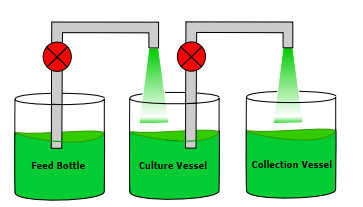
In this paper we study some chemostat models with random bounded fluctuations on the input flow. We start with the classical chemostat system and obtain new models incorporating, for instance, wall growth and different consumption functions, motivated by phenomena in real devices. In every case, we prove existence and uniqueness of positive global solution, existence of deterministic absorbing and attracting sets and we investigate the internal structure of the attracting sets to obtain detailed information about the long-time dynamics of the systems. This allows us to provide conditions under which either extinction or persistence of the species is ensured, the main goal for practitioners. In addition, we provide several numerical simulations to support the theoretical results.
Citation: Tomás Caraballo, Javier López-de-la-Cruz. Survey on chemostat models with bounded random input flow[J]. Mathematical Modelling and Control, 2021, 1(1): 52-78. doi: 10.3934/mmc.2021005
[1] | Bangxin Jiang, Yijun Lou, Jianquan Lu . Input-to-state stability of delayed systems with bounded-delay impulses. Mathematical Modelling and Control, 2022, 2(2): 44-54. doi: 10.3934/mmc.2022006 |
[2] | Mohamed COULIBALY, Modeste N'Zi . A stochastic model with jumps for smoking incorporating media coverage. Mathematical Modelling and Control, 2022, 2(3): 122-130. doi: 10.3934/mmc.2022013 |
[3] | Qin Xu, Xiao Wang, Yicheng Liu . Emergent behavior of Cucker–Smale model with time-varying topological structures and reaction-type delays. Mathematical Modelling and Control, 2022, 2(4): 200-218. doi: 10.3934/mmc.2022020 |
[4] | Pshtiwan Othman Mohammed . Some positive results for exponential-kernel difference operators of Riemann-Liouville type. Mathematical Modelling and Control, 2024, 4(1): 133-140. doi: 10.3934/mmc.2024012 |
[5] | Zhen Lin . On the sum of powers of the $ A_{\alpha} $-eigenvalues of graphs. Mathematical Modelling and Control, 2022, 2(2): 55-64. doi: 10.3934/mmc.2022007 |
[6] | Chunyu Tian, Lei Sun . Partitioning planar graphs with girth at least $ 9 $ into an edgeless graph and a graph with bounded size components. Mathematical Modelling and Control, 2021, 1(3): 136-144. doi: 10.3934/mmc.2021012 |
[7] | Hongyu Ma, Dadong Tian, Mei Li, Chao Zhang . Reachable set estimation for 2-D switched nonlinear positive systems with impulsive effects and bounded disturbances described by the Roesser model. Mathematical Modelling and Control, 2024, 4(2): 152-162. doi: 10.3934/mmc.2024014 |
[8] | Saïd Abbas, Mouffak Benchohra, Johnny Henderson . Random Caputo-Fabrizio fractional differential inclusions. Mathematical Modelling and Control, 2021, 1(2): 102-111. doi: 10.3934/mmc.2021008 |
[9] | Xingwen Liu, Shouming Zhong . Stability analysis of delayed switched cascade nonlinear systems with uniform switching signals. Mathematical Modelling and Control, 2021, 1(2): 90-101. doi: 10.3934/mmc.2021007 |
[10] | Qian Lin, Yan Zhu . Unicyclic graphs with extremal exponential Randić index. Mathematical Modelling and Control, 2021, 1(3): 164-171. doi: 10.3934/mmc.2021015 |
In this paper we study some chemostat models with random bounded fluctuations on the input flow. We start with the classical chemostat system and obtain new models incorporating, for instance, wall growth and different consumption functions, motivated by phenomena in real devices. In every case, we prove existence and uniqueness of positive global solution, existence of deterministic absorbing and attracting sets and we investigate the internal structure of the attracting sets to obtain detailed information about the long-time dynamics of the systems. This allows us to provide conditions under which either extinction or persistence of the species is ensured, the main goal for practitioners. In addition, we provide several numerical simulations to support the theoretical results.
Chemostat refers to a laboratory device used for growing microorganisms in a culture environment. It was invented at the same time by Monod (see [28]) and Novick and Szilard (see [29]) and provide a powerful tool to study microbial ecosystems at steady state with many applications, for instance, it can be used to investigate genetically altered microorganisms (see [34,35]), waste-water treatment processes (see [16,25]), models of mammalian large intestine (see [19,20]) and plays an important role in theoretical ecology (see [3,4,14,15,17,23,24,31,36,39,40,41]).
It is worth mentioning that the chemostat has been subject to a large number of scientific publications in applied sciences and Mathematics, being the focus of attention of several books and papers.
This good reputation is due to the fact that it is a very simple device that allows us to develop many different works reproducing the real devices in a very loyal manner. Moreover, it is also very interesting as a mathematical object, in fact it constitutes an active branch of applied mathematics which, moreover, proposes a recent formal framework called the theory of the chemostat.
Regarding the biological aspects, the chemostat device consists of three tanks, the feed bottle, the culture vessel and the collection vessel, which are interconnected by pumps, see Figure 1. The substrate is stored in the feed bottle and pumped to the culture vessel, where the interactions with the species take place. In addition, in order to keep the volume of the culture vessel constant, another flow is pumped from the culture vessel to the collection vessel. Then, our goal is to understand the dynamics of both the nutrient and the species in the culture vessel.
The classical chemostat model is given by the following system of ordinary differential equations (see [22,33])
dsdt=D(sin−s)−μ(s)x, | (1.1) |
dxdt=−Dx+μ(s)x, | (1.2) |
where s=s(t) and x=x(t) denote the concentration of the substrate and the species, respectively, D is the dilution rate, or input flow, sin describes the input concentration of substrate and μ is the consumption function of the species.
There are many works in the literature concerning the deterministic chemostat model (1.1)-(1.2). However, they assume strong restrictions. Among the different assumptions made when studying the system (1.1)-(1.2), one is to consider the input flow D fast enough such that species are removed from the culture vessel before having the opportunity to stick to the walls. This phenomenon, known as wall growth, was introduced by Pilyugin and Waltman (see [30]) and it is observed in real devices.
Hence, we divide the total concentration of species into two different groups, the planktonic biomass (species in the liquid media) and the attached biomass (species on the wall) such that we obtain the resulting chemostat (see [33])
dsdt=D(sin−s)−μ(s)yx1−μ(s)yx2+bνx1, | (1.3) |
dx1dt=−(ν+D)x1+μ(s)x1−r1x1+r2x2, | (1.4) |
dx2dt=−νx2+μ(s)x2+r1x1−r2x2, | (1.5) |
where s=s(t), x1=x1(t) and x2=x2(t) denote the concentration of the substrate, the planktonic biomass and the attached biomass, respectively, ν is the collective death rate, b∈(0,1) is the fraction of dead biomass which is recycled and r1 and r2 describe the rates at which the species stick on to and shear off the walls, respectively. D denotes again the input flow, sin is the input concentration of species, μ describes the consumption of the species and y∈(0,1] is the yield coefficient of transformation of substrate into biomass.
Another relevant ingredient when dealing with chemostats is the consumption function of the species. Even though there are different possibilities, the most typical one, called Monod, is given by the following expression
μ(s)=ˉμ0sa+s, | (1.6) |
where ˉμ0 denotes the maximum growth rate of the species and a is the so-called Monod constant.
However, it is very well-known that some species stop consuming when the concentration of substrate is high. This is very important in industrial setup, where large input concentrations sin can be observed. Hence, in order to model also this growth inhibition, we introduce the Haldane function (see [21])
μ(s)=ˉμ0sa+s+1kis2, | (1.7) |
where ˉμ0 denotes the growth rate coefficient of the species, a is again the Monod constant and ki describes the inhibition of species to take substrate if it is at high concentration (see [1]).
Once reached this point, we know now some important ingredients that could be incorporated to the classical chemostat (1.1)-(1.2) in order to obtain more realistic models. However, there is still another important strong assumption and it is that the input flow is constant. It is very well known that, in practice, some particles of dirt can remain inside the pumps and then the input flow is not constant, in fact, it is random (see Figure 2 where we present the evolution of the dilution rate in a real chemostat, where the red dashed line represents the nominal value D), in fact practitioners claim that the best approach would be to consider it to be random and bounded, as observed in real devices.
There are many different ways to model random fluctuations. The most typical one is to consider the standard Wiener process and replace D by D+α˙W(t), where α>0 denotes the intensity of the noise and ˙W(t) is the white noise. Nevertheless, since the Wiener process is unbounded with probability one, it could take arbitrary large values, both positive and negative, leading into several drawbacks from the biological point of view. We refer every interested reader to [11] (Section 3), where Caraballo et al consider the classical chemostat model (1.1)-(1.2) with Monod consumption function and perturb the input flow by means of the Wiener process, and [38] (Section 4) where López-de-la-Cruz investigates the chemostat model with wall growth (1.3)-(1.5) and Monod consumption function and models stochastic fluctuations on the input flow by using the Wiener process.
Some of the drawbacks found when using the Wiener process to perturb the input flow is that the solutions of the resulting stochastic systems may take negative values, which is completely unrealistic from the biological point of view, and persistence of species cannot be guaranteed due to the large fluctuations of the noise. For more details, see [11,38].
In order to overcome the previous drawbacks, another way to model perturbations on the input flow in chemostat models was proposed by Caraballo et al (see [5,10,13]). This new approach, based on the fact that such perturbations should be random but bounded, as the real ones, is explained in Section 2.
We recall that modeling bounded random perturbations is a very important issue for many researchers in applied sciences when trying to model real noises (see [5,6,12]). In addition, we also achieve important results when considering bounded random fluctuations, apart from obtaining models that fit the real perturbations in a very loyal manner. The most important one is to be able to prove the persistence of the species, the main goal for practitioners (see [5,6,7,12,26,27,38]).
Motivated by these explanations, our aim in this paper is to collect results concerning both chemostat models (1.1)-(1.2) and (1.3)-(1.5) where the input flow D is perturbed by bounded random fluctuations (as we will see in Section 2) and both the Monod and the Haldane consumption functions are considered. For every model, we prove first the existence and uniqueness of positive global solution of the corresponding random system, the existence of deterministic absorbing and attracting sets (that, in addition, do not depend on the noise) and we provide conditions under which both the extinction and the persistence of the species can be guaranteed, apart from depicting several numerical simulations supporting the theoretical part. In this way, this work is a complete survey on chemostat models with bounded random input flow.
