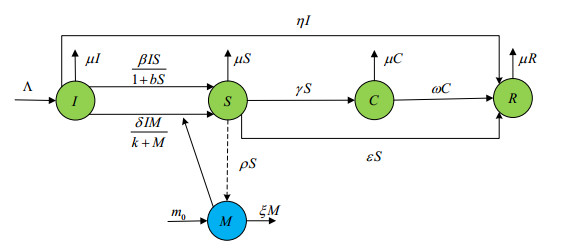
Citation: Yu-Ying Wang, Ah-Fur Lai, Rong-Kuan Shen, Cheng-Ying Yang, Victor R.L. Shen, Ya-Hsuan Chu. Modeling and verification of an intelligent tutoring system based on Petri net theory[J]. Mathematical Biosciences and Engineering, 2019, 16(5): 4947-4975. doi: 10.3934/mbe.2019250
[1] | Peter W. Bates, Jianing Chen, Mingji Zhang . Dynamics of ionic flows via Poisson-Nernst-Planck systems with local hard-sphere potentials: Competition between cations. Mathematical Biosciences and Engineering, 2020, 17(4): 3736-3766. doi: 10.3934/mbe.2020210 |
[2] | Yiwei Wang, Mingji Zhang . Finite ion size effects on I-V relations via Poisson-Nernst-Planck systems with two cations: A case study. Mathematical Biosciences and Engineering, 2024, 21(2): 1899-1916. doi: 10.3934/mbe.2024084 |
[3] | Dandan Fan, Dawei Li, Fangzheng Cheng, Guanghua Fu . Effects of congestion charging and subsidy policy on vehicle flow and revenue with user heterogeneity. Mathematical Biosciences and Engineering, 2023, 20(7): 12820-12842. doi: 10.3934/mbe.2023572 |
[4] | Tien-Wen Sung, Wei Li, Qiaoxin Liang, Chuanbo Hong, Qingjun Fang . Research on charging behavior of electric vehicles based on multiple objectives. Mathematical Biosciences and Engineering, 2023, 20(9): 15708-15736. doi: 10.3934/mbe.2023700 |
[5] | Chii-Dong Ho, Jr-Wei Tu, Hsuan Chang, Li-Pang Lin, Thiam Leng Chew . Optimizing thermal efficiencies of power-law fluids in double-pass concentric circular heat exchangers with sinusoidal wall fluxes. Mathematical Biosciences and Engineering, 2022, 19(9): 8648-8670. doi: 10.3934/mbe.2022401 |
[6] | Virginia González-Vélez, Amparo Gil, Iván Quesada . Minimal state models for ionic channels involved in glucagon secretion. Mathematical Biosciences and Engineering, 2010, 7(4): 793-807. doi: 10.3934/mbe.2010.7.793 |
[7] | Mette S. Olufsen, Ali Nadim . On deriving lumped models for blood flow and pressure in the systemic arteries. Mathematical Biosciences and Engineering, 2004, 1(1): 61-80. doi: 10.3934/mbe.2004.1.61 |
[8] | Guichen Lu, Zhengyi Lu . Permanence for two-species Lotka-Volterra cooperative systems with delays. Mathematical Biosciences and Engineering, 2008, 5(3): 477-484. doi: 10.3934/mbe.2008.5.477 |
[9] | Bindu Kumari, Chandrashekhar Sakode, Raghavendran Lakshminarayanan, Prasun K. Roy . Computational systems biology approach for permanent tumor elimination and normal tissue protection using negative biasing: Experimental validation in malignant melanoma as case study. Mathematical Biosciences and Engineering, 2023, 20(5): 9572-9606. doi: 10.3934/mbe.2023420 |
[10] | Suqing Lin, Zhengyi Lu . Permanence for two-species Lotka-Volterra systems with delays. Mathematical Biosciences and Engineering, 2006, 3(1): 137-144. doi: 10.3934/mbe.2006.3.137 |
A rumor refers to unsubstantiated information, which has the potential to rapidly and extensively disseminate through various communication channels [1]. In the past, rumors were disseminated through oral communication as well as various forms of print media, radio broadcasts, and other mediums. Contemporary social networks can function as an appropriate platform for the rumor propagation, while also satisfying individuals' informational needs [2]. For example, individuals' excessive salt consumption can be attributed to the panic of the Fukushima nuclear accident-related rumor. There have been numerous rumors that emerged a range of adverse impacts on the world since the outbreak of COVID-19 [3]. The security of individuals and their belongings is significantly compromised due to these rumors. Therefore, it is imperative to grasp the rules of communicating with scientists and create effective methods to intervene, defend, and prevent rumors from spreading.
Since rumor spreading is the analogy to the disease's transmission among individuals, many scholars employ similar rumor models. Early studies on rumor propagation focused a tiny community through individual interaction. According to rumor propagation, the crowd can be classified into three categories: ignorant, spreader, and stifler. In 1964, Daley and Kendall proposed the classic DK rumor-spreading model, which is the first cabin model in rumor propagation [4]. Furthermore, in 1973, Maki and Thompson proposed the MT model as an extension of the DK model [5]. According to the MT model, once interpersonal communication occurs, the former will no longer propagate. According to several scholars, the complexity of rumor propagation processes cannot be adequately captured by DK and MK models across various network settings, especially in the context of large-scale social networks. Zanette proposed a conceptual framework to simulate the dynamics of rumor propagation in small-world networks [6]. By integrating the MK model and the SIR model, Moreno et al. developed a novel rumor propagation model, demonstrating that network topologies can significantly influence the spread of rumors on scale-free networks [7]. To gain a deeper comprehension of rumor propagation, an increasing number of factors are being taken into account through the development of more rational models; for instance, hesitating mechanisms [8], inhibiting mechanisms, and attitude adjustment [9], etc. The innovations of these scholars, to a certain extent, promote rumor propagation to advance research under a variety of changing factors.
Nowadays, given the multiple channels of rumor propagation, individuals can quickly obtain information on social media platforms, thereby complicating the regulation of rumor propagation. Due to the significant damage caused by rumors, several control measures aimed at minimizing losses have been proposed by scholars; for instance, optimal control strategies [10], time-dependent controller [11], event-triggered control [12], and so on. In reality, when rumors prevail on social media platforms, the media coverage disseminates information to debunk these rumors and intervene in individuals' behavior, thereby mitigating social panic and upholding social harmony. Cheng et al. incorporated a nonlinear factor into the contact rate by integrating optimal control and time delay [13]. Huo et al. introduced a nonlinear function to effectively capture the impact of misinformation and media reports on the spread of rumors [14]. Pan et al. investigated a novel rumor model that integrated media coverage and rumor refuters [15]. Further on, Guo et al. proposed two models considering media coverage and a refutation mechanism, respectively [16,17]. Numerous mathematical models are available to describe how media coverage affects rumor propagation. Therefore, our proposed model must account for the impact of media coverage on the dissemination of rumors among individuals. Evidently, the aforementioned models have predominantly explored the propagation mechanism of rumors in an integer order.
However, the dynamic behavior of rumors is influenced by historical information. Fractional calculus, as an extension of integral calculus, provides innovative approaches for characterizing the memory effects, which is a generalization of integral calculus. Fractional order Caputo derivatives, extensively employed in various fields such as science, biology, engineering, computer science, chaos theory, and others, have witnessed significant advancements in recent decades [18,19,20,21,22,23,24,25]. The literature offers a wide range of models described by fractional derivatives, such as the SIR or SIRS model [26,27], the SEI model [28], the SEIR model [29,30,31], the SIQR model [32] and the SEIQRS model [33]. Rumor diffusion is similar to virus propagation and can be effectively modeled using fractional calculus. Singh proposed the dynamics of a rumor propagation in a social network, utilizing the Atangana-Baleanu derivative [34]. Ye et al. investigated a fractional-order reaction-diffusion rumor model in two linguistic contexts [35]. The impact of memory was investigated, and the findings clearly demonstrate that fractional calculus can be employed to more accurately characterize the process of rumor propagation.
Revisiting the findings of the previous study, we establish an Ignorants-Spreaders-Calmness-Removers-Media Reports (ISCRM) rumor propagation model with media reports under the Caputo sense by incorporating the memory effect. The subsequent sections of this manuscript are organized as follows: In Section 2, we provide a concise overview of fundamental definitions pertaining to fractional calculus. In Section 3, we propose an enhanced fractional-order ISCRM rumor model. In Section 4, the local and global asymptotic stability of the equilibria is investigated. In Section 5, the optimal control problem is addressed. In Section 6, numerical simulation is examined. In Section 7, the paper culminates with the presentation of our findings.
Various definitions exist regarding fractional derivatives, with the Caputo sense being provided as follows [36].
Definition 2.1. For Riemann-Liouville fractional integration with respect to order α, the function f(t) is presented as
t0Iαtf(t)=D−αtf(t)=1Γ(α)∫tt0f(τ)(t−τ)1−αdτ, | (2.1) |
where α∈R+, t0 represents the initial time, and Γ(⋅), which is established by Γ(z)=∫∞t0tz−1e−tdt, represents the Gamma function.
Definition 2.2. Define l−1<α⩽l, l∈N. The Riemann-Liouville fractional derivative with respect to order α is presented as [36]
RLt0Dαtf(t)=dαf(t)dtα=1Γ(m−α)dldtl∫tt0f(τ)(t−τ)α−l+1dτ, | (2.2) |
Remark 2.1. The combination of Definitions 2.1 and 2.2 yields RLt0Dαt(t0Iαtf(t))=f(t), giving the Riemann-Liouville fractional integrations and derivatives with respect to order α.
Definition 2.3. The Caputo fractional derivative with respect to order α is presented as follows:
Ct0Dαtf(t)={1Γ(l−α)∫tt0fl(τ)(t−τ)α−l+1dτ, l−1<α<l,dldtlf(t),α=l, | (2.3) |
where l denotes the smallest integer number such that α<l.
Remark 2.2. The equivalence between the definitions of Riemann-Liouville fractional integration and Caputo fractional integration are the same. Therefore, from Definitions 2.1 and 2.3, we obtain
Ct0Dαt(t0Iαtf(t))=f(t), | (2.4) |
giving the Caputo fractional integrations and derivatives with respect to order α.
