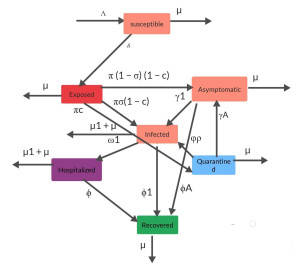
The Covid illness (COVID-19), which has emerged, is a highly infectious viral disease. This disease led to thousands of infected cases worldwide. Several mathematical compartmental models have been examined recently in order to better understand the Covid disease. The majority of these models rely on integer-order derivatives, which are incapable of capturing the fading memory and crossover behaviour observed in many biological phenomena. Similarly, the Covid disease is investigated in this paper by exploring the elements of COVID-19 pathogens using the non-integer Atangana-Baleanu-Caputo derivative. Using fixed point theory, we demonstrate the existence and uniqueness of the model's solution. All basic properties for the given model are investigated in addition to Ulam-Hyers stability analysis. The numerical scheme is based on Lagrange's interpolation polynomial developed to estimate the model's approximate solution. Using real-world data, we simulate the outcomes for different fractional orders in Matlab to illustrate the transmission patterns of the present Coronavirus-19 epidemic through graphs.
Citation: Ihtisham Ul Haq, Nigar Ali, Shabir Ahmad. A fractional mathematical model for COVID-19 outbreak transmission dynamics with the impact of isolation and social distancing[J]. Mathematical Modelling and Control, 2022, 2(4): 228-242. doi: 10.3934/mmc.2022022
[1] | Anil Chavada, Nimisha Pathak, Sagar R. Khirsariya . A fractional mathematical model for assessing cancer risk due to smoking habits. Mathematical Modelling and Control, 2024, 4(3): 246-259. doi: 10.3934/mmc.2024020 |
[2] | Abduljawad Anwar, Shayma Adil Murad . On the Ulam stability and existence of $ L^p $-solutions for fractional differential and integro-differential equations with Caputo-Hadamard derivative. Mathematical Modelling and Control, 2024, 4(4): 439-458. doi: 10.3934/mmc.2024035 |
[3] | Saïd Abbas, Mouffak Benchohra, Johnny Henderson . Random Caputo-Fabrizio fractional differential inclusions. Mathematical Modelling and Control, 2021, 1(2): 102-111. doi: 10.3934/mmc.2021008 |
[4] | Anil Chavada, Nimisha Pathak . Transmission dynamics of breast cancer through Caputo Fabrizio fractional derivative operator with real data. Mathematical Modelling and Control, 2024, 4(1): 119-132. doi: 10.3934/mmc.2024011 |
[5] | C. Kavitha, A. Gowrisankar . Fractional integral approach on nonlinear fractal function and its application. Mathematical Modelling and Control, 2024, 4(3): 230-245. doi: 10.3934/mmc.2024019 |
[6] | Mlyashimbi Helikumi, Paride O. Lolika . Dynamics and analysis of COVID-19 disease transmission: The effect of vaccination and quarantine. Mathematical Modelling and Control, 2023, 3(3): 192-209. doi: 10.3934/mmc.2023017 |
[7] | Ihtisham Ul Haq, Nigar Ali, Hijaz Ahmad . Analysis of a chaotic system using fractal-fractional derivatives with exponential decay type kernels. Mathematical Modelling and Control, 2022, 2(4): 185-199. doi: 10.3934/mmc.2022019 |
[8] | J. O. Akanni, S. Ajao, S. F. Abimbade, Fatmawati . Dynamical analysis of COVID-19 and tuberculosis co-infection using mathematical modelling approach. Mathematical Modelling and Control, 2024, 4(2): 208-229. doi: 10.3934/mmc.2024018 |
[9] | Mrutyunjaya Sahoo, Dhabaleswar Mohapatra, S. Chakraverty . Wave solution for time fractional geophysical KdV equation in uncertain environment. Mathematical Modelling and Control, 2025, 5(1): 61-72. doi: 10.3934/mmc.2025005 |
[10] | Erick Manuel Delgado Moya, Diego Samuel Rodrigues . Fractional order modeling for injectable and oral HIV pre-exposure prophylaxis. Mathematical Modelling and Control, 2023, 3(2): 139-151. doi: 10.3934/mmc.2023013 |
The Covid illness (COVID-19), which has emerged, is a highly infectious viral disease. This disease led to thousands of infected cases worldwide. Several mathematical compartmental models have been examined recently in order to better understand the Covid disease. The majority of these models rely on integer-order derivatives, which are incapable of capturing the fading memory and crossover behaviour observed in many biological phenomena. Similarly, the Covid disease is investigated in this paper by exploring the elements of COVID-19 pathogens using the non-integer Atangana-Baleanu-Caputo derivative. Using fixed point theory, we demonstrate the existence and uniqueness of the model's solution. All basic properties for the given model are investigated in addition to Ulam-Hyers stability analysis. The numerical scheme is based on Lagrange's interpolation polynomial developed to estimate the model's approximate solution. Using real-world data, we simulate the outcomes for different fractional orders in Matlab to illustrate the transmission patterns of the present Coronavirus-19 epidemic through graphs.
A novel coronavirus (SARS-CoV-2) emerged out of Wuhan city at the end of December, 2019 [1,2]. After spread to almost all countries and was declared a pandemic [3]. The outbreak has mostly been controlled in some countries since the end of April 2021, but still remains a series of public health and social-economic the problem in all over the world [4,5,6]. We know that most people infected with 2019-nCoV will experience mild to moderate respiratory illness, such as difficulty in breathing, sickness, cough, fever, and other symptoms [11,13,14,15]. When an infected person sneezes, coughs, or comes in touch with a contaminated surface, the virus is mostly spread to healthy people via the mouth, nose, and eyes [7,8]. The usual incubation time is 1 to 14 days, according to [9]. As the vaccine is not yet available everywhere, the control measures, for example, social distancing, wearing of masks, regular hand sanitation using sanitizer or soaps, and quarantine of the suspected individuals, are effective interventions that control the virus.
Mathematical models are useful tools for analysing outbreak dynamics. Mathematical models can be used to predict the virus's future spread, allowing the government to be prepared and take the necessary actions [18,19,20]. Several mathematical models were developed to better understand the behaviour of coronavirus disease. Researchers in [21] introduced a novel fractional model of COVID-19 while accounting for the effects of isolation and quarantine. The Mittag-Leffler, exponential kernels and power-law were used to simulate the proposed model. Mathematical modeling is an essential tool for formulating control strategies and forces guessing the spreading of COVID-19. To illustrate the dynamics of COVID-19, Chen and Zhao [7] established a susceptible, un-quarantine infected, quarantine infected, confirmed infected (SUQC) model. Similarly, Song et al. [17] formulated a mathematical model based on COVID-19 epidemiology, close contact, and the isolation of healthy persons. Acknowledge the means to control the spread of coronavirus in the people has been recorded in various mathematical modeling [12,22].
Fractional calculus is the computation of integrals or derivatives of any positive real order. In recent years, there has been a lot of interest in the use of fractional differential equations. Many researchers use fractional differential equations in their research [24,27,46]. Therefore, many researchers have shown more interest in studying fractional derivative and fractional integrals. In the investigation of differential equation for optimization, numerical and qualitative importance was contributed by researchers [25,26]. It is also notable that fractional differential equation has been characterized in various manners. It is well-known that regular kernels are not included in the definite integral. Therefore types of seeds have been included in different definitions. One crucial definition that has recently attracted the attention of researchers is the ABC derivative presented by Atangana, Baleanu, and Caputo [28] in 2016. This derivative exhibits the singular kernel by non-singular kernel, and So much has been studied [29,30]. Because most of the non-linear problems are challenging to solve for their analytical or exact solution, different numerical methods have been introduced to solve these problems [10,23,31,32]. The best numerical procedure has recently been developed to solve partial fractional differential equation under the ABC derivative; see for detail [33,34].
The following model investigates the COVID-19 transmission dynamic. The model included seven compartments: susceptible population S, expose population E, infected papulation I, Asymptomatic population A, Quarantined population Q, Hospitalized population H and the removed class R as:
{ABC0Dαt[S(t)]=Λ−(δ+μ)S,ABC0Dαt[E(t)]=δS−(π+μ)E,ABC0Dαt[I(t)]=πσ(1−c)E+ϕρQ+γ1A−(φ1+ω1+μ1+μ)I,ABC0Dαt[A(t)]=π(1−σ)(1−c)E+γAQ−(γ1+φA+μ)A,ABC0Dαt[Q(t)]=πcE−(γA+ϕρ+μ)Q,ABC0Dαt[H(t)]=ω1I−(φH+μ1+μ)H,ABC0Dαt[R(t)]=φ1I+φAA+φHH−μR, | (1.1) |
with the following initial conditions S(0)>0,E(0)≥0,I(0)≥0,A(0)≥0,Q(0)≥,H(t)≥0,R(0)≥0. The COVID-19 transmission dynamics are represented in the Figure 1 flow chart.
Some essential assumptions we put on the model are that all of the model (1.1) involved parameters be non-negative. The above model is examined from three perspectives. First, since the presented model (1.1) is recently defined, we use fixed point theory to demonstrate its existence. However, since stability is vital, we will examine Hyers-Ulam type stability for the proposed model. We refer to [43,44,45] for further information on the general models that use ordinary derivatives of fractional order. Furthermore, the model under consideration is nonlinear because it is sometimes impossible to discover an accurate solution to nonlinear situations. Therefore several numerical processes (methods) have been developed in the literature to deal with similar problems, see [39,40,41,42]. Therefore a Lagrange's interpolation polynomial approach is used to simulate the findings using Matlab-16.
We arranged the paper as Section 1 gives the introduction, section 2 describes preliminaries, section 3 shows the existence of the solution; section 4 offers uniqueness solution to the model, section 5 provides Hyers Ulams Stability analyses, and section 6 gives the numerical solution. Section 7 shows a graphical representation to support the analytical result. Finally, in section 8, we discuss the conclusion.
Parameters | Biological interpretations |
Describes the birth rate | |
The effective contact rate of social | |
distancing | |
Natural death rate | |
Shows the infected contact rate | |
The rate of symptoms among infected | |
people | |
Showed the death rate due to the | |
coronavirus | |
Recovery rates of individuals in the | |
infected class | |
Recovery rates of individuals in the | |
asymptomatic population | |
The transmission rate from the infected | |
class to the quarantined class | |
Shows the transition rate from | |
asymptomatic population to the | |
infected class | |
Represents the movement rate from the | |
quarantined quarantined population to the | |
asymptomatic population | |
Represented the rate of quarantine for | |
exposed persons | |
Recovery rate of individuals in the | |
hospitalized population |
Definition 2.1. Let F∈H1(a,b),b>a, for any α∈[0,1], the ABC fractional derivative is
ABC0DαtF(z)=ϰ(α)1−α∫t0F′(S)Eα[−α(t−S)1−α]dS, |
where E is a Mittag-Leffler function and ϰ(α) satisfied ϰ(0)=ϰ(1)=1.
