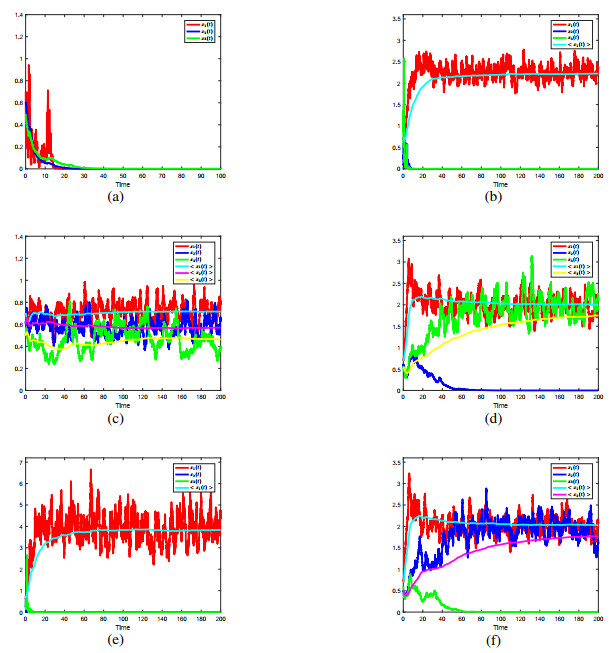
In this paper, well-posedness and asymptotic behaviors of a stochastic two-predator one-prey system with S-type distributed time delays are studied by using stochastic analytical techniques. First, the existence and uniqueness of global positive solution with positive initial condition is proved. Second, sufficient conditions for persistence in mean and extinction of each species are obtained. Then, sufficient conditions for global attractivity are established. Finally, some numerical simulations are provided to support the analytical results.
Citation: Zeyan Yue, Lijuan Dong, Sheng Wang. Stochastic persistence and global attractivity of a two-predator one-prey system with S-type distributed time delays[J]. Mathematical Modelling and Control, 2022, 2(4): 272-281. doi: 10.3934/mmc.2022026
[1] | Sheng Wang, Baoli Lei . Dynamics of a stochastic hybrid delay one-predator-two-prey model with harvesting and jumps in a polluted environment. Mathematical Modelling and Control, 2025, 5(1): 85-102. doi: 10.3934/mmc.2025007 |
[2] | Tengda Wei, Xiang Xie, Xiaodi Li . Persistence and periodicity of survival red blood cells model with time-varying delays and impulses. Mathematical Modelling and Control, 2021, 1(1): 12-25. doi: 10.3934/mmc.2021002 |
[3] | Tomás Caraballo, Javier López-de-la-Cruz . Survey on chemostat models with bounded random input flow. Mathematical Modelling and Control, 2021, 1(1): 52-78. doi: 10.3934/mmc.2021005 |
[4] | Qin Xu, Xiao Wang, Yicheng Liu . Emergent behavior of Cucker–Smale model with time-varying topological structures and reaction-type delays. Mathematical Modelling and Control, 2022, 2(4): 200-218. doi: 10.3934/mmc.2022020 |
[5] | Shaosan Xia, Xianyi Li . Complicate dynamics of a discrete predator-prey model with double Allee effect. Mathematical Modelling and Control, 2022, 2(4): 282-295. doi: 10.3934/mmc.2022027 |
[6] | Hongwei Zheng, Yujuan Tian . Exponential stability of time-delay systems with highly nonlinear impulses involving delays. Mathematical Modelling and Control, 2025, 5(1): 103-120. doi: 10.3934/mmc.2025008 |
[7] | Xianyi Li, Luyao Lv . Global attractivity of a rational difference equation with higher order and its applications. Mathematical Modelling and Control, 2024, 4(3): 260-272. doi: 10.3934/mmc.2024021 |
[8] | Yanchao He, Yuzhen Bai . Finite-time stability and applications of positive switched linear delayed impulsive systems. Mathematical Modelling and Control, 2024, 4(2): 178-194. doi: 10.3934/mmc.2024016 |
[9] | Xingwen Liu, Shouming Zhong . Stability analysis of delayed switched cascade nonlinear systems with uniform switching signals. Mathematical Modelling and Control, 2021, 1(2): 90-101. doi: 10.3934/mmc.2021007 |
[10] | Bangxin Jiang, Yijun Lou, Jianquan Lu . Input-to-state stability of delayed systems with bounded-delay impulses. Mathematical Modelling and Control, 2022, 2(2): 44-54. doi: 10.3934/mmc.2022006 |
In this paper, well-posedness and asymptotic behaviors of a stochastic two-predator one-prey system with S-type distributed time delays are studied by using stochastic analytical techniques. First, the existence and uniqueness of global positive solution with positive initial condition is proved. Second, sufficient conditions for persistence in mean and extinction of each species are obtained. Then, sufficient conditions for global attractivity are established. Finally, some numerical simulations are provided to support the analytical results.