The rest of the paper is organized in the following way: in Section 2 we present some preliminaries; in Section 3 we study the classical chemostat model with random bounded input flow and Monod consumption function; after that, in Section 4, we focus on the classical model with wall growth, apart from considering bounded random perturbations on the input flow and Monod consumption function; then, in Section 5, we investigate the classical chemostat model with bounded random input flow and non-monotonic consumption function; next, in Section 6, we focus on the chemostat model with wall growth, non-monotonic kinetics and bounded random perturbations on the input flow; finally, in Section 7, we provide some conclusions about the different results presented in this paper.
In this section we recall some results needed throughout the rest of this paper about deterministic chemostats with non-monotonic consumption function, the Ornstein-Uhlenbeck process and how to model bounded random fluctuations. Finally, we state some definitions of (uniform) persistence that are used in this work.
The next proposition recalls some classical results concerning the chemostat model (1.1)-(1.2) when μ has a non-monotonic growth. We refer interested readers to [22,32] for details.
Proposition 2.1. Assume that there exists ˆs∈(0,sin) such that μ is increasing on (0,ˆs) and decreasing on (ˆs,sin). Define the break-even concentrations λ−(D), λ+(D) as
λ−(D)=min{s∈[0,ˆs];μ(s)≥D},D∈[0,μ(ˆs)]λ+(D)=max{s∈[ˆs,sin];μ(s)≥D},D∈[μ(sin),μ(ˆs)] |
1. If D>μ(ˆs), the system (1.1)-(1.2) possesses a unique equilibrium E0:=(0,sin), which is globally asymptotically stable on R2+.
2. If D<μ(sin), the system (1.1)-(1.2) admits a unique positive equilibrium E−:=(sin−λ−(D),λ−(D)) which is globally asymptotically stable on R⋆+×R+.
3. If D∈[μ(sin),μ(ˆs)], the system (1.1)-(1.2) presents a bi-stability between E− and E0. From any initial condition in R⋆+×R+ excepted on a set of null measure, the solution converges asymptotically to E− or E0.
Remark 1. Note that only the second case is desirable to guarantee that the wash-out is avoided.
Remark 2. For the Haldane function (1.7), the break even concentrations λ± are given by
λ±(D)=(¯μ0−D)ki±√(¯μ0−D)2k2i−4D2aki2D. |
In the rest of the paper, we consider that μ is non-monotonic on [0,sin] when dealing with the Haldane function, otherwise the analysis is similar to monotonic growth function. In addition, we consider the following hypothesis.
Assumption 2.1. There exists sm∈(0,sin) such that μ is increasing on (0,sm) and decreasing on (sm,sin).
In this section we collect some results concerning the Ornstein-Uhlenbeck (OU) process. We refer readers to [5,7,8] for details.
The OU process is a stationary mean-reverting Gaussian stochastic process defined as
(t,ω)↦z(t,ω):=z∗(θtω)=−βν0∫−∞eβsθtω(s)ds, | (2.1) |
for all t∈R, ω∈Ω and β,ν>0, where ω denotes a standard Wiener process in a probability space (Ω,F,P), β is the mean reversion constant representing the strength with which the process is attracted by the mean, ν>0 is the volatility constant describing the variation or the size of the noise and θt denotes the usual Wiener shift flow given by
θtω(⋅)=ω(⋅+t)−ω(t),t∈R. |
We note that the OU process (2.1) can be obtained as the stationary solution of the Langevin equation
dz+βzdt=νdω. | (2.2) |
Typically, the OU process (2.1) can model the position of a particle by taking into account its friction in a fluid (which is the main difference with the typical standard Wiener process). Indeed, it can be considered as a generalization of the standard Wiener process and provides a link between the standard Wiener process (β=0, ν=1) and no noise at all (β=1, ν=0).
We recall in the next proposition some of its properties.
Proposition 2.2 (See [2,9]). There exists a θt-invariant set ˜Ω∈F of Ω of full measure such that for ω∈˜Ω and β,ν>0, we have
(i) the random variable |z∗(ω)| is tempered with respect to {θt}t∈R, i.e., for a.e. ω∈˜Ω,
limt→∞e−ηtsupt∈R|z∗(θ−tω)|=0,for all η>0. |
(ii) this mapping is a stationary solution of (2.2) with continuous trajectories
(t,ω)→z∗(θtω)=−βν0∫−∞eβs(θtω)(s)ds. |
(iii) for any ω∈˜Ω one has
limt→±∞|z∗(θtω)|t=0;limt→±∞1t∫t0z∗(θsω)ds=0; |
limt→±∞1t∫t0|z∗(θsω)|ds=E[|z∗|]<∞. |
From now on we consider β and ν fixed.
In this section we present a way to model bounded random fluctuations on the input flow of the chemostat model, fitting the real ones observed in practice.
Let us consider an interval [Dl,Dr] that is typically provided by practitioners from observations, where 0<Dl<D<Dr<∞.
Define now the function Φ:R→[−d,d] given by
Φ(z)=2dπarctan(z), | (2.3) |
where d=Dr−D=D−Dl>0.
Then, we have that
Dl<D+Φ(z∗(θtω))<Drfor allt∈R, | (2.4) |
which means that the perturbed input flow D+Φ(z∗(θtω)) is bounded in the desired interval [Dl,Dr] for every time, as in real devices.
In addition, we have the following ergodic property.
Proposition 2.3 (See [10,13]). Let be Φ(z)=2dπarctan(z) defined as in (2.3). Then
limt→+∞1t∫t0Φ(z∗(θsω))ds=0,a.s. inΩ. | (2.5) |
Remark 3. We remark that the ergodic property (2.5) remains true as long as Φ is an odd measurable function such that
limz→+∞Φ(z)=d>0. |
Remark 4. The resulting random systems, when using the previous way to model the perturbations on the input flow, generate a random dynamical system and then the theory of random dynamical systems and pullback attractors (see [8]) could be used. However, we investigate the resulting systems for every fixed realization of the noise ω∈Ω since, in this way, we can prove every result in forward sense, which is more natural than the pullback one in this case.
In this section, we recall some definitions of (uniform) persistence (see [18]) that we consider in the present work.
Definition 2.1. The dynamics of a chemostat model, where x=x(t) denotes the concentration of the species, is strongly persistent if there exists ϵ>0 such that for any initial condition and any realization of the D+Φ(z∗(θ(⋅)ω)), the solution verifies
lim inft→+∞x(t)>ϵ. |
Definition 2.2. The dynamics of a chemostat model, where x=x(t) denotes the concentration of the species, is weakly persistent if there exists ϵ>0 such that for any initial condition and any realization of D+Φ(z∗(θ(⋅)ω)), the solution verifies
lim supt→+∞x(t)>ϵ. |
In this section we consider the classical chemostat model (1.1)-(1.2) where the input flow D is replaced by D+Φ(z∗(θtω)), as explained in Section 2.3. Then, we obtain the following random chemostat
dsdt=[D+Φ(z∗(θtω))](sin−s)−μ(s)x, | (3.1) |
dxdt=−[D+Φ(z∗(θtω))]x+μ(s)x, | (3.2) |
where we consider the Monod function μ given by (1.6) to model the consumption of the species.
We recall that every state variable and parameter is defined as explained in the introductory section.
In the rest of this section X={(s,x)∈R2:s≥0,x≥0} denotes the positive cone in the two-dimensional space.
In this section we prove that the system (3.1)-(3.2) has a unique positive global solution.
Theorem 3.1. For any u0:=(s0,x0)∈X, the random system (3.1)-(3.2) possesses a unique global solution
u(⋅;0,ω,u0):=(s(⋅;0,ω,u0),x(⋅;0,ω,u0))∈C1([0,+∞),X) |
with u(0;0,ω,u0)=u0, where s0:=s(0;0,ω,u0) and x0:=x(0;0,ω,u0).
Proof. Set u(⋅;0,ω,u0):=(s(⋅;0,ω,u0),x(⋅;0,ω,u0)) and write the system (3.1)-(3.2) as
dudt=L(θtω)⋅u+F(u,θtω), |
where
L(θtω)=(−(D+Φ(z∗(θtω)))−ˉμ00−(D+Φ(z∗(θtω)))+ˉμ0) |
and F:X×[0,+∞)⟶R2 is given by
F(ξ,θtω)=(μ(ξ1)ξ2+sinD+sinΦ(z∗(θtω))−μ(ξ1)ξ2), |
where ξ=(ξ1,ξ2)∈X.
Since z∗(θtω) is continuous with respect to t (see Proposition 2.2 (ii)) and Φ is continuous, then Φ(z∗(θtω)) is continuous with respect to t and L generates an evolution system on R2. Moreover, F(⋅,θtω)∈C1(X×[0,+∞);R2) whence it is locally Lipschitz with respect to (ξ1,ξ2)∈X. Therefore, the system (3.1)-(3.2) has a unique local solution.
Now, define the new state variable q=s+x−sin satisfying the differential equation
dqdt=−[D+Φ(z∗(θtω))]q, |
whose solution is
q(t;0,ω,q0)=q0e−Dt−∫t0Φ(z∗(θsω))ds, | (3.3) |
for every t≥0 and q0=s0+x0−sin.
It is straightforward to check that q does not blow up at any finite time. In addition, from (3.2) we have
x(t;0,ω,x0)≤x0e−(D−ˉμ0)t−∫t0Φ(z∗(θsω))ds, |
since μ(s)≤ˉμ0 for any s≥0, thus x is bounded by an expression which does not blow up at any finite time.
Therefore, s does not blow up either and the unique local solution of the system (3.1)-(3.2) is a global one.
Moreover, x≡0 solves (3.2) and, from (2.4), we have
dsdt|s=0=[D+Φ(z∗(θtω))]sin>0, |
then the unique global solution of the system (3.1)-(3.2) remains inside X for every initial value u0∈X and t≥0.
Once proved the existence of a unique positive global solution of the random chemostat (3.1)-(3.2), we focus on proving the existence of absorbing and attracting sets for the solutions of such a system.
From now on, F⊂X denotes a bounded set.
Theorem 3.2. For any ε>0, there exists a deterministic compact absorbing set given by
Bε:={(s,x)∈X:sin−ε≤s+x≤sin+ε}, | (3.4) |
for the solutions of the system (3.1)-(3.2) in forward sense, i.e., there exists TF(ω,ε)>0 such that for every given u0∈F and ω∈Ω, the solution corresponding to u0 remains inside Bε for all t≥TF(ω,ε).
Proof. Consider again q=s+x−sin. Then, from (3.3), we obtain
limt→+∞q(t;0,ω,q0)=0. | (3.5) |
Thus, for every given u0∈F, ω∈Ω and any ε>0, there exists TF(ω,ε)>0 such that
−ε≤q(t;0,ω,q0)≤ε |
for every t≥TF(ω,ε), whence Bε (given by (3.4)) is a compact absorbing set in X for the solutions of the system (3.1)-(3.2).
