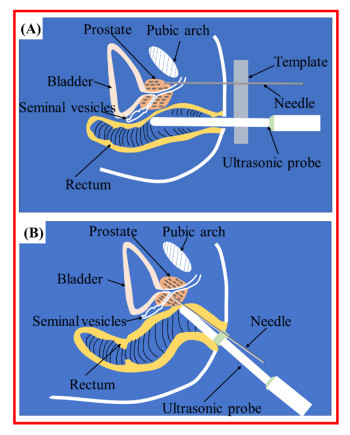
Citation: Li-Tao Zhang, Chao-Qian Li, Yao-Tang Li. A parameterized shift-splitting preconditioner for saddle point problems[J]. Mathematical Biosciences and Engineering, 2019, 16(2): 1021-1033. doi: 10.3934/mbe.2019048
[1] | Kai Cheng, Lixia Li, Yanmin Du, Jiangtao Wang, Zhenghua Chen, Jian Liu, Xiangsheng Zhang, Lin Dong, Yuanyuan Shen, Zhenlin Yang . A systematic review of image-guided, surgical robot-assisted percutaneous puncture: Challenges and benefits. Mathematical Biosciences and Engineering, 2023, 20(5): 8375-8399. doi: 10.3934/mbe.2023367 |
[2] | Xinran Zhang, Yongde Zhang, Jianzhi Yang, Haiyan Du . A prostate seed implantation robot system based on human-computer interactions: Augmented reality and voice control. Mathematical Biosciences and Engineering, 2024, 21(5): 5947-5971. doi: 10.3934/mbe.2024262 |
[3] | Jun Gao, Qian Jiang, Bo Zhou, Daozheng Chen . Convolutional neural networks for computer-aided detection or diagnosis in medical image analysis: An overview. Mathematical Biosciences and Engineering, 2019, 16(6): 6536-6561. doi: 10.3934/mbe.2019326 |
[4] | Wenlu Zhang, Ziyue Ma, Hong Wang, Juan Deng, Pengfei Li, Yu Jia, Yabin Dong, Hong Sha, Feng Yan, Wenjun Tu . Study on automatic ultrasound scanning of lumbar spine and visualization system for path planning in lumbar puncture surgery. Mathematical Biosciences and Engineering, 2023, 20(1): 613-623. doi: 10.3934/mbe.2023028 |
[5] | Jiashuai Li, Xiuyan Peng, Bing Li, Victor Sreeram, Jiawei Wu, Ziang Chen, Mingze Li . Model predictive control for constrained robot manipulator visual servoing tuned by reinforcement learning. Mathematical Biosciences and Engineering, 2023, 20(6): 10495-10513. doi: 10.3934/mbe.2023463 |
[6] | Bethan Morris, Lee Curtin, Andrea Hawkins-Daarud, Matthew E. Hubbard, Ruman Rahman, Stuart J. Smith, Dorothee Auer, Nhan L. Tran, Leland S. Hu, Jennifer M. Eschbacher, Kris A. Smith, Ashley Stokes, Kristin R. Swanson, Markus R. Owen . Identifying the spatial and temporal dynamics of molecularly-distinct glioblastoma sub-populations. Mathematical Biosciences and Engineering, 2020, 17(5): 4905-4941. doi: 10.3934/mbe.2020267 |
[7] | Zhen Yang, Junli Li, Liwei Yang, Qian Wang, Ping Li, Guofeng Xia . Path planning and collision avoidance methods for distributed multi-robot systems in complex dynamic environments. Mathematical Biosciences and Engineering, 2023, 20(1): 145-178. doi: 10.3934/mbe.2023008 |
[8] | Fang Zhu, Wei Liu . A novel medical image fusion method based on multi-scale shearing rolling weighted guided image filter. Mathematical Biosciences and Engineering, 2023, 20(8): 15374-15406. doi: 10.3934/mbe.2023687 |
[9] | Leonardo Schultz, Antonio Gondim, Shigui Ruan . Gompertz models with periodical treatment and applications to prostate cancer. Mathematical Biosciences and Engineering, 2024, 21(3): 4104-4116. doi: 10.3934/mbe.2024181 |
[10] | Yong Tao, Fan Ren, Youdong Chen, Tianmiao Wang, Yu Zou, Chaoyong Chen, Shan Jiang . A method for robotic grasping based on improved Gaussian mixture model. Mathematical Biosciences and Engineering, 2020, 17(2): 1495-1510. doi: 10.3934/mbe.2020077 |
Globally, prostate cancer (PCa) is the fifth leading cause of cancer mortality in men and the second most common cancer type with more than 900,000 new cases of PCa each year [1]. The urologist performs a puncture biopsy of the prostate based on a positive digital rectal examination (DRE) and elevated prostate-specific antigen (PSA) levels in the patient to definitively diagnose PCa [2]. Prostate biopsy is a method in which urologists use a biopsy needle to collect tissue samples from a specific area of the prostate for the diagnosis of PCa which is very important for the early detection of PCa.
At present, clinical urologists usually choose two methods for prostate biopsy: one is a transperineal prostate biopsy (TPPB) and the other is a transrectal prostate biopsy (TRPB) [3]. That is, the biopsy needle can enter the prostate through the perineal or rectal access, as shown in Figure 1 [4]. In TPPB, the patient is placed in the lithotomy position, requiring general anesthesia and a longer needle path resulting in higher biopsy cost, increased patient discomfort and longer duration of biopsy. But TPPB can insert multiple needles at the same time and can retrieve more tissue from the surrounding area of the prostate [5]. In TRPB the urologist needs to apply anesthesia to the local area of the patient's rectum and place the patient in the lithotomy or lateral decubitus position. In contrast to general anesthesia, patients experience pain during the biopsy and may experience complications such as rectal infection and bleeding [6]. The study by Emiliozzi et al. [7] showed that TPPB was more effective than TRPB in detecting PCa. In clinical practice, image guidance is usually used to assist doctors in biopsy surgery. Compared with traditional blind insertion of biopsy, it can avoid damage to surrounding normal tissues and improve the accuracy of PCa detection. This article uses MRI-guided TRPB as an example to illustrate, the simplified process as follows: At present, many advanced medical institutions conduct multi-parametric MRI (mpMRI) scans on patients before performing biopsies to identify suspicious areas in the prostate. Urologists make biopsy plans based on the scan results. mpMRI is an advanced imaging technology that can provide doctors with more accurate and comprehensive diagnostic information than traditional MRI imaging technology. After the mpMRI scan is complete, the doctor cleans and sterilizes the biopsy equipment, administers local anesthesia to the patient and places the patient on the MRI scanner table. Subsequently, the urologist inserts the MRI-compatible biopsy device into the patient's rectum and scans the patient with MRI. The doctor observes the relative position of the biopsy needle and the target point, controls the insertion depth and direction of the biopsy needle, repeats the above steps to complete the biopsy plan and obtains multiple biopsy samples from patients for pathological evaluation.
Since the introduction of transrectal ultrasound (TRUS)-guided sextant prostate biopsy by Hodge et al. [8] TRUS-guided biopsy has become the main method for PCa diagnosis due to its low cost, dynamic real-time imaging and ease of use. At present, TRUS-guided urologists manually operating biopsy needles and ultrasound probes is the gold standard of prostate biopsy technology [9]. However, TRUS image resolution is low and the biopsy process is blindly operated by urologists which completely depends on their experience and skills. Manual operation sometimes shows uncertainty [10]. Errors in centimeters are common in clinical practice [11]. Other than that, it is limited by the low sensitivity of 60% cancer detection. As a result, the diagnostic rate of patients with PSA values of 4–10 ng/ml was only 20%–30% [12,13]. Moreover, urologists usually need to perform more than two biopsies on the patient to be able to diagnose PCa which brings great pain to the patient [14,15,16].
In traditional prostate biopsy surgery, the low soft tissue resolution of the US image may lead to a missed diagnosis or repeated biopsy problems which not only causes unnecessary harm to patients but also delays the optimal timing of treatment. In addition, the biopsy process is performed manually by urologists which requires the doctor to have a certain degree of experience in the operation which greatly limits the reliability and accuracy of the biopsy [17]. A clinical study by Blumenfeld et al. [18] demonstrated a manual targeting accuracy of only 6.5 mm for biopsy. However, the placement plan of seeds in brachytherapy is based on biopsy results so biopsy requires higher precision and accuracy. In clinical practice, due to the low diagnostic rate of traditional biopsy, doctors may over-treat patients to control the progression of cancer resulting in complications such as impotence and urinary incontinence [19].
Magnetic resonance imaging (MRI) has a high contrast of soft tissue and high accuracy in detecting PCa. However, due to its slow imaging speed, limited workspace and the need for good MRI compatibility of biopsy equipment the cost of using MRI alone to guide prostate biopsy is high [20]. However, MRI-TRUS fusion image guided biopsy can combine the high sensitivity of MRI in detecting PCa with the advantages of TRUS low-cost real-time imaging and has high accuracy in the localization and detection of PCa [21,22]. At present, MRI-TRUS fusion image guided prostate biopsy is increasingly used in patients who are highly suspicious of PCa but the operating software used in each biopsy platform is different [23]. The research of Hanske et al. [24] shows that the operation software based on elastic fusion has higher accuracy in detecting PCa than that based on rigid fusion. Shoji et al. [21] showed that in the patients of the prostate imaging and reporting and data system 5 (PI-RADS 5), the detection rate of the use of flexible fusion operation software PCa was 80%. Zhang et al. [25] showed that in patients with PI-RADS > = 3, MRI-TRUS fusion image guided biopsy has a higher PCa detection rate than systematic biopsy.