In 1977 and 1984, Freedman and Waltman ([1,2]) studied the following two-predator one-prey system:
{dx1(t)dt=x1(t)[r1−a11x1(t)−a12x2(t)−a13x3(t)],dx2(t)dt=x2(t)[−r2+a21x1(t)−a22x2(t)−a23x3(t)],dx3(t)dt=x3(t)[−r3+a31x1(t)−a32x2(t)−a33x3(t)], | (1.1) |
where xi(t) stands for the size of the ith population and all the parameters are positive constants.
However, the deterministic system has its limitation in mathematical modeling of ecosystems since the parameters involved in the system are unable to capture the influence of environmental noises [3,4,5]. Therefore, it is of enormous importance to study the effects of environmental noises on the dynamics of population systems. Assume that the parameters ri are affected by white noises, i.e., r1↪r1+σ1˙B1(t), −r2↪−r2+σ2˙B2(t), −r3↪−r3+σ3˙B3(t), where Bi(t) are mutually independent standard Wiener processes defined on a complete probability space (Ω,F,P) with a filtration {Ft}t≥0 satisfying the usual conditions. Then, the stochastic two-predator one-prey system with white noises can be expressed as follows:
{dx1(t)=x1(t)[r1−a11x1(t)−a12x2(t)−a13x3(t)]dt+σ1x1(t)dB1(t),dx2(t)=x2(t)[−r2+a21x1(t)−a22x2(t)−a23x3(t)]dt+σ2x2(t)dB2(t),dx3(t)=x3(t)[−r3+a31x1(t)−a32x2(t)−a33x3(t)]dt+σ3x3(t)dB3(t). | (1.2) |
On the other hand, "all species should exhibit time delay" in the real world, and incorporating time delays in biological systems makes the systems much more realistic than those without time delays ([6,7,8,9,10]). Hence, in this paper we concern the dynamics of the following stochastic two-predator one-prey system with S-type distributed time delays:
{dx1(t)=x1(t)[r1−D11(x1)(t)−D12(x2)(t)−D13(x3)(t)]dt+σ1x1(t)dB1(t),dx2(t)=x2(t)[−r2+D21(x1)(t)−D22(x2)(t)−D23(x3)(t)]dt+σ2x2(t)dB2(t),dx3(t)=x3(t)[−r3+D31(x1)(t)−D32(x2)(t)−D33(x3)(t)]dt+σ3x3(t)dB3(t), | (1.3) |
where Dji(xi)(t)=ajixi(t)+∫0−τjixi(t+θ)dμji(θ), ∫0−τjixi(t+θ)dμji(θ) are Lebesgue-Stieltjes integrals, τji>0 are time delays, μji(θ) are nondecreasing bounded variation functions defined on [−τ,0], τ=maxi,j=1,2,3{τji}.
Denote Aij=aij+∫0−τijdμij(θ), D1=r1−σ212, Di=ri+σ2i2 (i=2,3) and
{Θ=|A11A12A13−A21A22A23−A31A32A33|,Θ1=|D1A12A13−D2A22A23−D3A32A33|,Θ2=|A11D1A13−A21−D2A23−A31−D3A33|,Θ3=|A11A12D1−A21A22−D2−A31A32−D3|. |
Assume that Θ>0. For the matrix corresponding to Θ (respectively, Θk), denote by MΘij (respectively, MΘkij) the complement minor of the element at the i-th row and the j-th column (i,j,k=1,2,3).
Theorem 2.1. For any (ξ1,ξ2,ξ3)T∈C([−τ,0],R3+), system (1.3) has a unique global solution (x1(t),x2(t),x3(t))T∈R3+ on t∈[0,+∞) a.s. Moreover, for any constant p>0, there are Ki(p)>0 such that
supt≥0E[xpi(t)]≤Ki(p)(i=1,2,3). | (2.1) |
Proof. The proof is rather standard and here is omitted (see, e.g., [11] and [12]).
Lemma 2.1. ([13]) Suppose Z(t)∈C(Ω×[0,+∞),R+) and limt→+∞o(t)t=0.
(i) If there exists constant δ0>0 such that for t≫1,
lnZ(t)≤δt−δ0∫t0Z(s)ds+o(t), | (2.2) |
then
{lim supt→+∞t−1∫t0Z(s)ds≤δδ0a.s.(δ≥0);limt→+∞Z(t)=0a.s.(δ<0). | (2.3) |
(ii) If there exist constants δ>0 and δ0>0 such that for t≫1,
lnZ(t)≥δt−δ0∫t0Z(s)ds+o(t), | (2.4) |
then
lim inft→+∞t−1∫t0Z(s)ds≥δδ0a.s. | (2.5) |
Lemma 2.2. Consider the following auxiliary system:
{dX1(t)=X1(t)[r1−D11(X1)(t)]dt+σ1X1(t)dB1(t),dXi(t)=Xi(t)[−ri+Di1(X1)(t)−Dii(Xi)(t)]dt+σiXi(t)dBi(t),(i=2,3). | (2.6) |
(a) If D1<0, then limt→+∞Xi(t)=0 a.s. (i=1,2,3).