From Theorem 3.2, we have that
A:={(s,x)∈X:s+x=sin} | (3.6) |
is a deterministic attracting set for the solutions of the system (3.1)-(3.2) in forward sense, i.e.,
limt→+∞supu0∈Finfb0∈A|u(t;0,ω,u0)−b0|X=0. |
Now, we are interested in investigating the random dynamics inside the attracting set A given by (3.6), in order to obtain more detail information about the long-time behavior of the system (3.1)-(3.2).
To this end, we first state the following theorem providing conditions under which the extinction of the species happens.
Theorem 3.3. Provided
D>μ(sin), | (3.7) |
the attracting set (3.6) is reduced to A0={(sin,0)}, which means the extinction of the species.
Proof. Since Bε, given by (3.4), is a compact deterministic absorbing set for the solutions of the system (3.1)-(3.2), for every ε>0, ω∈Ω and s0>0, there exists TF(ω,ε)>0 such that s(t;0,ω,s0)≤sin+ε for all t≥TF(ω,ε). Then, since μ is monotonic, μ(s(t;0,ω,s0))≤μ(sin+ε) for every t≥TF(ω,ε), ω∈Ω and s0>0. Thus, from (3.2) we have
dxdt≤−[D+Φ(z∗(θtω))]x+μ(sin+ε)x, |
whose solution is
x(t;0,ω,x0)≤x0e−(D−μ(sin+ε))t−∫t0Φ(z∗(θsω))ds. |
Then, as long as condition (3.7) holds true, there exists ε0>0 such that D>μ(sin+ε) for every ε∈(0,ε0), whence x tends to zero when t goes to infinity.
Therefore, the attracting set A given by (3.6) is reduced to a singleton component A0={(sin,0)}.
Next, we provide conditions under which the persistence of the species can be ensured, the main goal for practitioners.
Theorem 3.4. Assume that
ˉs<sin | (3.8) |
holds true, where ˉs=μ−1(Dr). Then, for any ε>0, there exists a compact deterministic absorbing set
ˆBε={(s,x)∈X:sin−ε≤s+x≤sin+ε,s_≤s≤ˉs,sin−ˉs−ε≤x≤sin−s_+ε} | (3.9) |
for the solutions of the system (3.1)-(3.2), where s_=μ−1(Dl).
Proof. Recall that q=s+x−sin. From (3.5), for any ε>0, ω∈Ω and u0∈F, there exists TF(ω,ε)>0 such that
−ε≤q(t;0,ω,q0)≤ε | (3.10) |
for every t≥TF(ω,ε).
Now, from (3.1) we have
dsdt=(sin−s)(D+Φ(z∗(θtω)))−μ(s)x=(sin−s)(D+Φ(z∗(θtω)))−μ(s)q−μ(s)(sin−s). |
Hence, from (3.10) we have
dsdt≤(sin−s)(D+Φ(z∗(θtω)))−μ(s)(sin−s)+εˉμ0 |
and
dsdt≥(sin−s)(D+Φ(z∗(θtω)))−μ(s)(sin−s)−εˉμ0 |
for every u0∈F, ε>0, ω∈Ω and for all t≥TF(ω,ε).
On the one hand, thanks to (3.8), we have
dsdt|s=ˉs≤(sin−ˉs)(D+Φ(z∗(θtω)))−μ(ˉs)(sin−ˉs)+εˉμ0≤(sin−ˉs)π−+εˉμ0, |
for every u0∈F, ε>0, ω∈Ω and for all t≥TF(ω,ε), where
π−:=supt≥0((D+Φ(z∗(θtω)))−μ(ˉs)). |
Then, by considering ε∈(0,−(sin−ˉs)π−/ˉμ0), we have (sin−ˉs)π−+εˉμ0<0 and
dsdt|s=ˉs<0, | (3.11) |
whence we have a strictly positive upper bound ˉs for the concentration of the substrate.
On the other hand, from (3.8) we have sin>s_ then
dsdt|s=s_≥(sin−s_)(D+Φ(z∗(θtω)))−μ(s_)(sin−s_)−εˉμ0≥(sin−s_)π+−εˉμ0, |
for every u0∈F, ε>0, ω∈Ω and for all t≥TF(ω,ε), where
π+:=inft≥0((D+Φ(z∗(θtω)))−μ(s_)). |
Now, for ε∈(0,(sin−s_)π+/ˉμ0) we obtain (sin−s_)π+−εˉμ0>0 and
dsdt|s=s_>0, | (3.12) |
whence we have a strictly positive lower bound s_ for the substrate.
Note that it is straightforward to prove that
dsdt|s=s∗>0anddsdt|s=˜s<0 |
for every s∗∈(0,s_) and ˜s>ˉs.
Hence, from (3.11) and (3.12) we have that
s_<s(t;0,ω,s0)<ˉs |
for every given ε∈(0,min{(sin−ˉs)π+/ˉμ0,−(sin−ˉs)π−/ˉμ0}), ω∈Ω, t≥TF(ω,ε) and s0>0.
Now, we focus on the species. From the previous reasoning,
−ˉs+sin−ε<x(t;0,ω,x0)<−s_+sin+ε, |
for every given ε∈(0,min{(sin−ˉs)π+/ˉμ0,−(sin−ˉs)π−/ˉμ0}), ω∈Ω, t≥TF(ω,ε) and x0>0.
Then, ˆBε (given by (3.9)) is a compact deterministic absorbing set for the solutions of the system (3.1)-(3.2) in forward sense.
As a corollary of Theorem 3.4, we have that the attracting set A in (3.6) is reduced to
˜A={(s,x)∈A:s_≤s≤ˉs,sin−ˉs≤x≤sin−s_} | (3.13) |
as long as (3.8) holds true, whence we ensure the persistence of the species.
Remark 5. It is worth mentioning that some improvements are achieved when comparing this work with the deterministic framework. More precisely, we recall that the washout equilibrium (sin,0) is attractive if D=μ(sin) in the deterministic case, and then we obtain extinction of the microbial biomass, see [22,32]. However, in the random case we prove conditions under which the attracting set has several points (all of them except the wash-out) inside the positive cone.
In this section we illustrate the theoretical results with some numerical simulations. The blue dashed lines correspond to the solutions of the deterministic systems and the rest are different realizations of the random ones.
In Figure 3 we depict the evolution on time of both the substrate and the species with sin=1, a=1, ˉμ0=3.1, D=1.7, d=0.4, Dr=2.1, Dl=1.3, s0=1 and x0=5. In this case, D=1.7>1.55=μ(sin) then the species become extinct as proved in Theorem 3.3.
Figure 4 shows the phase plane (s,x) for the previous values of the parameters. We also depict an arrow pointing the initial condition (s0,x0).
In Figure 5 we display the evolution of both the substrate and the species with sin=4, a=0.6, ˉμ0=4, D=1.7, d=0.25, Dr=1.95, Dl=1.45, s0=4 and x0=5. In this case we include a zoom of an interval closed to the final time to observe the dynamics of the solutions better. Since ˉs=0.57072<sin=4, we have persistence of the species, as proved in Theorem 3.4.
Figure 6 displays the phase plane where the values of the parameters are the ones in the previous figure. In addition, we also depict a zoom to observe better the dynamics around the attracting set (3.13), in fact, it is easy to notice how the solutions approach to the line s+x=sin.
In this section, we consider the chemostat model with wall growth (1.3)-(1.5) where the input flow D is replaced by D+Φ(z∗(θtω)), as in Section 2.3. Then, we obtain the following random system
dsdt=(D+Φ(z∗(θtω)))(sin−s)−μ(s)y(x1+x2)+bνx1, | (4.1) |
dx1dt=−(ν+D+Φ(z∗(θtω)))x1+μ(s)x1−r1x1+r2x2, | (4.2) |
dx2dt=−νx2+μ(s)x2+r1x1−r2x2, | (4.3) |
where μ is given by (1.6).
In this section X={(x,y,z)∈R3:x≥0,y≥0,z≥0} denotes the positive cone in the three-dimensional space.
In this section we prove that the system (4.1)-(4.3) has a unique positive global solution.
Theorem 4.1. For any u0:=(s0,x10,x20)∈X, the system (4.1)-(4.3) possesses a unique global solution u(⋅;0,ω,u0)∈C1([0,+∞),X) given by
u(⋅;0,ω,u0):=(s(⋅;0,ω,u0),x1(⋅;0,ω,u0),x2(⋅;0,ω,u0)), |
with u(0;0,ω,u0)=u0, where s0:=s(0;0,ω,u0), x10:=x1(0;0,ω,u0) and x20:=x2(0;0,ω,u0).
Proof. Write the system (4.1)-(4.3) as
dudt=L(θtω)u+F(u,θtω), |
where L(θtω) is given by
(−D(θtω)−μ0+bν−μ00−(ν+D(θtω))−r1+ˉμ0r20r1−ν+ˉμ0−r2), |
with D(θtω):=D+Φ(z∗(θtω)), and F:X×[0,+∞)→R3 is defined by
F(ξ,θtω)=((D+Φ(z∗(θtω))sin+μ(ξ1)yξ2+μ(ξ1)yξ3−μ(ξ1)ξ2−μ(ξ1)ξ3), |
where ξ=(ξ1,ξ2,ξ3)∈X.
Since Φ(z∗(θtω)) is continuous, L generates an evolution system on R3. Moreover, F(⋅,θtω)∈C1(X×[0,+∞);R3) then it is locally Lipschitz with respect to (ξ1,ξ2,ξ3)∈X. Thus, the system (4.1)-(4.3) has a unique local solution.
Define now q=s+μ0ˉμ0(x1+x2) satisfying the following differential inequalities
dqdt≤(D+Φ(z∗(θtω))sin−Dls−μ0ˉμ0Dlx1−μ0ˉμ0νx2≤(D+Φ(z∗(θtω))sin−ϑ[s+μ0ˉμ0x1+μ0ˉμ0x2]=(D+Φ(z∗(θtω))sin−ϑq, |
where ϑ:=min{Dl,ν}>0, whose solution is given by
q(t;0,ω,q0)≤q0e−ϑt+sin∫t0(D+Φ(z∗(θsω)))e−ϑ(t−s)ds. | (4.4) |
Thus, q does not blow up at any finite time, then s, x1 and x2 do not blow up at any finite time either and the unique local solution of the system (4.1)-(4.3) is defined globally in time.
In order to prove that every solution of the system (4.1)-(4.3) remains in X for any u0∈X, consider x1≥0 and x2≥0 whence
dsdt|s=0=(D+Φ(z∗(θtω)))sin+bνx1>0, |
since the random input flow is bounded from below by a positive constant. On the other hand, for any s≥0 and x2≥0, we have
dx1dt|x1=0=r2x2≥0 |
and, for every s≥0 and x1≥0, we obtain
dx2dt|x2=0=r1x1≥0. |
Thus, every solution of the random system (4.1)-(4.3) remains positive for every t≥0.