Definition 2.2. Let F∈H1(a,b),b>a, and 0≤α≤1, the ABC fractional integral is
ABC0IαtF(z)=1−αϰ(α)F(z)+αϰ(α)Γ(α)∫t0F(S)(t−S)α−1dS. |
Lemma 2.1. The following Newton Leibniz formula is satisfied for any F(t)∈H1(a,b)
ABC0Iαt(ABC0DαtF(t))=F(0)−F(t). |
Applying the Lemma (2.1) to (1.1), we get
S(t)−S(0)=1−αϰ(α)(Λ−(δ+μ)S)+αϰ(α)Γ(α)∫t0(t−S)α−1(Λ−(δ+μ)S)dS.E(t)−E(0)=1−αϰ(α)(δS−(π+μ)E)+αϰ(α)Γ(α)∫t0(t−S)α−1(Λ−(δ+μ)S)dS.I(t)−I(0)=1−αϰ(α)(πσ(1−c)E+ϕρQ+γ1A−(φ1+ω1+μ1+μ)I)+αϰ(α)Γ(α)∫ta(t−S)α−1(πσ(1−c)E+ϕρQ+γ1A−(φ1+ω1+μ1+μ)I)dS.A(t)−A(0)=1−αϰ(α)(π(1−σ)(1−c)E+γAQ−(γ1+φA+μ)A)+αϰ(α)Γ(α)∫t0(t−S)α−1(π(1−σ)(1−c)E+γAQ−(γ1+φA+μ)A)dS.Q(t)−Q(0)=1−αϰ(α)(πcE−(γA+ϕρ+μ)Q)∫t0(t−S)α−1(πcE−(γA+ϕρ+μ)Q)dS.H(t)−H(0)=1−αϰ(α)(ω1I−(φH+μ1+μ)H)+αϰ(α)Γ(α)+αϰ(α)Γ(α)∫t0(t−S)α−1(ω1I−(φH+μ1+μ)H)dS.R(t)−R(0)=1−αϰ(α)(φ1I+φAA+φHH−μR)+αϰ(α)Γ(α)∫t0(t−S)α−1(φ1I+φAA+φHH−μR)dS. |
Suppose the function Gi for i=1,2...7 given below
P1(t,S)=Λ−(δ+μ)SG2(t,E)=δS−(π+μ)EG3(t,I)=πσ(1−c)E+ϕρQ+γ1A−(φ1+ω1+μ1+μ)IG4(t,A)=π(1−σ)(1−c)E+γAQ−(γ1+φA+μ)AG5(t,Q)=πcE−(γA+ϕρ+μ)QG6(t,H)=ω1I−(φH+μ1+μ)HG7(t,R)=φ1I+φAA+φHH−μR. |
We defined
{Θ1=|δ+μ|Θ2=|π+μ|Θ3=|φ1+ω1+μ1+μ|Θ4=|γ1+φA+μ|Θ5=|γA+ϕρ+μ|Θ6=|φH+μ1+μ|Θ7=|μ|. | (3.1) |
Let
● (p), let, for S,S∗,E,E∗,I,I∗,A,A∗,Q,Q∗,H, H∗,R,R∗∈L[0,1], there exits constants Wi>0, for i=1,2,3,4,5,6,7 such that ‖S‖≤W1,‖E‖≤W2,‖I‖≤W3,‖A‖≤W4,‖Q‖≤W5,‖H‖≤W6,‖R‖≤W7 and η1,η2>0.
‖S+E+I+Q+H‖≤η1 | (3.2) |
‖A+R‖≤η2. | (3.3) |
The Gi for i∈N71 satisfy the Lipschitz condition provided that (p) is obeyed. First, we take for G1(t,S), we have
G1(S)S−G1(S∗)=‖(Λ−(δ+μ)S)−(Λ−(δ+μ)S∗)‖≤|(δ+μ)|‖(S−S∗)‖≤Θ1‖(S−S∗)‖. |
For the G2(t,E), we have
G2(E)−G2(E∗)=‖(δS−(π+μ)E)−(δS−(π+μ)E∗)‖≤|(π+μ)|‖(E−E∗)‖≤Θ2‖(E−E∗)‖. |
For the G3(t,I), we have
G3(I)−G3(I∗)=‖(πσ(1−c)E+ϕρQ+γ1A(φ1+ω1+μ1+μ)I)−(πσ(1−c)E+ϕρQ+γ1A−(φ1+ω1+μ1+μ)I∗)‖≤|(φ1+ω1+μ1+μ)|‖(I−I∗)‖≤Θ3‖(I−I∗)‖. |
For the G4(t,A), we have
G4(A)−G4(A∗)=‖(π(1−σ)(1−c)E+γAQ−(γ1+φA+μ)A)−(π(1−σ)(1−c)E+γAQ−(γ1+φA+μ)A∗)‖≤|(γ1+φA+μ)|‖(A−A∗)‖≤Θ4‖(A−A∗)‖. |
Now, for G5(t,Q), we have
G5(Q)−G5(Q∗)=‖(πcE−(γA+ϕρ+μ)Q)−(πcE−(γA+ϕρ+μ)Q∗)‖≤|(γA+ϕρ+μ)|‖(Q−Q∗)‖≤Θ5‖(Q−Q∗)‖. |
For the G6(t,H), we have
G6(H)−G6(H∗)=‖(ω1I−(φH+μ1+μ)H)−(ω1I−(φH+μ1+μ)H∗)‖≤|(φH+μ1+μ)|‖(H−H∗)‖≤Θ6‖(H−H∗)‖. |
Similarly, for the G7(t,R), we have
G7(R)−G7(R∗)=‖(φ1I+φAA+φHH−μR)−(φ1I+φAA+φHH−μR∗)‖≤|μ|‖(R−R∗)‖≤Θ7‖(R−R∗)‖. |
Hence, the Gi, for i=1,2,...7. satisfies the lipschitz condition. We suppose that S(0)=E(0)=A(0)=I(0)=Q(0)=H(0)=R(0)=0, we have
S(t)=1−αϰ(α)G1(t,S(t))+αϰ(α)Γ(α)∫t0(t−z)α−1G1(z,S(z))dzE(t)=1−αϰ(α)G2(t,E(t))+αϰ(α)Γ(α)∫t0(t−z)α−1G2(z,E(z))dzI(t)=1−αϰ(α)G3(t,I(t))+αϰ(α)Γ(α)∫t0(t−z)α−1G3(z,I(z))dzA(t)=1−αϰ(α)G4(t,A(t))+αϰ(α)Γ(α)∫t0(t−z)α−1G4(z,A(z))dzQ(t)=1−αϰ(α)G5(t,Q(t))+αϰ(α∗5)Γ(α)∫ta(t−z)α−1G5(z,Q(z))dzH(t)=1−αϰ(α)G6(t,H(t))+αϰ(α)Γ(α)∫t0(t−z)α−1G6(z,H(z))dzR(t)=1−αϰ(α)G7(t,R(t))+αϰ(α)Γ(α)∫t0(t−z)α−1G7(z,R(z))dz. |
We defined the following iterative relation for (1.1) as
Sn(t)=1−αϰ(α)G1(t,Sn−1(t))+αϰ(α)Γ(α)∫t0(t−z)α−1G1(z,Sn−1(z))dzEn(t)=1−αϰ(α)G2(t,En−1(t))+αϰ(α)Γ(α)∫t0(t−z)α−1G2(z,En−1(z))dzIn(t)=1−αϰ(α)G3(t,In−1(t))+αϰ(α)Γ(α)∫t0(t−z)α−1G3(z,In−1(z))dzAn(t)=1−αϰ(α)G4(t,An−1(t))+αϰ(α)Γ(α)∫t0(t−z)α−1G4(z,An−1(z))dzQn(t)=1−αϰ(α)G5(t,Qn−1(t))+αϰ(α)Γ(α)∫t0(t−z)α−1G5(z,Qn−1(z))dzHn(t)=1−αϰ(α)G6(t,Hn−1(t))+αϰ(α)Γ(α)∫t0(t−z)α−1G6(z,Hn−1(z))dzRn(t)=1−αϰ(α)G7(t,Rn−1(t))+αϰ(α)Γ(α)∫t0(t−z)α−1G7(z,Rn−1(z))dz. |
Using the supposition (P) and (3.1), the model (1.1) has a solution Ω=max The functions we defined as
\begin{equation} \begin{alignedat}{1} & \varPi1_{n}(t) = \mathscr{S}_{n+1}-\mathscr{S}\\ & \varPi2_{n}(t) = \mathscr{E}_{n+1}-\mathscr{E},\\ & \varPi3_{n}(t) = \mathscr{I}_{n+1}-\mathscr{I},\\ & \varPi4_{n}(t) = \mathscr{A}_{n+1}-\mathscr{A},\\ & \varPi5_{n}(t) = \mathscr{Q}_{n+1}-\mathscr{Q},\\ & \varPi6_{n}(t) = \mathscr{H}_{n+1}-\mathscr{H},\\ & \varPi7_{n}(t) = \mathscr{R}_{n+1}-\mathscr{R}. \end{alignedat} \end{equation} | (3.4) |
Now, we using the definition (2.1) and (3.4), we get
\begin{eqnarray*} \begin{alignedat}{1}\left\Vert \varPi1_{n}\right\Vert = &\frac{1-\alpha}{\varkappa(\alpha)}\left\Vert \mathscr{G}_{1}(\mathscr{S}_{n})-\mathscr{G}_{1}(\mathscr{S}_{n-1})\right\Vert +\frac{\alpha}{\varkappa(\alpha)\varGamma(\alpha)}\\ &\int_{0}^{t}\left(t-z\right)^{\alpha-1}\left\Vert \mathscr{G}_{1}\left(z,\mathscr{S}_{n}(z)\right)-\mathscr{G}_{1}\left(z,\mathscr{S}_{n-1}(z)\right)\right\Vert dz\\ \leq& \left[\frac{1-\alpha}{\varkappa(\alpha)}+\frac{\alpha}{\varkappa(\alpha)\varGamma(\alpha)}\right]\varTheta_{1}\left\Vert \mathscr{S}_{n}-\mathscr{S}\right\Vert . \end{alignedat} \end{eqnarray*} |
Similarly, using the same procedure for the other compartments of the model (1.1)
\begin{eqnarray*} \begin{alignedat}{1}\left\Vert \varPi2_{n}\right\Vert = &\frac{1-\alpha}{\varkappa(\alpha)}\left\Vert \mathscr{G}_{2}(\mathscr{E}_{n})-x_{2}(\mathscr{E}_{n-1})\right\Vert +\frac{\alpha}{\varkappa(\alpha)\varGamma(\alpha)}\\ & \int_{0}^{t}\left(t-z\right)^{\alpha-1}\left\Vert \mathscr{G}_{2}\left(z,\mathscr{E}_{n}(z)\right)-\mathscr{G}_{2}\left(z,\mathscr{E}_{n-1}(z)\right)\right\Vert dz\\ \leq& \left[\frac{1-\alpha}{\varkappa(\alpha)}+\frac{\alpha}{\varkappa(\alpha_{2}^{*})\varGamma(\alpha)}\right]\varTheta_{2}\left\Vert \mathscr{E}_{n}-\mathscr{E}\right\Vert . \\ \left\Vert \varPi3_{n}\right\Vert = &\frac{1-\alpha}{\varkappa(\alpha)}\left\Vert \mathscr{G}_{3}(\mathscr{I}_{n})-\mathscr{G}_{3}(\mathscr{I}_{n-1})\right\Vert +\frac{\alpha}{\varkappa(\alpha)\varGamma(\alpha)}\\ & \int_{0}^{t}\left(t-z\right)^{\alpha-1}\left\Vert \mathscr{G}_{3}\left(z,\mathscr{I}_{n}(z)\right)-\mathscr{G}_{3}\left(z,\mathscr{I}_{n-1}(z)\right)\right\Vert dz\\ \leq&\left[\frac{1-\alpha}{\varkappa(\alpha)}+\frac{\alpha}{\varkappa(\alpha)\varGamma(\alpha)}\right]\varTheta_{3}\left\Vert \mathscr{I}_{n}-\mathscr{I}\right\Vert . \\ \left\Vert \varPi4_{n}\right\Vert = &\frac{1-\alpha}{\varkappa(\alpha)}\left\Vert \mathscr{G}_{4}\mathscr{A}_{n})-\mathscr{G}_{4}(\mathscr{A}_{n-1})\right\Vert +\frac{\alpha}{\varkappa(\alpha)\varGamma(\alpha)}\\ & \int_{0}^{t}\left(t-z\right)^{\alpha-1}\left\Vert \mathscr{G}_{4}\left(z,\mathscr{A}_{n}(z)\right)-\mathscr{G}_{4}\left(z,\mathscr{A}_{n-1}(z)\right)\right\Vert dz\\ \leq& \left[\frac{1-\alpha}{\varkappa(\alpha)}+\frac{\alpha}{\varkappa(\alpha)\varGamma(\alpha)}\right]\varTheta_{4}\left\Vert \mathscr{A}_{n}-\mathscr{A}\right\Vert . \end{alignedat} \end{eqnarray*} |