(b) If D1≥0, −Di+Ai1D1A11<0, then
limt→+∞t−1∫t0X1(s)ds=D1A11,limt→+∞Xi(t)=0a.s.(i=2,3). |
(c) If D1≥0, −Di+Ai1D1A11≥0, then
limt→+∞t−1∫t0X1(s)ds=D1A11,limt→+∞t−1∫t0Xi(s)ds=A−1ii(−Di+Ai1D1A11)a.s.(i=2,3). |
Proof. By Itô's formula, we have
{lnX1(t)=D1t−A11∫t0X1(s)ds−T11(X1)(t)+o(t),lnXi(t)=−Dit+Ai1∫t0X1(s)ds−Aii∫t0Xi(s)ds+Ti1(X1)(t)−Tii(Xi)(t)+o(t),(i=2,3), | (2.7) |
where
Tji(Xi)(t)=∫0−τji∫0θXi(s)dsdμji(θ)−∫0−τji∫tt+θXi(s)dsdμji(θ). |
Case(i): D1<0. Then limt→+∞X1(t)=0 a.s. Hence, for ∀ϵ∈(0,Di2) and t≫1,
lnXi(t)≤(−Di+ϵ)t−aii∫t0Xi(s)ds,(i=2,3). | (2.8) |
So, limt→+∞Xi(t)=0 a.s. (i=2,3).
Case(ii): D1≥0. Then,
limt→+∞t−1∫t0X1(s)ds=D1A11a.s. | (2.9) |
Consider the following SDDE:
d~Xi(t)=~Xi(t)(−ri+Di1(X1)(t)−aii~Xi(t))dt+σi~Xi(t)dBi(t),(i=2,3). |
Then, Xi(t)≤~Xi(t) a.s. (i=2,3). By Itô's formula,
ln~Xi(t)=(−Di+Ai1D1A11)t−aii∫t0~Xi(s)ds+o(t). |
In view of Lemma 2.1, we obtain:
(1)† If D1≥0, −Di+Ai1D1A11<0, then limt→+∞~Xi(t)=0 a.s. (i=2,3).
(2)† If D1≥0, −Di+Ai1D1A11≥0, then
limt→+∞t−1∫t0~Xi(s)ds=a−1ii(−Di+Ai1D1A11)a.s.(i=2,3). |
Therefore, for arbitrary constant γ>0,
limt→+∞t−1∫tt−γXi(s)ds=0a.s.(i=1,2,3). | (2.10) |
Based on (2.10) and system (2.7), for i=2,3,
lnXi(t)=(−Di+Ai1D1A11)t−Aii∫t0Xi(s)ds+o(t). |
Thanks to Lemma 2.1, we obtain:
(1)‡ If D1≥0, −Di+Ai1D1A11<0, then limt→+∞Xi(t)=0 a.s. (i=2,3).
(2)‡ If D1≥0, −Di+Ai1D1A11≥0, then
limt→+∞t−1∫t0Xi(s)ds=A−1ii(−Di+Ai1D1A11)a.s.(i=2,3). |
Therefore, the desired assertion (b) follows from combining (2.9) with (1)‡, and (c) follows from combining (2.9) with (2)‡.
Lemma 2.3. For system (1.3), lim supt→+∞t−1lnxi(t)≤0 a.s. (i=1,2,3).
Proof. Thanks to Lemma 2.2 and (2.7), system (2.6) satisfies limt→+∞t−1lnXi(t)=0 a.s. (i=1,2,3). From the stochastic comparison theorem, we obtain the desired assertion.
Theorem 2.2. For system (1.3):
(i) If D1<0, then limt→+∞xi(t)=0 a.s. (i=1,2,3).
