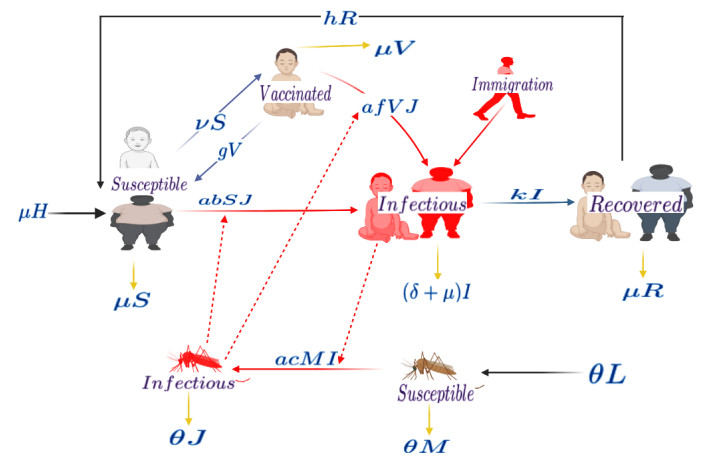
The world is aiming to eliminate malaria by 2030. The introduction of the pilot project on malaria vaccination for children in Kenya, Ghana, and Malawi presents a significant thrust to the elimination efforts. In this work, a susceptible, infectious and recovered (SIR) human-vector interaction mathematical model for malaria was formulated. The model was extended to include a compartment of vaccinated humans and an influx of infected immigrants. Qualitative and quantitative analysis was performed on the model. When there was no influx of infected immigrants, the model had a disease-free equilibrium point that was globally asymptotically stable when a threshold known as the basic reproductive number denoted by R0 was less than one. When there was an influx of infected immigrants, the model had endemic equilibrium points only. Parameter sensitivity analysis on R0 was performed and results showed that strategies must be implemented to reduce contact between mosquitoes and humans. Results from different vaccine coverage indicated that in the absence of an influx of infected immigrants, it is possible to achieve a malaria-free society when more children get vaccinated and the influx of infected humans is avoided. The analysis of the optimal control model showed that the combined use of vaccination, personal protective equipment, and treatment is the best way to curb malaria incidence, provided the influx of infected humans is completely stopped.
Citation: Pride Duve, Samuel Charles, Justin Munyakazi, Renke Lühken, Peter Witbooi. A mathematical model for malaria disease dynamics with vaccination and infected immigrants[J]. Mathematical Biosciences and Engineering, 2024, 21(1): 1082-1109. doi: 10.3934/mbe.2024045
[1] | Suxia Zhang, Hongbin Guo, Robert Smith? . Dynamical analysis for a hepatitis B transmission model with immigration and infection age. Mathematical Biosciences and Engineering, 2018, 15(6): 1291-1313. doi: 10.3934/mbe.2018060 |
[2] | Yicang Zhou, Zhien Ma . Global stability of a class of discrete age-structured SIS models with immigration. Mathematical Biosciences and Engineering, 2009, 6(2): 409-425. doi: 10.3934/mbe.2009.6.409 |
[3] | Peter Witbooi, Gbenga Abiodun, Mozart Nsuami . A model of malaria population dynamics with migrants. Mathematical Biosciences and Engineering, 2021, 18(6): 7301-7317. doi: 10.3934/mbe.2021361 |
[4] | Chenwei Song, Rui Xu, Ning Bai, Xiaohong Tian, Jiazhe Lin . Global dynamics and optimal control of a cholera transmission model with vaccination strategy and multiple pathways. Mathematical Biosciences and Engineering, 2020, 17(4): 4210-4224. doi: 10.3934/mbe.2020233 |
[5] | Shina D. Oloniiju, Olumuyiwa Otegbeye, Absalom E. Ezugwu . Investigating the impact of vaccination and non-pharmaceutical measures in curbing COVID-19 spread: A South Africa perspective. Mathematical Biosciences and Engineering, 2022, 19(1): 1058-1077. doi: 10.3934/mbe.2022049 |
[6] | Yaoyao Qin, Xin Pei, Mingtao Li, Yuzhen Chai . Transmission dynamics of brucellosis with patch model: Shanxi and Hebei Provinces as cases. Mathematical Biosciences and Engineering, 2022, 19(6): 6396-6414. doi: 10.3934/mbe.2022300 |
[7] | Lili Liu, Xi Wang, Yazhi Li . Mathematical analysis and optimal control of an epidemic model with vaccination and different infectivity. Mathematical Biosciences and Engineering, 2023, 20(12): 20914-20938. doi: 10.3934/mbe.2023925 |
[8] | Zhiping Liu, Zhen Jin, Junyuan Yang, Juan Zhang . The backward bifurcation of an age-structured cholera transmission model with saturation incidence. Mathematical Biosciences and Engineering, 2022, 19(12): 12427-12447. doi: 10.3934/mbe.2022580 |
[9] | Eunha Shim . A note on epidemic models with infective immigrants and vaccination. Mathematical Biosciences and Engineering, 2006, 3(3): 557-566. doi: 10.3934/mbe.2006.3.557 |
[10] | C. K. Mahadhika, Dipo Aldila . A deterministic transmission model for analytics-driven optimization of COVID-19 post-pandemic vaccination and quarantine strategies. Mathematical Biosciences and Engineering, 2024, 21(4): 4956-4988. doi: 10.3934/mbe.2024219 |
The world is aiming to eliminate malaria by 2030. The introduction of the pilot project on malaria vaccination for children in Kenya, Ghana, and Malawi presents a significant thrust to the elimination efforts. In this work, a susceptible, infectious and recovered (SIR) human-vector interaction mathematical model for malaria was formulated. The model was extended to include a compartment of vaccinated humans and an influx of infected immigrants. Qualitative and quantitative analysis was performed on the model. When there was no influx of infected immigrants, the model had a disease-free equilibrium point that was globally asymptotically stable when a threshold known as the basic reproductive number denoted by R0 was less than one. When there was an influx of infected immigrants, the model had endemic equilibrium points only. Parameter sensitivity analysis on R0 was performed and results showed that strategies must be implemented to reduce contact between mosquitoes and humans. Results from different vaccine coverage indicated that in the absence of an influx of infected immigrants, it is possible to achieve a malaria-free society when more children get vaccinated and the influx of infected humans is avoided. The analysis of the optimal control model showed that the combined use of vaccination, personal protective equipment, and treatment is the best way to curb malaria incidence, provided the influx of infected humans is completely stopped.