\begin{eqnarray*} \begin{alignedat}{1}\left\Vert \varPi5_{n}\right\Vert = &\frac{1-\alpha}{\varkappa(\alpha)}\left\Vert \mathscr{G}_{5}(\mathscr{Q}_{n})-\mathscr{G}_{5}(\mathscr{Q}_{n-1})\right\Vert +\frac{\alpha}{\varkappa(\alpha)\varGamma(\alpha)}\\ & \int_{0}^{t}\left(t-z\right)^{\alpha-1}\left\Vert \mathscr{G}_{5}\left(z,\mathscr{Q}_{n}(z)\right)-\mathscr{G}_{5}\left(z,\mathscr{Q}_{n-1}(z)\right)\right\Vert dz\\ \leq&\left[\frac{1-\alpha}{\varkappa(\alpha)}+\frac{\alpha}{\varkappa(\alpha)\varGamma(\alpha)}\right]\varTheta_{5}\left\Vert \mathscr{Q}_{n}-\mathscr{Q}\right\Vert . \\ \left\Vert \varPi6_{n}\right\Vert = & \frac{1-\alpha}{\varkappa(\alpha)}\left\Vert \mathscr{G}_{6}(\mathscr{H}_{n})-\mathscr{G}_{1}(\mathscr{H}_{n-1})\right\Vert +\frac{\alpha}{\varkappa(\alpha)\varGamma(\alpha)}\\ & \int_{0}^{t}\left(t-z\right)^{\alpha-1}\left\Vert \mathscr{G}_{6}\left(z,\mathscr{H}_{n}(z)\right)-\mathscr{G}_{6}\left(z,\mathscr{H}_{n-1}(z)\right)\right\Vert dz\\ \leq&\left[\frac{1-\alpha}{\varkappa(\alpha)}+\frac{\alpha}{\varkappa(\alpha)\varGamma(\alpha)}\right]\varTheta_{6}\left\Vert \mathscr{H}_{n}-\mathscr{H}\right\Vert . \\ \left\Vert \varPi7_{n}\right\Vert = &\frac{1-\alpha}{\varkappa(\alpha)}\left\Vert \mathscr{G}_{7}(\mathscr{R}_{n})-\mathscr{G}_{7}(\mathscr{R}_{n-1})\right\Vert +\frac{\alpha}{\varkappa(\alpha)\varGamma(\alpha)}\\ & \int_{0}^{t}\left(t-z\right)^{\alpha-1}\left\Vert \mathscr{G}_{7}\left(z,\mathscr{R}_{n}(z)\right)-\mathscr{G}_{1}\left(z,\mathscr{R}_{n-1}(z)\right)\right\Vert dz\\ \leq&\left[\frac{1-\alpha}{\varkappa(\alpha)}+\frac{\alpha}{\varkappa(\alpha)\varGamma(\alpha)}\right]\varTheta_{7}\left\Vert \mathscr{R}_{n}-\mathscr{R}\right\Vert . \end{alignedat} \end{eqnarray*} |
Hence, we have \varPi_{n}(t)\rightarrow0 as n\rightarrow \infty .
Theorem 4.1. With the supposition ( P ) the model (1.1) has unique solution if
\begin{equation} \left[\frac{1-\phi_{i}}{\mathscr{B}(\phi_{i})}+\frac{1}{\mathscr{B}(\phi_{i})\varGamma(\phi_{i})}\right]\varTheta_{i}\leq1,i\in\mathbb{\mathbb{N}}_{i}^{7}. \end{equation} | (4.1) |
Proof. We suppose that the solution of (1.1) is not unique, Let \{\mathscr{S}^{*}(t), \mathscr{E}^{*}(t), \mathscr{I}^{*}(t), \mathscr{A}^{*}(t), \mathscr{Q}^{*}(t), \mathscr{H}^{*}(t), \mathscr{R}^{*}(t)\} be another solution of (1.1), such that
\begin{align*} \mathscr{S}(t) = &\frac{1-\alpha}{\varkappa(\alpha)}\mathscr{G}_{1}\left(t,\mathscr{S}^{*}(t)\right) +\frac{\alpha}{\varkappa(\alpha)\varGamma(\alpha)}\int_{0}^{t}\left(t-z\right)^{\alpha-1} \\ &\mathscr{G}_{1}\left(z,\mathscr{S}^{*}(z)\right)dz \\ \mathscr{E}(t) = &\frac{1-\alpha}{\varkappa(\alpha)}\mathscr{G}_{2}\left(t,\mathscr{E}^{*}(t)\right) +\frac{\alpha}{\varkappa(\alpha)\varGamma(\alpha)}\int_{0}^{t}\left(t-z\right)^{\alpha-1} \\ &\mathscr{G}_{2}\left(z,\mathscr{E}^{*}(z)\right)dz \\ \mathscr{I}(t) = &\frac{1-\alpha}{\varkappa(\alpha)}\mathscr{G}_{3}\left(t,\mathscr{I}^{*}(t)\right) +\frac{\alpha}{\varkappa(\alpha)\varGamma(\alpha)}\int_{0}^{t}\left(t-z\right)^{\alpha-1} \\ &\mathscr{G}_{3}\left(z,\mathscr{I}^{*}(z)\right)dz \\ \mathscr{A}(t) = &\frac{1-\alpha}{\varkappa(\alpha)}\mathscr{G}_{4}\left(t,\mathscr{A}^{*}(t)\right) +\frac{\alpha}{\varkappa(\alpha)\varGamma(\alpha)}\int_{0}^{t}\left(t-z\right)^{\alpha-1} \\ &\mathscr{G}_{4}\left(z,\mathscr{G}^{*}(z)\right)dz \\ \mathscr{Q}(t) = &\frac{1-\alpha}{\varkappa(\alpha)}\mathscr{G}_{5}\left(t,\mathscr{Q}^{*}(t)\right) +\frac{\alpha}{\varkappa(\alpha)\varGamma(\alpha)}\int_{0}^{t}\left(t-z\right)^{\alpha-1} \\ &\mathscr{G}_{5}\left(z,\mathscr{Q}^{*}(z)\right)dz \\ \mathscr{H}(t) = &\frac{1-\alpha}{\varkappa(\alpha)}\mathscr{G}_{6}\left(t,\mathscr{H}^{*}(t)\right) +\frac{\alpha}{\varkappa(\alpha)\varGamma(\alpha)}\int_{0}^{t}\left(t-z\right)^{\alpha-1} \\ &\mathscr{G}_{6}\left(z,\mathscr{H}^{*}(z)\right)dz \\ \mathscr{R}(t) = &\frac{1-\alpha}{\varkappa(\alpha)}\mathscr{G}_{7}\left(t,\mathscr{R}^{*}(t)\right) +\frac{\alpha}{\varkappa(\alpha)\varGamma(\alpha)}\int_{0}^{t}\left(t-z\right)^{\alpha-1} \\ &\mathscr{G}_{7}\left(z,\mathscr{R}^{*}(z)\right)dz. \end{align*} |
Then,
\begin{eqnarray*} \begin{alignedat}{1}\left\Vert \mathscr{S}-\mathscr{S}^{*}\right\Vert = &\frac{1-\alpha}{\varkappa(\alpha)}\left\Vert \mathscr{G}_{1}(t,\mathscr{S}(t))-\mathscr{G}_{1}(t,\mathscr{S}^{*}(t))\right\Vert \\ & +\frac{\alpha}{\varkappa(\alpha)\varGamma(\alpha)}\int_{a}^{t}\left(t-z\right)^{\alpha-1}\Vert \mathscr{G}_{1}\left(z,\mathscr{S}(z)\right)\\ &-\mathscr{G}_{1}\left(z,\mathscr{S}^{*}(z)\right)\Vert dz\\ \leq&\left[\frac{1-\alpha}{\varkappa(\alpha)}+\frac{\alpha}{\varkappa(\alpha)\varGamma(\alpha)}\right]\varTheta_{1}\left\Vert \mathscr{S}-\mathscr{S}^{*}\right\Vert . \end{alignedat} \end{eqnarray*} |
Which implies that
\begin{equation} \left[\frac{1-\alpha}{\varkappa(\alpha)}\varTheta_{1}+\frac{\varTheta_{1}}{\varkappa(\alpha)\varGamma(\alpha)}-1\right]\left\Vert \mathscr{S}-\mathscr{S}^{*}\right\Vert \geq0. \end{equation} | (4.2) |
By (4.1), (4.2) is vaild for \left\Vert \mathscr{S}-\mathscr{S}^{*}\right\Vert = 0 , which means that \mathscr{S} = \mathscr{S}^{*} . Similarly, we repeat the same fashion for the remaining compartment of the model (1.1), we have
\begin{eqnarray*} \begin{alignedat}{1}\left\Vert \mathscr{E}-\mathscr{E}^{*}\right\Vert = &\frac{1-\alpha}{\varkappa(\alpha)}\left\Vert \mathscr{G}_{1}(t,\mathscr{E}(t))-\mathscr{G}_{1}(t,\mathscr{E}^{*}(t))\right\Vert \\ & +\frac{\alpha}{\varkappa(\alpha)\varGamma(\alpha)}\int_{a}^{t}\left(t-z\right)^{\alpha-1}\Vert \mathscr{G}_{2}\left(z,\mathscr{E}(z)\right) \\ &-\mathscr{G}_{2}\left(z,\mathscr{E}^{*}(z)\right)\Vert dz\\ \leq&\left[\frac{1-\alpha}{\varkappa(\alpha)}+\frac{\alpha}{\varkappa(\alpha)\varGamma(\alpha)}\right]\varTheta_{2}\left\Vert \mathscr{E}-\mathscr{E}^{*}\right\Vert . \end{alignedat} \end{eqnarray*} |
