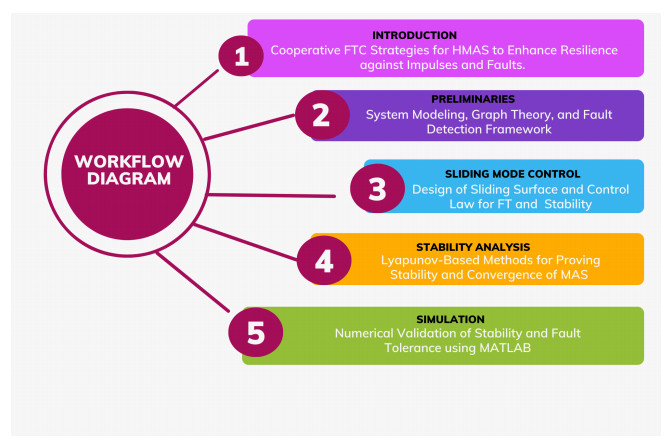
This study dealt with the problem of fault-tolerant control in multi-agent systems impacted also by factor stochastic impulsive disturbances together with actuator and sensor malfunctions. It is known that impulsive events can cause the agents to become unsynchronized and controlled coordination using given fault-tolerant methods is difficult. To enhance the robustness and safety of fault models, this research presented an impulsive fault-tolerant control. This controller aims to eliminate the effect of actuator faults, sensor faults, and impulsive disturbances. We also provided an overview of the sliding mode control technique, which is necessary for managing nonlinear uncertainties in impulses and faults in actuators and sensors. To describe the characteristics of the sliding mode controller, the Lyapunov function was employed to analyze its stability. The stability effect demonstrated in our paper is asymptotic stability rather than semi-global uniform ultimate boundedness because the function derivative of the Lyapunov function and its included term ensures it is negative semi-definite except for the bounded switching control terms, confirming asymptotic convergence to zero. Numerical examples were included to demonstrate the effectiveness and benefits of the proposed approach.
Citation: Meraa Arab, Aasma Zaman, Azmat Ullah Khan Niazi, Marwa Balti. Impulsive fault-tolerant control for multi-agent systems with stochastic disturbances[J]. AIMS Mathematics, 2025, 10(3): 7414-7429. doi: 10.3934/math.2025340
[1] | Zuo Wang, Hong Xue, Yingnan Pan, Hongjing Liang . Adaptive neural networks event-triggered fault-tolerant consensus control for a class of nonlinear multi-agent systems. AIMS Mathematics, 2020, 5(3): 2780-2800. doi: 10.3934/math.2020179 |
[2] | Qiangqiang Zhang, Yiyan Han, Chuandong Li, Le You . Constraint impulsive consensus of nonlinear multi-agent systems with impulsive time windows. AIMS Mathematics, 2020, 5(4): 3682-3701. doi: 10.3934/math.2020238 |
[3] | Xing Zhang, Lei Liu, Yan-Jun Liu . Adaptive NN control based on Butterworth low-pass filter for quarter active suspension systems with actuator failure. AIMS Mathematics, 2021, 6(1): 754-771. doi: 10.3934/math.2021046 |
[4] | Le You, Chuandong Li, Xiaoyu Zhang, Zhilong He . Edge event-triggered control and state-constraint impulsive consensus for nonlinear multi-agent systems. AIMS Mathematics, 2020, 5(5): 4151-4167. doi: 10.3934/math.2020266 |
[5] | Hongjie Li . H-infinity bipartite consensus of multi-agent systems with external disturbance and probabilistic actuator faults in signed networks. AIMS Mathematics, 2022, 7(2): 2019-2043. doi: 10.3934/math.2022116 |
[6] | Chunli You, Linxin Shu, Xiao-bao Shu . Approximate controllability of second-order neutral stochastic differential evolution systems with random impulsive effect and state-dependent delay. AIMS Mathematics, 2024, 9(10): 28906-28930. doi: 10.3934/math.20241403 |
[7] | Mohamed Kharrat, Hadil Alhazmi . Neural networks-based adaptive fault-tolerant control for a class of nonstrict-feedback nonlinear systems with actuator faults and input delay. AIMS Mathematics, 2024, 9(6): 13689-13711. doi: 10.3934/math.2024668 |
[8] | Qiushi Wang, Hongwei Ren, Zhiping Peng . Event-triggered impulsive control for second-order nonlinear multi-agent systems under DoS attacks. AIMS Mathematics, 2024, 9(6): 13998-14011. doi: 10.3934/math.2024680 |
[9] | Xiaogang Liu, Muhammad Ahsan, Zohaib Zahid, Shuili Ren . Fault-tolerant edge metric dimension of certain families of graphs. AIMS Mathematics, 2021, 6(2): 1140-1152. doi: 10.3934/math.2021069 |
[10] | Snezhana Hristova, Kremena Stefanova, Angel Golev . Dynamic modeling of discrete leader-following consensus with impulses. AIMS Mathematics, 2019, 4(5): 1386-1402. doi: 10.3934/math.2019.5.1386 |
This study dealt with the problem of fault-tolerant control in multi-agent systems impacted also by factor stochastic impulsive disturbances together with actuator and sensor malfunctions. It is known that impulsive events can cause the agents to become unsynchronized and controlled coordination using given fault-tolerant methods is difficult. To enhance the robustness and safety of fault models, this research presented an impulsive fault-tolerant control. This controller aims to eliminate the effect of actuator faults, sensor faults, and impulsive disturbances. We also provided an overview of the sliding mode control technique, which is necessary for managing nonlinear uncertainties in impulses and faults in actuators and sensors. To describe the characteristics of the sliding mode controller, the Lyapunov function was employed to analyze its stability. The stability effect demonstrated in our paper is asymptotic stability rather than semi-global uniform ultimate boundedness because the function derivative of the Lyapunov function and its included term ensures it is negative semi-definite except for the bounded switching control terms, confirming asymptotic convergence to zero. Numerical examples were included to demonstrate the effectiveness and benefits of the proposed approach.