Malaria is a deadly mosquito-borne disease caused by Plasmodium parasites transmitted by Anopheles female mosquitoes between humans. Once an infected mosquito bites a human, the parasites multiply in the host's liver, destroying red blood cells during infection [1]. Parasites that infect humans are Plasmodium falciparum (P. falciparum), Plasmodium vivax (P. vivax), Plasmodium ovale (P. ovale), Plasmodium knowlesi (P. knowlesi) and Plasmodium malariae (P. malariae) [2]. P. falciparum and P. vivax are unicellular protozoan parasites of humans, and are the most important Plasmodium species causing malaria in humans [2]. Malaria is most common in tropical regions with high temperatures and humidity such as Africa, Central South America, the Dominican Republic, areas in the Caribbean, Eastern Europe, South Asia, and islands in the central and South Pacific Ocean [3]. However, other regions such as Southeast Asia, the Eastern Mediterranean, the Western Pacific and America also reported significant numbers of cases and deaths [4]. Information gathered by the World Health Organization (WHO) [4] indicated that there was an estimated 241 million cases of malaria in 2020 while deaths stood at 627,000, with most cases and deaths being recorded in Sub-Saharan Africa. The Sub-Saharan African region accounted for 95% of cases and 96% of malaria deaths with children under the age of five years, being the most affected, accounting for about 80% of malaria deaths [4], which has motivated us to carryout this study. People who live in poverty and do not have access to healthcare are more likely to have complications from the disease [3]. It is less common for pregnant women with malaria to transfer the parasite to their children before or during birth [3]. After a person has been infected, malaria symptoms usually appear within 10 to 30 days [3]. However, some people do not feel sick for up to a year after infection. Some studies suggest that parasites can live in the host body for several years without causing any symptoms [3]. Malaria often has mild flu-like symptoms such as headache, cough, and nausea, which may be difficult to recognize as malaria [4]. If not attended to in 24 hours, susceptible specimens like P. falciparum can progress to severe illness with symptoms such as high fever, fatigue, chest pains, breathing problems, diarrhea and vomiting [5], and when not treated, P. falciparum can cause anemia and jaundice [3], which can lead to death [4]. Children with severe malaria frequently develop severe anemia, respiratory distress to metabolic acidosis or cerebral malaria [4]. People residing in malaria-endemic areas may develop partial immunity, allowing asymptomatic infections to develop [4]. Some population groups such as infants, children under the age of five, patients living with HIV/AIDS, pregnant women and nonimmune migrants are considered to have a higher risk of contracting malaria and having a severe disease [6]. The first ordinary differential equation (ODE) model of malaria transmission was proposed and analyzed by Ross [7] and refinements of this model were produced by MacDonald [8]. The result became known as the Ross-MacDonald model, which has become a basis for modeling of mosquito-borne diseases. Several models of malaria have been studied and improvements on the formulations and analysis of the models keep on evolving. Models of malaria disease with vaccination have been studied by several authors; for instance, Mohammed [9], Tchoumi [10] and models with infected immigrants presented by, for instance; Witbooi [11] and Traoré [12]. De la Sen et al. (2015) [14] studied a susceptible, exposed, infectious and recovered (SEIR) epidemic model with finitely distributed delays and eventual vaccination with a pseudo-mass action type, where the infective transmission rate does not depend directly on the total population. The proposed model was analyzed qualitatively, and they further proposed general time varying feedback vaccination rules. In this paper, we present a new deterministic model with an influx of malaria parasites via infected immigrants as well as vaccination. We investigate the effectiveness of the malaria vaccine called RTS, S/AS01 or Mosquirix, in the presence of infected immigrants. The name RTS is due to the fact that the vaccine was manufactured using genes from the repeat ("R") and T-cell epitope ("T") of the pre-erythrocytic circumsporozoite protein (CSP) of the P. falciparum, together with a surface antigen ("S") of the hepatitis B virus, and AS01 is a chemical adjuvant added to it to increase the immune system response [13]. Our proposed model consists of a system of first-order ordinary differential equations. When there is zero influx of infectives and R0<1, the model was shown to have a disease-free equilibrium point that is globally asymptotically stable. The existence of endemic equilibria for the system is deduced and the local dynamics are proved using the center manifold theorem. Numerical simulations are also performed to investigate the effect of immigration in controlling the malaria disease.
Malaria interventions have been of great use over the years but have mostly not succeeded in eliminating the disease, and these efforts are being challenged by insecticide and drug resistance [15]. In complementing anti-malarial methods, the European Medicines Agency (EMA) [16] approved the use of a malaria vaccine known as RTS, S/AS01, or Mosquirix. This vaccine candidate is intended for use against malaria caused by the P. falciparum parasite to reduce severe cases of the disease in infants aged from six weeks to 17 months in areas where malaria is dominant [16]. Formal recommendations were issued by the WHO [17] on the pilot implementations of the four-dose schedule of Mosquirix in children below the age of five years. This pilot project was carried out in three Sub-Saharan African countries, namely Ghana, Kenya and Malawi, where three doses were given to children between one and 59 months old, at least four weeks apart from the first dose, with the fourth dose being issued 15–18 months after the third dose [18].
At a particular time t, the total human population under study is of size N(t). The human population is divided into susceptible individuals (S) who can be infected; fully vaccinated individuals (V) who have a reduced chance of being infected; infectious individuals (I) who have malaria and can infect mosquitoes and recovered individuals (R) who are free of malaria and have gained a partial immunity, such that N(t)=S(t)+V(t)+I(t)+R(t). The total vector population is constant and is given by L(t). It is divided into susceptible mosquitoes (M) and infectious mosquitoes (J) such that L(t)=M(t)+J(t). When susceptible and vaccinated individuals interact with infectious mosquitoes, they get infected. The rate at which vaccinated individuals become infected is much less than the rate at which unvaccinated susceptible individuals may be infected. The movement of individuals and mosquitoes between compartments and within the two sub-populations is given in Figure 1. Our model differs from existing models in the literature, such as, [10,11,19,20,21] in that it studies the dynamics of the malaria disease in the presence of immigration and vaccination simultaneously. This makes our model more realistic with respect to the Sub-Saharan African region, as the region is characterized by movement of both infected and uninfected individuals across different regions; at the same time, authorities are working on curbing malaria infections by using different control measures including vaccination.
The population of susceptible individuals is increased by a constant recruitment μH and the natural recovery of infected individuals at a rate h. We assume that susceptible individuals can contract the malaria parasite through a successful bite by an infectious female Anopheles mosquito at a rate a, where each bite has a probability b of bringing an infection. These individuals will die a natural death at a rate of μ. The class of vaccinated children is increased by vaccination of susceptible humans at a rate ν. Vaccinated children contract the disease when they are bitten by an infectious mosquito at a rate a, where each bite has a probability f of bringing an infection, where f<<b. These individuals are decreased by a proportion progressing back to being susceptible when the antibody titers decrease at a rate g and a natural mortality, at a rate μ. The compartment of infectious individuals is increased by both susceptible and vaccinated individuals who have been bitten by infectious mosquitoes J at a rate a. Infected immigrants increase the population of humans in this class at a rate μG. This class is reduced by a natural death at a rate μ, death due to the disease at a rate δ and recovery at a rate k. The class of recovered individuals is increased by the recovery of infectious individuals at a rate of k. We assume a recovery with temporary immunity, meaning recovered individuals have a chance of being susceptible again at a rate h. Recovered individuals may die a natural death at a rate μ. On the other hand, the population of susceptible mosquitoes is increased by a constant birth rate at a rate θL. When a susceptible mosquito bites an infectious individual (I) at a rate a, with a probability of bringing an infection c, it eventually become infectious and will progress to the class of infectious mosquitoes (J). Susceptible mosquitoes will die a natural death at a rate θ or will become infectious through a force of infection ac. The population of infectious mosquitoes increases when susceptible mosquitoes interact with infectious humans. These mosquitoes acquire the infection through a successful bite at a rate a, with a probability of infection c. The model equations describing the dynamics of malaria are given by system (1.1):
dS(t)dt=μH−abS(t)J(t)−(μ+ν)S(t)+gV(t)+hR(t)dV(t)dt=νS(t)−afV(t)J(t)−(μ+g)V(t)dI(t)dt=μG+a[bS(t)+fV(t)]J(t)−(μ+k+δ)I(t)dR(t)dt=kI(t)−(μ+h)R(t)dM(t)dt=θL−acM(t)I(t)−θM(t)dJ(t)dt=acM(t)I(t)−θJ(t), | (1.1) |
with initial conditions S(0)=S0, V(0)=V0, I(0)=I0, R(0)=R0, M(0)=M0, J(0)=J0, where
N(t)=S(t)+V(t)+I(t)+R(t)andL(t)=M(t)+J(t). |
In this section we give a summary of the descriptions of the parameters and variables used in system (1.1). We also give the values of the parameters. The natural death rate of humans is calculated as
μ=1lifeexpectancy×365=166.2×365=0.00004per day, | (2.1) |
where the life expectancy is 66.2 years, with Kenya as an example [22].
System (1.1) describes the population of living organisms; therefore, it is important to show that all state variables S(t),V(t),I(t),R(t),M(t),J(t) are always positive for every time t≥0.
Theorem 3.1. The solutions S(t),V(t),I(t),R(t),M(t),J(t) of system (1.1) exist for all time and are always positive, smooth and unique for all t>0, subject to v,G >0 and the initial conditions S0>0,V0>0,I0>0,R0>0,M0>0,J0>0.
Lemma 3.2. Let U⊆Rn be open and consider a solution x=(x1,…,xn)∈C1((0,T);U)∩C([0,T);U) to the ODE
{˙x(t)=f(t,x(t))x(0)=x0, |
where x0=(x1,0,…,xn,0)∈U, with xi,0>0 for all i∈{1,…,n} and f=(f1,…,fn):(0,T)×U→Rn. With the property that fi(t,y)>0 for all y∈U such that y∈Ri−1>0×{0}×Rn−i≥0 , t∈(0,T) and i∈{1,…,n}, then x is positive for all time, i.e., xi(t)>0 for all t∈[0,T) and i∈{1,…,n}.
Proof. of Lemma 3.2.
Suppose x is not positive for all time, then by continuity there exists s∈(0,T) and j∈{1,…,n} such that xj(s)=0 and, thus, the set of all the zeros of all the xi; that is, ∪ni=1x−1i({0}), is closed, nonempty and bounded below and, thus, has a minimum zero, for τ∈(0,T). Therefore, we have that xi(τ)≥0, xi(t)>0 for all t∈(0,τ) and i∈{1,…,n}.
