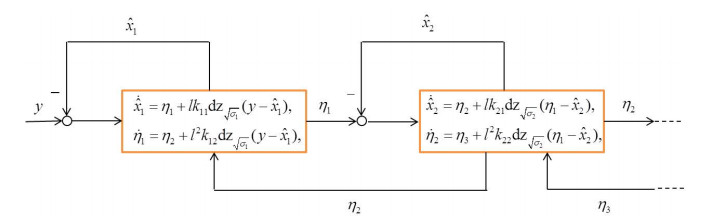
For high frequency noise, a new 2n-th order cascade extended state observer with dynamic dead-zone structure is proposed in this paper. Dead zone dynamic consists of two parts. One is to "trim" the effect of noise by cutting off the part that falls in the dead zone. The other part pushes the dead zone amplitude to converge to 0 as soon as possible to ensure the convergence of the estimation error. Moreover, in the cascade structure, the high-gain parameter grows only to a second power, thus avoiding excessive amplification of the measurement noise and solving numerical implementation problems. The design procedure ensures that the extended state observer is input-to-state stable. Numerical simulations show the improvement in terms of total disturbance estimation and noise attenuation. The frequency-domain analysis of the proposed ESO using the describing function method investigates the effect of the dead zone nonlinear parameter on the performance of a closed-loop system.
Citation: Shihua Zhang, Xiaohui Qi, Sen Yang. A cascade dead-zone extended state observer for a class of systems with measurement noise[J]. AIMS Mathematics, 2023, 8(6): 14300-14320. doi: 10.3934/math.2023732
[1] | Wei Zhao, Lei Liu, Yan-Jun Liu . Adaptive neural network control for nonlinear state constrained systems with unknown dead-zones input. AIMS Mathematics, 2020, 5(5): 4065-4084. doi: 10.3934/math.2020261 |
[2] | Xiaohang Su, Peng Liu, Haoran Jiang, Xinyu Yu . Neighbor event-triggered adaptive distributed control for multiagent systems with dead-zone inputs. AIMS Mathematics, 2024, 9(4): 10031-10049. doi: 10.3934/math.2024491 |
[3] | Hadil Alhazmi, Mohamed Kharrat . Echo state network-based adaptive control for nonstrict-feedback nonlinear systems with input dead-zone and external disturbance. AIMS Mathematics, 2024, 9(8): 20742-20762. doi: 10.3934/math.20241008 |
[4] | Jingjing Yang, Jianqiu Lu . Stabilization in distribution of hybrid stochastic differential delay equations with Lévy noise by discrete-time state feedback controls. AIMS Mathematics, 2025, 10(2): 3457-3483. doi: 10.3934/math.2025160 |
[5] | Andrey Borisov . Filtering of hidden Markov renewal processes by continuous and counting observations. AIMS Mathematics, 2024, 9(11): 30073-30099. doi: 10.3934/math.20241453 |
[6] | Chuang Liu, Jinxia Wu, Weidong Yang . Robust $ {H}_{\infty} $ output feedback finite-time control for interval type-2 fuzzy systems with actuator saturation. AIMS Mathematics, 2022, 7(3): 4614-4635. doi: 10.3934/math.2022257 |
[7] | Tian Xu, Ailong Wu . Stabilization of nonlinear hybrid stochastic time-delay neural networks with Lévy noise using discrete-time feedback control. AIMS Mathematics, 2024, 9(10): 27080-27101. doi: 10.3934/math.20241317 |
[8] | Zigui Kang, Tao Li, Xiaofei Fan . Delay-dependent anti-disturbance control of electric vehicle based on collective observers. AIMS Mathematics, 2023, 8(6): 14684-14703. doi: 10.3934/math.2023751 |
[9] | Yanxin Li, Shangkun Liu, Jia Li, Weimin Zheng . Congestion tracking control of multi-bottleneck TCP networks with input-saturation and dead-zone. AIMS Mathematics, 2024, 9(5): 10935-10954. doi: 10.3934/math.2024535 |
[10] | Xiao Yu, Yan Hua, Yanrong Lu . Observer-based robust preview tracking control for a class of continuous-time Lipschitz nonlinear systems. AIMS Mathematics, 2024, 9(10): 26741-26764. doi: 10.3934/math.20241301 |
For high frequency noise, a new 2n-th order cascade extended state observer with dynamic dead-zone structure is proposed in this paper. Dead zone dynamic consists of two parts. One is to "trim" the effect of noise by cutting off the part that falls in the dead zone. The other part pushes the dead zone amplitude to converge to 0 as soon as possible to ensure the convergence of the estimation error. Moreover, in the cascade structure, the high-gain parameter grows only to a second power, thus avoiding excessive amplification of the measurement noise and solving numerical implementation problems. The design procedure ensures that the extended state observer is input-to-state stable. Numerical simulations show the improvement in terms of total disturbance estimation and noise attenuation. The frequency-domain analysis of the proposed ESO using the describing function method investigates the effect of the dead zone nonlinear parameter on the performance of a closed-loop system.
The extended state observer (ESO) proposed by Han Jingqing appeared in 1995. In active disturbance rejection control (ADRC), simultaneous reconstruction of the states of a plant as well as total disturbance using an ESO is one of the most important strategies. Since then, ESO has attracted a lot of research attention because it requires minimal information about the plant and is simple to implement. ESO based controls have been widely applied in various areas involving urban traffic [1], motion [2], power [3], and so on. At first, the classic ESO was only proposed for uncertain systems in series integrator form. The convergence of the classic ESO has been theoretically validated by using various tools like singular perturbation [4] and Lyapunov techniques [5]. Recently, many studies have been done on ESO design methods for systems that cannot be transformed into series integrators, such as mismatched uncertain systems [6], time-delay systems [7], multi-coupled subsystems [8] and non-minimal phase (NMP) systems [9]. In addition, in recent years, some scholars began to pay attention to finite time estimation in order to achieve safety and high steady state accuracy. [10] proposed a continuous finite time ESO. The time-varying gain of the observer was designed by defining the time-varying transformation so that the observation error approached zero in finite time. Furthermore, Razmjooei, Palli, Shafiei et al. proposed an adaptive fast finite time ESO to ensure convergence within a short time interval regardless of initial conditions ([11,12]).
In practical engineering, the measurement noise will be unavoidable. Measurement noise can be amplified by the ESO and entered the outer loop system, which can have adverse effects such as saturation of input actuators, vibration of mechanical systems, and even system instability. Therefore, improving the robustness to measurement noise of the ESO is still an active research topic. In fact, to a large extent, satisfactory system state reconstruction and total disturbance compensation usually result in high gain forms of the ESO ([5,13]). When the system has a high dimension or a large high gain parameter must be selected to achieve rapid estimation, this high gain leads to some limitations in applications, such as peaking phenomenon, numerical problems and sensitivity to high frequency measurement noise. Therefore, in order to suppress the noise, many strategies have been proposed, mainly the error-based gain-regulation techniques ([14,15,16]) and the use of cascade structures ([17,18,19]). On the other hand, combining the ESO with a Kalman filter [20] or adding integral terms to the ESO [21] are other solutions to attenuate the effects of measurement noise. While the above methods have shown effectiveness in certain control scenarios, they have some limitations, including the introduction of additional system lags, the complexity of tuning methods, and additional design knowledge requirements.
Inspired by Astolfi and Marconi [17], [18] proposed a new extended state observer with the low power structure (LPESO), which consisted of n second order linear observers. When high frequency noise is present, the LPESO improves the estimation performance by adopting a structure whose gain grows only to the second power. Thus it relaxes the digital implementation requirements. However, linear feedback is used in this low power structure. It can be seen from the numerical simulation in [18] that LPESO can reduce the peaking more effectively compared with traditional LESO, but it is still sensitive to persistent high-frequency noise. This is also verified in our numerical simulations.
