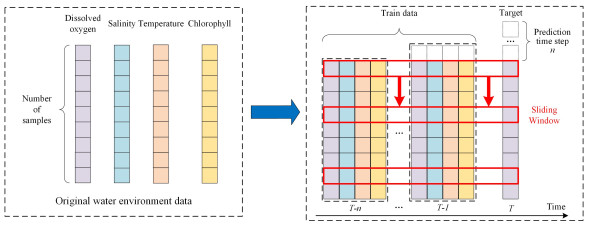
Citation: Jie Wang, Xin Pang, Hamid Jahed. Surface protection of Mg alloys in automotive applications: A review[J]. AIMS Materials Science, 2019, 6(4): 567-600. doi: 10.3934/matersci.2019.4.567
[1] | Daniela De Silva, Ovidiu Savin . Uniform density estimates and Γ-convergence for the Alt-Phillips functional of negative powers. Mathematics in Engineering, 2023, 5(5): 1-27. doi: 10.3934/mine.2023086 |
[2] | Vito Crismale, Gianluca Orlando . A lower semicontinuity result for linearised elasto-plasticity coupled with damage in W1,γ, γ > 1. Mathematics in Engineering, 2020, 2(1): 101-118. doi: 10.3934/mine.2020006 |
[3] | Matteo Novaga, Marco Pozzetta . Connected surfaces with boundary minimizing the Willmore energy. Mathematics in Engineering, 2020, 2(3): 527-556. doi: 10.3934/mine.2020024 |
[4] | Fernando Farroni, Giovanni Scilla, Francesco Solombrino . On some non-local approximation of nonisotropic Griffith-type functionals. Mathematics in Engineering, 2022, 4(4): 1-22. doi: 10.3934/mine.2022031 |
[5] | Kyungkeun Kang, Dongkwang Kim . Existence of generalized solutions for Keller-Segel-Navier-Stokes equations with degradation in dimension three. Mathematics in Engineering, 2022, 4(5): 1-25. doi: 10.3934/mine.2022041 |
[6] | Massimo Frittelli, Ivonne Sgura, Benedetto Bozzini . Turing patterns in a 3D morpho-chemical bulk-surface reaction-diffusion system for battery modeling. Mathematics in Engineering, 2024, 6(2): 363-393. doi: 10.3934/mine.2024015 |
[7] | Serena Della Corte, Antonia Diana, Carlo Mantegazza . Global existence and stability for the modified Mullins–Sekerka and surface diffusion flow. Mathematics in Engineering, 2022, 4(6): 1-104. doi: 10.3934/mine.2022054 |
[8] | Chiara Caracciolo . Normal form for lower dimensional elliptic tori in Hamiltonian systems. Mathematics in Engineering, 2022, 4(6): 1-40. doi: 10.3934/mine.2022051 |
[9] | Emilio N. M. Cirillo, Giuseppe Saccomandi, Giulio Sciarra . Compact structures as true non-linear phenomena. Mathematics in Engineering, 2019, 1(3): 434-446. doi: 10.3934/mine.2019.3.434 |
[10] | M. M. Bhatti, Efstathios E. Michaelides . Oldroyd 6-constant Electro-magneto-hydrodynamic fluid flow through parallel micro-plates with heat transfer using Darcy-Brinkman-Forchheimer model: A parametric investigation. Mathematics in Engineering, 2023, 5(3): 1-19. doi: 10.3934/mine.2023051 |
According to the Food and Agriculture Organization of the United Nations (FAO), more than one billion people worldwide rely on fish to supplement their bodies with animal protein [1]. Over the past 30 years, aquaculture has been the fastest-growing sector in agriculture. It is one of the pillar industries driving China's economy, creating many jobs in rural areas and bringing stable income to farmers [2]. With the development of artificial intelligence and big data technology, how to increase aquaculture production based on modern information technology and improve the information management of fisheries and fishery administration has become a hot research topic.
For aquatic animals, DO is essential in sustaining their lives, and survival and reproduction can only occur under oxygenated conditions. At the same time, too high or too low dissolved oxygen concentrations can be fatal to the health of aquatic products and must be kept within a reasonable range [3]. When DO levels are too high, fish are prone to bubble disease [4]. On the contrary, when DO is below the standard index for a long time, the growth of aquatic organisms will be slowed down, disease resistance will be reduced, and death will result in severe cases. Therefore, aquaculturists would be very convenient if the trend of DO concentration could be accurately predicted in advance. However, accurately predicting DO concentration trends are challenging. Since aquaculture is in an open-air environment some microorganisms in the water will increase the DO content through photosynthesis; meanwhile, fish and phytoplankton will also accelerate the oxygen consumption through respiration [5]. Different depths and water temperatures will also lead to uneven DO distribution within the culture water [6]. Therefore, the DO time series monitored by water quality sensors will show a nonlinear characteristic [7,8]. With the increase in the prediction time, the nonlinear characteristics will gradually decrease the model's accuracy [9,10].
In order to reduce the influence of nonlinear characteristics on prediction results, researchers have gradually designed various water quality prediction models for various application scenarios, which can be divided into mechanistic and nonmechanistic models according to their working principles [11,12]. The mechanical model is derived from the system structure based on the physical, chemical, biological and other reaction processes of the water environment system with the help of many hydrological, water quality, meteorological and other monitoring data [13]. Because the mechanistic model requires a large amount of basic information about the water environment, which is usually very complex, it limits the further application in water quality prediction.
With the development and application of computer technology, more and more non-mechanical models are being applied to water quality prediction [14]. The non-mechanical water quality prediction method does not consider the physical and chemical changes of the water body. It builds a corresponding model based on historical data to predict the changing trend. The process is simple, and the effect is good. Mainly including time series, regression, probabilistic statistical, machine learning, and deep learning [15,16,17]. For example, Shi et al. [18] propose the softplus extreme learning machine (ELM) model based on clustering to accurately predict changes in DO for the nonlinear characteristics of the DO data in aquaculture waters. The model employs partial least squares while using a new Softplus activation function to improve the ELM, which solves the nonlinearity problem in time series data streams and avoids the instability of the output weight coefficients. However, this model is not suitable for training a large amount of historical data, so that the deep learning model may be more suitable for actual aquatic data. Li et al. [19] developed three deep learning models, recurrent neural network (RNN), long short-term memory neural network (LSTM) and gated recurrent unit (GRU), to predict DO in fish ponds. The results showed that the performance of GRU is similar to LSTM, but the time cost and the number of parameters used by GRU are much lower than LSTM, which is more suitable for DO prediction in natural fish ponds. Although the above three deep learning models can obtain excellent prediction results while training a large amount of aquatic data, the accuracy will decrease significantly with the increase in prediction time.
In recent years, many scholars have also decomposed the raw DO data first and then used different machine learning models or deep learning models to predict the characteristics of the decomposition results. For example, Li et al. [20] proposed a hybrid model of integrated empirical mode decomposition based on multiscale features. Ren et al. [21] proposed a prediction model based on variational modal decomposition and deep belief network combined prediction model. Huang et al. [22] proposed a combined prediction model based on fully integrated empirical mode decomposition, adaptive noise and GRU combined with an improved particle swarm algorithm. Compared with various benchmark models, this method can effectively consider complex time-series data and predict DO variation trends reliably. This decomposition-based method can effectively separate and denoise the original data and enhance the neural network input data quality, thus improving the model's prediction accuracy. However, the method based on decomposition before prediction can cause the problem of boundary distortion, and there is a possibility that future data will be used in the model training [23]. Because of the excellent performance of the attention mechanism in artificial intelligence, many scholars have introduced it to DO prediction in aquaculture. For example, Bi et al. [24] added an attention mechanism after the LSTM network for multi-steps prediction of DO. Liu et al. [25] built a combined prediction model combining attention mechanism and RNN network and obtained excellent short-term and long-term prediction.
Based on previous studies, we investigate a combined model combining convolutional neural network, attention mechanism, and bidirectional long-term and short-term memory neural networks for short-term and long-term prediction of DO concentrations in aquaculture. It consists of a one-dimensional convolutional neural network (1D-CNN), a BiLSTM, and the AM, called the CNN-BiLSTM-AM model. The 1D-CNN helps the model extract important feature data from multiple input vectors. The BiLSTM adds forward and backward propagation to the LSTM, which can learn the input data's backward and forward adjacency relationships in the input data to achieve the purpose of fully mining the multiple data features. After this, the BiLSTM network is combined with the AM. The model's attention is focused on the moving step to capture the effect of different time steps on DO concentration prediction and improve the accuracy and stability of the model in long-term prediction.
The remainder of this paper is organized as follows: Section 2 describes the materials and methods. Section 3 details the experiments and analysis of results. Finally, Section 4 gives conclusions and directions for future work.
The time series is a set of numerical sequences formed by arranging them in chronological order [26]. Time series are divided into unary time series and multivariate time series, and multivariate time series is the combination of multiple unary time series, which can be regarded as a sampling process to obtain multiple observed variables from different sources [27]. The multidimensional DO concentration prediction model for aquaculture proposed in this paper is a multivariate time series prediction problem. For a given time series data with N characteristic variables, multivariate single-step prediction can be defined by the Eq (2.1):
⌢yt+1=f(x00,x11,⋅⋅⋅,xN−1t) | (2.1) |
where ⌢ yt+1 represents the model's estimate of DO concentration for one future moment, xji=(xj0,xj1,⋅⋅⋅,xjt)T represents the data vector for the j∈[0,N) eigenvariable at the moment i∈{0,1,2,⋅⋅⋅,t}.
The multivariate multi-step prediction is based on the multivariate single-step prediction. It uses k⋅N characteristic variables in the last k moments as inputs to predict the DO concentration for the next k moments. The Eq (2.2) demonstrates the k steps prediction.
(⌢yt+1,⋅⋅⋅,⌢yt+k)=f(X0,X1,⋅⋅⋅,Xk) | (2.2) |
where Xi∈{X1,X2,⋅⋅⋅,Xk} is a multi-step supervised learning dataset constructed i moments in advance, model f(⋅) is usually estimated using a supervised learning strategy and multi-step prediction, using training data and corresponding labels for estimation.
The data used in this paper were obtained from two aquaculture farms in Yantai, Shandong Province, China. The marine farm is equipped with multi-parameter water quality monitoring sensors to collect various water environment data in real-time, including water temperature, salinity, chlorophyll concentration, and DO concentration. All data are collected at a frequency of 10 minutes. This paper uses the first 80% of the data collected in the experiment as the training set samples. The last 20% of the data collected is divided into validation and test set samples. The training set is used to adjust the model's internal parameters, such as the weights and bias vectors of the neural network. The validation data check whether the model is overfitted or underfitting. The test set data is used to evaluate the model's predictive performance.
Multivariate multi-step prediction can be defined as a supervised learning problem. The multi-dimensional data are stitched together to form a matrix. Specifically, the inputs to the model are DO concentration, salinity, water temperature and chlorophyll content at the past time. The output of the model is the DO concentration at the current time. T denotes the current moment and n represents the prediction time step. Figure 1 illustrates the construction process of the supervised learning dataset in this paper, using multi-dimensional data from the previous (T−n) moments to predict the DO concentration at the moment. The data surrounded by red boxes represent a set of constructed training data and target labels, and the sliding window technique is used to repeat the operation to complete the construction of all data.