In the subsequent sections of this paper, the notation Dα represents the Riemann-Liouville fractional derivative.
Lemma 2.1. Provide a definition for a function f(t) and a nonzero interval t∈(0,T), the condition |f(t)|⩾F1, where F1>0, is a constant. Next, a positive constant is given L=F1TαΓ(1+α). Thus, the inequality 0Iαt1|f(t)|⩾L is held for t∈(0,t1), t1⩾T.
Proof. The equality is evidently apparent:
0Iαt1|f(t)|=1Γ(α)∫t10|f(τ)|(t−τ)1−αdτ. | (2.5) |
By referring to Eq (2.1), which defines fractional integration, the value of 0Iαt1|f(t)| can be determined. Due to the condition |f(t)|⩾F1, for at least one nonzero interval t∈(0,T), it acquires
0Iαt1|f(t)|=1Γ(α)∫t10|f(τ)|(t−τ)1−αdτ⩾1Γ(α)∫T0|f(τ)|(t−τ)1−αdτ⩾F1Γ(α)∫T01(t−τ)1−αdτ=F1TαΓ(1+α). | (2.6) |
Therefore, the inequality 0Iαt1|f(t)|⩾F1TαΓ(1+α) holds for f(t).
Definition 2.4. Let F(τ) be the Laplace transform of the function f(t). Then, the Caputo fractional derivative Laplace transform is provided as [37]
L[Dαf(t)]=ταF(τ)−n−1∑i=0f(i)(0)τα−n−1, n−1<α⩽n, n∈N. | (2.7) |
Definition 2.5. The expressions for the Mittag-Leffler functions in both one-parameter and two-parameter forms are provided as [36]
Eα(z)=∞∑k=0zkΓ(αk+1), Eα,l(z)=∞∑k=0zkΓ(αk+l), | (2.8) |
where z,α,β∈C.
Theorem 2.1. [36] Consider the autonomous linear fractional-order equation Dαx=Ax, x(0)=x0, where 0<α<1, x∈Rn and A∈Rn×n. The asymptotic stability of this system implies that
|arg(eigA)|⩾απ2. | (2.9) |
In this scenario, each component of the state's decays toward 0 as t−α.
Theorem 2.2. [38] Let x=0 denote an equilibrium point for the equilibrium of the non-autonomous fractional-order equation
Dαx(t)=f(x,t), | (2.10) |
where f(x,t) satisfies the Lipschitz constant l>0 and α∈(0,1). Provided that it constitutes a Lyapunov function V(t,x(t)) and class-K functions α1, α2 and α3 satisfying the condition
α1(‖ |
where \beta \in \left({0, 1} \right) , the equilibrium point of Eq (2.10) is asymptotic stable.
The proposed framework in this section is an Ignorants-Spreaders-Calmness-Removers-Media Reports (ISCRM). All of the crowd are divided into four groups: Ignorants are those who are unfamiliar with the rumors but are willing to believe them, denoted by I\left(t \right) ; Spreaders are those who acquire the rumors and spread them, denoted by S\left(t \right) ; Calmness are those who calm down before they refrain from disseminating rumors [39], denoted by C\left(t \right) ; Removers are those who are aware of the rumors and either refute them or have become disinterested in rumor, denoted by R\left(t \right) . Since rumor propagation varies over time, media reports will undergo changes over time. We employ a quantization method to measure the impact of media reports, denoted by M\left(t \right).
The rules for rumor propagation are as follows:
(ⅰ) When an ignorant contacts a spreader, it will be affected and become a spreader with the exposure rate of \beta /\left({1 + bS} \right) , which reflects the reduced amount of contact rate due to the scale of the rumor propagation. \beta denotes the rumor contact rate and b is the measure of inhibition.
(ⅱ) \delta /\left({k + M} \right) represents the influence of the media reports, where \delta > 1 and k \geqslant 0 . \delta /k means the highest availability of media influence effect. \delta represents the rate of contact between the ignorant and the media reports and k is the effect of media reports. \gamma represents calm down rate of the spreader. \varepsilon and \omega represent the forgetting rates.
(ⅲ) We define that the immigrate rate is \Lambda and the emigrate rate is \mu in our study. Furthermore, \eta represents the influence of rumors under the rumor control measures. {m_0} represents the initial value of the media reports. \rho represents the influence of rumor propagation on media reports. The parameters of the model are assumed to be constant and positive.
The mathematical dynamics of rumor propagation under the Caputo sense is derived based on the propagation rules in Figure 1 as follows:
\begin{array}{l} {D^\alpha }I\left( t \right) = \Lambda - \frac{{\beta I\left( t \right)S\left( t \right)}}{{1 + bS\left( t \right)}} - \frac{{\delta I\left( t \right)M\left( t \right)}}{{k + M\left( t \right)}} - \eta I\left( t \right) - \mu I\left( t \right), \\ {D^\alpha }S\left( t \right) = \frac{{\beta I\left( t \right)S\left( t \right)}}{{1 + bS\left( t \right)}} + \frac{{\delta I\left( t \right)M\left( t \right)}}{{k + M\left( t \right)}} - \gamma S\left( t \right) - \varepsilon S\left( t \right) - \mu S\left( t \right), \\ {D^\alpha }C\left( t \right) = \gamma S\left( t \right) - \omega C\left( t \right) - \mu C\left( t \right), \\ {D^\alpha }R\left( t \right) = \eta I\left( t \right) + \varepsilon S\left( t \right) + \omega C\left( t \right) - \mu R\left( t \right), \\ {D^\alpha }M\left( t \right) = {m_0} + \rho S\left( t \right) - \xi M\left( t \right), \\ \end{array} | (3.1) |
where the initial conditions are denoted by \left({I\left(0 \right), S\left(0 \right), C\left(0 \right), R\left(0 \right), M\left(0 \right)} \right) = \left({{I_0}, {S_0}, {C_0}, {R_0}, {M_0}} \right) .
In this model, the non-negative initial conditions of Eq (3.1) are obtained as
I\left( 0 \right) = {I_0} \geqslant 0, {\text{ }}S\left( 0 \right) = {S_0} \geqslant 0, {\text{ }}C\left( 0 \right) = {C_0} \geqslant 0, {\text{ }}R\left( 0 \right) = {R_0} \geqslant 0, {\text{ }}M\left( 0 \right) = {M_0} \geqslant 0. | (3.2) |
Theorem 3.1. The solution of the Caputo sense Eq (3.1) subject to the conditions (3.2) is non-negative within the closed set
\Omega = \left\{ {\left( {I, S, C, R, M} \right) \in R_ + ^4:N\left( t \right) = I\left( t \right) + S\left( t \right) + C\left( t \right)} \right. \left. { + R\left( t \right) \leqslant \frac{\Lambda }{\mu }, M\left( t \right) \leqslant \frac{{{m_0}}}{\xi } + \frac{{\rho \Lambda }}{{\mu \xi }}} \right\}. |
Proof. For Caputo fractional derivatives (3.1), one obtains
\left\{ \begin{array}{l} {\left. {{D^\alpha }I\left( t \right)} \right|_{I\left( t \right) = 0}} = \Lambda \geqslant 0, \\ {\left. {{D^\alpha }S\left( t \right)} \right|_{S\left( t \right) = 0}} = \frac{{\delta I\left( t \right)M\left( t \right)}}{{k + M\left( t \right)}} \geqslant 0, \\ {\left. {{D^\alpha }S\left( t \right)} \right|_{S\left( t \right) = 0}} = \gamma S\left( t \right) \geqslant 0, \\ {\left. {{D^\alpha }R\left( t \right)} \right|_{R\left( t \right) = 0}} = \eta I\left( t \right) + \varepsilon S\left( t \right) + \omega C\left( t \right) \geqslant 0, \\ {\left. {{D^\alpha }M\left( t \right)} \right|_{M\left( t \right) = 0}} = {m_0} + \rho S\left( t \right) \geqslant 0. \\ \end{array} \right. | (3.3) |
Based on the Laplace transform, it can be demonstrated that I, S, C, R, M \geqslant 0, {\text{ }}t \in \left[{{t_0}, T} \right). Additionally, the total population is determined as N\left(t \right) = I\left(t \right) + S\left(t \right) + C\left(t \right) + R\left(t \right) . Thus, adding the first four equations of Eq (3.1), we obtain
\begin{array}{l} {}_0^CD_t^\alpha N\left( t \right) = \Lambda - \mu \left( {I\left( t \right) + S\left( t \right) + C\left( t \right) + R\left( t \right)} \right) \\ \;\;\;\;\;\;\;\;\;\;\;\;\;\; = \Lambda - \mu N\left( t \right). \\ \end{array} |
By employing the Laplace transform, we obtain N\left(t \right) \leqslant N\left(0 \right){E_\alpha }\left({ - \mu {t^\alpha }} \right) + \frac{\Lambda }{\mu }\left({1 - {E_\alpha }\left({ - \mu {t^\alpha }} \right)} \right). {E_\alpha }\left({ - \mu {t^\alpha }} \right) is the Mittag-Leffler function of parameter \alpha . Since 0 \leqslant {E_\alpha }\left({ - \mu {t^\alpha }} \right) \leqslant 1 , we obtain N\left(t \right) \leqslant \frac{\Lambda }{\mu } + N\left(0 \right). Subsequently, by applying the fifth equation of Eq (3.1), we obtain {D^\alpha }M\left(t \right) \leqslant {m_0} + \frac{{\rho \Lambda }}{\mu } - \xi M\left(t \right), and then, 0 \leqslant M\left(t \right) \leqslant \frac{{{m_0}}}{\xi } + \frac{{\rho \Lambda }}{{\mu \xi }} - M\left(0 \right), where M\left(0 \right) denotes the initial value of the message of media reports. Thus, \mathop {\sup }\limits_{t \to \infty } M\left(t \right) \leqslant \frac{{{m_0}}}{\xi } + \frac{\Lambda }{{\mu \xi }} . This implies that N\left(t \right) is bounded, thus I\left(t \right), S\left(t \right), C\left(t \right), R\left(t \right) and M\left(t \right) are bounded in
\Omega = \left\{ {\left( {I, S, C, R, M} \right) \in R_ + ^4:N\left( t \right) = I\left( t \right) + S\left( t \right) + C\left( t \right) + R\left( t \right) \leqslant \frac{\Lambda }{\mu }, M\left( t \right) \leqslant \frac{{{m_0}}}{\xi } + \frac{{\rho \Lambda }}{{\mu \xi }}} \right\}. |
So, all solutions of Eq (3.1) are non-negative. Now, we will show that equation has a unique solution.