In this paper, in order to suppress the persistent bounded measurement noise, a total of n observers of second order are designed using dead-zone nonlinear feedback and connected into a cascade structure. The dynamic dead-zone was originally applied to the high gain observer in [22], but in this paper, the dead zone nonlinear feedback is designed in the cascade structure, which is a generalization of [22]. The dead zone dynamic is composed of two parts. One is to cut down the noise that falls in the zone to improve noise attenuation. The other is to push the dead zone amplitude to converge to 0 as soon as possible to ensure the convergence of errors. In addition, the cascade structure avoids excessive power increase of the high gain parameter, inherits the good performance of non-amplifying noise, and solves numerical implementation problems. Theoretical analysis shows that LPESO in [18] is a linear form of the extended state observer proposed in this paper. By perturbation theory, the convergence of the proposed ESO is proved. Numerical simulations reveal that the cascade ESO with the dynamic dead zone can significantly improve the suppression of persistent bounded measurement noise. Description function method is used to analyze the proposed ESO in the quasi-frequency domain, and the effect of the dead zone nonlinear parameter on the closed-loop system performance is studied. This analysis provides a more intuitive view of its high-frequency noise suppression capability.
This paper is organized as follows. Section 2 formulates a problem for nth-order nonlinear system. Section 3 shows input-to-state stability when there is measurement noise. Section 4 provides an example of numerical validation and a quasi-frequency domain analysis is carried out based on Laplace transform and description function. Section 5 offers the conclusion.
Notation. Diagn>0 represents the set of n×n-dimensional diagonal positive definite matrices. col(a1,⋅⋅⋅,an) denotes a column vector. The Euclidean norm of the vector a is defined as ‖a‖=√aTa. ‖X‖=(λmax(XTX))12 represents the l2 norm of the matrix X. Given a∈R,b∈R≥0, define sata(b):=max{−a,min{b,a}} and dza(b):=b−sata(b). For a given vector c=[c1,⋯,cn]T, there is satb(c):=[satb(c1),⋯,satb(cn)]T.
Definition. [19] The following system
˙x(t)=f(x,u1,u2,t), |
defined on x∈X⊂Rn,u1∈U1⊂Rm1, u2∈U2⊂Rm2, and t∈[0,+∞) is locally input-to-state stable (ISS) on some bounded sets X,U1,U2 if there exist a function β of class KL, and functions γ1,γ2 of class K, such that for any initial condition x(0)∈X and any bounded inputs u1(t),u2(t), solution x(t) exists for all t, satisfying
‖x(t)‖≤β(x(0),t)+γ1(supt≥0‖u1(t)‖)+γ2(supt≥0‖u2(t)‖). |
Consider a nonlinear uncertain system of nth-order, which can be written in phase-variable form after coordinate changes:
{˙xi(t)=xi+1(t),i=1,⋯,n−1,˙xn(t)=f(t,x)+d∗(t)+u(t),y(t)=x1(t)+v(t), | (2.1) |
where x=(x1,x2,⋅⋅⋅,xn)T∈Rn is the state vector, f(t,x) involves uncertain linear or nonlinear internal dynamics, y∈R is the measured output, u∈R is a control signal, d∗∈R is the unknown external disturbance and v represents an unknown measurement noise. Define
d(t,x)=f(t,x)+d∗(t), |
as the total disturbance. We are interested in designing the ESO to simultaneously estimate the state vector as well as the total disturbance despite the measurement noise v(t).
Assumption 1. For all x∈D and t>0, the total disturbance d(t,x) is bounded by |d(t,x)|≤md, and the bound of its derivative is m˙d, i.e., |ddtd(t,x)|≤m˙d.
Assumption 2. Measurement noise v(t) is bounded, that is |v(t)|≤mv.
In order to estimate the states and total disturbance of system (2.1), the order of the traditional ESO is n+1. Therefore, when the plant order is large, the high gain parameter of the ESO will grow to a power of n+1, resulting in the amplification of measurement noise and pollution of the estimates. To solve this problem, inspired by [17,18,22,23], we design an observer with dynamic dead-zone in a cascade structure for persistent bounded high frequency noise that affects the measurement output. This is a structure consisting of a cascade of n second-order observers with dynamic dead zone feedback. For i≤n−1, if xi and xi+2 are known, design second-order dynamic dead zone observers for xi and xi+1 as follows
˙ˆxi=ηi+lki1dz√σi(xi−ˆxi),˙ηi=xi+2+l2ki2dz√σi(xi−ˆxi), | (2.2) |
where ˆxi and ηi are the estimations of xi and xi+1, l is the high gain parameter, ki1 and ki2 are parameters to be designed. √σi is the threshold, satisfying the following dynamic system
˙σi=−lλσi+lw(xi−ˆxi)2. | (2.3) |
In the above system, λ>0 is a constant, w is the parameter to be designed. Similarly, second-order dynamic dead zone observer of xn and d(t,x) takes the form as
˙ˆxn=ηn+lkn1dz√σn(xn−ˆxn),˙ηn=l2kn2dz√σn(xn−ˆxn),˙σn=−lλσn+lw(xn−ˆxn)2, | (2.4) |
in which l,kn1,kn2,λ and w are defined as above. In fact, we take the total disturbance d(t,x) as an extended state of system (2.1). If xn is known, (2.4) is an extended state observer for xn and d(t,x), ˆxn and ηn are estimations, respectively. However, only x1 is measurable, and x2,x3,⋯,xn are unknown state variables in system (2.1). Thus, we replace xi and xi+2 with the second state ηi−1 and ηi+1 in the (i−1)the observer and the (i+1)th observer, respectively. Meanwhile, xn is replaced by the second state ηn−1 in the (n−1)th observer. Then the above n second-order observers (2.2)–(2.4) are connected into a dynamic dead zone observer with a cascaded structure (Figure 1).
As can be seen from Figure 1, a total of n dead-zone dynamics are needed for n output injection channels. To simplify the design, we define the same dead-zone amplitude determined by the sum of the squares of output injection terms. Thus, an extended state observer with dynamic "dead-zonated" output injection (DZ-CESO) is introduced to deal with persistent bounded measurement noise affecting the measurement output, as shown below
{˙z1=Az1+Nz2+D(l)K1dz√σ(y−Cz1),˙zi=Azi+Nzi+1+D(l)Kidz√σ(BTzi−1−Czi),i=2,⋅⋅⋅,n−2,⋮˙zn−1=Azn−1+Nzn+D(l)Kn−1dz√σ(BTzn−2−Czn−1)+Bu(t),˙zn=Azn+D(l)Kndz√σ(BTzn−1−Czn)+CTu(t), | (2.5) |
where zi=(ˆxi,ηi)T∈R2,z=(z1,⋅⋅⋅,zn)T denotes a state of the proposed DZ-CESO. In (2.5),
A=(0100),N=(0001), |
B=(0,1)T,C=(1,0), D(l)=diag(l,l2)∈Diag2>0. The parameters Ki=(ki1,ki2)(i=1,⋅⋅⋅,n) are designed later. Define two block diagonal matrices in Rn×2n,
L1=blockdiag(C,⋅⋅⋅,C),L2=blockdiag(BT,⋅⋅⋅,BT,(0,0)). |
From the above analysis we can see that ˆx=L1z can be seen as a correction for ˆx′=L2z. Then we take ˆx=L1z and ηn=BTzn as the estimations of the state vector and total disturbance of system (2.1), respectively, and ˆx′ as the redundant estimation. σ∈R≥0 defines the dead-zone amplitude on the output channel, whose adaptation law is
˙σ=−lλσ+lw[(y−Cz1)2+(BTz1−Cz2)2+⋅⋅⋅+(BTzn−1−Czn)2]. | (2.6) |
Note that √σ is always well defined because σ is non-negative.