At the same time, the DO concentration at the current moment can be predicted in several time steps; the one-time step is 10 min, using sliding windows of different sizes. In order to verify the performance of the model in short-term prediction, three-step prediction (30 minutes) and six-steps prediction (1 hour) are selected for experimental analysis. The three-step prediction uses the historical data of the first three moments to predict the DO trend of the current moment 30 minutes in advance. The six-step prediction uses the historical data of the first six moments in advance to predict the DO trend of the current moment. Based on this, this paper chooses 36 steps ahead (6 hours) and 72 steps ahead (12 hours) to verify the performance of the CNN-BiLSTM-AM model in long-term prediction.
The CNN is an improvement of Lecun on multilayer perceptron (MLP) [28]. Due to its structural features of local area connectivity, weight sharing, and downsampling, the CNN excels in image processing. The application scenarios of CNN are specifically in image classification, face recognition, autonomous driving, and target detection [29,30,31]. There are three types of convolution operations: 1D, 2D convolution and 3D convolution [32]. The 1D convolution is used to process sequential data, such as in natural language processing; 2D convolution is often used in computer vision and image processing; 3D convolution is often used in medicine and video processing.
Since this paper focuses on predicting DO concentration sequences in aquaculture and is one-dimensional data, 1D-CNN is used for feature extraction. The 1D-CNN is calculated as shown in Eq (2.3):
ht=σ(W∗xt+b) | (2.3) |
where W denotes the convolution kernel, also called the weight coefficient of the filter in the convolution layer. b denotes the bias vector. xt represents the sample data of the tth input network. ∗ denotes the convolution operator. σ represents the activation function. ht represents the output result after the convolution operation.
Figure 2 shows the structure of 1D-CNN. In this paper, the 1D-CNN is used to extract the features of DO concentration for the characteristics of time series data. Firstly, the historical data of the aquaculture water environment with multiple features are stitched into a matrix. In order to feed the constructed supervised learning data into the interior of the CNN network, the dimensions of the matrix are therefore converted into k tensors. At the same time, each tensor has m rows and n columns. The k represents the size of the one-dimensional convolution kernel, m is a variety of water environment data from the past few days, and n represents the length of the time step. After the convolution operation, this paper uses maximum pooling to retain the most vital features and eliminate the weak ones. The purpose is to reduce the complexity and avoid overfitting the model. The step of maximum pooling is to place the pooling window on the sequence and use the maximum value within that window as the output value of the pooling. The window is then slid, and the above steps are repeated until the end of the sequence.
Although the RNN can learn the relationship between the current moment and the earlier moment information in the long time series prediction problem, the longer the time goes, the more difficult it is for RNN to learn this relationship. Researchers call this phenomenon the long-term dependence problem [33]. It is like a person with weak memory who cannot remember the past is the same. The root cause of the long-term dependency problem is that the gradient tends to disappear or explode after the RNN has propagated through many stages. To solve the long-term dependency problem, Hochreiter and Schmidhuber [34] proposed the LSTM in 1997. LSTM is a modification of RNN, and Figure 3 illustrates the basic structural unit of LSTM. The LSTM network consists of several structural units, each containing three gating mechanisms: forgetting, input, and output.
The forgetting gate mainly determines the degree of forgetting of previous information. The forgetting gate decides which information from the past is discarded after receiving the last moment output ht−1 and the current moment input xt. The forgetting gate of LSTM is calculated as follows:
ft=σ(Wf⋅[ht−1,xt]+bf) | (2.4) |
where ft represents the output of the forgetting gate and σ represents the activation function. The output of the sigmoid function in the forgetting gate ranges between (0,1) so that selective discarding of the data can be achieved.
The role of the input gate is to select which current information is input to the internal network after the forgetting gate has discarded some of the information [35,36]. The input gate of LSTM consists of two steps. Firstly, determining which values need to be updated according to Eqs (2.5) and (2.6). Secondly, updating the cell state of the last moment to the cell state of the current moment according to Eq {(2.7)}.
it=σ(Wi⋅[ht−1,xt]+bi) | (2.5) |
˜Ct=tanh(WC⋅[ht−1,xt]+bC) | (2.6) |
Ct=ft∗Ct−1+it∗˜Ct | (2.7) |
As the last part inside the LSTM network, the output gate obtains the model's output value, and the calculation formula is as follows: Eqs {(2.8) and (2.9)}. The initial output value ot is first calculated using the output ht−1 of the previous moment and the input xt of the current moment, which is used to control the information to be output. The hyperbolic tangent activation function (tanh) then scales the cell state ct at the current moment between −1 and 1 and multiplies the result by the initial output value ot to obtain the final output of the output gate.
ot=σ(Wo[ht−1,xt])+bo | (2.8) |
ht=ot∗tanh(Ct) | (2.9) |
where wf, wi, wc, wo are the weight matrices of oblivion gate, input gate, state update, and output gate. bf, bi, bc, and bo represent the corresponding bias vectors.
Although the LSTM network internally solves the long-term dependency problem by adding a threshold mechanism, the LSTM can only handle temporal information in one direction. For the long-time sequence problem, the current state may relate to the previous information and involve the following information. The BiLSTM combines the bi-directional recurrent neural network [37] and LSTM to fully use the sequence's historical and future information to improve the model's prediction performance [38]. Since BiLSTM networks can obtain the before-and-after features of the input sequences, they have been widely used in machine translation and speech recognition.
The BiLSTM neural network adds a forward layer and backward layer to the LSTM structure, and the main structure is shown in Figure 4. The principle of each propagation layer in BiLSTM is precisely the same as the structure of the one-way propagation LSTM model. As shown in Eq {(2.10)}, the final output consists of a superposition of LSTMs in both directions.
ht=→ht⊗←ht | (2.10) |
where t represents the moment in the time series; →h and ← h represent the output values of the forward and backward propagation layers, respectively. ht represents the output results after the forward and backward superposition at moment t.
When we look through a photo album quickly, we may not see the whole picture but often focus on the most beautiful parts of the picture. The AM references the above idea explained in mathematical language as X=[x1,x2,x3,⋅⋅⋅,xn] representing n inputs of information. In order to save computational resources, we do not want the neural network to process all the inputs but only select some information from the most relevant inputs to the task. In recent years, the AM mechanism has been widely used in image enhancement, text classification, and machine translation [39,40].
In this paper, we use soft attention, the most common attention method. It means that when information selection is performed, instead of selecting one of the n input information, the weighted sum of the n input information is calculated and then input to the neural network for calculation. In this paper, attention is assigned to the prediction step of the model based on the CNN-BiLSTM network.
The calculation steps are as follows: the first step is to calculate the similarity between the output hi(i=1,2,⋅⋅⋅,n) of the BiLSTM network at each time and the output ht of the current moment to get the corresponding weight si(i=1,2,⋅⋅⋅,n). The second step normalizes the weights si using the softmax(⋅) function. Obtain the output ht at the current moment with the weights αi(i=1,2,⋅⋅⋅,n) of the outputs hi at each moment. The third step weights the weight αi and the output hi of the BiLSTM neural network at each moment to obtain the final output ci. The formulas for the attention mechanism are given in Eqs {(2.11)–(2.13)}.
si=tanh(Whiht+bhi) | (2.11) |
αi=softmax(si) | (2.12) |
ct=n∑i=1αihi | (2.13) |
Figure 5 illustrates the CNN-BiLSTM-AM model framework built in this paper. Table 1 collates the details of the main parameters of the CNN-BiLSTM-AM model. In addition to the input and output layers, the model contains a convolutional layer, a BiLSTM layer, and an Attention layer.
Hyperparameter | Value |
Filter size of CNN | [128, 64] |
Kernel size of CNN | 1 |
Padding | same |
Activation function | Relu |
Unit number for BiLSTM | 128 |
Optimization function | adam |
Learning-rate | 0.001 |
Batch size | 64 |
Epoch number | 100 |
The first step is the data input layer, which uses multi-feature data from aquaculture water environments to construct a supervised learning dataset {(Xi,Yi)|i=1,2,⋅⋅⋅,n}, and constructs the features X and labels Y for model training. The second step is the convolution layer, which extracts the features in the sequence using one-dimensional convolution (Conv1D) while adding a pooling (MaxPooling) layer to reduce the complexity of the features to avoid overfitting of the model. Although the convolution and pooling stages of the convolution layer can fully extract the time-series features and enrich the diversity of features, CNN only considers the correlation links between adjacent data in the sequence and does not consider the problem of long-term information dependence of the time series.
In order to remedy this deficiency, the third step of this paper connects the BiLSTM layer to the CNN layer. The forward and reverse LSTM networks in the BiLSTM layer are utilized to fully consider the sequences' past and future information features. Since the BiLSTM network is based on the bi-directional recurrent neural network and the LSTM neural network, the core of the network is still the LSTM, so the feature information of the output of the CNN layer needs to be changed accordingly, as the tensor form of the data is consistent with the input format [number of samples, prediction time step size, and input feature dimension] required by the LSTM. In the BiLSTM layer, the BiLSTM network firstly traverses the data output from the CNN layer from left to right, and secondly traverses the data from right to left in the reverse direction, and finally stitches the output results from the above two directions together and submits them to the attention layer.
In this paper, we study the multi-dimensional multi-step time series prediction of DO concentration, and different time steps affect the model's accuracy. Therefore, the fourth step of this paper incorporates the AM after the BiLSTM layer, specifically, the soft attention mechanism. The AM is used to capture the importance of different prediction steps in the time series on the impact of the model and improve the model's overall prediction accuracy. The fifth step uses the Flatten Layer to transform the multi-dimensional input data into one dimension. Finally, the fully connected layer is added to obtain the model's output.
In this paper, the mean absolute error (MAE), root mean square error (RMSE), mean absolute percentage error (MAPE) and determination coefficient (R2) are chosen to assess the prediction accuracy of the model. Their calculation methods are shown in Eqs {(2.14)–(2.17)}.
MAE=1NN∑k=1|ˆyk−yk| | (2.14) |
RMSE=√1NN∑k=1(yk−ˆyk)2 | (2.15) |
MAPE=1NN∑k=1|ˆyk−ykyk|×100% | (2.16) |
R2=1−∑Nk=1(yk−⌢yk)2∑Nk=1(yk−ˉy2)2 | (2.17) |
where N is the number of samples in the test set, ⌢ yk is the actual value, and yk represents the prediction result. The model has higher prediction accuracy when the MAE and RMSE results are negligible. Conversely, a higher R2 value represents a better fit of the model on the test set and more accurate prediction results.
Aquaculture enterprises in the daily production and operation process, power outages, poor network signals and water quality sensor failure and other factors can easily lead to the collection of raw water environmental data missing values and abnormal. This paper uses the Lagrangian interpolation method to fill in the missing and abnormal data, calculated as in Eq (3.1). Table 2 collates the statistical information on DO concentrations monitored in aquaculture farms above.
Cases | Datasets | Numbers | Statistical indicators | |||||
Mean | Std. | Max. | Min. | Kurtosis | Skewness | |||
Dataset 1 | All Samples | 20851 | 8.09 | 1.20 | 10.25 | 5.02 | -0.64 | -0.62 |
Training Set | 16681 | 8.57 | 0.77 | 10.25 | 6.37 | -0.37 | -0.47 | |
Validation Set | 2085 | 6.54 | 0.25 | 7.09 | 5.84 | -0.53 | -0.04 | |
Testing Set | 2085 | 5.81 | 0.35 | 6.44 | 5.02 | -0.88 | -0.21 | |
Dataset 2 | All Samples | 21673 | 7.28 | 1.35 | 10.32 | 4.12 | -0.99 | 0.46 |
Training Set | 17339 | 6.75 | 0.94 | 9.08 | 4.12 | -0.69 | 0.48 | |
Validation Set | 2167 | 8.99 | 0.24 | 9.71 | 8.49 | -0.03 | 0.97 | |
Testing Set | 2167 | 9.70 | 0.22 | 10.32 | 9.32 | -1.47 | 0.19 |
At the same time, the different units of data in different water environments and the possible existence of odd sample data in the original data can negatively affect the model's training. For example, when gradient descent is performed, the gradient direction tends to deviate from the direction of the minimum value, resulting in a long training time for the model. Therefore, this paper adopts the normalization operation for the preprocessed data, and Eq (3.2) describes its calculation process.