In this section, we are interested in studying the existence of a deterministic attracting set for the solutions of the random system (4.1)-(4.3), in order to obtain details about the asymptotic behavior of such a system.
In this section, F⊂X denotes again a bounded set.
Next theorem proves the existence of a compact deterministic absorbing set for the solutions of the system (4.1)-(4.3).
Theorem 4.2. For any ε>0, there exists a deterministic compact absorbing set for the solutions of the system (4.1)-(4.3) given by
Bε:={(s,x1,x2)∈X:s+μ0ˉμ0(x1+x2)≤sinDrϑ+ε}. | (4.5) |
Proof. Consider again q=s+μ0ˉμ0(x1+x2). Then, from (4.4) we have
0≤q(t;0,ω,q0)≤q0e−ϑt+sinDrϑ[1−e−ϑt] |
for every t≥0, ω∈Ω and q0>0, whence there exists TF(ω,ε)>0 such that
0≤q(t;0,ω,q0)≤sinDrϑ+ε |
for all t≥TF(ω,ε), ω∈Ω and q0>0. Then Bε (given by (4.5)) is a deterministic compact absorbing set (forwards in time) for the solutions of the system (4.1)-(4.3).
As a corollary of Theorem 4.2, we have that
A:={(s,x1,x2)∈X:s+μ0ˉμ0(x1+x2)≤sinDrϑ} | (4.6) |
is a deterministic attracting set (forwards in time) for the solutions of the system (4.1)-(4.3).
In this section we study the random dynamics of the system (4.1)-(4.3) inside the attracting set (4.6). To this end, we first define two state variables
x=x1+x2andp=x1x1+x2, | (4.7) |
the total concentration of species x and the proportion of the planktonic species p.
By differentiation, we obtain the random system
dsdt=(D+Φ(z∗(θtω)))(sin−s)−μ0sa+sx+bνpx, | (4.8) |
dxdt=−νx−(D+Φ(z∗(θtω)))px+ˉμ0sa+sx, | (4.9) |
dpdt=−(D+Φ(z∗(θtω)))p(1−p)−r1p+r2(1−p), | (4.10) |
where μ0:=ˉμ0/y>ˉμ0.
Let us first start studying the equation (4.10) which is uncoupled of the rest of the system.
Theorem 4.3. There exists a deterministic compact absorbing set
Bp=[pl,pr] | (4.11) |
for the solutions of (4.10), where pl and pr are constants given by
pl:=r2Dr+r1+r2andpr:=Dl+r2Dl+r1+r2. | (4.12) |
Proof. From (4.10), we have
dpdt=−(D+Φ(z∗(θtω)))p(1−p)−r1p+r2(1−p)≤−(Dl+r1+r2)p+Dl+r2, |
whence
dpdt|p=p∗<0 |
for every p∗>pr and
dpdt|p=pr≤0. |
On the other hand, from (4.10) we obtain
dpdt=−(D+Φ(z∗(θtω)))p(1−p)−r1p+r2(1−p)≥−(Dr+r1+r2)p+r2, |
whence
dpdt|p=˜p>0 |
for every ˜p<pl and
dpdt|p=pl≥0. |
Hence, Bp (given by (4.11)) is a deterministic compact absorbing set for the solutions of (4.10).
Next, we study the system (4.8)-(4.9). To this end, we define
z=ˉμ0s+μ0x. |
Theorem 4.4. For any ε>0, there exists a deterministic compact absorbing set
B(s,x)ε:={(s,x)∈R2+:zl−ε≤ˉμ0s+μ0x≤zr+ε} | (4.13) |
for the solutions of the system (4.8)-(4.9).
Proof. By differentiation, since b≤1, ˉμ0≤μ0 and p(t;0,ω,p0)≤1 for every t≥0, ω∈Ω and p0∈(0,1), we have
dzdt≤−(D+Φ(z∗(θtω)))plz+ˉμ0sin(D+Φ(z∗(θtω))) | (4.14) |
and
dzdt≥−[ν+(D+Φ(z∗(θtω)))−ˉμ0bνμ0pl]z+ˉμ0(D+Φ(z∗(θtω)))sin | (4.15) |
for every time t large enough.
By solving (4.14) and (4.15), we have
z(t;0,ω,z0)≤z0e−Dplt−pl∫t0Φ(z∗(θrω))dr+ˉμ0sin∫t0(D+Φ(z∗(θsω)))e−Dpl(t−s)−pl∫tsΦ(z∗(θrω))drds≤z0e−Dlplt+ˉμ0sinDrplDl[1−e−plDlt] |
and
z(t;0,ω,z0)≥z0e−(ν+D−ˉμ0bνμ0pl)t−∫t0Φ(z∗(θrω))dr+ˉμ0sin∫t0(D+Φ(z∗(θsω)))e−(ν+D−ˉμ0bνμ0pl)(t−s)−∫tsΦ(z∗(θrω))drds≥z0e−(Dr+ν−ˉμ0bνμ0pl)t+ˉμ0sinDlDr+ν−ˉμ0bνμ0pl[1−e−(Dr+ν−ˉμ0bνμ0pl)t], |
respectively, for every time t large enough, ω∈Ω and z0=ˉμ0s0+μ0x0>0.
Thus, for every z0=ˉμ0s0+μ0x0>0, ε>0 and ω∈Ω, there exists TF(ω,ϵ)>0 such that
zl−ε≤z(t;0,ω,z0)≤zr+ε | (4.16) |
for all t≥TF(ω,ε), where
zl:=ˉμ0sinDlDr+ν−ˉμ0bνμ0pl,zr:=ˉμ0sinDrplDl | (4.17) |
and Dr+ν−ˉμ0bνμ0pl>0.
Then, B(s,x)ε (given by (4.13)) is a deterministic compact absorbing set (forwards in time) for the solutions of the system (4.8)-(4.9).
Therefore, we have that
A(s,x):={(s,x)∈R2+:zl≤ˉμ0s+μ0x≤zr} | (4.18) |
is a deterministic attracting set for the solutions of the system (4.8)-(4.9).
Now, we are interested in studying the internal structure of the deterministic attracting set (4.18) in order to provide conditions under which either the extinction or the persistence of species can be proved.
Next theorem provides conditions under which the extinction of the species cannot be avoided.
Theorem 4.5. Assume that
ν+Dpl>ˉμ0 | (4.19) |
holds true. Then, the attracting set (4.6) for the solutions of the system (4.1)-(4.3) is reduced to
A0=[zlˉμ0,zrˉμ0]×{0}×{0}. |
Proof. From (4.9) we have
dxdt≤−[ν+(D+Φ(z∗(θtω)))pl−ˉμ0]x |
for every time t large enough, whose solution is
x(t;0,ω,x0)≤x0e−(ν+Dpl−ˉμ0)t−pl∫t0Φ(z∗(θsω))ds, |
whence
limt→+∞x(t;0,ω,x0)=0 |
as long as (4.19) is fulfilled, which means the extinction of both the planktonic and the attached biomass.
Now we provide conditions to ensure the persistence of the total concentration of species.
Theorem 4.6. Assume that
ν+Dr<zla+zrˉμ0 | (4.20) |
is fulfilled, where zl and zr are given as in (4.17). Then, there exists a deterministic compact absorbing set
ˆB(s,x)ε:={(s,x)∈R2+:x≥˜x,s≥˜s,zl−ε≤ˉμ0s+μ0x≤zr+ε} | (4.21) |
for the solutions of the system (4.8)-(4.9), where
˜x:=zl−(ν+Dr)(a+zrˉμ0)μ0+ˉμ0,˜s:=DlsinDr+2zra. | (4.22) |
Proof. Recall that
0≤p(t;0,ω,p0)≤1 |
for every t≥0, ω∈Ω and p0∈(0,1).
Thus, from (4.9), we obtain
dxdt≥−νx−Drx+ˉμ0sa+sx | (4.23) |
for all t≥0, ω∈Ω and x0>0.
By definition, we have
z(t;0,ω,z0)=ˉμ0s(t;0,ω,s0)+μ0x(t;0,ω,x0) |
and, thanks to (4.16), for each z0=ˉμ0s0+μ0x0>0, ω∈Ω and ε>0, there exists TF(ω,ε)>0 such that
zl−ε≤ˉμ0s(t;0,ω,s0)+μ0x(t;0,ω,x0)≤zr+ε |
for every t≥TF(ω,ε).
Thus, we have
ˉμ0s(t;0,ω,s0)≥zl−ε−μ0x(t;0,ω,x0) |
and
s(t;0,ω,s0)≤zrˉμ0+εˉμ0−x(t;0,ω,x0) |
for every initial value (s0,x0)∈F, ω∈Ω, ε>0 and t≥TF(ω,ε).
Then, from (4.23), we have
dxdt≥−νx−Drx+zl−μ0x−εa+zrˉμ0+εˉμ0−xx |
for every x0>0, ω∈Ω, ε>0 and t≥TF(ω,ε).
Now, consider ε<ˉμ0˜x, where ˜x is defined as in (4.22), and we obtain
dxdt|x=˜x≥[−(ν+Dr)+zl−μ0˜x−εa+zrˉμ0+εˉμ0−˜x]˜x>[−(ν+Dr)+zl−μ0˜x−ˉμ0˜xa+zrˉμ0+ˉμ0˜xˉμ0−˜x]˜x=0 |
for every x0>0, ε∈(0,ˉμ0˜x), ω∈Ω and t≥TF(ω,ε).
Hence, as long as (4.20) is fulfilled, we have
x(t;0,ω,x0)>˜x, |
for any ε∈(0,ˉμ0˜x), x0>0, ω∈Ω and t≥TF(ω,ε).
We note that it is straightforward to prove that
dxdt|x=x∗>0 |
for every x∗∈(0,˜x).
This proves a positive lower bound ˜x for the total concentration of species.
Now, recall that
dsdt=(D+Φ(z∗(θtω)))sin−(D+Φ(z∗(θtω)))s−μ0sa+sx(t;0,ω,x0)+bνpx, |
for all t≥0, ω∈Ω and every initial value s0>0.
In addition, from (4.16), for each x0>0, ε>0 and ω∈Ω, there exists TF(ω,ε)>0 such that
x(t;0,ω,x0)≤zrμ0+εμ0 |
for every t≥TF(ω,ε).
Then, from (4.8) we have
dsdt≥Dlsin−Drs−μ0sazr+εμ0, |
for every s0>0, ε>0, ω∈Ω and t≥TF(ω,ε).
Hence, for any ε∈(0,zr), s0>0 and ω∈Ω, there exists TF(ω,ε)>0 such that
dsdt|s=˜s>0 |
for all t≥TF(ω,ε), where ˜s is defined in (4.22), whence
s(t;0,ω,s0)>˜s |
for any ε∈(0,zr), s0>0, ω∈Ω and t≥TF(ω,ε).
