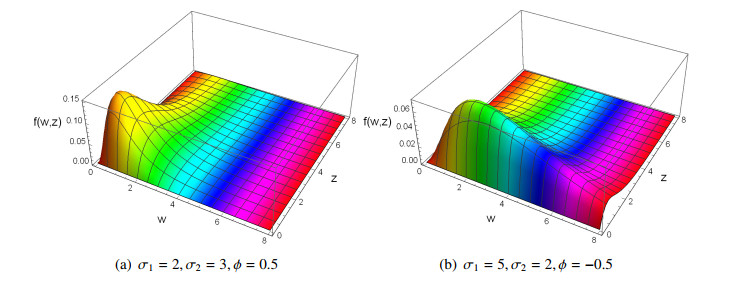
Ranked set sampling is a well-known and efficient method compared to simple random sampling for estimating population parameters. In this study, we focus on the challenge of estimating the scale parameter of the primary variable Z using a multistage ranked set sample obtained by ordering the marginal observations of an auxiliary variable W, where the pair (W,Z) follows the Farlie–Gumbel–Morgenstern bivariate Bilal distribution. Assuming that the dependence parameter ϕ is known, we introduce the best linear unbiased estimator for the scale parameter of the primary variable, utilizing a multistage ranked set sample. We also compare the efficiency of the proposed estimator with that of the maximum likelihood estimator based on the same number of measured units. It is found that the suggested estimators are more efficient than the classical estimators considered in this study.
Citation: S. P. Arun, M. R. Irshad, R. Maya, Amer I. Al-Omari, Shokrya S. Alshqaq. Parameter estimation in the Farlie–Gumbel–Morgenstern bivariate Bilal distribution via multistage ranked set sampling[J]. AIMS Mathematics, 2025, 10(2): 2083-2097. doi: 10.3934/math.2025098
[1] | M. Nagy, H. M. Barakat, M. A. Alawady, I. A. Husseiny, A. F. Alrasheedi, T. S. Taher, A. H. Mansi, M. O. Mohamed . Inference and other aspects for $ q- $Weibull distribution via generalized order statistics with applications to medical datasets. AIMS Mathematics, 2024, 9(4): 8311-8338. doi: 10.3934/math.2024404 |
[2] | Rasha Abd-Elwahaab Attwa, Taha Radwan, Esraa Osama Abo Zaid . Bivariate q-extended Weibull morgenstern family and correlation coefficient formulas for some of its sub-models. AIMS Mathematics, 2023, 8(11): 25325-25342. doi: 10.3934/math.20231292 |
[3] | H. M. Barakat, M. A. Alawady, I. A. Husseiny, M. Nagy, A. H. Mansi, M. O. Mohamed . Bivariate Epanechnikov-exponential distribution: statistical properties, reliability measures, and applications to computer science data. AIMS Mathematics, 2024, 9(11): 32299-32327. doi: 10.3934/math.20241550 |
[4] | Aisha Fayomi, Ehab M. Almetwally, Maha E. Qura . A novel bivariate Lomax-G family of distributions: Properties, inference, and applications to environmental, medical, and computer science data. AIMS Mathematics, 2023, 8(8): 17539-17584. doi: 10.3934/math.2023896 |
[5] | Areej M. AL-Zaydi . On concomitants of generalized order statistics arising from bivariate generalized Weibull distribution and its application in estimation. AIMS Mathematics, 2024, 9(8): 22002-22021. doi: 10.3934/math.20241069 |
[6] | I. A. Husseiny, M. Nagy, A. H. Mansi, M. A. Alawady . Some Tsallis entropy measures in concomitants of generalized order statistics under iterated FGM bivariate distribution. AIMS Mathematics, 2024, 9(9): 23268-23290. doi: 10.3934/math.20241131 |
[7] | Fiaz Ahmad Bhatti, Azeem Ali, G. G. Hamedani, Mustafa Ç. Korkmaz, Munir Ahmad . The unit generalized log Burr XII distribution: properties and application. AIMS Mathematics, 2021, 6(9): 10222-10252. doi: 10.3934/math.2021592 |
[8] | Salem A. Alyami, Amal S. Hassan, Ibrahim Elbatal, Naif Alotaibi, Ahmed M. Gemeay, Mohammed Elgarhy . Estimation methods based on ranked set sampling for the arctan uniform distribution with application. AIMS Mathematics, 2024, 9(4): 10304-10332. doi: 10.3934/math.2024504 |
[9] | Haidy A. Newer, Mostafa M. Mohie El-Din, Hend S. Ali, Isra Al-Shbeil, Walid Emam . Statistical inference for the Nadarajah-Haghighi distribution based on ranked set sampling with applications. AIMS Mathematics, 2023, 8(9): 21572-21590. doi: 10.3934/math.20231099 |
[10] | Nuran M. Hassan, M. Nagy, Subhankar Dutta . Statistical inference for the bathtub-shaped distribution using balanced and unbalanced sampling techniques. AIMS Mathematics, 2024, 9(9): 25049-25069. doi: 10.3934/math.20241221 |
Ranked set sampling is a well-known and efficient method compared to simple random sampling for estimating population parameters. In this study, we focus on the challenge of estimating the scale parameter of the primary variable Z using a multistage ranked set sample obtained by ordering the marginal observations of an auxiliary variable W, where the pair (W,Z) follows the Farlie–Gumbel–Morgenstern bivariate Bilal distribution. Assuming that the dependence parameter ϕ is known, we introduce the best linear unbiased estimator for the scale parameter of the primary variable, utilizing a multistage ranked set sample. We also compare the efficiency of the proposed estimator with that of the maximum likelihood estimator based on the same number of measured units. It is found that the suggested estimators are more efficient than the classical estimators considered in this study.