Ln(x)=n∑k=0yk⋅(x−x0)(x−x1)⋅⋅⋅(x−xk−1)(x−xk+1)⋅⋅⋅(x−xn)(xk−x0)(xk−x1)⋅⋅⋅(xk−xk−1)(xk−xk+1)⋅⋅⋅(xk−xn) | (3.1) |
z′=z−min(z)max(z)−min(z) | (3.2) |
where xk(k=0,1,⋅⋅⋅,n) is several independent variables, yk is the value of the dependent variable corresponding to the independent variables, x is the interpolation node, and Ln(x) is the result after interpolation. z represents the different water environment parameters in the original data, min(z) and max(z) represent the minimum and maximum values under the same feature, respectively, z′ representing the normalized data results.
In the experiments of this paper, several classical prediction models were trained and predicted to compare and evaluate the prediction performance of CNN-BiLSTM-AM models. They are SVR, RNN, LSTM, CNN-LSTM and CNN-BiLSTM models.
Meanwhile, to ensure the fairness of the experiments, we use the same training set, validation set and test set for all the models and choose the same values for the same hyperparameters in these models. The MSE is used as the loss function for model training, and the weights of the models are optimized using the adaptive moment estimation method. The learning rate was 0.001 and the training period was 100 times. All models were done in a Python programming environment and implemented on the Keras framework.
In order to visualize the prediction results, Figure 6 shows the short-term prediction fitting curves of different comparison models and the CNN-BiLSTM-AM model proposed in this paper on the test set. The four subplots (a)–(d) represent 3-step prediction, 6-step prediction, 36-step prediction and 72-step prediction, respectively. It can be seen from the Figure 6 that the dissolved oxygen concentration in the water environment of this aquaculture farm shows a cyclic variation, fluctuating up and down in a particular range. Although all models could predict the overall trend of DO correctly, the prediction accuracy of different models varied considerably at the peaks and valleys of different time steps. We can see that the prediction curves of the SVR model deviate the farthest from the actual values at the peaks and valleys, and the rest of the deep learning models and the combined models are closer to the actual values.
Table 3 collates the short-term prediction results of the CNN-BiLSTM-Attention model proposed in this paper for dissolved oxygen concentration in an aquaculture water environment, including three steps ahead (30 minutes) and six steps ahead (1 hour). Based on the prediction results of 3 steps ahead (30 minutes), it can be seen that the MAE, RMSE and MAPE of the deep learning RNN model are reduced by 10.12, 4.34 and 13.19%, respectively, compared with the machine learning SVR model. The three metrics of LSTM were reduced by another 13.85, 9.59 and 12.66%, respectively, compared to the RNN model. It is verified that LSTM alleviates the long-term dependence problem of RNN by increasing the threshold mechanism and improving the model's prediction accuracy. Compared with the combined model, none of the three single prediction models has higher prediction accuracy than the combined model at 30 minutes of prediction. It reflects that the combined model can utilize different network structures to improve the overall prediction of short-term forecasts.
Methods | 3 steps | 6 steps | ||||||
MAE | RMSE | MAPE | R2 | MAE | RMSE | MAPE | R2 | |
SVR | 0.0514 | 0.0599 | 0.0091 | 0.9711 | 0.1238 | 0.1333 | 0.0219 | 0.8565 |
RNN | 0.0462 | 0.0573 | 0.0079 | 0.9736 | 0.0555 | 0.0638 | 0.0096 | 0.9671 |
LSTM | 0.0398 | 0.0518 | 0.0069 | 0.9799 | 0.0552 | 0.0627 | 0.0094 | 0.9683 |
CNN-LSTM | 0.0309 | 0.0394 | 0.0053 | 0.9875 | 0.0452 | 0.0575 | 0.0078 | 0.9733 |
CNN-BiLSTM | 0.0231 | 0.0342 | 0.0041 | 0.9907 | 0.0303 | 0.0429 | 0.0053 | 0.9851 |
Proposed | 0.0135 | 0.0187 | 0.0023 | 0.9971 | 0.0202 | 0.0293 | 0.0034 | 0.9931 |
To verify whether the model's prediction performance is improved after adding AM, a CNN-BiLSTM comparison model is introduced in this paper. The experimental results show that the prediction performance of the CNN-BiLSTM-AM model after adding AM improves by 41.56, 45.32 and 43.9%, respectively, when predicting 30 minutes in the short term. It demonstrates that focusing the model's attention on the time step can improve the short-term prediction performance of the model. In the prediction results of 6 steps ahead (1 hour), SVR reduced 11.43% in R2 metric compared to LSTM in deep learning models. The CNN-BiLSTM-AM model proposed in this paper also improved 33.33, 31.7 and 35.85% over the best-performing CNN-BiLSTM model in the combined neural network.
To verify whether the CNN-BiLSTM-AM model proposed in this paper still has excellent prediction performance for long-term prediction, we take 36 steps ahead (6 hours) and 72 steps ahead (12 hours) for multiple time steps of DO concentration prediction. According to Table 4, the prediction accuracy of the SVR model decreases sharply as the prediction time step increases. In the R2 index, its accuracy decreased by 21.97%, from 97.11% for 3-step prediction to 75.77% for 72-step prediction. RNN and LSTM closely follow this.
Methods | 36 steps | 72 steps | ||||||
MAE | RMSE | MAPE | R2 | MAE | RMSE | MAPE | R2 | |
SVR | 0.1352 | 0.1490 | 0.0240 | 0.8142 | 0.1365 | 0.1713 | 0.0247 | 0.7577 |
RNN | 0.0794 | 0.0923 | 0.0135 | 0.9287 | 0.0881 | 0.1063 | 0.0154 | 0.9067 |
LSTM | 0.0633 | 0.0765 | 0.0109 | 0.9513 | 0.0675 | 0.0797 | 0.0115 | 0.9476 |
CNN-LSTM | 0.0448 | 0.0599 | 0.0078 | 0.9756 | 0.0479 | 0.0601 | 0.0082 | 0.9702 |
CNN-BiLSTM | 0.0347 | 0.0451 | 0.0062 | 0.9835 | 0.0452 | 0.0584 | 0.0079 | 0.9719 |
Proposed | 0.0156 | 0.0219 | 0.0027 | 0.9968 | 0.0283 | 0.0381 | 0.0049 | 0.9883 |
The 36-step predictions of RNN and LSTM decrease by 3.97 and 1.75%, respectively, in R2 compared to their 6-step predictions for short-term prediction of DO concentration. Although the accuracy of long-term prediction was reduced, LSTM had higher stability than RNN, verifying that LSTM alleviated the long-term dependence problem by its unique gating mechanism. The combined neural network models all outperformed the single model in long-term prediction. Compared with LSTM, CNN-BiLSTM improved by 45.18, 41.06 and 43.12% in 36-step prediction, and 33.04, 26.73 and 31.3% in 72-step prediction.
Happily, the performance of the CNN-BiLSTM-AM model used in this paper is 55.04, 51.44 and 56.45% higher than that of CNN-BiLSTM without attention mechanism in 36-step prediction. In the 72-step prediction, each index improved by 37.39, 34.76 and 37.97%, respectively. The above results indicate that the CNN-BiLSTM-AM model has more robust prediction performance on longer prediction steps and has higher stability than similar models.
Based on the above, this paper explores the generalization ability of CNN-BiLSTM-AM model on different datasets, and we use the water environment data monitored by another farm in Yantai. As shown in Figure 8, it can be seen that the periodicity of this dataset is not apparent, and the overall dissolved oxygen content is higher than that of the first dataset.
Tables 5 and 6 summarize the performance metrics of the different models for short-term and long-term forecasting on this dataset. We can clearly see that the CNN-BiLSTM model with the added attention mechanism has excellent performance in all metrics. In terms of MAE metrics, it improves 25.29%, 26.10%, 33.43% and 21.59% in short-term and long-term predictions, respectively, over the CNN-BiLSTM model without the added attention mechanism. It improved 30.76%, 48.85%, 49.31% and 44.24% in short-term and long-term predictions, respectively, over the RNN model. The growth trend of the prediction steps shows that adding the attention mechanism to the CNN-BiLSTM model in this paper can effectively enhance the long-term prediction ability of the model and has higher prediction accuracy compared with the benchmark model.
Methods | 3 steps | 6 steps | ||||||
MAE | RMSE | MAPE | R2 | MAE | RMSE | MAPE | R2 | |
SVR | 0.0431 | 0.0514 | 0.0043 | 0.9473 | 0.0518 | 0.0607 | 0.0053 | 0.9264 |
RNN | 0.0273 | 0.0368 | 0.0028 | 0.9729 | 0.0393 | 0.0502 | 0.0040 | 0.9497 |
LSTM | 0.0208 | 0.0321 | 0.0021 | 0.9793 | 0.0334 | 0.0452 | 0.0034 | 0.9592 |
CNN-LSTM | 0.0257 | 0.0323 | 0.0027 | 0.9791 | 0.0329 | 0.0413 | 0.0034 | 0.9659 |
CNN-BiLSTM | 0.0253 | 0.0314 | 0.0026 | 0.9803 | 0.0272 | 0.0352 | 0.0028 | 0.9753 |
Proposed | 0.0189 | 0.0257 | 0.0019 | 0.9869 | 0.0201 | 0.0266 | 0.0021 | 0.9858 |
Methods | 36 steps | 72 steps | ||||||
MAE | RMSE | MAPE | R2 | MAE | RMSE | MAPE | R2 | |
SVR | 0.0849 | 0.0971 | 0.0086 | 0.8126 | 0.1013 | 0.0118 | 0.0104 | 0.7519 |
RNN | 0.0436 | 0.0555 | 0.0045 | 0.9395 | 0.0495 | 0.0642 | 0.0051 | 0.9184 |
LSTM | 0.0409 | 0.0499 | 0.0042 | 0.9505 | 0.0420 | 0.0532 | 0.0043 | 0.9443 |
CNN-LSTM | 0.0394 | 0.0494 | 0.0041 | 0.9516 | 0.0422 | 0.0504 | 0.0044 | 0.9497 |
CNN-BiLSTM | 0.0332 | 0.0402 | 0.0034 | 0.9683 | 0.0352 | 0.0446 | 0.0036 | 0.9606 |
Proposed | 0.0221 | 0.0298 | 0.0023 | 0.9824 | 0.0276 | 0.0372 | 0.0028 | 0.9726 |
Both Figure 7 and Figure 9 show a combination of bar and line graphs that visualize the prediction accuracy of the different prediction models on the two data sets, respectively. Subplots (a) to (d) in the figures represent 3-step forecasting, 6-step forecasting, 36-step forecasting, and 72-step forecasting, respectively. Starting from the legend, MAE and RMSE correspond to the orange and green bars, whose values correspond to the left y-axis. mape corresponds to the purple beads, whose values correspond to the right y-axis. The red line graphs represent the coefficients of determination of the indicators, and higher values represent better fitting results of the model.