At present, robots are successfully applied in many fields of human diseases [26,27]. The use of robot assisted urologists for prostate biopsy can make up for many shortcomings of traditional biopsy procedures. One of the biggest advantages is accuracy. The puncture point and biopsy point can be defined and the puncture path can be planned by creating a three-dimensional model through preoperative images. The path error can be compensated in real time during the operation and human error can be reduced during the operation, reaching the preset point with minimum placement error of the biopsy needle [11]. Compared with manual biopsy, the use of robot-assisted prostate biopsy allows the biopsy needle to reach the biopsy site with minimal placement errors not depending on the doctor's experience and skills [11] which greatly improves the accuracy and reliability of the biopsy. A clinical trial study by Tokuda et al. [28,29] showed that prostate biopsies performed using a robot had higher targeting accuracy than those performed manually under the same conditions and environment.
MRI-guided prostate biopsy procedures have been reported to take anywhere from 55 minutes to two hours depending on the technical proficiency of the urologist, the patient's specific situation, etc. [30]. The main problem with performing biopsies manually is that each biopsy requires moving the patient out of the MRI hole twice which not only makes the prostate biopsy procedure last longer but also results in less accurate placement of the biopsy needle due to patient movement which may result in the patient needing repeat biopsies [31]. The use of a fully actuated robot speeds up and simplifies the biopsy procedure, eliminating the need to remove the patient from the MRI hole during the procedure and reducing the time required for the prostate biopsy procedure [10,32,33]. Patel et al. [34] made a clinical evaluation of the MRI-guided prostate biopsy robot designed by his team. The clinical test results show that the operation duration of obtaining two biopsy samples from patients by this robot system is 19 minutes and the positioning accuracy is higher than that by manual operation. As a result, more patients can be diagnosed in the same amount of time with a robot-assisted urologist performing a prostate biopsy. It also frees up the hands of the urologist, reducing the labor intensity of urologists and the pain of patients and increases the accuracy of biopsy.
From the above analysis, it can be seen that the image-guided biopsy robot has the advantages of a high degree of visualization and automation, does not rely on the skills and experience of operators, avoids medical accidents caused by human error, reduces the work intensity and operation time of urologists and so on. This ensures patient safety and improves the reliability and accuracy of biopsy operations. In summary, the use of image-guided robots instead of manual biopsy operations is an inevitable trend in the future. The ultimate purpose of this article's research is to present the reader with research advances and technical trends in image-guided prostate biopsy robotics to help the reader with more in-depth research. To facilitate quick reading, the overall structure of this paper is shown in Figure 2. The main contributions of this paper are outlined as follows:
1) MRI, ultrasound (US) and image fusion are the main medical image guidance modalities for prostate biopsy robots and this paper provides a quantitative literature review of the technologies and principles of the three medical image-guided prostate biopsy robotic systems.
2) As actuators and materials are the main differences between different image-guided robots, this paper presents and compares the actuators and materials of different types of image-guided robots.
3) Image-guided positioning technology is one of the main research directions for prostate biopsy robots which is analyzed and discussed in this paper.
4) This paper summarizes the research progress of prostate biopsy robots and looks forward to the future development trend.
In this paper, we used percutaneous, prostate biopsy, image-guided and puncture as index terms, each of which contains the word robot. The periodical publications and conference papers of image-guided prostate biopsy robot system published in the databases of Engineering Village, IEEE Xplore, Web of Science, PubMed, Google Scholar, Science Direct and Elsevier from 1995 to 2023 were reviewed quantitatively. The reason for choosing this time range is that in April 1995, the robot-assisted urologist was used for the first time to perform prostate biopsy on patients. In order to limit the scope, the following contents are excluded from this quantitative article review including patents, unpublished commercial systems, surgical training simulators and the interaction between biopsy needles and soft tissues during the biopsy. The reason the patent is not included is that it only contains the system design of the prostate biopsy robot and there is no necessary information such as the compatibility of clinical trial results with imaging to understand the overall usability of the system.
Since the success of the first clinical trial in 1995 by Rovetta et al. [10] of the Politecnico di Milano using a robot to perform the prostate biopsy in a patient, researchers have made great progress in the development of a robotic for prostate biopsy, As shown in Table 1.
Year | FA | Institution | IM | PS | NTPA | Actuation | DOF | Status | Reference |
2012 | Seifabadi | Queen's University | MRI | Perineum | 6 | Virtual prototyping design | [31] | ||
2010 | Krieger | Johns Hopkins University | MRI | Rectum | 2.4 mm | Piezoelectric actuators | 3 | Phantom test | [32] |
2019 | Patel | Worcester Polytechnic Institute | MRI | Perineum | 3.7 mm | Piezoelectric actuators | 6 | Phantom test | [34] |
2005 | Fichtinger | Johns Hopkins University | MRI | Rectum | 1.8 mm | 3 | Clinical patient test | [35] | |
2011 | Krieger | Johns Hopkins University | MRI | Rectum | 1.1 mm | 6 | Clinical patient test | [38] | |
2013 | Krieger | Johns Hopkins University | MRI | Rectum | 2.4 mm | Piezoelectric actuators | 8 | Phantom test | [39] |
2012 | Bohren | Johns Hopkins University | MRI | Rectum | Piezoelectric actuators | 9 | Virtual prototyping design | [40] | |
2007 | Elhawary | University of Navarra | MRI | Rectum | 2.3 mm | Piezoelectric actuators | 5 | Phantom test | [41,42,43] |
2008 | Goldenberg | University of Toronto | MRI | Perineum | < 2 mm | Piezoelectric actuators | 6 | Phantom test | [44,45] |
2008 | Fischer | Johns Hopkins University | MRI | Perineum | 0.94 mm | Pneumatic actuators | 4 | Phantom test | [46,47,48] |
2010 | Schouten | Radboud University | MRI | Rectum | 3.0 mm | Pneumatic actuators | 5 | Phantom test | [49,50,51] |
2010 | Song | Johns Hopkins University | MRI | Perineum | < 0.5 mm | Pneumatic actuators | 4 | Phantom test | [52] |
2016 | Seifabadi | Queen's University | MRI | Perineum | 0.9 mm | Pneumatic actuators | 6 | Phantom test | [55,56] |
2012 | Su | Worcester Polytechnic Institute | MRI | Perineum | 1 mm | Piezoelectric actuators | 6 | Phantom test | [57,58,59] |
2013 | Eslami | Johns Hopkins University |
MRI | Perineum | 4 | Virtual prototyping design | [60] | ||
2015 | Li | Johns Hopkins University |
MRI | Perineum | 0.92 mm | Piezoelectric actuators | 7 | Phantom test | [62] |
2014 | Stoianovici | Johns Hopkins University | MRI | Rectum | < 2.58 mm | Pneumatic actuators | 3 | Live animal tests | [64] |
2018 | Stoianovici | Johns Hopkins University | MRI | Rectum | 0.645 mm | Pneumatic actuators | 3 | Phantom test | [65] |
2015 | Chen | Fraunhofer MEVIS Institute | MRI | Rectum | Pneumatic actuators | 5 | Virtual prototyping design | [66] | |
2016 | Stoianovici | Johns Hopkins University | MRI | Perineum | 2.55 mm | Pneumatic actuators | 6 | Clinical patient test | [67] |
2022 | Aleong | University of Toronto | MRI | Perineum | 5 mm | Piezoelectric actuators | 6 | Phantom test | [69] |
2022 | Biswas | University of Central Florida | MRI | Perineum | motors | 4 | Virtual prototyping design | [70] | |
2005 | Phee | Nanyang Technological University | US | Perineum | < 2.5 mm | 9 | Clinical patient test | [88] | |
2009 | Ho | Singapore General Hospital |
US | Perineum | < 1 mm | motors | 6 | Phantom test | [89,90] |
2012 | Zhang | Harbin University of Science and Technology | US | 5 | Virtual prototyping design | [91] | |||
2012 | Long | Grenoble University Hospital | US | Perineum | 2.73 mm | motors | 7 | Phantom test | [92] |
2013 | Poquet | University of Pittsburgh Medical Center | US | Rectum | 3.1 mm | motors | 6 | Clinical patient test | [93] |
2019 | Lim | Johns Hopkins University |
US | Rectum | 1 mm | motors | 4 | Clinical patient test | [97] |
2022 | Yan | Harbin Institute of Technology | US | Rectum | 1.594 mm | motors | 8 | Phantom test | [98] |
2017 | Pisla | Technical University of Cluj-Napoca | MRI-US | Perineum | 1–2 mm | motors | 10 | Phantom test | [101] |
2021 | Maris | University of Verona | MRI-US | Perineum | 1.30 ± 0.44 mm | motors | 5 | Phantom test | [104] |
2021 | Wang | Harbin Institute of Technology | MRI-US | Perineum | 1.44 mm | motors | 5 | Phantom test | [105] |
2021 | Xiao | Southern University of Science and Technology | MRI-US | Urethra | 1.2 mm | motors | 3 | Phantom test | [106,107] |
Note: Abbreviations: FA, First author; IM, Imaging modality; PS, Puncture site; NTPA, Needle tip positioning accuracy; DOF, Degree of freedom. |
During MRI-guided prostate biopsy, patients need to frequently enter and exit the imaging space which affects the accuracy of tumor localization and greatly reduces the reliability of the biopsy. The use of robotic-assisted prostate biopsy could eliminate the need to move the patient out of the imaging space, simplifies the biopsy procedure and provides higher biopsy accuracy. However, due to the limited internal space and working space of the MRI hole coupled with the limitation of the high-intensity magnetic field, the design of the robot requires a small footprint and good MRI compatibility. The prostate biopsy robot structure for MRI-guided is shown in Figure 3.