(ii) If D1≥0, MΘ233<0, MΘ322<0, then
limt→+∞t−1∫t0x1(s)ds=D1A11,limt→+∞xi(t)=0a.s.(i=2,3). |
(iii) If D1≥0, MΘ13≤0, Θ3<0, MΘ233>0, then
limt→+∞t−1∫t0xi(s)ds=MΘi33MΘ33,limt→+∞x3(t)=0a.s.(i=1,2). |
(iv) If Θ1>0, Θ2>0, Θ3>0, MΘ11>0, then
limt→+∞t−1∫t0xi(s)ds=ΘiΘa.s.(i=1,2,3). |
(v) If D1≥0, MΘ12≥0, Θ2<0, MΘ322<0, then
limt→+∞t−1∫t0x1(s)ds=D1A11,limt→+∞xi(t)=0a.s.(i=2,3). |
(vi) If D1≥0, MΘ12≥0, Θ2<0, MΘ322≥0, then
limt→+∞t−1∫t0xi(s)ds=MΘi22MΘ22,limt→+∞x2(t)=0a.s.(i=1,3). |
Proof. According to (2.10), for arbitrary constant γ>0,
limt→+∞t−1∫tt−γxi(s)ds=0a.s.(i=1,2,3). | (2.11) |
By Itô's formula and (2.11), we derive
{lnx1(t)=D1t−A11∫t0x1(s)ds−A12∫t0x2(s)ds−A13∫t0x3(s)ds+o(t),lnx2(t)=−D2t+A21∫t0x1(s)ds−A22∫t0x2(s)ds−A23∫t0x3(s)ds+o(t),lnx3(t)=−D3t+A31∫t0x1(s)ds−A32∫t0x2(s)ds−A33∫t0x3(s)ds+o(t). | (2.12) |
Case(i): D1<0. From Lemma 2.2 (a), limt→+∞xi(t)=0 a.s. (i=1,2,3).
Case(ii): D1≥0, MΘ233<0, MΘ322<0. Based on system (2.12), for ∀ϵ∈(0,1) and t≫1,
lnx1(t)≤(D1+ϵ)t−A11∫t0x1(s)ds. | (2.13) |
By Lemma 2.1 and the arbitrariness of ϵ, we obtain
lim supt→+∞t−1∫t0x1(s)ds≤D1A11a.s. | (2.14) |
Hence, for ∀ϵ∈(0,1) and t≫1,
{lnx2(t)≤(MΘ233A11+ϵ)t−A22∫t0x2(s)ds,lnx3(t)≤(MΘ322A11+ϵ)t−A33∫t0x3(s)ds. | (2.15) |
According to Lemma 2.1 and the arbitrariness of ϵ, we have
limt→+∞xi(t)=0a.s.(i=2,3). | (2.16) |
Based on (2.16) and system (2.12), for ∀ϵ∈(0,1) and t≫1,
{lnx1(t)≤(D1+ϵ)t−A11∫t0x1(s)ds,lnx1(t)≥(D1−ϵ)t−A11∫t0x1(s)ds. | (2.17) |
In view of Lemma 2.1 and the arbitrariness of ϵ, we obtain
limt→+∞t−1∫t0x1(s)ds=D1A11a.s. | (2.18) |
Case(iii): D1≥0, MΘ13≤0, Θ3<0, MΘ233>0. Compute
MΘ13lnx1(t)−MΘ23lnx2(t)+MΘ33lnx3(t)=Θ3t−Θ∫t0x3(s)ds+o(t). |
By Lemma 2.3, for ∀ϵ∈(0,1) and t≫1,
MΘ33lnx3(t)≤(Θ3+ϵ)t−Θ∫t0x3(s)ds. | (2.19) |
From Lemma 2.1 and the arbitrariness of ϵ, we deduce
limt→+∞x3(t)=0a.s. | (2.20) |
By (2.20) and system (2.12), for ∀ϵ∈(0,1) and t≫1,
{lnx1(t)≤(D1+ϵ)t−A11∫t0x1(s)ds−A12∫t0x2(s)ds,lnx1(t)≥(D1−ϵ)t−A11∫t0x1(s)ds−A12∫t0x2(s)ds,lnx2(t)≤(−D2+ϵ)t+A21∫t0x1(s)ds−A22∫t0x2(s)ds,lnx2(t)≥(−D2−ϵ)t+A21∫t0x1(s)ds−A22∫t0x2(s)ds. | (2.21) |
According to (2.21), for ∀ϵ∈(0,1) and t≫1,
A21lnx1(t)+A11lnx2(t)≥[MΘ233−(A11+A21)ϵ]t−MΘ33∫t0x2(s)ds,A22lnx1(t)−A12lnx2(t)≤[MΘ133+(A12+A22)ϵ]t−MΘ33∫t0x1(s)ds. |
Thanks to Lemma 2.3, for ∀ϵ∈(0,1) and t≫1,
A11lnx2(t)≥[MΘ233−(A11+2A21)ϵ]t−MΘ33∫t0x2(s)ds,A22lnx1(t)≤[MΘ133+(2A12+A22)ϵ]t−MΘ33∫t0x1(s)ds. |
In view of Lemma 2.1 and the arbitrariness of ϵ, we obtain
lim inft→+∞t−1∫t0x2(s)ds≥MΘ233MΘ33a.s. | (2.22a) |
lim supt→+∞t−1∫t0x1(s)ds≤MΘ133MΘ33a.s. | (2.22b) |
Thanks to (2.21) and (2.22b), for ∀ϵ∈(0,1) and t≫1,
lnx2(t)≤(A22MΘ233MΘ33+2ϵ)t−A22∫t0x2(s)ds. | (2.23) |
Based on Lemma 2.1 and the arbitrariness of ϵ, we obtain
lim supt→+∞t−1∫t0x2(s)ds≤MΘ233MΘ33a.s. | (2.24) |
Combining (2.22-1) with (2.24) yields