Observer (2.5) is obtained by "dead-zonating" the output errors using a dynamic dead-zone level √σ. Around y−Cz1=0 and BTzi−1−Czi=0,i=2,⋅⋅⋅,n, zero output correction terms provided by the dead zone filter out high frequency noise. However, a fixed dead-zone amplitude will make the tracking and tracked signals never synchronize. σ needs to approach 0 fast to ensure convergence to zero. The adaptation law (2.6) weighs two adversarial effects: the first item is to push the dead-zone amplitude σ to zero, and the second item is to filter out noise by appropriately increasing σ using the output estimation error. Therefore, σ converges to 0 fast when λ is large. In particular, if there is only the first term, the dead zone function deforms to a linear form in a very short time, and then DZ-CESO deforms to LPESO in [18]. On the other hand, setting w large increases the amplitude of the dead zone, thereby improving the ability of noise suppression. Note that the dead-zone amplitude adaptive law (2.6) is different from that in [22]. We correct the amplitude using the quadratic sum of the estimation errors of n output channels to have the same threshold. In this way, the estimation error of each second-order observer is considered and the design of observer DZ-CESO is simplified.
Remark 1. The high gain parameter which is defined by D(l), can only grow up to the second order. Thus, the peaking phenomenon and the numerical problems in the implementation which are caused by ln can be solved. Furthermore, by cutting down the high frequency noise that falls in the dead-zone, the dynamic dead-zone reduces the high sensitivity to measurement noise and improves the noise attenuation. The numerical simulation and frequency domain analysis in Section 4 will show the benefits of the dead-zone nonlinearity by comparing LPESO and DZ-CESO.
We study the ISS property of the estimation error for the proposed observer (2.5), and give an upper bound of the estimation error in this section.
Change variables as the following
˜zi=zi−col(xi,xi+1),i=1,⋅⋅⋅,n−1,˜zn=zn−col(xn,d). |
By the definition of dz√σ(⋅), system (2.5) can be transformed as follows
{˙˜z1=(A−D(l)K1C)˜z1+N˜z2+D(l)K1v−D(l)K1sat√σ(v−C˜z1),˙˜zi=D(l)KiBT˜zi−1+(A−D(l)KiC)˜zi+N˜zi+1−D(l)Kisat√σ(BT˜zi−1−C˜zi),i=2,⋅⋅⋅,n−1,⋮˙˜zn=D(l)KnBT˜zn−1+(A−D(l)KnC)˜zn−col(0,˙d)−D(l)Knsat√σ(BT˜zn−1−C˜zn). |
Rescale the variables as
ζi=l2−iD(l)−1˜zi,i=1,⋅⋅⋅,n,ζ=col(ζ1,⋅⋅⋅,ζn)∈R2n, | (3.1) |
and rewrite the error dynamic system for (2.1) and (2.5) in a compact form:
˙ζ=lMζ−Δ(l,t,x)−lˆKD−1n(l)sat√σ(Dn(l)Gζ)+l¯K[sat√σ(Gζ)−sat√σ(Gζ+Bnv)]+l¯KBnv, | (3.2) |
in which
Δ(l,t,x)=col(02×1,⋅⋅⋅,02×1,l−ncol(0,˙d))∈R2n,Dn(l)=diag(1,l,⋅⋅⋅,ln−1)∈Diagn>0,Bn=(1,0,⋅⋅⋅,0)∈R2n×1,ˆK=blockdiag(K1,⋅⋅⋅,Kn)∈R2n×n,¯K=blockdiag(K1,02×1,⋅⋅⋅,02×1)∈R2n×n. |
Define the matrices G and M:
G=(−C00⋯0BT−C0⋯00BT−C⋯0⋮⋮⋮⋱⋮00⋯BT−C)n×2n, |
M=(E1N00⋯0Q2E2N0⋯00Q3E3N⋯0⋯⋯⋯⋯⋯⋯00⋯Qn−1En−1N00⋯0QnEn)2n×2n, | (3.3) |
where 0 represents the zero matrix of the corresponding dimension, and
Ei=A−KiC=(−ki11−ki20),Qi=KiBT=(0ki10ki2). | (3.4) |
Correspondingly, the adaptation law of σ is transformed into
˙σ=−lλσ+lw[(v−Cζ1)2+l2(BTζ1−Cζ2)2+⋅⋅⋅+l2(n−1)(BTζn−1−Cζn)2]. | (3.5) |
Remark 2. The eigenvalues of M can be arbitrarily assigned by selecting Ki(i=1,2,⋯,n). The constructive procedure was given in the appendix of [17]. Based on this algorithm, [18] gave a simple selection rule.
Theorem 1. Selecting (ki1,ki2)(i=1,⋯,n) in system (2.5) makes M defined in (3.3) a Hurwitz matrix. Then there exist l∗>1,λ>0,w∗>0, if l>l∗ and 0<w<w∗, for any initial conditions x(0)∈Rn,z(0)∈R2n,σ(0)∈R, and some positive constants μi(i=3,4,5,6), the following bounds hold
|ˆxi−xi|≤μ6κlie−μ3lt+μ4l−n−12+im˙d+μ5l12+imv,i=1,⋯,n,|ηn−d|≤μ6κlne−μ3lt+μ4l−12m˙d+μ5l12+nmv, | (3.6) |
where
κ=n∑i=1|xi(0)−ˆxi(0)|+n−1∑i=1|xi+1(0)−ηi(0)|+|ηn(0)|+√σ(0)+md. |
Proof. Using the time-rescaling
t↦τ:=lt |
on systems (3.2) and (3.5) leads to
˙ζ=dζdτ=Mζ−l−1Δ(l,t,x)−ˆKD−1n(l)sat√σ(Dn(l)Gζ)+¯K[sat√σ(Gζ)−sat√σ(Gζ+Bnv)]+¯KBnv,˙σ=dσdτ=−λσ+w(ζTGTD2n(l)Gζ)+w(v2−2vCζ1). | (3.7) |
Choose Ki(i=1,2,⋯,n) so that M is a Hurwitz matrix. Then solving the Lyapunov equation PM+MTP=−I yields a positive definite matrix P∈R2n. Define Lyapunov function,
V(τ,ζ,σ)=ζTPζ+ξσ, |
in which ξ>0 is a constant, whose value is determined later. It turns out that
λmin(P)‖ζ‖2+ξσ≤V(τ,ζ,σ)≤λmax(P)‖ζ‖2+ξσ. | (3.8) |
Along the solution of (3.7), take the derivative of V(τ,ζ,σ) and get that
˙V=ζT(PM+MTP)ζ−2l−1ζTPΔ(l,t,x)−2ζTPˆKD−1n(l)sat√σ(Dn(l)Gζ)+2ζTP¯K[sat√σ(Gζ)−sat√σ(Gζ+Bnv)]+2ζTP¯KBnv−ξλσ+ξw(ζTGTD2n(l)Gζ)+ξw(v2−2vCζ1). | (3.9) |
According to Assumption 1 and Young's inequality, the second term of (3.9) can be enlarged to
|−2l−1ζTPΔ(l,t,x)|≤2l−1‖ζ‖⋅‖P‖⋅‖Δ(l,t,x)‖≤2‖ζ‖⋅‖P‖⋅1ln+1m˙d≤2(12‖ζ‖)(2l−(n+1)‖P‖m˙d)≤14‖ζ‖2+4l−2(n+1)‖P‖2m2˙d. |
For the third term, since ‖D−1n(l)‖≤1 and ‖sat√σ(Dn(l)Gζ)‖≤√nσ based on the definition of the norm of the matrix and the vector, it can be written that
−2ζTPˆKD−1n(l)sat√σ(Dn(l)Gζ)≤2|ζTPˆKD−1n(l)sat√σ(Dn(l)Gζ)|≤2‖ζ‖⋅‖PˆK‖⋅‖D−1n(l)‖⋅‖sat√σ(Dn(l)Gζ)‖≤2‖ζ‖⋅‖PˆK‖⋅√nσ=2(12‖ζ‖)⋅(2√nσ‖PˆK‖)≤14‖ζ‖2+4n‖PˆK‖2σ. |
Note that
|sat√σ(Gζ)−sat√σ(Gζ+Bnv)|≤‖Bnv‖≤mv. |
Thus, it is easy to get that
2ζTP¯K[sat√σ(Gζ)−sat√σ(Gζ+Bnv)]+2ζTP¯KBnv≤4‖ζ‖⋅‖P¯K‖⋅mv=2(14‖ζ‖)⋅(4‖P¯K‖⋅2mv)≤116‖ζ‖2+64‖P¯K‖2m2v. |