It is easy to prove that
dsdt|s=s∗>0 |
for every s∗∈(0,˜s).
Thus, by considering ε∈(0,min{ˉμ0˜x,zr}), u0∈F and ω∈Ω, there exists TF(ω,ε)>0 such that
x(t;0,ω,x0)>˜xands(t;0,ω,s0)>˜s |
for all t≥TF(ω,ε) and then ˆB(s,x)ε (given by (4.21)) is a deterministic compact absorbing set (forwards in time) for the solutions of the system (4.8)-(4.9).
Therefore, we have that
ˆA(s,x):={(s,x)∈R2+:x≥˜x,s≥˜s,zl≤ˉμ0s+μ0x≤zr} | (4.24) |
is a deterministic attracting set (forwards in time) for the solutions of the system (4.8)-(4.9).
Remark 6. It is not difficult to check that both ˜x<zlμ0 and ˜s<zlˉμ0 are satisfied.
Finally, next theorem ensures the persistence of both the planktonic and the attached biomass individually.
Theorem 4.7. As long as (4.20) is fulfilled, we have
x1(t;0,ω,x10)>pl˜x>0 |
and
x2(t;0,ω,x20)>(1−pr)˜x>0 |
for every t large enough, ω∈Ω and x10,x20>0, where pl, pr and ˜x are defined as in (4.12) and (4.22).
Proof. From (4.7), Theorem 4.3 and Theorem 4.6, we have
x1(t;0,ω,x10)=p(t;0,ω,p0)x(t;0,ω,x0)>pl˜x>0 |
and
x2(t;0,ω,x20)=x(t;0,ω,x0)(1−p(t;0,ω,p0))>(1−pr)˜x>0 |
for every t large enough, ω∈Ω and x10,x20>0.
Theorem 4.7 gives conditions to ensure the persistence of both the planktonic and the attached biomass.
Remark 7. We highlight that the bound ˜x in (4.22) can be finer by considering ε∈(0,ˉμ0n˜x), for any n∈N, instead of ε∈(0,ˉμ0˜x) in the proof. In this case, the bound ˜x can be replaced by ˜xn given by
˜xn:=zl−(ν+Dr)(a+zrˉμ0)μ0+ˉμ0n>˜x>0. |
Similarly, by considering ε∈(0,1nzr), for any n∈N, instead of ε∈(0,zr) in the proof, we obtain ˜sn given by
˜sn:=DlsinDr+zra(1+1n)>˜s>0. |
In this section we illustrate the theoretical results with numerical simulations. The blue dashed lines correspond to the solution of the deterministic system and the rest are different realizations of the random one.
In Figure 7 we show the dynamics of both the substrate, the planktonic biomass and the attached biomass on time. In this case we set sin=4, a=1.8, μ0=2, ˉμ0=1.7, b=1, ν=1.7, r1=0.6, r2=0.4, D=1.7, d=0.25, Dr=1.95, Dl=1.45, s0=2.5, x10=2 and x10=2. We can observe the extinction of the species, which is not surprising from Theorem 4.5 since ν+Dpl=1.9305>1.7=ˉμ0.
In Figures 8 and 9 we illustrate the phase plane for the previous values of the parameters (Figure 8) and the dynamics of the substrate versus the planktonic biomass and the attached biomass (Figure 9. The arrow points the initial condition.
In Figure 10 we plot again the evolution of the substrate, the planktonic biomass and the attached biomass on time. We also add little zooms of an interval closed to the final time to observe better the dynamics of the solutions. In this case we consider sin=10, a=1.4, μ0=7.7, ˉμ0=6.7, b=0.7, ν=0.1, r1=0.4, r2=0.5, D=0.7, d=0.1, Dr=0.8, Dl=0.6, s0=2.5, x10=2 and x20=2. From Theorems 4.6 and 4.7, since (4.20) holds true, we observe that the species persist.
In Figures 11 and 12 we consider the same values of the parameters that the ones in the previous figure. However, in this case we show the phase plane (Figure 11) and the dynamics of the substrate versus the planktonic and the attached biomass (Figure 12) with little panels to observe better how the solutions approach the attracting set (4.24).
In this section we consider the classical chemostat model (1.1)-(1.2) where the input flow D is replaced by D+Φ(z∗(θtω)), as in Section 2.3, and the consumption function is given by (1.7). Then, the resulting model is
dsdt=(D+Φ(z∗(θtω)))(sin−s)−ˉμ0sa+s+s2kix, | (5.1) |
dxdt=(D+Φ(z∗(θtω)))x+ˉμ0sa+s+s2kix. | (5.2) |
Our aim in this section is to collect some known results about the system (5.1)-(5.2) to complement the results in Section 3. Then, we present a sketch of the proofs that can be found in [10].
From now on X:={(s,x):s≥0,x≥0} denotes the positive cone in the two-dimensional space.
Theorem 5.1 (See [10]). For any u0:=(s0,x0)∈X, the system (5.1)-(5.2) possesses a unique global solution
u(t;0,ω,u0):=(s(t;0,ω,u0),x(t;0,ω,u0))∈C1([0,+∞);X). |
The proof of this theorem is based on classical arguments from the theory of ordinary differential equations, thanks to the continuity and boundedness of the perturbed input flow.
Now, we focus on the existence of deterministic absorbing and attracting sets for the solutions of the system (5.1)-(5.2).
Theorem 5.2 (See [10]). The system (5.1)-(5.2) possesses a deterministic absorbing set
Bε:={(s,x)∈X:sin−ε≤s+x≤sin+ε} | (5.3) |
and then
A:={(s,x)∈X:s+x=sin}. | (5.4) |
is a deterministic attracting set for the solutions of the system.
Sketch of the proof. Define q=s−sin+x satisfying
dqdt=−(D+Φ(z∗(θtω)))q |
whose solution is given by
q(t;0,ω,q0)=q0e−∫t0(D+Φ(z∗(θsω)))ds, |
whence
limt→+∞q(t;0,ω,q0)=0. |
Thus, Bε (given by (5.3)) is a forward deterministic absorbing set for the solutions of the system (5.1)-(5.2).
Our aim now is to study the internal structure of the deterministic attracting set (5.4), in order to obtain details about the long-time dynamics of the system (5.1)-(5.2).
To this end, we first present conditions under which extinction of species cannot be avoided.
Theorem 5.3 (See [10]). Assume that
Dl>μ(sm) | (5.5) |
is fulfilled, where sm=√kia. Then, the singleton
A0:={(sin,0)} |
is a forward attracting set for the solutions of the system (5.1)-(5.2).
Sketch of the proof. From (5.2), we have
dxdt≤−(Dl−μ(sm))x. |
whose solution is given by
x(t;0,ω,x0)≤x0e−(Dl−μ(sm))t, |
whence we conclude since (5.5) is fulfilled.
Finally, we present some results providing conditions under which strong and weak (uniform) persistence of the species is ensured.
Theorem 5.4 (See [10]). Assume that
μ(sin)>D. |
is fulfilled. Then, the random system (5.1)-(5.2) is weakly (uniform) persistent.
Sketch of the proof. Let ε>0 be such that μ(s)>D for all s∈[sin−ε,sin+ε] and define
η:=min{μ(s)−D:s∈[sin−ε,sin+ε]}>0. |
From Theorem 5.2, there exists T(ω,ε)>0 such that s(t;0,ω,s0)<sin+ε for any t>T(ω,ε), ω∈Ω and s0>0.
Now, for t>T(ω,ε), define the sets
U(t):={τ∈[T(ω,ε),t]:s(τ;0,ω,s0)<sin−ε},V(t):={τ∈[T(ω,ε),t]:s(τ;0,ω,s0)∈[sin−ε,sin+ε]} |
and the functions in [0,1]
u(t):=meas(U(t))t−T(ω,ε),v(t):=meas(V(t))t−T(ω,ε)=1−u(t). |
From (5.2), we have
dxdt≥{−(D+Φ(z∗(θtω)))x(t),t∈U(t)(η−Φ(z∗(θtω)))x(t),t∈V(t) |
and, by integration between T(ω,ε) and t>T(ω,ε), one obtains
x(t)≥x(T(ω,ε))e(t−T(ω,ε))[u(t)(−D)+(1−u(t))η−1t−T(ω,ε)∫tT(ω,ε)Φ(z∗(θrω))dr] | (5.6) |
for t>T(ω,ε).
Assume now that
limt→+∞u(t)=0. |
Then, from Proposition 2.3, we have that
limt→+∞e−1t−T(ω,ε)∫tT(ω,ε)Φ(z∗(θrω))dr=1, |
whence, from (5.6), we obtain
limt→+∞x(t;0,ω,x0)=+∞ |
which is a contradiction since x is bounded. Then
limt→+∞meas(U(t))=+∞, |
that is
limt→+∞meas({t:s(t;0,ω,s0)<sin−ε})=+∞, |
whence
lim inft→+∞s(t;0,ω,s0)≤sin−ε |
or equivalently
lim supt→+∞x(t;0,ω,x0)≥ε>0 |
since s+x converges to sin (see Theorem 5.2).
Remark 8. Recall that the weak (uniform) persistence of species can be proved in the deterministic case if D<μ(sin), as in Theorem 5.4. It is worth mentioning that the upper bound Dr of the variations of the removal rate do not need to fulfill this and then we can have realizations of the perturbed dilution rate with effective value above μ(sin) on large periods of time. This means that the species could be arbitrary closed to the extinction but it always persist.
Next we assume a stronger condition on the removal rate to ensure the strong (uniform) persistence of the species.
Theorem 5.5 (See [10]). Assume that
Dr<μ(sin) |
is fulfilled. Then, the random system (5.1)-(5.2) is strongly (uniform) persistent and the set
ˆA:={(x,y)∈X:s+x=sin,x≥sin−λ−(Dr)} | (5.7) |
is (forward) attracting, where the function λ− is defined in Proposition 2.1.
Sketch of the proof. Consider ε>0 such that μ(s)>Dr for any s∈[sin−ε,sin+ε] and
η:=min{μ(s)−Dr:s∈[sin−ε,sin+ε]}>0. |
From Theorem 5.2, s converges to [0,sin]. Thus, there exists T(ω,ε)>0 such that
s(t;0,ω,s0)<sin+ε,q(t;0,ω,q0)>−κ, |
for all t>T(ω,ε), ω∈Ω, s0>0 and q0=s0+x0−sin, where
κ:=μ(sin−ε/2)−Drμ(sin−ε/2)(ε/2)>0. |
Now, if s(t;0,ω,s0)∈[sin−ε,sin+ε] for any t>T(ω,ε), ω∈Ω and s0>0, from (5.2) we have
dxdt>ηx |
for any t>T(ω,ε), whence x is unbounded and we obtain a contradiction. Then, there exists T(ω)≥T(ω,ε) such that s(T(ω))≤sin−ε/2.