limt→+∞t−1∫t0x2(s)ds=MΘ233MΘ33a.s. | (2.25) |
From (2.21) and (2.25), for ∀ϵ∈(0,1) and t≫1,
lnx1(t)≥(A11MΘ133MΘ33−2ϵ)t−A11∫t0x1(s)ds. | (2.26) |
Thanks to Lemma 2.1 and the arbitrariness of ϵ, we have
lim inft→+∞t−1∫t0x1(s)ds≥MΘ133MΘ33a.s. | (2.27) |
Combining (2.22-2) with (2.27) yields
limt→+∞t−1∫t0x1(s)ds=MΘ133MΘ33a.s. | (2.28) |
Case(iv): Θ1>0, Θ2>0, Θ3>0, MΘ11>0. According to Lemma 2.1, (2.19) and the arbitrariness of ϵ, we obtain
lim supt→+∞t−1∫t0x3(s)ds≤Θ3Θa.s. | (2.29) |
In view of system (2.12), we compute
MΘ11lnx1(t)−MΘ21lnx2(t)+MΘ31lnx3(t)=Θ1t−Θ∫t0x1(s)ds+o(t).−MΘ12lnx1(t)+MΘ22lnx2(t)−MΘ32lnx3(t)=Θ2t−Θ∫t0x2(s)ds+o(t). |
Then, for ∀ϵ∈(0,1) and t≫1,
MΘ11lnx1(t)≤(Θ1+ϵ)t−Θ∫t0x1(s)ds,MΘ22lnx2(t)≤(Θ2+ϵ)t−Θ∫t0x2(s)ds. |
According to Lemma 2.1 and the arbitrariness of ϵ, we deduce
lim supt→+∞t−1∫t0x1(s)ds≤Θ1Θa.s. | (2.30a) |
lim supt→+∞t−1∫t0x2(s)ds≤Θ2Θa.s. | (2.30b) |
Therefore, for ∀ϵ∈(0,1) and t≫1,
t−1∫t0xi(s)ds≤ΘiΘ+ϵa.s.(i=1,2,3). | (2.31) |
Based on (2.31) and system (2.12), for ∀ϵ∈(0,1) and t≫1,
lnx1(t)≥(A11Θ1Θ−3∑i=1A1iϵ)t−A11∫t0x1(s)ds. | (2.32) |
Thanks to Lemma 2.1 and the arbitrariness of ϵ, we obtain
lim inft→+∞t−1∫t0x1(s)ds≥Θ1Θa.s. | (2.33) |
Combining (2.30-1) with (2.33) yields
limt→+∞t−1∫t0x1(s)ds=Θ1Θa.s. | (2.34) |
According to (2.31), (2.33) and system (2.12), for ∀ϵ∈(0,1) and t≫1,
lnx2(t)≥(A22Θ2Θ−3∑i=1A2iϵ)t−A22∫t0x2(s)ds. | (2.35) |
From Lemma 2.1 and the arbitrariness of ϵ, we have
lim inft→+∞t−1∫t0x2(s)ds≥Θ2Θa.s. | (2.36) |
Combining (2.30-2) with (2.36) yields
limt→+∞t−1∫t0x2(s)ds=Θ2Θa.s. | (2.37) |
Similarly, for ∀ϵ∈(0,1) and t≫1,
lnx3(t)≥(A33Θ3Θ−3∑i=1A3iϵ)t−A33∫t0x3(s)ds. | (2.38) |
Thanks to Lemma 2.1 and the arbitrariness of ϵ, we obtain
lim inft→+∞t−1∫t0x3(s)ds≥Θ3Θa.s. | (2.39) |
Combining (2.29) with (2.39) yields
limt→+∞t−1∫t0x3(s)ds=Θ3Θa.s. | (2.40) |
Case(v): D1≥0, MΘ12≥0, Θ2<0, MΘ322<0. By Lemma 2.1, (2.30) and the arbitrariness of ϵ, we have
limt→+∞x2(t)=0a.s. | (2.41) |
Hence, for ∀ϵ∈(0,1) and t≫1,
{lnx1(t)≤(D1+ϵ)t−A11∫t0x1(s)ds−A13∫t0x3(s)ds,lnx1(t)≥(D1−ϵ)t−A11∫t0x1(s)ds−A13∫t0x3(s)ds,lnx3(t)≤(−D3+ϵ)t+A31∫t0x1(s)ds−A33∫t0x3(s)ds,lnx3(t)≥(−D3−ϵ)t+A31∫t0x1(s)ds−A33∫t0x3(s)ds. | (2.42) |
Based on (2.42), we deduce
A33lnx1(t)−A13lnx3(t)≤[MΘ122+(A13+A33)ϵ]t−MΘ22∫t0x1(s)ds. | (2.43) |
Therefore, for ∀ϵ∈(0,1) and t≫1,
A33lnx1(t)≤[MΘ122+(2A13+A33)ϵ]t−MΘ22∫t0x1(s)ds. | (2.44) |
In view of Lemma 2.1 and the arbitrariness of ϵ, we deduce
lim supt→+∞t−1∫t0x1(s)ds≤MΘ122MΘ22a.s. | (2.45) |
According to (2.42) and (2.45), for ∀ϵ∈(0,1) and t≫1,
lnx3(t)≤(A33MΘ322MΘ22+2ϵ)t−A33∫t0x3(s)ds. | (2.46) |
Clearly, (2.20) is true. From (2.20) and (2.42), for ∀ϵ∈(0,1) and t≫1,
{lnx1(t)≤(D1+2ϵ)t−A11∫t0x1(s)ds,lnx1(t)≥(D1−2ϵ)t−A11∫t0x1(s)ds. | (2.47) |
In view of Lemma 2.1 and the arbitrariness of ϵ, we obtain (2.18).