As for the seventh term, it is can be seen that
ξw(ζTGTD2n(l)Gζ)≤ξw|(Cζ1)2+l2(BTζ1−Cζ2)2+⋯+l2(n−1)(BTζn−1−Cζn)2|=ξw|ζ21,1+l2(ζ21,2+ζ22,1−2ζ1,2ζ2,1)+⋯+l2(n−1)(ζ2n−1,2+ζ2n,1−2ζn−1,2ζn,1)|≤ξwl2(n−1)[|ζ21,1+ζ21,2+ζ22,1+⋯+ζ2n−1,2+ζ2n,1|+|2ζ1,2ζ2,1+⋯+2ζn−1,2ζn,1|]≤ξwl2(n−1)[‖ζ‖2+(ζ21,2+ζ22,1+⋯+ζ2n−1,2+ζ2n,1)]≤2ξwl2(n−1)‖ζ‖2. |
Now, we calculate the last term, that is
ξw(v2−2vCζ1)≤ξwv2+2(14‖ζ‖)⋅(4ξwmv)≤116‖ζ‖2+(ξw+16ξ2w2)m2v. |
As a result, we have that
˙V≤(−38+2ξwl2(n−1))‖ζ‖2+(−ξλ+4n‖PˆK‖2)σ+4l−2(n+1)‖P‖2m2˙d+(64‖P¯K‖2+ξw+16ξ2w2)m2v. | (3.10) |
In order to make both the first and second expressions of (3.10) negative, let l≥l∗>1, and
ξ>ρ+4n‖PˆK‖2λ,w<18ξl2(n−1), | (3.11) |
in which ρ is a positive constant. Then (3.10) can be written as
˙V≤−18‖ζ‖2−ρσ+4l−2(n+1)‖P‖2m2˙d+(64‖P¯K‖2+ξw+16ξ2w2)m2v. | (3.12) |
Let
μ1=min{18λmax(P),ρξ}. | (3.13) |
By using (3.8), (3.12) and (3.13), it leads to that
˙V≤−μ1(λmax(P)‖ζ‖2+ξσ)+4l−2(n+1)‖P‖2m2˙d+(64‖P¯K‖2+ξw+16ξ2w2)m2v≤−μ1V+4l−2(n+1)‖P‖2m2˙d+(64‖P¯K‖2+ξw+16ξ2w2)m2v. |
Since τ=lt, we have that
dVdt=˙Vdτdt=l˙V≤−μ1lV+4l−2n−1‖P‖2m2˙d+l(64‖P¯K‖2+ξw+16ξ2w2)m2v. |
By the comparison theorem, it is obtained that
V(t,ζ(t),σ(t))≤V(0,ζ(0),σ(0))e−μ1lt+lμ1[4l−2(n+1)‖P‖2m2˙d+(64‖P¯K‖2+ξw+16ξ2w2)m2v]. | (3.14) |
From (3.8) and (3.14), it is concluded that
‖ζ(t)‖≤μ2e−μ3lt+μ4l−n−12m˙d+μ5l12mv, |
in which
μ2=√λmax(P)‖ζ(0)‖2+ξσ(0)λmin(P),μ3=μ12,μ4=2‖P‖√μ1λmin(P),μ5=√64‖P¯K‖2+ξw+16ξ2w2μ1λmin(P). |
From (3.1), for l>1, note that 1li‖˜zi‖≤‖ζi‖≤‖˜zi‖. Therefore, we have
|ˆxi−xi|≤μ2lie−μ3lt+μ4l−n−12+im˙d+μ5l12+imv,i=1,⋯,n,|ηn−d|≤μ2lne−μ3lt+μ4l−12m˙d+μ5l12+nmv. | (3.15) |
Define
μ6=√max{λmax(P),ξ}λmin(P). | (3.16) |
Since
‖ζ(0)‖≤n∑i=1|xi(0)−ˆxi(0)|+n−1∑i=1|xi+1(0)−ηi(0)|+|ηn(0)|+md, | (3.17) |
combining (3.15)–(3.17), we can obtain the inequalities in (3.6). The proof is complete.
Remark 3. Note that taking σ≡0 in (2.5), DZ-CESO is reduced to LPESO in [18], which indicates that LPESO is a linear form of DZ-CESO. From the proof of Theorem 1, if the matrix M is Hurwitz, the convergence of LPESO can be obtained.
The parameters to be designed for DZ-CESO are Ki(i=1,⋯,n) and w. To summarize the DZ-CESO design methods discussed in Theorem 1, a parameter tuning procedure is given as follows:
step 1: According to Remark 3, select Ki such that eigenvalues of the matrix M are assigned to the specified positions.
step 2: Based on (3.3) and (3.4), establish matrix M and solve the Lyapunov equation PM+MTP=−I to obtain the matrix P.
step 3: Choose l∗>1, ρ>0 and λ>0.
step 4: Select ξ to satisfy ξ>ρ+4n‖PˆK‖2λ.
step 5: Finally choose w∗=18ξl2(n−1).
This section first presents an example to evaluate the effectiveness of the cascade ESO with dead-zone mechanism proposed in Section 2 in terms of total disturbance estimation and noise attenuation. The parameter selection methods of the LESO, LPESO and DZ-CESO are given. Then simulation results of three ESOs are presented to illustrate the advantage of DZ-CESO. At the end of this section, DZ-CESO (4.2) is analyzed in the quasi-frequency domain based on Laplace transform and description function, so as to show its ability of suppressing high-frequency noise more intuitively.
The following second-order nonlinear uncertain system in form of (2.1) is considered:
{˙x1(t)=x2(t),˙x2(t)=f(x1(t),x2(t))+d∗(t)+u(t),y(t)=x1(t)+v(t), | (4.1) |
with d∗(t)=t4π+sin(4t+1) and f(x1(t),x2(t))=5(1−x21(t))x2(t)−x1(t). The total disturbance is
d(t,x)=5(1−x21(t))x2(t)−x1(t)+t4π+sin(4t+1). |
The control objective is to estimate the total disturbance d(t,x), and the states x1(t),x2(t) despite the measurement noise v(t). We selected x(0)=(0,0) in the simulation. Define the same bounded control action u(t)=3sin(2t) for all the tested cases. Measurement noise starts at ts=10s, ends at te=20s during the simulation.
Due to total disturbance compensation is the essential purpose of ESO, parameter selection here is based on providing similar reconstruction quality of d(t,x) at steady state in terms of minimizing integral criterion ∫Tt0|e3(t)|dt, with e3(t) being the total disturbance observation error, t0=25s being the integration start time and T=30s being its finish time.
We implement the proposed DZ-CESO as specified in Section 2, and get that
{˙ˆx1(t)=η1(t)+lk11dz√σ(y(t)−ˆx1(t)),˙η1(t)=η2(t)+l2k12dz√σ(y(t)−ˆx1(t))+u(t),˙ˆx2(t)=η2(t)+lk21dz√σ(η1(t)−ˆx2(t))+u(t),˙η2(t)=l2k22dz√σ(η1(t)−ˆx2(t)), | (4.2) |
with the adaption law
˙σ(t)=−lλσ(t)+lω[(ˆx1(t)−y(t))2+(ˆx2(t)−η1(t))2]. | (4.3) |
According to the parameter tuning procedure given in Section 3, determine the coefficients and parameters to be designed in (4.2) and (4.3). Firstly, the matrix M for (4.2) is
M=(−k11100−k120010k21−k2110k22−k220), |
with characteristic polynomial
fM(λ)=λ4+(k11+k21)λ3+(k12+k11k21)λ2+k12k21λ+k12k22. |
Place all four of the poles of matrix M at −1, and then the coefficients Ki can be set as k11=2,k12=2,k21=2,k22=0.5. Secondly, the matrix P is obtained in the following by solving Lyapunov equation
P=(1.6250−1.37500.3750−2.6250−1.37501.9062−0.09372.12500.3750−0.09370.9062−2.6250−2.62502.1250−2.625012.1250). |
We obtained ‖PˆK‖2=2.7143. Let l=10,λ=10,ρ=0.01. According to steps 4 and 5, there is w=0.001. Choose initial conditions as ˆx1(0)=0,η1(0)=5,ˆx2(0)=−5,η2(0)=−5, and σ(0)=5.