In 2013, [1] introduced a new lifetime distribution of one parameter, known as the Bilal distribution. The Bilal distribution is derived as a member of the family of distributions for the median of a random sample drawn from an exponential distribution. The cumulative distribution function (cdf) of the Bilal distribution with the scale parameter σ is given by:
F(x;σ)=1−e−2xσ(3−2e−xσ);σ>0,x≥0. | (1.1) |
The corresponding probability density function (pdf) is defined by:
f(x;σ)=6σe−2xσ(1−e−xσ);σ>0,x≥0, | (1.2) |
with survival function given by:
S(x;σ)=e−2xσ(3−2e−xσ);σ>0,x≥0. |
The Bilal distribution exhibits lower skewness and kurtosis compared to the exponential distribution and belongs to the class of distributions characterized as new, better than used in failure rates. Despite having only one parameter, the Bilal distribution demonstrates a strong capacity to fit two distinct real-world data sets: the first comprising 30 consecutive precipitation measurements (in inches) provided by [2], and the second consisting of waiting times before service for 100 bank customers, as reported by [3]. The closed-form expressions for all its statistical properties, includes key functions such as the quantile function, the hazard rate function (HRF), and a simple expression for the moments attracted several researchers and developed its various extensions. Abd-Elrahman and Niazi [4] proposed various estimators of the parameter for the Bilal distribution based on using Type-2 censored sample. To address the unimodal HRF of the Bilal distribution, Abd-Elrahman [5] proposed a two-parameter generalization referred to as the general Bilal distribution. A three-parameter generalization, called the Harris extended Bilal distribution was introduced, by [6], and its various properties have been discussed. Irshad et al. [7] proposed the Marshal–Olkin Bilal distribution, its applications in statistical process control, and the associated minification process. The proficiency of the univariate Bilal distribution has been firmly established in the literature, demonstrating its superiority over competing models in both theoretical and applied perspectives.
Maya et al. [8] introduced a bivariate version of the one-parameter Bilal distribution using the Morgenstern framework, called the Farlie–Gumbel–Morgenstern bivariate Bilal distribution (FGMBBD), and studied its inferential aspects based on concomitants of order statistics (COS). The pdf of FGMBBD is expressed as follows:
f(w,z)={36σ1σ2e−2wσ1(1−e−wσ1)e−2zσ2(1−e−zσ2)×[1+ϕ(2e−2wσ1{3−2e−wσ1}−1)(2e−2zσ2{3−2e−zσ2}−1)],w>0,z>0;σ1>0,σ2>0;−1≤ϕ≤1.0, otherwise. | (1.3) |
Figure 1 presents 3D plots of the FGMBBD pdf for various parameter values. We can see that the distribution has many shapes depending on the parameters values.
Arun et al. [9] evaluated the performance of the FGMBBD in comparison to the well-known FGM bivariate exponential distribution using two real-world data sets. The first data set, sourced from [10], consists of mineral content measurements in the dominant ulna (X) and non-dominant ulna (Y) of 25 elderly women. The second data set, also from [10], includes tail length (X) and wing length (Y) measurements in millimeters for a sample of 45 female hook-billed kites.