Thus, we have that (x2(τ),…,xn(τ))∈Rn−1≥0 and, if x1(τ)=0, then we have satisfied the conditions to apply the property of f to get f1(τ,x(τ))>0. Thus,
˙x1(τ)=f1(τ,x(τ))>0. |
By continuity, there exists 0<ε<τ such that ˙x1(t)>0, for all t∈(τ−ε,τ+ε). Thus, by the fundamental theorem of calculus, we have that
x1(τ−ε)=x1(τ)−∫ττ−ε˙x1(t)dt<0. |
This is a contradiction, as x1(t)≥0 for all t∈(0,τ) and, thus, x1(τ)>0.
Suppose (x1(τ),…,xi−1(τ))∈Ri−1>0 and (xi(τ),…,xn(τ))∈Rn−i+1≥0 for i∈{2,…,n}. If xi(τ)=0, then similarly to before, we satisfy the conditions to apply the property of f to get fi(τ,x(τ))>0. The same as before, we derive a contradiction and conclude xi(τ)>0 and that (x1(τ),…,xi(τ))∈Ri>0 and (xi+1(τ),…,xn(τ))∈Rn−i≥0. Thus, by induction, we obtain that x1(τ),…,xn(τ)>0. This contradicts that τ∈∪ni=1x−1i({0}); thus, there must be no zeros, so x is positive for all time.
Next, we prove Theorem 3.1.
Proof. of Theorem 3.1
We consider the ODE system
x=(x1x2x3x4x5x6)=(MISVJR),x0=(M0I0S0V0J0R0) and f=(f1f2f3f4f5f6)=(θL−(acI+θ)MμG+a[bS+fV]J−(μ+k+δ)IμH+gV+hR−(abJ+μ+ν)SνS−(afJ+μ+g)VacMI−θJkI−(μ+h)R). |
We define the set U=BN0+L+1 as the open ball of radius N+L+1, where N0 is the human population and L is the mosquito population; we can see from the ODE that these remain constant. We see that x0∈U, and that on U, f is a bounded function, Lipschitz continuous and is smooth. By the Picard-Lindelöf theorem, there exists a T>0 and unique solution x=(x1,…,xn)∈C1((0,T);U)∩C([0,T);U) to the system of ODEs with given initial conditions. Since the system of ODEs is in the format of Lemma 3.2 with n=6, we have the positivity of the solutions of system (1.1). Since we have positivity of solutions and N(t)=S(t)+V(t)+I(t)+R(t) and L=M(t)+J(t), then x(t)∈¯BN0+L+H+G⋐U bu Eq (4.2), so by the Corollary in section 17.4 of the book [24], we have that the solution is defined for all time and we have a global positive unique solution. By applying a bootstrap argument, we obtain that the solution is smooth in time. That is, since f is smooth and
x(t)=x0+∫t0f(x(s))ds, | (3.1) |
if x(t) is Cn, then from (3.1) we have that x(t) is Cn+1. Thus, we obtain that x(t) is C∞.
We aim to analyze system (1.1) in a suitable feasible region D of biological interest.
Theorem 4.1. The feasible region D=DH×DV, where
DH=[(S(t),V(t),I(t),R(t))∈R4+:S+V+I+R≤(H+G)]and |
DV=[(M(t),J(t))∈R2+:M+J=L] |
is attracting and positively invariant with respect to system (1.1) for all values of t≥0.
Proof.
N=S+V+I+R⟹dNdt=dSdt+dVdt+dIdt+dRdt. |
Substituting values of the derivatives of state variables of system (1.1), we get
dNdt=μ(H+G)−μN−δI⟹dNdt+μN≤μ(H+G). | (4.1) |
Solving Eq (4.1) by using the integrating factor If=e∫t0μdτ=eμt, we have:
ddt[N(t)eμt]≤μ(H+G)eμt⟹∫t0dds[N(s)eμs]≤∫t0μ(H+G)eμsds. |
Evaluating the integrals on both sides, we get:
N(t)eμt−N(0)≤(H+G)[eμt−1], |
which reduces to:
N(t)≤(H+G)+[N(0)−(H+G)]e−μt. |
For all t≥0, the total population N(t)≤(H+G) if N(0)≤(H+G). Thus, the region DH is positively invariant. If N(0)>(H+G), then N(t) approaches DH asymptotically. Thus, the region DH attracts all solutions in R4+.
Similarly, for the vector population,
M+J=L⟹dMdt+dJdt=dLdt⟹dLdt=0. |
The mosquito population will remain constant and will stay in DV for all t≥0.
Thus, D is positively invariant and system (1.1) is well-posed mathematically and biologically; hence, it is sufficient to study the dynamics of system (1.1) in D.
It is very important to check if the disease under study has steady states. These are points at which the disease is neither increasing nor decreasing. We equate the changes in the state variables to zero and we solve for the corresponding state variables such that:
dS(t)dt=dV(t)dt=dI(t)dt=dR(t)dt=dM(t)dt=dJ(t)dt=0. | (5.1) |
Observing that M+J=L⟹M=L−J, system (1.1) can be reduced to a system of five equations by eliminating the equation for dMdt so that we have
μH−abS∗(t)J∗(t)−(μ+v)S∗(t)+gV∗(t)+hR∗(t)=0vS∗(t)−afV∗(t)J∗(t)−(μ+g)V∗(t)=0μG+a[bS∗(t)+fV∗(t)]J∗(t)−(μ+k+δ)I∗(t)=0kI∗(t)−(μ+h)R∗(t)=0ac[L−J∗(t)]I∗(t)−θJ∗(t)=0. | (5.2) |
The solutions (S∗,V∗,I∗,R∗,M∗,J∗) of equations described in Eq (5.2) are the steady states of system (1.1).
NB: In the rest of this section we restrict to the case when there is no influx of infected immigrants, i.e., (G=0).
In this case, we have a disease-free equilibrium point
(S∗,V∗,I∗,R∗,M∗,J∗)=(H(g+μ)g+μ+ν,νHg+μ+ν,0,0,L,0). |
The reproductive number can be defined as the average number of new infections of the disease caused by one typically infected individual or mosquito in a completely susceptible population. We make use of the next-generation matrix method by Van den Driessche and Watmough [25]. This is done by considering transmissions and transitions between and within classes. Considering infectious classes only, we have
F=[a(bS∗+fV∗)J∗acM∗I∗]andV=[(μ+k+δ)I∗θJ∗]. |
Taking partial derivatives, we define the matrix F as the matrix of new infections and matrix V as the matrix of transitions, such that
F=(0a(bS∗+fV∗)acM∗0)andV=((δ+k+μ)00θ). |
R0=ρ(FV−1), where ρ is the spectral radius or largest eigenvalue. Thus,
R0=√a2HcL(bg+bμ+fν)θ(k+δ+μ)(g+μ+ν). | (5.3) |
● The term RH=√aH(bg+bμ+fν)(k+δ+μ)(g+μ+ν) is the average number of new infections caused by one infectious human in a completely susceptible mosquito population.
● The term RV=√acLθ is the average number of new infections caused by one infectious mosquito in a completely susceptible human population.
Theorem 5.1. The disease-free equilibrium point is locally asymptotically stable when R0<1, otherwise it is unstable.
Proof. To prove the local dynamics of our proposed model, we compute the Jacobian matrix J evaluated at the disease-free equilibrium point and we obtain:
J=(−(μ+ν)g0h0−abS∗ν0000−afV∗00−(δ+k+μ)00abS∗+afV∗00k−(h+μ)0000−acM∗0−θ000acM∗00−θ). |
The eigenvalues of J are:
{λ1=−θλ2=−(h+μ)λ3=−μλ4=−(g+μ+ν)λ5=−(δ+θ+μ+k)2−√((δ+θ+μ+k)2)2−[θ(δ+μ+k)−a2HcL(bg+bμ+fν)g+μ+ν]λ6=−(δ+θ+μ+k)2+√((δ+θ+μ+k)2)2−[θ(δ+μ+k)−a2HcL(bg+bμ+fν)g+μ+ν]. |
Following Allen [26], all eigenvalues are negative if they are real, or have negative real parts if they are complex, except for λ6, which is negative or has a negative real part whenever the term
θ(δ+μ+k)−a2HcL(bg+bμ+fν)g+μ+ν>0⟹a2HcL(bg+bμ+fν)θ(δ+μ+k)(g+μ+ν)<1⟹R20<1. |
Thus the disease-free equilibrium point is locally asymptotically stable when R0<1.