In summary, we can conclude that both short-term prediction (30 minutes, 1 hour) and long-term prediction (6 hours, 12 hours). The CNN-BiLSTM-AM model proposed in this paper has excellent prediction performance and better stability in long-term prediction.
This paper proposed a multi-step prediction model of dissolved oxygen concentration based on the combination of attention mechanisms and a combined neural network to improve the prediction accuracy of dissolved oxygen concentration in an aquaculture water environment. The model incorporates an attention mechanism in the BiLSTM network to focus the model's attention on the time step of prediction, which enhances the long-term prediction ability of the model. By comparing the SVR, RNN, LSTM, CNN-LSTM and CNN-BiLSTM prediction models, the CNN-BiLSTM-AM model established in this paper has higher prediction accuracy. As the time step increases, the model's superiority becomes more evident.
In the study process, this paper only used four water quality parameters as the input of the model. Future studies can consider on-farm weather factors and more water quality factors for the model.
This work was supported by the Yantai Science and Technology Innovation Development Plan Project (Grant No. 2022XDRH015).
The authors declare that there is no conflict of interests regarding the publication of this paper.
[1] | Kumar DS, Sasanka CT, Ravindra K, et al. (2015) Magnesium and its alloys in automotive applications-a review. Am J Mater Sci Technol 4: 12–30. |
[2] | Narayanan TS, Park IS, Lee MH (2015) Surface modification of magnesium and its alloys for biomedical applications: opportunities and challenges, In: Surface Modification of Magnesium and its Alloys for Biomedical Applications, Woodhead Publishing, 29–87. |
[3] |
Sakintuna B, Lamari-Darkrim F, Hirscher M (2007) Metal hydride materials for solid hydrogen storage: a review. Int J Hydrogen Energ 32: 1121–1140. doi: 10.1016/j.ijhydene.2006.11.022
![]() |
[4] |
Huie MM, Bock DC, Takeuchi ES, et al. (2015) Cathode materials for magnesium and magnesium-ion based batteries. Coordin Chem Rev 287: 15–27. doi: 10.1016/j.ccr.2014.11.005
![]() |
[5] |
Kulekci MK (2008) Magnesium and its alloys applications in automotive industry. Int J Adv Manuf Tech 39: 851–865. doi: 10.1007/s00170-007-1279-2
![]() |
[6] | Asian Metal, Magnesium uses, 2018. Available from: http://metalpedia.asianmetal.com/metal/magnesium/application.shtml. |
[7] | Brady MP, Joost WJ, David Warren C (2016) Insights from a recent meeting: current status and future directions in magnesium corrosion research. Corrosion 73: 452–462. |
[8] | Merdian, Magnesium Alloys in the Automotive Market, 2015. Available from: https://www.amm.com/events/download.ashx/document/speaker/7981/a0ID000000X0kJUMAZ/Presentation. |
[9] | Du CP, Xu DF (2013) Application of energy-saving magnesium alloy in automotive industry. Adv Mater Res 734: 2244–2247. |
[10] | Blawert C, Hort N, Kainer KU (2004) Automotive applications of magnesium and its alloys. Trans Indian Inst Met 57: 397–408. |
[11] | Luo AA (2002) Magnesium: current and potential automotive applications. JOM 54: 42–48. |
[12] | Hussein RO, Northwood DO (2014) Improving the performance of magnesium alloys for automotive applications. WIT T Built Environ 137: 531–544. |
[13] | Beecham M, Magnesium in car production-a weighting game, Bromsgrove (UK): Arop Ltd, 2017. Available from: https://www.just-auto.com/analysis/magnesium-in-car-production-a-weighting game_id176723.aspx. |
[14] | Carpenter JA, Jackman J, Li NY, et al. (2007) Automotive Mg research and development in North America. Mater Sci Forum 546: 11–24. |
[15] |
Friedrich H, Schumann S (2001) Research for a "new age of magnesium" in the automotive industry. J Mater Process Tech 117: 276–281. doi: 10.1016/S0924-0136(01)00780-4
![]() |
[16] | Brownell B, How Dangerous Are Magnesium Body Panels? 2016. Available from: http://www.thedrive.com/flat-six-society/6302/how-dangerous-are-magnesium-body-panels. |
[17] |
Luo AA (2013) Magnesium casting technology for structural applications. J Magnesium Alloy 1: 2–22. doi: 10.1016/j.jma.2013.02.002
![]() |
[18] |
Joost WJ, Krajewski PE (2017) Towards magnesium alloys for high-volume automotive applications. Scripta Mater 128: 107–112. doi: 10.1016/j.scriptamat.2016.07.035
![]() |
[19] | Cole GS (2013) Magnesium (Mg) corrosion protection techniques in the automotive industry, In: Corrosion Prevention of Magnesium Alloys, Woodhead Publishing, 489–508. |
[20] |
Esmaily M, Svensson JE, Fajardo S, et al. (2017) Fundamentals and advances in magnesium alloy corrosion. Prog Mater Sci 89: 92–193. doi: 10.1016/j.pmatsci.2017.04.011
![]() |
[21] |
Song G, Atrens A (2003) Understanding magnesium corrosion-a framework for improved alloy performance. Adv Eng Mater 5: 837–858. doi: 10.1002/adem.200310405
![]() |
[22] |
Song G (2005) Recent progress in corrosion and protection of magnesium alloys. Adv Eng Mater 7: 563–586. doi: 10.1002/adem.200500013
![]() |
[23] |
Atrens A, Song GL, Cao F, et al. (2013) Advances in Mg corrosion and research suggestions. J Magnesium Alloy 1: 177–200. doi: 10.1016/j.jma.2013.09.003
![]() |
[24] | Nowak P, Mosiałek M, Nawrat G (2015) Corrosion of magnesium and its alloys: new observations and ideas. Ochrona przed Korozją 11: 371–377. |
[25] | Skar JI, Albright D (2016) Emerging trends in corrosion protection of magnesium die-castings, In: Essential Readings in Magnesium Technology, Springer, Cham, 585–591. |
[26] |
Gray J, Luan B (2002) Protective coatings on magnesium and its alloys-a critical review. J Alloy Compd 336: 88–113. doi: 10.1016/S0925-8388(01)01899-0
![]() |
[27] | Abela S (2011) Protective coatings for magnesium alloys, In: Magnesium Alloys-Corrosion and Surface Treatments, InTech. |
[28] | Chen XB, Birbilis N, Abbott TB (2011) Review of corrosion-resistant conversion coatings for magnesium and its alloys. Corrosion 67: 035005-1. |
[29] |
Blawert C, Dietzel W, Ghali E, et al. (2006) Anodizing treatments for magnesium alloys and their effect on corrosion resistance in various environments. Adv Eng Mater 8: 511–533. doi: 10.1002/adem.200500257
![]() |
[30] | Gadow R, Gammel FJ, Lehnert F, et al. (2000) Coating system for magnesium diecastings in class A surface quality. Magnesium Alloy Appl 492–498. |
[31] | Höche D, Nowak A, John-Schillings T (2013) Surface cleaning and pre-conditioning surface treatments to improve the corrosion resistance of magnesium (Mg) alloys, In: Corrosion Prevention of Magnesium Alloys, Woodhead Publishing, 87–109. |
[32] |
Wu CY, Zhang J (2011) State-of-art on corrosion and protection of magnesium alloys based on patent literatures. T Nonferr Metal Soc 21: 892–902. doi: 10.1016/S1003-6326(11)60799-1
![]() |
[33] |
Ke C, Song MS, Zeng RC, et al. (2019) Interfacial study of the formation mechanism of corrosion resistant strontium phosphate coatings upon Mg-3Al-4.3Ca-0.1Mn. Corros Sci 151: 143–153. doi: 10.1016/j.corsci.2019.02.024
![]() |
[34] |
Lehr IL, Saidman SB (2018) Corrosion protection of AZ91D magnesium alloy by a cerium-molybdenum coating-The effect of citric acid as an additive. J Magnesium Alloy 6: 356–365. doi: 10.1016/j.jma.2018.10.002
![]() |
[35] |
Zeng R, Lan Z, Kong L, et al. (2011) Characterization of calcium-modified zinc phosphate conversion coatings and their influences on corrosion resistance of AZ31 alloy. Surf Coat Tech 205: 3347–3355. doi: 10.1016/j.surfcoat.2010.11.027
![]() |
[36] |
Pommiers S, Frayret J, Castetbon A, et al. (2014) Alternative conversion coatings to chromate for the protection of magnesium alloys. Corros Sci 84: 135–146. doi: 10.1016/j.corsci.2014.03.021
![]() |
[37] |
Van Phuong N, Lee K, Chang D, et al. (2013) Zinc phosphate conversion coatings on magnesium alloys: a review. Met Mater Int 19: 273–281. doi: 10.1007/s12540-013-2023-0
![]() |
[38] |
Jian SY, Chu YR, Lin CS (2015) Permanganate conversion coating on AZ31 magnesium alloys with enhanced corrosion resistance. Corros Sci 93: 301–309. doi: 10.1016/j.corsci.2015.01.040
![]() |
[39] |
Zeng RC, Zhang F, Lan ZD, et al. (2014) Corrosion resistance of calcium-modified zinc phosphate conversion coatings on magnesium–aluminum alloys. Corros Sci 88: 452–459. doi: 10.1016/j.corsci.2014.08.007
![]() |
[40] |
Lin CS, Lin HC, Lin KM, et al. (2006) Formation and properties of stannate conversion coatings on AZ61 magnesium alloys. Corros Sci 48: 93–109. doi: 10.1016/j.corsci.2004.11.023
![]() |
[41] | Greene JA, Vonk DR (2004) Conversion coatings including alkaline earth metal fluoride complexes. U.S. Patent No. 6,749,694. 2014-6-15. |
[42] | Morris WC (1942) Process for coating metal surfaces. U.S. Patent No. 2,294,760. 1942-9-1. |