The marginal distributions of the variables W and Z are univariate Bilal distributions, with their respective pdf's given by:
fW(w)=6σ1e−2wσ1(1−e−wσ1);ifσ1>0,w≥0, |
and
fZ(z)=6σ2e−2zσ2(1−e−zσ2);ifσ2>0,z≥0. | (1.4) |
Clearly, E(W)=56σ1,Var(W)=1336σ21,
E(Z)=56σ2, | (1.5) |
and
Var(Z)=1336σ22. | (1.6) |
Note that the mean and variance values of Z depend on σ2. The ranked set sampling (RSS) method, originally developed by [11], is designed to improve the precision of the sample mean as an estimator of the population mean. In this approach, a total of n sets of units, each containing n units, are selected. The units within each set are ordered using a judgmental method or a cost-effective technique that does not involve actual measurements of the selected observations. Subsequently, the unit ranked first in the first set is measured, followed by the unit ranked second in the second set, and this process continues until the unit ranked n largest in the n-th set is measured. The observations obtained through this process form a ranked set sample (rss) of size n. Hence, we only measured n rss units: X1(1:n),X2(2:n),…,Xn(n:n), which are obtained from n2 units. For simplicity, we will use X(i:n) to denote the rss units.
Let the random variable (rv) X have a pdf f(x) and cdf F(x), with mean μ and variance σ2. Consider a simple random sample (srs) of size n, denoted as X1, X2, …, Xn. Let Xj(i:n) represent the ith largest unit in a set of size n in the jth sample. Takahasi and Wakimoto [12] demonstrated that the rss estimator of the population mean, when based on perfect ranking, is unbiased and outperforms the estimator based on srs because it has a lower variance. The mean estimators based on srs and rss are, respectively given by: ˉXSRS=1nn∑i=1Xi, and ˉXRSS=1nn∑i=1X(i:n), with respective variances given by Var(ˉXSRS)=σ2n, and Var(ˉXRSS)=σ2n−1n2n∑i=1(μ(i:n)−μ)2, where μ(i:k)=∞∫−∞xf(i:k)(x)dx. Clearly, Var(ˉXRSS) ≤ Var(ˉXSRS).
Stokes [13] introduced an alternative RSS scheme designed for situations where the primary variable of interest, denoted as Z, is challenging to measure directly, while an auxiliary variable, W, which is correlated with Z can be easily measured. The process involves selecting n independent bivariate sets, each containing n units. In the first set, the Z variable corresponding to the smallest ordered W is measured, followed by the Z variable associated with the second smallest W in the second set, and so on, until the Z variable corresponding to the largest W in the n-th set is measured. The resulting measurements on the Z variable from this new set of n units, selected according to the described method, constitute a rss, as proposed by [13]. Let W(i:n)i represent the observation on the auxiliary variable W from the unit obtained from the i-th set represent Z[i:n]i is used to denote the corresponding measurement of the study variable Z for that unit. Thus, Z[i:n]i for i=1,2,…,n, collectively constitutes the rss of size n. David and Nagaraja [14] mentioned Z[i:n]i as the concomitant variable of the i-th order statistic obtain from the i-th sample. Using Stoke's procedure of RSS, [15] proposed estimators of the scale parameter associated with the Z variable, when (W,Z) follows the FGMBBD.
Suppose that the bivariate random vector (W,Z) follows the FGMBBD with pdf given in (1.3). Draw a rss using [13] scheme. Let W(i:n)i represent the observation obtained on the auxiliary variate W from the ith unit of the rss, and let Z[i:n]i denote the corresponding measurement of the variable related to W(i:n)i,i=1,2,⋯,n. Hence, Z[i:n]i is the ith COS of a random sample of size n drawn from the FGMBBD, with pdf given by (see, [8]) as:
f[i:n](z)=6σ2e−2zσ2(1−e−zσ2)[1+ϕ(n−2i+1)(n+1)(2e−2zσ2{3−2e−zσ2}−1)]. | (1.7) |
Figure 2 shows 3D plots of the f[i:n](z) for some choices of n=10,i=1,10,ϕ=−0.9,0.9. The figure reflects the effect of these selections on the shape of this function.