Multi-agents have a unique structure and capacity to collaborate to focus on coordination for different tasks. Multi-agent control theory has advanced with vast research works. In recent decades, control theory has had a lot of applications in various fields like robotics, engineering, space research, etc. [1] and has discussed the different techniques for fault-tolerant control. In multi-agent control theory, many agents collaborate to perform a task and become the foundation of every industry. As the agents contain many system components, their quality and reliability demand has been increasing. That is why a fault-tolerant control (FTC) strategy has been developed to improve the reliability of multi-agent systems. The evolution of fault-tolerant control is significant because it refines the stability and reliability of the system. There are two types of fault-tolerant control systems: passive and active. Passive fault control does not adopt the various types of faults while active fault tolerant control involves the detection and isolation of faults. Recent research work focused on developing control laws based on real-time data. Currently, research on FTC is to improve fault detection accuracy and retaliation on time for systems like independent vehicle systems. For instance, metaheuristic optimization algorithms based on swarm intelligence have demonstrated improved unmanned aerial vehicle (UAV) control performance [2,3]. Observer designs for systems with infinite delays have shown effective disturbance mitigation [4]. The Padé-approximation-based optimal preview repetitive control method has also proven effective in enhancing system stability [5]. Intelligent fault detection methods for ground vehicles and non-intrusive appliance load monitoring approaches have enhanced reliability and identification accuracy [6,7]. Moreover, advanced machine learning techniques have improved classification accuracy under imbalanced conditions [8].
Vehicle systems include UAVs and unmanned ground vehicles (UGVs). Over the past few years, there has been heightened interest in heterogeneous multi-agent systems (HMASs) which leverage the unique capabilities of both UAVs and UGVs. Robots like drones can perform the multiple tasks in worst-case scenarios to mitigate the catastrophic effects. These vehicles are capable of bringing important towing capacity and executing commands with precision. The utility of these UAVs assists in military and civilian fields including agriculture, disasters, and environmental monitoring. To enhance the resilience of HMASs, various fault-tolerant control schemes including passive, active, and hybrid approaches have been developed. Furthermore, finite-time stability theories have been introduced to accelerate the convergence rates of these systems, although their effectiveness is often contingent upon the initial conditions of the system. Adaptive control strategies in dynamic systems [9] and robust event-triggered mechanisms further contribute to improved stability and resilience in multi-agent networks [10,11].
The system will be reliable when its sensor and actuator are functioning properly. Faults in these components can cause system instability leading to failures. Impulses can represent sudden, unpredictable events, such as communication packet drops and abrupt environmental changes. The concept of scholastic impulses introduces a vital layer of dynamism and adaptability to HMASs by enabling sudden yet calculated adjustments in system states. These impulses serve as deliberate interventions designed to redirect or stabilize agents when conventional continuous-control strategies encounter limitations. Mechanical system performance degradation models were discussed in [12]. In the context of tracking, scholastic impulses can optimize formation adjustments for UAVs and UGVs, allowing the system to respond promptly to unpredictable changes in fire behavior. By temporarily overriding standard control dynamics, impulses facilitate swift recovery from actuator or sensor faults, thereby minimizing the risk of extensive system disruptions. This approach is particularly beneficial in the case of HMASs which have to work in uncertain environments where external disturbances and faults in the system are inevitable. The inclusion of advanced methods, like higher- order sliding mode observation and fractional-order non-singular fast terminal sliding mode control, and stochastic impulses allow UAV and UGV systems to preserve formation and perform tasks even in difficult situations. Adaptive control for UAV path tracking was explored in [13]. Deep reinforcement learning for UAV navigation was introduced in [14], while an adaptive event-triggered control for heavy trucks was proposed in [15]. Finally, the concepts of fault tolerance allow the design of the HMAS to be more robust and adaptive, allowing the system to be fault tolerant when deployed in real-life missions with a high degree of accuracy and efficiency.