Which implies that
\begin{equation} \left[\frac{1-\alpha}{\varkappa(\alpha)}\varTheta_{2}+\frac{\varTheta_{2}}{\varkappa(\alpha)\varGamma(\alpha)}-1\right]\left\Vert \mathscr{E}-\mathscr{E}^{*}\right\Vert \geq0. \end{equation} | (4.3) |
By using (4.1) which implies the (4.3) is valid for \left\Vert \mathscr{E}-\mathscr{E}^{*}\right\Vert = 0 , which means that \mathscr{E} = \mathscr{E}^{*} . Similarly, for \mathscr{I} , we have
\begin{eqnarray*} \begin{alignedat}{1}\left\Vert \mathscr{I}-\mathscr{I}^{*}\right\Vert = &\frac{1-\alpha}{\varkappa(\alpha)}\left\Vert \mathscr{G}_{3}(t,\mathscr{I}(t))-\mathscr{G}_{3}(t,\mathscr{I}^{*}(t))\right\Vert \\ & +\frac{\alpha}{\varkappa(\alpha)\varGamma(\alpha)}\int_{a}^{t}\left(t-z\right)^{\alpha-1}\Vert \mathscr{G}_{3}\left(z,\mathscr{I}(z)\right) \\ &-\mathscr{G}_{3}\left(z,\mathscr{I}^{*}(z)\right)\Vert dz\\ \leq&\left[\frac{1-\alpha}{\varkappa(\alpha)}+\frac{\alpha}{\varkappa(\alpha)\varGamma(\alpha)}\right]\varTheta_{3}\left\Vert \mathscr{I}-\mathscr{I}^{*}\right\Vert . \end{alignedat} \end{eqnarray*} |
Which implies that
\begin{equation} \left[\frac{1-\alpha}{\varkappa(\alpha)}\varTheta_{3}+\frac{\varTheta_{3}}{\varkappa(\alpha)\varGamma(\alpha)}-1\right]\left\Vert \mathscr{I}-\mathscr{I}^{*}\right\Vert \geq0. \end{equation} | (4.4) |
From (4.1), (4.4) result is valid for \left\Vert \mathscr{I}-\mathscr{I}^{*}\right\Vert = 0 , which means that \mathscr{I} = \mathscr{I}^{*} . Now, for \mathscr{A} , we have
\begin{eqnarray*} \begin{alignedat}{1}\left\Vert \mathscr{A}-\mathscr{A}^{*}\right\Vert = &\frac{1-\alpha}{\varkappa(\alpha)}\left\Vert \mathscr{G}_{4}(t,\mathscr{A}(t))-\mathscr{G}_{4}(t,\mathscr{A}^{*}(t))\right\Vert \\ & +\frac{\alpha}{\varkappa(\alpha)\varGamma(\alpha)}\int_{a}^{t}\left(t-z\right)^{\alpha-1}\Vert \mathscr{G}_{4}\left(z,\mathscr{A}(z)\right)\\ &-\mathscr{G}_{4}\left(z,\mathscr{A}^{*}(z)\right)\Vert dz\\ \leq&\left[\frac{1-\alpha}{\varkappa(\alpha)}+\frac{\alpha}{\varkappa(\alpha)\varGamma(\alpha)}\right]\varTheta_{4}\left\Vert \mathscr{A}-\mathscr{A}\mathscr{A}^{*}\right\Vert . \end{alignedat} \end{eqnarray*} |
Which implies that
\begin{equation} \left[\frac{1-\alpha}{\varkappa(\alpha)}\varTheta_{4}+\frac{\varTheta_{4}}{\varkappa(\alpha)\varGamma(\alpha)}-1\right]\left\Vert \mathscr{A}-\mathscr{A}^{*}\right\Vert \geq0. \end{equation} | (4.5) |
By using (4.1), the (4.5) is valid for \left\Vert \mathscr{A}-\mathscr{A}^{*}\right\Vert = 0 , which means that \mathscr{A} = \mathscr{A}^{*} . Similarly, for \mathscr{Q} ,
\begin{eqnarray*} \begin{alignedat}{1}\left\Vert \mathscr{Q}-\mathscr{Q}^{*}\right\Vert = &\frac{1-\alpha}{\varkappa(\alpha)}\left\Vert \mathscr{G}_{5}(t,\mathscr{Q}(t))-\mathscr{G}_{5}(t,\mathscr{Q}^{*}(t))\right\Vert \\ & +\frac{\alpha}{\varkappa(\alpha)\varGamma(\alpha)}\int_{a}^{t}\left(t-z\right)^{\alpha-1}\Vert \mathscr{G}_{5}\left(z,\mathscr{Q}(z)\right)\\ &-\mathscr{G}_{5}\left(z,\mathscr{Q}^{*}(z)\right)\Vert dz\\ \leq&\left[\frac{1-\alpha}{\varkappa(\alpha)}+\frac{\alpha}{\varkappa(\alpha)\varGamma(\alpha)}\right]\varTheta_{5}\left\Vert \mathscr{Q}-\mathscr{Q}^{*}\right\Vert . \end{alignedat} \end{eqnarray*} |
Which implies that
\begin{equation} \left[\frac{1-\alpha}{\varkappa(\alpha)}\varTheta_{5}+\frac{\varTheta_{5}}{\varkappa(\alpha)\varGamma(\alpha)}-1\right]\left\Vert \mathscr{Q}-\mathscr{Q}^{*}\right\Vert \geq0. \end{equation} | (4.6) |
By using (4.1) which implies the (4.6) is valid for \left\Vert \mathscr{Q}-\mathscr{Q}^{*}\right\Vert = 0 , which means that \mathscr{Q} = \mathscr{Q}^{*} . Similarly, for \mathscr{H} , we have
\begin{eqnarray*} \begin{alignedat}{1}\left\Vert \mathscr{H}-\mathscr{H}^{*}\right\Vert = &\frac{1-\alpha}{\varkappa(\alpha)}\left\Vert \mathscr{G}_{6}(t,\mathscr{H}(t))-\mathscr{G}_{6}(t,\mathscr{H}^{*}(t))\right\Vert \\ & +\frac{\alpha}{\varkappa(\alpha)\varGamma(\alpha)}\int_{a}^{t}\left(t-z\right)^{\alpha-1}\Vert \mathscr{G}_{6}\left(z,\mathscr{H}(z)\right) \\ &-\mathscr{G}_{6}\left(z,\mathscr{H}^{*}(z)\right)\Vert dz\\ \leq&\left[\frac{1-\alpha}{\varkappa(\alpha)}+\frac{\alpha}{\varkappa(\alpha)\varGamma(\alpha)}\right]\varTheta_{7}\left\Vert \mathscr{H}-\mathscr{H}^{*}\right\Vert . \end{alignedat} \end{eqnarray*} |
Which implies that
\begin{equation} \left[\frac{1-\alpha}{\varkappa(\alpha)}\varTheta_{6}+\frac{\varTheta_{6}}{\varkappa(\alpha)\varGamma(\alpha)}-1\right]\left\Vert \mathscr{H}-\mathscr{H}^{*}\right\Vert \geq0. \end{equation} | (4.7) |
By using (4.1), the (4.7) result is valid for \left\Vert \mathscr{H}-\mathscr{H}^{*}\right\Vert = 0 , which means that \mathscr{H} = \mathscr{H}^{*} . Similarly, for \mathscr{R} , we have
\begin{eqnarray*} \begin{alignedat}{1}\left\Vert R-R^{*}\right\Vert = &\frac{1-\alpha}{\varkappa(\alpha)}\left\Vert \mathscr{G}_{7}(t,\mathscr{R}(t))-\mathscr{G}_{7}(t,\mathscr{R}^{*}(t))\right\Vert \\ & +\frac{\alpha}{\varkappa(\alpha)\varGamma(\alpha)}\int_{a}^{t}\left(t-z\right)^{\alpha-1}\Vert \mathscr{G}_{7}\left(z,\mathscr{R}(z)\right) \\ &-\mathscr{G}_{7}\left(z,\mathscr{R}^{*}(z)\right)\Vert dz\\ \leq&\left[\frac{1-\alpha}{\varkappa(\alpha)}+\frac{\alpha}{\varkappa(\alpha)\varGamma(\alpha)}\right]\varTheta_{7}\left\Vert \mathscr{R}-\mathscr{R}^{*}\right\Vert . \end{alignedat} \end{eqnarray*} |
Which implies that
\begin{equation} \left[\frac{1-\alpha}{\varkappa(\alpha)}\varTheta_{7}+\frac{\varTheta_{7}}{\varkappa(\alpha)\varGamma(\alpha)}-1\right]\left\Vert \mathscr{R}-\mathscr{R}^{*}\right\Vert \geq0. \end{equation} | (4.8) |
By using (4.1) which implies that (4.8) results is valid for \left\Vert \mathscr{R}-\mathscr{R}^{*}\right\Vert = 0 , which shows that \mathscr{R} = \mathscr{R}^{*} . Thus the model (1.1) has a unique solution.