The mean and variance of Z[i:n]i for i=1,2,⋯,n, are obtained as
E[Z[i:n]i]=σ2[56−1960ϕ(n−2i+1)(n+1)], | (1.8) |
and
Var[Z[i:n]i]=σ22[1336−2531800ϕ(n−2i+1)(n+1)−3613600ϕ2(n−2i+1)2(n+1)2]. | (1.9) |
Since, Z[i:n]i and Z[j:n]j for i≠j are arising from two independent samples, we obtain
Cov[Z[i:n]i,Z[j:n]j]=0,i≠j. |
Al-Saleh and Al-kadiri [16] extended McIntyre's [11] concept of RSS, by introducing the double-stage ranked set sampling (DSRSS). Their findings affirmed that estimators based on the DSRSS scheme exhibit greater efficiency in estimating population parameters compared to those based on both RSS and simple random sampling (SRS) schemes based on the same number of measured units. In a subsequent work, Al-Saleh and Al-Omari [17] extended the DSRSS to the multistage ranked set sampling (MSRSS) and demonstrated an increase in the precision of MSRSS estimators compared to both DSRSS and RSS estimators without needing to increase the sample size. Al-Saleh [18] introduced the concept of steady-state RSS and its corresponding parametric inference. Jemain and Al-Omari [19,20] proposed multistage percentile and quartile RSS methods for estimating the population mean. Jemain [21] suggested multistage median RSS for estimating the population median. For a deeper exploration of RSS and its variations, refer to [22], [23] for the varied L RSS method, [24] for an analysis of generalized robust-regression-type estimators under different RSS methods, and [25] for research on estimating a decreasing mean residual life based on RSS, with applications to survival analysis. Zamanzade et al. [26] considered nonparametric estimation of the mean residual lifetime in RSS with a concomitant variable. Zamanzade et al. [27] considered a ranked-based estimator of the mean past lifetime.
The aforementioned generalizations of RSS play a crucial role in developing inferential aspects of parent bivariate distributions in both theoretical and applied perspectives. Consequently, the objectives of this study are as follows:
(1) To identify the unit that has maximum Fisher information (FI) when ϕ is positive and negative.
(2) Building on the insights from the FI, we define the MSRSS and a steady-state RSS. Using these methodologies, we estimate the scale parameter associated with the study variable Z.
(3) To compare the efficiency of the proposed estimator based on MSRSS and steady-state RSS with the maximum likelihood estimator (MLE).
The rest of this paper is structured as follows. The FI included in the concomitant of a specific order statistic from a random sample arising from a distribution provides essential insight for selecting the most warranted unit(s) from a group of units to establish an appropriate RSS. Thus, the FI about the parameter σ2, included in the concomitant of the ith order statistic from a random sample of size n drawn from the FGMBBD, has been derived and is presented in Section 2. As per the knowledge disseminated from the FI, we have identified that for the FGMBBD, the maximum amount of information about the parameter σ2 is contained in the concomitant of the largest order statistic or the concomitant of the smallest order statistic, according as ϕ is positive or negative. Accordingly, we define an MSRSS in Section 2 and used its observations to introduce the best linear unbiased estimator (BLUE) of σ2 when the dependence parameter ϕ is positive and negative. Here, we have additionally obtained the efficiency of the estimator of σ2 based on the MSRSS and compared it with the MLE of σ2. In Section 3, we proposed BLUE of σ2 based on steady-state RSS and compared the efficiency of the same with respect to the MLE of σ2. The article concludes in Section 4 with some suggestions for future works.
Here, we define the MSRSS and propose the BLUE of σ2 when ϕ is positive and negative based on observations generated by the MSRSS. The MSRSS at r stages (see, [17]) is defined as follows:
(1) Randomly select nr+1 sample units from the target population, where r denotes the number of stages in the MSRSS process. These selected units are then randomly divided into nr−1 sets, each containing n2 units.
(2) For each set from Step 1, adopt Stoke's RSS scheme, to obtain an RSS of size n. This step results in nr−1 ranked sets, each of size n.
(3) Randomly organize the nr−1 ranked sets, each of size n obtained from Step 2, into nr−2 sets, each of size n2. Without performing any actual measurements, apply the RSS scheme to each of the nr−2 sets to produce nr−2 second-stage ranked sets, each of size n.
(4) The process continues, without conducting any actual measurements, until we obtain an rth stage ranked set of size n.
(5) Finally, the n identified units from Step 4 are actually quantified for the variable of interest.
To identify the most suitable unit from a group of units for measurements, we derive the FI regarding the scale parameter σ2 found in the concomitant of the ith order statistic from the FGMBBD.