Theorem 3.2. For any given initial condition X\left(0 \right) = \left({{I_0}, {H_0}, {S_0}, {R_0}, {M_0}} \right), {\text{ }}\forall t \geqslant 0 , Eq (3.1) has a unique solution X\left(t \right) \in \Omega .
Proof. The approach employed in [40] is adopted to validate the existence and uniqueness.
Let H\left(X \right) = \left({{H_1}\left(X \right), {H_2}\left(X \right), {H_3}\left(X \right), {H_4}\left(X \right), {H_5}\left(X \right)} \right) as follows:
\begin{array}{l} {H_1}\left( X \right) = \Lambda - \frac{{\beta I\left( t \right)S\left( t \right)}}{{1 + bS\left( t \right)}} - \frac{{\delta I\left( t \right)M\left( t \right)}}{{k + M\left( t \right)}} - \eta I\left( t \right) - \mu I\left( t \right), \\ {H_2}\left( X \right) = \frac{{\beta I\left( t \right)S\left( t \right)}}{{1 + bS\left( t \right)}} + \frac{{\delta I\left( t \right)M\left( t \right)}}{{k + M\left( t \right)}} - \gamma S\left( t \right) - \varepsilon S\left( t \right) - \mu S\left( t \right), \\ {H_3}\left( X \right) = \gamma S\left( t \right) - \omega C\left( t \right) - \mu C\left( t \right), \\ {H_4}\left( X \right) = \eta I\left( t \right) + \varepsilon S\left( t \right) + \omega C\left( t \right) - \mu R\left( t \right), \\ {H_5}\left( X \right) = {m_0} + \rho S\left( t \right) - \xi M\left( t \right). \\ \end{array} |
Furthermore, for any given X, {X_1} \in \Omega , a function can be constructed as follows:
\begin{array}{l} \left\| {H\left( X \right) - H\left( Y \right)} \right\| = \left| {{H_1}\left( X \right) - {H_1}\left( {{X_1}} \right)} \right| + \left| {{H_2}\left( X \right) - {H_2}\left( {{X_1}} \right)} \right| + \left| {{H_3}\left( X \right) - {H_3}\left( {{X_1}} \right)} \right| \\ \;\;\;\;\;\;\;\;\;\;\;\;\;\;\;\;\;\;\;\;\;\;\;\;\;{\text{ }} + \left| {{H_4}\left( X \right) - {H_4}\left( {{X_1}} \right)} \right| + \left| {{H_5}\left( X \right) - {H_5}\left( {{X_1}} \right)} \right| \\ \;\;\;\;\;\;\;\;\;\;\;\;\;\;\;\;\;\;\;\;\;\;\;\;\; = \left| {\Lambda - \frac{{\beta IS}}{{1 + bS}} - \frac{{\delta IM}}{{k + M}} - \eta I - \mu I - \Lambda + \frac{{\beta {I_1}{S_1}}}{{1 + b{S_1}}} + \frac{{\delta {I_1}{M_1}}}{{k + {M_1}}} + \eta {I_1} + \mu {I_1}} \right| \\ \;\;\;\;\;\;\;\;\;\;\;\;\;\;\;\;\;\;\;\;\;\;\;\;\;{\text{ }} + \left| {\frac{{\beta IS}}{{1 + bS}} + \frac{{\delta IM}}{{k + M}} - \gamma S - \varepsilon S - \mu S - \frac{{\beta {I_1}{S_1}}}{{1 + b{S_1}}} - \frac{{\delta {I_1}{M_1}}}{{k + {M_1}}} + \gamma {S_1} + \varepsilon {S_1} + \mu {S_1}} \right| \\ \;\;\;\;\;\;\;\;\;\;\;\;\;\;\;\;\;\;\;\;\;\;\;\;\;{\text{ }} + \left| {\gamma S - \omega C - \mu C - \gamma {S_1} + \omega {C_1} + \mu {C_1}} \right| \\ \;\;\;\;\;\;\;\;\;\;\;\;\;\;\;\;\;\;\;\;\;\;\;\;{\text{ }}\; + \left| {\eta I + \varepsilon S + \omega C - \mu R - \eta {I_1} - \varepsilon {S_1} - \omega {C_1} + \mu {R_1}} \right| \\ \;\;\;\;\;\;\;\;\;\;\;\;\;\;\;\;\;\;\;\;\;\;\;\;\;{\text{ }} + \left| {{m_0} + \rho S - \xi M - {m_0} - \rho {S_1} + \xi {M_1}} \right| \\ \;\;\;\;\;\;\;\;\;\;\;\;\;\;\;\;\;\;\;\;\;\;\;\;\; \leqslant {l_1}\left| {I - {I_1}} \right| + {l_2}\left| {S - {S_1}} \right| + {l_3}\left| {C - {C_1}} \right| + {l_4}\left| {R - {R_1}} \right| + {l_5}\left| {M - {M_1}} \right| \\ \;\;\;\;\;\;\;\;\;\;\;\;\;\;\;\;\;\;\;\;\;\;\;\;\; \leqslant L\left\| {\left( {I, S, C, R, M} \right) - \left( {{I_1}, {S_1}, {C_1}, {R_1}, {M_1}} \right)} \right\| \\ \;\;\;\;\;\;\;\;\;\;\;\;\;\;\;\;\;\;\;\;\;\;\;\;\; \leqslant L\left\| {X - {X_1}} \right\|, \\ \end{array} |
where
\begin{array}{c}{l_1} = \frac{{2\beta }}{b} + 2\delta + 2\eta + \mu , {\text{ }}{l_2} = \frac{{2\beta \Lambda }}{\mu } + 2\gamma + 2\varepsilon + \rho + \mu , {\text{ }}{l_3} = 2\omega + \mu , {\text{ }}{l_4} = \mu , {\text{ }}{l_5} = \frac{{2\delta \Lambda }}{{k\mu }} + \xi ,\\ L = \max \left( {{l_1}, {l_2}, {l_3}, {l_4}, {l_5}} \right). \end{array} |
Therefore, H\left(X \right) satisfies the Lipschitz condition with any initial condition X\left(0 \right) = \left({{I_0}, {H_0}, {S_0}, {R_0}, {M_0}} \right), {\text{ }}\forall t \geqslant 0 . Equations (3.1) always have a unique solution.
In order to explore the rumor-free and rumor-permanence equilibrium points of the fractional order Eq (3.1), we solve the following equations:
\begin{array}{l} \Lambda - \frac{{\beta I\left( t \right)S\left( t \right)}}{{1 + bS\left( t \right)}} - \frac{{\delta I\left( t \right)M\left( t \right)}}{{k + M\left( t \right)}} - \eta I\left( t \right) - \mu I\left( t \right) = 0, \\ \frac{{\beta I\left( t \right)S\left( t \right)}}{{1 + bS\left( t \right)}} + \frac{{\delta I\left( t \right)M\left( t \right)}}{{k + M\left( t \right)}} - \gamma S\left( t \right) - \varepsilon S\left( t \right) - \mu S\left( t \right) = 0, \\ \gamma S\left( t \right) - \omega C\left( t \right) - \mu C\left( t \right) = 0, \\ \eta I\left( t \right) + \varepsilon S\left( t \right) + \omega C\left( t \right) - \mu R\left( t \right) = 0, \\ {m_0} + \rho S\left( t \right) - \xi M\left( t \right) = 0. \\ \end{array} |
By denoting the aforementioned Eq (3.1) with {S_0} = 0 , the rumor-free equilibrium point can be calculated as: {E_0} = \left({\frac{\Lambda }{{\eta + \mu }}, 0, 0, 0, \frac{{{m_0}}}{\xi } + \frac{{\rho \Lambda }}{{\xi \left({\eta + \mu } \right)}}} \right). By employing the next generation approach [41], the basic reproduction number {R_0} is calculated. If Q = {\left({S, C, M} \right)^T} , the original equation can be reformulated as
\frac{{dQ}}{{dt}} = \mathcal{F}\left( x \right) - \mathcal{V}\left( x \right), |
where
\mathcal{F}\left( x \right) = \left( \begin{array}{l} \frac{{\beta I\left( t \right)S\left( t \right)}}{{1 + bS\left( t \right)}} + \frac{{\delta I\left( t \right)M\left( t \right)}}{{k + M\left( t \right)}} \\ \;\;\;\;\;\;\;\;\;\;\;\;\;\;\;\;0 \\ \;\;\;\;\;\;\;\;\;\;\;\;\;\;\;\;0 \\ \end{array} \right), {\text{ }}\mathcal{V}\left( x \right) = \left( \begin{array}{l} \;\;\;\;\left( {\gamma + \varepsilon + \mu } \right)S\left( t \right) \\ \left( {\omega + \mu } \right)C\left( t \right) - \gamma S\left( t \right) \\ \;{m_0} + \xi M\left( t \right) - \rho S\left( t \right) \\ \end{array} \right). |
The Jacobian matrices of \mathcal{F}\left(x \right) and \mathcal{V}\left(x \right) at the rumor-free equilibrium {E_0} , respectively, are
{F_0} = \left( \begin{array}{l} 0\;\;\;\;\beta {I_0}\;\;\;\;0\;\;\;\;\;\;0\;\;\;\;\;\frac{{\delta {I_0}}}{k} \\ 0\;\;\;\;\;0\;\;\;\;\;\;\;0\;\;\;\;\;\;0\;\;\;\;\;\;\;0 \\ 0\;\;\;\;\;0\;\;\;\;\;\;\;0\;\;\;\;\;\;0\;\;\;\;\;\;\;0 \\ \end{array} \right), {\text{ }}{V_0} = \left( \begin{array}{l} 0\;\;\gamma + \varepsilon + \mu \;\;\;\;\;\;0\;\;\;\;\;\;0\;\;\;\;0 \\ 0\;\;\;\;\; - \gamma \;\;\;\;\;\;\;\;\omega + \mu \;\;\;0\;\;\;\;0 \\ 0\;\;\;\; - \rho \;\;\;\;\;\;\;\;\;\;\;0\;\;\;\;\;\;\;0\;\;\;\;\xi \; \\ \end{array} \right). |
The next-generation matrix for Eq (3.1) is presented as follows:
{F_0}V_0^{ - 1} = \left( \begin{array}{l} \;\;\;\;\;\;\;\;\;\;\;\;0\;\;\;\;\;\;\;\;\;\;\;\;\;\;\; 0 \;\;\;\;\;\; 0 \\ \frac{{\beta {I_0}}}{{\gamma + \varepsilon + \mu }} + \frac{{\rho \delta {I_0}}}{{k\xi \left( {\gamma + \varepsilon + \mu } \right)}}\;\;\;\;0\;\;\;\;\frac{{\delta {I_0}}}{{k\xi }} \\ \;\;\;\;\;\;\;\;\;\;\;\;\;0\;\;\;\;\;\;\;\;\; \;\;\;\;\;0\;\;\;\;\;\;0 \\ \end{array} \right). |
Subsequently, the basic reproduction number of Eq (3.1) is determined:
{R_0} = \rho \left( {{F_0}V_0^{ - 1}} \right) = \frac{{\beta {I_0}}}{{\gamma + \varepsilon + \mu }} + \frac{{\rho \delta {I_0}}}{{k\xi \left( {\gamma + \varepsilon + \mu } \right)}} = \frac{{\Lambda \left( {k\xi \beta + \rho \delta } \right)}}{{k\xi \left( {\eta + \mu } \right)\left( {\gamma + \varepsilon + \mu } \right)}}. |
The following theorem is utilized to analyze the local and global stability of the two equilibrium points.