Remark 4. Considering the initial value of ˆx1(t) is generally taken from sensors, so the error (x1(0)−ˆx1(0)) is small. While for the DZ-CESO, there exist other initial errors besides the first term (x1(0)−ˆx1(0)). Therefore, we set the initial value of first state for all ESOs equal to x1(0) in system (4.1) and keep the other initial states unequal to compare the peaking phenomenon in simulations.
For convenience, we keep the notations concise, and treat the LPESO in [18] as case dz√σ(y(t)−ˆx1(t))=y(t)−ˆx1(t), dz√σ(η1(t)−ˆx2(t))=η1(t)−ˆx2(t), and no adaption law (4.3). The coefficients Ki and l are the same as the values in (4.2). The initial state is also placed at (0,5,−5,−5).
Based on (4.1), the LESO can be designed accordingly from [13]:
{˙z1(t)=z2(t)+lβ1(y−z1),˙z2(t)=z3+l2β2(y−z1)+u(t),˙z3(t)=l3β3(y−z1). | (4.4) |
Since the LESO is different from the DZ-CESO and LPESO in structure and number of tuning parameters, we first set β1=3,β2=3,β3=1 to place the poles of the following matrix at −1
(−β110−β201−β300). |
The initial state is (z1(0),z2(0),z3(0))=(0,5,−5). It is found in simulations that gain parameter l=10 provides a similar total disturbance reconstruction quality, as confirmed by Table 1.
LESO | LPESO | DZ-CESO | |
l=10(ˆx1(0)=0) | 5.5644 | 5.3976 | 5.4053 |
l=20(ˆx1(0)=0.5) | 2.9436 | 2.9332 | 2.9679 |
l=30(ˆx1(0)=0.5) | 1.9972 | 1.9936 | 2.0053 |
We perform the following comparison simulations. In the first case, the output y(t) is polluted by "Band-Limited White Noise" with power 1e−3. Figure 2 shows the comparison of the estimated state and total disturbance of the three observers. As can be seen from Figure 2 that, although the estimations are affected by the measurement noise, the DZ-CESO outperforms the LESO and LPESO in terms of noise attenuation. In particular, for x2 and the total disturbance, estimations of the DZ-CESO are significantly better than the other two methods when high frequency noise is present, despite some lag in tracking speed.
Tables 2–4 show the peakings of estimation errors of x1(t),x2(t) and d(t,x) for three ESOs under noiseless conditions, respectively. It is can be seen that when l=10, there is no significant difference in the error peakings of the three ESOs, although the initial error of the DZ-CESO is larger. In order to characterize the peaking phenomenon, we set ˆx1(0)=0.5 for three ESOs, leaving the other initial values unchanged. Simulations are performed under criteria with similar total disturbance reconstruction qualities, as shown in Table 1. As can be seen from Tables 2–4, with the increase of l, the peakings of e2(t) and e3(t) provided by the LESO obviously increase, while those provided by LPESO and DZ-CESO barely change. Note that the largest coefficient that needs to be implemented in LPESO and DZ-CESO is k12l2=1800, while in the LESO, l3=27000. This implies DZ-CESO can solve the peaking phenomenon and the numerical problems in the implementation which are caused by ln.
l=10(ˆx1(0)=0) | l=20(ˆx1(0)=0.5) | l=30(ˆx1(0)=0.5) | |
‖e1(t)‖∞ | 0.1172 | 0.5000 | 0.5000 |
‖e2(t)‖∞ | 5 | 5.7394 | 9.5657 |
‖e3(t)‖∞ | 46.8049 | 63.3846 | 127.9371 |
l=10(ˆx1(0)=0) | l=20(ˆx1(0)=0.5) | l=30(ˆx1(0)=0.5) | |
‖e1(t)‖∞ | 0.2022 | 0.5000 | 0.5000 |
‖e2(t)‖∞ | 5.6699 | 5 | 5.7548 |
‖e3(t)‖∞ | 46.5124 | 48.2156 | 42.9333 |
l=10(ˆx1(0)=0) | l=20(ˆx1(0)=0.5) | l=30(ˆx1(0)=0.5) | |
‖e1(t)‖∞ | 0.1891 | 0.5446 | 0.5296 |
‖e2(t)‖∞ | 5 | 5 | 5 |
‖e3(t)‖∞ | 39.3851 | 42.5383 | 48.6312 |
In order to compare the attenuation effect of the observer on noise with different frequencies, we did a lot of simulations in three different scenarios. All the parameters and initial conditions of ESOs are the same as in Section 4.1. In the scenario (a), the noise is generated by "Band-Limited White Noise" with power 1e−3. In the scenarios (b) and (c), the measurement noise v(t) is generated by a sinusoidal signal v(t)=5sin(ωt). In the scenario (b), ω=1000 represents high-frequencies, while in the scenario (c), ω=100 represents medium frequencies. Tables 5–7 show the effect of the measurement noise on the steady-state behaviour of the three observers, respectively. ‖ei‖∞(i=1,2,3) represent the maximum estimated errors of states and the total disturbance under measurement noise (from t=10s to t=20s). We use the error noise ratio (ENR) to measure the extent to which the error is polluted by noise. The ENR is defined as ENR:=‖e‖2‖v‖2. It can be seen from Tables 5–7 that in the scenario (a), e2 and e3 are more seriously polluted by noise than that of e1 for all three ESOs. Compared to LESO and LPESO, the DZ-CESO greatly decreases the error noise ratio of e2 and e3. In the scenario (b), for the observers LPESO and DZ-CESO, the high frequency noise is attenuated on all estimation errors ei(t)(i=1,2,3). In the scenario (c), the DZ-CESO is superior to LPESO in suppressing medium frequency noise. Through these simulations, the benefits of the dynamic dead-zone mechanism with the low power structure can be recognized.