[43] | Guerci G, Mus C, Stewart K (2000) Surface treatments for large automotive magnesium components. Magnesium Alloy Appl 484–491. |
[44] |
Rudd AL, Breslin CB, Mansfeld F (2000) The corrosion protection afforded by rare earth conversion coatings applied to magnesium. Corros Sci 42: 275–288. doi: 10.1016/S0010-938X(99)00076-1
![]() |
[45] |
Takenaka T, Ono T, Narazaki Y, et al. (2007) Improvement of corrosion resistance of magnesium metal by rare earth elements. Electrochim Acta 53: 117–121. doi: 10.1016/j.electacta.2007.03.027
![]() |
[46] |
Doerre M, Hibbitts L, Patrick G, et al. (2018) Advances in Automotive Conversion Coatings during Pretreatment of the Body Structure: A Review. Coatings 8: 405. doi: 10.3390/coatings8110405
![]() |
[47] |
Milošev I, Frankel GS (2018) Review-Conversion Coatings Based on Zirconium and/or Titanium. J Electrochem Soc 165: C127–C144. doi: 10.1149/2.0371803jes
![]() |
[48] | Giles TR, Goodreau BH, Fristad WE, et al. (2009) An update of new conversion coating for the automotive industry. SAE Int J Mater Manuf 1: 575–581. |
[49] |
Brady MP, Leonard DN, Meyer III HM, et al. (2016) Advanced characterization study of commercial conversion and electrocoating structures on magnesium alloys AZ31B and ZE10A. Surf Coat Tech 294: 164–176. doi: 10.1016/j.surfcoat.2016.03.066
![]() |
[50] | Li N, Chen X, Hubbert T, et al. (2005) 2005 Ford GT Magnesium Instrument Panel Cross Car Beam. SAE Technical Paper 2005-01-0341. |
[51] | Environmental Friendly Conversion Coating on Mg Alloy. Institute of Metal Research Chinese Academy of Sciences, 2018. Available from: http://english.imr.cas.cn/news/newsrelease/201408/t20140829_126968.html. |
[52] |
Chiu KY, Wong MH, Cheng FT, et al. (2007) Characterization and corrosion studies of fluoride conversion coating on degradable Mg implants. Surf Coat Tech 202: 590–598. doi: 10.1016/j.surfcoat.2007.06.035
![]() |
[53] |
Chen XB, Yang HY, Abbott TB, et al. (2014) Corrosion protection of magnesium and its alloys by metal phosphate conversion coatings. Surf Eng 30: 871–879. doi: 10.1179/1743294413Y.0000000235
![]() |
[54] | Dolan SE (1995) Composition and process for treating metals. U.S. Patent No. 5,449,415. 1995-9-12. |
[55] | Walter M, Kurze P (2004) MAGPASS-COAT® as a Chrome-free Pre-treatment for Paint Layers and an Adhesive Primer for Subsequent Bonding. SAE Technical Paper 2004-01-0134. |
[56] | Kainer KU (2007) Magnesium: proceedings of the 7th International Conference on Magnesium Alloys and their Applications, John Wiley & Sons. |
[57] | Chemetall. Oxsilan® . Chemetall GmbH, 2018. Available from: http://www.chemetall.com/Products/Trademarks/Oxsilan/index.jsp. |
[58] | CHEMEON TCP-HF. NALTIC Industrials, LLC, 2018. Available from: http://naltic.com/chemeon-tcp-hf.html. |
[59] | SurTec. SurTec 650: Chromium (VI)-Free Passivation for Aluminium for the Electronics,-Automotive and Aerospace Industry. SurTec, 2018. Available from: https://www.surtec.com/en/products/product-highlights/628/. |
[60] | ATOTECH. Interlox® 5707: Phosphate-free paint pretreatment for multimetal applocations. ATOTECH, 2018. Available from: https://www.atotech.com/paint-support-technologies/interlox-5707/. |
[61] | PPG Industrial Coatings. ZIRCOBOND® and X-BONDTM: Zirconium-Based Thin-Film Pretreatment System. PPG Industrial Coatings, 2018. Available from: http://www.ppgindustrialcoatings.com/getmedia/591ca2e3-ec3d-4f60-9315-5bd2b9e37159/Zircobond-X-Bond-Sell-Sheet_v2-02-06-16LowRes.pdf.aspx. |
[62] |
Cui LY, Zeng RC, Guan SK, et al. (2017) Degradation mechanism of micro-arc oxidation coatings on biodegradable Mg-Ca alloys: The influence of porosity. J Alloy Compd 695: 2464–2476. doi: 10.1016/j.jallcom.2016.11.146
![]() |
[63] |
Ding ZY, Cui LY, Chen XB, et al. (2018) In vitro corrosion of micro-arc oxidation coating on Mg-1Li-1Ca alloy-The influence of intermetallic compound Mg2Ca. J Alloy Compd 764: 250–260. doi: 10.1016/j.jallcom.2018.06.073
![]() |
[64] |
Cui LY, Liu HP, Zhang WL, et al. (2017) Corrosion resistance of a superhydrophobic micro-arc oxidation coating on Mg-4Li-1Ca alloy. J Mater Sci Technol 33: 1263–1271. doi: 10.1016/j.jmst.2017.10.010
![]() |
[65] |
Cui LY, Gao SD, Li PP, et al. (2017) Corrosion resistance of a self-healing micro-arc oxidation/polymethyltrimethoxysilane composite coating on magnesium alloy AZ31. Corros Sci 118: 84–95. doi: 10.1016/j.corsci.2017.01.025
![]() |
[66] | Xue Y, Pang X, Jiang B, et al. (2018) Corrosion performances of micro-arc oxidation coatings on Az31B, Az80 and Zk60 cast Mg alloys. CSME Conference Proceedings. |
[67] | Xue Y, Pang X, Jiang B, et al. (2018) Influence of micro-arc oxidation coatings on corrosion performances of AZ80 cast alloy. Int J Electrochem Sc 13: 7265–7281. |
[68] | Song GL, Shi Z (2013) Anodization and corrosion of magnesium (Mg) alloys, In: Corrosion Prevention of Magnesium Alloys, Woodhead Publishing, 232–281. |
[69] | Jiang BL, Ge YF (2013) Micro-arc oxidation (MAO) to improve the corrosion resistance of magnesium (Mg) alloys, In: Corrosion Prevention of Magnesium Alloys, Woodhead Publishing, 163–196. |
[70] | Shapiro J. Alodine EC2 Prevents Corrosion on Volvo Penta's Lighter Greener Pleasure Craft. MachineDesign, 2010. Available from: https://www.machinedesign.com/news/alodine-ec2-prevents-corrosion-volvo-penta-s-lighter-greener-pleasure-craft. |
[71] | Coating Applications. Technology Applications Group, 2018. Available from: http://www.tagnite.com/applications/#?201,115. |
[72] | Azumi K, Elsentriecy HH, Tang J (2013) Plating techniques to protect magnesium (Mg) alloys from corrosion, In: Corrosion Prevention of Magnesium Alloys, Woodhead Publishing, 347–369. |
[73] | Chen XB, Easton MA, Birbilis N, et al. (2013) Corrosion-resistant electrochemical plating of magnesium (Mg) alloys, In: Corrosion Prevention of Magnesium Alloys, Woodhead Publishing, 315–346. |
[74] |
El Mahallawy N (2008) Surface Treatment of Magnesium Alloys by Electroless Ni–P Plating Technique with Emphasis on Zinc Pre-treatment: a Review. Key Eng Mater 384: 241–262. doi: 10.4028/www.scientific.net/KEM.384.241
![]() |
[75] |
Lei XP, Yu G, Zhu YP, et al. (2010) Successful cyanide free plating protocols on magnesium alloys. T IMF 88: 75–80. doi: 10.1179/174591910X12646055765330
![]() |
[76] |
Hu RG, Zhang S, Bu JF, et al. (2012) Recent progress in corrosion protection of magnesium alloys by organic coatings. Prog Org Coat 73: 129–141. doi: 10.1016/j.porgcoat.2011.10.011
![]() |
[77] | Wang GG, Stewart K, Berkmortel R, et al. (2001) Corrosion prevention for external magnesium automotive components. SAE Technical Paper 2001-01-0421. |
[78] | Magnesium Coatings Suppliers. Thomas, 2018. Available from: https://www.thomasnet.com/products/magnesium-coatings-15800535-1.html. |
[79] | High pressure: magnesium diecasting from STIHL. STIHL team, 2017. Available from: http://blog.stihl.com/products-/2017/11/stihl-magnesium-diecasting-plant/. |
[80] |
Guo L, Wu W, Zhou Y, et al. (2018) Layered double hydroxide coatings on magnesium alloys: A review. J Mater Sci Technol 34: 1455–1466. doi: 10.1016/j.jmst.2018.03.003
![]() |
[81] |
Zhang G, Wu L, Tang A, et al. (2017) A novel approach to fabricate protective layered double hydroxide films on the surface of anodized Mg‐Al alloy. Adv Mater Interfaces 4: 1700163. doi: 10.1002/admi.201700163
![]() |
[82] |
Wu L, Zhang G, Tang A, et al. (2017) Communication-fabrication of protective layered double hydroxide films by conversion of anodic films on magnesium alloy. J Electrochem Soc 164: C339–C341. doi: 10.1149/2.0921707jes
![]() |
[83] |
Wu L, Yang D, Zhang G, et al. (2018) Fabrication and characterization of Mg-M layered double hydroxide films on anodized magnesium alloy AZ31. Appl Surf Sci 431: 177–186. doi: 10.1016/j.apsusc.2017.06.244
![]() |
[84] |
Zeng RC, Liu ZG, Zhang F, et al. (2014) Corrosion of molybdate intercalated hydrotalcite coating on AZ31 Mg alloy. J Mater Chem A 2: 13049–13057. doi: 10.1039/C4TA01341G
![]() |
[85] |
Adsul SH, Raju KRCS, Sarada BV, et al. (2018) Evaluation of self-healing properties of inhibitor loaded nanoclay-based anticorrosive coatings on magnesium alloy AZ91D. J Magnesium Alloy 6: 299–308. doi: 10.1016/j.jma.2018.05.003
![]() |
[86] |
Bala N, Singh H, Karthikeyan J, et al. (2014) Cold spray coating process for corrosion protection: a review. Surf Eng 30: 414–421. doi: 10.1179/1743294413Y.0000000148
![]() |
[87] |
Hassani-Gangaraj SM, Moridi A, Guagliano M (2015) Critical review of corrosion protection by cold spray coatings. Surf Eng 31: 803–815. doi: 10.1179/1743294415Y.0000000018
![]() |
[88] |
Spencer K, Fabijanic DM, Zhang MX (2009) The use of Al–Al2O3 cold spray coatings to improve the surface properties of magnesium alloys. Surf Coat Tech 204: 336–344. doi: 10.1016/j.surfcoat.2009.07.032