In 2005, Fichtinger et al. [35] designed a 3-degree-of-freedom (DOF) prostate biopsy robot system called ATP-MRI. The needle guide in the system contains two needle channels for the proximal and distal portions of the prostate. This is the first successful robotic prostate biopsy system that combines MRI imaging and tracking coils for real-time localization of anatomical structures during the biopsy. The advantage of this device is that it allows multi-angle needle insertion, increasing the flexibility of the biopsy procedure. However, the ATP-MRI is a manual prostate biopsy device and the accuracy of the biopsy depends on the operator's proficiency. Its in vivo clinical in-plane displacement error is 1.8 mm and the system is in phase I clinical trials for prostate biopsy [36,37]. In 2010, Krieger et al. [32] used a similar mechanism to the APT-MRI manipulator, combining drive needle alignment with manual needle insertion through a drive needle guide, reducing the intervention time for biopsy procedures and improving biopsy needle placement accuracy. In 2011, the team designed a 6-DOF robotic system called APT-II using a hybrid tracking approach which solved the problem of needing to customize the active tracking MRI scanner sequence in the APT-MRI system, simplifies the workflow and makes APT-II system error and operation time lower than APT-MRI system [38]. This system can be used on any MRI scanner without extensive system integration and calibration. In 2013, the team proposed the APT-III system. Based on APT-II, a 2-DOF piezoelectric ceramic motor-driven needle guide was designed and manual biopsy needle insertion is performed after positioning along the desired trajectory [39]. Subsequently, the team Bohren et al. [40] designed the APT-IV virtual prototype, adding a drive needle insertion module to the APT-III system. It reduces the workload and human intervention of the urologist during the procedure, enhances the automation of the ATP system and effectively improves the accuracy of the biopsy procedure but this system is still in the virtual prototype stage and needs to be further developed for use in the clinic.
In 2007, Elhawary et al. [41,42] developed a 5-DOF prostate biopsy robotic system based on a modular design, each module consisting of a 1-DOF stage with actuators and position coding device. To improve the localization accuracy of the system within the MRI scanner, a real-time device with passive micro-coil markers was used to track the position and orientation of the needle in three dimensions thereby improving patient safety during prostate biopsy procedures. In 2010, the team achieved real-time image guidance during prostate biopsy through the development of an imaging pulse sequence [43]. Capable of positioning and driving within the scanner's field of view close to the patient without affecting the imaging quality of the MRI scanner but also providing real-time tracking of end-effector positions and updating of scan plane images, enabling biopsy needle trajectories and target anatomy dynamic updating of structures. The advantage of this system is that it provides a clear, targeted biopsy of the suspected area of the prostate in real time, avoiding the multiple blind insertions of conventional TRUS guidance. However, this system is more expensive and the biopsy procedure takes longer.
In 2008, Goldenberg et al. [44,45] designed an MRI-guided 6-DOF prostate biopsy robotic system. The advantages of the robot are its lightweight and compact structure. Its tip position error is less than 2 mm and the prostate biopsy can be performed in the MRI hole during the operation. However, due to the obvious noise generated by the motor operation, image artifacts occurred in this system during the biopsy process which affected the accuracy of the biopsy and increased the duration of the operation.
In 2008, Fischer et al. [46,47,48] designed a 4-DOF prostate biopsy robotic system consisting of two sets of linkages. The robot is installed on a manual linear slide rail to quickly collect prostate biopsy tissue. This system uses a pneumatic drive that has better MRI compatibility and the patient does not need to be moved out of the imaging space during the biopsy process which has a similar surgical process to traditional TRUS-guided prostate biopsy. In addition, the team also conducted a comprehensive evaluation of the system's working space, placement accuracy, MRI compatibility and workflow and the evaluation results show that the system has good MRI compatibility. However, its software interface and control system needs to be further improved to shorten the operation time and enhance the targeting accuracy of biopsy.
In 2010, Schouten et al. [49,50,51] developed a 5-DOF prostate biopsy robotic system made of plastic, the first robotic system for real-time MRI-guided needle positioning. The advantage of this system is that it can be controlled remotely and a safety mechanism is built into the needle catheter which effectively improves the safety of the biopsy process. The team performed risk analysis, mechanical testing and RF safety testing related to needle tip heating and also assessed the MRI compatibility, accuracy and speed of the system, showing that this system meets patient safety requirements, effectively reduces procedure time and has good MRI compatibility. However, the size of the robot is large, the needle tip cannot completely cover the patient's prostate during the biopsy process and the targeting accuracy is not high.
In 2010, Song et al. [52] designed a 4-DOF prostate biopsy robot system based on a modular and damping mechanism, using a mechanism with external damping synchronously and optimizing the working space to solve the problem of poor robot controllability. The advantage of this system is that it takes up less space but has lower target positioning accuracy. That same year, the team tested the system's MRI signal-to-noise ratio (SNR) and detailed the overall procedure and system integration [53]. In order to improve the system accuracy, optimize the workspace and shorten the operation time, in 2012, the team added a 1-DOF master-slave needle driver module on top of the robot to realize the remote operation of the robot system. On this basis, the team evaluated the accuracy of the system and solved the disinfection problem of the robotic system [28,31,54]. To reduce the number of times the patient is removed from the scanner during biopsy procedures and to continuously compensate for positioning errors caused by needle-tissue interaction. In 2016 the team developed a 2-DOF needle steering module to integrate it with a previously proposed robotic system [52]. The needle-steering module employs fiber Bragg grating (FBG) force sensors to address the inherent friction of piezoelectric actuators. The system can be controlled by the urologist through a teleoperation method. The positioning error was reduced from 4.2 millimeters to 0.9 millimeters compared to the system previously proposed by the team [55,56].
In 2012, Su et al. [57] designed a 6-DOF prostate biopsy robotic system based on a modular design. The system consists of a modular 3-DOF casing driver with a fiducial tracking frame and a 3-DOF-driven Cartesian platform. It enables MRI-guided placement of a curved, steerable active cannula and allows simultaneous cannula movement and imaging. This system has the advantage that the puncture path can be changed in real time during the procedure but image artifacts may occur. In 2013, the team designed a bending mechanism with an integrated Fabry-Perot interferometric (FPI) sensor fiber for measuring needle insertion force and integrated it into the original robotic system, performing finite element analysis to optimize its correct force-deformation relationship [58]. In the same year, the team conducted phantom experiments to evaluate the feasibility of the system's workflow and the flexibility of the modular design approach [59].
In 2013, Eslami et al. [60] designed a 4-DOF prostate biopsy robot consisting of two anterior and posterior parallel trapezoidal platforms, each with 2-DOF. Compared with the basic robot designed by Song et al. [52,53] the team replaced the pneumatic actuation with a non-magnetic ultrasonic motor and the trapezoidal-link mechanism in the system replaced the original triangular mechanism. As a result, the lateral displacement is converted into vertical motion, the overall size of the system is reduced and better control performance and accuracy are obtained but the overall manufacturing error of the robot is larger which affects the target positioning accuracy to some extent. To reduce the positioning error of the needle tip and ensure the rigidity of the needle entry structure, the team made corresponding improvements and designs at the weak points of the structure through finite element analysis of the structure. Subsequently, the team lowered the height of the front and rear platforms by about 26 mm which solved the interference of this manipulator with the legs and retained the same working space [61]. In 2015, the team designed an MRI-compatible 3-DOF biopsy needle driver. The basic robot and the biopsy needle driver can be sterilized separately. Since both the patient and the robot remain in the MRI scanner bore during the biopsy, there is no need to move the patient out of the imaging space thereby reducing the time required for clinical biopsy procedures and improving biopsy accuracy [33,62]. In 2016, the team designed a multi-DOF robot drive system controlled by one motor driving, effectively reducing the number of motors required for the robot system [63]. In 2019, based on the original robotic [15,61], the team developed a 4-DOF prostate biopsy robotic system based on a modular approach [34]. The team describes the clinical workflow, and the system has received Institutional Review Board (IRB) approval for clinical trials, using phantom testing to yield two target localization errors of 4.0 mm and 3.7 mm, respectively. However, the needle of this system requires manual insertion which is time-consuming.
In 2014, Stoianovici et al. [64] developed a 3-DOF prostate biopsy robotic system called MRI-Safe in which 2-DOF was used to position the needle guide and 1-DOF was used to remotely preset the needle insertion depth. Needle insertion and biopsy are performed manually and the device assists physicians with biopsy by automatically positioning the biopsy needle on the target and setting the depth of needle insertion under MRI-guided. Since the robot is made of non-magnetic, non-conductive materials and uses a pneumatic drive, this system has the advantage of good MRI compatibility and can be used safely in MRI environments but the robot structure is less rigid. In 2018, the team designed a manipulator with a remote center of motion (RCM) parallelogram structure. Compared with the original manipulator [64], the structural rigidity and lateral clearance of the patient were greatly improved which is more in line with the expected clinical prostate biopsy workflow [65]. In 2015, Chen et al. [66] developed an MRI-guided 5-DOF prostate biopsy robotic system based on pneumatic actuation. Each part was tested and confirmed to be non-magnetic before robotic assembly and this system has good MRI compatibility. Compared with the ATP-MRI robotic system [35], this system has a more flexible needle trajectory and a larger working space.
In 2016, Stoianovici et al. [67] developed a 6-DOF prostate biopsy robotic system consisting of a parallel link structure. This system is pneumatically driven. When a malfunction occurs during the surgical process, the power supply of the pneumatic valve is disabled to ensure the patient's safety. The insertion of the biopsy needle is performed manually by the physician and the system is approved by the Food and Drug Administration (FDA) for use in the MRI setting. In 2017, the team conducted a feasibility and safety study of the system on five patientst The advantage of this system is that it has good MRI compatibility and allows safe and accurate manipulation of the robot in the MRI environment. However, the biopsy process is time-consuming [68].