The integral system Eqs. (3.4)-(3.4) is Hyers -ulam Stable if for \eta_{i} > 0, \epsilon_{i} > 0 , for i\in N_{1}^{7} [47], with
\begin{align} &|\mathscr{S}(t)-\frac{1-\alpha}{\varkappa(\alpha)}\mathscr{G}_{1}\left(t,\mathscr{S}(t)\right)- \frac{\alpha}{\varkappa(\alpha)\varGamma(\alpha)}\int_{0}^{t}\left(t-z\right)^{\alpha-1} \\ &\mathscr{G}_{1}\left(z,\mathscr{S}(z)\right)dz|\leq\eta_{1} \end{align} | (5.1) |
\begin{align} &|\mathscr{E}(t)-\frac{1-\alpha}{\varkappa(\alpha)}\mathscr{G}_{2}\left(t,\mathscr{E}(t)\right) -\frac{\alpha}{\varkappa(\alpha)\varGamma(\alpha)}\int_{0}^{t}\left(t-z\right)^{\alpha-1} \\ &\mathscr{G}_{2}\left(z,\mathscr{E}(z)\right)dz|\leq\eta_{2} \end{align} | (5.2) |
\begin{align} &|\mathscr{I}(t)-\frac{1-\alpha}{\varkappa(\alpha)}\mathscr{G}_{3}\left(t,\mathscr{I}(t)\right)- \frac{\alpha}{\varkappa(\alpha)\varGamma(\alpha)}\int_{0}^{t}\left(t-z\right)^{\alpha-1} \\ &\mathscr{G}_{3}\left(z,\mathscr{I}(z)\right)dz|\leq\eta_{3} \end{align} | (5.3) |
\begin{align} &|\mathscr{A}(t)-\frac{1-\alpha}{\varkappa(\alpha)}\mathscr{G}_{4}\left(t,\mathscr{A}(t)\right)- \frac{\alpha}{\varkappa(\alpha)\varGamma(\alpha)}\int_{a}^{t}\left(t-z\right)^{\alpha-1} \\ &\mathscr{G}_{4}\left(z,\mathscr{A}(z)\right)dz|\leq\eta_{4} \end{align} | (5.4) |
\begin{align} &|\mathscr{Q}(t)-\frac{1-\alpha}{\varkappa(\alpha)}\mathscr{G}_{5}\left(t,\mathscr{Q}(t)\right)- \frac{\alpha}{\varkappa(\alpha)\varGamma(\alpha)}\int_{0}^{t}\left(t-z\right)^{\alpha-1} \\ &\mathscr{G}_{5}\left(z,\mathscr{Q}(z)\right)dz|\leq\eta_{5} \end{align} | (5.5) |
\begin{align} &|\mathscr{H}(t)-\frac{1-\alpha}{\varkappa(\alpha)}\mathscr{G}_{6}\left(t,\mathscr{H}(t)\right)- \frac{\alpha}{\varkappa(\alpha)\varGamma(\alpha)}\int_{0}^{t}\left(t-z\right)^{\alpha-1} \\ &\mathscr{G}_{6}\left(z,\mathscr{H}(z)\right)dz|\leq\eta_{6} \end{align} | (5.6) |
\begin{align} &|\mathscr{R}(t)-\frac{1-\alpha}{\varkappa(\alpha)}\mathscr{G}_{7}\left(t,\mathscr{R}(t)\right)- \frac{\alpha}{\varkappa(\alpha)\varGamma(\alpha)}\int_{0}^{t}\left(t-z\right)^{\alpha-1} \\ &\mathscr{G}_{7}\left(z,\mathscr{R}(z)\right)dz|\leq\eta_{7} \end{align} | (5.7) |
Now, we have \dot{\mathscr{S}}, \dot{\mathscr{E}}, \dot{\mathscr{I}}, \dot{\mathscr{A}}, \dot{\mathscr{Q}}, \dot{\mathscr{H}}, \dot{\mathscr{R}} , which implies that
\begin{align} \dot{\mathscr{S}}(t) = &\frac{1-\alpha}{\varkappa(\alpha)}\mathscr{G}_{1} \left(t,\dot{\mathscr{S}}(t)\right)+\frac{\alpha}{\varkappa(\alpha)\varGamma(\alpha)} \int_{0}^{t}\left(t-z\right)^{\alpha-1}\mathscr{G}_{1} \\ &(z,\dot{\mathscr{S}}(z))dz \end{align} | (5.8) |
\begin{align} \dot{\mathscr{E}}(t) = &\frac{1-\alpha}{\varkappa(\alpha)}\mathscr{G}_{2} \left(t,\dot{\mathscr{E}}(t)\right)+\frac{\alpha}{\varkappa(\alpha)\varGamma(\alpha)} \int_{0}^{t}\left(t-z\right)^{\alpha-1}\mathscr{G}_{2} \\ &\left(z,\dot{\mathscr{E}}(z)\right)dz \end{align} | (5.9) |
\begin{align} \dot{\mathscr{I}}(t) = &\frac{1-\alpha}{\varkappa(\alpha)}\mathscr{G}_{3} \left(t,\dot{\mathscr{I}}(t)\right)+\frac{\alpha}{\varkappa(\alpha)\varGamma(\alpha)} \int_{a}^{t}\left(t-z\right)^{\alpha-1}\mathscr{G}_{3} \\ &\left(z,\dot{\mathscr{I}}(z)\right)dz \end{align} | (5.10) |
\begin{align} \dot{\mathscr{A}}(t) = &\frac{1-\alpha}{\varkappa(\alpha)}\mathscr{G}_{4} \left(t,\dot{\mathscr{A}}(t)\right)+\frac{\alpha}{\varkappa(\alpha)\varGamma(\alpha)} \int_{a}^{t}\left(t-z\right)^{\alpha-1}\mathscr{G}_{4} \\ &\left(z,\dot{\mathscr{A}}(z)\right)dz \end{align} | (5.11) |
\begin{align} \dot{\mathscr{Q}}(t) = &\frac{1-\alpha}{\varkappa(\alpha)}\mathscr{G}_{5} \left(t,\dot{\mathscr{Q}}(t)\right)+\frac{\alpha}{\varkappa(\alpha)\varGamma(\alpha)} \int_{0}^{t}\left(t-z\right)^{\alpha-1}\mathscr{G}_{5} \\ &\left(z,\dot{\mathscr{Q}}(z)\right)dz \end{align} | (5.12) |
\begin{align} \dot{\mathscr{H}}(t) = &\frac{1-\alpha}{\varkappa(\alpha)}\mathscr{G}_{6} \left(t,\dot{\mathscr{H}}(t)\right)+\frac{\alpha}{\varkappa(\alpha)\varGamma(\alpha)} \int_{0}^{t}\left(t-z\right)^{\alpha-1}\mathscr{G}_{6} \\ &\left(z,\dot{\mathscr{H}}(t)\right)dz \end{align} | (5.13) |
\begin{align} \dot{\mathscr{R}}(t) = &\frac{1-\alpha}{\varkappa(\alpha)}\mathscr{G}_{7} \left(t,\dot{\mathscr{R}}(t)\right)+\frac{\alpha}{\varkappa(\alpha)\varGamma(\alpha)} \int_{0}^{t}\left(t-z\right)^{\alpha-1}\mathscr{G}_{7} \\ &\left(z,\dot{\mathscr{R}}(t)\right)dz. \end{align} | (5.14) |
Such that
\begin{eqnarray*} & & \left|\mathscr{S}-\dot{\mathscr{S}}\right|\leq\epsilon_{1}\eta_{1},\left|\mathscr{E}-\dot{\mathscr{E}} \right|\leq\epsilon_{2}\eta_{2},\left|\mathscr{I}-\dot{\mathscr{I}}\right|\leq\epsilon_{3}\eta_{3}, \\ & & \left|\mathscr{A}-\dot{\mathscr{A}}\right|\leq\epsilon_{4}\eta_{4}, \left|\mathscr{Q}-\dot{\mathscr{Q}}\right|\leq\epsilon_{5}\eta_{5}, \left|\mathscr{H}-\dot{\mathscr{H}}\right|\leq\epsilon_{6}\eta_{6}, \\ & & \left|\mathscr{R}-\dot{\mathscr{R}}\right|\leq\epsilon_{7}\eta_{7}. \end{eqnarray*} |
Theorem 5.1. We assume that (P), Satisfied then the FOM (1.1) is Hyers Ulam stable.
Proof. By using (4.1), the FOM (1.1) has a unique solution, say \left(\mathscr{S}, \mathscr{E}, \mathscr{I}, \mathscr{A}, \mathscr{Q}, \mathscr{H}, \mathscr{R}\right) . Let we suppose that \left(\dot{\mathscr{S}}, \dot{\mathscr{E}}, \dot{\mathscr{I}}, \dot{\mathscr{A}}, \dot{\mathscr{Q}}, \dot{\mathscr{H}}, \dot{\mathscr{R}}\right) be an another solution for the considered model. We go ahead with first equation of the (1.1), satisfying Eqs. (3.4). Then
\begin{eqnarray*} \begin{alignedat}{1}\left\Vert \mathscr{S}-\dot{\mathscr{S}}\right\Vert = &\frac{1-\alpha}{\varkappa(\alpha)}\left\Vert \mathscr{G}_{1}(t,\mathscr{S}(t))-\mathscr{G}_{1}(t,\dot{\mathscr{S}}(t))\right\Vert +\frac{\alpha}{\varkappa(\alpha)\varGamma(\alpha)} \\ & \int_{0}^{t}\left(t-z\right)^{\alpha-1}\left\Vert \mathscr{G}_{1}\left(z,\mathscr{S}(z)\right)-\mathscr{G}_{1}\left(z,\dot{\mathscr{S}}(z)\right)\right\Vert dz\\ \leq &\left[\frac{1-\alpha}{\varkappa(\alpha)}+\frac{\alpha}{\varkappa(\alpha)\varGamma(\alpha)}\right]\varTheta_{1}\left\Vert \mathscr{S}-\dot{\mathscr{S}}\right\Vert . \end{alignedat} \end{eqnarray*} |
Now, we taking \eta_{1} = \Theta_{1} and \frac{1-\alpha}{\varkappa(\alpha)}+\frac{\alpha}{\varkappa(\alpha)\varGamma(\alpha)} = \Delta_{1} , this implies \left\Vert \mathscr{S}-\dot{\mathscr{S}}\right\Vert \leq\eta_{1}\Delta_{1} .
Similarly, for \mathscr{E}(t), \dot{\mathscr{E}}(t), \mathscr{I}(t), \dot{\mathscr{I}}(t), \mathscr{A}(t), \dot{\mathscr{A}}(t), \mathscr{Q}(t), \dot{\mathscr{Q}}(t) , \mathscr{H}(t), \dot{\mathscr{H}}(t), \mathscr{R}(t), \dot{\mathscr{R}}(t) , we have
\begin{equation} \begin{aligned}\begin{cases} & \left\Vert \mathscr{E}-\dot{\mathscr{E}}\right\Vert \leq\eta_{2}\Delta_{2}\\ & \left\Vert \mathscr{I}-\dot{\mathscr{I}}\right\Vert \leq\eta_{3}\Delta_{3}\\ & \left\Vert \mathscr{A}-\dot{\mathscr{A}}\right\Vert \leq\eta_{4}\Delta_{4}\\ & \left\Vert \mathscr{Q}-\dot{\mathscr{Q}}\right\Vert \leq\eta_{5}\Delta_{5}\\ & \left\Vert \mathscr{H}-\dot{\mathscr{H}}\right\Vert \leq\eta_{6}\Delta_{6}\\ & \left\Vert \mathscr{R}-\dot{\mathscr{R}}\right\Vert \leq\eta_{7}\Delta_{7}. \end{cases}\end{aligned} \end{equation} | (5.15) |
Thus, the solution of the considered FOM is stable.