Case(vi): D1≥0, MΘ12≥0, Θ2<0, MΘ322≥0. Thanks to Lemma 2.1, (2.46) and the arbitrariness of ϵ,
lim supt→+∞t−1∫t0x3(s)ds≤MΘ322MΘ22a.s. | (2.48) |
According to (2.42) and (2.48), for ∀ϵ∈(0,1) and t≫1,
lnx1(t)≥(A11MΘ122MΘ22−2ϵ)t−A11∫t0x1(s)ds. | (2.49) |
Based on Lemma 2.1 and the arbitrariness of ϵ, we have
lim inft→+∞t−1∫t0x1(s)ds≥MΘ122MΘ22a.s. | (2.50) |
Combining (2.45) with (2.50) yields
limt→+∞t−1∫t0x1(s)ds=MΘ122MΘ22a.s. | (2.51) |
By (2.42) and (2.50), for ∀ϵ∈(0,1) and t≫1,
lnx3(t)≥(A33MΘ322MΘ22−2ϵ)t−A33∫t0x3(s)ds. | (2.52) |
Thanks to Lemma 2.1 and the arbitrariness of ϵ, we deduce
lim inft→+∞t−1∫t0x3(s)ds≥MΘ322MΘ22a.s. | (2.53) |
Combining (2.48) with (2.53) yields
limt→+∞t−1∫t0x3(s)ds=MΘ322MΘ22a.s. | (2.54) |
The proof is complete.
Theorem 3.1. Assume that 2ajj>∑3i=1Aij (j=1,2,3). Let X(t;ϕ)=:(x1(t;ϕ),x2(t;ϕ),x3(t;ϕ))T be the solution to system (1.3) with initial condition ϕ∈C([−τ,0],R3+). Then, for any ϕ and ϕ∗∈C([−τ,0],R3+),
limt→+∞E[‖X(t;ϕ)−X(t;ϕ∗)‖]=0. | (3.1) |
Proof. We only need to show
limt→+∞E|xi(t;ϕ)−xi(t;ϕ∗)|=0(i=1,2,3). | (3.2) |
Define
W(t;ϕ,ϕ∗)=3∑i=1|ln(xi(t;ϕ∗)xi(t;ϕ))|+3∑i,j=1∫0−τji∫tt+θ|xi(s;ϕ∗)−xi(s;ϕ)|dsdμji(θ). |
From Itô's formula, we derive
L[W(t;ϕ,ϕ∗)]≤−3∑j=1(2ajj−3∑i=1Aij)|xj(t;ϕ∗)−xj(t;ϕ)|. | (3.3) |
According to (3.3), we have
E[W(t;ϕ,ϕ∗)]−E[W(0;ϕ,ϕ∗)]≤−3∑j=1(2ajj−3∑i=1Aij)∫t0E[|xj(s;ϕ∗)−xj(s;ϕ)|]ds, |
which implies
∫+∞0E[|xi(t;ϕ∗)−xi(t;ϕ)|]dt<+∞(i=1,2,3). | (3.4) |
Define Gi(t)=E[|xi(t;ϕ∗)−xi(t;ϕ)|] (i=1,2,3). Then,
|Gi(t2)−Gi(t1)|≤E[|xi(t2;ϕ∗)−xi(t1;ϕ∗)|]+E[|xi(t2;ϕ)−xi(t1;ϕ)|]. | (3.5) |
Based on Hölder's inequality, for t2>t1 and p>1,
(E[|xj(t2)−xj(t1)|])p≤E[|xj(t2)−xj(t1)|p]≤2p−1E[(∫t2t1xj(s)(rj+3∑i=1ajixi(s)+3∑i=1∫0−τjixi(s+θ)dμji(θ))ds)p]+2p−1E[|∫t2t1σjxj(s)dBj(s)|p]≜2p−1Υ1+2p−1Υ2(j=1,2,3). | (3.6) |
In view of Theorem 7.1 in [14], for p≥2, we obtain
Υ2≤|σj|p(p(p−1)2)p2(t2−t1)p−22∫t2t1E[xpj(s)]ds. | (3.7) |
From Hölder's inequality, we derive
Υ1≤7p−1rpj(t2−t1)p−1∫t2t1E[xpj(s)]ds+3∑i=17p−1apji(t2−t1)p−1∫t2t1E[xpi(s)xpj(s)]ds+3∑i=17p−1(t2−t1)p−1E[∫t2t1(∫0−τjixi(s+θ)xj(s)dμji(θ))pds]. | (3.8) |
According to Hölder's inequality, we get
E[∫t2t1(∫0−τjixj(s)xi(s+θ)dμji(θ))pds]≤12(∫0−τjidμji(θ))p∫t2t1E[x2pj(s)]ds+12(∫0−τjidμji(θ))p−1∫t2t1∫0−τjiE[x2pi(s+θ)]dμji(θ)ds. | (3.9) |