Effective trajectory controllability of a MAS involves making sure that the predefined paths are tracked regardless of the stochastic impulses, actuator/sensor faults, or uncertainty that the surrounding environment poses. An observer-based control strategy for interconnected systems was presented in [16]. Secure consensus algorithms under sensor and actuator attacks were highlighted in [17]. The achievement of trajectory controllability in multi-agent systems is particularly difficult because of the existence of system uncertainties, actuator or sensor faults, and impulsive disturbances. A decentralized framework for multi-agent systems was developed in [18] and event-triggered control to minimize communication costs were applied in [19]. A lot of research work has been invested in making trajectory control robust in its application to autonomous vehicles, coordinated UAV swarms, and robotics, where movement and coordination precision matter most. Recently, some advancements have been made regarding the usage of fault tolerance, combining it with the adaptive control methods and the sliding mode control to accomplish better trajectory tracking. A distributed anomaly detection framework using federated learning was introduced in [20] while Particle Swarm Optimization (PSO) with the Long Short-Term Memory (LSTM) network for Quadrotor unmanned aerial vehicles (QUAVs) control was integrated in [21]. Resilient state estimation strategies are investigated in [22], and a hybrid consensus protocol enhancing system robustness was proposed in [23]. Recent developments in this area have demonstrated its importance in a UAV-UGV coordination application where heterogeneous agents need to robustly adapt to dynamic constraints while being able to follow predefined trajectories. In the context of UAV swarms, applied stochastic impulse removal techniques to achieve improved controllability of the trajectory formation for maintenance of stringent formation repositioning constraints under packet losses and sensor outages. All these studies depict the issues and challenges regarding the ability to maintain trajectory controllability of HMASs for faulty and impulsive disturbance-affected environments as well as without unduly compromising the consensus dynamics. Recent studies related to this paper include the investigation of fixed and prescribed stability criteria for stochastic systems with time-delay [24], the development of a hybrid event-triggering control approach for prescribed-time synchronization in complex dynamic networks of piecewise smooth systems [25], and the design of a memory-based quantized dynamic event-triggered control strategy for practical finite-time synchronization of Lur'e systems with performance constraints and actuator faults [26]. Additionally, relevant works explore active device detection and performance analysis in massive non-orthogonal transmissions for internet of things (IoT) networks [27], uplink and downlink communication design for federated learning [28], distributed machine learning for multiuser mobile edge computing systems [29], and federated unfolding learning for channel state information (CSI) feedback in distributed edge networks [30].
In this study, to estimate dispersal within the system actually, an advanced fractional-order non-singular terminal sliding mode control strategy was used. These techniques are effective for dealing with most uncertainties and external disturbances. This method has been devised to enhance speed while ensuring that the desired region is stable irrespective of the changes in operating conditions. This work explains exhaustively the dynamics that control the UAV maneuvers. An integrated multi-body dynamic model is established that solves several problems such as the presence of sensor errors that degrade information quality, actuator failures that hamper control systems, impulsive systems, and several systems that may be external to the system under consideration. To counter these problems, a more sophisticated higher-order sliding mode observer is proposed which helps to determine the state of the system in the presence of uncertainties and defects. Then a non-singular fast terminal sliding mode surface is designed, along with an interconnected control strategy desired to effectively stabilize the UAV demeanor. To show the stability of the sliding surface, extensive numerical simulations are conducted. The framework of this paper is represented in Figure 1.
This paper presents a novel approach for impulsive fault-tolerant control (FTC) in multi-agent systems (MAS) subjected to stochastic disturbances, actuator malfunctions, and sensor faults. The key contributions of this study are summarized as follows:
● The control framework is extended to handle stochastic impulsive disturbances, making it more adaptable for practical applications. Unlike conventional FTC methods that primarily focus on deterministic faults, our study explicitly incorporates stochastic impulses, ensuring robust performance under abrupt disturbances.
● A high-order sliding mode controller is designed to enhance trajectory tracking precision and fault compensation efficiency. Fractional order non-singular fast terminal sliding mode control (FO-NFTSMC) as compares to conventional sliding mode control (SMC) achieves faster error convergence and reduces chattering effects, which are common issues in classical SMC.
● A sliding mode-based control method is introduced to address impulsive disturbances and faults in actuators and sensors. This distributed control strategy with centralized methods, showcases its advantages in scalability, resilience, and real-time fault adaptation in multi-agent systems.
● A rigorous mathematical analysis is performed to prove the asymptotic stability of the system. Our stability analysis, demonstrating a Lyapunov-based approach accounts for impulsive effects and stochastic uncertainties, unlike many existing models that primarily handle deterministic faults.
● Simulation studies are conducted to compare system performance under fault conditions, demonstrating the robustness of the proposed method.
A mathematical foundation for examining interactions in systems with multiple agents (HMASs) is provided by graph theory. An HMAS can be represented as a directed graph with the form G=(V,E), in which the set of nodes representing agents, like UAVs, is represented by V={V1,V2,…,VN}, and the set of pointed edges representing communication linkages between the agents is represented by E⊆V.
An edge (Vi,Vj) exists if agent Vj receives information from agent vi. Each edge may have a weight ωij if (Vi,Vj)∈E, which indicates the strength of interaction or the reliability of communication between agents Vi and Vj.The adjacency matrix A∈RN×N of the graph G describes the structure of the communication network. The diagonal matrix D (degree matrix) represents the sum of outgoing edge weights for each node:
D=diag∑Aij. |
The Laplacian matrix L is defined as:
Lij=D−Aij,if i=j. |
The Laplacian matrix is essential for analyzing the stability of a system. Particularly, the eigenvalues of the Laplacian matrix determine whether agents in a network can reach consensus. In cooperative control systems, the Laplacian matrix aids in analyzing the convergence of agent dynamics. A positive semi-definite Laplacian ensures stability.