![]() |
[89] |
Wang Q, Spencer K, Birbilis N, et al. (2010) The influence of ceramic particles on bond strength of cold spray composite coatings on AZ91 alloy substrate. Surf Coat Tech 205: 50–56. doi: 10.1016/j.surfcoat.2010.06.008
![]() |
[90] |
DeForce BS, Eden TJ, Potter JK (2011) Cold spray Al-5% Mg coatings for the corrosion protection of magnesium alloys. J Therm Spray Techn 20: 1352–1358. doi: 10.1007/s11666-011-9675-4
![]() |
[91] |
Bu H, Yandouzi M, Lu C, et al. (2012) Cold spray blended Al + Mg17Al12 coating for corrosion protection of AZ91D magnesium alloy. Surf Coat Tech 207: 155–162. doi: 10.1016/j.surfcoat.2012.06.050
![]() |
[92] |
Zhan W, Tian F, Ou-Yang G, et al. (2018) Effects of Nickel Additive on Micro-Arc Oxidation Coating of AZ63B Magnesium Alloy. Int J Precis Eng Man 19: 1081–1087. doi: 10.1007/s12541-018-0128-6
![]() |
[93] |
Tomozawa M, Hiromoto S (2011) Microstructure of hydroxyapatite-and octacalcium phosphate-coatings formed on magnesium by a hydrothermal treatment at various pH values. Acta Mater 59: 355–363. doi: 10.1016/j.actamat.2010.09.041
![]() |
[94] |
Cui X, Liu C, Yang R, et al. (2013) Duplex-layered manganese phosphate conversion coating on AZ31 Mg alloy and its initial formation mechanism. Corros Sci 76: 474–485. doi: 10.1016/j.corsci.2013.07.024
![]() |
[95] |
Lee YL, Chu YR, Li WC, et al. (2013) Effect of permanganate concentration on the formation and properties of phosphate/permanganate conversion coating on AZ31 magnesium alloy. Corros Sci 70: 74–81. doi: 10.1016/j.corsci.2013.01.014
![]() |
[96] |
Zhao M, Wu S, Luo JR, et al. (2006) A chromium-free conversion coating of magnesium alloy by a phosphate–permanganate solution. Surf Coat Tech 200: 5407–5412. doi: 10.1016/j.surfcoat.2005.07.064
![]() |
[97] |
Cui X, Liu C, Yang R, et al. (2012) Phosphate film free of chromate, fluoride and nitrite on AZ31 magnesium alloy and its corrosion resistance. T Nonferr Metal Soc 22: 2713–2718. doi: 10.1016/S1003-6326(11)61522-7
![]() |
[98] | Li L, Qu Q, Fang Z, et al. (2012) Enhanced corrosion resistance of AZ31B magnesium alloy by cooperation of rare earth cerium and stannate conversion coating. Int J Electrochem Sci 7: 12690–12705. |
[99] |
Zeng R, Yan HU, Zhang F, et al. (2016) Corrosion resistance of cerium-doped zinc calcium phosphate chemical conversion coatings on AZ31 magnesium alloy. T Nonferr Metal Soc 26: 472–483. doi: 10.1016/S1003-6326(16)64102-X
![]() |
[100] |
Ba Z, Dong Q, Zhang X, et al. (2017) Cerium-based modification treatment of Mg-Al hydrotalcite film on AZ91D Mg alloy assisted with alternating electric field. J Alloy Compd 695: 106–113. doi: 10.1016/j.jallcom.2016.10.139
![]() |
[101] |
Ardelean H, Frateur I, Marcus P (2008) Corrosion protection of magnesium alloys by cerium, zirconium and niobium-based conversion coatings. Corros Sci 50: 1907–1918. doi: 10.1016/j.corsci.2008.03.015
![]() |
[102] |
Zhao M, Wu S, An P, et al. (2006) Microstructure and corrosion resistance of a chromium-free multi-elements complex coating on AZ91D magnesium alloy. Mater Chem Phys 99: 54–60. doi: 10.1016/j.matchemphys.2005.08.078
![]() |
[103] |
Jiang X, Guo R, Jiang S (2015) Microstructure and corrosion resistance of Ce–V conversion coating on AZ31 magnesium alloy. Appl Surf Sci 341: 166–174. doi: 10.1016/j.apsusc.2015.02.195
![]() |
[104] |
Zhao M, Li J, He G, et al. (2013) Nano Al2O3/phosphate composite conversion coating formed on magnesium alloy for enhancing corrosion resistance. J Electrochem Soc 160: C553–C559. doi: 10.1149/2.059311jes
![]() |
[105] |
Li K, Liu J, Lei T, et al. (2015) Optimization of process factors for self-healing vanadium-based conversion coating on AZ31 magnesium alloy. Appl Surf Sci 353: 811–819. doi: 10.1016/j.apsusc.2015.07.052
![]() |
[106] |
Cheng Y, Wu H, Chen Z, et al. (2006) Phosphating process of AZ31 magnesium alloy and corrosion resistance of coatings. T Nonferr Metal Soc 16: 1086–1091. doi: 10.1016/S1003-6326(06)60382-8
![]() |
[107] |
Amini R, Sarabi AA (2011) The corrosion properties of phosphate coating on AZ31 magnesium alloy: the effect of sodium dodecyl sulfate (SDS) as an eco-friendly accelerating agent. Appl Surf Sci 257: 7134–7139. doi: 10.1016/j.apsusc.2011.03.072
![]() |
[108] |
Wang C, Zhu S, Jiang F, et al. (2009) Cerium conversion coatings for AZ91D magnesium alloy in ethanol solution and its corrosion resistance. Corros Sci 51: 2916–2923. doi: 10.1016/j.corsci.2009.08.003
![]() |
[109] |
Hsiao HY, Tsai WT (2005) Characterization of anodic films formed on AZ91D magnesium alloy. Surf Coat Tech 190: 299–308. doi: 10.1016/j.surfcoat.2004.03.010
![]() |
[110] |
Zhang Y, Yan C, Wang F, et al. (2002) Study on the environmentally friendly anodizing of AZ91D magnesium alloy. Surf Coat Tech 161: 36–43. doi: 10.1016/S0257-8972(02)00342-0
![]() |
[111] |
Wu H, Cheng Y, Li L, et al. (2007) The anodization of ZK60 magnesium alloy in alkaline solution containing silicate and the corrosion properties of the anodized films. Appl Surf Sci 253: 9387–9394. doi: 10.1016/j.apsusc.2007.05.085
![]() |
[112] | Su Y, Li G, Lian J (2012) A chemical conversion hydroxyapatite coating on AZ60 magnesium alloy and its electrochemical corrosion behaviour. Int J Electrochem Sci 7: 11497–11511. |
[113] |
Guo X, Du K, Wang Y, et al. (2012) A new nanoparticle penetrant used for plasma electrolytic oxidation film coated on AZ31 Mg alloy in service environment. Surf Coat Tech 206: 4833–4839. doi: 10.1016/j.surfcoat.2012.05.063
![]() |
[114] |
Sun RX, Wang PF, Zhao DD, et al. (2015) An environment‐friendly calcium phosphate conversion coating on AZ91D alloy and its corrosion resistance. Mater Corros 66: 383–386. doi: 10.1002/maco.201307424
![]() |
[115] |
Mu S, Du J, Jiang H, et al. (2014) Composition analysis and corrosion performance of a Mo–Ce conversion coating on AZ91 magnesium alloy. Surf Coat Tech 254: 364–370. doi: 10.1016/j.surfcoat.2014.06.044
![]() |
[116] |
Hu J, Li Q, Zhong X, et al. (2009) Composite anticorrosion coatings for AZ91D magnesium alloy with molybdate conversion coating and silicon sol–gel coatings. Prog Org Coat 66: 199–205. doi: 10.1016/j.porgcoat.2009.07.003
![]() |
[117] |
Seifzadeh D, Rajabalizadeh Z (2013) Environmentally-friendly method for electroless Ni–P plating on magnesium alloy. Surf Coat Tech 218: 119–126. doi: 10.1016/j.surfcoat.2012.12.039
![]() |
[118] |
Wang L, Zhang K, Sun W, et al. (2013) Hydrothermal synthesis of corrosion resistant hydrotalcite conversion coating on AZ91D alloy. Mater Lett 106: 111–114. doi: 10.1016/j.matlet.2013.05.018
![]() |
[119] |
Gao HF, Tan HQ, Li J, et al. (2012) Synergistic effect of cerium conversion coating and phytic acid conversion coating on AZ31B magnesium alloy. Surf Coat Tech 212: 32–36. doi: 10.1016/j.surfcoat.2012.09.008
![]() |
[120] |
Sun J, Wang G (2014) Preparation and corrosion resistance of cerium conversion coatings on AZ91D magnesium alloy by a cathodic electrochemical treatment. Surf Coat Tech 254: 42–48. doi: 10.1016/j.surfcoat.2014.05.054
![]() |
[121] |
Sun J, Wang G (2015) Preparation and characterization of a cerium conversion film on magnesium alloy. Anti-Corros Method M 62: 253–258. doi: 10.1108/ACMM-12-2013-1336
![]() |
[122] |
Pan SJ, Tsai WT, Kuo JC, et al. (2013) Material characteristics and corrosion performance of heat-treated Al-Zn coatings electrodeposited on AZ91D magnesium alloy from an ionic liquid. J Electrochem Soc 160: D320–D325. doi: 10.1149/2.100308jes
![]() |
[123] |
Tao Y, Xiong T, Sun C, et al. (2010) Microstructure and corrosion performance of a cold sprayed aluminium coating on AZ91D magnesium alloy. Corros Sci 52: 3191–3197. doi: 10.1016/j.corsci.2010.05.023
![]() |
[124] |
Krishna LR, Poshal G, Jyothirmayi A, et al. (2013) Compositionally modulated CGDS + MAO duplex coatings for corrosion protection of AZ91 magnesium alloy. J Alloy Compd 578: 355–361. doi: 10.1016/j.jallcom.2013.06.036
![]() |
[125] |
Tao Y, Xiong T, Sun C, et al. (2009) Effect of α-Al2O3 on the properties of cold sprayed Al/α-Al2O3 composite coatings on AZ91D magnesium alloy. Appl Surf Sci 256: 261–266. doi: 10.1016/j.apsusc.2009.08.012
![]() |
[126] |
Lei XP, Yu G, Zhu YP, et al. (2010) Successful cyanide free plating protocols on magnesium alloys. T IMF 88: 75–80. doi: 10.1179/174591910X12646055765330
![]() |
[127] |
Chen F, Zhou H, Yao B, et al. (2007) Corrosion resistance property of the ceramic coating obtained through microarc oxidation on the AZ31 magnesium alloy surfaces. Surf Coat Tech 201: 4905–4908. doi: 10.1016/j.surfcoat.2006.07.079
![]() |
[128] |
Zhao H, Huang Z, Cui J (2007) A new method for electroless Ni–P plating on AZ31 magnesium alloy. Surf Coat Tech 202: 133–139. doi: 10.1016/j.surfcoat.2007.05.001
![]() |
[129] |