Theorem 4.1. Equation (3.1) exhibit local stability of the rumor-free equilibrium {E_0} if {R_0} < 1 , and if {R_0} > 1 it is unstable.
Proof. The stability of the equilibrium point is investigated by examining the Jacobian matrix of Eq (3.1).
J\left( {{E_0}} \right) = \left( \begin{array}{l} - \eta - \mu \;\;\;\;\;\;\;\;\;\;\;\; - \beta {I_0}\;\;\;\;\;\;\;\;\;\;\;\;\;\;\;0\;\;\;\;\;\;\;\;\;\;\;0\;\;\;\;\; - \frac{{\delta {I_0}}}{k} \\ \;\;\;\;0\;\;\;\;\;\;\beta {I_0} - \left( {\gamma + \varepsilon + \mu } \right)\;\;\;\;\;\;\;\;\;\;0\;\;\;\;\;\;\;\;\;\;\;0\;\;\;\;\;\;\frac{{\delta {I_0}}}{k} \\ \;\;\;\;0\;\;\;\;\;\;\;\;\;\;\;\;\;\;\;\;\;\;\;\;\gamma \;\;\;\;\;\;\;\;\;\;\;\;\; - \omega - \mu \;\;\;\;\;\;0\;\;\;\;\;\;\;\;0 \\ \;\;\;\;\eta \;\;\;\;\;\;\;\;\;\;\;\;\;\;\;\;\;\;\;\varepsilon \;\;\;\;\;\;\;\;\;\;\;\;\;\;\;\;\;\;\omega \;\;\;\;\;\;\;\;\; - \mu \;\;\;\;\;\;0\;\;\;\; \\ \;\;\;\;0\;\;\;\;\;\;\;\;\;\;\;\;\;\;\;\;\;\;\;\rho \;\;\;\;\;\;\;\;\;\;\;\;\;\;\;\;\;\;0\;\;\;\;\;\;\;\;\;\;\;\;0\;\;\;\;\;\; - \xi \\ \end{array} \right). |
The characteristic polynomial of J\left({{E_0}} \right) is derived as follows:
Det\left( {\lambda I - J\left( {{E_0}} \right)} \right) = \left( {\lambda + \mu } \right)\left( {\lambda + \eta + \mu } \right)\left( {\lambda + \omega + \mu } \right)\left[ {\left( {\lambda - \beta {I_0} + \left( {\gamma + \varepsilon + \mu } \right)} \right)\left( {\lambda + \xi } \right) - \frac{{\rho \delta {I_0}}}{k}} \right]. | (4.1) |
If all roots of Det\left({\lambda I - J\left({{E_0}} \right)} \right) possess negative real parts, the rumor-free equilibrium {E_0} can be considered asymptotically stable.
When Det\left({\lambda I - J\left({{E_0}} \right)} \right) = 0 , we obtain
{\lambda _1} = - \mu , {\text{ }}{\lambda _2} = - \eta - \mu , {\text{ }}{\lambda _3} = - \omega - \mu , |
and
{\lambda ^2} + \left( {\gamma + \varepsilon + \mu + \xi - \beta {I_0}} \right)\lambda + \xi \left( {\gamma + \varepsilon + \mu } \right) - \xi \beta {I_0} - \frac{{\rho \delta {I_0}}}{k} = 0. | (4.2) |
It is obvious that {\lambda _1}, {\text{ }}{\lambda _2} and {\lambda _3} are negatives roots and thus, the real component of each of Eq (4.2) roots plays a crucial role.
According to Eq (4.1), it defines
{b_0} = 1, {\text{ }}{b_1} = \gamma + \varepsilon + \mu + \xi - \beta {I_0}, {\text{ }}{b_2} = \xi \left( {\gamma + \varepsilon + \mu } \right) - \xi \beta {I_0} - \frac{{\rho \delta {I_0}}}{k}. |
Evidently, we obtain
{b_0}{\lambda ^2} + {b_1}\lambda + {b_2} = 0. | (4.3) |
When {R_0} < 1 , we acquire
\xi \left( {\gamma + \varepsilon + \mu } \right) - \xi \beta {I_0} - \frac{{\rho \delta {I_0}}}{k} > 0. | (4.4) |
Thus, {b_2} > 0 is deducted from Eq (4.2) by adding \frac{{\rho \delta {I_0}}}{k} to Eq (4.4), and we obtain
\xi \left( {\gamma + \varepsilon + \mu } \right) - \xi \beta {I_0} > \frac{{\rho \delta {I_0}}}{k} > 0. | (4.5) |
From Eq (4.5), we obtain
\gamma + \varepsilon + \mu - \beta {I_0} > \frac{{\rho \delta {I_0}}}{{k\xi }} > 0. | (4.6) |
By adding the parameter \xi to Eq (4.6), we obtain
\gamma + \varepsilon + \mu + \xi - \beta {I_0} > \xi > 0. | (4.7) |
Therefore, {b_1} > 0 is deduced based on Eq (4.7). After evaluation of different coefficients of Eq (4.2), {b_0} > 0, {\text{ }}{b_1} > 0, {\text{ }}{b_2} > 0 and {b_1}{b_2} > 0 are obtained. According to the Routh-Hurwitz conditions [42], all eigenvalues of J\left({{E_0}} \right) have negative real part. The condition \left| {\arg \left({{\lambda _i}} \right)} \right| \geqslant \frac{{\alpha \pi }}{2} is satisfied and the rumor-free equilibrium {E_0} is locally asymptotically stable if {R_0} < 1 , and unstable if {R_0} > 1 .
Theorem 4.2. The rumor-free equilibrium {E_0} of Eq (3.1) is globally asymptotically stable if {R_0} < 1 .