In 2022, Aleong et al. [69] designed a modular 6-DOF prostate intervention robot system guided by MRI. The robot consists of a base, an arm and an end-effector, all parts of which are made of MR safe material and have good MRI compatibility. The advantage of this system is the small footprint and the ability to perform multiple non-parallel needle insertions under real-time MRI guidance with each needle being able to be angled to completely cover the prostate, compared to other robotic systems that insert parallel needles. However, needle insertion requires manual manipulation by the urologist and requires the patient to be moved out of the imaging space during the procedure which increases the procedure duration and needle placement errors to some extent.
In 2022, Biswas et al. [70] designed an MRI-guided 4-DOF prostate robot prototype consisting of a stack of four parallel disks. The advantage of this prototype is that it has high structural rigidity and can be tilted ± 15° during needle insertion to avoid vital organs and sensitive tissues and completely cover the patient's prostate. However, this prototype is currently driven by a DC motor which has poor MRI compatibility and cannot perform biopsy operations in an MRI environment. It needs to be replaced with an MRI-compatible piezoelectric motor in further development and tested for MRI compatibility. In addition, the controllability of the needle during puncture is poor which reduces the accuracy of the biopsy to a certain extent.
In the MRI environment, traditional electromagnetic induction motors cannot be used in actuators for prostate biopsy due to the high density of the magnetic field and the limited space in the bore. The actuator is required to have a compact size and non-magnetic properties and cannot affect the imaging quality of the scanner such as the difference in magnetic susceptibility of surrounding objects and metals leading to image artifacts caused by magnetic field interference [71]. This paper summarizes the driving methods of the MRI-guided prostate biopsy robot including pneumatic, hydraulic and piezoelectric driving methods.
Pneumatic actuation. Pneumatic actuation is widely used in industrial and medical equipment due to its low maintenance and low cost [72]. Both pneumatic and hydraulic drives are fluid drives and both require larger pumps, resulting in more complex systems [73]. Compared with hydraulic drives, pneumatic drives have better connectivity and cleanliness [74]. Pneumatic actuation uses compressed air to drive biopsy robots and has good MRI compatibility. But the control precision is lower due to the compressibility of the air in the classic pneumatic drive. However, biopsy robots require high positioning accuracy so pneumatic actuation requires precise servo control and expensive proportional valves. In 2007, Stoianovici et al. [67,72] developed a pneumatic stepper motor (PneuStep) with optical sensor coding for a 6-DOF biopsy robot system which is driven by a pneumatic drive to generate pulsed pressure to drive the motor to work without electricity intervention with good MRI compatibility. Compared with the traditional pneumatic drive, PneuStep greatly improves the control accuracy but the price is relatively expensive. In 2007, Plante et al. [75,76] developed a robotic system for prostate biopsy using a dielectric elastomer actuator (DEA) with 12 DEA actuators to operate within the MRI cavity. The team evaluated the prototype of the system in an MRI environment with an average positioning error of 3 mm. The experimental results show that the system has good MRI compatibility but there are problems such as insufficient reliability and driving force in the biopsy process. In 2009, in order to improve the driving force and reduce the structural size of this system, the team replaced DEA with 12 pneumatic air muscles (PAM) of similar cost. Compared with the original system, this system has greater rigidity and working space. The positioning error is 3 mm which meets clinical needs [77,78,79]. In 2012, to improve the positioning accuracy of the system, the team updated the 12 PAMs in the system to 20 PAMs and verified through experiments that the closed-loop error of the system under MRI guidance was less than 0.5 mm [80,81].
Hydraulic actuation. The hydraulic actuation can reasonably design the pipeline according to the requirements of the system and has the advantages of good MRI compatibility and stable transmission. However, it is rarely used in prostate biopsy robotic systems due to its fluid leakage and cavitation problems [82].
Piezo actuation. Piezoelectric actuation is also called ultrasonic drives. Different from electromagnetic induction motors, piezoelectric motors are made of non-magnetic ceramics. Piezoelectric drive and pneumatic drive are the main driving methods used in prostate biopsy robots. Compared with the pneumatic drive, the piezoelectric drive has significant advantages such as compact structure, scalability and easy control [74,83]. However, piezoelectric driving requires high-frequency pulse voltage to generate sufficient driving force and the control circuit has electrical noise which can cause serious image artifacts [84]. Piezo drives can only be used in an MRI environment when the piezo motor is a safe distance from the MRI bore or properly shielded [82]. In order to reduce the electrical noise of the control circuit, Su et al. [85] designed a 6-DOF prostate biopsy robot system in 2014. This system uses a piezoelectric driver and designed a low-noise driver board. The phantom test results show that the system has good MRI compatibility. To make the piezoelectric motor have a certain safe distance from the MRI bore, the researchers used long-distance transmission. The most commonly used method is cable transmission. In 2019, Velazco-Garcia et al. [86] designed a 4-DOF robotic virtual prototype for prostate biopsy based on cable transmission. To meet the requirements of different hospitals, this prototype adopts a modular design.
Due to the limitation of the strong magnetic field in the MRI environment during the MRI scanning process, the incorrect selection of robotic materials will affect the imaging quality of the scanner and produce image artifacts. Therefore, the use of ferromagnetic materials is prohibited. The selection of MRI-compatible materials must have non-magnetic and dielectric properties [87]. MRI-guided prostate biopsy robots usually use materials with good MRI compatibility such as high-strength plastics, titanium and ceramics.
US imaging has the advantages of low cost and good dynamic real-time imaging capability. Urologists need to constantly adjust the TRUS probe manually to make the US image clear enough during the biopsy operation but the manual operation of the TRUS probe and the biopsy needle is less safe. However, a robot-assisted prostate biopsy can reduce the work intensity of urologists and improve the reliability of biopsy procedures. The prostate biopsy robot structure for US-guided is shown in Figure 4.
In 2005, Phee et al. [11,88] designed a 9-DOF prostate biopsy robot system. In this system, the gantry structure, the TRUS probe and the control of the biopsy needle all have 3-DOF. This system can collect continuous two-dimensional (2D) images of the patient's prostate by controlling the TRUS probe to create a three-dimensional (3D) model. Pre-biopsy, urologists can use this system to define the biopsy point and needle entry point within the 3D model and predict the required trajectory of the biopsy needle during the biopsy. Manual biopsy of the prostate is performed on the patient with the aid of this system if the doctor considers the results to be feasible. The advantage of this system is that multiple core biopsies can be performed at a single puncture site but the accuracy of the biopsy is more dependent on the proficiency of the urologist.
In 2009, Ho et al. [89,90] designed a US-guided 6-DOF prostate biopsy robotic system which consisted of a gantry equipped with a positioning system, a gun holder for fixing the biopsy gun and needle and a TRUS probe holder. To ensure the biopsy needle can reach any position of the prostate and reduce the risk of urethral injury, the system uses TP combined with the proposed bipyramidal approach for prostate biopsy. The advantages of this system are that it is highly safe, does not damage the patient's urethra during the biopsy and has a reproducible accuracy of < 1 mm. However, it is still in the phantom test phase and actual tissue models still need to be used to verify the accuracy of the system.
In 2012, Zhang et al. [91] designed a miniature cartesian type 5-DOF prostate biopsy robot based on TRIZ theory, determined the structural design scheme through TRIZ theory and improved the function of the needle insertion system. However, this robot is still in the virtual prototyping design phase and its feasibility needs to be verified by further practical experiments. In the same year, Long et al. [92] developed a US-guided 7-DOF prostate biopsy robotic system named Prosper. The robot consists of a biopsy needle insertion module and a location module. This system with needle rotation and angled insertion has the advantage that for the first time intraoperative tracking of prostate motion has been achieved, improving biopsy accuracy. However, targeting on the left side of the prostate is low and still needs further improvement.
In 2013, Poquet et al. [93] designed a US-guided 6-DOF prostate biopsy robotic system called Apollo. Based on the concept of cooperative operation, this system has two operation modes free and blocked to meet the needs of urologists in different situations. Apollo adopts a hybrid drive mode, using motors and brakes for the first three joints and the rear three joints respectively. The experimental results in vivo and in vitro show that the robot has high positioning accuracy for a given direction and position in the locked mode and conforms to the doctor's operating habits in the free mode [94,95]. In 2016, the team conducted a trial of TRPB in 10 patients and the results showed that urologists were significantly more accurate with the Apollo-assisted prostate biopsy than without the Apollo-assisted [96]. However, this system does not have automatic updating of the ultrasound probe position and requires human intervention which reduces the accuracy of needle tip positioning to some extent.
In 2019, Lim et al. [97] designed a US-guided 6-DOF prostate biopsy robotic system. The robot consists of drivers and an RCM module. The RCM module adopts a belt drive and is more compact than the classic rod-type RCM. The advantage of this system is that the operator can accurately guide the biopsy needle to the biopsy point through this system whether the operator is skilled in clinical biopsy. The positioning accuracy of the needle in the clinical trial is about 1 mm and the results show that this system is very feasible and safe for assisting prostate biopsy. However, this system does not have a support structure to maintain the patient's leg posture which to some extent affects the accuracy of needle tip positioning.
In 2022, Yan et al. [98] designed a US-guided 8-DOF parallel prostate interventional robot system, which consists of a 2-DOF needle insertion structure, a 2-DOF ultrasound probe movement structure, and a 4-DOF needle positioning mechanism composition. The advantage of this robot is that the structural design is relatively compact, and the hidden danger of collision between the patient and the robot during the operation is eliminated due to the parallel structure. However, this system is still in the phantom test phase and animal experiments are still needed to further study its feasibility in clinical operations.
Because there is no limitation of the strong magnetic field in the US environment, the driving device will not affect the imaging of the US scanner so researchers usually use motors for driving.
In the US environment, the material of the robot does not affect the imaging of the US scanner. In order to meet the required stiffness of the robot and reduce the weight of the equipment, researchers mostly choose dense materials such as ceramics, aluminum alloys and engineering plastics.