Liu Q, Chen D, Kang Z (2015) One-step electrodeposition process to fabricate corrosion-resistant superhydrophobic surface on magnesium alloy. ACS Appl Mater Inter 7: 1859–1867. doi: 10.1021/am507586u
![]() |
[130] |
Zhang J, Wu C (2010) Corrosion and Protection of Magnesium Alloys-A Review of the Patent Literature. Recent Pat Corros Sci 2: 55–68. doi: 10.2174/1877610801002010055
![]() |
[131] | Forsmark JH, Li M, Su X, et al. (2014) The USAMP Magnesium Front End Research and Development Project-Results of the Magnesium "Demonstration" Structure, In: Magnesium Technology, Springer, Cham, 517–524. |
[132] |
Montemor MF (2014) Functional and smart coatings for corrosion protection: a review of recent advances. Surf Coat Tech 258: 17–37. doi: 10.1016/j.surfcoat.2014.06.031
![]() |
[133] |
Jiang C, Cao Y, Xiao G, et al. (2017) A review on the application of inorganic nanoparticles in chemical surface coatings on metallic substrates. RSC Adv 7: 7531–7539. doi: 10.1039/C6RA25841G
![]() |
[134] |
Unigovski Y, Eliezer A, Abramov E, et al. (2003) Corrosion fatigue of extruded magnesium alloys. Mat Sci Eng A-Struct 360: 132–139. doi: 10.1016/S0921-5093(03)00409-X
![]() |
[135] |
Nan ZY, Ishihara S, Goshima T (2008) Corrosion fatigue behavior of extruded magnesium alloy AZ31 in sodium chloride solution. Int J Fatigue 30: 1181–1188. doi: 10.1016/j.ijfatigue.2007.09.005
![]() |
[136] |
Bhuiyan MS, Mutoh Y, Murai T, et al. (2010) Corrosion fatigue behavior of extruded magnesium alloy AZ80-T5 in a 5% NaCl environment. Eng Fract Mech 77: 1567–1576. doi: 10.1016/j.engfracmech.2010.03.032
![]() |
[137] |
Chamos AN, Pantelakis SG, Spiliadis V (2010) Fatigue behavior of bare and pre-corroded magnesium alloy AZ31. Mater Design 31: 4130–4137. doi: 10.1016/j.matdes.2010.04.031
![]() |
[138] |
He XL, Wei YH, Hou LF, et al. (2014) Investigation on corrosion fatigue property of epoxy coated AZ31 magnesium alloy in sodium sulfate solution. Theor Appl Fract Mec 70: 39–48. doi: 10.1016/j.tafmec.2014.03.002
![]() |
[139] |
He XL, Wei YH, Hou LF, et al. (2014) Corrosion fatigue behavior of epoxy-coated Mg–3Al–1Zn alloy in NaCl solution. Rare Metals 33: 276–286. doi: 10.1007/s12598-014-0278-3
![]() |
[140] |
He XL, Wei YH, Hou LF, et al. (2014) Corrosion fatigue behavior of epoxy-coated Mg–3Al–1Zn alloy in gear oil. T Nonferr Metal Soc 24: 3429–3440. doi: 10.1016/S1003-6326(14)63486-5
![]() |
[141] |
Uematsu Y, Kakiuchi T, Teratani T, et al. (2011) Improvement of corrosion fatigue strength of magnesium alloy by multilayer diamond-like carbon coatings. Surf Coat Tech 205: 2778–2784. doi: 10.1016/j.surfcoat.2010.10.040
![]() |
[142] |
Dayani SB, Shaha SK, Ghelichi R, et al. (2018) The impact of AA7075 cold spray coating on the fatigue life of AZ31B cast alloy. Surf Coat Tech 337: 150–158. doi: 10.1016/j.surfcoat.2018.01.008
![]() |
[143] | Borhan Dayani S (2017) Improvement of fatigue and corrosion-fatigue resistance of AZ31B cast alloy by cold spray coating and top coating [Master thesis]. University of Waterloo. |
[144] |
Ishihara S, Masuda K, Namito T, et al. (2014) On corrosion fatigue strength of the anodized and painted Mg alloy. Int J Fatigue 66: 252–258. doi: 10.1016/j.ijfatigue.2014.03.007
![]() |
[145] |
Khan SA, Miyashita Y, Mutoh Y, et al. (2008) Fatigue behavior of anodized AM60 magnesium alloy under humid environment. Mat Sci Eng A-Struct 498: 377–383. doi: 10.1016/j.msea.2008.08.015
![]() |
[146] |
Khan SA, Miyashita Y, Mutoh Y (2015) Corrosion fatigue behavior of AM60 magnesium alloy with anodizing layer and chemical‐conversion‐coating layer. Mater Corros 66: 940–948. doi: 10.1002/maco.201407946
![]() |
[147] |
Bhuiyan MS, Mutoh Y (2011) Corrosion fatigue behavior of conversion coated and painted AZ61 magnesium alloy. Int J Fatigue 33: 1548–1556. doi: 10.1016/j.ijfatigue.2011.06.011
![]() |
[148] | Ishihara S, Notoya H, Namito T (2011) Improvement in Corrosion Fatigue Resistance of Mg Alloy due to Plating, In: Magnesium Alloys-Corrosion and Surface Treatments, IntechOpen. |
[149] | Yerokhin AL, Shatrov A, Samsonov V, et al. (2004) Fatigue properties of Keronite® coatings on a magnesium alloy. Surf Coat Tech 182: 78–84. |
[150] |
Bhuiyan MS, Ostuka Y, Mutoh Y, et al. (2010) Corrosion fatigue behavior of conversion coated AZ61 magnesium alloy. Mat Sci Eng A-Struct 527: 4978–4984. doi: 10.1016/j.msea.2010.04.059
![]() |
[151] |
Khan SA, Bhuiyan MS, Miyashita Y, et al. (2011) Corrosion fatigue behavior of die-cast and shot-blasted AM60 magnesium alloy. Mat Sci Eng A-Struct 528: 1961–1966. doi: 10.1016/j.msea.2010.11.033
![]() |
[152] | Shaha SK, Dayani SB, Jahed H (2018) Influence of Cold Spray on the Enhancement of Corrosion Fatigue of the AZ31B Cast Mg Alloy, In: TMS Annual Meeting & Exhibition, Springer, Cham, 541–550. |
[153] |
Diab M, Pang X, Jahed H (2017) The effect of pure aluminum cold spray coating on corrosion and corrosion fatigue of magnesium (3% Al-1% Zn) extrusion. Surf Coat Tech 309: 423–435. doi: 10.1016/j.surfcoat.2016.11.014
![]() |
[154] |
Němcová A, Skeldon P, Thompson GE, et al. (2014) Influence of plasma electrolytic oxidation on fatigue performance of AZ61 magnesium alloy. Corros Sci 82: 58–66. doi: 10.1016/j.corsci.2013.12.019
![]() |
[155] |
Klein M, Lu X, Blawert C, et al. (2017) Influence of plasma electrolytic oxidation coatings on fatigue performance of AZ31 Mg alloy. Mater Corros 68: 50–57. doi: 10.1002/maco.201609088
![]() |
[156] |
Okada H, Uematsu Y, Tokaji K (2010) Fatigue behaviour in AZ80A magnesium alloy with DLC/thermally splayed WC-12Co hybrid coating. Procedia Eng 2: 283–290. doi: 10.1016/j.proeng.2010.03.031
![]() |
[157] |
Ceschini L, Morri A, Angelini V, et al. (2017) Fatigue behavior of the rare earth rich EV31A Mg alloy: influence of plasma electrolytic oxidation. Metals 7: 212. doi: 10.3390/met7060212
![]() |
[158] |
Huang CA, Chuang CH, Yeh YH, et al. (2016) Low-cycle fatigue fracture behavior of a Mg alloy (AZ61) after alkaline Cu, alkaline followed by acidic Cu, Ni/Cu, and Cr-C/Cu electroplating. Mat Sci Eng A-Struct 662: 111–119. doi: 10.1016/j.msea.2016.03.064
![]() |
[159] | Chen YL, Zhang Y, Li Y, et al. (2011) Influences of micro-arc oxidation on pre-corroded fatigue property of magnesium alloy AZ91D. Adv Mater Res 152: 51–57. |
[160] |
Wang BJ, Wang SD, Xu DK, et al. (2017) Recent progress in fatigue behavior of Mg alloys in air and aqueous media: A review. J Mater Sci Technol 33: 1075–1086. doi: 10.1016/j.jmst.2017.07.017
![]() |
[161] |
LeBozec N, Blandin N, Thierry D (2008) Accelerated corrosion tests in the automotive industry: a comparison of the performance towards cosmetic corrosion. Mater Corros 59: 889–894. doi: 10.1002/maco.200804168
![]() |
[162] | ASTM B117 (1997) Standard Practice for Operating Salt Spray (Fog) Apparatus. ASTM International. |
[163] | ASTM D870 (2009) Standard Practice for Testing Water Resistance of Coatings Using Water Immersion. ASTM International. |
[164] | ASTM D (2002) Standard practice for testing water resistance of coatings in 100% relative humidity. |
[165] |
Liu M, Uggowitzer PJ, Nagasekhar AV, et al. (2009) Calculated phase diagrams and the corrosion of die-cast Mg–Al alloys. Corros Sci 51: 602–619. doi: 10.1016/j.corsci.2008.12.015
![]() |
[166] | VDA 233-102 Cyclic corrosion testing of materials and components in automotive construction. ascot, 2018. Available from: http://www.vda233-102.com/. |
[167] | Townsend HE, McCune DC (1997) Round-Robin Evaluation of a New Standard Laboratory Test for Cosmetic Corrosion. SAE Trans 106: 1249–1262. |
[168] |
Bovard FS, Smith KA, Courval GJ, et al. (2010) Cosmetic Corrosion Test for Aluminum Autobody Panels. SAE Intl J Passeng Cars-Mech Syst 3: 544–553. doi: 10.4271/2010-01-0726
![]() |
[169] | Weiler JP, Wang G, Berkmortel R (2018) Assessment of OEM Corrosion Test Protocols for Magnesium Substrates. SAE Technical Paper 2018-01-0103. |
[170] | Standard Corrosion Tests, 2018. Available from: https://www.ascott-analytical.com/test_standard/. |
[171] |
LeBozec N, Blandin N, Thierry D (2008) Accelerated corrosion tests in the automotive industry: a comparison of the performance towards cosmetic corrosion. Mater Corros 59: 889–894. doi: 10.1002/maco.200804168
![]() |
[172] | SAE J2334 (2003) Laboratory Cyclic Corrosion Test. |
[173] | Cyclic Corrosion Test. Quebec (CA): Micom Laboratories Inc., 2018. Available from: https://www.micomlab.com/micom-testing/cyclic-corrosion-testing/. |
[174] |
Micone N, De Waele W (2017) Evaluation of Methodologies to Accelerate Corrosion Assisted Fatigue Experiments. Exp Mech 57: 547–557. doi: 10.1007/s11340-016-0241-3
![]() |
[175] |
LeBozec N, Thierry D (2015) A new device for simultaneous corrosion fatigue testing of joined materials in accelerated corrosion tests. Mater Corros 66: 893–898. doi: 10.1002/maco.201407984