Theorem 5.2. The disease-free equilibrium point is globally asymptotically stable when R0<1.
Proof. We use a function of the form P(I,J)=α1I+α2J, where α1,α2>0 are constants to be determined. Since we proved in Lemma (3.1) that I,J>0, then P is positive definite. Taking first derivatives on P, we get
˙P=α1˙I+α2˙J. |
Substituting the right hand side of the third and sixth equations of system (1.1), we get;
˙P=α1[abSJ+afVJ−(k+δ+μ)I]+α2[acMI−θJ] |
=[α2acM−α1(k+δ+μ)]I+[α1abS+α1afV−α2θ]J. |
Evaluating ˙P at the disease-free equilibrium point, we get
˙P≤[α2acM∗−α1(k+δ+μ)]I+[α1abS∗+α1afV∗−α2θ]J |
=[α2acL−α1(k+δ+μ)]I+[α1abH(g+μ)g+μ+ν+α1afνHg+μ+ν−α2θ]J. |
Equating the coefficients of I and J to zero and solving for the nontrivial values of α1 and α2 by setting α1=1, we have α2=aH[bg+bμ+fν]θ(g+μ+ν).
Simplifying ˙P, we have
˙P≤[a2cLH(bg+bμ+fν)θ(g+μ+ν)−(k+δ+μ)]I+[0]J=[a2cLH(bg+bμ+fν)θ(g+μ+ν)(k+δ+μ)−1](k+δ+μ)I, |
⟹˙P≤[R20−1](k+δ+μ)I. |
We note that ˙P<0 if, and only if, R20<1⟹R0<1. When R0=1, then P=0. Thus, by [27], the disease free equilibrium point is globally asymptotically stable when R0<1.
In our study of the existence of endemic equilibrium points, we encounter the number R2i, which is:
R2i=2μG(g+μ+ν)+acLfGμ+aLb(G+H)(g+μ)+aLfν(G+H)θ(g+μ+ν)(δ+k+μ). | (6.1) |
This invariant is the indicator that determines the existence, or not, of a unique endemic equilibrium point. Note that if G=0, then Ri=R0.
Theorem 6.1. (a) Suppose that G=0. There exists a unique positive equilibrium point if R0>1.
(b) Suppose that G>0. There exists a unique positive equilibrium point if, and only if, Ri>1.
Proof. At the steady states, we equate the derivatives of the state variables of system (1.1) to zero, with G>0. Since M=L−J, we can eliminate the fifth equation of system (1.1) so that we have
μH−abS∗(t)J∗(t)−(μ+v)S∗(t)+gV∗(t)+hR∗(t)=0vS∗(t)−afV∗(t)J∗(t)−(μ+g)V∗(t)=0a[bS∗(t)+fV∗(t)]J∗(t)−(μ+k+δ)I∗(t)+μG=0kI∗(t)−(μ+h)R∗(t)=0ac[L−J∗(t)]I∗(t)−θJ∗(t)=0. | (6.2) |
From the last equation of system (6.2), we have
J∗=acLI∗acI∗+θ.FromtheequationforR∗,wehaveR∗=kI∗μ+h. |
By back substitution, we get
V∗=ν(acI∗+θ)2[μH(μ+h)+hkI∗](μ+h)[(a2bcLI∗+(μ+ν)(acI∗+θ))(a2fcLI∗+(μ+g)(acI∗+θ))−νg(acI∗+θ)2],
S∗=[a2fcLI∗+(μ+g)(acI+θ)][μH(μ+h)+hkI∗](acI∗+θ)(μ+h)[(a2bcLI∗+(μ+ν)(acI∗+θ))(a2fcLI∗+(μ+g)(acI∗+θ))−νg(acI∗+θ)2].
Substituting the values of S∗,V∗,R∗,J∗ into the equation for infective humans, we get the polynomial P(I∗), with
P(I∗) = Gμ(h+μ){[a2bcLI∗+(μ+ν)(acI∗+θ)][a2cfLI∗+(g+μ)(acI∗+θ)]
−gν(acI∗+θ)2}+a2bcLI∗{[Hμ(h+μ)+hkI∗]([a2cfLI∗+(g+μ)(acI∗+θ)]}
−(δ+k+μ)I∗(h+μ){[a2bcLI∗+(μ+ν)(acI∗+θ)][a2cfLI∗+(g+μ)(acI∗+θ)]
−gν(acI∗+θ)2}+a2cfLνI∗(acI∗+θ)[Hμ(h+μ)+hkI∗]=0. This simplifies to,
P(I∗)=A3I∗3+A2I∗2+A1I∗+A0=0, | (6.3) |
where
A3 = −a2c2{[(μ+δ)(μ+h)+μk][a2bfL2+abL(μ+g)+afLν]+μ(μ+h)(μ+δ+k)[afL+μ+g+ν]<0,
A2=ac(a(bL(hμ(ac(G+H)(afL+g)+θk)+acμ2(G+H)(afL+g+h)
+acμ3(G+H)+ghθk)+cμ(h+μ)(Gμ(afL+g+μ)+afLν(G+H)
+Gμν)+fhθkLν)−θ(δ+k+μ)(h+μ)(aL(b(g+μ)+f(μ+ν))+2μ(g+μ+ν))),
A1=acθ2μ(h+μ)(g+μ+ν)(δ+k+μ)[R2i−1],
A0=Gθ2μ2(h+μ)(g+μ+ν)>0.
For case (b), the number of positive solutions of Eq (6.3) depends on the signs of A3,A2,A1 and A0. We apply Descartes rule of signs by [28] to investigate the number of sign changes of Eq (6.3), which will be the possible solutions of the polynomial. Possible signs of the coefficients of Eq (6.3) are shown in Table 2. It can be observed that A0>0 and A3<0. With Ri>1, we have A1>0. By the Descartes rule of signs, it follows that P(I∗) has a unique positive root. Thus, the statement (b) is proved.
Case | A3 | A2 | A1 | A0 | Ri | Number of Sign Changes | Number of Positive Roots |
1 | − | + | + | + | >1 | 1 | 1 |
2 | − | − | + | + | >1 | 1 | 1 |
3 | − | + | + | + | >1 | 1 | 1 |
4 | − | − | + | + | >1 | 1 | 1 |
5 | − | + | − | + | <1 | 3 | 1, 3 |
6 | − | − | − | + | <1 | 1 | 1 |
7 | − | + | − | + | <1 | 3 | 1, 3 |
8 | − | − | − | + | <1 | 1 | 1 |
Now, for case (a) with G=0, the polynomial of interest becomes:
Q(I∗)=A3I∗2+A2I∗+A1=0. |
It can be observed that A3<0 with R0>1, thus A1>0. By the Descartes rule of signs, it follows that Q(I∗) has a unique positive root. Thus, the claim (a) is also true.
We first present the case when there is no immigration of infectives, i.e. G=0. This is done by applying a change of variable to system (1.1):
(f1,f2,f3,f4,f5,f6)=(dSdt,dVdt,dIdt,dRdt,dMdt,dJdt)and(S,V,I,R,M,J)=(x1,x2,x3,x4,x5,x6). |
We choose the bifurcation point a=ϕ∗⟹a2=ϕ∗2 and R0=1.