In this section, we find the numerical solution by using the numerical method of Lagrange's interpolation. For the solution of the system (1.1), we consider
\begin{equation} \begin{cases} _{0}^{ABC}\mathscr{D}_{t}^{\alpha}[\mathscr{S}(t)] = \mathscr{G}_{1}(t,\mathscr{S}),\\ _{0}^{ABC}\mathscr{D}_{t}^{\alpha}[\mathscr{E}(t)] = \mathscr{G}_{2}(t,\mathscr{E}),\\ _{0}^{ABC}\mathscr{D}_{t}^{\alpha}[\mathscr{I}(t)] = \mathscr{G}_{3}(t,\mathscr{I}),\\ _{0}^{ABC}\mathscr{D}_{t}^{\alpha}[\mathscr{A}(t)] = \mathscr{G}_{4}(t,\mathscr{A}),\\ _{0}^{ABC}\mathscr{D}_{t}^{\alpha}[\mathscr{Q}(t)] = \mathscr{G}_{5}(t,\mathscr{Q}),\\ _{0}^{ABC}\mathscr{D}_{t}^{\alpha}[\mathscr{H}(t)] = \mathscr{G}_{6}(t,\mathscr{H}),\\ _{0}^{ABC}\mathscr{D}_{t}^{\alpha}[\mathscr{R}(t)] = \mathscr{G}_{7}(t,\mathscr{R}). \end{cases} \end{equation} | (6.1) |
Using the Lemma (2.1) and (6.1), we get
\begin{align} \mathscr{S}(t)-\mathscr{S}(0) = &\frac{1-\alpha}{\varkappa(\alpha)}x_{1}\left(t,\mathscr{S}\right) +\frac{\alpha}{\varkappa(\alpha)\varGamma(\alpha)}\int_{0}^{t}\left(t-z\right)^{\alpha-1} \\ &\mathscr{G}_{1}\left(z,\mathscr{S}\right)dz \end{align} | (6.2) |
\begin{align} \mathscr{E}(t)-\mathscr{E}(0) = &\frac{1-\alpha}{\varkappa(\alpha)}\mathscr{G}_{2} \left(t,\mathscr{E}\right)+\frac{\alpha}{\varkappa(\alpha)\varGamma(\alpha)} \int_{0}^{t}\left(t-z\right)^{\alpha-1} \\ &\mathscr{G}_{2}\left(z,\mathscr{E}\right)dz \end{align} | (6.3) |
\begin{align} \mathscr{I}(t)-\mathscr{I}(0) = &\frac{1-\alpha}{\varkappa(\alpha)}\mathscr{G}_{3} \left(t,\mathscr{I}\right)+\frac{\alpha}{\varkappa(\alpha)\varGamma(\alpha)}\int_{0}^{t} \left(t-z\right)^{\alpha-1} \\ &\mathscr{G}_{3}\left(z,\mathscr{I}\right)dz \end{align} | (6.4) |
\begin{align} \mathscr{A}(t)-\mathscr{A}(0) = &\frac{1-\alpha}{\varkappa(\alpha)}\mathscr{G}_{4} \left(t,\mathscr{A}\right)+\frac{\alpha}{\varkappa(\alpha)\varGamma(\alpha)} \int_{0}^{t}\left(t-z\right)^{\alpha-1} \\ &\mathscr{G}_{4}\left(z,\mathscr{A}\right)dz \end{align} | (6.5) |
\begin{align} \mathscr{Q}(t)-\mathscr{Q}(0) = &\frac{1-\alpha}{\varkappa(\alpha)}\mathscr{G}_{5} \left(t,\mathscr{Q}\right)+\frac{\alpha}{\varkappa(\alpha)\varGamma(\alpha)}\int_{0}^{t} \left(t-z\right)^{\alpha-1} \\ &\mathscr{G}_{5}\left(z,\mathscr{Q}\right)dz \end{align} | (6.6) |
\begin{align} \mathscr{H}(t)-\mathscr{H}(0) = &\frac{1-\alpha}{\varkappa(\alpha)}\mathscr{G}_{6} \left(t,\mathscr{H}\right)+\frac{\alpha}{\varkappa(\alpha)\varGamma(\alpha)} \int_{0}^{t}\left(t-z\right)^{\alpha-1} \\ &\mathscr{G}_{6}\left(z,H\right)dz \end{align} | (6.7) |
\begin{align} \mathscr{R}(t)-\mathscr{R}(0) = &\frac{1-\alpha}{\varkappa(\alpha)}\mathscr{G}_{7} \left(t,\mathscr{R}\right)+\frac{\alpha}{\varkappa(\alpha)\varGamma(\alpha)} \int_{0}^{t}\left(t-z\right)^{\alpha-1} \\ &\mathscr{G}_{7}\left(z,\mathscr{R}\right)dz. \end{align} | (6.8) |
Let [0, T] be the interval which we want to find the solution of the system (1.1). For this we divided the given interval as a set of points t_{m+1} , for m = 0, 1, 2, ..n, we have
\begin{align} \mathscr{S}(t_{m+1}) = &\frac{1-\alpha}{\varkappa(\alpha)}\mathscr{G}_{1} \left(t_{m},\mathscr{S}\right)+\frac{\alpha}{\varkappa(\alpha)\varGamma(\alpha)} \sum\limits_{k = 0}^{n}\int_{t_{k}}^{t_{k+1}}\left(t_{m+1}-z\right)^{\alpha-1} \\ &\mathscr{G}_{1}\left(z,\mathscr{S}\right)dz \end{align} | (6.9) |
\begin{align} \mathscr{E}(t_{m+1}) = &\frac{1-\alpha}{\varkappa(\alpha)}\mathscr{G}_{2} \left(t_{m},\mathscr{E}\right)+\frac{\alpha}{\varkappa(\alpha)\varGamma(\alpha)} \sum\limits_{k = 0}^{n}\int_{t_{k}}^{t_{k+1}}\left(t_{m+1}-z\right)^{\alpha-1} \\ &\mathscr{G}_{2}\left(z,\mathscr{E}\right)dz \end{align} | (6.10) |
\begin{align} \mathscr{I}(t_{m+1}) = &\frac{1-\alpha}{\varkappa(\alpha)}\mathscr{G}_{3} \left(t_{m},\mathscr{I}\right)+\frac{\alpha}{\varkappa(\alpha)\varGamma(\alpha)} \sum\limits_{k = 0}^{n}\int_{t_{k}}^{t_{k+1}}\left(t_{m}-z\right)^{\alpha-1} \\ &\mathscr{G}_{3}\left(z,\mathscr{I}\right)dz \end{align} | (6.11) |
\begin{align} \mathscr{A}(t_{m+1}) = &\frac{1-\alpha}{\varkappa(\alpha)}\mathscr{G}_{4} \left(t_{m},\mathscr{A}\right)+\frac{\alpha}{\varkappa(\alpha)\varGamma(\alpha)} \sum\limits_{k = 0}^{n}\int_{t_{k}}^{t_{k+1}}\left(t_{m}-z\right)^{\alpha-1} \\ &\mathscr{G}_{4}\left(z,\mathscr{A}\right)dz \end{align} | (6.12) |
\begin{align} \mathscr{Q}(t_{m+1}) = &\frac{1-\alpha}{\varkappa(\alpha)}\mathscr{G}_{5} \left(t_{m},\mathscr{Q}\right)+\frac{\alpha}{\varkappa(\alpha)\varGamma(\alpha)} \sum\limits_{k = 0}^{n}\int_{t_{k}}^{t_{k+1}}\left(t_{m}-z\right)^{\alpha-1} \\ &\mathscr{G}_{5}\left(z,\mathscr{Q}\right)dz \end{align} | (6.13) |
\begin{align} \mathscr{H}(t_{m+1}) = &\frac{1-\alpha}{\varkappa(\alpha)}\mathscr{G}_{6} \left(t_{m},\mathscr{H}(t)\right)+\frac{\alpha}{\varkappa(\alpha)\varGamma(\alpha)} \sum\limits_{k = 0}^{n}\int_{t_{k}}^{t_{k+1}}\left(t_{m}-z\right)^{\alpha-1} \\ &\mathscr{G}_{6}\left(z,\mathscr{H}\right)dz \end{align} | (6.14) |
\begin{align} \mathscr{R}(t_{m+1}) = &\frac{1-\alpha}{\varkappa(\alpha)}\mathscr{G}_{7} \left(t_{m},\mathscr{R}\right)+\frac{\alpha}{\varkappa(\alpha)\varGamma(\alpha)} \sum\limits_{k = 0}^{n}\int_{t_{k}}^{t_{k+1}}\left(t_{m}-z\right)^{\alpha-1} \\ &\mathscr{G}_{7}\left(z,\mathscr{R}\right)dz. \end{align} | (6.15) |
Using lagrange's interpolation, we get
\begin{align} \mathscr{S}(t_{m+1}) = \mathscr{S}_{0}+\frac{1-\alpha}{\varkappa(\alpha)}\mathscr{G}_{1}\left(t_{m},\mathscr{S}\right) +\frac{\alpha}{\varkappa(\alpha)}\sum\limits_{\zeta = 1}^{m}[\frac{h^{\alpha} \mathscr{G}_{1}(t_{\zeta},\mathscr{S})}{\varGamma(\alpha+2)} \\ ((1+m-\zeta)^{\alpha}(2+m-\zeta+\alpha)-(m-\zeta)^{\alpha}(m-\zeta\\+2+2\alpha)) -\frac{h^{\alpha}\mathscr{G}_{1}(t_{\zeta-1},\mathscr{S})}{\varGamma(\alpha+2)} ((1+m-\zeta)^{\alpha} \\-(m-\zeta)^{\alpha}(1+m-\zeta+\alpha))].\end{align} |
\begin{align} \mathscr{E}(t_{m+1}) = \mathscr{E}_{0}+\frac{1-\alpha}{\varkappa(\alpha)}\mathscr{G}_{2}\left(t_{m},\mathscr{E}\right) +\frac{\alpha}{\varkappa(\alpha)}\sum\limits_{\zeta = 0}^{m}[\frac{h^{\alpha} \mathscr{G}_{2}(t_{\zeta},\mathscr{E})}{\varGamma(\alpha+2)}\\((1+m-\zeta)^{\alpha}(2+m-\zeta+\alpha) -(m-\zeta)^{\alpha}(m-\zeta\\+2+2\alpha))-\frac{h^{\alpha} \mathscr{G}_{1}(t_{\zeta-1},\mathscr{E})}{\varGamma(\alpha+2)} ((m+1-\zeta)^{\alpha}\\-(m-\zeta)^{\alpha}(m+1-\zeta+\alpha))]. \end{align} |
\begin{align} \mathscr{I}(t_{m+1}) = \mathscr{I}_{0}+\frac{1-\alpha}{\varkappa(\alpha)}\mathscr{G}_{3}\left(t_{m},\mathscr{I}\right) +\frac{\alpha}{\varkappa(\alpha)}\sum\limits_{\zeta = 0}^{m}[\frac{h^{\alpha} \mathscr{G}_{3}(t_{\zeta},\mathscr{I})}{\varGamma(\alpha+2)}\\((1+m-\zeta)^{\alpha}(2+m-\zeta+\alpha) -(m-\zeta)^{\alpha}(m-\zeta\\+2+2\alpha))-\frac{h^{\alpha} \mathscr{G}_{3}(t_{\zeta-1},\mathscr{I})}{\varGamma(\alpha+2)} ((m+1-\zeta)^{\alpha}\\-(m-\zeta)^{\alpha}(m+1-\zeta+\alpha))]. \end{align} |
\begin{align} \mathscr{A}(t_{m+1}) = \mathscr{A}_{0}+\frac{1-\alpha}{\varkappa(\alpha)}\mathscr{G}_{4}\left(t_{m},\mathscr{A}\right) +\frac{\alpha}{\varkappa(\alpha)}\sum\limits_{\zeta = 0}^{m}[\frac{h^{\alpha} \mathscr{G}_{4}(t_{\zeta},\mathscr{A})}{\varGamma(\alpha+2)}\\((1+m-\zeta)^{\alpha}(2+m-\zeta+\alpha) .-(m-\zeta)^{\alpha}(m-\zeta\\+2+2\alpha))-\frac{h^{\alpha} \mathscr{G}_{4}(t_{\zeta-1},\mathscr{A})}{\varGamma(\alpha+2)} ((m+1-\zeta)^{\alpha} \\-(m-\zeta)^{\alpha}(m+1-\zeta+\alpha))].\end{align} |
\begin{align} \mathscr{Q}(t_{m+1}) = \mathscr{Q}_{0}+\frac{1-\alpha}{\varkappa(\alpha)}\mathscr{G}_{5} \left(t_{m},\mathscr{Q}\right)+\frac{\alpha}{\varkappa(\alpha)}\sum\limits_{\zeta = 0}^{n} [\frac{h^{\alpha}\mathscr{G}_{5}(t_{\zeta},\mathscr{Q})}{\varGamma(\alpha+2)} \\((1+m-\zeta)^{\alpha}(2+m-\zeta+\alpha) -(m-\zeta)^{\alpha}(m-\zeta\\+2+2\alpha))-\frac{h^{\alpha} \mathscr{G}_{5}(t_{\zeta-1},\mathscr{Q})}{\varGamma(\alpha+2)} ((m+1-\zeta)^{\alpha}\\-(m-\zeta)^{\alpha}(m+1-\zeta+\alpha))]. \end{align} |
\begin{align} \mathscr{H}(t_{m+1}) = \mathscr{H}_{0}+\frac{1-\alpha}{\varkappa(\alpha)}\mathscr{G}_{6}\left(t_{m},\mathscr{H}\right) +\frac{\alpha}{\varkappa(\alpha)}\sum\limits_{\zeta = 0}^{n}[\frac{h^{\alpha} \mathscr{G}_{6}(t_{\zeta},\mathscr{H})}{\varGamma(\alpha+2)}\\((1+m-\zeta)^{\alpha}(2+m-\zeta+\alpha)-(m-\zeta)^{\alpha}(m-\zeta\\+2+2\alpha))-\frac{h^{\alpha} \mathscr{G}_{6}(t_{\zeta-1},\mathscr{H})}{\varGamma(\alpha+2)}((m+1-\zeta)^{\alpha}\\-(m-\zeta)^{\alpha}(m+1-\zeta+\alpha))]. \end{align} |
\begin{align} \mathscr{R}(t_{m+1}) = \mathscr{R}_{0}+\frac{1-\alpha}{\varkappa(\alpha)}\mathscr{G}_{7}\left(t_{m},\mathscr{R}\right) +\frac{\alpha}{\varkappa(\alpha)}\sum\limits_{\zeta = 0}^{n}[\frac{h^{\alpha} \mathscr{G}_{7}(t_{\zeta},\mathscr{R})}{\varGamma(\alpha+2)} \\ ((1+m-\zeta)^{\alpha}(2+m-\zeta+\alpha)-(m-\zeta)^{\alpha}(m-\zeta \\ +2+2\alpha))- \frac{h^{\alpha}\mathscr{G}_{7}(t_{\zeta-1},\mathscr{R})}{\varGamma(\alpha+2)} ((1+m-\zeta)^{\alpha} \\ -(m-\zeta)^{\alpha}(m+1-\zeta+\alpha))]. \end{align} |
This numerical scheme will be used in the next section.