Proof. Let V\left({I, S, C, R, M} \right) , with positive constants {c_1} and {c_2} , serve as a Lyapunov function
V\left( {I, S, C, R, M} \right) = \left( {I - {I_0} - {I_0}\ln \frac{I}{{{I_0}}}} \right) + \left( {C - {C_0} - {C_0}\ln \frac{C}{{{C_0}}}} \right)\; + \left( {R - {R_0} - {R_0}\ln \frac{R}{{{R_0}}}} \right) + {c_1}S + {c_2}M. |
By differentiating the Lyapunov function with respect to time, we obtain
{D^\alpha }V = \left( {1 - \frac{{{I_0}}}{I}} \right){D^\alpha }I\left( t \right) + \left( {1 - \frac{{{C_0}}}{C}} \right){D^\alpha }C\left( t \right) + \left( {1 - \frac{{{R_0}}}{R}} \right){D^\alpha }R\left( t \right)\; + {c_1}{D^\alpha }S\left( t \right) + {c_2}{D^\alpha }M\left( t \right), | (4.8) |
where {c_1} \geqslant 0, {\text{ }}{c_2} \geqslant 0. Substituting {D^\alpha }I\left(t \right), {D^\alpha }S\left(t \right), {D^\alpha }C\left(t \right), {D^\alpha }R\left(t \right) , and {D^\alpha }M\left(t \right) in Eq (3.1) into Eq (4.8) yields
\begin{array}{l} {D^\alpha }V = \left( {1 - \frac{{{I_0}}}{I}} \right)\left[ {\Lambda - \frac{{\beta I\left( t \right)S\left( t \right)}}{{1 + bS\left( t \right)}} - \frac{{\delta I\left( t \right)M\left( t \right)}}{{k + M\left( t \right)}} - \eta I\left( t \right) - \mu I\left( t \right)} \right] \\ \;\;\;\;\;\;\;\;\;\;\;\; + \left( {1 - \frac{{{C_0}}}{C}} \right)\left[ {\gamma S\left( t \right) - \omega C\left( t \right) - \mu C\left( t \right)} \right] \\ \;\;\;\;\;\;\;\;\;\;\;\; + \left( {1 - \frac{{{R_0}}}{R}} \right)\left[ {\eta I\left( t \right) + \varepsilon S\left( t \right) + \omega C\left( t \right) - \mu R\left( t \right)} \right] \\ \;\;\;\;\;\;\;\;\;\;\;\; + {c_1}\left[ {\frac{{\beta I\left( t \right)S\left( t \right)}}{{1 + bS\left( t \right)}} + \frac{{\delta I\left( t \right)M\left( t \right)}}{{k + M\left( t \right)}} - \gamma S\left( t \right) - \varepsilon S\left( t \right) - \mu S\left( t \right)} \right] \\ \;\;\;\;\;\;\;\;\;\;\;\; + {c_2}\left[ {\rho S\left( t \right) - \xi M\left( t \right)} \right], \\ \end{array} |
{D^\alpha }V \leqslant \left[ {\frac{{{c_1}\delta I\left( t \right)}}{{k + M\left( t \right)}} - {c_2}\xi } \right]M\left( t \right) + \left[ {\frac{{{c_1}\beta I\left( t \right)}}{{1 + bS\left( t \right)}} - {c_1}\left( {\gamma + \varepsilon + \mu } \right) + {c_2}\rho } \right]S\left( t \right). | (4.9) |
From Eq (4.9), we obtain
{d_1} = \frac{{{c_1}\delta I\left( t \right)}}{{k + M\left( t \right)}} - {c_2}\xi , {\text{ }}{d_2} = \frac{{{c_1}\beta I\left( t \right)}}{{1 + bS\left( t \right)}} - {c_1}\left( {\gamma + \varepsilon + \mu } \right) + {c_2}. |
Equating the coefficient {d_1} to zero, we obtain
\frac{{{c_1}\delta I\left( t \right)}}{{k + M\left( t \right)}} = {c_2}\xi . |
At the rumor-free equilibrium {E_0} , I \leqslant {I^0} and M \leqslant {M^0} = 0 , we obtain \frac{{{c_1}\delta {I_0}}}{k} = {c_2}\xi . By considering {c_1} = \xi, {\text{ }}{c_2} = \frac{{\delta {I_0}}}{k}, and substituting {c_1} and {c_2} into Eq (4.9), the obtained result is as follows:
\begin{array}{l} {c_2} = \frac{{\xi \beta {I_0}}}{{1 + bS\left( t \right)}} + \frac{{\rho \delta {I_0}}}{k} - \xi \left( {\gamma + \varepsilon + \mu } \right) \\ \;\;\;\; \leqslant \xi \beta {I_0} + \frac{{\rho \delta {I_0}}}{k} - \xi \left( {\gamma + \varepsilon + \mu } \right){\text{ }}\left( {for{\text{ }}1 + bS\left( t \right) \geqslant 1} \right) \\ \;\;\;\; = \xi \left( {\gamma + \varepsilon + \mu } \right)\left( {{R_0} - 1} \right). \\ \end{array} |
If {R_0} \leqslant 1 , then {D^\alpha }V\left(t \right) \leqslant 0 . Specially, {D^\alpha }V\left(t \right) = 0 if and only if S\left(t \right) = 0 . Therefore, the largest compact invariant set in \left\{ {V\left({I, S, C, R, M} \right) \in \Omega :{D^\alpha }V\left(t \right) \leqslant 0} \right\} is the singleton set \left\{ {{E_0}} \right\} . According to LaSalle's invariance principle [43], {E_0} is globally asymptotically stable in \Omega if {R_0} \leqslant 0 .
Theorem 4.3. If {R_0} > 1 and {l_1}{l_2} - {l_3} > 0, {\text{ }}{l_1}{l_2}{l_3} - l_1^2{l_3} - l_3^2 > 0 , Eq (3.1) exhibits local stability of rumor-permanence equilibrium {E^*} .
Proof. Equation (3.1) at the rumor-permanence equilibrium {E^*} can be further simplified as follows:
\begin{array}{l} \Lambda - \frac{{\beta {I^*}{S^*}}}{{1 + b{S^*}}} - \frac{{\delta {I^*}{M^*}}}{{k + {M^*}}} - \eta {I^*} - \mu {I^*} = 0, \\ \frac{{\beta {I^*}{S^*}}}{{1 + b{S^*}}} + \frac{{\delta {I^*}{M^*}}}{{k + {M^*}}} - \gamma {S^*} - \varepsilon {S^*} - \mu {S^*} = 0, \\ \gamma {S^*} - \omega {C^*} - \mu {C^*} = 0, \\ {m_0} + \rho {S^*} - \xi {M^*} = 0. \\ \end{array} |
The Jacobian matrix evaluated at the rumor-permanence equilibrium {E^*} persistence is presented in [16] as
J\left({E}^{*}\right) = \left(\begin{array}{l}{J}_{11}^{*}{J}_{12}^{*}0{J}_{14}^{*}\\ {J}_{21}^{*}{J}_{22}^{*}0{J}_{24}^{*}\\ 0{J}_{32}^{*}{J}_{33}^{*}0\\ 0{J}_{42}^{*}0{J}_{44}^{*}\end{array}\right), |
where
\begin{array}{l} J_{11}^* = - \frac{{\beta {S^*}}}{{1 + b{S^*}}} - \frac{{\delta {M^*}}}{{k + {M^*}}} - \eta - \mu , {\text{ }}J_{12}^* = - \frac{{\beta {I^*}}}{{{{\left( {1 + b{S^*}} \right)}^2}}}, {\text{ }}J_{14}^* = - \frac{{k\delta {I^*}}}{{{{\left( {k + {M^*}} \right)}^2}}}, \\ J_{21}^* = \frac{{\beta {S^*}}}{{1 + b{S^*}}} + \frac{{\delta {M^*}}}{{k + {M^*}}}, {\text{ }}J_{22}^* = \frac{{\beta {I^*}}}{{{{\left( {1 + b{S^*}} \right)}^2}}} - \gamma - \varepsilon - \mu , {\text{ }}J_{24}^* = \frac{{k\delta {I^*}}}{{{{\left( {k + {M^*}} \right)}^2}}}, \\ J_{32}^* = \gamma , {\text{ }}J_{33}^* = - \omega , {\text{ }}J_{42}^* = \rho , {\text{ }}J_{44}^* = - \xi . \\ \end{array} |
The corresponding characteristic equation can be derived as
{\lambda ^4} + {l_1}{\lambda ^3} + {l_2}{\lambda ^2} + {l_3}\lambda + {l_4} = 0, | (4.10) |
where
\begin{array}{l} {l_1} = - J_{11}^* - J_{22}^* - J_{33}^* - J_{44}^*, \\ {l_2} = J_{11}^*J_{22}^* - J_{12}^*J_{21}^* + J_{11}^*J_{33}^* + J_{11}^*J_{44}^* + J_{22}^*J_{33}^* + J_{22}^*J_{44}^* - J_{24}^*J_{42}^* + J_{33}^*J_{44}^*, \\ {l_3} = - J_{11}^*J_{22}^*J_{33}^* + J_{12}^*J_{21}^*J_{33}^* - J_{11}^*J_{24}^*J_{42}^* + J_{12}^*J_{21}^*J_{44}^* - J_{14}^*J_{21}^*J_{42}^* \\ \;\;\;\;\;\; - J_{11}^*J_{33}^*J_{44}^* - J_{22}^*J_{33}^*J_{44}^* + J_{24}^*J_{33}^*J_{42}^*, \\ {l_4} = J_{11}^*J_{22}^*J_{33}^*J_{44}^* - J_{11}^*J_{24}^*J_{33}^*J_{42}^* - J_{12}^*J_{21}^*J_{33}^*J_{44}^* + J_{14}^*J_{21}^*J_{33}^*J_{42}^*. \\ \end{array} |
For {R_0} > 1 , J_{ii}^*{\text{ }}\left({i = 1, 2, 3, 4} \right), J_{12}^* and J_{14}^* are all negative, while J_{21}^*, J_{24}^*, J_{32}^* and J_{42}^* are positive. Subsequently, we obtain
\begin{array}{l} {\Delta _1} = {l_1} = - J_{11}^* - J_{22}^* - J_{33}^* - J_{44}^* > 0, \\ {\Delta _2} = \left| \begin{array}{l} {l_1}\;\;\;\;1 \\ {l_3}\;\;\;\;{l_2} \\ \end{array} \right| = {l_1}{l_2} - {l_3}, \\ {\Delta _3} = \left( \begin{array}{l} {l_1}\;\;\;\;1\;\;\;\;0 \\ {l_3}\;\;\;\;{l_2}\;\;\;{l_1} \\ 0\;\;\;\;{l_4}\;\;\;\;{l_3} \\ \end{array} \right) = {l_1}{l_2}{l_3} - l_1^2{l_3} - l_3^2. \\ \end{array} |
Obviously, it is {\Delta _1} > 0 . If {l_1}{l_2} - {l_3} > 0, {\text{ }}{l_1}{l_2}{l_3} - l_1^2{l_3} - l_3^2 > 0 , then {\Delta _2} > 0, {\text{ }}{\Delta _3} > 0 . According to the Routh-Hurwitz criterion [42], it can be inferred that all eigenvalues of the eigenequation in Eq (4.10) possess negative real parts.
Therefore, the rumor-permanence equilibrium {E^*} is proven to be locally asymptotically stable and Theorem 4.3 is proven.
The objective of our study is to identify the optimal control method and approach in order to minimize the overall cost associated with rumor spreading. The feasible intervention method aims to minimize the dissemination of rumors by individuals. The primary objective of fractional optimal control is to mitigate the relative density of individuals who spread rumors. Furthermore, the consideration of cost control measures is imperative in light of the current circumstances. Therefore, the optimal control strategy for Eq (5.1) is proposed, and its investigation can be facilitated through the application of Pontryagin's maximum principle [44].