The pdf of the concomitant of the ith order statistic given in (1.7) can be written as:
f[i:n](z)=6σ2e−2zσ2(1−e−zσ2)[d+2(1−d)e−2zσ2(3−2e−zσ2)], |
where d=d(ϕ,i,n)=1−ϕ(n−2i+1)(n+1). By taking the logarithm, and then the first derivative partially with respect to σ2, we obtain:
∂logf[i:n](z)∂σ2=1σ2[−1+2zσ2−ze−zσ2σ2(1−e−zσ2)+12(1−d)ze−2zσ2(1−e−zσ2)σ2{d+2(1−d)e−2zσ2(3−2e−zσ2)}]. |
Thus,
(∂logf[i:n](z)∂σ2)2=1σ22[−1+2u−ue−u(1−e−u)+12(1−d)ue−2u(1−e−u){d+2(1−d)e−2u(3−2e−u)}]2, |
where u=zσ2. The pdf of the transformed rv U=Zσ2 is obtained by
g(u)=6e−2u(1−e−u)[d+2(1−d)e−2u(3−2e−u)]. |
Then, the FI about the parameter σ2 contained in the concomitant of the ith order statistic is given by:
Jσ2(Z[i:n],ϕ)=E(∂logf[i:n](Z)∂σ2)2=σ2−2q(d), |
where
q(d)=∞∫0[−1+2u−ue−u(1−e−u)+12(1−d)ue−2u(1−e−u){d+2(1−d)e−2u(3−2e−u)}]2×6e−2u(1−e−u)[d+2(1−d)e−2u(3−2e−u)]du. | (2.1) |
Now, fix ϕ>0. Since, d=1−ϕ(n−2i+1)(n+1), i=1,2,⋯,n, the values of d lie in the interval 0≤1−ϕ≤d≤1+ϕ≤2. A graph of the q(d) on the interval 0≤1−ϕ≤d≤1+ϕ≤2 is presented in Figure 3.
From the graph, it is easily observed that the maximum value of the q(d) reaches at the upper extreme point of d, that is when i=n. Thus, one can conclude that when ϕ>0, the maximum FI is obtained in the concomitants of the largest order statistic. Hence, we consider the estimator based on the concomitants of the largest order statistic. Since d(ϕ,i,n)=d(−ϕ,n−i+1,n), we have Jσ2(Z[i:n],ϕ)=Jσ2(Z[n−i+1:n],−ϕ). Hence ϕ<0, the maximum FI is obtained in the concomitants of the smallest order statistic.
By assuming that the rv (W,Z) has a FGMBBD with pdf given in (1.3), where Z is the variable of primary interest and W is an auxiliary variable, in this section first we consider ϕ>0 and carry out a MSRSS based on measurements made on an auxiliary variate to choose the rss to estimate σ2 contained in FGMBBD based on the measurements made on the variable of primary interest. At each stage, from each set, we select the unit with the highest value on the auxiliary variable as the units of the ranked sets, aiming to maximize the FI about σ2 for the final chosen rss.
Let X(r)i,i=1,2,⋯,n be the MSRSS units chosen at the rth stage. Since, the actual measurement of the auxiliary variable on each unit X(r)i,i=1,2,⋯,n has the largest value, we can write Z(r)[n:n]i as the value measured on the variable of the primary interest to X(r)i,i=1,2,⋯,n. Thus, easily one can verify that Z(r)[n:n]i is the same as that of Z[nr:nr], the concomitant of the largest order statistic of nr independent and identically distributed bivariate rvs from the FGMBBD. Also, Z(r)[n:n]i,i=1,2,⋯,n are independently distributed with a pdf given by:
f(r)[n:n]i(z;ϕ)=6σ2e−2zσ2(1−e−zσ2)[1+ϕ(nr−1nr+1)(1−2e−2zσ2{3−2e−zσ2})]. | (2.2) |
Thus, the mean and variance of Z(r)[n:n]i,i=1,2,⋯,n are obtained as:
E[Z(r)[n:n]i]=σ2[56+1960ϕ(nr−1nr+1)], | (2.3) |
and
Var[Z(r)[n:n]i]=σ22[1336+2531800ϕ(nr−1nr+1)−3613600ϕ2(nr−1nr+1)2]. | (2.4) |
If we write
ζnr=56+1960ϕ(nr−1nr+1), | (2.5) |