Based on (3.6)-(3.9), for p≥2 and |t2−t1|≤δ,
(E[|xj(t2)−xj(t1)|])p≤Mj|t2−t1|p2, | (3.10) |
where
Mj=14p−1{rpjKj(p)+3∑i=1[apji2+12(∫0−τjidμji(θ))p][Ki(2p)+Kj(2p)]}δp2+2p−1|σj|p(p(p−1)2)p2Kj(p). |
Combining (3.5) with (3.10) yields
|Gj(t2)−Gj(t1)|≤2p√Mj√|t2−t1|. | (3.11) |
Therefore, (3.2) follows from (3.4), (3.11) and Barbalat's conclusion in [15].
In this section we provide some numerical simulations to show the effectiveness of our main theoretical results by using the Milstein approach mentioned in [16]. Let τji=ln2, μji(θ)=μjieθ. Denote
Param(i)=(r1a11a12a13μ11μ12μ13r2a21a22a23μ21μ22μ23r3a31a32a33μ31μ32μ33). |
Let
Param(1)=(0.90.20.40.20.40.80.20.20.60.30.10.60.60.20.10.40.30.20.80.40.4), |
subject to x1(θ)=0.7eθ, x2(θ)=0.6eθ, x3(θ)=0.5eθ, θ∈[−ln2,0].
Case1. σ1=1.4, σ2=0.1, σ3=0.1. Then, D1=−0.08. By Theorem 2.2 (i), all three species are extinctive. See Figure 1(a).
Case2. σ1=0.1, σ2=2.0, σ3=1.9. Then, D1=0.895, MΘ233=−0.0745, MΘ322=−0.046. From Theorem 2.2 (ii), x1(t) is persistent in mean, while x2(t) and x3(t) are extinctive. See Figure 1(b).
Case3. σ1=0.1, σ2=0.1, σ3=0.1. Then, Θ=0.225, Θ1=0.16225, Θ2=0.13375, Θ3=0.09825, MΘ11=0.14. In view of Theorem 2.2 (iv), all three species are persistent in mean. See Figure 1(c).
Let
Param(2)=(0.80.10.20.10.20.20.20.30.20.40.20.40.40.20.20.20.20.10.20.40.2), |
subject to x1(θ)=0.7eθ, x2(θ)=0.6eθ, x3(θ)=0.5eθ, θ∈[−ln2,0].
Case4. σ1=0.1, σ2=0.1, σ3=0.1. Then, Θ=0.001, D1=0.795, MΘ12=0.01, Θ2=−0.00975, MΘ322=0.1975. According to Theorem 2.2 (vi), both x1(t) and x3(t) are persistent in mean, while x2(t) is extinctive. See Figure 1(d).
Case5. σ1=0.2, σ2=1.8, σ3=1.5. Then, Θ=0.001, D1=0.78, MΘ12=0.01, Θ2=−0.0143, MΘ322=−0.031. Based on Theorem 2.2 (v), x1(t) is persistent in mean, while both x2(t) and x3(t) are extinctive. See Figure 1(e).
Let
Param(3)=(0.80.10.10.20.20.20.20.20.20.10.20.20.20.40.30.20.20.40.40.20.4), |
subject to x1(θ)=0.7eθ, x2(θ)=0.5eθ, x3(θ)=0.6eθ, θ∈[−ln2,0].
Case6. σ1=0.1, σ2=0.1, σ3=0.1. Then, Θ=0.001, D1=0.795, MΘ13=−0.01, Θ3=−0.00975, MΘ233=0.1975. Thanks to Theorem 2.2 (iii), both x1(t) and x2(t) are persistent in mean, while x3(t) is extinctive. See Figure 1(f).
All mentioned above can be confirmed by Figure 1.