The formation control problem within a multi-agent system leverages non-linear system dynamics to effectively address the diverse characteristics and varying dimensions of each unmanned aerial vehicle (UAV). This modeling approach is intentionally designed to capture and accommodate the unique attributes present in the system, ensuring that each UAV can operate seamlessly within the group. Additionally, a detailed description of the models related to actuators, sensors, and stochastic impulse faults is critical for understanding the system's behavior and robustness under various conditions. Stochastic impulse control problems represent a class of continuous-time optimization challenges. In these scenarios, a stochastic system—one that evolves unpredictably over time—is manipulated by a limited number of impulses. Each impulse results in a sudden and discontinuous shift in the state of the system. The UAV dynamical model with stochastic impulses and other faults can be written as:
dxi(t)=vi(t)d(t), | (3.1) |
dvi(t)=σi(t)[fi(xj−xi,t)+ϵi(t)ui(t)+ζi+δi(t)]d(t)+γiN∑j=1aij(xj(t)−xi(t))d(t)+Biσwdw(t), | (3.2) |
where σw is the impulse intensity, vi(t)=σww(t) is the standard Wiener process (stochastic noise), ϵi(t)ui(t) is the actuator fault, σi(t) is the sensor fault, fi(xj−xi,t) refers to the system disturbances that arise from both internal and external factors affecting the ith agent, ζi denotes the system perturbation, aj(i) represents the communication topology between agents i, Bi represents the impulse effect matrix of the system, j, γi is the formation control gain, and w(t) is the stochastic impulse process.
The sliding surface serves as a mathematical model that illustrates how the system is expected to behave under various conditions. It defines the desired trajectory and performance characteristics that the system should follow to achieve its objectives. Sliding mode control objectives include maintaining formation despite actuator or sensor faults and stochastic impulses, as well as ensuring the convergence of formation errors Eij,k to zero.
The position error tracking in the course of the formation of agents is given as:
εij,k=εx,ij−εx,jp, | (3.3) |
where εij,k is the formation error between agents j and k where εij,k=xj−xi, xi and xj are the positions of agents i and j, and εx,jp is the desired relative position.
Now the sliding surface is given as
si(t)=λiεij,k+dxi(t)−vid(t). | (3.4) |
The term λi represents the positive observer gain, dxi(t) denotes the velocity of the ith agent, and vid(t) is the desired velocity. In the case of two predictive models, sufficient conditions require λ<0 to ensure convergence of the formation errors. In control theory, equivalent control is the command that must be injected to keep the system on the enforced sliding surface. This control is important in dynamically adjusting systems to maintain a set course where they are expected to perform at a specified point in time. That is, si(t)=0 is maintained through real-time adjustments made through this feedback control system allowing it to be invariant.
Differentiating (3.4), we have
˙si(t)=λi˙εi(t)+d˙xi(t)−˙vid(t). | (3.5) |
Now substituting system dynamics (3.1), (3.2) in the above equation gives us
˙si(t)=λi[vi(t)−vid(t)]+σi(t)[fi(xj−xi,t)+ϵi(t)ui(t)+ζi+δi(t)]d(t)+γiN∑j=1aij(xj(t)−xi(t))d(t)+Biσwdw(t). | (3.6) |
The equivalent control ueqi(t) that maintains the system on the sliding surface, i.e., ˙si(t)=0,
ueqi(t)=λi[vid(t)−vi(t)]−σi(t)−1[fi(xj−xi,t)+ϵi(t)ui(t)+ζi+δi(t)]d(t)−γiN∑j=1aij(xj(t)−xi(t))d(t)−Biσwdw(t). | (3.7) |
A switching control term to handle uncertainties and faults is
uswi(t)=−ρisgn(si(t)), | (3.8) |
where ρi is the positive gain Combining the equivalent and switching control and total control input, we have
ui(t)=ueqi(t)+uswi(t). | (3.9) |
Using Eq (3.9) in Eq (3.6) for the control input gives us
˙si(t)=λi[vi(t)−vid(t)]+σi(t)ϵi(t)[ueqi(t)+uswi(t)]+σi(t)[fi(xj−xi,t)+ζi+δi(t)]d(t)+γiN∑j=1aij(xj(t)−xi(t))d(t)+Biσwdw(t). | (3.10) |
Lyapunov theory is a practical technique for verifying the stability and convergence of dynamical systems. The stability of the closed-loop system can be effectively evaluated by developing a suitable Lyapunov function. This function serves as a useful tool for analyzing how the system behaves over time and helps us determine if it will remain stable when faced with perturbations or disturbances.
V(t)=12N∑i=1s2i(t). |
The rate of change of a Lyapunov function is given as:
˙V(t)=N∑i=1si(t)˙si(t). |
Using Eq (3.10) in the above Lyapunov function, we have
˙V(t)=N∑i=1si(t)[λi(vi(t)−vid(t))+σi(t)ϵi(t)(ueqi(t)+uswi(t))]+σi(t)[fi(xj−xi,t)+ζi+δi(t)]d(t)+γiN∑j=1aij(xj(t)−xi(t))d(t)+Biσwdw(t), |
w(t)≤N∑i=1|si(t)|[λi|vi(t)−vid(t)|+|σi(t)ϵi(t)|(|ueqi(t)|+|uswi(t)|)]. |
To prove the stability of the Lyapunov function, the bounds on σi(t), ϵi(t), and ρi are used where |vi(t)−vid(t)|≤|ei(t)| in the formation error, |ueqi(t)|≤βi in the equivalent control bound, |uswi(t)|≤ρi in the switching control, and |σi(t)ϵi(t)|≤σmaxϵmax, and the final result will be:
dw(t)≤−ηV(t)+N∑i=1ρi|si(t)|, |
where η=minλi−σmaxϵmaxβi−ρi is a positive constant so that Lyapunov function V(t) is bounded and si(t) converges to zero as t→∞.