Moreover, from (5.1)
dsdt=F(t,s):=(D+Φ(z∗(θtω))−μ(s))(sin−s)−μ(s)q. | (5.8) |
Since
F(t,sin−ε/2)≤(Dr−μ(sin−ε/2))(ε/2)+μ(sin−ε/2)κ=0 |
for t>T(ω,ε), then [0,sin−ε/2] is forward invariant for the semi-flow {˙s=F(t,s),t>T(ω,ε)} whence
s(t;0,ω,s0)≤sin−ε/2, |
for all t>T(ω), ω∈Ω and s0>0.
Then, from (5.8),
dsdt≤(Dr−μ(s))(sin−s)−μ(s)q |
for every t>T(ω), whence s(t;0,ω,s0)≤s+(t;0,ω,s0) for any t>T(ω), ω∈Ω and s0>0, where s+ solves
ds+dt=(Dr−μ(s+))(sin−s+)−μ(s+)q,s+(T(ω))=s(T(ω)). |
Note that s+(t;0,ω,s0) belongs to [0,sin] for any t≥T(ω), ω∈Ω and s0>0, then it is bounded, and its dynamics is asymptotic autonomous with limiting dynamics
ds†dt=(Dr−μ(s†))(sin−s†). |
If Dr<μ(sin), we have λ−(Dr)<sin and
(Dr−μ(σ))(λ−(Dr)−σ)>0,∀σ∈[0,sin]∖{λ−(Dr)} |
is fulfilled.
Finally, s† goes to λ−(Dr) when t goes to infinity. Then, from the theory of asymptotically autonomous dynamical systems [37], one concludes that s+ converges to λ−(Dr) when t goes to infinity, whence
lim supt→+∞s(t;0,ω,s0)≤λ−(Dr) |
i.e.,
lim inft→+∞x(t;0,ω,x0)≥sin−λ−(Dr)>0. |
Finally, next theorem provides an upper bound for the species.
Theorem 5.6 (See [10]). Assume x0>0. Then,
lim supt→+∞x(t;0,ω,x0)≤sin−λ−(Dl) |
for any solution of the system (5.1)-(5.2) as long as Dl<μ(sm).
Sketch of the proof. Recall that q=sin−x−s. From (5.2) one has
dxdt=(μ(s)−(D+Φ(z∗(θtω))))x=(μ(sin−x−q)−(D+Φ(z∗(θtω))))x:=F(t,x). |
Since q converges to zero when t goes to infinty, for every ε>0, ω∈Ω and q0=s0+x0−sin, there exists T(ω,ε)>0 such that |q(t;0,ω,q0)|<ε for every t>T(ω,ε) and then x(t;0,ω,x0)<sin+ε for every t>T(ω,ε), ω∈Ω and x0>0.
Moreover, for all x∈(sin−λ−(Dl)+ε,sin+ε), we obtain sin−x−q∈(−q−ε,λ−(Dl)−q−ε), in fact, since sin−x−q=s≥0, we have sin−x−q∈(0,λ−(Dl)−q−ε)⊂(0,λ−(Dl)) whence F(t,x)<0 since μ(0,λ−(Dl))<Dl and D+Φ(z∗(θtω))≥Dl.
Thus, for every ε>0,
lim supt→+∞x(t;0,ω,x0)≤sin−λ−(Dl)+ε. |
In this section we illustrate the theoretical results with numerical simulations. Again, the blue dashed lines correspond to the solution of the deterministic systems and the rest are different realizations of the random ones.
In Figure 13 we plot the evolution of the substrate and the species on time with sin=14, a=7, ˉμ0=4, ki=5, D=1.7, d=0.25, Dr=1.95, Dl=1.45, s0=14 and x0=5. In this case Dl=1.45>0.9302=μ(sm) then we have extinction of the species, as proved in Theorem 5.3.
Figure 14 shows the phase plane for the same values of the parameters, where the arrow points the initial condition.
In Figure 15 we display the evolution of the substrate and the species with sin=16.7, a=7, ˉμ0=7, ki=7, D=1.4, d=0.25, Dr=1.65, Dl=1.15, s0=16.7 and x0=5. Thanks to Theorem 5.5, since Dr=1.65<1.8397=μ(sin), we have (uniform) strong persistence of the species. In addition, we include a little panel with a zoom of the dynamics closed to the final time.
In Figure 16 we show the phase phase plane for the values of the parameters of the previous figure, with a little panel to see better the dynamics around the attracting set (5.7).
Finally, we present some numerical simulations to prove that bi-stability can also observed. To this end, in Figures 17 and 18 we set sin=20.1, a=7, ˉμ0=4, ki=7, D=1, d=0.25, Dr=1.25, Dl=0.75 and s0=20.1. We can observe how the species become extinct in Figure 17 with x0=0.01 and persist in Figure 18 if x0=5.
In this section we consider the chemostat model with wall growth (1.3)-(1.5) and replace the input flow D by D+Φ(z∗(θtω)), as in Section 2.3. In addition, we assume that the consumption function μ is non-monotonic given by (1.7). Then, we obtain the following random system
dsdt=(D+Φ(z∗(θtω)))(sin−s)−μ(s)yx1−μ(s)yx2+bνx1, | (6.1) |
dx1dt=−(ν+D+Φ(z∗(θtω)))x1+μ(s)x1−r1x1+r2x2, | (6.2) |
dx2dt=−νx2+μ(s)x2+r1x1−r2x2. | (6.3) |
Our aim in this section is to present some results concerning the system (6.1)-(6.3) to complement the ones in Section 4. Then, we present a sketch of the proofs and refer readers to [13] for details.
In this section we prove the existence and uniqueness of solution of the random system (6.1)-(6.3).
Now X={(s,x1,x2)∈R3:s≥0,x1≥0,x2≥0} denotes the positive cone in the three dimensional space.
Theorem 6.1 (See [13]). For every u0:=(s0,x10,x20)∈X, the random system (6.1)-(6.3) possesses a unique global solution u(⋅;0,ω,u0)∈C1([0,+∞);X) given by
u(⋅;0,ω,u0):=(s(⋅;0,ω,s0),x1(⋅;0,ω,x10),x2(⋅;0,ω,x20)). |
The proof of this theorem is based on standard arguments from the theory of ordinary differential equations, thanks to the continuity and boundedness of the perturbed input flow.
In this section, we are interested in investigating the existence of absorbing and attracting sets for the solutions of the random system (6.1)-(6.3), in order to obtain more information about the long-time dynamics of the system in study.
Theorem 6.2. For any ε>0, the random system (6.1)-(6.3) possesses a deterministic absorbing set (forwards in time) given by
Bε:={(s,x1,x2)∈X:s+x1+x2≤Drsinm+ε}, | (6.4) |
where m=min{Dl,ν}. As a consequence,
A:={(s,x1,x2)∈X:s+x1+x2≤Drsinm} | (6.5) |
is a deterministic attracting set for the solutions of the random system (6.1)-(6.3) forwards in time.
Sketch of the proof. Define q=s+x1+x2 satisfying
dqdt≤Drsin−mq, |
where m=min{Dl,ν}, whose solution is given by
q(t;0,ω,q0)≤q0e−mt+Drsinm(1−e−mt) |
for all t>0, ω∈Ω and q0=s0+x10+x20>0.
Then, for any ε>0 and ω∈Ω, there exists T(ω,ε)>0 such that
s(t;0,ω,s0)+x1(t;0,ω,x10)+x2(t;0,ω,x20)≤Drsinm+ε |
for any t≥T(ω,ε) and s0,x10,x20>0, whence Bε (given by (6.4)) is a forward absorbing set for the solutions of the random system (6.1)-(6.3).
Now, we study the internal structure of the deterministic attracting set (6.5) in order to obtain more detailed information about the random dynamics inside it.
To this end, as we did in Section 4, we consider the total concentration of species x and the proportion of the planktonic biomass p defined as
x=x1+x2andp=x1x1+x2. | (6.6) |
By differentiation, we obtain the random system
dsdt=(D+Φ(z∗(θtω)))(sin−s)−μ0ss+a+1kis2x+bνpx, | (6.7) |
dxdt=−(ν+(D+Φ(z∗(θtω)))p)x+ˉμ0ss+a+1kis2x, | (6.8) |
dpdt=−(D+Φ(z∗(θtω)))p(1−p)−r1p+r2(1−p), | (6.9) |
where we recall that μ0:=ˉμ0/y>μ0 as in Section 4.
Our goal now is to investigate the long-time dynamics of the random system (6.7)-(6.9).
We first focus on studying the dynamics of the equation for the proportion (6.9) since it is uncoupled of the rest of the system.
Theorem 6.3 (See [13]). The interval
Bp:=[pl,pr] | (6.10) |
is a deterministic absorbing set for the solutions of the random equation (6.9), where
pl:=Dr+r1+r2−√(Dr+r1+r2)2−4Dlr22Dl |
and
pr:=Dl+r1+r2−√(Dl+r1+r2)2−4Dlr22Dl. |
Sketch of the proof. From (6.9) we have
dpdt≥Dlp2−(Dr+r1+r2)p+r2. |
Define H:p∈[0,1]→H(p)∈R as H(p)=Dlp2−(Dr+r1+r2)p+r2 which is a convex parabolic function.
By Bolzano's Theorem, it is easy to prove that there exists a unique pl∈(0,1) such that
H(p){>0forp∈[0,pl),=0forp=pl,<0forp∈(pl,1], |
where
pl=Dr+r1+r2−√(Dr+r1+r2)2−4Dlr22Dl. |
Then, we have
dpdt|p=ˉp>0 |
for all ˉp<pl, whence
p(t;0,ω,p0)≥pl |
for every t large enough, ω∈Ω and p0∈(0,1).
A similar argument works to obtain the upper bound pr and then Bp (given by (6.10)) is a deterministic absorbing set for the solutions of the random equation (6.9).
Once investigated the equation for the proportion, we study the system (6.7)-(6.8).
Theorem 6.4 (See [13]). For any ε>0, the random system (6.7)-(6.9) has a deterministic absorbing set (forwards in time) given by
B(s,x)ε:={(s,x)∈R2+:zl−ε≤ˉμ0s+μ0x≤zr+ε}, | (6.11) |
where
zl:=ˉμ0sinDrplDlandzr:=ˉμ0sinDlDr+ν−ˉμ0bνμ0pl. |
Then
A(s,x):={(s,x)∈R2+:zl≤ˉμ0s+μ0x≤zr} | (6.12) |
is a deterministic attracting set (forwards in time) for the solutions of the random system (6.7)-(6.9).