Equation (3.1), incorporating the control mechanism, is thus formulated as
\begin{array}{l} {D^\alpha }I\left( t \right) = \Lambda - \frac{{\beta \left( {1 - {u_1}\left( t \right)} \right)I\left( t \right)S\left( t \right)}}{{1 + bS\left( t \right)}} - \frac{{\delta I\left( t \right)M\left( t \right)}}{{k + M\left( t \right)}} - \eta I\left( t \right) - \mu I\left( t \right), \\ {D^\alpha }S\left( t \right) = \frac{{\beta \left( {1 - {u_1}\left( t \right)} \right)I\left( t \right)S\left( t \right)}}{{1 + bS\left( t \right)}} + \frac{{\delta I\left( t \right)M\left( t \right)}}{{k + M\left( t \right)}} - \gamma S\left( t \right) - \varepsilon {u_2}\left( t \right)S\left( t \right) - \mu S\left( t \right), \\ {D^\alpha }C\left( t \right) = \gamma S\left( t \right) - \omega {u_3}\left( t \right)C\left( t \right) - \mu C\left( t \right), \\ {D^\alpha }R\left( t \right) = \eta I\left( t \right) + \varepsilon {u_2}\left( t \right)S\left( t \right) + \omega {u_3}\left( t \right)C\left( t \right) - \mu R\left( t \right), \\ {D^\alpha }M\left( t \right) = {m_0} + \rho S\left( t \right) - \xi M\left( t \right), \\ \end{array} | (5.1) |
where the control strengths to the rumor propagation are represented by {u_1}\left(t \right), {\text{ }}{u_2}\left(t \right) and {u_3}\left(t \right) through education or government management. The objective function of the optimal control is formulated as
Minimize \;J\left({u}_{1}, {u}_{2}, {u}_{3}\right) = {\displaystyle {\int }_{0}^{T}\left({A}_{1}S\left(t\right)+{A}_{2}C\left(t\right)+{K}_{1}\frac{{u}_{1}^{2}}{2}+{K}_{2}\frac{{u}_{2}^{2}}{2}+{K}_{3}\frac{{u}_{3}^{2}}{2}\right)dt}\text{,} | (5.2) |
where {A_1} and {A_2} are the non-negative weights related to S\left(t \right) and C\left(t \right) in Eq (5.2). {K_1}, {\text{ }}{K_2}, and {K_3} are relative to cost of the measures.
To determine the optimal interventions proposed in Eq (5.1),
U\left\{ {\left. {\left( {{u_1}\left( t \right), {u_2}\left( t \right), {u_3}\left( t \right)} \right)} \right|0 \leqslant {u_1}\left( t \right) \leqslant u_1^{\max }, 0 \leqslant {u_2}\left( t \right) \leqslant u_2^{\max }, 0 \leqslant {u_3}\left( t \right) \leqslant u_3^{\max }, t \in \left( {0, T} \right]} \right\}, | (5.3) |
where u_1^{\max } < 1, {\text{ }}u_2^{\max } < 1, and u_3^{\max } < 1 are the upper bounds of {u_1}\left(t \right), {\text{ }}{u_2}\left(t \right), and {u_3}\left(t \right) , respectively. Optimal controls u_1^*, u_2^*, and u_3^* satisfy J\left({u_1^*, u_2^*, u_2^*} \right) = \min \left\{ {J\left({{u_1}\left(t \right), {u_2}\left(t \right), {u_3}\left(t \right)} \right):} \right. \left. {\left({{u_1}\left(t \right), {u_2}\left(t \right), {u_3}\left(t \right)} \right) \in U} \right\} .
We derive the optimal controls for minimizing the rumor propagation at minimal cost. Consequently, the following Lagrangian function is obtained:
\begin{array}{l}{\rm H} = {A}_{1}S\left(t\right)+{A}_{2}C\left(t\right)+{K}_{1}\frac{{u}_{1}^{2}}{2}+{K}_{2}\frac{{u}_{2}^{2}}{2}+{K}_{3}\frac{{u}_{3}^{2}}{2}\\ \text{ }+{\lambda }_{1}\left(\Lambda -\frac{\beta \left(1-{u}_{1}\left(t\right)\right)I\left(t\right)S\left(t\right)}{1+bS\left(t\right)}-\frac{\delta I\left(t\right)M\left(t\right)}{k+M\left(t\right)}-\eta I\left(t\right)-\mu I\left(t\right)\right)\\ \text{ }+{\lambda }_{2}\left(\frac{\beta \left(1-{u}_{1}\left(t\right)\right)I\left(t\right)S\left(t\right)}{1+bS\left(t\right)}+\frac{\delta I\left(t\right)M\left(t\right)}{k+M\left(t\right)}-\left(\gamma +\varepsilon {u}_{2}\left(t\right)+\mu \right)S\left(t\right)\right)\\ \text{ }+{\lambda }_{3}\left(\gamma S\left(t\right)-\omega {u}_{3}\left(t\right)C\left(t\right)-\mu C\left(t\right)\right)\\ \text{ }+{\lambda }_{4}\left(\eta I\left(t\right)+\varepsilon {u}_{2}\left(t\right)S\left(t\right)+\omega {u}_{3}\left(t\right)C\left(t\right)-\mu R\left(t\right)\right)\\ \text{ }+{\lambda }_{5}\left({m}_{0}+\rho S\left(t\right)-\xi M\left(t\right)\right)\text{,}\end{array} | (5.4) |
where {\lambda _1}, {\lambda _2}, {\lambda _3}, {\lambda _4}, {\lambda _5} are adjoint variable functions.
Theorem 5.1. There exists an optimal control with the solution \left({I, S, C, R, M} \right) corresponding to the state equation in Eq (5.1) and the adjoint variables {\lambda _1}, {\lambda _2}, {\lambda _3}, {\lambda _4}, {\lambda _5} , we obtain
\begin{array}{l} {D^\alpha }{\lambda _1} = - {\lambda _1}\left( {\frac{{\beta \left( {1 - {u_1}} \right)S}}{{1 + bS}} - \frac{{\delta M}}{{k + M}} + \eta + \mu } \right) - {\lambda _2}\rho \left( {\frac{{\beta \left( {1 - {u_1}} \right)S}}{{1 + bS}} - \frac{{\delta M}}{{k + M}}} \right) + {\lambda _4}\eta , \\ {D^\alpha }{\lambda _2} = \frac{{ - {\lambda _1}\beta \left( {1 - {u_1}} \right)I}}{{{{\left( {1 + bS} \right)}^2}}} + {\lambda _2}\left( {\frac{{\beta \left( {1 - {u_1}} \right)I}}{{{{\left( {1 + bS} \right)}^2}}} - \left( {\gamma + \varepsilon {u_2}\left( t \right) + \mu } \right)} \right) - {\lambda _3}\gamma - {\lambda _4}\varepsilon {u_2}\left( t \right) - \;{\lambda _5}\rho , \\ {D^\alpha }{\lambda _3} = {\lambda _3}\left( {\omega {u_3}\left( t \right) + \mu } \right) - {\lambda _4}\omega {u_3}\left( t \right), \\ {D^\alpha }{\lambda _4} = {\lambda _4}\mu , \\ {D^\alpha }\;{\lambda _5} = {\lambda _1}\frac{{k\delta I}}{{{{\left( {k + M} \right)}^2}}} - {\lambda _2}\frac{{k\delta I}}{{{{\left( {k + M} \right)}^2}}} + \;{\lambda _5}\xi M, \\ \end{array} |
with boundary conditions {\lambda _1}\left(t \right) = {\lambda _2}\left(t \right) = {\lambda _3}\left(t \right) = {\lambda _4}\left(t \right) = {\lambda _5}\left(t \right) = 0. According to the adjoint variables I\left(t \right), S\left(t \right), C\left(t \right), R\left(t \right) and M\left(t \right) , we differentiate the Hamiltonian {\rm H} in Eq (5.2) with respect to each variable.