(a) | (b) | (c) | |
‖v(t)‖∞ | 9.6790 | 5 | 5 |
‖e1(t)‖∞ | 4.3875 | 0.2762 | 1.8548 |
‖e2(t)‖∞ | 38.9742 | 5.4794 | 18.7378 |
‖e3(t)‖∞ | 125.9236 | 55.1586 | 98.4120 |
ENR of e1 | 0.4331 | 0.0301 | 0.2961 |
ENR of e2 | 3.9908 | 0.3005 | 2.9569 |
ENR of e3 | 13.0557 | 1.0016 | 9.8504 |
(a) | (b) | (c) | |
‖v(t)‖∞ | 9.6790 | 5 | 5 |
‖e1(t)‖∞ | 3.5098 | 0.3120 | 1.3656 |
‖e2(t)‖∞ | 20.6755 | 5.9730 | 7.8698 |
‖e3(t)‖∞ | 46.9397 | 49.7482 | 52.8344 |
ENR of e1 | 0.3779 | 0.0201 | 0.2010 |
ENR of e2 | 2.3023 | 0.0123 | 0.3972 |
ENR of e3 | 5.5629 | 0.0295 | 0.9919 |
(a) | (b) | (c) | |
‖v(t)‖∞ | 9.6790 | 5 | 5 |
‖e1(t)‖∞ | 3.0672 | 0.2867 | 1.2620 |
‖e2(t)‖∞ | 4.2285 | 5 | 5 |
‖e3(t)‖∞ | 41.6543 | 41.8767 | 43.1932 |
ENR of e1 | 0.3156 | 0.0199 | 0.1955 |
ENR of e2 | 0.6097 | 0.0106 | 0.1748 |
ENR of e3 | 2.3798 | 0.0258 | 0.4233 |
The error dynamics system of (4.1) and (4.2) is
{˙e1(t)=e2(t)+lk11dz√σ(v(t)−e1(t)),˙e2(t)=e4(t)+l2k12dz√σ(v(t)−e1(t)),˙e3(t)=e4(t)+lk21dz√σ(e2(t)−e3(t)),˙e4(t)=l2k22dz√σ(e2(t)−e3(t))−˙d(t,x), | (4.5) |
where e1(t)=ˆx1(t)−x1(t),e2(t)=η1(t)−x2(t),e3(t)=ˆx2(t)−x2(t),e4(t)=η2(t)−d(t,x). Substituting ˙d(t,x)=0 into (4.5), Laplace transform and description function are used to transform the error system (4.5) into quasi-frequency domain as follows,
{Ea(s)=V(s)−E1(s),Eb(s)=E2(s)−E3(s),E1(s)s=E2(s)+lk11N(Ea),E2(s)s=E4(s)+l2k12N(Ea),E3(s)s=E4(s)+lk21N(Eb),E4(s)s=l2k22N(Eb), | (4.6) |
in which s is the Laplace variable; E1(s),E2(s),E3(s) and E4(s) are the outputs of (4.6); V(s) is the measurement noise. All of above are in the frequency domain. N(Ea) and N(Eb) are the describing functions of the dead zone nonlinearity with amplitudes of Ea(s) and Eb(s) as the input, respectively. The describing function of the dead zone nonlinearity in (4.2) is denoted as
N(E)=2π[π2−arcsin√σE−√σE√1−(√σE)2],E⩾√σ. | (4.7) |
The block diagram description of system (4.6) is shown in Figure 3.
Notice that if √σ=0 in (4.7), we have N(Ea)=1,N(Eb)=1 in Figure 3, then the system is reduced to be linear. The transfer functions from v(t) to ei(t)(i=1,2,3,4) can be directly derived from Figure 3
G1linear(s)=lk11s3+l2(k11k21+k12)s2+l3k12k21s+l4k12k22s4+l(k11+k21)s3+l2(k11k21+k12)s2+l3k12k21s+l4k12k22,G2linear(s)=l2k12s3+l3k12k21s2+l4k12k22ss4+l(k11+k21)s3+l2(k11k21+k12)s2+l3k12k21s+l4k12k22,G3linear(s)=l3k12k21s2+l4k12k22ss4+l(k11+k21)s3+l2(k11k21+k12)s2+l3k12k21s+l4k12k22,G4linear(s)=l4k12k22s2s4+l(k11+k21)s3+l2(k11k21+k12)s2+l3k12k21s+l4k12k22. | (4.8) |
The frequency response of the linear system can be easily obtained. If we are mainly concerned with high frequency, only the highest order of numerator and denominator is important. Then it is can be seen from (4.8) that the DZ-CESO in linear form can be approximated as a high order low-pass filter, so it has better noise attenuation ability than LESO. Furthermore, the dead zone nonlinearity on the improvement of noise suppression will be studied in the following.
However, for a nonlinear system, it is not straightforward because the outputs of nonlinear blocks N(Ea) and N(Eb) are related to the amplitudes Ea and Eb of their inputs. Therefore, we use a numerical method which was proposed in [24] to calculate the frequency response.
For simplicity of computation, assume that
ea(t)=v(t)−e1(t)=Easin(ωt) | (4.9) |
is known. The following derivation is to obtain the input signal v(t), denoted as v0sin(ωt+θv). When the signal ea(t) goes through different channels of the block diagram, we can get that
d11(t)=A11Easin(ωt+θG),d12(t)=A12Easin(ωt+2θG),d21(t)=A21Easin(ωt+θG), | (4.10) |
where
A11=N(Ea)lk11|G(s)|,A12=N(Ea)l2k12|G(s)|2,A21=N(Ea)l2k12|G(s)|, | (4.11) |
in which |G(s)| and θG are the magnitude and phase angle of 1/s, respectively. Denote
eb(t)=e2(t)−e3(t)=Ebsin(ωt+θEb), | (4.12) |
where Eb and θEb will be determined later. Let the signal eb(t) go through different channels of the block diagram to obtain that
e4(t)=A4Ebsin(ωt+θEb+θG),d13(t)=A13Ebsin(ωt+θEb+3θG),d22(t)=A22Ebsin(ωt+θEb+2θG),d31(t)=A31Ebsin(ωt+θEb+θG), | (4.13) |
where
A4=N(Eb)l2k22|G(s)|,A13=N(Eb)l2k22|G(s)|3,A22=N(Eb)l2k22|G(s)|2,A31=N(Eb)lk21|G(s)|. | (4.14) |
From Figure 3 we can see that
e1(t)=e10sin(ωt+θe1)=d11(t)+d12(t)+d13(t),e2(t)=e20sin(ωt+θe2)=d21(t)+d22(t),e3(t)=e30sin(ωt+θe3)=d31(t)+d22(t). | (4.15) |
Using the Eqs (4.12) and (4.15), it is easy to get that
eb(t)=d21(t)−d31(t). | (4.16) |
Thus, the phase angle and the amplitude of the signal eb(t) can be obtained after deduction and simplification and are described by
θEb=−π4,N(Eb)Eb=N(Ea)Ealk12sinθGk21sin(θEb+θG). | (4.17) |
Next, from (4.9) and (4.15), the input signal can be denoted as
v0sin(ωt+θv)=ea(t)+d11(t)+d12(t)+d13(t). | (4.18) |
Therefore, after deduction and simplification, the phase angle and the amplitude of the signal v(t) are as following
θv=arctan(Ea(1+A11sinθG+A12sin2θG)+A13Ebsin(θEb+3θG)Ea(1+A11cosθG+A12cos2θG)+A13Ebcos(θEb+3θG)),v0=(Ea(1+A11sinθG+A12sin2θG)+A13Ebsin(θEb+3θG))/sinθv. | (4.19) |
Furthermore, using the aforementioned numerical method and (4.10), (4.13) and (4.15), the phase angles and the amplitudes of outputs of the nonlinear system can be denoted as
θe1=arctan(Ea(A11sinθG+A12sin2θG)+A13Ebsin(θEb+3θG)Ea(A11cosθG+A12cos2θG)+A13Ebcos(θEb+3θG)),e10=(Ea(A11sinθG+A12sin2θG)+A13Ebsin(θEb+3θG))/sinθe1,θe2=arctan(A21EasinθG+A22Ebsin(θEb+2θG)A21EacosθG+A22Ebcos(θEb+2θG)),e20=(A21EasinθG+A22Ebsin(θEb+2θG))/sinθe2,θe3=arctan(A31Ebsin(θEb+θG)+A22Ebsin(θEb+2θG)A31Ebcos(θEb+θG)+A22Ebcos(θEb+2θG)),e30=(A31Ebsin(θEb+θG)+A22Ebsin(θEb+2θG))/sinθe3. | (4.20) |
At this moment, the magnitude frequency response for the nonlinear system can be described as
G1nonlinear=e10v0,G2nonlinear=e20v0,G3nonlinear=e30v0,G4nonlinear=A4Ebv0, | (4.21) |
which change for various values of the frequency ω and the dead zone nonlinearity parameter √σ.
Using the transfer functions for the linear system in (4.8) and the numerical solution for the nonlinear system, the frequency responses of both |Glinear| and |Gnonlinear| can be achieved. Figure 4 shows the adaptation of the dead zone parameter (left) and the magnitude bode-plots of |G4nonlinear| with different σ (right). In this figure, we set Ea=1, and other parameters are the same as in Section 4.1. Note that the curve for the the nonlinear system with σ=0 is the numerical solution for the linear system. As can be seen from Figure 4 that with an increase in σ, the magnitude in the frequency response decreases gradually. Thus, increasing σ results in the increase of the high frequency noise suppression ability. From this point of view, the DZ-CESO is superior to LPESO in noise suppression ability due to the advantage of the dynamic dead-zone mechanism.