The bound shows that the derivative of the Lyapunov function is negative definite, except for the term ∑Ni=1ρi|si(t)|, which represents the effect of the switching control. The system is asymptotically stable, and the sliding surface si(t) converges to zero as t→∞.
Assumption 3.1. The spanning tree in the directed graph can be enhanced by designating a leader to serve as the root node, providing clear direction for all agents involved.
Assumption 3.2. The efficiency factor ϵi(t), sensor fault σi(t), and bias fault βi are unknown but bounded.
Assumption 3.3. The external disturbances δi(t) encountered by the UAV are bounded and satisfy the |δi(t)|≤¯δi(t), where ¯δi(t) is the upper bound of the external disturbances.
Remark 3.1. Including fault-tolerant features in the control system helps prevent issues with sensors and actuators from affecting the entire system. This approach keeps the formation performing well.
Lemma 3.2. For the following non-linear system [31,32]:
˙z=f(z(t)),z(0)=zo. |
In the context of the given system, let z0 represent the initial state. If there is a continuous function V(z) that is both positive and unbounded, specific conclusions can be made about the behavior of the system based on the equation previously mentioned. This indicates that as the state of the system evolves, the value of V(z) does not approach a finite limit and continues to increase indefinitely.
● V(z)=0⟺zz=0,
● V≤−βVa1−λV,
where β, λ>0, and a1∈(0,1] (the origin of the system) is globally fixed, time-stable, and the time convergence satisfies the condition:
T≤1λ(1−a1)lnβ+λoVoa1β. |
Lemma 3.3. [33,34] Let ki>0,i=1,2,3,⋯,n and 0<K<2. Then
(n∑i=1ki2)K≤(n∑i=1kiK)2. |
Remark 3.4. The function fi(x,t) satisfies a Lipschitz condition with respect to x, meaning there exists a constant L>0 such that
||fi(x1,t)−fi(x2,t)||≤L||x1−x2||. |
This ensures that the system does not exhibit chaotic behavior and guarantees the existence and uniqueness of solutions based on fundamental results in differential equations. It is assume that f(x, t) is bounded for all x and t, ensuring that the system states remain finite. This is critical in preventing uncontrolled growth in state variables.
This section presents numerical simulations to validate and demonstrate the effectiveness of the proposed control scheme outlined in the previous sections. The Laplacian matrix L, adjacency matrix Aij, diagonal matrix D, and the directed topology of the leader and follower matrices, as illustrated in Figure 2, are set as follows:
The adjacency matrix is given as
Aij=(00.30.30.30.30.30.3000000.3000000.3000000.3000000.300000). |
The diagonal matrix is
D=(200000010000001000000000000010000000). |
The Laplacian matrix is given as
L=(1.5−0.3−0.3−0.3−0.3−0.3−0.30.30000−0.300.3000−0.3000.300−0.30000.30−0.300000.3). |
Figure 3 represents the graph which illustrates individually and in aggregate a possible evolution of states of agents in multi-agent systems, as this is affected by the proposed fault tolerant control schemes. xi(t) represents the coordinates of the center of mass or the velocity of the mass center of selected agents or groups of agents. Gains λ,γ are parameters that affect agents. ϵij,k indicates the disparities between the ones that were commanded to be tracked and the things that were tracked. Phase shifts or oscillation, or non-phase shifts or oscillation, means that when the trajectories of multiple agents merge over time, the fault-tolerant control convergence has been achieved. The rate of convergence was determined by the rate at which the trajectories of the agents stabilized to a point after they had been disturbed. Trajectories sharply changed and then settled at a new level which corresponded to actuator and sensor faults and recovery trends that followed prospects demonstrating the efficacy of sliding mode control.
Convergence is faster in the impulsive stochastic case since the time duration is shorter and shows sharp peaks which indicate sudden blows. Roundly designed control systems include fault tolerance and stabilize rapidly after both actuator, sensor fault, and stochastic impulse since all trajectories tend to flatten or merge within a very small time frame. Deviations caused by actuator faults appear in the form of steady-state errors, e.g., shifts in trajectory until the fault-tolerant mechanism propels in and makes the compensations. The aim of stating the definition of the system sliding mode control in the control task is that the equation in it contains the following terms, which allow replacing the influences of faults on the actuator by an equivalent control term ueqi(t), adjusting the switching control law and handling the sensor fault by switching control term uswi(t). This enables the robust nature of the sliding mode control system to formulate against sensor faults. The bounded principle which underlies the stochastic term \(\sigma_i(t) w(t) \) subject to certain bounds further enhances resilience in the control model. The new observer combined with a high-order sliding mode design, reduces the influence of these random poorly defined factors as part of disturbance inputs.