and
ψnr=1336+2531800ϕ(nr−1nr+1)−3613600ϕ2(nr−1nr+1)2. | (2.6) |
Then, for i≤i≤n, (2.3) and (2.4) can be written as:
E[Z(r)[n:n]i]=σ2ζnr, | (2.7) |
and
Var[Z(r)[n:n]i]=σ22ψnr. | (2.8) |
Since Z(r)[n:n]i and Z(r)[n:n]j (for i≠j) are measurements on Z made from two units involved in two independent samples, we have
Cov(Z(r)[n:n]i,Z(r)[n:n]j)=0,fori≠j. | (2.9) |
Assume that Z(r)[n,n]=(Z(r)[n:n]1,Z(r)[n:n]2,⋯,Z(r)[n:n]n)′. Then, by using (2.7), we obtain the mean vector of Z(r)[n,n] as:
E[Z(r)[n,n]]=σ2ζnr1, | (2.10) |
and by using (2.8) and (2.9), the dispersion matrix of Z(r)[n,n] can be obtained as:
D[Z(r)[n,n]]=σ22ψnrI, | (2.11) |
where 1 is a column vector of n ones and I is a unit matrix of order n. If ϕ>0 is involved in ζnr and ψnr, then (2.10) and (2.11) together defines a generalized Gauss–Markov setup, and hence the BLUE of σ2 is obtained as:
˜σn(r)2=1nζnrn∑i=1Z(r)[n:n]i, | (2.12) |
and its variance is obtained as
Var(˜σn(r)2)=ψnrn(ζnr)2σ22. | (2.13) |
If we take r=1 in the MSRSS method elucidated above, in this case, we get the usual single-stage RSS. Hence, the BLUE ˜σn(1)2 of σ2 is given by
˜σn(1)2=1nζnn∑i=1Z[n:n]i, | (2.14) |
with variance given by
Var(˜σn(1)2)=ψnn(ζn)2σ22, | (2.15) |
where we write Z[n:n]i instead of Z(1)[n:n]i that represents the measurement of the variable of primary interest of the unit selected by the RSS. Also, ζn and ψn obtained by putting r=1 in (2.5) and (2.6), respectively. Irshad et al. [15] computed the asymptotic variance of the MLE ^σ2 of σ2. Using those values, we have evaluated the ratio e(˜σn(1)2|^σ2)=Var(^σ2)Var(˜σn(1)2) for ϕ=0.25(0.25)1; n=2(2)20 as a measure of the efficiency of our estimator ˜σn(1)2 compared to the MLE ^σ2 based on n observations and the results are given in Table 1. From the table one can see that the efficiency increases with increases in ϕ and n. For example, when ϕ=0.5 and n=2,8, we have ˜σn(1)2=1.0596 and 1.1773, respectively. Also, for n=6 and ϕ=0.25,1, the efficiency values are ˜σn(1)2=1.0720 and 1.3580, respectively. However, it is clear that there is not much difference in the efficiency values for negative and positive values of ϕ.
n | ϕ | e(˜σn(1)2|ˆσ2) | ϕ | e(˜σ1(1)2|ˆσ2) |
0.25 | 1.0302 | -0.25 | 1.0302 | |
2 | 0.50 | 1.0596 | -0.50 | 1.0596 |
0.75 | 1.0863 | -0.75 | 1.0863 | |
1.00 | 1.1026 | -1.00 | 1.1030 | |
0.25 | 1.0588 | -0.25 | 1.0588 | |
4 | 0.50 | 1.1259 | -0.50 | 1.1259 |
0.75 | 1.1989 | -0.75 | 1.1989 | |
1.00 | 1.2702 | -1.00 | 1.2710 | |
0.25 | 1.0720 | -0.25 | 1.0720 | |
6 | 0.50 | 1.1577 | -0.50 | 1.1577 |
0.75 | 1.2563 | -0.75 | 1.2563 | |
1.00 | 1.3580 | -1.00 | 1.3600 | |
0.25 | 1.0800 | -0.25 | 1.0800 | |
8 | 0.50 | 1.1773 | -0.50 | 1.1773 |
0.75 | 1.2901 | -0.75 | 1.2901 | |
1.00 | 1.4123 | -1.00 | 1.4150 | |
0.25 | 1.0858 | -0.25 | 1.0858 | |
10 | 0.50 | 1.3273 | -0.50 | 1.3273 |
0.75 | 1.3119 | -0.75 | 1.3119 | |
1.00 | 1.4461 | -1.00 | 1.4490 | |
0.25 | 1.0882 | -0.25 | 1.0882 | |
12 | 0.50 | 1.1950 | -0.50 | 1.1950 |