System (1.1) becomes
f1=dx1(t)dt=μH−ϕ∗bx1(t)x6(t)−(μ+ν)x1(t)+gx2(t)+hx4(t)f2=dx2(t)dt=νx1(t)−ϕ∗fx2(t)x6(t)−(μ+g)x2(t)f3=dx3(t)dt=ϕ∗[bx1(t)+fx2(t)]x6(t)−(μ+k+δ)x3(t)f4=dx4(t)dt=kx3(t)−(μ+h)x4(t)f5=dx5(t)dt=θL−ϕ∗cx5(t)x3(t)−θx5(t)f6=dx6(t)dt=ϕ∗cx5(t)x3(t)−θx6(t). | (7.1) |
The Jacobian matrix of system (7.1) evaluated at the disease-free equilibrium point is
J=(−(μ+ν)g0h0−ϕ∗bH(g+μ)g+μ+νν−(g+μ)000−ϕ∗fHνg+μ+ν00−(δ+k+μ)00ϕ∗H(b(g+μ)+fν)g+μ+ν00k−(h+μ)0000−ϕ∗cL0−θ000ϕ∗cL00−θ). |
The eigenvalues of the system at R0=1 are
λ1=−θ,λ2=−(h+μ),λ3=−μ,λ4=−(g+μ+ν),λ5=−(θ+k+δ+μ),λ6=0. |
We note that the system has a simple eigenvalue, while the other eigenvalues have negative real parts. The right eigenvector associated with the simple eigenvalue is given by w=(w1,w2,w3,w4,w5,w6), where
{w1=hk(g+μ)μ(h+μ)(g+μ+ν)−ϕ∗2HcL[b(g+μ)2+νfg]θμ(g+μ+ν)2w2=−ν[hμ+hδ+μδ+μk+μ2]μ(h+μ)(g+μ+ν)w3=1w4=kh+μw5=−ϕ∗cLθw6=ϕ∗cLθ. |
Similarly, the left eigenvector corresponding the simple eigenvalue is given by v=(v1,v2,v3,v4,v5,v6), where
{v1=0v2=0v3=θθ+δ+k+μv4=0v5=0v6=θ(δ+k+μ)ϕ∗cL(θ+δ+k+μ). |
The nonzero second order partial derivatives are:
∂2f3∂x1∂x6=∂2f3∂x6∂x1=ϕ∗b,∂2f3∂x2∂x6=∂2f3∂x6∂x2=ϕ∗f,∂2f6∂x3∂x5=∂2f6∂x5∂x3=ϕ∗c, |
∂2f3∂x6∂ϕ∗=H[bg+bμ+fν]g+μ+ν,∂2f6∂x3∂ϕ∗=cL. |
a=v3[2w1w6ϕ∗b+2w2w6ϕ∗f]+v6[2w3w5ϕ∗c], |
b=v3w6∂2f3∂x6∂ϕ∗+v6w3∂2f6∂x3∂ϕ∗. |
Substituting the values of w1,w2,w3,w5,w6,v3,v6 and evaluating b at the disease-free equilibrium point, we get,
a=[2ϕ∗2cL[θbghk+θbμhk+δfh+μfh+μfk+μfδ+μ2f]θμ(g+μ+ν)2(k+δ+μ+θ)]− |
[2ϕ∗4bc2HL2[b(g+μ)2+fνg]+2ϕ∗cθμ(g+μ+ν)2(k+δ+μ)θμ(g+μ+ν)2(k+δ+μ+θ)]. |
b=v3[w6H(bg+bμ+fν)g+μ+ν+v6w3cL]>0. |
We note that b is always positive. The type of bifurcation of system (1.1) depends on the sign of a.
Theorem 7.1. Following [29],
(i) When a>0, meaning,
[2ϕ∗2cL[θbghk+θbμhk+δfh+μfh+μfk+μfδ+μ2f]θμ(g+μ+ν)2(k+δ+μ+θ)]> |
[2ϕ∗4bc2HL2[b(g+μ)2+fνg]+2ϕ∗cθμ(g+μ+ν)2(k+δ+μ)θμ(g+μ+ν)2(k+δ+μ+θ)], |
system (1.1) undergoes a backward bifurcation when R0=1, a=ϕ∗.
(ii) When a<0, meaning,
[2ϕ∗2cL[θbghk+θbμhk+δfh+μfh+μfk+μfδ+μ2f]θμ(g+μ+ν)2(k+δ+μ+θ)]< |
[2ϕ∗4bc2HL2[b(g+μ)2+fνg]+2ϕ∗cθμ(g+μ+ν)2(k+δ+μ)θμ(g+μ+ν)2(k+δ+μ+θ)], |
system (1.1) undergoes a transcritical bifurcation when R0=1, a=ϕ∗.
Therefore the endemic equilibrium point is locally asymptotically stable when R0>1 but sufficiently close to one.
Now we consider the case G>0.
Since μG is a constant, its partial derivatives will be zero. We inherit the proof of the local dynamics of the endemic equilibrium points when G>0 and the bifurcation analysis of system (1.1) from the center manifold studied for the case when G=0. Thus, the endemic equilibrium point for system (1.1) when G>0 is locally asymptotically stable.
In order to implement control strategies, it is important to know the parameters that influence the basic reproductive number. This will help in providing a clear picture regarding the factors that need to be addressed in order to reduce malaria incidents. In the event of a low budget, sensitivity analysis helps us identify key factors that can be prioritized in the control of a disease. In this section we carry out sensitivity analysis of system (1.1) using the Latin hypercube sampling (LHS) method described in [30,31,32,33], with 1000 simulations per run. The partial rank correlation coefficient (PRCC) is an efficient and reliable sampling-dependent method that performs global sensitivity analysis, which allows us to investigate the monotonicity between parameters of the model and the model output, or in this case, the reproductive number when all parameters are varied [33,34]. In general, a standard correlation coefficient ρ for any two variables, x and y, is calculated using the formula:
ρ=∑i(xi−ˉx)(yi−ˉy)√∑i(xi−ˉx)2∑i(yi−ˉy)2, |
where {(xi,yi):xi∈x,yi∈y} are a set of paired sampled data [33]. The method of PRCCs is explained in detail in [34]. Figure 2 shows the tornado plot of the PRCCs of the parameters of our proposed model.
Parameters with positive PRCCs increase the basic reproductive number R0 when they are increased, while parameters with negative PRCCs decrease the reproductive number when they are increased. Parameter values used are displayed in Table 2. It can be observed that factors such as the mosquito biting rate, probabilities of successful bites leading to both new mosquito infections and human infections are the most sensitive factors. Interventions should be introduced to reduce these factors. On the other hand, factors such as the mosquito mortality rate and vaccination rate are also sensitive to the reproductive number. Increasing these factors will decrease the reproductive number and, hence reduce malaria, provided there is no immigration of infected humans.
The scatter plots shown in Figure 3 show the relationship between the most sensitive parameters and the logR0. It can been seen that the mosquito biting rate and the probability of new mosquito infections are monotonically increasing, meaning if we increase these parameters, the disease will also increase. Similarly, the mosquito mortality rate and vaccination rate monotonically decreasing and increasing their values will lead to a decrease in the number of new cases.
In this section we carry out numerical simulations for our system (1.1). Parameters used in the simulations are obtained from literature, with some being reasonable estimates. The values are listed in Table 2. We start by investigating the impact of immigration of infectives in the population.
One of our objectives is to investigate the impact of influx of infected immigrants into the population. Figure 4 below shows the population of infectious individuals when there is a constant influx of infected immigrants and the case when there is no influx of infected immigrants. The Python notebook for the codes used to produce the plot below can be obtained here.
It can be observed that the population of infectious human beings can be reduced when there is no influx of infected immigrants. In the presence of a constant flow of infected immigrants, the population of infectious humans will increase and malaria will remain a pandemic and a huge threat to human lives. The shaded region between the two graphs indicates the number of infections that can be prevented by curbing or reducing the influx of infected humans.
We investigate the impact of introducing the mosquirix malaria vaccine when there is no influx of infected immigrants. This is done by varying values of ν, the rate at which susceptible humans are vaccinated. Figure 5 below indicates the population of infectious humans in the absence of the influx infected immigrants. The Python notebook for the code used to produce this plot can be obtained here.
Immigration is part of our daily lives. It is difficult for people not to migrate because of other factors that can include poverty and search for greener pastures. We investigate the effectiveness of the malaria vaccine when there is an influx of infected immigrants.
It can be observed that in the presence of infected immigrants, a higher vaccine coverage also helps in reducing malaria infections. This is evidenced by a decrease in the number of cases, as shown in Figure 6. The disease will remain endemic because of the influx of infected humans, but vaccination reduces the rate at which new infections emerge in the population. The code used to produce this plot can be obtained on this link.
In this section we carry out an optimal control analysis of different strategies to control malaria. We wish to choose the best scenario that, if well implemented, could help control the spread of malaria. To investigate the potential impact of the implemented intervention measures, the following control variables are incorporated into our model system:
● p1(t): Represent the use of personal protection measures to prevent mosquito bites during the day and night, such as the use of insecticide-treated nets, application of repellents to skin or spraying of insecticides,
● p2(t): Represent the treatment and
● p3(t): Represent the use of vaccination to prevent malaria.