The formation control gains used are as λ=1.5,γ=0.5,ρ=1.0,α=0.1. Actuator, sensor faults, and stochastic impulse values are ϵ=0.5,σ=0.1,μ=0.1,σw=0.05. The time interval is taken as t=[0,10]. The formation error is calculated by
εij,k=εx,ij−εx,jp. |
The sliding surface is calculated using Eq (3.4), equivalent control and switching control are calculated using Eqs (3.7) and (3.8), and the control input by using Eq (3.9). The agent state is solved by a numerical technique called the R-K method.
Figure 4 illustrates the undertaking system's performance with regards to different scenarios: fault or impulse free, having the fault tolerant control and without it, and after and before having external disturbances or faults, demonstrating the performance of the system in holding or achieving the designated states formation even when there is an externally applied force or there is a fault that happens to the system. Time-stamped responses indicate the response of the system after an impulse and differences in trajectory behaviors indicate the ability of the system to withstand faults. This figure underlines regions that are sharply deviated, showing the impulse effects, and examines the recovery dynamics of the system when its required dynamics are restored after the disturbance. Comparative curves assist in evaluating the effectiveness enhancement under the fault tolerant strategy and better disturbance reduction shows better fault tolerance. Lyapunov stability eliminates any trajectory movement other than fractal motion, so by default si(t)→0. Error converges to zero. In the figure above, irregular fluctuations of the system are presented as a stochastic impulse mainly due to the response of the system. These fluctuations are earmarks of stochastic impulses which affect the stability and performance of the system. Figure 5 represents that the estimation tracking error converges to zero. Table 1 shows the comparative analysis of our method with different existing methods.
Method | Max Tracking Error | Convergence Time (s) | Chattering Effect | Fault Compensation Efficiency | Scalability |
PID-based FTC | High | Slow | None | Low | Poor |
Traditional SMC | Medium | Moderate | High | Medium | Limited |
Proposed FO-NFTSMC (Our Method) | Low | Fast | Minimal | High | Excellent |
In this study, a robust control strategy for non-linear systems was developed utilizing a non-singular fast terminal sliding mode approach. As they are combined with Lyapunov techniques, the resulting control architectures present finite-time stability and resourcefulness to faults and disturbances even in the presence of random impulses. It was demonstrated that the proposed control technique was effective in formation maintenance and fault repair in the presence of system and external interference. By means of appropriate Lyapunov functions, stability proofs of the systems and their fault tolerance are provided. The simulation results exhibited the usefulness of the approach whereby the agents succeeded in maintaining the desired formation while the effects of actuator and sensor faults and random impulses were reduced. These observations are enough to show the promise fractional-order control has in the development of robust and dependable multi-agent systems applicable in the real world.
Conceptualization, Marwa Balti; Software, Aasma Zaman; Validation, Meraa Arab; Formal analysis, Azmat Ullah Khan Niazi; Resources, Meraa Arab; Data curation, Aasma Zaman; Writing—original draft, Aasma Zaman; Writing—review and editing, Azmat Ullah Khan Niazi, Aasma Zaman; Supervision, Azmat Ullah Khan Niazi, Project administration, Meraa Arab. All authors have read and approved the final version of the manuscript for publication.
The authors declare that they have not used Artificial Intelligence (AI) tools in the creation of this article.
This work was supported by the Deanship of Scientific Research, Vice Presidency for Graduate Studies and Scientific Research, King Faisal University, Saudi Arabia [Grant No. KFU251257].
The authors declare no conflicts of interest.
The code is considered an intellectual property of the University of Lahore, Sargodha, and therefore is not publicly available.
[1] |
G. K. Fourlas, G. C. Karras, A survey on fault diagnosis and fault-tolerant control methods for unmanned aerial vehicles, Machines, 9 (2021), 197. https://doi.org/10.3390/machines9090197 doi: 10.3390/machines9090197
![]() |
[2] |
J. Lv, X. Ju, C. Wang, Neural network prescribed-time observer-based output-feedback control for uncertain pure-feedback nonlinear systems, Expert Syst. Appl., 264 (2025), 125813. https://doi.org/10.1016/j.eswa.2024.125813 doi: 10.1016/j.eswa.2024.125813