In this part of the paper, we apply the numerical method described in the previous section to demonstrate the graphical results of the model (1.1).
The parameters used in simulations are given in Table.2, most of which are taken from previous published work [35,36,37,38]. Plotting in the Figure 2, the behavior of the model with two activate parameters, isolation rate ( c\neq0 ) and social distancing rate ( \delta\neq 0 ), is shown by the bold blue line. In contrast, the bold red line shows the behavior of the model with parameters c and \delta equal to zero.
Parameters | Parameters Values |
Individuals in the exposed, asymptomatic, infected, and hospitalized classes declined considerably with isolation and social distance parameters, but the suspectable and recovered population increased fast. These graphs demonstrate that these factors are useful in reducing Covid-19 infection in the population.
From Figures 3-9, we see that the susceptible, infection, asymptotic, quarantined, and hospitalized population will decrease, and consequently, the recovery will increase. We observed a rapid decrease in the population of exposed, asymptomatic, quarantined, hospitalized, and infected classes, which becomes more significant for fractional value compared to integer order \alpha = 1 .
From Figure 10, we noted that the susceptible, exposed, and asymptomatic individuals are the smallest due to the smallest value of \Lambda . Otherwise, the largest value of the \Lambda could be high population density, close contact with the people, improper social distancing, and insufficient preventive measures. This will lead to a relatively high spread of the disease.
This work studied the transmission dynamics of the COVID-19 pandemic with asymptomatic, quarantine, and hospitalization individuals through an ABC fractional model. Initially, we formulated a mathematical model and analyzed the model using the fractional operator with the power-law kernel. We have used fixed point theory to conclude the existence of such a model in the real world. Then we have shown the unique solution of the model. Further, we investigated significant conditions for the Ulam Hyers type stability via non-linear functional analysis. The computational scheme is derived for the numerical simulation and is checked for available data. Further, We simulate the COVID-19 model for different transmission rate values to evaluate the dynamics of the separate compartments in the model. The dynamics of the COVID-19 pandemic and the impact of various control strategies by including additional classes into the current model will be addressed in future research. The vaccinated population and the number of pathogens in the environment are two compartments. The suggested model will include memory characteristics and non-locality and be an expanded version of the current model. This study's findings will help health care centers forecast how the coronavirus may affect the world in the future.
This research received no specific grant from any funding agency in the public, commercial, or not-for-profit sectors.
We declare that we have no competing interests.
[1] | J. Li, Y. Wang, S. Gilmour, M. Wang, D. Yoneoka, Y. Wang, et al., Estimation of the epidemic properties of the 2019 novel coronavirus: A mathematical modeling study, J. Am. Math. Soc., (2020). |
[2] |
Q. Lin, S. Zhao, D. Gao, Y. Lou, S. Yang, S. S. Musa, et al., A conceptual model for the coronavirus disease 2019 (COVID-19) outbreak in Wuhan, China with individual reaction and governmental action, Int. J. Infect. Dis., 93 (2020), 211–216. https://doi.org/10.1016/j.ijid.2020.02.058 doi: 10.1016/j.ijid.2020.02.058
![]() |
[3] | World Health Organization and others, Coronavirus disease 2019 (COVID-19): situation report, 51 World Health Organization, (2020). |
[4] | J. Li, Y. Wang, S. Gilmour, M. Wang, D. Yoneoka, Y. Wang, et al., Interim guidance for homeless service providers to plan and respond to coronavirus disease 2019 (COVID-19), J. Am. Math. Soc., (2020), 1–10. |
[5] |
A. J. Kucharski, T. W. Russell, C. Diamond, Y. Liu, J. Edmunds, S. Funk, et al., Early dynamics of transmission and control of COVID-19: a mathematical modelling study, The lancet infectious diseases, 20 (2020), 553–558. https://doi.org/10.1016/S1473-3099(20)30144-4 doi: 10.1016/S1473-3099(20)30144-4
![]() |
[6] | Y. Liu, A. A. Gayle, A. Wilder-Smith, J. Rocklov, The reproductive number of COVID-19 is higher compared to SARS coronavirus, J. Travel Med., (2020). |
[7] | S. Zhao, H. Chen, Modeling the epidemic dynamics and control of COVID-19 outbreak in China, Quant. Biol., 1 (2020), 1–9. |
[8] | H. Song, F. Liu, F. Li, X. Cao, H. Wang, Z. Jia, et al., The impact of isolation on the transmission of COVID-19 and estimation of potential second epidemic in China, Preprints, (2020). |
[9] |
S. A. Sarkodie, P. A. Owusu, Global assessment of environment, health and economic impact of the novel coronavirus (COVID-19), Environment, Development and Sustainability, 23 (2021), 5005–5015. https://doi.org/10.1007/s10668-020-00801-2 doi: 10.1007/s10668-020-00801-2
![]() |
[10] |
I. U. Haq, N. Ali, S. Ahmad, T. Akram, A Hybrid Interpolation Method for Fractional PDEs and Its Applications to Fractional Diffusion and Buckmaster Equations, Math. Probl. Eng., 2022 (2022). https://doi.org/10.1155/2022/2517602 doi: 10.1155/2022/2517602
![]() |
[11] |
Y. Chen, D. Guo, Molecular mechanisms of coronavirus RNA capping and methylation, Virol. Sin., 31 (2016), 3–11. https://doi.org/10.1007/s12250-016-3726-4 doi: 10.1007/s12250-016-3726-4
![]() |
[12] | I. U. Haq, N. Ali, K. S. Nisar, An optimal control strategy and Grünwald-Letnikov finite-difference numerical scheme for the fractional-order COVID-19 model, Mathematical Modelling and Numerical Simulation with Applications, 2 (2022), 108–116. |
[13] |
H. Lu, C. W. Stratton, Y.-W. Tang, Outbreak of pneumonia of unknown etiology in Wuhan, China: the mystery and the miracle, J. Med. Virol., 92 (2020), 401–402. https://doi.org/10.1002/jmv.25678 doi: 10.1002/jmv.25678
![]() |
[14] |
E. Rinott, I. Youngster, Y. E. Lewis, Reduction in COVID-19 patients requiring mechanical ventilation following implementation of a national COVID-19 vaccination program—Israel, December 2020–February 2021, Morbidity and Mortality Weekly Report, 70 (2021), 326. https://doi.org/10.15585/mmwr.mm7009e3 doi: 10.15585/mmwr.mm7009e3
![]() |
[15] |
P. Ingrid Johnston, Australia's public health response to COVID-19: what have we done, and where to from here? Aust. NZ J. Publ. Heal., 44 (2020), 440. https://doi.org/10.1111/1753-6405.13051 doi: 10.1111/1753-6405.13051
![]() |
[16] | S. Zhao, H. Chen, Modeling the epidemic dynamics and control of COVID-19 outbreak in China, Quant. Biol., 2 (2020), 1–9. |
[17] | H. Song, F. Liu, F. Li, X. Cao, H. Wang, Z. Jia, et al., The impact of isolation on the transmission of COVID-19 and estimation of potential second epidemic in China, Preprints, (2020). |
[18] |
J. Li, Y. Wang, S. Gilmour, M. Wang, D. Yoneoka, Y. Wang, et al., A new study on two different vaccinated fractional-order COVID-19 models via numerical algorithms, J. King Saud Univ. Sci., 34 (2022), 101914. https://doi.org/10.1016/j.jksus.2022.101914 doi: 10.1016/j.jksus.2022.101914
![]() |
[19] |
J. Li, Y. Wang, S. Gilmour, M. Wang, D. Yoneoka, Y. Wang, et al., Projections and fractional dynamics of COVID-19 with optimal control strategies, Chaos, Solitons & Fractals, 145 (2022), 110689. https://doi.org/10.1016/j.chaos.2021.110689 doi: 10.1016/j.chaos.2021.110689
![]() |
[20] |
K. N. Nabi, H. Abboubakar, P. Kumar, Forecasting of COVID-19 pandemic: From integer derivatives to fractional derivatives, Chaos, Solitons & Fractals, 141 (2020), 110283. https://doi.org/10.1016/j.chaos.2020.110283 doi: 10.1016/j.chaos.2020.110283
![]() |
[21] |
N. P. Jewell, J. A. Lewnard, B. L. Jewell, Predictive mathematical models of the COVID-19 pandemic: underlying principles and value of projections, Jama, 323 (2020), 1893–1894. https://doi.org/10.1001/jama.2020.6585 doi: 10.1001/jama.2020.6585
![]() |
[22] |
M. S. Khan, M. Ozair, T. Hussain, J. Gomez-Aguilar, Bifurcation analysis of a discrete-time compartmental model for hypertensive or diabetic patients exposed to COVID-19, The European Physical Journal Plus, 136 (2021), 1–26. https://doi.org/10.1140/epjp/s13360-021-01862-6 doi: 10.1140/epjp/s13360-021-01862-6
![]() |
[23] |
R. P. Yadav, R. Verma, A numerical simulation of fractional order mathematical modeling of COVID-19 disease in case of Wuhan China, Chaos, Solitons & Fractals, 140 (2020), 110124. https://doi.org/10.1016/j.chaos.2020.110124 doi: 10.1016/j.chaos.2020.110124
![]() |
[24] |