We proposed a cascade dead-zone ESO for nth-order nonlinear uncertain systems with measurement noise in this paper. For simplicity, we considered the plant in the phase-variable form. In fact, design method of DZ-CESO can be generalized to address more general forms of observability. Although there is a tradeoff between tracking speed and noise amplification, the low power structure and dynamic dead-zone mechanism improve the estimation effect when there exists high frequency noise. Therefore, a bigger high gain parameter l can be selected to better reject the disturbance. The proposed observer can be used for various settings, such as output regulation, output feedback stabilization and fault detection.
In this paper, an ESO algorithm is designed for continuous compound disturbance and bounded measurement noise. The assumption of bounded derivative of the total disturbance is conservative. In the following work, we will relax this assumption to further improve the DZ-CESO, then design the controller and investigate the stability of the whole closed loop system.
The authors have no conflicts of interest.
[1] |
A. Q. Liu, T. Li, Y. Gu, H. H. Dai, Cooperative extended state observer based control of vehicle platoons with arbitrarily small time headway, Automatica, 129 (2021), 109678. https://doi.org/10.1016/j.automatica.2021.109678 doi: 10.1016/j.automatica.2021.109678
![]() |
[2] |
K. Rsetam, Z. W. Cao, Z. H. Man, Cascaded-extended-state-observer-based sliding-mode control for underactuated flexible joint robot, IEEE Trans. Ind. Electron., 67 (2020), 10822–10832. https://doi.org/10.1109/TIE.2019.2958283 doi: 10.1109/TIE.2019.2958283
![]() |
[3] |
Y. Cheng, X. M. Ren, D. D. Zheng, L. W. Li, Non-linear bandwidth extended-state-observer based non-smooth funnel control for motor-drive servo systems, IEEE Trans. Ind. Electron., 69 (2022), 6215–6224. https://doi.org/10.1109/TIE.2021.3095811 doi: 10.1109/TIE.2021.3095811
![]() |
[4] |
S. Shao, Z. Gao, On the conditions of exponential stability in active disturbance rejection control based on singular perturbation analysis, Int. J. Control, 90 (2017), 2085–2097. https://doi.org/10.1080/00207179.2016.1236217 doi: 10.1080/00207179.2016.1236217
![]() |
[5] |
Z. L. Zhao, B. Z. Guo, A nonlinear extended state observer based on fractional power functions, Automatica, 81 (2017), 286–296. https://doi.org/10.1016/j.automatica.2017.03.002 doi: 10.1016/j.automatica.2017.03.002
![]() |
[6] |
J. X. Wang, S. H. Li, J. Yang, B. Wu, Q. Li, Extended state observer-based sliding mode control for PWM-based DC–DC buck powerCc onverter systems with mismatched disturbances, IET Control Theory Appl., 9 (2015), 579–586. https://doi.org/10.1049/iet-cta.2014.0220 doi: 10.1049/iet-cta.2014.0220
![]() |
[7] |
S. Chen, W. C. Xue, S. Zhong, Y. Huang, On comparison of modified ADRCs for nonlinear uncertain systems with time delay, Sci. China Inf. Sci., 61 (2018), 1–15. https://doi.org/10.1007/s11432-017-9403-x doi: 10.1007/s11432-017-9403-x
![]() |
[8] |
X. Y. Zhang, H. Pan, W. Y. Bai, S. Zhong, Y. Huang, W. C. Xue, On observability analysis for a class of uncertain systems with coupling dynamics of rigid body and elastic vibration, 2020 39th Chinese Control Conference (CCC), 2020,730–735. https://doi.org/10.23919/CCC50068.2020.9189008 doi: 10.23919/CCC50068.2020.9189008
![]() |
[9] |
W. C. Xue, Y. Huang, Z. Q. Gao, On ADRC for non-minimum phase systems: canonical form selection and stability conditions, Control Theory Technol., 14 (2016), 199–208. https://doi.org/10.1007/s11768-016-6041-6 doi: 10.1007/s11768-016-6041-6
![]() |
[10] |
H. Razmjooei, G. Palli, E. Abdi, Continuous finite-time extended state observer design for electro-hydraulic systems, J. Franklin Inst., 359 (2022), 5036–5055. https://doi.org/10.1016/j.jfranklin.2022.04.030 doi: 10.1016/j.jfranklin.2022.04.030
![]() |
[11] |
H. Razmjooei, G. Palli, E. Abdi, M. Terzo, S. Strano, Design and experimental validation of an adaptive fast-finite-time observer on uncertain electro-hydraulic systems, Control Eng. Pract., 131 (2023), 105391. https://doi.org/10.1016/j.conengprac.2022.105391 doi: 10.1016/j.conengprac.2022.105391
![]() |
[12] |
H. Razmjooei, G. Palli, F. Janabi-Sharifi, S. Alirezaee, Adaptive fast-finite-time extended state observer design for uncertain electro-hydraulic systems, Eur. J. Control, 69 (2023), 100749. https://doi.org/10.1016/j.ejcon.2022.100749 doi: 10.1016/j.ejcon.2022.100749
![]() |
[13] |
Z. Q. Gao, Scaling and bandwidth-parameterization based controller tuning, Proceedings of the 2003 American Control Conference, 2003, 4989–4996. https://doi.org/10.1109/ACC.2003.1242516 doi: 10.1109/ACC.2003.1242516
![]() |
[14] |
A. A. Prasov, H. K. Khalil, A nonlinear high-gain observer for systems with measurement noise in a feedback control framework, IEEE Trans. Autom. Control, 58 (2013), 569–580. https://doi.org/10.1109/TAC.2012.2218063 doi: 10.1109/TAC.2012.2218063
![]() |
[15] |
S. Battilotti, Robust observer design under measurement noise with gain adaptation and saturated estimates, Automatica, 81 (2017), 75–86. https://doi.org/10.1016/j.automatica.2017.02.008 doi: 10.1016/j.automatica.2017.02.008
![]() |
[16] |
J. Ahrens, H. Khalil, High-gain observers in the presence of measurement noise: a switched-gain approach, Automatica, 45 (2009), 936–943. https://doi.org/10.1016/j.automatica.2008.11.012 doi: 10.1016/j.automatica.2008.11.012