![]() |
[3] |
A. Tong, J. Zhang, L. Xie, Intelligent fault diagnosis of rolling bearing based on Gramian angular difference field and improved dual attention residual network, Sensors, 24 (2024), 2156. https://doi.org/10.3390/s24072156 doi: 10.3390/s24072156
![]() |
[4] |
X. Ju, Y. Jiang, L. Jing, P. Liu, Quantized predefined-time control for heavy-lift launch vehicles under actuator faults and rate gyro malfunctions, ISA Trans., 138 (2023), 133–150. https://doi.org/10.1016/j.isatra.2023.02.022 doi: 10.1016/j.isatra.2023.02.022
![]() |
[5] |
Q. Wang, Q. Chen, G. Xiao, P. Wang, P. Gu, J. Lu, Elevator fault diagnosis based on digital twin and PINNs-e-RGCN, Sci. Rep., 14 (2024), 30713. https://doi.org/10.1038/s41598-024-78784-7 doi: 10.1038/s41598-024-78784-7
![]() |
[6] |
T. Guan, B. Li, Y. Song, G. R. Duan, Fixed-time spacecraft attitude control with unwinding-free performance, IEEE Trans. Automat. Control, 70 (2024), 1898–1904. https://doi.org/10.1109/TAC.2024.3471333 doi: 10.1109/TAC.2024.3471333
![]() |
[7] |
X. Xu, B. Li, PDE-based observation and predictor-based control for linear systems with distributed infinite input and output delays, Automatica, 170 (2024), 111845. https://doi.org/10.1016/j.automatica.2024.111845 doi: 10.1016/j.automatica.2024.111845
![]() |
[8] |
Y. H. Lan, J. Y. Zhao, Improving track performance by combining padé-approximation-based preview repetitive control and equivalent-input-disturbance, J. Electr. Eng. Technol., 19 (2024), 3781–3794. https://doi.org/10.1007/s42835-024-01830-x doi: 10.1007/s42835-024-01830-x
![]() |
[9] |
X. Hu, T. Tang, L. Tan, H. Zhang, Fault detection for point machines: a review, challenges, and perspectives, Actuators, 12 (2023), 391. https://doi.org/10.3390/act12100391 doi: 10.3390/act12100391
![]() |
[10] |
L. Lin, J. Liu, N. Huang, S. Li, Y. Zhang, Multiscale spatio-temporal feature fusion based non-intrusive appliance load monitoring for multiple industrial industries, Appl. Soft Comput., 167 (2024), 112445. https://doi.org/10.1016/j.asoc.2024.112445 doi: 10.1016/j.asoc.2024.112445
![]() |
[11] |
L. Lin, X. Ma, C. Chen, J. Xu, N. Huang, Imbalanced industrial load identification based on optimized CatBoost with entropy features, J. Electr. Eng. Technol., 19 (2024), 4817–4832. https://doi.org/10.1007/s42835-024-01933-5 doi: 10.1007/s42835-024-01933-5
![]() |
[12] |
T. Li, H. Shi, X. Bai, N. Li, K. Zhang, Rolling bearing performance assessment with degradation twin modeling considering interdependent fault evolution, Mech. Syst. Signal Process., 224 (2025), 112194. https://doi.org/10.1016/j.ymssp.2024.112194 doi: 10.1016/j.ymssp.2024.112194
![]() |
[13] |
G. Du, H. Zhang, H. Yu, P. Hou, J. He, S. Cao, Study on automatic tracking system of microwave deicing device for railway contact wire, IEEE Trans. Instrum. Meas., 73 (2024), 1–11. https://doi.org/10.1109/TIM.2024.3446638 doi: 10.1109/TIM.2024.3446638
![]() |
[14] |
Y. Yin, Z. Wang, L. Zheng, Q. Su, Y. Guo, Autonomous UAV navigation with adaptive control based on deep reinforcement learning, Electronics, 13 (2024), 2432. https://doi.org/10.3390/electronics13132432 doi: 10.3390/electronics13132432
![]() |
[15] |
F. Ding, K. Zhu, J. Liu, C. Peng, Y. Wang, J. Lu, Adaptive memory event-triggered output feedback finite-time lane-keeping control for autonomous heavy truck with roll prevention, IEEE Trans. Fuzzy Syst., 32 (2024), 6607–6621. https://doi.org/10.1109/TFUZZ.2024.3454344 doi: 10.1109/TFUZZ.2024.3454344
![]() |
[16] |
L. Zhang, C. Ma, J. Liu, Enhancing four-axis machining center accuracy through interactive fusion of spatiotemporal graph convolutional networks and an error-controlled digital twin system, J. Manuf. Process., 112 (2024), 14–31. https://doi.org/10.1016/j.jmapro.2024.01.024 doi: 10.1016/j.jmapro.2024.01.024
![]() |
[17] |
X. Zhang, Y. Liu, X. Chen, Z. Li, C. Y. Su, Adaptive pseudoinverse control for constrained hysteretic nonlinear systems and its application on dielectric elastomer actuator, IEEE/ASME Trans. Mech., 28 (2023), 2142–2154. https://doi.org/10.1109/TMECH.2022.3231263 doi: 10.1109/TMECH.2022.3231263
![]() |
[18] |
L. Ji, Z. Lin, C. Zhang, S. Yang, J. Li, H. Li, Data-based optimal consensus control for multiagent systems with time delays: using prioritized experience replay, IEEE Trans. Syst., Man, Cybern.: Syst., 54 (2024), 3244–3256. https://doi.org/10.1109/TSMC.2024.3358293 doi: 10.1109/TSMC.2024.3358293
![]() |
[19] |