Sketch of the proof. Define z=ˉμ0s+μ0x satisfying the differential equation
dzdt=ˉμ0(D+Φ(z∗(θtω)))sin−ˉμ0(D+Φ(z∗(θtω)))s+ˉμ0bνpx−μ0νx−μ0(D+Φ(z∗(θtω)))px. |
From the previous equation, it is easy to obtain
dzdt≤ˉμ0(D+Φ(z∗(θtω)))sin−(D+Φ(z∗(θtω)))plz |
and
dzdt≥−(ν+D+Φ(z∗(θtω))−ˉμ0bνμ0pl)z+ˉμ0(D+Φ(z∗(θtω)))sin | (6.13) |
for t large enough.
Hence, by solving (6.3) and (6.13), it is not difficult to prove that, for any ε>0, ω∈Ω and (s0,x0)∈R2+, there exists T(ω,ε)>0 such that
zl−ε≤ˉμ0s(t;0ω,s0)+μ0x(t;0,ω,x0)≤zr+ε | (6.14) |
for all t≥T(ω,ε), whence B(s,x)ε (given by (6.11)) is a deterministic absorbing set (forwards in time) for the solutions of the random system (6.7)-(6.8).
Now, we are interested in providing conditions under which the extinction of the species cannot be avoided.
Theorem 6.5 (See [13]). Assume
ν+D>μ(sm). | (6.15) |
Then, for any ω∈Ω and x0=x10+x20>0,
limt→+∞x(t;0,ω,x0)=0, | (6.16) |
which means the extinction of the species.
Sketch of the proof. From (6.8) one has
dxdt≤−(ν+D+Φ(z∗(θtω))−μ(sm))x, | (6.17) |
whose solution is given by
x(t;0,ω,x0)≤e−(ν+D−μ(sm))t−∫t0Φ(z∗(θrω))dr | (6.18) |
for every t>0, ω∈Ω and x0=x10+x20>0.
Hence, as long as (6.15) is fulfilled, we have
limt→+∞x(t;0,ω,x0)=0. |
Finally, we provide conditions under which not only the total concentration of species persists, but also both the planktonic and the attached biomass individually.
Theorem 6.6 (See [13]). Provided
ˉμ0ν+Drprzl>zr+ˉμ0a+(zr)2kiˉμ0, | (6.19) |
where every constant was already defined, the attracting set (6.12) is reduced to
ˆA(s,x):={(s,x1,x2)∈A(s,x):s≥ˉs,x≥ˉx}, | (6.20) |
where
ˉs:=−a1−√a21−4a2a02a2andˉx:=−b1−√b21−4b2b02b2, |
with
a2:=−Dr−2ˉμ0μ0bνpl,a0:=Dlsina+zlabνplμ0 |
a1:=Dlsin−Dra−zr+zlbνplμ0−2ˉμ0μ0abνpl, |
b2:=−μ20kiˉμ0,b1:=−ˉμ0μ0ν+Drpr+μ0+2zrμ0kiˉμ0, |
b0:=ˉμ0zlν+Drpr−zr−ˉμ0a−(zr)2kiˉμ0 |
Sketch of the proof. From (6.7), we obtain
dsdt≥Dlsin−Drs−s(zr+ε−ˉμ0s)s+a+1μ0bνpl(zl−ε−ˉμ0s), | (6.21) |
where we used (2.4) and (6.14).
Consider now ε<ˉμ0s∗, where s∗>0 such that
Dlsin−Drs∗−s∗zrs∗+a+1μ0bνpl(zl−2ˉμ0s∗)≥0. | (6.22) |
Hence, from (6.21) we have
dsdt|s=s∗≥0. |
Then, we prove the existence of s∗>0 such that (6.22) holds or, in other words,
a2(s∗)2+a1s∗+a0≥0, |
where a2, a1 and a0 are defined as in the statement of Theorem 6.7.
To this end, define F:s∈[0,+∞)↦F(s)∈R as F(s)=a2s2+a1s+a0 which is a concave parabolic function. It is not difficult to prove that there exists ˉs>0 such that F(s)>0 for every s∈[0,ˉs), F(s)<0 when s∈(ˉs,+∞) and F(ˉs)=0, where
ˉs=−a1−√a21−4a2a02a2. | (6.23) |
Hence (6.22) fulfills for every s∗∈[0,ˉs], in fact
dsdt|s=s∗>0 |
for s∗∈[0,ˉs) and
dsdt|s=ˉs≥0, |
whence
s(t;0,ω,s0)≥ˉs |
for every t large enough, ω∈Ω and s0>0.
A similar argument works to provide the lower bound for the total concentration of species ˉx.
Finally, the next theorem ensures the persistence of both the planktonic and the attached biomass.
Theorem 6.7 (See [13]). Assume that (6.19) holds true. Then, for every x10>0, x20>0, ω∈Ω and t>0 large enough, the following lower bounds hold true
x1(t;0,ω,x10)≥ˉx1andx2(t;0,ω,x20)≥ˉx2, |
where
ˉx1:=plˉxandˉx2:=(1−pr)ˉx, |
i.e., both the planktonic biomass and the attached biomass persist, and pl, pr, ˉx are given in Theorems 6.3 and 6.6.
This theorem can be easily proved from the definition of the proportion (6.6), Theorem 6.3 and Theorem 6.6. A complete proof can be found in [13].
In this section we illustrate the theoretical results with numerical simulations. The blue dashed lines correspond to the solution of the deterministic systems and the rest are different realizations of the random one.
In Figure 19 we plot the evolution of the substrate, the planktonic biomass and the attached biomass on time. We set sin=4, a=0.4, μ0=4, ki=0.5, ˉμ0=1.4, b=0.1, ν=1.4, r1=0.4, r2=0.8, D=1.7, d=0.1, Dr=1.8, Dl=1.6, s0=2, x10=5 and x20=2. In this case ν+D=3.1>1.4343=μ(sm) and then we have extinction of the species from Theorem 6.5.
In Figures 20 and 21 we show the phase plane (Figure 20) and the dynamics of the substrate versus both the planktonic and the attached biomass (Figure 21). The arrows point the initial conditions.
In Figure 22 we illustrate the evolution of the substrate, the planktonic biomass and the attached biomass on time with little panels showing a zoom of the interval closed to the final time. In this case sin=5, a=1.7, μ0=8, ki=7, ˉμ0=7.5, b=0.1, ν=0.1, r1=0.4, r2=0.8, D=0.7, d=0.1, Dr=0.8, Dl=0.6, s0=2, x10=5 and x20=2. Since (6.19) holds, we have persistence of the species from Theorems 6.6 and 6.7.
In Figures 23 and 24 we show the phase plane (Figure 23) and the dynamics of the substrate versus both the planktonic biomass and the attached biomass (Figure 24) with zooms to observe better the dynamics around the attracting set (6.20).
Finally, we present some numerical simulations to show that bi-stability can be also observed. We set sin=10, a=1.8, μ0=3.4, ki=7.7, ˉμ0=2.3, b=0.1, ν=0.4, r1=0.4, r2=0.8, D=1.7, d=0.4, Dr=2.1, Dl=1.3 and s0=5. In Figure 25 we have extinction of the species with x10=0.01 and x20=0.01 and we observe persistence of the species if x10=4 and x20=1 in Figure 26.
In this paper we present results about different chemostat models where the input flow is perturbed by bounded random fluctuations, as in real life. We start with the classical chemostat model with Monod kinetics in Section 3. Then, in Section 4, we incorporate wall growth. After this, in Section 5 we revisit the classical chemostat but in this case we consider the Haldane consumption function. Finally, in Section 6 we investigate the chemostat model with wall growth and Haldane kinetics.
We prove for every model the existence and uniqueness of positive global solution. Then, we focus on the existence of absorbing and attracting sets for the solutions of the systems which, moreover, do not depend on the realization of the noise. After that, we study the internal structure of the corresponding attracting set, in order to obtain more detailed information about the asymptotic behavior of the state variables. This allows us to provide conditions under which the extinction of the species cannot be avoided and, what is the main goal in practice, conditions to guarantee the persistence of the species.
We recall that the approach in which real noise (bounded) is modeled in this paper helps us to achieve important improvements, specially when comparing the results in this paper with the ones when using the usual standard Wiener process, as explained in the introduction.
Now, we divide this section in four different parts to highlight the results in the different sections of the paper.
In Section 3 we consider the classical chemostat model with Monod consumption function. In this case, the extinction of the species cannot be avoided if D>μ(sin) (see Theorem 3.3) and we can ensure the persistence of the species as long as ˉs<sin (see Theorem 3.4). This essentially means that the input flow D cannot be too large compared with the input concentration of substrate sin, which is logical from the biological point of view. Of course, if we do not supply enough substrate to the culture vessel and the input flow is large, the species cannot have good access to the nutrient and, moreover, they are removed fast from the culture vessel.
It is worth mentioning that some improvements are achieved in this section when comparing with the deterministic framework. More precisely, the washout equilibrium (sin,0) is attractive if D=μ(sin) in the deterministic case, which means extinction of species. However, in the random case we prove conditions under which the attracting set has several points (all of them except the washout) inside the positive cone.
In Section 4, we prove that condition ν+Dpl>ˉμ0 leads into the extinction of the species (see Theorem 4.5) whereas the persistence can be ensured if
ν+Dr<zla+zrˉμ0 |
holds true (see Theorems 4.6 and 4.7), in fact both the planktonic and the attached biomass persist.
The condition needed to have persistence basically imposes some restrictions on the dilution rate D and the death collective rate ν, which is totally reasonable. Recall that the dilution rate cannot be too large since, otherwise, the species would not have a suitable access to the substrate and, moreover, they would be removed fast from the culture vessel. Concerning the collective death rate, it is not surprising that it needs to be small enough to preserve the species from the extinction.
In Section 5 we prove that the species become extinct if Dl>μ(sm) holds true (see Theorem 5.3). In addition, the weakly uniform persistence of species is ensured for D<μ(sin) (see Theorem 5.4). This means that species can be temporarily arbitrary closed to the extinction but they persist despite of the random disturbances on the input flow, in fact, D<μ(sin) ensures the persistence of the species in the deterministic case but the effective input flow does not necessarily satisfies this condition. Eventually, we prove the (uniform) strong persistence of the species as long as Dr<μ(sin) (see Theorem 5.5) and provide lower bounds for the concentration of species that can be useful for practitioners.
In Section 6 we show that the species become extinct if ν+D>μ(sin) (see Theorem 6.5) and persist provided that
ˉμ0ν+Drprzl>zr+ˉμ0a+(zr)2kiˉμ0, |
fulfills, see Theorems 6.6 and 6.7, which means that both the collective death rate ν and the input flow D cannot be too large.
It is worth mentioning that, as in the deterministic case (see [22]), in both Sections 5 and 6 dealing with Haldane kinetics, we observe bi-stability for certain values of the input flow (see numerical simulations in both sections) such that the species can converge either to the wash-out (which means extinction) or a positive equilibrium. This phenomenon is an important issue in industrial setup since it requires a careful monitoring of the system to prevent it from going to the wash-out.