Clinical studies have shown that MRI-TRUS fusion image-guided biopsy increases the detection rate of high-risk PCa by nearly 30% compared to non-targeted methods [99]. Since the fusion image can provide more detailed anatomical information than the single modality image, it can greatly improve the precision and accuracy of biopsy surgery [100]. At present, fusion image-guided prostate biopsy robots have attracted great attention from researchers and the prostate biopsy robot structure used for fusion image guidance is shown in Figure 5.
In 2017, Pisla et al. [101] designed an MRI-TRUS fusion image-guided 10-DOF prostate biopsy robotic system named BIO-PROS-1. The robot consists of two parallel fully automatic modules that work together to control the ultrasound probe and biopsy gun respectively. The advantage of this system is that the urologist only needs to check the reasonableness of the sampling points and supervise the safety of the system and the robot can automatically select the sampling points for the biopsy procedure, effectively reducing the duration of the procedure and the labour intensity of the doctor. However, the control system for this system needs to be further developed. In the same year, the team carried out the development of the BIO-PROS-1 control system [9] and the verification of the closed-loop control system [102]. The test results show that the system has high positioning accuracy. Subsequently, the team improved the guided ultrasound probe module of BIO-PROS-1 and named it BIO-PROS-2 so that the robot could easily enter between the patient's legs [103].
In order to minimize human errors, give robots more autonomy. In 2021, Maris et al. [104] designed an MRI-TRUS fusion image-guided 5-DOF prostate biopsy robotic system called PROST. The robot consists of two articulated arms that move along parallel planes. In the planning and execution phase, the needle guide is automatically executed by the robot and the determination of the needle entry point and the biopsy point and the insertion of the biopsy needle are completed by the doctor. The positioning accuracy of PROST is about 1 mm. The advantage of this system is that the robot has a certain degree of autonomy. However, the needle insertion still needs to be done manually by the urologist which has not completely eliminated human errors. It is currently in the phantom test phase and the clinical feasibility needs to be further verified by cadavers experiments.
In 2021, Wang et al. [105] designed an MRI-TRUS fusion image-guided 5-DOF prostate biopsy robotic system. In this system, the biopsy needle clamping mechanism is designed on the TRUS probe so that the rotation of the TRUS probe can drive the biopsy needle to complete the yaw movement. The advantage of this system is that it takes up less space and reduces the number of needle insertions compared to traditional biopsy procedures. However, this system is still in the phantom test phase and further experiments with animals are needed to verify the safety and accuracy of the system.
In 2021, Xiao et al. [106,107] designed an MRI-TRUS fusion image-guided 3-DOF prostate biopsy robotic system. The robot uses a tubular double-roller bending mechanism that allows the flexible needle designed by the team to rotate and bend simultaneously. This flexible needle is integrated into a continuous tube, allowing the biopsy needle to bend arbitrarily in the range of 16.0°–55.8°. The translational movement combined with the bending system can reach any point in the workspace. The advantage of this system is that it takes up less space, can launch biopsy needles from different angles and has a shorter needle path. This is the first robot for transurethral prostate biopsy. However, because the prototype is a double model and has a large size, it cannot be used for clinical transurethral prostate biopsy at present.
In recent years, with the continuous development and improvement of image processing technology [108,109,110,111,112], it has been widely used in the field of robotics research and one of the important applications is for lesion localization. The robot obtains the relative position information of itself and the lesion by processing and analyzing the image to perform more accurate motion control. In addition, the accuracy of lesion location plays an important role in making preoperative biopsy plans and intraoperative real-time guidance.
MRI is a 3D imaging technique which has the advantages of high soft tissue resolution, no radiation and accurate display of prostate tissue boundaries. Due to the limited working space and high magnetic field, the structure of the robot is required to be compact enough to operate in the MRI hole and the materials and actuators must be compatible with MRI which leads to high design costs for the robot. The degree of automation and system functions of the robot designed by the researchers are different and the guidance process is generally summarized as follows: First, the patient is placed on the MRI scanner table, an initial MRI scan is performed on the patient to locate suspicious tissue and the urologist makes a biopsy plan based on the MRI images and cleans and disinfects the biopsy equipment. To realize the automatic biopsy of the robot, it is necessary to register the robot coordinate system with the MRI image coordinate system so that the two can be unified into the same coordinate space. Then, the doctor selects a biopsy position and the robot automatically sets the insertion direction and depth of the biopsy needle according to the biopsy position and automatically places the biopsy needle through the coordinated movement of the joints. After the setting is completed, the robot triggers the biopsy device to obtain the patient's pathological tissue. At the same time, the patient is scanned by MRI to determine whether the needle reaches the target point. If it fails, the process is repeated. After completing the above steps, the robot repositions to the next biopsy position and repeats the above process for subsequent biopsies under the supervision of doctors.
In 2007, DiMaio et al. [113] used a two-step non-rigid registration technique based on finite element and thin-plate spline techniques to register preoperative 0.5T MRI images with 1.5T MRI images. On this basis, a prostate biopsy robot-assisted system was developed. This system is visualized through 3DSlicer. Urologists can identify the tumor location through visualization and make a biopsy plan before surgery. The phantom test results show that with the assistance of this system, the needle point positioning error of the robot is within 2 mm.
In 2008, Mewes et al. [114] developed a prostate biopsy robot-assisted system. The system generates biopsy needle puncture trajectories as well as intraoperative visualization and correction of biopsy needle puncture paths. In 2010, Tokuda et al. [47] developed a prostate biopsy robot-assisted system. This system registers the robot to the patient image coordinate system based on the calibration of the Z-frame and can visualize the 3D puncture trajectory of the intraoperative biopsy needle in real time. The experimental results show that the registration error is 2.6 mm. In 2015, Patel et al. [115] developed an intraoperative continuous MRI-guided robotic-assisted system that segmented the needle tip of the intraoperative MRI image and overlayed the original image to guide the needle path in real time. The phantom test showed that with the assistance of the system the target positioning error was 2.5 ± 0.47 mm.
Segmentation of prostate MRI images plays a crucial role in the target location but the complexity of MRI image structures and the lack of partial anatomy boundaries make accurate prostate segmentation in MRI images very challenging. To this end, in 2022, Qin et al. [116] combined the statistical shape model (SSM) with the convolutional neural network (CNN) to propose a two-branch prostate MRI segmentation model. The first branch uses SSM-Net to generate the prostate border to obtain a border distance map and the second branch uses ResU-Net to obtain a probability map from the input image. Perform the optimal weighted sum of the results of the two branches to obtain the final segmentation result. Experimental results show that the results of dice similarity coefficient and average surface distance are 0.907 and 1.85 mm respectively which is very competitive with other methods.
In 2023, Wang et al. [117] proposed a two-step CNN model where the first step used a classification model containing a squeeze excitation module for determining whether the MRI images contained prostate and the second part used an attention and residual module to improve the U-Net segmentation model named RAU-Net. Experimental results showed that the values of the Dice similarity coefficient and true positive rate indices were 0.860 and 0.882, both higher than nnUNets. In the same year, Li et al. [118] proposed a segmentation model consisting of a contrastive learning module, a generator and a discriminator to improve the segmentation accuracy of PCa localization and edge recognition. Among them, the generator adopts the U-Net segmentation model with parallel expansion convolution module named dila-UNet which effectively improves the positioning ability of the model. The experimental results show that the Dice, pixel accuracy, intersection over union and 95% hausdoff distance of the method proposed by the author are 81.1%, 85.3%, 70.8%, and 9.48 respectively.
The US has the advantages of dynamic real-time imaging, no radiation and low cost and is the preferred method for prostate biopsy. The degree of automation and system functions of the robot designed by the researchers are different, and its guidance process is generally summarized as follows: First, the biopsy protocol is selected by the urologist such as the standard systematic 12-core needle biopsy protocol. Subsequently, the doctor cleans and disinfects the biopsy equipment and mounts the ultrasound probe on the robot. The ultrasound probe is inserted into the patient's rectum by a robot and the ultrasound probe needs to be close to the patient's rectal wall to obtain a relatively clear US image. In order to realize the automatic biopsy of the robot, it is necessary to register the robot coordinate system with the US image coordinate system so that the two can be unified into the same coordinate space. On this basis, the doctor selects a biopsy location and the robot automatically places the biopsy needle through the coordinated movement of the joints according to the biopsy location. In the real-time US image, the biopsy needle appears as a point with echo shadows and the robot automatically sets the insertion depth and direction of the needle. After the setting is completed, the robot triggers the biopsy device to obtain the patient's pathological tissue. After completing the above steps, the robot repositions to the next biopsy position and repeats the above process for subsequent biopsy under the supervision of the doctor.
In 2005, Xiao et al. [119] developed a US-guided prostate biopsy assist system written in C++. This system acquires transverse US image frames with pre-defined spacing from a US scanner and models the prostate in 3D based on a non-uniform rational B-splines algorithm. The puncture point is defined by the urologist and the feasible puncture path is established in the 3D scene through this system. The clinical test results show that the robot's needle tip positioning error is less than 2.5 mm with the assistance of this system which shows the feasibility of this system. In 2011, Baumann et al. [120] proposed a 3D TRUS-guided prostate biopsy-assist system that employs a coarse-to-fine registration strategy to rapidly estimate prostate motion during biopsy procedures. In 2014, Abayazid et al. [121] designed a framework for 3D localization of preoperative targets for prostate biopsy based on US-guided. This framework combines needle tip tracking algorithms, target localization and control algorithms. The centroid of the target is obtained from the US image of each frame through image processing technology. Then, the centroid is input into the control algorithm to guide the orientation of the needle. In the phantom test with an inclined surface, the average positioning error is 0.85 mm. In 2018, Busam et al. [122] designed a 3D TRUS-guided prostate biopsy assist system. This system, based on visual SLAM, does not rely on specific markers and can track the position of the TRUS probe in real-time during the biopsy.