F. Wang, K. Chen, S. Zhen, X. Chen, H. Zheng, Z. Wang, Prescribed performance adaptive robust control for robotic manipulators with fuzzy uncertainty, IEEE Trans. Fuzzy Syst., 32 (2024), 1318–1330. https://doi.org/10.1109/TFUZZ.2023.3323090 doi: 10.1109/TFUZZ.2023.3323090
![]() |
[20] |
J. Hao, P. Chen, J. Chen, X. Li, Effectively detecting and diagnosing distributed multivariate time series anomalies via Unsupervised Federated Hypernetwork, Inform. Process. Manag., 62 (2025), 104107. https://doi.org/10.1016/j.ipm.2025.104107 doi: 10.1016/j.ipm.2025.104107
![]() |
[21] |
Z. Zou, S. Yang, L. Zhao, Dual-loop control and state prediction analysis of QUAV trajectory tracking based on biological swarm intelligent optimization algorithm, Sci. Rep., 14 (2024), 19091. https://doi.org/10.1038/s41598-024-69911-5 doi: 10.1038/s41598-024-69911-5
![]() |
[22] |
H. B. Zeng, Z. J. Zhu, T. S. Peng, W. Wang, X. M. Zhang, Robust tracking control design for a class of nonlinear networked control systems considering bounded package dropouts and external disturbance, IEEE Trans. Fuzzy Syst., 32 (2024), 3608–3617. https://doi.org/10.1109/TFUZZ.2024.3377799 doi: 10.1109/TFUZZ.2024.3377799
![]() |
[23] |
Y. Liu, Q. Hu, G. Feng, Navigation functions on 3-manifold with boundary as a disjoint union of hopf tori, IEEE Trans. Automat. Control, 70 (2024), 219–234. https://doi.org/10.1109/TAC.2024.3419817 doi: 10.1109/TAC.2024.3419817
![]() |
[24] |
Y. Zhao, H. Wu, Fixed/prescribed stability criterions of stochastic system with time-delay, AIMS Math., 9 (2024), 14425–14453. https://doi.org/10.3934/math.2024701 doi: 10.3934/math.2024701
![]() |
[25] |
X. Zhao, H. Wu, J. Cao, L. Wang, Prescribed-time synchronization for complex dynamic networks of piecewise smooth systems: a hybrid event-triggering control approach, Qual. Theory Dyn. Syst., 24 (2025), 11. https://doi.org/10.1007/s12346-024-01166-x doi: 10.1007/s12346-024-01166-x
![]() |
[26] |
X. Hou, H. Wu, J. Cao, Practical finite-time synchronization for Lur'e systems with performance constraint and actuator faults: a memory-based quantized dynamic event-triggered control strategy, Appl. Math. Comput., 487 (2025), 129108. https://doi.org/10.1016/j.amc.2024.129108 doi: 10.1016/j.amc.2024.129108
![]() |
[27] |
D. Cai, P. Fan, Q. Zou, Y. Xu, Z. Ding, Z. Liu, Active device detection and performance analysis of massive non-orthogonal transmissions in cellular internet of things, Sci. China Inf. Sci., 65 (2022), 182301. https://doi.org/10.1007/s11432-021-3328-y doi: 10.1007/s11432-021-3328-y
![]() |
[28] |
S. Zheng, C. Shen, X. Chen, Design and analysis of uplink and downlink communications for federated learning, IEEE J. Sel. Areas Commun., 39 (2020), 2150–2167. https://doi.org/10.1109/JSAC.2020.3041388 doi: 10.1109/JSAC.2020.3041388
![]() |
[29] |
Y. Guo, R. Zhao, S. Lai, L. Fan, X. Lei, G. K. Karagiannidis, Distributed machine learning for multiuser mobile edge computing systems, IEEE J. Sel. Top. Signal Process., 16 (2022), 460–473. https://doi.org/10.1109/JSTSP.2022.3140660 doi: 10.1109/JSTSP.2022.3140660
![]() |
[30] |
C. Tan, D. Cai, F. Fang, Z. Ding, P. Fan, Federated unfolding learning for CSI feedback in distributed edge networks, IEEE Trans. Commun., 73 (2025), 410–424. https://doi.org/10.1109/TCOMM.2024.3429170 doi: 10.1109/TCOMM.2024.3429170
![]() |
[31] |
A. Polyakov, Nonlinear feedback design for fixed-time stabilization of linear control systems, IEEE Trans. Automat. Control, 57 (2011), 2106–2110. https://doi.org/10.1109/TAC.2011.2179869 doi: 10.1109/TAC.2011.2179869
![]() |
[32] |
N. Ali, I. Tawiah, W. Zhang, Finite-time extended state observer-based nonsingular fast terminal sliding mode control of autonomous underwater vehicles, Ocean Eng., 218 (2020), 108179. https://doi.org/10.1016/j.oceaneng.2020.108179 doi: 10.1016/j.oceaneng.2020.108179
![]() |
[33] |
Y. Liu, G. H. Yang, Fixed-time fault-tolerant consensus control for multi-agent systems with mismatched disturbances, Neurocomputing, 366 (2019), 154–160. https://doi.org/10.1016/j.neucom.2019.07.093 doi: 10.1016/j.neucom.2019.07.093
![]() |
[34] |
S. T. Venkataraman, S. Gulati, Control of nonlinear systems using terminal sliding modes, J. Dyn. Syst., Meas., Control, 115 (1993), 554–560. https://doi.org/10.1115/1.2899138 doi: 10.1115/1.2899138
![]() |
Method | Max Tracking Error | Convergence Time (s) | Chattering Effect | Fault Compensation Efficiency | Scalability |
PID-based FTC | High | Slow | None | Low | Poor |
Traditional SMC | Medium | Moderate | High | Medium | Limited |
Proposed FO-NFTSMC (Our Method) | Low | Fast | Minimal | High | Excellent |