0.75 | 1.3292 | -0.75 | 1.3292 | |
1.00 | 1.4733 | -1.00 | 1.4733 | |
0.25 | 1.0882 | -0.25 | 1.0882 | |
14 | 0.50 | 1.2026 | -0.50 | 1.2026 |
0.75 | 1.3395 | -0.75 | 1.3395 | |
1.00 | 1.4979 | -1.00 | 1.4979 | |
0.25 | 1.0909 | -0.25 | 1.0909 | |
16 | 0.50 | 1.2105 | -0.50 | 1.2105 |
0.75 | 1.3475 | -0.75 | 1.3475 | |
1.00 | 1.5122 | -1.00 | 1.5122 | |
0.25 | 1.0951 | -0.25 | 1.0951 | |
18 | 0.50 | 1.2119 | -0.50 | 1.2119 |
0.75 | 1.3606 | -0.75 | 1.3606 | |
1.00 | 1.5249 | -1.00 | 1.5249 | |
0.25 | 1.0928 | -0.25 | 1.0928 | |
20 | 0.50 | 1.2170 | -0.50 | 1.2170 |
0.75 | 1.3583 | -0.75 | 1.3583 | |
1.00 | 1.5309 | -1.00 | 1.5309 |
Al-Saleh [18] has proposed the steady-state RSS by letting r go to +∞. If we apply the steady state RSS, then the asymptotic distribution of Z(r)[n:n]i is obtained from (2.2) with a pdf given by
f(∞)[n:n]i(z;ϕ)=6σ2e−2zσ2(1−e−zσ2)[1+ϕ(1−2e−2zσ2{3−2e−zσ2})]. | (3.1) |
Clearly, Z(∞)[n:n]i,i=1,2,⋯,n are independent and identically distributed (iid) rvs, each with pdf defined in (3.1). Then, Z(∞)[n:n]i,i=1,2,⋯,n, may be considered as steady-state rss of size n. Therefore, from (2.3) and (2.4), the mean and variance of Z(∞)[n:n]i for i=1,2,⋯,n are obtained as:
E[Z(∞)[n:n]i]=σ2[56+1960ϕ], | (3.2) |
and
Var[Z(∞)[n:n]i]=σ22[1336+2531800ϕ−3613600ϕ2]. | (3.3) |
Let Z(∞)[n:n]=(Z(∞)[n:n]1,Z(∞)[n:n]2,⋯,Z(∞)[n:n]n)′. Then, the BLUE ˜σn(∞)2 based on Z(∞)[n:n] and the variance of ˜σn(∞)2 is obtained by taking the limit as r→∞ in (2.12) and (2.13), respectively, and are given by
˜σn(∞)2=1n[56+1960ϕ]n∑i=1Z(∞)[n:n]i, | (3.4) |
and
Var(˜σn(∞)2)=[1336+2531800ϕ−3613600ϕ2]n[56+1960ϕ]2σ22. | (3.5) |
Using the results of [15], we obtain the efficiency of ˜σn(∞)2 relative to ^σ2 by taking the ratio of Var(^σ2) with respect to Var(˜σn(∞)2) and is given by:
E(˜σn(∞)2|^σ2)=Var(^σ2)Var(˜σn(∞)2)=I(−1)σ2(ϕ)[56+1960ϕ]2[1336+2531800ϕ−3613600ϕ2]. |
Thus, the efficiency e(˜σn(∞)2|^σ2) is independent of the sample size n, and that is for a fixed ϕ, the e(˜σn(∞)2|^σ2) has the same values for all n.
Additionally, we have computed the value of e(˜σn(∞)2|^σ2) for ϕ=0.25(0.25)1, and the results are given in Table 2. From the table one can observe that the efficiency of ˜σn(∞)2 increases as ϕ increases. Moreover, the estimator ˜σn(∞)2 possesses the highest efficiency among other estimators of σ2 considered in this study, and the value of the efficiencies varies from 1.1073 to 1.6352.
ϕ | e(˜σn(∞)2|^σ2) | ϕ | e(˜σ1(∞)2|^σ2) |
0.25 | 1.1073 | -0.25 | 1.1073 |
0.50 | 1.2472 | -0.50 | 1.2472 |
0.75 | 1.4223 | -0.75 | 1.4226 |
1.00 | 1.6352 | -1.00 | 1.6365 |
As mentioned earlier in the case of FGMBBD, when ϕ<0, the concomitant of the smallest order statistic contains the maximum FI about σ2. Consequently, we explore an MSRSS in this scenario, where we select a unit of a sample with the smallest value on the auxiliary variable as the units of ranked sets at each step and from each set, with the goal of maximizing FI on the final selected rss.