Due to the fuzzy boundary of the prostate in US images, the low signal-to-noise ratio and low contrast, this poses a great challenge to the accurate segmentation of the prostate. To improve segmentation accuracy, in 2022, Peng et al. [123] proposed a semi-automatic prostate TRUS segmentation method called H-ProMed based on data point priors which combined an evolutionary neural network with an improved master curve method. The experimental results show that the average Dice similarity coefficient is 96.8%, compared with 95% and 92% for the other two methods. The accuracy and Jaccard similarity coefficient were 96.4% and 95.7%, respectively. In the same year, to alleviate the cost of data annotation for prostate US image segmentation, Xu et al. [124] proposed for the first time a semi-supervised learning based on shadow loss and shadow enhancement for prostate US image segmentation named SCO-SSL. The input image and intermediate feature images were processed by a shadow loss and shadow enhancement mechanism and experimental results showed that compared with other advanced fully supervised methods this team proposed method achieves competitive results using only 20% of the labeled training data and a Dice similarity coefficient reduction of about 0.6%.
In 2023, Wang et al. [125] improved the U-Net model named DSU-Net by combining deformable convolution with shear transform to replace the convolution of the original segmentation model. The improved segmentation model is more sensitive to prostate US image boundary information and experimental results show that the Dice coefficient and Jaccard similarity coefficient of DSU-Net are 0.957 and 0.925, respectively, which are 0.034 and 0.039 higher than those of U-Net.
MRI has the advantage of high soft tissue contrast and can obtain relatively clear three-dimensional images of the prostate. Urologists can accurately obtain the distribution of lesions in patients based on MRI images. However, the limited operating space in the MRI hole and the limitation of MRI compatibility lead to higher design costs for the robot, longer operation duration and the inability to use conventional medical devices. TURS has the advantage of low-cost real-time imaging but has poor soft tissue contrast. In contrast, the fusion of the two images can take full advantage of the high soft tissue contrast of MRI images and the advantages of low-cost real-time imaging of TRUS. Compared with using MRI-guided prostate biopsy surgery, the advantages of fused images are lower surgical cost and dynamic real-time imaging capabilities. Compared with using US-guided prostate biopsy surgery, the advantage of fusion image is that it has higher targeting ability, reduces the number of biopsies and avoids excessive biopsy and missed detection. The degree of automation and system functions of the robot designed by the researchers are different and the guidance process is generally summarized as follows: Before surgery, the patient undergoes an MRI scan and the urologist makes a biopsy plan based on the patient's preoperative MRI images. After completing the preoperative MRI image acquisition, the doctor cleans and disinfects the biopsy equipment and mounts the ultrasound probe on the robot. The ultrasound probe is inserted into the patient's rectum by a robot and the real-time US images collected during the operation are registered and fused with the preoperative MRI images so that the spatial positions of the two modal images are consistent and clear suspicious areas of the preoperative MRI images are fused into the real-time US images. In order to realize the automatic biopsy of the robot, it is necessary to register the coordinate system of the robot with the coordinate system of the fused image so that the two can be unified into the same coordinate space. Then, the doctor selects a biopsy position and the robot automatically sets the insertion direction and depth of the biopsy needle according to the biopsy position and automatically places the biopsy needle through the coordinated movement of the joints. After the setting is completed, the robot triggers the biopsy device to obtain the patient's pathological tissue. After completing the above steps, the robot repositions to the next biopsy position and repeats the above process for subsequent biopsy under the supervision of the doctor. However, image fusion requires additional software which is expensive, time-consuming and has the risk of fusion errors. Researchers need to develop fast and accurate fusion algorithms.
In 2020, Bi et al. [126] developed a prostate biopsy robot-assisted system, based on which they proposed an improved active demons non-rigid registration method named ADOP combining optic flow with active demons for preoperative MRI and intraoperative US prostate image fusion. The experimental results show that the root mean square error of ADOP and active demons are 3.15 mm and 3.48 mm respectively. In addition, the results of phantom experiments show that with the assistance of this system, the needle point positioning error of the robot is less than 2.5 mm which shows the feasibility of this system.
In 2022, Altini et al. [127] proposed a deformable hyperellipse formula for semi-automatic segmentation of prostate US images that requires only a small amount of data sets. Subsequently, the nnU-Net model was used to automatically segment patient MRI images. Finally, the team fused the segmentation results of US and MRI images using rigid registration. The experimental results show that the Hausdorff distance of all fusion data is less than 4 mm and the Dice coefficient is greater than 91%.
At present, with the development of clinical surgery and robotics and the increase of PCa cases worldwide. In order to improve the accuracy and reliability of biopsy operations and reduce the labor intensity of doctors, the research and development of prostate biopsy robots have attracted great attention from researchers. At present, most prostate biopsy robots are developed by hospitals and universities with different degrees of automation and functions and have not been applied to actual clinical biopsy. Image guidance is the key to determining the tumor positioning and planning the biopsy puncture path in the prostate biopsy robotic system. Therefore, this article reviews the development status of MRI, US and fusion image-guided prostate biopsy robots and discusses their advantages and limitations in detail. In the MRI-guided prostate biopsy robotic, due to the limitation of the strong magnetic field in the MRI environment, to ensure low image distortion and high signal-to-noise ratio, the drive and materials of the robot are the main concerns of researchers. Piezoelectric drive and pneumatic drive are the two main drivers methods and the materials are usually high-strength plastics and non-ferrous metals. In the US-guided prostate biopsy robot, there are no restrictions on the choice of drive and material and motors are usually used for the drive. Compared to other image-guided robotic, there is no need to worry about the stiffness of the device [128]. The accuracy of fusion is limited due to the difference in the position and shape of the prostate between the MRI image acquired before surgery and the image obtained by the intraoperative TRUS probe. Thus, fusion accuracy is the main problem in MRI-TRUS fusion image-guided prostate biopsy robotic systems.
To make the robot better meet the needs of urologists in the clinical biopsy, future researchers need to design a prostate biopsy robot that is more accurate, more compact and has higher automation, safety, reliability and autonomous decision-making capabilities. This paper provides the following 4 suggestions for the future development of prostate biopsy robots here:
Efficient and high-precision image fusion algorithm. Due to the complex calculation of image processing in prostate biopsy surgery, it is a challenge to achieve real-time image fusion. Therefore, a more efficient image fusion algorithm is proposed, combining new technologies such as machine learning, GPU and parallel computing to optimize the computational efficiency of image fusion. In addition, with the rapid development of deep learning technology, high-precision image fusion algorithms based on deep learning have become a current and future research hotspot. At present, the image-guided prostate biopsy robot has an urgent practical demand for real-time and high-precision image fusion algorithms to improve the robot's three-dimensional perception ability.
Intelligent interaction. The human-computer interaction in the existing prostate biopsy robot is not intuitive and it is difficult to fully display the relative pose relationship between the biopsy needle and the target organ. In the future, researchers need to conduct in-depth research on low-cost and fast information interaction between robots and other smart devices and design robot interaction methods according to doctors' operating habits during biopsy operations. For example, through mixed reality technology combined with voice control and gesture recognition technologies, virtual target information is superimposed on the position of real objects in 3D form and the intuitiveness of doctors' operations and surgical efficiency are improved through voice and gesture control.
Telemedicine. In the future, with the continuous updating of technologies such as communication technology, artificial intelligence, wearable medical devices, sensors and robots how to effectively integrate and improve various technologies to realize remote prostate biopsy across regions so that more people can obtain high-quality medical resources are the focus that researchers should pay attention to.
Robot intelligence. With the continuous updating of artificial intelligence and hardware technology, the design of future image-guided prostate biopsy robots should pay more attention to autonomy and intelligence and researchers should continue to strengthen the learning and independent decision-making capabilities of prostate biopsy robots. Establish a three-dimensional model of the patient's prostate through imaging, accurately locate suspicious areas in the prostate, define and visualize biopsy points, plan biopsy paths and make independent decisions based on intraoperative tissue movement and feedback from needle insertion to avoid important organs such as the pubic bone.
Through high-precision real-time imaging guidance, efficient intelligent interaction, mixed reality, remote control and robot intelligence and other technologies to efficiently integrate the system, it has become an inevitable trend to establish a robot-patient-environment multimodal fusion sensing prostate biopsy robot.
The authors declare they have not used Artificial Intelligence (AI) tools in the creation of this article.
This research was supported by the Reserve Leader Funding Project of Leading Talent Echelon of Heilongjiang Province of China (Grant No. 2501050628) and the National Natural Science Foundation of China (Grant No. 52275015) and the Science and Technology Innovation Team Project of Foshan City of China (Grant No. 2018IT100302).
The authors declare there is no conflict of interest.