The model with optimal control is shown in Figure 7 and system (11.1):
dS(t)dt=μH−(1−p1)abS(t)J(t)−(μ+νp3)S(t)+gV(t)+hR(t)dV(t)dt=νp3S(t)−(1−p1)afV(t)J(t)−(μ+g)V(t)dI(t)dt=μG+a(1−p1)[bS(t)+fV(t)]J(t)−(μ+p2k+δ)I(t)dR(t)dt=p2kI(t)−(μ+h)R(t)dM(t)dt=θL−a(1−p1)cM(t)I(t)−θM(t)dJ(t)dt=(1−p1)acM(t)I(t)−θJ(t). | (11.1) |
We want to investigate the impact of the control strategies at different levels of immigration. We consider the following quadratic objective functional:
W(p1,p2,p3)=∫T0[M1I+M2J+M3L+ω12p21+ω22p22+ω32p23]dt. | (11.2) |
The objective functional measures the cost of our proposed strategies from time t=0 to the terminal time t=T. For realism, we need to know the unit cost of the intervention, but for now, the cost is not available and, hence, we base our experiments on nominal values. The constants M1,M2,M3 are the weight constants for the state variables and are positive for all time t, while ω1,ω2,ω3 are the constants for the three strategies. We derive the necessary conditions that must be satisfied by an optimal control problem from the Pontryagin's maximum principle [35,36], which converts system (11.1) and the objective functional defined in Eq (11.2) into the problem of minimizing pointwise Hamiltonian H, with respect to the control variables (p1,p2,p3)∈U at a minimum cost:
W(p∗1,p∗2,p∗3)=minW(p1,p2,p3)|(p1,p2,p3)∈U, |
where U is the set of admissible controls. The Hamiltonian (H) is given by
H=A1I+A2J+A3L+ω12p21+ω22p22+ω32p23+[μH−(1−p1)abS(t)J(t)−(μ+νp3)S(t)+gV(t)+hR(t)]λ1+[νp3S(t)−(1−p1)afV(t)J(t)−(μ+g)V(t)]λ2+[μG+a(1−p1)[bS(t)+fV(t)]J(t)−(μ+p2k+δ)I(t)]λ3+[p2kI(t)−(μ+h)R(t)]λ4+[θL−a(1−p1)cM(t)I(t)−θM(t)]λ5+[(1−p1)acM(t)I(t)−θJ(t)]λ6, | (11.3) |
where the variables λ1,λ2,λ3,λ4,λ5,λ6 are the adjoints to the state variables S,V,I,R,M,J, respectively. The adjoint variables should satisfy
λ′1=−∂H∂S;λ′2=−∂H∂V;λ′3=−∂H∂I;λ′4=−∂H∂R;λ′5=−∂H∂M;λ′6=−∂H∂J, |
implying that:
{λ′1=ab(1−p1)J[λ1−λ3]+νp3[λ1−λ2]+μλ1,λ′2=afJ(1−p1)[λ2−λ3]+g[λ2−λ1]+μλ2,λ′3=ac(1−p1)M(λ5−λ6)−p2kλ4−A1,λ′4=h(λ4−λ1),λ′5=ac(1−p1)I[λ5+λ6]−θλ6,λ′6=ab(1−p1)S[λ1−λ3]+af(1−p1)V(λ2−λ3)+θλ6, |
with transversality conditions
λ1(T)=λ2(T)=λ3(T)=λ4(T)=λ5(T)=λ6(T)=0. |
Moreover, p∗1,p∗2,p∗3 satisfy the conditions
∂H∂p1=0,∂H∂p2=0and∂H∂p3=0, |
implying that;
{abS∗J∗[λ1−λ3]+afV∗J∗[λ2−λ3]+acM∗I∗[λ5−λ6]+p1w1=0,kI∗[λ4−λ3]+w2p2=0,νS∗[λ2−λ1]+w3p3=0, | (11.4) |
in the interior of the control set U. Solving Eq (11.4) for (p1,p2,p3) in U, we get the optimal solution as:
p∗1=min{1,max[0,−afVJ(ξ2−ϵ3)+abSJ(ξ1−ξ3)+acMI(ξ5−ξ6)ω1]}, |
p∗2=min{1,max[0,−kI(ξ4−ξ3)ω2]}, |
p∗3=min{1,max[0,−νS(ξ2−ξ1)ω3]}. |
Using parameters described in Table 1, we obtain the following simulations for the different strategies under consideration. The code used in these plots can be obtained here.
Parameter | Definition | Units | Value/Range | Source |
H | Recruitment of susceptible individuals | day−1 | 3994200 | [11] |
G | Recruitment of infected immigrants | day−1 | [0−100000] | Nominal |
μ | Natural death rate in the human population | day−1 | 0.000041 | Calculated in (2.1) |
ν | Vaccination rate of susceptible individuals | day−1 | [0−1.0] | Nominal |
g | Rate at which vaccinated children become susceptible | day−1 | 0.0026 | Estimated |
a | Mosquito biting rate | day−1 | 0.0030 | Estimated |
θ | Natural death rate of mosquitoes | day−1 | 0.04 | [23] |
b | Probability that a susceptible human become infected | — | 0.009 | Estimated |
c | Probability that a susceptible mosquito becomes infectious | — | 0.00098 | Estimated |
δ | Malaria induced mortality on humans | day−1 | 0.05 | [23] |
f | Probability that a vaccinated human becomes infectious | — | 0.0004 | Estimated |
h | Loss of immunity by recovered humans | day−1 | 0.0010 | [23] |
k | Recovery rate of infectious humans | day−1 | 0.006 | [23] |
It can be observed that even though the use of personal protective equipment, treatment and vaccination of young children can help reduce the burden of malaria, the combined use of these strategies is the best way to achieve better results.
When there is immigration of infected humans, the three strategies do reduce malaria incidence, but malaria remains endemic in the population. Even the combined use of all strategies at the same time is not enough to completely eliminate malaria because all these available strategies are not 100% efficient. Thus, the best strategy is to use the three strategies at the same time, but making sure that immigration of infected humans is stopped.
We have presented a new model for which we have proved that the solutions are well-behaved and the equilibria has good stability properties. Existence of endemic equilibria for system (1.1) was deduced and the local dynamics were proven using the center manifold theorem. When there is immigration, the model had no disease free equilibrium point, indicating that malaria would remain endemic in the population. Numerical simulations were also performed to investigate the effect of immigration in controlling the malaria disease. The results showed that completely stopping the influx of infected immigrants will be very useful in reducing the burden of malaria in malaria endemic regions. We performed a sensitivity analysis to R0 and our results showed that interventions must be introduced with an aim of reducing contacts between mosquitoes and humans. An investigation of the vaccine coverage indicated that when there is no influx of infected immigrants, vaccination will be essential in eliminating malaria. We then analyzed the effectiveness of the vaccine by considering different rates of coverage of the vaccine when there is an influx of immigrants. Results from this analysis showed that a wide coverage rate will reduce infections, although malaria will remain endemic. Following the results from the sensitivity analysis, we introduced optimal control strategies to curb malaria incidence. The strategies proposed are the use of personal protection measures to prevent mosquito bites during the day and night, such as the use of insecticide-treated nets, application of repellents to skin or spraying of insecticides, treatment of infected humans and vaccination of young children below the age of five years. Results from this optimal control showed that the combined use of the three strategies is the best way to reduce malaria incidence, provided the influx of infected humans is completely stopped. In conclusion, it is evident that a malaria free population can be achieved when more children get vaccinated, infected people get treatment, people living in malaria endemic regions continue using personal protective measures that prevent mosquito bites during the day and night and when immigration of infected humans is completely stopped. The modeling can be improved by also considering the effect of climatic factors on malaria dynamics. Several such models, but without vaccination or immigration of infected humans, are already in the literature, e.g., [19,20,37]. Also, when malaria spreads within a population, individuals gain knowledge and a better understanding of the disease. Thus, this work can be extended by means of investigating the memory effect on the dynamics of malaria, using fractional order differential equations described in [38,39,40,41]. It is also interesting to consider stochastic models and their optimal control, as described in [42].
The authors declare they have not used any Artificial Intelligence (AI) tools in the creation of this article.
Pride Duve would like to acknowledge the African Institute for Mathematical Sciences (AIMS) South Africa for the scholarship he obtained during his Masters degree studies, which made it possible for him to carry out this work. Furthermore, the project is funded by the Federal Ministry of Education and Research of Germany (BMBF) under the project NEED (Grant Number 01Kl2022) and the German Research Foundation (JO 1276/5-1).
All authors declare no conflicts of interest in this paper.