![]() |
[17] |
D. Astolfi, L. Marconi, A high-gain nonlinear observer with limited gain power, IEEE Trans. Autom. Control, 60 (2015), 3059–3064. https://doi.org/10.1109/TAC.2015.2408554 doi: 10.1109/TAC.2015.2408554
![]() |
[18] |
X. Y. Li, H. Xia, A new extended state observer with low sensitivity to high frequency noise and low gain power, IFAC PapersOnLine, 53 (2020), 4929–4934. https://doi.org/10.1016/j.ifacol.2020.12.1072 doi: 10.1016/j.ifacol.2020.12.1072
![]() |
[19] |
K. Łakomy, R. Madonski, Cascade extended state observer for active disturbance rejection control applications under measurement noise, ISA Trans., 109 (2021), 1–10. https://doi.org/10.1016/j.isatra.2020.09.007 doi: 10.1016/j.isatra.2020.09.007
![]() |
[20] |
H. Sun, R. Madonski, S. H. Li, Y. Zhang, W. C. Xue, Composite control design for systems with uncertainties and noise using combined extended state observer and kalman filter, IEEE Trans. Ind. Electron., 69 (2022), 4119–4128. https://doi.org/10.1109/TIE.2021.3075838 doi: 10.1109/TIE.2021.3075838
![]() |
[21] |
R. Madonski, P. Herman, Method of sensor noise attenuation in high-gain observers-experimental verification on two laboratory systems, 2012 IEEE International Symposium on Robotic and Sensors Environments Proceedings, 2012,121–126. https://doi.org/10.1109/ROSE.2012.6402616 doi: 10.1109/ROSE.2012.6402616
![]() |
[22] |
M. Cocetti, S. Tarbouriech, L. Zaccarian, High-gain dead-zone observers for linear and nonlinear plants, IEEE Control. Syst. Lett., 3 (2019), 356–361. https://doi.org/10.1109/LCSYS.2018.2880931 doi: 10.1109/LCSYS.2018.2880931
![]() |
[23] |
D. Astolfi, L. Marconi, L. Praly, A. R. Teel, Low-power peaking-free high-gain observers, Automatica, 98 (2018), 169–179. https://doi.org/10.1016/j.automatica.2018.09.009 doi: 10.1016/j.automatica.2018.09.009
![]() |
[24] |
D. Wu, K. Chen, Frequency-domain analysis of nonlinear active disturbance rejection control via the describing function method, IEEE Trans. Ind. Electron., 60 (2013), 3906–3914. https://doi.org/10.1109/TIE.2012.2203777 doi: 10.1109/TIE.2012.2203777
![]() |
1. | Shihang Li, Zeyi Li, Zhiheng Zhang, Peng Liu, Jianfeng Cui, 2023, Distributed Lattice Kalman Filtering, 979-8-3503-0375-9, 3212, 10.1109/CAC59555.2023.10451589 | |
2. | Chong Zhao, Fan Zhou, Yanjun Shen, Fuzzy observer design for sampled nonlinear systems with measurement uncertainty, 2024, 26, 1561-8625, 1939, 10.1002/asjc.3312 |
LESO | LPESO | DZ-CESO | |
l=10(ˆx1(0)=0) | 5.5644 | 5.3976 | 5.4053 |
l=20(ˆx1(0)=0.5) | 2.9436 | 2.9332 | 2.9679 |
l=30(ˆx1(0)=0.5) | 1.9972 | 1.9936 | 2.0053 |
l=10(ˆx1(0)=0) | l=20(ˆx1(0)=0.5) | l=30(ˆx1(0)=0.5) | |
‖e1(t)‖∞ | 0.1172 | 0.5000 | 0.5000 |
‖e2(t)‖∞ | 5 | 5.7394 | 9.5657 |
‖e3(t)‖∞ | 46.8049 | 63.3846 | 127.9371 |
l=10(ˆx1(0)=0) | l=20(ˆx1(0)=0.5) | l=30(ˆx1(0)=0.5) | |
‖e1(t)‖∞ | 0.2022 | 0.5000 | 0.5000 |
‖e2(t)‖∞ | 5.6699 | 5 | 5.7548 |
‖e3(t)‖∞ | 46.5124 | 48.2156 | 42.9333 |
l=10(ˆx1(0)=0) | l=20(ˆx1(0)=0.5) | l=30(ˆx1(0)=0.5) | |
‖e1(t)‖∞ | 0.1891 | 0.5446 | 0.5296 |
‖e2(t)‖∞ | 5 | 5 | 5 |
‖e3(t)‖∞ | 39.3851 | 42.5383 | 48.6312 |
(a) | (b) | (c) | |
‖v(t)‖∞ | 9.6790 | 5 | 5 |
‖e1(t)‖∞ | 4.3875 | 0.2762 | 1.8548 |
‖e2(t)‖∞ | 38.9742 | 5.4794 | 18.7378 |
‖e3(t)‖∞ | 125.9236 | 55.1586 | 98.4120 |
ENR of e1 | 0.4331 | 0.0301 | 0.2961 |
ENR of e2 | 3.9908 | 0.3005 | 2.9569 |
ENR of e3 | 13.0557 | 1.0016 | 9.8504 |
(a) | (b) | (c) | |
‖v(t)‖∞ | 9.6790 | 5 | 5 |
‖e1(t)‖∞ | 3.5098 | 0.3120 | 1.3656 |
‖e2(t)‖∞ | 20.6755 | 5.9730 | 7.8698 |
‖e3(t)‖∞ | 46.9397 | 49.7482 | 52.8344 |
ENR of e1 | 0.3779 | 0.0201 | 0.2010 |
ENR of e2 | 2.3023 | 0.0123 | 0.3972 |
ENR of e3 | 5.5629 | 0.0295 | 0.9919 |
(a) | (b) | (c) | |
‖v(t)‖∞ | 9.6790 | 5 | 5 |
‖e1(t)‖∞ | 3.0672 | 0.2867 | 1.2620 |
‖e2(t)‖∞ | 4.2285 | 5 | 5 |
‖e3(t)‖∞ | 41.6543 | 41.8767 | 43.1932 |
ENR of e1 | 0.3156 | 0.0199 | 0.1955 |
ENR of e2 | 0.6097 | 0.0106 | 0.1748 |
ENR of e3 | 2.3798 | 0.0258 | 0.4233 |
LESO | LPESO | DZ-CESO | |
l=10(ˆx1(0)=0) | 5.5644 | 5.3976 | 5.4053 |
l=20(ˆx1(0)=0.5) | 2.9436 | 2.9332 | 2.9679 |
l=30(ˆx1(0)=0.5) | 1.9972 | 1.9936 | 2.0053 |
l=10(ˆx1(0)=0) | l=20(ˆx1(0)=0.5) | l=30(ˆx1(0)=0.5) | |
‖e1(t)‖∞ | 0.1172 | 0.5000 | 0.5000 |
‖e2(t)‖∞ | 5 | 5.7394 | 9.5657 |
‖e3(t)‖∞ | 46.8049 | 63.3846 | 127.9371 |
l=10(ˆx1(0)=0) | l=20(ˆx1(0)=0.5) | l=30(ˆx1(0)=0.5) | |
‖e1(t)‖∞ | 0.2022 | 0.5000 | 0.5000 |
‖e2(t)‖∞ | 5.6699 | 5 | 5.7548 |
‖e3(t)‖∞ | 46.5124 | 48.2156 | 42.9333 |
l=10(ˆx1(0)=0) | l=20(ˆx1(0)=0.5) | l=30(ˆx1(0)=0.5) | |
‖e1(t)‖∞ | 0.1891 | 0.5446 | 0.5296 |
‖e2(t)‖∞ | 5 | 5 | 5 |
‖e3(t)‖∞ | 39.3851 | 42.5383 | 48.6312 |
(a) | (b) | (c) | |
‖v(t)‖∞ | 9.6790 | 5 | 5 |
‖e1(t)‖∞ | 4.3875 | 0.2762 | 1.8548 |
‖e2(t)‖∞ | 38.9742 | 5.4794 | 18.7378 |
‖e3(t)‖∞ | 125.9236 | 55.1586 | 98.4120 |
ENR of e1 | 0.4331 | 0.0301 | 0.2961 |
ENR of e2 | 3.9908 | 0.3005 | 2.9569 |
ENR of e3 | 13.0557 | 1.0016 | 9.8504 |
(a) | (b) | (c) | |
‖v(t)‖∞ | 9.6790 | 5 | 5 |
‖e1(t)‖∞ | 3.5098 | 0.3120 | 1.3656 |
‖e2(t)‖∞ | 20.6755 | 5.9730 | 7.8698 |
‖e3(t)‖∞ | 46.9397 | 49.7482 | 52.8344 |
ENR of e1 | 0.3779 | 0.0201 | 0.2010 |
ENR of e2 | 2.3023 | 0.0123 | 0.3972 |
ENR of e3 | 5.5629 | 0.0295 | 0.9919 |
(a) | (b) | (c) | |
‖v(t)‖∞ | 9.6790 | 5 | 5 |
‖e1(t)‖∞ | 3.0672 | 0.2867 | 1.2620 |
‖e2(t)‖∞ | 4.2285 | 5 | 5 |
‖e3(t)‖∞ | 41.6543 | 41.8767 | 43.1932 |
ENR of e1 | 0.3156 | 0.0199 | 0.1955 |
ENR of e2 | 0.6097 | 0.0106 | 0.1748 |
ENR of e3 | 2.3798 | 0.0258 | 0.4233 |