Proof. From the adjoint variables I\left(t \right), S\left(t \right), C\left(t \right), R\left(t \right) and M\left(t \right) , we differentiate the Hamiltonian {\rm H} in Eq (5.2) with respect to each variable:
\begin{array}{l} {D^\alpha }{\lambda _1} = \frac{{ - \partial {\rm H}}}{{\partial I}} = {\lambda _1}\left( {\frac{{\beta \left( {1 - {u_1}} \right)S}}{{1 + bS}} - \frac{{\delta M}}{{k + M}} + \eta + \mu } \right) - {\lambda _2}\rho \left( {\frac{{\beta \left( {1 - {u_1}} \right)S}}{{1 + bS}} - \frac{{\delta M}}{{k + M}}} \right) + {\lambda _4}\eta , \\ {D^\alpha }{\lambda _2} = \frac{{ - \partial {\rm H}}}{{\partial S}} = \frac{{ - {\lambda _1}\beta \left( {1 - {u_1}} \right)I}}{{{{\left( {1 + bS} \right)}^2}}} + {\lambda _2}\left( {\frac{{\beta \left( {1 - {u_1}} \right)I}}{{{{\left( {1 + bS} \right)}^2}}} - \left( {\gamma + \varepsilon {u_2}\left( t \right) + \mu } \right)} \right) - {\lambda _3}\gamma - {\lambda _4}\varepsilon {u_2}\left( t \right) - \;{\lambda _5}\rho , \\ {D^\alpha }{\lambda _3} = \frac{{ - \partial {\rm H}}}{{\partial C}} = {\lambda _3}\left( {\omega {u_3}\left( t \right) + \mu } \right) - {\lambda _4}\omega {u_3}\left( t \right), \\ {D^\alpha }{\lambda _4} = \frac{{ - \partial {\rm H}}}{{\partial R}} = {\lambda _4}\mu , \\ {D^\alpha }\;{\lambda _5} = \frac{{ - \partial {\rm H}}}{{\partial M}} = {\lambda _1}\frac{{k\delta I}}{{{{\left( {k + M} \right)}^2}}} - {\lambda _2}\frac{{k\delta I}}{{{{\left( {k + M} \right)}^2}}} + \;{\lambda _5}\xi M. \\ \end{array} |
Moreover, the optimal control of the control variables set {u_1}, {u_2}, {u_3} is attained by partial differential equation as follows:
\frac{{\partial {\rm H}\left( {I, S, C, R, M} \right)}}{{\partial {u_i}}} = 0, {\text{ }}i = 1, 2, 3. |
For u_1^*, {\text{ }}\frac{{\partial {\rm H}\left({I, S, C, R, M} \right)}}{{\partial {u_1}}} = 0,
u_1^* = \frac{{\beta SI\left( {{\lambda _1} - {\lambda _2}} \right)}}{{{K_1}}}. |
For u_2^*, \frac{{\partial {\rm H}\left({I, S, C, R, M} \right)}}{{\partial {u_2}}} = 0,
u_2^* = \frac{{\varepsilon S\left( { - {\lambda _2} + \;{\lambda _4}} \right)}}{{{K_2}}}. |
For u_3^*, \frac{{\partial {\rm H}\left({I, S, C, R, M} \right)}}{{\partial {u_3}}} = 0,
u_3^* = \frac{{\omega C\left( { - {\lambda _3} + {\lambda _4}} \right)}}{{{K_3}}}. |
Therefore,
\begin{array}{l} u_1^* = \max \left\{ {0, \min \left( {1, \frac{{\omega C\left( { - {\lambda _3} + {\lambda _4}} \right)}}{{{K_3}}}} \right)} \right\}, \\ u_2^* = \max \left\{ {0, \min \left( {1, \frac{{\varepsilon S\left( { - {\lambda _2} + \;{\lambda _4}} \right)}}{{{K_2}}}} \right)} \right\}, \\ u_3^* = \max \left\{ {0, \min \left( {1, \frac{{\omega C\left( { - {\lambda _3} + {\lambda _4}} \right)}}{{{K_3}}}} \right)} \right\}. \\ \end{array} |
The uniqueness of the optimal control has been established based on the boundedness and adjoint variables. The standard control with a bound is obtained as follows:
{u}_{1}^{*} = \left\{ \begin{array}{l}0, \;\;\;\;\;\;\;\;\;if\;\;\;\frac{\beta SI\left({\lambda }_{1}-{\lambda }_{2}\right)}{{K}_{1}} < 0, \\ \left(0, 1\right),\;\;\;if\;\;\;\frac{\beta SI\left({\lambda }_{1}-{\lambda }_{2}\right)}{{K}_{1}}\le 1, \\ 1, \;\;\;\;\;\;\;\;\;if\;\;\;\frac{\beta SI\left({\lambda }_{1}-{\lambda }_{2}\right)}{{K}_{1}} > 1, \end{array} \right. |
{u}_{2}^{*} = \left\{ \begin{array}{l}0, \;\;\;\;\;\;\;\;\;if\;\;\;\frac{\varepsilon S\left(-{\lambda }_{2}+{\lambda }_{4}\right)}{{K}_{2}} < 0, \\ \left(0, 1\right),\;\;\;if\;\;\;\frac{\varepsilon S\left(-{\lambda }_{2}+{\lambda }_{4}\right)}{{K}_{2}}\le 1, \\ 1, \;\;\;\;\;\;\;\;\;if\;\;\;\frac{\varepsilon S\left(-{\lambda }_{2}+{\lambda }_{4}\right)}{{K}_{2}} > 1, \end{array} \right. |
{u}_{3}^{*} = \left\{ \begin{array}{l}0, \;\;\;\;\;\;\;\;\;if\;\;\;\frac{\omega C\left(-{\lambda }_{3}+{\lambda }_{4}\right)}{{K}_{3}} < 0, \\ \left(0, 1\right),\;\;\;if\;\;\;\frac{\omega C\left(-{\lambda }_{3}+{\lambda }_{4}\right)}{{K}_{3}}\le 1, \\ 1, \;\;\;\;\;\;\;\;\;if\;\;\;\frac{\omega C\left(-{\lambda }_{3}+{\lambda }_{4}\right)}{{K}_{3}} > 1.\end{array} \right. |
The sensitivity of the parameters in the rumor propagation model is investigated in this section. The following conditions are satisfied:
\begin{array}{l} {A_\beta } = \frac{\beta }{{{R_0}}}\frac{{\partial {R_0}}}{{\partial \beta }} = \frac{{k\xi \beta }}{{k\xi \beta + \rho \delta }} > 0, \\ {A_\rho } = \frac{\rho }{{{R_0}}}\frac{{\partial {R_0}}}{{\partial \rho }} = \frac{{\rho \delta }}{{k\xi \beta + \rho \delta }} > 0, \\ {A_\delta } = \frac{\delta }{{{R_0}}}\frac{{\partial {R_0}}}{{\partial \delta }} = \frac{{\rho \delta }}{{k\xi \beta + \rho \delta }} > 0, \\ {A_\eta } = \frac{\eta }{{{R_0}}}\frac{{\partial {R_0}}}{{\partial \eta }} = - \frac{\eta }{{\left( {\eta + \mu } \right)}} < 0. \\ \end{array} |
In conclusion, \beta , \rho , and \delta are sensitive, and \eta , analogous to \gamma and \varepsilon are not sensitive. Sensitivity analysis shows that it is possible to reduce the rumor contact rate \beta and parameters \rho and \delta , thus lowering the value of {R_0} .
In this section, we present the fractional Euler method [45], which is employed in solving fractional-order differential equations and obtaining a discretized equation, as described below:
\begin{array}{l} I\left( {{t_p}} \right) = I\left( {{t_0}} \right) + \frac{{{h^\alpha }}}{{\Gamma \left( {\alpha + 1} \right)}}\sum\limits_{j = 0}^{p - 1} {{c_{j, p}}\left[ {\Lambda - \frac{{\beta I\left( {{t_j}} \right)S\left( {{t_j}} \right)}}{{1 + bS\left( {{t_j}} \right)}} - \frac{{\delta I\left( {{t_j}} \right)M\left( {{t_j}} \right)}}{{k + M\left( {{t_j}} \right)}} - \left( {\eta + \mu } \right)I\left( {{t_j}} \right)} \right]} , \\ S\left( {{t_p}} \right) = S\left( {{t_0}} \right) + \frac{{{h^\alpha }}}{{\Gamma \left( {\alpha + 1} \right)}}\sum\limits_{j = 0}^{p - 1} {{c_{j, p}}\left[ {\frac{{\beta I\left( {{t_j}} \right)S\left( {{t_j}} \right)}}{{1 + bS\left( {{t_j}} \right)}} + \frac{{\delta I\left( {{t_j}} \right)M\left( {{t_j}} \right)}}{{k + M\left( {{t_j}} \right)}} - \left( {\gamma + \varepsilon + \mu } \right)S\left( {{t_j}} \right)} \right]} , \\ C\left( {{t_p}} \right) = C\left( {{t_0}} \right) + \frac{{{h^\alpha }}}{{\Gamma \left( {\alpha + 1} \right)}}\sum\limits_{j = 0}^{p - 1} {{c_{j, p}}\left[ {\gamma S\left( {{t_j}} \right) - \left( {\omega + \mu } \right)C\left( {{t_j}} \right)} \right]} , \\ R\left( {{t_p}} \right) = R\left( {{t_0}} \right) + \frac{{{h^\alpha }}}{{\Gamma \left( {\alpha + 1} \right)}}\sum\limits_{j = 0}^{p - 1} {{c_{j, p}}\left[ {\eta I\left( {{t_j}} \right) + \varepsilon S\left( {{t_j}} \right) + \omega C\left( {{t_j}} \right) - \mu R\left( {{t_j}} \right)} \right]} , \\ M\left( {{t_p}} \right) = M\left( {{t_0}} \right) + \frac{{{h^\alpha }}}{{\Gamma \left( {\alpha + 1} \right)}}\sum\limits_{j = 0}^{p - 1} {{c_{j, p}}\left[ {{m_0} + \rho M\left( {{t_j}} \right) - \xi M\left( {{t_j}} \right)} \right]} . \\ \end{array} |
for p = 1, 2, \ldots, N where {c_{j, p}} = {\left({p - j} \right)^\alpha } - {\left({p - 1 - j} \right)^\alpha } . The fractional Euler method is employed for the subsequent numerical solutions of the fractional-order differential equations.
In this section, we conduct numerical simulations to validate the findings presented in the preceding sections. The initial values are considered as I\left(0 \right) = 10500, {\text{ }}S\left(0 \right) = 1500, C\left(0 \right) = 750, {\text{ }}R\left(0 \right) = 750, and M\left(0 \right) = 1500 . Additionally, the parametric values are listed in Table 1. Obviously, the basic reproduction number is {{\text{R}}_0}{\text{ = 0}}{\text{.4}} < 1 . Figure 2 provides graphs of the evolution of (a) Ignorants, (b) Spreaders, (c) Calmness, (d) Removers, and (e) Media Reports in the rumor-free case for Eq (3.1), employing various values of the differentiation parameter \alpha = 0.6, 0.7, 0.8, 0.9, 1. With the decrease of the order \alpha , the convergence rate of the rumor propagation curve slows down and eventually tends to stabilize, which shows the memory effect. From the perspective of public opinion dissemination, it implies that such rumors tend to persist for an extended duration. Moreover, it demonstrates an anomalous propagation pattern at a lower order \alpha , characterized by an exponentially rapid growth in the initial phase as depicted in Figure 3(b). Meanwhile, the subsequent phase exhibits a deceleration. When the order is \alpha = 1 , the fractional-order differential equation turns into an integer-order one.
Parameter | Value | Parameter | Value |
\Lambda | 0.4 | \omega | 0.4 |
\beta | 0.1 | \eta | 0.2 |
\delta | 0.2 | \varepsilon | 0.5 |
b | 0.5 | {m_0} | 0.2 |
k | 0.5 | \rho | 0.2 |
\xi | 0.3 | \mu | 0.05 |
\gamma | 0.5 |
The initial values are also set to I\left(0 \right) = 10500, {\text{ }}S\left(0 \right) = 1500, {\text{ }}C\left(0 \right) = 750, {\text{ }}R\left(0 \right) = 750, and M\left(0 \right) = 1500 . Furthermore, the parametric values are provided in Table 2. The calculation result of the basic reproduction number is {{\text{R}}_0}{\text{ = 1}}{\text{.32}} > 1 . The results corresponding to the simulation of the rumors case are presented in Figure 3 using various values of the differentiation parameter \alpha = 0.6, 0.7, 0.8, 0.9, 1. The convergence rates of rumor propagation vary depending on the order value \alpha . The impact of the fractional order is observed through numerical simulation in Figure 3, from which it is demonstrated that reducing the value \alpha can enhance the stability range of model (3.1). The larger the order \alpha , the more rapidly the curve approaches stability in the rumor-permanence case. Therefore, it is imperative to consistently and promptly disseminate scientific knowledge while actively debunking rumors in order to effectively suppress rumor propagation.