The work is supported by National Natural Science Foundation of China (No.11901166).
The authors declare that there are no conflicts of interest.
[1] |
H. Freedman, P. Waltman, Mathematical analysis of some three-species food-chain models, Math. Biosci., 33 (1977), 257–276. http://doi.org/10.1016/0025-5564(77)90142-0 doi: 10.1016/0025-5564(77)90142-0
![]() |
[2] |
H. Freedman, P. Waltman, Persistence in models of three interacting predator-prey populations, Math. Biosci., 68 (1984), 213–231. http://doi.org/10.1016/0025-5564(84)90032-4 doi: 10.1016/0025-5564(84)90032-4
![]() |
[3] |
J. Roy, D. Barman, S. Alam, Role of fear in a predator-prey system with ratio-dependent functional response in deterministic and stochastic environment, Biosystems, 197 (2020), 104176. http://doi.org/10.1016/j.biosystems.2020.104176 doi: 10.1016/j.biosystems.2020.104176
![]() |
[4] |
Q. Zhang, D. Jiang, Dynamics of stochastic predator-prey systems with continuous time delay, Chaos Solitons Fractals, 152 (2021), 111431. http://doi.org/10.1016/j.chaos.2021.111431 doi: 10.1016/j.chaos.2021.111431
![]() |
[5] |
Y. Cai, S. Cai, X. Mao, Analysis of a stochastic predator-prey system with foraging arena scheme, Stochastics, 92 (2020), 193–222. http://doi.org/10.1080/17442508.2019.1612897 doi: 10.1080/17442508.2019.1612897
![]() |
[6] |
F. A. Rihan, H. J. Alsakaji, Stochastic delay differential equations of three-species prey-predator system with cooperation among prey species, Discret. Contin. Dyn. Syst. Ser., 15 (2022), 245. http://doi.org/10.3934/dcdss.2020468 doi: 10.3934/dcdss.2020468
![]() |
[7] |
H. J. Alsakaji, S. Kundu, F. A. Rihan, Delay differential model of one-predator two-prey system with Monod-Haldane and holling type Ⅱ functional responses, Appl. Math. Comput., 397 (2021), 125919. http://doi.org/10.1016/j.amc.2020.125919 doi: 10.1016/j.amc.2020.125919
![]() |
[8] |
J. Geng, M. Liu, Y.Q. Zhang, Stability of a stochastic one-predator-two-prey population model with time delays, Commun. Nonlinear Sci. Numer. Simul., 53 (2017), 65–82. http://doi.org/10.1016/j.cnsns.2017.04.022 doi: 10.1016/j.cnsns.2017.04.022
![]() |
[9] | K. Golpalsamy, Stability and Oscillations in Delay Differential Equations of Population Dynamics, Dordrecht: Kluwer Academic Publishers, 1992. https://doi.org/10.1007/978-94-015-7920-9 |
[10] |
S. Wang, L. Wang, T. Wei, Optimal harvesting for a stochastic logistic model with S-type distributed time delay, J. Differ. Equ. Appl., 23 (2017), 618–632. http://doi.org/10.1080/10236198.2016.1269761 doi: 10.1080/10236198.2016.1269761
![]() |
[11] |
L. C. Hung, Stochastic delay population systems, Appl. Anal., 88 (2009), 1303–1320. http://doi.org/10.1080/00036810903277093 doi: 10.1080/00036810903277093
![]() |
[12] |
S. Wang, L. Wang, T. Wei, Optimal Harvesting for a Stochastic Predator-prey Model with S-type Distributed Time Delays, Methodol. Comput. Appl. Probab., 20 (2018), 37–68. http://doi.org/10.1007/s11009-016-9519-2 doi: 10.1007/s11009-016-9519-2
![]() |
[13] |
M. Liu, K. Wang, Q. Wu, Survival Analysis of Stochastic Competitive Models in a Polluted Environment and Stochastic Competitive Exclusion Principle, Bull. Math. Biol., 73 (2011), 1969–2012. http://doi.org/10.1007/s11538-010-9569-5 doi: 10.1007/s11538-010-9569-5
![]() |
[14] | X. Mao, Stochastic differential equations and applications, England: Horwood Publishing Limited, 2007. |
[15] | I. Barbalat, Systems dequations differentielles d'osci d'oscillations, Rev. Roumaine Math. Pures Appl., 4 (1959), 267–270. |
[16] |
D. Higham, An algorithmic introduction to numerical simulation of stochastic differential equations, SIAM Rev., 43 (2001), 525–546. http://doi.org/10.1137/S0036144500378302 doi: 10.1137/S0036144500378302
![]() |
1. | Zhiqiang Hang, Xiaolin Wang, Fangfei Li, Yi-ang Ren, Haitao Li, Learning-based DoS attack game strategy over multi-process systems, 2024, 4, 2767-8946, 424, 10.3934/mmc.2024034 |