Finally, we would like to remark that the way in which bounded random fluctuations are modeled in this paper has proved again to be a very realistic and powerful approach that allows us to obtain models fitting the real ones in a very loyal manner. In addition, it also helps us to provide conditions to ensure persistence, which is the main goal from the point of view of applications.
This work has been partially supported by Project PGC2018-096540-B-I00 from FEDER and Ministerio Ministerio de Ciencia, Innovación y Universidades (Spanish government), Project I+D+i FEDER US-1254251 from Consejería de Economía y Conocimiento (Junta de Andalucía) and Project P18-FR-4509 from Consejería de Economía y Conocimiento (Junta de Andalucía).
The authors declare there is no conflict of interests.
[1] | J. Andrews, A mathematical model for the continuous culture of microorganisms utilizing inhibitory substrates, Biotechnology & Bioengineering, 10 (1968), 707–723. |
[2] | L. Arnold, Random Dynamical Systems, Springer Berlin Heidelberg, 1998. |
[3] | J. Barlow, F. de Noyelles, B. Peterson, J. Peterson and W. Schaffner, Continuous flow nutrient bioassays with natural phytoplankton populations, G. Glass (Editor): Bioassay Techniques and Environmental Chemistry, John Wiley & Sons Ltd., 1973. |
[4] |
H. R. Bungay and M. L. Bungay, Microbial interactions in continuous culture, Advances in Applied Microbiology, 10 (1968), 269–290. doi: 10.1016/S0065-2164(08)70194-1
![]() |
[5] |
T. Caraballo, R. Colucci, J. López-de-la-Cruz and A. Rapaport, A way to model stochastic perturbations in population dynamics models with bounded realizations, Communications in Nonlinear Science and Numerical Simulation, 77 (2019), 239–257. doi: 10.1016/j.cnsns.2019.04.019
![]() |
[6] |
T. Caraballo, R. Colucci, J. López-de-la-Cruz and A. Rapaport, Study of the chemostat model with non-monotonic growth under random disturbances on the removal rate, Mathematical Biosciences and Engineering, 17 (2020), 7480–7501. doi: 10.3934/mbe.2020382
![]() |
[7] | T. Caraballo, M. J. Garrido-Atienza, J. López-de-la-Cruz and A. Rapaport, Modeling and analysis of random and stochastic input flows in the chemostat model, Discrete & Continuous Dynamical Systems - Series B, 24 (2018), 3591–3614. |
[8] | T. Caraballo and X. Han, Applied Nonautonomous and Random Dynamical Systems, Applied Dynamical Systems, Springer International Publishing, 2016. |
[9] |
T. Caraballo, P. E. Kloeden and B. Schmalfuss, Exponentially stable stationary solutions for stochastic evolution equations and their perturbation, Applied Mathematics and Optimization, 50 (2004), 183–207. doi: 10.1007/s00245-004-0802-1
![]() |
[10] |
T. Caraballo, R. Colucci, J. López-de-la-Cruz and A. Rapaport, Study of the chemostat model with non-monotonic growth under random disturbances on the removal rate, AIMS Mathematical Biosciences and Engineering, 17 (2020), 7480–7501. doi: 10.3934/mbe.2020382
![]() |
[11] | T. Caraballo, M. J. Garrido-Atienza and J. López-de-la-Cruz, Some Aspects Concerning the Dynamics of Stochastic Chemostats, vol. 69, chapter 11,227–246, Springer International Publishing, 2016. |
[12] | T. Caraballo, J.López-de-la-Cruz and A. Rapaport, Modeling bounded random fluctuations in biological systems: application to the chemostat model with two species, IFAC-PapersOnLine, 52 (2019), 187–192. |
[13] |
T. Caraballo and J. López-de-la-Cruz, Bounded random fluctuations on the input flow in chemostat models with wall growth and non-monotonic kinetics, AIMS Mathematics, 6 (2021), 4025–4052. doi: 10.3934/math.2021239
![]() |
[14] |
I. F. Creed, D. M. McKnight, B. A. Pellerin, M. B. Green, B. A. Bergamaschi, G. R. Aiken et al., The river as a chemostat: fresh perspectives on dissolved organic matter flowing down the river continuum, Canadian Journal of Fisheries and Aquatic Sciences, 72 (2015), 1272–1285. doi: 10.1139/cjfas-2014-0400
![]() |
[15] | A. Cunningham and R. M. Nisbet, Transients and oscillations in continuous cultures, Mathematics in Microbiology, 77–103. |
[16] | G. D'Ans, P. Kokotovic and D. Gottlieb, A nonlinear regulator problem for a model of biological waste treatment, IEEE Transactions on Automatic Control, 16 (1971), 341–347. |
[17] | A. Fredrickson and G. Stephanopoulos, Microbial competition, Science, 213 (1981), 972–979. |
[18] |
H. I. Freedman and P. Moson, Persistence definitions and their connections, Proceedings of the American Mathematical Society, 109 (1990), 1025–1033. doi: 10.1090/S0002-9939-1990-1012928-6
![]() |
[19] | R. Freter, Human Intestinal Microflora in Health and Disease, chapter Mechanisms that control the microflora in the large intestine, 33–54, Academic Press, New York, 1983. |
[20] | R. Freter, An understanding of colonization of the large intestine requires mathematical analysis, Microecology and Therapy, 16 (1986), 147–155. |
[21] | J. S. H. Haldane, Enzymes, Longmans Green and Co, London, 1930. |
[22] | J. Harmand, C. Lobry, A. Rapaport and T. Sari, The Chemostat: Mathematical Theory of Micro-organisms Cultures, Wiley, Chemical Engineering Series, John Wiley & Sons, Inc., 2017. |
[23] |
H. W. Jannasch, Steady state and the chemostat in ecology, Limnology and Oceanography, 19 (1974), 716–720. doi: 10.4319/lo.1974.19.4.0716
![]() |
[24] |
J. Kalff and R. Knoechel, Phytoplankton and their dynamics in oligotrophic and eutrophic lakes, Annual Review of Ecology and Systematics, 9 (1978), 475–495. doi: 10.1146/annurev.es.09.110178.002355
![]() |
[25] | J. W. M. La Rivière, Microbial ecology of liquid waste treatment, in Advances in Microbial Ecology, vol. 1, Springer US, 1977,215–259. |
[26] |
X. Li, J. Shen and R. Rakkiyappan, Persistent impulsive effects on stability of functional differential equations with finite or infinite delay, Applied Mathematics and Computation, 329 (2018), 14–22. doi: 10.1016/j.amc.2018.01.036
![]() |
[27] |
X. Li, X. Yang and T. Huang, Persistence of delayed cooperative models: Impulsive control method, Applied Mathematics and Computation, 342 (2019), 130–146. doi: 10.1016/j.amc.2018.09.003
![]() |
[28] | J. Monod, La technique de culture continue: Théorie et applications, Annales de l'Institute Pasteur, 79 (1950), 390–410. |
[29] |
A. Novick and L. Szilard, Experiments with the chemostat on spontaneous mutations of bacteria, Proceedings of the National Academy of Sciences, 36 (1950), 708–719. doi: 10.1073/pnas.36.12.708
![]() |
[30] | S. Pilyugin and P. Waltman, The simple chemostat with wall growth, Siam Journal on Applied Mathematics - SIAMAM, 59. |
[31] |
E. Rurangwa and M. C. J. Verdegem, Microorganisms in recirculating aquaculture systems and their management, Reviews in Aquaculture, 7 (2015), 117–130. doi: 10.1111/raq.12057
![]() |
[32] | H. L. Smith and P. Waltman, The theory of the chemostat: dynamics of microbial competition, Cambridge University Press, 1995. |
[33] | V. Sree Hari Rao and P. Raja Sekhara Rao, Dynamic Models and Control of Biological Systems, Springer-Verlag, Heidelberg, 2009. |
[34] |
G. Stephanopoulos, R. Aris and A. Fredrickson, A stochastic analysis of the growth of competing microbial populations in a continuous biochemical reactor, Mathematical Biosciences, 45 (1979), 99–135. doi: 10.1016/0025-5564(79)90098-1
![]() |
[35] |
F. Stewart and B. Levin, The population biology of bacterial plasmids: A priori conditions for the existence of conjugationally transmitted factors, Genetics, 87 (1977), 209–28. doi: 10.1093/genetics/87.2.209
![]() |
[36] |
P. A. Taylor and J. L. Williams, Theoretical studies on the coexistence of competing species under continuous flow conditions, Cadandian Journal of Microbiology, 21 (1975), 90–98. doi: 10.1139/m75-013
![]() |
[37] | H. R. Thieme, Convergence results and a poincare-bendixson trichotomy for asymptotically autonomous differential equations, Journal of Mathematical Biology, 30. |
[38] |
J. López-de-la-Cruz, Random and stochastic disturbances on the input flow in chemostat models with wall growth, Stochastic Analysis and Applications, 37 (2019), 668–698. doi: 10.1080/07362994.2019.1605911
![]() |
[39] |
H. Veldcamp, Ecological studies with the chemostat, Advances in Microbial Ecology, 1 (1977), 59–95. doi: 10.1007/978-1-4615-8219-9_2
![]() |
[40] | P. Waltman, Competition Models in Population Biology, CBMS-NSF Regional Conference Series in Applied Mathematics, 1983, Society for Industrial and Applied Mathematics, Philadelphia. |
[41] | P. Waltman, S. P. Hubbel and S. B. Hsu, Theoretical and experimental investigations of microbial competition in continuous culture, Modeling and Differential Equations in Biology (Conf., southern Illinois Univ. Carbonadle, III., 1978), 58 (1980), 107–152. |
1. | Tomás Caraballo, Javier López-de-la-Cruz, Verónica Caraballo-Romero, Non-autonomous chemostat models with non-monotonic growth, 2022, 0020-7160, 1, 10.1080/00207160.2022.2148467 | |
2. | Tomás Caraballo, Javier López-de-la-Cruz, Alain Rapaport, Study of the dynamics of two chemostats connected by Fickian diffusion with bounded random fluctuations, 2022, 22, 0219-4937, 10.1142/S0219493722400020 | |
3. | Tomás Caraballo, Javier López‐de‐la‐Cruz, Verónica Caraballo‐Romero, Chemostat models with Monod and Haldane consumption functions and random environmental fluctuations, 2023, 0170-4214, 10.1002/mma.9061 | |
4. | Tomás Caraballo, Javier López-de-la-Cruz, Verónica Caraballo-Romero, Effects of real random perturbations on Monod and Haldane consumption functions in the chemostat model, 2024, 218, 03784754, 482, 10.1016/j.matcom.2023.11.035 |