![]() |
1. | K Ganpati Shrinivas Sharma, Surekha Bhusnur, 2023, Data Ensemble Model for Prediction of Oxygen Content in Gas fired Boiler for Efficient Combustion, 979-8-3503-9874-8, 1, 10.1109/SCEECS57921.2023.10062991 | |
2. | Zheng Li, Min Yao, Zhenmin Luo, Qianrui Huang, Tongshuang Liu, Ultra-early prediction of the process parameters of coal chemical production, 2024, 10, 24058440, e30821, 10.1016/j.heliyon.2024.e30821 | |
3. | Rui Tan, Zhaocai Wang, Tunhua Wu, Junhao Wu, A data-driven model for water quality prediction in Tai Lake, China, using secondary modal decomposition with multidimensional external features, 2023, 47, 22145818, 101435, 10.1016/j.ejrh.2023.101435 | |
4. | Mingyan Wang, Qing Xu, Yingying Cao, Shahbaz Gul Hassan, Wenjun Liu, Min He, Tonglai Liu, Longqin Xu, Liang Cao, Shuangyin Liu, Huilin Wu, An Ensemble Model for Water Temperature Prediction in Intensive Aquaculture, 2023, 11, 2169-3536, 137285, 10.1109/ACCESS.2023.3339190 | |
5. | Wenhao Li, Yin Zhao, Yining Zhu, Zhongtian Dong, Fenghe Wang, Fengliang Huang, Research progress in water quality prediction based on deep learning technology: a review, 2024, 31, 1614-7499, 26415, 10.1007/s11356-024-33058-7 | |
6. | Yane Li, Lijun Guo, Jiyang Wang, Yiwei Wang, Dayu Xu, Jun Wen, An Improved Sap Flow Prediction Model Based on CNN-GRU-BiLSTM and Factor Analysis of Historical Environmental Variables, 2023, 14, 1999-4907, 1310, 10.3390/f14071310 | |
7. | Itunu C. Adedeji, Ebrahim Ahmadisharaf, Clayton J. Clark, A unified subregional framework for modeling stream water quality across watersheds of a hydrologic subregion, 2025, 958, 00489697, 177870, 10.1016/j.scitotenv.2024.177870 | |
8. | Sirisak Pangvuthivanich, Wirachai Roynarin, Promphak Boonraksa, Terapong Boonraksa, Deep Learning‐Driven Forecasting for Compressed Air Oxygenation Integrating With Floating PV Power Generation System, 2025, 7, 2516-8401, 10.1049/esi2.70000 | |
9. | Kaixuan Shao, Daoliang Li, Hao Tang, Yonghui Zhang, Bo Xu, Uzair Aslam Bhatti, Improving multi-step dissolved oxygen prediction in aquaculture using adaptive temporal convolution and optimized transformer, 2025, 235, 01681699, 110329, 10.1016/j.compag.2025.110329 |
Hyperparameter | Value |
Filter size of CNN | [128, 64] |
Kernel size of CNN | 1 |
Padding | same |
Activation function | Relu |
Unit number for BiLSTM | 128 |
Optimization function | adam |
Learning-rate | 0.001 |
Batch size | 64 |
Epoch number | 100 |
Cases | Datasets | Numbers | Statistical indicators | |||||
Mean | Std. | Max. | Min. | Kurtosis | Skewness | |||
Dataset 1 | All Samples | 20851 | 8.09 | 1.20 | 10.25 | 5.02 | -0.64 | -0.62 |
Training Set | 16681 | 8.57 | 0.77 | 10.25 | 6.37 | -0.37 | -0.47 | |
Validation Set | 2085 | 6.54 | 0.25 | 7.09 | 5.84 | -0.53 | -0.04 | |
Testing Set | 2085 | 5.81 | 0.35 | 6.44 | 5.02 | -0.88 | -0.21 | |
Dataset 2 | All Samples | 21673 | 7.28 | 1.35 | 10.32 | 4.12 | -0.99 | 0.46 |
Training Set | 17339 | 6.75 | 0.94 | 9.08 | 4.12 | -0.69 | 0.48 | |
Validation Set | 2167 | 8.99 | 0.24 | 9.71 | 8.49 | -0.03 | 0.97 | |
Testing Set | 2167 | 9.70 | 0.22 | 10.32 | 9.32 | -1.47 | 0.19 |
Methods | 3 steps | 6 steps | ||||||
MAE | RMSE | MAPE | R2 | MAE | RMSE | MAPE | R2 | |
SVR | 0.0514 | 0.0599 | 0.0091 | 0.9711 | 0.1238 | 0.1333 | 0.0219 | 0.8565 |
RNN | 0.0462 | 0.0573 | 0.0079 | 0.9736 | 0.0555 | 0.0638 | 0.0096 | 0.9671 |
LSTM | 0.0398 | 0.0518 | 0.0069 | 0.9799 | 0.0552 | 0.0627 | 0.0094 | 0.9683 |
CNN-LSTM | 0.0309 | 0.0394 | 0.0053 | 0.9875 | 0.0452 | 0.0575 | 0.0078 | 0.9733 |
CNN-BiLSTM | 0.0231 | 0.0342 | 0.0041 | 0.9907 | 0.0303 | 0.0429 | 0.0053 | 0.9851 |
Proposed | 0.0135 | 0.0187 | 0.0023 | 0.9971 | 0.0202 | 0.0293 | 0.0034 | 0.9931 |
Methods | 36 steps | 72 steps | ||||||
MAE | RMSE | MAPE | R2 | MAE | RMSE | MAPE | R2 | |
SVR | 0.1352 | 0.1490 | 0.0240 | 0.8142 | 0.1365 | 0.1713 | 0.0247 | 0.7577 |
RNN | 0.0794 | 0.0923 | 0.0135 | 0.9287 | 0.0881 | 0.1063 | 0.0154 | 0.9067 |
LSTM | 0.0633 | 0.0765 | 0.0109 | 0.9513 | 0.0675 | 0.0797 | 0.0115 | 0.9476 |
CNN-LSTM | 0.0448 | 0.0599 | 0.0078 | 0.9756 | 0.0479 | 0.0601 | 0.0082 | 0.9702 |
CNN-BiLSTM | 0.0347 | 0.0451 | 0.0062 | 0.9835 | 0.0452 | 0.0584 | 0.0079 | 0.9719 |
Proposed | 0.0156 | 0.0219 | 0.0027 | 0.9968 | 0.0283 | 0.0381 | 0.0049 | 0.9883 |
Methods | 3 steps | 6 steps | ||||||
MAE | RMSE | MAPE | R2 | MAE | RMSE | MAPE | R2 | |
SVR | 0.0431 | 0.0514 | 0.0043 | 0.9473 | 0.0518 | 0.0607 | 0.0053 | 0.9264 |
RNN | 0.0273 | 0.0368 | 0.0028 | 0.9729 | 0.0393 | 0.0502 | 0.0040 | 0.9497 |
LSTM | 0.0208 | 0.0321 | 0.0021 | 0.9793 | 0.0334 | 0.0452 | 0.0034 | 0.9592 |
CNN-LSTM | 0.0257 | 0.0323 | 0.0027 | 0.9791 | 0.0329 | 0.0413 | 0.0034 | 0.9659 |
CNN-BiLSTM | 0.0253 | 0.0314 | 0.0026 | 0.9803 | 0.0272 | 0.0352 | 0.0028 | 0.9753 |
Proposed | 0.0189 | 0.0257 | 0.0019 | 0.9869 | 0.0201 | 0.0266 | 0.0021 | 0.9858 |
Methods | 36 steps | 72 steps | ||||||
MAE | RMSE | MAPE | R2 | MAE | RMSE | MAPE | R2 | |
SVR | 0.0849 | 0.0971 | 0.0086 | 0.8126 | 0.1013 | 0.0118 | 0.0104 | 0.7519 |
RNN | 0.0436 | 0.0555 | 0.0045 | 0.9395 | 0.0495 | 0.0642 | 0.0051 | 0.9184 |
LSTM | 0.0409 | 0.0499 | 0.0042 | 0.9505 | 0.0420 | 0.0532 | 0.0043 | 0.9443 |
CNN-LSTM | 0.0394 | 0.0494 | 0.0041 | 0.9516 | 0.0422 | 0.0504 | 0.0044 | 0.9497 |
CNN-BiLSTM | 0.0332 | 0.0402 | 0.0034 | 0.9683 | 0.0352 | 0.0446 | 0.0036 | 0.9606 |
Proposed | 0.0221 | 0.0298 | 0.0023 | 0.9824 | 0.0276 | 0.0372 | 0.0028 | 0.9726 |
Hyperparameter | Value |
Filter size of CNN | [128, 64] |
Kernel size of CNN | 1 |
Padding | same |
Activation function | Relu |
Unit number for BiLSTM | 128 |
Optimization function | adam |
Learning-rate | 0.001 |
Batch size | 64 |
Epoch number | 100 |
Cases | Datasets | Numbers | Statistical indicators | |||||
Mean | Std. | Max. | Min. | Kurtosis | Skewness | |||
Dataset 1 | All Samples | 20851 | 8.09 | 1.20 | 10.25 | 5.02 | -0.64 | -0.62 |
Training Set | 16681 | 8.57 | 0.77 | 10.25 | 6.37 | -0.37 | -0.47 | |
Validation Set | 2085 | 6.54 | 0.25 | 7.09 | 5.84 | -0.53 | -0.04 | |
Testing Set | 2085 | 5.81 | 0.35 | 6.44 | 5.02 | -0.88 | -0.21 | |
Dataset 2 | All Samples | 21673 | 7.28 | 1.35 | 10.32 | 4.12 | -0.99 | 0.46 |
Training Set | 17339 | 6.75 | 0.94 | 9.08 | 4.12 | -0.69 | 0.48 | |
Validation Set | 2167 | 8.99 | 0.24 | 9.71 | 8.49 | -0.03 | 0.97 | |
Testing Set | 2167 | 9.70 | 0.22 | 10.32 | 9.32 | -1.47 | 0.19 |
Methods | 3 steps | 6 steps | ||||||
MAE | RMSE | MAPE | R2 | MAE | RMSE | MAPE | R2 | |
SVR | 0.0514 | 0.0599 | 0.0091 | 0.9711 | 0.1238 | 0.1333 | 0.0219 | 0.8565 |
RNN | 0.0462 | 0.0573 | 0.0079 | 0.9736 | 0.0555 | 0.0638 | 0.0096 | 0.9671 |
LSTM | 0.0398 | 0.0518 | 0.0069 | 0.9799 | 0.0552 | 0.0627 | 0.0094 | 0.9683 |
CNN-LSTM | 0.0309 | 0.0394 | 0.0053 | 0.9875 | 0.0452 | 0.0575 | 0.0078 | 0.9733 |
CNN-BiLSTM | 0.0231 | 0.0342 | 0.0041 | 0.9907 | 0.0303 | 0.0429 | 0.0053 | 0.9851 |
Proposed | 0.0135 | 0.0187 | 0.0023 | 0.9971 | 0.0202 | 0.0293 | 0.0034 | 0.9931 |
Methods | 36 steps | 72 steps | ||||||
MAE | RMSE | MAPE | R2 | MAE | RMSE | MAPE | R2 | |
SVR | 0.1352 | 0.1490 | 0.0240 | 0.8142 | 0.1365 | 0.1713 | 0.0247 | 0.7577 |
RNN | 0.0794 | 0.0923 | 0.0135 | 0.9287 | 0.0881 | 0.1063 | 0.0154 | 0.9067 |
LSTM | 0.0633 | 0.0765 | 0.0109 | 0.9513 | 0.0675 | 0.0797 | 0.0115 | 0.9476 |
CNN-LSTM | 0.0448 | 0.0599 | 0.0078 | 0.9756 | 0.0479 | 0.0601 | 0.0082 | 0.9702 |
CNN-BiLSTM | 0.0347 | 0.0451 | 0.0062 | 0.9835 | 0.0452 | 0.0584 | 0.0079 | 0.9719 |
Proposed | 0.0156 | 0.0219 | 0.0027 | 0.9968 | 0.0283 | 0.0381 | 0.0049 | 0.9883 |
Methods | 3 steps | 6 steps | ||||||
MAE | RMSE | MAPE | R2 | MAE | RMSE | MAPE | R2 | |
SVR | 0.0431 | 0.0514 | 0.0043 | 0.9473 | 0.0518 | 0.0607 | 0.0053 | 0.9264 |
RNN | 0.0273 | 0.0368 | 0.0028 | 0.9729 | 0.0393 | 0.0502 | 0.0040 | 0.9497 |
LSTM | 0.0208 | 0.0321 | 0.0021 | 0.9793 | 0.0334 | 0.0452 | 0.0034 | 0.9592 |
CNN-LSTM | 0.0257 | 0.0323 | 0.0027 | 0.9791 | 0.0329 | 0.0413 | 0.0034 | 0.9659 |
CNN-BiLSTM | 0.0253 | 0.0314 | 0.0026 | 0.9803 | 0.0272 | 0.0352 | 0.0028 | 0.9753 |
Proposed | 0.0189 | 0.0257 | 0.0019 | 0.9869 | 0.0201 | 0.0266 | 0.0021 | 0.9858 |
Methods | 36 steps | 72 steps | ||||||
MAE | RMSE | MAPE | R2 | MAE | RMSE | MAPE | R2 | |
SVR | 0.0849 | 0.0971 | 0.0086 | 0.8126 | 0.1013 | 0.0118 | 0.0104 | 0.7519 |
RNN | 0.0436 | 0.0555 | 0.0045 | 0.9395 | 0.0495 | 0.0642 | 0.0051 | 0.9184 |
LSTM | 0.0409 | 0.0499 | 0.0042 | 0.9505 | 0.0420 | 0.0532 | 0.0043 | 0.9443 |
CNN-LSTM | 0.0394 | 0.0494 | 0.0041 | 0.9516 | 0.0422 | 0.0504 | 0.0044 | 0.9497 |
CNN-BiLSTM | 0.0332 | 0.0402 | 0.0034 | 0.9683 | 0.0352 | 0.0446 | 0.0036 | 0.9606 |
Proposed | 0.0221 | 0.0298 | 0.0023 | 0.9824 | 0.0276 | 0.0372 | 0.0028 | 0.9726 |