Parameter | Value | Parameter | Value |
\Lambda | 1.2 | \omega | 0.4 |
\beta | 0.6 | \eta | 0.2 |
\delta | 0.5 | \varepsilon | 0.6 |
b | 1 | {m_0} | 0.6 |
k | 1 | \rho | 0.2 |
\xi | 0.3 | \mu | 0.3 |
\gamma | 0.5 |
To illustrate the effectiveness of the media reports, for the basic reproduction number {R_0} > 1, {\text{ }}\alpha {\text{ = }}0.95 is established with the parametric values in Table 2. The media reports have an impact on Eq (3.1) governing fractional-order \alpha {\text{ = }}0.95 as illustrated in Figure 4. The peak of the Spreaders group decreases as the value of k increases, as shown in Figure 4(b). The statement suggests that there is a positive correlation between the level of media reports and the influence on rumor propagation. Therefore, employing appropriate media strategies can effectively suppress the rumor propagation.
To demonstrate the effectiveness of the fractional optimal control strategy, for the basic reproduction number {R_0} > 1, {\text{ }}\alpha {\text{ = }}0.95 is established with the parametric values in Table 2. Figure 5 shows the populations of I\left(t \right), S\left(t \right), C\left(t \right), R\left(t \right), and M\left(t \right) , with or without control mechanism. Compared with Eq (5.1), with or without control strategy, the populations of the Ignorants and the Spreaders are significantly influenced. This implies that implementing an effective control mechanism can significantly restrict the rumor diffusion.
A Caputo sense-based ISCRM rumor propagation model incorporating media reports is proposed. The existence of positive solutions is rigorously established in this study, and the equilibrium solutions are determined for both the rumor-free equilibrium and rumor-permanence equilibrium, along with their local properties. The rumor-free equilibrium {E_0} is locally and globally asymptotically stable if {R_0} < 1 . The instability of the unique rumor-permanence equilibrium {E^*} exists when {R_0} > 1 , and for {l_1}{l_2} - {l_3} > 0, {\text{ }}{l_1}{l_2}{l_3} - l_1^2{l_3} - l_3^2 > 0 , it is locally asymptotically stable. The analytical research was validated by conducting numerical simulations, encompassing a range of fractional orders and initial compartment sizes. In the case of {R_0} < 1 , stability is rapidly achieved at lower fractional orders, whereas in the case of {R_0} > 1 , the attainment of stability is rapidly achieved at higher orders. The significance of fractional analysis has been underscored by the achievement of significantly improved and more realistic outcomes. Additionally, a comprehensive analysis of the impact of media reports and an optimal control strategy is presented. The effectiveness of rumor diffusion prevention can be significantly enhanced through the implementation of a control strategy and media reports, thereby mitigating the risk associated with rumor propagation. In future research, the investigation of time delay effects [46] and stochastic factors [47,48] could be expanded within the framework of complex networks in Caputo sense systems.
The authors declare they have not used Artificial Intelligence (AI) tools in the creation of this article.
The authors declare that they have no known competing financial interests or personal relationships that could have appeared to influence the work reported in this paper.
[1] | H. K. Wu, S. W. Y. Lee, H. Y. Chang, et al., Current status, opportunities and challenges of augmented reality in education, Comput. Educ., 62 (2013), 41–49. |
[2] | Y. B. Kim and S. Y. Rhee, Augmented reality on a deformable surface by considering self-occlusion and realistic illu, J. Internet Technol., 12 (2011), 733–740. |
[3] | B. Koo and T. Shon, A structural health monitoring framework using 3D visualization and augmented reality in wireless sensor networks, J. Internet Technol., 11 (2010), 801–807. |
[4] | G. Schall, E. Mendez, E. Kruijff, et al., Handheld augmented reality for underground infrastructure visualization, Pers. Ubiquit. Comput., 13 (2009), 281–291. |
[5] | K. J. L. Nevelsteen, Virtual world, defined from a technological perspective and applied to video games, mixed reality, and the Metaverse, Comput. Animat. Virt. W., 29 (2017). |
[6] | J. Luo, Q. Zhang, X. Chen, et al., Modeling and race detection of ladder diagrams via ordinary Petri nets, IEEE T. Syst., Man. Cy. S., 48 (2018), 1166–1176. |
[7] | B. J. Boom, S. Orts-Escolano, X. X. Ning, et al., Interactive light source position estimation for augmented reality with an RGB-D camera, Comput. Animat. Virt. W., 28 (2017). |
[8] | P. C. Xiong, Y. S. Fan and M. C. Zhou, A Petri net approach to analysis and composition of Web services, IEEE T. Syst. Man. Cy. A., 40 (2010), 376–387. |
[9] | Y. Ru and C. N. Hadjicostis, Bounds on the number of markings consistent with label observations in Petri nets, IEEE T. Autom. Sci. Eng., 6 (2009), 334–344. |
[10] | J. Rosell, Assembly and task planning using Petri nets: A survey, J. Eng. Manuf., 218 (2004), 987–994. |
[11] | L. Li, Y. Ru and C. N. Hadjicostis, Least-cost firing sequence estimation in labeled Petri nets, Process of the 45th IEEE International Conference on Decision and Control, (2006), 416–421. |
[12] | D. M. Xiang, G. J. Liu, C. G. Yan, et al., Detecting data-flow errors based on Petri nets with data operations, IEEE/CAA JAS, 5 (2018), 251–260. |
[13] | T. Murata, Petri nets: properties, analysis, and applications, IEEE, 77 (1989), 541–580. |
[14] | N. Wu and M. C. Zhou, System modeling and control with resource-oriented Petri nets, Int. J. Prod. Res., 49 (2011), 6585–6586. |
[15] | WoPeD, Mar. 2017. Available from: http://woped.dhbw-karlsruhe.de/woped/. |
[16] | F. Suro and Y. Ono, Japanese EFL learners' uses of text-to-speech technology and their learning behaviors: A pilot study, Process of the 2016 5th IIAI International Congress on Advanced Applied Informatics (IIAI-AAI), (2016), 296–301. |
[17] | Y. Lee, S. Lee and S. H. Lee, Multifinger interaction between remote users in avatar-mediated telepresence, Comput. Animat. Virt. W., 28 (2017). |
[18] | Speaker independent connected speech recognition-fifth generation computer corporation, May 2017. Available from: http://www.fifthgen.com/speaker-independent-connected-s-r.htm. |
[19] | F. J. Yang, N. Q. Wu, Y. Qiao, et al., Polynomial approach to optimal one-wafer cyclic scheduling of treelike hybrid multi-cluster tools via Petri nets, IEEE/CAA JAS, 5 (2018), 270–280. |
[20] | P. Milgram and A. F. Kishino, Taxonomy of mixed reality visual displays, IEICE T. Inform. Syst., (1994), 1321–1329. |
[21] | K. C. Li, C. W. Tsai, C. T. Chen, et al., The design of immersive English learning environment using augmented reality, Process of 8th IEEE International Conference on UMEDIA, (2015), 174–179. |
[22] | C. S. C. Dalim, A. Dey and T. Piumsomboon, TeachAR: An interactive augmented reality tool for teaching basic English to non-native children, Process of IEEE International Symposium on Mixed and Augmented Reality Adjunct, (2016), 67–72. |
[23] | F. Sorrentino, L. D. Spano and R. Scateni, Speaky notes learn languages with augmented reality, Process of IEEE International Conference Interactive Mobile Communication Technologies and Learning (IMCL), (2015), 56–61. |
1. | Hong Li, Zhantao Li, Chaohong Pan, Jie Song, Mingji Zhang, Cubic-like Features of I–V Relations via Classical Poisson–Nernst–Planck Systems Under Relaxed Electroneutrality Boundary Conditions, 2024, 13, 2075-1680, 790, 10.3390/axioms13110790 | |
2. | Xiangshuo Liu, Jie Song, Lijun Zhang, Mingji Zhang, Roles Played by Critical Potentials in the Study of Poisson–Nernst–Planck Models with Steric Effects Under Relaxed Neutral Boundary Conditions, 2025, 14, 2075-1680, 69, 10.3390/axioms14010069 |
Parameter | Value | Parameter | Value |
\Lambda | 0.4 | \omega | 0.4 |
\beta | 0.1 | \eta | 0.2 |
\delta | 0.2 | \varepsilon | 0.5 |
b | 0.5 | {m_0} | 0.2 |
k | 0.5 | \rho | 0.2 |
\xi | 0.3 | \mu | 0.05 |
\gamma | 0.5 |
Parameter | Value | Parameter | Value |
\Lambda | 1.2 | \omega | 0.4 |
\beta | 0.6 | \eta | 0.2 |
\delta | 0.5 | \varepsilon | 0.6 |
b | 1 | {m_0} | 0.6 |
k | 1 | \rho | 0.2 |
\xi | 0.3 | \mu | 0.3 |
\gamma | 0.5 |
Parameter | Value | Parameter | Value |
\Lambda | 0.4 | \omega | 0.4 |
\beta | 0.1 | \eta | 0.2 |
\delta | 0.2 | \varepsilon | 0.5 |
b | 0.5 | {m_0} | 0.2 |
k | 0.5 | \rho | 0.2 |
\xi | 0.3 | \mu | 0.05 |
\gamma | 0.5 |
Parameter | Value | Parameter | Value |
\Lambda | 1.2 | \omega | 0.4 |
\beta | 0.6 | \eta | 0.2 |
\delta | 0.5 | \varepsilon | 0.6 |
b | 1 | {m_0} | 0.6 |
k | 1 | \rho | 0.2 |
\xi | 0.3 | \mu | 0.3 |
\gamma | 0.5 |