[1] | M. Arioli, I. S. Du and P. P. M. de Rijk, On the augmented system approach to sparse leastsquares problems, Numer. Math., 55 (1989), 667–684. |
[2] | Z. Z. Bai, B. N. Parlett and Z. Q. Wang, On generalized successive overrelaxation methods for augmented linear systems, Numer. Math., 102 (2005), 1–38. |
[3] | Z. Z. Bai and Z. Q. Wang, On parameterized inexact Uzawa methods for generalized saddle point problems, Linear Algebra Appl., 428 (2008), 2900–2932. |
[4] | Z. Z. Bai and X. Yang, On HSS-based iteration methods for weakly nonlinear systems, Appl. Numer. Math., 59 (2009), 2923–2936. |
[5] | Z. Z. Bai, G. H. Golub and K. N. Michael, On inexact hermitian and skew-Hermitian splitting methods for non-Hermitian positive definite linear systems, Linear Algebra Appl., 284 (2008), 413–440. |
[6] | Z. Z. Bai, Several splittings for non-Hermitian linear systems, Science in China, Series A: Math., 51 (2008), 1339–1348. |
[7] | Z. Z. Bai, G. H. Golub, L. Z. Lu and J. F. Yin, Block-Triangular and skew-Hermitian splitting methods for positive definite linear systems, SIAM J. Sci. Comput., 26 (2005), 844–863. |
[8] | Z. Z. Bai, G. H. Golub and M. K.Ng, Hermitian and skew-Hermitian splitting methods for non- Hermitian positive definite linear systems, SIAM J. Matrix Anal. A., 24 (2003), 603–626. |
[9] | Z. Z. Bai, G. H. Golub and M. K. Ng, On successive-overrelaxation acceleration of the Hermitian and skew-Hermitian splitting iteration. Available from: http://www.sccm.stanford.edu/wrap/pubtech. html. |
[10] | Z. Z. Bai, G. H. Golub and C. K. Li, Optimal parameter in Hermitian and skew-Hermitian splitting method for certain twoby- two block matrices, SIAM J. Sci. Comput., 28 (2006), 28:583–603. |
[11] | Z. Z. Bai, G. H. Golub and M. K. Ng, On successive-overrelaxation acceleration of the Hermitian and skew-Hermitian splitting iterations, Numer. Linear Algebra Appl., 14 (2007), 319–335. |
[12] | Z. Z. Bai, G. H. Golub and J. Y. Pan, Preconditioned Hermitian and skew-Hermitian splitting methods for non-Hermitian positive semidefinite linear systems, Numer. Math., 98 (2004), 1–32. |
[13] | Z. Z. Bai and M. K. Ng, On inexact preconditioners for nonsymmetric matrices, SIAM J. Sci. Comput., 26 (2005), 1710–1724. |
[14] | Z. Z. Bai, M. K. Ng and Z. Q.Wang, Constraint preconditioners for symmetric indefinite matrices, SIAM J. Matrix Anal. Appl., 31 (2009), 410–433. |
[15] | Z. Z. Bai, Optimal parameters in the HSS-like methods for saddle-point problems, Numer. Linear Algebra Appl., 16 (2009), 447–479. |
[16] | Z. Z. Bai, J. F. Yin and Y. F. Su, A shift-splitting preconditioner for non-Hermitian positive definite matrices, J. Comput. Math., 24 (2006), 539–552. |
[17] | Z. Z. Bai, G. H. Golub and J. Y. Pan, Preconditioned Hermitian and skew-Hermitian splitting methods for non-Hermitian positive semidefinite linear systems. Technical Report SCCM-02-12, Scientific Computing and Computational Mathematics Program, Department of Computer Science, Stanford University, Stanford, CA, 2002. |
[18] | Z. Z. Bai, M. Benzi and F. Chen, Modified HSS iteration methods for a class of complex symmetric linear systems, Comput., 87 (2010), 93–111. |
[19] | Y. Cao, J. Du and Q. Niu, Shift-splitting preconditioners for saddle point problems, J. Comput. Appl. Math., 272 (2014), 239–250. |
[20] | Y. Cao, L. Q. Yao and M. Q. Jiang, A modified dimensional split preconditioner for generalized saddle point problems, J. Comput. Appl. Math., 250 (2013), 70–82. |
[21] | Y. Cao, L. Q. Yao, M. Q. Jiang and Q. Niu, A relaxed HSS preconditioner for saddle point problems from meshfree discretization, J. Comput. Math., 31 (2013), 398–421. |
[22] | C. R. Chen and C. F. Ma, A generalized shift-splitting preconditioner for saddle point problems, Appl. Math. Lett., 43 (2015), 49–55. |
[23] | F. Chen and Y. L. Jiang, A generalization of the inexact parameterized Uzawa methods for saddle point problems, Appl. Math. Comput., 206 (2008), 765–771. |
[24] | L. B. Cui, C. Chen, W. Li and M.K. Ng, An eigenvalue problem for even order tensors with its applications, Linear Multilinear Algebra, 64 (2016), 602–621. |
[25] | L. B. Cui, W. Li and M. K. Ng, Primitive tensors and directed hypergraphs, Linear Algebra Appl., 471 (2015), 96–108. |
[26] | L. B. Cui, C. X. Li and S. L. Wu, The relaxation convergence of multisplitting AOR method for linear complementarity problem, Linear Multilinear Algebra, DOI: 10.1080/03081087.2018.1511680. |
[27] | L. B. Cui and Y. S. Song, On the uniqueness of the positive Z-eigenvector for nonnegative tensors, J. Comput. Appl. Math., 352 (2019), 72C78. |
[28] | M. T. Darvishi and P. Hessari, Symmetric SOR method for augmented systems, Appl. Math. Comput., 183 (2006), 409–415. |
[29] | H. Elman and D. Silvester, Fast nonsymmetric iterations and preconditioning for Navier-Stokes equations, SIAM J. Sci. Comput., 17 (1996), 33–46. |
[30] | H. Elman and G. H. Golub, Inexact and preconditioned Uzawa algorithms for saddle point problems, SIAM J. Numer. Anal., 31 (1994), 1645–1661. |
[31] | B. Fischer, A. Ramage, D. J. Silvester and A. J.Wathen, Minimum residual methods for augmented systems, BIT, 38 (1998), 527–543. |
[32] | G. H. Golub, X. Wu and J. Y. Yuan, SOR-like methods for augmented systems, BIT, 55 (2001), 71–85. |
[33] | M. Q. Jiang and Y. Cao, On local Hermitian skew-Hermitian splitting iteration methods for generalized saddle point problems, J. Comput. Appl. Math., 231 (2009), 973–982. |
[34] | X.Y. Li, L. Gao, Q. K. Pan, L. Wan and K. M. Chao, An e ective hybrid genetic algorithm and variable neighborhood search for integrated process planning and scheduling in a packaging machine workshop, IEEE Transactions on Systems, Man and Cybernetics: Systems, 2018, DOI 10.1109/TSMC.2018.2881686. |
[35] | X. Y. Li, C. Lu, L. Gao, S. Q. Xiao and L.Wen, An E ective Multi-Objective Algorithm for Energy Efficient Scheduling in a Real-Life Welding Shop, IEEE T. Ind. Inform., 14 (2018), 5400–5409. |
[36] | X. Y. Li and L. Gao, An E ective Hybrid Genetic Algorithm and Tabu Search for Flexible Job Shop Scheduling Problem, Int. J. Prod. Econ., 174 (2016), 93–110. |
[37] | X. F. Peng and W. Li, On unsymmetric block overrelaxation-type methods for saddle point, Appl. Math. Comput., 203 (2008), 660–671. |
[38] | C. H. Santos, B. P. B. Silva and J. Y. Yuan, Block SOR methods for rank deficient least squares problems, J. Comput. Appl. Math., 100 (1998), 1–9. |
[39] | H. A. Van der Vorst, Iterative Krylov Methods for Large Linear Systems, Cambridge Monographs on Applied and Computational Mathematics, Cambridge University Press, Cambridge, UK, 2003. |
[40] | L. Wang and Z. Z. Bai, Convergence conditions for splitting iteration methods for non-Hermitian linear systems, Linear Algebra Appl., 428 (2008), 453–468. |
[41] | S. Wright, Stability of augmented system factorizations in interior-point methods, SIAM J. Matrix Anal. Appl., 18 (1997), 191–222. |
[42] | S. L.Wu, T. Z. Huang and X. L. Zhao, A modified SSOR iterative method for augmented systems, J. Comput. Appl. Math., 228 (2009), 424–433. |
[43] | D. M. Young, Iteratin Solution for Large Systems, Academic Press, New York, 1971. |
[44] | J. Y. Yuan, Numerical methods for generalized least squares problems, J. Comput. Appl. Math., 66 (1996), 571–584. |
[45] | J. Y. Yuan and A. N. Iusem, Preconditioned conjugate gradient method for generalized least squares problems, J. Comput. Appl. Math., 71 (1996), 287–297. |
[46] | G. F. Zhang and Q. H. Lu, On generalized symmetric SOR method for augmented systems, J. Comput. Appl. Math., 1 (2008), 51–58. |
[47] | L. T. Zhang, A new preconditioner for generalized saddle matrices with highly singular(1,1) blocks, Int. J. Comput. Math., 91 (2014), 2091–2101. |
[48] | L. T. Zhang, T. Z. Huang, S. H. Cheng and Y. P. Wang, Convergence of a generalized MSSOR method for augmented systems, J. Comput. Appl. Math., 236 (2012), 1841–1850. |
[49] | B. Zheng, Z. Z. Bai and X. Yang, On semi-convergence of parameterized Uzawa methods for singular saddle point problems, Linear Algebra Appl., 431 (2009), 808–817. |
[50] | Y. Z. Zhou, W. C. Yi, L. Gao and X. Y. Li, Adaptive di erential evolution with sorting crossover rate for continuous optimization problems, IEEE T. Cybernetics, 47 (2017), 2742–2753. |
1. | Nihat Türkmen, Taner Hacıosmanoğlu, What may be Related to Patient Satisfaction in Prostate Biopsies?, 2024, 16, 2148-0532, 50, 10.54233/endourolbull-1423624 | |