[1] | J. Vinetz, What to Know About Malaria, 2022. Available from: https://www.medicalnewstoday.com/articles/150670 |
[2] | Centers for Disease Control and Prevention, Frequently Asked Questions, 2022. Available from: https://www.cdc.gov/malaria/about/faqs.html |
[3] | Cleveland Clinic, Malaria Overview, 2022. Available from: https://my.clevelandclinic.org/health/diseases/15014-malaria |
[4] | World Health Organization, Frequently Asked Questions, 2022. Available from: https://www.who.int/health-topics/malaria |
[5] |
S. Looareesuwan, J. D. Chulay, C. J. Canfield, D. B. Hutchinson, Malarone (atovaquone and proguanil hydrochloride): A review of its clinical development for treatment of malaria. Malarone Clinical Trials Study Group, Am. J. Trop. Med. Hyg., 60 (1999), 533–541. https://doi.org/10.4269/ajtmh.1999.60.533 doi: 10.4269/ajtmh.1999.60.533
![]() |
[6] |
S. Dini, S. Zaloumis, P. Cao, R. N. Price, J. Freya, I. Fowkes, et al., Investigating the efficacy of triple artemisinin-based combination therapies for treating Plasmodium falciparum malaria patients using mathematical modeling, Antimicrob. Agents Chemother., 62 (2018). https://doi.org/10.1128/aac.01068-18 doi: 10.1128/aac.01068-18
![]() |
[7] |
R. Ross, An application of the theory of probabilities to the study of a priori pathometry, Proc. R. Soc. London, 92 (1916), 204–230. https://dx.doi.org/10.1098/rspa.1916.0007 doi: 10.1098/rspa.1916.0007
![]() |
[8] |
G. Macdonald, Epidemiological basis of malaria control, Bull. W. H. O., 15 (1956), 613. https://dx.doi.org/10.1098/rspa.1916.0007 doi: 10.1098/rspa.1916.0007
![]() |
[9] |
J. Mohammed-Awel, E. Numfor, R. Zhao, S. Lenhart, A new mathematical model studying imperfect vaccination: Optimal control analysis, J. Math. Anal. Appl., 500 (2021), 125132. https://doi.org/10.1016/j.jmaa.2021.125132 doi: 10.1016/j.jmaa.2021.125132
![]() |
[10] |
S. Y. Tchoumi, C. W. Chukwu, M. L. Diagne, H. Rwezaura, M. L. Juga, J. M. Tchuenche, Optimal control of a two? Group malaria transmission model with vaccination, Network Model. Anal. Health Inf. Bioinf., 12 (2022), 7. https://doi.org/10.1007/s13721-022-00403-0 doi: 10.1007/s13721-022-00403-0
![]() |
[11] |
P. Witbooi, G. Abiodun, M. Nsuami, A model of malaria population dynamics with migrants, Math. Biosci. Eng., 18 (2021), 7301–7317. https://dx.doi.org/10.3934/mbe.2021361 doi: 10.3934/mbe.2021361
![]() |
[12] |
A. Traoré, Analysis of a vector-borne disease model with human and vectors immigration, J. Appl. Math. Comput., 64 (2020), 411–428. https://doi.org/10.1007/s12190-020-01361-4 doi: 10.1007/s12190-020-01361-4
![]() |
[13] |
D. Heppnerjr, K. Kester, C. Ockenhouse, N. Tornteporth, O. Ofori, J. Lyon, et al., Towards an RTS, S-based, multi-stage, multi-antigen vaccine against falciparum malaria: Progress at the Walter Reed Army Institute of Research, Vaccine, 23 (2005), 2243–2250. http://dx.doi.org/10.1016/j.vaccine.2005.01.142 doi: 10.1016/j.vaccine.2005.01.142
![]() |
[14] |
M. De la Sen, S. Alonso-Quesada, A. Ibeas, On the stability of an SEIR epidemic model with distributed time-delay and a general class of feedback vaccination rules, Appl. Math. Comput., 270 (2015), 953–976. https://dx.doi.org/10.1016/j.amc.2015.08.099 doi: 10.1016/j.amc.2015.08.099
![]() |
[15] |
S. Dhiman, Are malaria elimination efforts on right track? An analysis of gains achieved and challenges ahead, Infect. Dis. Poverty, 17 (2019), 1–19. https://doi.org/10.1186/s40249-019-0524-x doi: 10.1186/s40249-019-0524-x
![]() |
[16] |
European Medicines Agency, First malaria vaccine receives positive scientific opinion, Pharm. J., 2015 (2015). http://dx.doi.org/10.1211/pj.2015.20069061 doi: 10.1211/pj.2015.20069061
![]() |
[17] | World Health Organization, WHO Malaria Policy Advisory Committee (MPAC) Meeting: Meeting Report, 2022. Available from: https://apps.who.int/iris/handle/10665/312198 |
[18] |
World Health Organization, Malaria vaccine: WHO position paper–January 2016, Vaccine, 36 (2018), 3576–3577. https://dx.doi.org/10.1016/j.vaccine.2016.10.047 doi: 10.1016/j.vaccine.2016.10.047
![]() |
[19] |
G. J. Abiodun, P. Witbooi, K. O. Okosun, Modeling and analyzing the impact of temperature and rainfall on mosquito population dynamics over Kwazulu-Natal, South Africa, Int. J. Biomath., 10 (2017), 1750055. https://doi.org/10.1142/S1793524517500553 doi: 10.1142/S1793524517500553
![]() |
[20] |
K. Okuneye, A. B. Gumel, Analysis of a temperature and rainfall dependent model for malaria transmission dynamics, Math. Biosci., 287 (2017), 72–92. https://doi.org/10.1016/j.mbs.2016.03.013 doi: 10.1016/j.mbs.2016.03.013
![]() |
[21] |
S. M. Ndiaye, E. M. Parilina, An epidemic model of malaria without and with vaccination. Pt 2. A model of malaria with vaccination, Appl. Math. Comput. Sci. Control Process., 18 (2022), 555–567. https://dx.doi.org/10.21638/11701/spbu10.2022.410 doi: 10.21638/11701/spbu10.2022.410
![]() |
[22] | World Health Organization, Life Expectancy and Healthy Life Expectancy Data by Country, 2020. Available from: https://apps.who.int/gho/data/node.main.688 |
[23] |
G. Otieno, J. K. Koske, J. M. Mutiso, Transmission dynamics and optimal control of malaria in Kenya, Discrete Dyn. Nat. Soc., Hindawi Ltd., 2016 (2016), 1–27. https://doi.org/10.1155/2016/8013574 doi: 10.1155/2016/8013574
![]() |
[24] | M. W. Hirsch, S. Smale, R. L. Devaney, Discrete dynamical systems, in Differential Equations, Dynamical Systems, and an Introduction to Chaos, Academic Press, (2013), 329–-359. https://dx.doi.org/10.1016/b978-0-12-382010-5.00015-4 |
[25] |
P. van den Driessche, J. Watmough, Reproduction numbers and sub-threshold endemic equilibria for compartmental models of disease transmission, Math. Biosci., 180 (2002), 29–48. https://doi.org/10.1016/S0025-5564(02)00108-6 doi: 10.1016/S0025-5564(02)00108-6
![]() |
[26] | L. Allen, An Introduction to Mathematical Biology, Prentice Hall, 2007. |
[27] | J. P. La Salle, The Stability of Dynamical Systems, Society for Industrial and Applied Mathematics, 1976. https://dx.doi.org/10.1137/1.9781611970432 |
[28] | R. Descartes, La Géométrie, livre premier, édition 1637 publiée dans The geometry of Rene Descartes de David Eugene Smith et Marcia L, 1637. |
[29] |
C. Castillo-Chavez, B. Song, Dynamical models of tuberculosis and their applications, Math. Biosci. Eng., 1 (2004), 361–404. https://dx.doi.org/10.3934/mbe.2004.1.361 doi: 10.3934/mbe.2004.1.361
![]() |
[30] |
S. M. Blower, H. Dowlatabadi, Sensitivity and uncertainty analysis of complex models of disease transmission: An HIV model, as an example, Int. Stat. Rev., 62 (1994), 229–243. https://dx.doi.org/10.2307/1403510 doi: 10.2307/1403510
![]() |
[31] |
S. M. Blower, D. Hartel, H. Dowlatabadi, R. M. Anderson, R.M. May, Sex and HIV: A mathematical model for New York City, Philos. Trans. R. Soc. London Ser. B Biol. Sci., 331 (1991), 171–187. https://dx.doi.org/10.1098/rstb.1991.0006 doi: 10.1098/rstb.1991.0006