P. Pandey, Y.-M. Chu, J. Gomez-Aguilar, H. Jahanshahi, A. A. Aly, A novel fractional mathematical model of COVID-19 epidemic considering quarantine and latent time, Results Phys., 26 (2021), 104286. https://doi.org/10.1016/j.rinp.2021.104286 doi: 10.1016/j.rinp.2021.104286
![]() |
[25] |
A. Khan, H. M. Alshehri, T. Abdeljawad, Q. M. Al-Mdallal, H. Khan, Stability analysis of fractional nabla difference COVID-19 model, Results Phys., 122 (2021), 103888. https://doi.org/10.1016/j.rinp.2021.103888 doi: 10.1016/j.rinp.2021.103888
![]() |
[26] |
M. S. Abdo, K. Shah, H. A. Wahash, S. K. Panchal, On a comprehensive model of the novel coronavirus (COVID-19) under Mittag-Leffler derivative, Chaos, Solitons and Fractals, 135 (2020), 109867. https://doi.org/10.1016/j.chaos.2020.109867 doi: 10.1016/j.chaos.2020.109867
![]() |
[27] |
P. Pandey, J. Gomez-Aguilar, M. K. Kaabar, Z. Siri, A. M. Abd Allah, Mathematical modeling of COVID-19 pandemic in India using Caputo-Fabrizio fractional derivative, Comput. Biol. Med., 145 (2022), 105518. https://doi.org/10.1016/j.compbiomed.2022.105518 doi: 10.1016/j.compbiomed.2022.105518
![]() |
[28] |
M. Al-Refai, T. Abdeljawad, Analysis of the fractional diffusion equations with fractional derivative of non-singular kernel, Advances in Difference Equations, 2017 (2017), 1–12. https://doi.org/10.1186/s13662-017-1356-2 doi: 10.1186/s13662-017-1356-2
![]() |
[29] |
S. Hasan, A. El-Ajou, S. Hadid, M. Al-Smadi, S. Momani, Atangana-Baleanu fractional framework of reproducing kernel technique in solving fractional population dynamics system, Chaos, Solitons & Fractals, 133 (2020), 109624. https://doi.org/10.1016/j.chaos.2020.109624 doi: 10.1016/j.chaos.2020.109624
![]() |
[30] |
S. A. Khan, K. Shah, G. Zaman, F. Jarad, Existence theory and numerical solutions to smoking model under Caputo-Fabrizio fractional derivative, Chaos: An Interdisciplinary Journal of Nonlinear Science, 29 (2019), 013128. https://doi.org/10.1063/1.5079644 doi: 10.1063/1.5079644
![]() |
[31] |
M. L. Morgado, N. J. Ford, P. M. Lima, Analysis and numerical methods for fractional differential equations with delay, J. Comput. Appl. Math., 252 (2020), 159–168. https://doi.org/10.1016/j.cam.2012.06.034 doi: 10.1016/j.cam.2012.06.034
![]() |
[32] |
R. Garrappa, Trapezoidal methods for fractional differential equations: Theoretical and computational aspects, Math. Comput. Simulat., 110 (22015), 96–112. https://doi.org/10.1016/j.matcom.2013.09.012 doi: 10.1016/j.matcom.2013.09.012
![]() |
[33] |
K. Shah, Z. A. Khan, A. Ali, R. Amin, H. Khan, A. Khan, Haar wavelet collocation approach for the solution of fractional order COVID-19 model using Caputo derivative, Alex. Eng. J., 59 (2020), 3221–3231. https://doi.org/10.1016/j.aej.2020.08.028 doi: 10.1016/j.aej.2020.08.028
![]() |
[34] |
A. Atangana, J. Gomez-Aguilar, Numerical approximation of Riemann-Liouville definition of fractional derivative: from Riemann-Liouville to Atangana-Baleanu, Numerical Methods for Partial Differential Equations, 34 (2018), 1502–1523. https://doi.org/10.1002/num.22195 doi: 10.1002/num.22195
![]() |
[35] |
R. Resmawan, L. Yahya, Sensitivity analysis of mathematical model of coronavirus disease (COVID-19) transmission, Cauchy, 6 (2020), 91–99. https://doi.org/10.18860/ca.v6i2.9165 doi: 10.18860/ca.v6i2.9165
![]() |
[36] |
A. S. Shaikh, I. N. Shaikh, K. S. Nisar, A mathematical model of COVID-19 using fractional derivative: outbreak in India with dynamics of transmission and control, Advances in Difference Equations, 2020 (2020), 1–19. https://doi.org/10.1186/s13662-020-02834-3 doi: 10.1186/s13662-020-02834-3
![]() |
[37] |
B. Tang, X. Wang, Q. Li, N. L. Bragazzi, S. Tang, Y. Xiao, et al., Estimation of the transmission risk of the 2019-nCoV and its implication for public health interventions, J. Clin. Med., 9 (2020), 462. https://doi.org/10.3390/jcm9020462 doi: 10.3390/jcm9020462
![]() |
[38] |
C. N. Ngonghala, E. Iboi, S. Eikenberry, M. Scotch, C. R. MacIntyre, M. H. Bonds, et al., Mathematical assessment of the impact of non-pharmaceutical interventions on curtailing the 2019 novel Coronavirus, Math. Biosci., 135 (2020), 108364. https://doi.org/10.1016/j.mbs.2020.108364 doi: 10.1016/j.mbs.2020.108364
![]() |
[39] |
I. ul Haq, Analytical Approximate Solution Of Non-Linear Problem By Homotopy Perturbation Method (Hpm), Matrix Science Mathematic, 3 (2029), 20–24. https://doi.org/10.26480/msmk.01.2019.20.24 doi: 10.26480/msmk.01.2019.20.24
![]() |
[40] |
J. Solıs-Perez, J. Gomez-Aguilar, A. Atangana, X. You, J. Gu, C. Hao, et al., Novel numerical method for solving variable-order fractional differential equations with power, exponential and Mittag-Leffler laws, Chaos, Solitons & Fractals, 114 (2018), 175–185. https://doi.org/10.1016/j.chaos.2018.06.032 doi: 10.1016/j.chaos.2018.06.032
![]() |
[41] |
J. Solıs-Perez, J. Gomez-Aguilar, A. Atangana, Novel numerical method for solving variable-order fractional differential equations with power, exponential and Mittag-Leffler laws, Chaos, Solitons & Fractals, 114 (2018), 175–185. https://doi.org/10.1016/j.chaos.2018.06.032 doi: 10.1016/j.chaos.2018.06.032
![]() |
[42] |
C. Li, A. Chen, Numerical methods for fractional partial differential equations, International Journal of Computer Mathematics, 95 (2018), 1048–1099. https://doi.org/10.1080/00207160.2017.1343941 doi: 10.1080/00207160.2017.1343941
![]() |
[43] | J. Wang, K. Shah, A. Ali, Existence and Hyers-Ulam stability of fractional nonlinear impulsive switched coupled evolution equations, Math. Method. Appl. Sci., 41 (2018), 2392–2402. |
[44] |
M. Ahmad, A. Zada, J. Alzabut, Hyers–Ulam stability of a coupled system of fractional differential equations of Hilfer–Hadamard type, Demonstratio Mathematica, 52 (2019), 283–295. https://doi.org/10.1515/dema-2019-0024 doi: 10.1515/dema-2019-0024
![]() |
[45] |
A. Khan, H. Khan, J. Gomez-Aguilar, T. Abdeljawad, Existence and Hyers-Ulam stability for a nonlinear singular fractional differential equations with Mittag-Leffler kernel, Chaos, Solitons & Fractals, 127 (2019), 422–427. https://doi.org/10.1016/j.chaos.2019.07.026 doi: 10.1016/j.chaos.2019.07.026
![]() |
[46] |
V. S. Panwar, P. S. Uduman, J. G omez-Aguilar, Mathematical modeling of coronavirus disease COVID-19 dynamics using CF and ABC non-singular fractional derivatives, Chaos, Solitons & Fractals, 145 (2021), 110757. https://doi.org/10.1016/j.chaos.2021.110757 doi: 10.1016/j.chaos.2021.110757
![]() |
[47] |
M. Ahmad, A. Zada, J. Alzabut, Hyers–Ulam stability of a coupled system of fractional differential equations of Hilfer–Hadamard type, Demonstratio Mathematica, 52 (2019), 283–295. https://doi.org/10.1515/dema-2019-0024 doi: 10.1515/dema-2019-0024
![]() |
1. | Mahmoud A. Ibrahim, Attila Dénes, Mathematical Modeling of SARS-CoV-2 Transmission between Minks and Humans Considering New Variants and Mink Culling, 2023, 8, 2414-6366, 398, 10.3390/tropicalmed8080398 | |
2. | Hua He, Wendi Wang, Asymptotically periodic solutions of fractional order systems with applications to population models, 2024, 476, 00963003, 128760, 10.1016/j.amc.2024.128760 |
Parameters | Biological interpretations |
Describes the birth rate | |
The effective contact rate of social | |
distancing | |
Natural death rate | |
Shows the infected contact rate | |
The rate of symptoms among infected | |
people | |
Showed the death rate due to the | |
coronavirus | |
Recovery rates of individuals in the | |
infected class | |
Recovery rates of individuals in the | |
asymptomatic population | |
The transmission rate from the infected | |
class to the quarantined class | |
Shows the transition rate from | |
asymptomatic population to the | |
infected class | |
Represents the movement rate from the | |
quarantined quarantined population to the | |
asymptomatic population | |
Represented the rate of quarantine for | |
exposed persons | |
Recovery rate of individuals in the | |
hospitalized population |
Parameters | Parameters Values |
Parameters | Biological interpretations |
Describes the birth rate | |
The effective contact rate of social | |
distancing | |
Natural death rate | |
Shows the infected contact rate | |
The rate of symptoms among infected | |
people | |
Showed the death rate due to the | |
coronavirus | |
Recovery rates of individuals in the | |
infected class | |
Recovery rates of individuals in the | |
asymptomatic population | |
The transmission rate from the infected | |
class to the quarantined class | |
Shows the transition rate from | |
asymptomatic population to the | |
infected class | |
Represents the movement rate from the | |
quarantined quarantined population to the | |
asymptomatic population | |
Represented the rate of quarantine for | |
exposed persons | |
Recovery rate of individuals in the | |
hospitalized population |
Parameters | Parameters Values |