2. | Weirong Wang, Bo Pan, Yue Ai, Gonghui Li, Yili Fu, Yanjie Liu, LightCM-PNet: A lightweight pyramid network for real-time prostate segmentation in transrectal ultrasound, 2024, 156, 00313203, 110776, 10.1016/j.patcog.2024.110776 | |
3. | Yibo Wang, Zhichao Ye, Mingwei Wen, Huageng Liang, Xuming Zhang, TransVFS: A spatio-temporal local–global transformer for vision-based force sensing during ultrasound-guided prostate biopsy, 2024, 94, 13618415, 103130, 10.1016/j.media.2024.103130 |
Year | FA | Institution | IM | PS | NTPA | Actuation | DOF | Status | Reference |
2012 | Seifabadi | Queen's University | MRI | Perineum | 6 | Virtual prototyping design | [31] | ||
2010 | Krieger | Johns Hopkins University | MRI | Rectum | 2.4 mm | Piezoelectric actuators | 3 | Phantom test | [32] |
2019 | Patel | Worcester Polytechnic Institute | MRI | Perineum | 3.7 mm | Piezoelectric actuators | 6 | Phantom test | [34] |
2005 | Fichtinger | Johns Hopkins University | MRI | Rectum | 1.8 mm | 3 | Clinical patient test | [35] | |
2011 | Krieger | Johns Hopkins University | MRI | Rectum | 1.1 mm | 6 | Clinical patient test | [38] | |
2013 | Krieger | Johns Hopkins University | MRI | Rectum | 2.4 mm | Piezoelectric actuators | 8 | Phantom test | [39] |
2012 | Bohren | Johns Hopkins University | MRI | Rectum | Piezoelectric actuators | 9 | Virtual prototyping design | [40] | |
2007 | Elhawary | University of Navarra | MRI | Rectum | 2.3 mm | Piezoelectric actuators | 5 | Phantom test | [41,42,43] |
2008 | Goldenberg | University of Toronto | MRI | Perineum | < 2 mm | Piezoelectric actuators | 6 | Phantom test | [44,45] |
2008 | Fischer | Johns Hopkins University | MRI | Perineum | 0.94 mm | Pneumatic actuators | 4 | Phantom test | [46,47,48] |
2010 | Schouten | Radboud University | MRI | Rectum | 3.0 mm | Pneumatic actuators | 5 | Phantom test | [49,50,51] |
2010 | Song | Johns Hopkins University | MRI | Perineum | < 0.5 mm | Pneumatic actuators | 4 | Phantom test | [52] |
2016 | Seifabadi | Queen's University | MRI | Perineum | 0.9 mm | Pneumatic actuators | 6 | Phantom test | [55,56] |
2012 | Su | Worcester Polytechnic Institute | MRI | Perineum | 1 mm | Piezoelectric actuators | 6 | Phantom test | [57,58,59] |
2013 | Eslami | Johns Hopkins University |
MRI | Perineum | 4 | Virtual prototyping design | [60] | ||
2015 | Li | Johns Hopkins University |
MRI | Perineum | 0.92 mm | Piezoelectric actuators | 7 | Phantom test | [62] |
2014 | Stoianovici | Johns Hopkins University | MRI | Rectum | < 2.58 mm | Pneumatic actuators | 3 | Live animal tests | [64] |
2018 | Stoianovici | Johns Hopkins University | MRI | Rectum | 0.645 mm | Pneumatic actuators | 3 | Phantom test | [65] |
2015 | Chen | Fraunhofer MEVIS Institute | MRI | Rectum | Pneumatic actuators | 5 | Virtual prototyping design | [66] | |
2016 | Stoianovici | Johns Hopkins University | MRI | Perineum | 2.55 mm | Pneumatic actuators | 6 | Clinical patient test | [67] |
2022 | Aleong | University of Toronto | MRI | Perineum | 5 mm | Piezoelectric actuators | 6 | Phantom test | [69] |
2022 | Biswas | University of Central Florida | MRI | Perineum | motors | 4 | Virtual prototyping design | [70] | |
2005 | Phee | Nanyang Technological University | US | Perineum | < 2.5 mm | 9 | Clinical patient test | [88] | |
2009 | Ho | Singapore General Hospital |
US | Perineum | < 1 mm | motors | 6 | Phantom test | [89,90] |
2012 | Zhang | Harbin University of Science and Technology | US | 5 | Virtual prototyping design | [91] | |||
2012 | Long | Grenoble University Hospital | US | Perineum | 2.73 mm | motors | 7 | Phantom test | [92] |
2013 | Poquet | University of Pittsburgh Medical Center | US | Rectum | 3.1 mm | motors | 6 | Clinical patient test | [93] |
2019 | Lim | Johns Hopkins University |
US | Rectum | 1 mm | motors | 4 | Clinical patient test | [97] |
2022 | Yan | Harbin Institute of Technology | US | Rectum | 1.594 mm | motors | 8 | Phantom test | [98] |
2017 | Pisla | Technical University of Cluj-Napoca | MRI-US | Perineum | 1–2 mm | motors | 10 | Phantom test | [101] |
2021 | Maris | University of Verona | MRI-US | Perineum | 1.30 ± 0.44 mm | motors | 5 | Phantom test | [104] |
2021 | Wang | Harbin Institute of Technology | MRI-US | Perineum | 1.44 mm | motors | 5 | Phantom test | [105] |
2021 | Xiao | Southern University of Science and Technology | MRI-US | Urethra | 1.2 mm | motors | 3 | Phantom test | [106,107] |
Note: Abbreviations: FA, First author; IM, Imaging modality; PS, Puncture site; NTPA, Needle tip positioning accuracy; DOF, Degree of freedom. |
Year | FA | Institution | IM | PS | NTPA | Actuation | DOF | Status | Reference |
2012 | Seifabadi | Queen's University | MRI | Perineum | 6 | Virtual prototyping design | [31] | ||
2010 | Krieger | Johns Hopkins University | MRI | Rectum | 2.4 mm | Piezoelectric actuators | 3 | Phantom test | [32] |
2019 | Patel | Worcester Polytechnic Institute | MRI | Perineum | 3.7 mm | Piezoelectric actuators | 6 | Phantom test | [34] |
2005 | Fichtinger | Johns Hopkins University | MRI | Rectum | 1.8 mm | 3 | Clinical patient test | [35] | |
2011 | Krieger | Johns Hopkins University | MRI | Rectum | 1.1 mm | 6 | Clinical patient test | [38] | |
2013 | Krieger | Johns Hopkins University | MRI | Rectum | 2.4 mm | Piezoelectric actuators | 8 | Phantom test | [39] |
2012 | Bohren | Johns Hopkins University | MRI | Rectum | Piezoelectric actuators | 9 | Virtual prototyping design | [40] | |
2007 | Elhawary | University of Navarra | MRI | Rectum | 2.3 mm | Piezoelectric actuators | 5 | Phantom test | [41,42,43] |
2008 | Goldenberg | University of Toronto | MRI | Perineum | < 2 mm | Piezoelectric actuators | 6 | Phantom test | [44,45] |
2008 | Fischer | Johns Hopkins University | MRI | Perineum | 0.94 mm | Pneumatic actuators | 4 | Phantom test | [46,47,48] |
2010 | Schouten | Radboud University | MRI | Rectum | 3.0 mm | Pneumatic actuators | 5 | Phantom test | [49,50,51] |
2010 | Song | Johns Hopkins University | MRI | Perineum | < 0.5 mm | Pneumatic actuators | 4 | Phantom test | [52] |
2016 | Seifabadi | Queen's University | MRI | Perineum | 0.9 mm | Pneumatic actuators | 6 | Phantom test | [55,56] |
2012 | Su | Worcester Polytechnic Institute | MRI | Perineum | 1 mm | Piezoelectric actuators | 6 | Phantom test | [57,58,59] |
2013 | Eslami | Johns Hopkins University |
MRI | Perineum | 4 | Virtual prototyping design | [60] | ||
2015 | Li | Johns Hopkins University |
MRI | Perineum | 0.92 mm | Piezoelectric actuators | 7 | Phantom test | [62] |
2014 | Stoianovici | Johns Hopkins University | MRI | Rectum | < 2.58 mm | Pneumatic actuators | 3 | Live animal tests | [64] |
2018 | Stoianovici | Johns Hopkins University | MRI | Rectum | 0.645 mm | Pneumatic actuators | 3 | Phantom test | [65] |
2015 | Chen | Fraunhofer MEVIS Institute | MRI | Rectum | Pneumatic actuators | 5 | Virtual prototyping design | [66] | |
2016 | Stoianovici | Johns Hopkins University | MRI | Perineum | 2.55 mm | Pneumatic actuators | 6 | Clinical patient test | [67] |
2022 | Aleong | University of Toronto | MRI | Perineum | 5 mm | Piezoelectric actuators | 6 | Phantom test | [69] |
2022 | Biswas | University of Central Florida | MRI | Perineum | motors | 4 | Virtual prototyping design | [70] | |
2005 | Phee | Nanyang Technological University | US | Perineum | < 2.5 mm | 9 | Clinical patient test | [88] | |
2009 | Ho | Singapore General Hospital |
US | Perineum | < 1 mm | motors | 6 | Phantom test | [89,90] |
2012 | Zhang | Harbin University of Science and Technology | US | 5 | Virtual prototyping design | [91] | |||
2012 | Long | Grenoble University Hospital | US | Perineum | 2.73 mm | motors | 7 | Phantom test | [92] |
2013 | Poquet | University of Pittsburgh Medical Center | US | Rectum | 3.1 mm | motors | 6 | Clinical patient test | [93] |
2019 | Lim | Johns Hopkins University |
US | Rectum | 1 mm | motors | 4 | Clinical patient test | [97] |
2022 | Yan | Harbin Institute of Technology | US | Rectum | 1.594 mm | motors | 8 | Phantom test | [98] |
2017 | Pisla | Technical University of Cluj-Napoca | MRI-US | Perineum | 1–2 mm | motors | 10 | Phantom test | [101] |
2021 | Maris | University of Verona | MRI-US | Perineum | 1.30 ± 0.44 mm | motors | 5 | Phantom test | [104] |
2021 | Wang | Harbin Institute of Technology | MRI-US | Perineum | 1.44 mm | motors | 5 | Phantom test | [105] |
2021 | Xiao | Southern University of Science and Technology | MRI-US | Urethra | 1.2 mm | motors | 3 | Phantom test | [106,107] |
Note: Abbreviations: FA, First author; IM, Imaging modality; PS, Puncture site; NTPA, Needle tip positioning accuracy; DOF, Degree of freedom. |