![]() |
[32] |
A. Hoare, D. G. Regan, D. P. Wilson, Sampling and sensitivity analyses tools (SaSAT) for computational modelling, Theor. Biol. Med. Modell., 5 (2008), 1742–4682. https://dx.doi.org/10.1186/1742-4682-5-4 doi: 10.1186/1742-4682-5-4
![]() |
[33] |
J. Wu, R. Dhingra, M. Gambhir, J. V. Remais, Sensitivity analysis of infectious disease models: Methods, advances and their application, J. R. Soc. Interface, 10, (2013), 1742–5662. https://dx.doi.org/10.1098/rsif.2012.1018 doi: 10.1098/rsif.2012.1018
![]() |
[34] |
S. Marino, I. B. Hogue, C. J. Ray, D. E. Kirschner, A methodology for performing global uncertainty and sensitivity analysis in systems biology, J. Theor. Biol., 254 (2008), 178–196. https://dx.doi.org/10.1016/j.jtbi.2008.04.011 doi: 10.1016/j.jtbi.2008.04.011
![]() |
[35] | L. S. Pontryagin, Mathematical Theory of Optimal Processes, CRC press, 1987. |
[36] | L. S. Pontryagin, V. G. Boltyanskii, R. V. Gamkrelidze, E. F. Mischenko, The Mathematical Theory of Optimal Processes, Cambridge University Press, 1963. |
[37] |
A. Y. Mukhtar, J. B. Munyakazi, R. Ouifki, Assessing the role of climate factors on malaria transmission dynamics in South Sudan, Math. Biosci., 310 (2019), 13–23. https://doi.org/10.1016/j.mbs.2019.01.002 doi: 10.1016/j.mbs.2019.01.002
![]() |
[38] |
K. Shah, M. Arfan, A. Ullah, Q. Al-Mdallal, K. J. Ansari, T. Abdeljawad, Computational study on the dynamics of fractional order differential equations with applications, Chaos Solitons Fractals, 157 (2022), 111955. https://dx.doi.org/10.1016/j.chaos.2022.111955 doi: 10.1016/j.chaos.2022.111955
![]() |
[39] |
K. Hattaf, A new class of generalized fractal and fractal-fractional derivatives with non-singular kernels, Fractal Fractional, 5 (2023), 395. https://dx.doi.org/10.3390/fractalfract7050395 doi: 10.3390/fractalfract7050395
![]() |
[40] |
M. Semlali, K. Hattaf, E. K. Mohamed, Modeling and analysis of the dynamics of COVID-19 transmission in presence of immigration and vaccination, Commun. Math. Biol. Neurosci., 2002 (2022). https://dx.doi.org/10.28919/cmbn/7270 doi: 10.28919/cmbn/7270
![]() |
[41] |
N. Sene, SIR epidemic model with Mittag-Leffler fractional derivative, Chaos Solitons Fractals, 137 (2020), 109833. https://dx.doi.org/10.1016/j.chaos.2020.109833 doi: 10.1016/j.chaos.2020.109833
![]() |
[42] |
P. J. Witbooi, S. M. Vyambwera, G. J. van Schalkwyk, G. E. Muller, Stability and control in a stochastic model of malaria population dynamics, Adv. Contin. Discrete Models, 1 (2023), 45. https://dx.doi.org/10.1186/s13662-023-03791-3 doi: 10.1186/s13662-023-03791-3
![]() |
1. | Muhammad Asad Ullah, Nauman Raza, Mohammad Y. Alshahrani, Andrew Omame, Analysis and interpretation of a novel malaria transmission mathematical model with socioeconomic structure, 2024, 0924-090X, 10.1007/s11071-024-10807-4 | |
2. | Nnaemeka Stanley Aguegboh, Walter Okongo, Diallo Boubacar, Munkaila Dasumani, Kingsley Chinedu Nnamaga, Daniel Ugochukwu Nnaji, Netochukwu Onyiaji, Anthonia Uchenna Nwachukwu, A novel approach to modeling malaria with treatment and vaccination as control strategies in Africa using the Atangana–Baleanu derivative, 2025, 11, 2363-6203, 10.1007/s40808-024-02273-z | |
3. | Esaie Naroum, Ebenezer Maka Maka, Hamadjam Abboubakar, Paul Dayang, Appolinaire Batoure Bamana, Benjamin Garga, Hassana Daouda Daouda, Mohsen Bakouri, Ilyas Khan, Comparative analysis of deep learning and machine learning techniques for forecasting new malaria cases in Cameroon’s Adamaoua region, 2025, 11, 26665212, 100220, 10.1016/j.ibmed.2025.100220 | |
4. | Kamel Guedri, Rahat Zarin, Mowffaq Oreijah, Samaher Khalaf Alharbi, Hamiden Abd El-Wahed Khalifa, Modeling syphilis progression and disability risk with neural networks, 2025, 314, 09507051, 113220, 10.1016/j.knosys.2025.113220 | |
5. | Rahat Zarin, Usa Wannasingha Humphries, Analyzing spatial diffusion and vaccination strategies in malaria epidemics: a numerical approach, 2025, 11, 2363-6203, 10.1007/s40808-025-02315-0 |
Case | A3 | A2 | A1 | A0 | Ri | Number of Sign Changes | Number of Positive Roots |
1 | − | + | + | + | >1 | 1 | 1 |
2 | − | − | + | + | >1 | 1 | 1 |
3 | − | + | + | + | >1 | 1 | 1 |
4 | − | − | + | + | >1 | 1 | 1 |
5 | − | + | − | + | <1 | 3 | 1, 3 |
6 | − | − | − | + | <1 | 1 | 1 |
7 | − | + | − | + | <1 | 3 | 1, 3 |
8 | − | − | − | + | <1 | 1 | 1 |
Parameter | Definition | Units | Value/Range | Source |
H | Recruitment of susceptible individuals | day−1 | 3994200 | [11] |
G | Recruitment of infected immigrants | day−1 | [0−100000] | Nominal |
μ | Natural death rate in the human population | day−1 | 0.000041 | Calculated in (2.1) |
ν | Vaccination rate of susceptible individuals | day−1 | [0−1.0] | Nominal |
g | Rate at which vaccinated children become susceptible | day−1 | 0.0026 | Estimated |
a | Mosquito biting rate | day−1 | 0.0030 | Estimated |
θ | Natural death rate of mosquitoes | day−1 | 0.04 | [23] |
b | Probability that a susceptible human become infected | — | 0.009 | Estimated |
c | Probability that a susceptible mosquito becomes infectious | — | 0.00098 | Estimated |
δ | Malaria induced mortality on humans | day−1 | 0.05 | [23] |
f | Probability that a vaccinated human becomes infectious | — | 0.0004 | Estimated |
h | Loss of immunity by recovered humans | day−1 | 0.0010 | [23] |
k | Recovery rate of infectious humans | day−1 | 0.006 | [23] |
Case | A3 | A2 | A1 | A0 | Ri | Number of Sign Changes | Number of Positive Roots |
1 | − | + | + | + | >1 | 1 | 1 |
2 | − | − | + | + | >1 | 1 | 1 |
3 | − | + | + | + | >1 | 1 | 1 |
4 | − | − | + | + | >1 | 1 | 1 |
5 | − | + | − | + | <1 | 3 | 1, 3 |
6 | − | − | − | + | <1 | 1 | 1 |
7 | − | + | − | + | <1 | 3 | 1, 3 |
8 | − | − | − | + | <1 | 1 | 1 |
Parameter | Definition | Units | Value/Range | Source |
H | Recruitment of susceptible individuals | day−1 | 3994200 | [11] |
G | Recruitment of infected immigrants | day−1 | [0−100000] | Nominal |
μ | Natural death rate in the human population | day−1 | 0.000041 | Calculated in (2.1) |
ν | Vaccination rate of susceptible individuals | day−1 | [0−1.0] | Nominal |
g | Rate at which vaccinated children become susceptible | day−1 | 0.0026 | Estimated |
a | Mosquito biting rate | day−1 | 0.0030 | Estimated |
θ | Natural death rate of mosquitoes | day−1 | 0.04 | [23] |
b | Probability that a susceptible human become infected | — | 0.009 | Estimated |
c | Probability that a susceptible mosquito becomes infectious | — | 0.00098 | Estimated |
δ | Malaria induced mortality on humans | day−1 | 0.05 | [23] |
f | Probability that a vaccinated human becomes infectious | — | 0.0004 | Estimated |
h | Loss of immunity by recovered humans | day−1 | 0.0010 | [23] |
k | Recovery rate of infectious humans | day−1 | 0.006 | [23] |