Let Z(r)[1:n]i,i=1,2,⋯,n, represents the value measured on the primary variable of interest for the units selected at the rth stage of the MSRSS. Then, it is simple to see that Z(r)[1:n]i, the concomitant of the least order statistic of nr iid bivariate random variables with FGMBBD, has the same distribution as Z[1:nr]. Moreover, Z(r)[1:n]i,i=1,2,⋯,n, are also independently distributed with pdf given by:
f(r)[1:n]i(z;ϕ)=6σ2e−2zσ2(1−e−zσ2)[1−ϕ(nr−1nr+1)(1−2e−2zσ2{3−2e−zσ2})]. | (3.6) |
Clearly, from (2.2) and (3.6), we have:
f(r)[1:n]i(z;ϕ)=f(r)[n:n]i(z;−ϕ). | (3.7) |
Hence, it is obvious that E(Z(r)[n:n]i) for ϕ>0 and E(Z(r)[1:n]i) for ϕ<0 are the same. Similarly, Var(Z(r)[n:n]i) for ϕ>0 and Var(Z(r)[1:n]i) for ϕ<0 are the same. Consequently, if ˜σ1(r)2 is the BLUE of σ2 involved in the FGMBBD for ϕ<0 based on the MSRSS observations Z(r)[1:n]i,i=1,2,⋯,n, then the coefficients of Z(r)[1:n]i,i=1,2,⋯,n in the BLUE ˜σ1(r)2 for ϕ<0 the same as the coefficients of Z(r)[n:n]i,i=1,2,⋯,n in the BLUE ˜σn(r)2 for ϕ>0.
Further, we have Var(˜σ1(r)2)=Var(˜σn(r)2). Hence, Var(˜σ1(1)2)=Var(˜σn(1)2) and Var(˜σ1(∞)2)=Var(˜σn(∞)2), where ˜σ1(1)2 is the BLUE of σ2 for ϕ<0 is the usual single-stage rss observations Z(r)[1:n]i,i=1,2,⋯,n and ˜σ1(∞)2 is the BLUE of σ2 for ϕ<0 based on the steady-state rss observations Z(∞)[1:n]i,i=1,2,⋯,n. We have computed the efficiency e(˜σ1(r)2|ˆσ2) of the BLUE ˜σ1(r)2 relative to ˆσ2, the MLE of σ2 for ϕ=−1,−0.75,−0.50,−0.25; n=2(2)20 and the results are included in Table 1. Also, we have computed e(˜σ1(∞)2|ˆσ2) for ϕ=−1,−0.75,−0.50,and−0.25, and the results are given in Table 2. Based on Table 2, one can infer that the efficiency increases as |ϕ| increases, and the value of the efficiency varies from 1.1073 to 1.6365.
Remark 3.1. If (W,Z) follows a FGMBBD with pdf given in (1.3), then the correlation coefficient between W and Z is given by
Corr(W,Z)=3611300ϕ,−1≤ϕ≤1. |
Obviously, the correlation coefficient is maximum when |ϕ| is as large as 1. From the tables, we can observe that the efficiencies of all estimators increase as |ϕ| increases for a given sample size. Consequently, we observe that more information about σ2 can be extracted from the RSS when |ϕ| is large. Therefore, we conclude that concomitant ranking is more effective for estimating σ2 when the modulus value of the dependence parameter ϕ is large (ϕ approaches to ±1).
Remark 3.2. The assumption that ϕ is known may be considered unrealistic in certain real-life situations. [8] addressed this issue by proposing a moment-type estimator for ϕ, which is expressed as follows:
ˆϕ={−1,ifϰ<−36113001300361ϰ,if−3611300≤ϰ≤36113001,ifϰ>3611300, |
where ϰ is the sample correlation from (W(i:n)i,Z[i:n]i) for i=1,2,⋯,n.
This study focuses on estimating the scale parameter of the primary variable Z using the MSRSS, where the ranking is based on the marginal observations of an auxiliary variable W. It is assumed that the joint distribution of (W,Z) follows the FGMBBD. Considering the dependence parameter ϕ is known, the BLUE for the scale parameter of Z under MSRSS as well as steady-state RSS schemes is presented. The efficiency of the suggested estimators is compared to the MLE based on the same number of measured units. The results indicate that the proposed estimators are more efficient than the classical estimators considered in this study. For further studies, one can estimate other parameters or use different modifications of RSS to estimate the same parameter.
Conceptualization, S.P.A., M.R.I., R.M., S.S.A., and A.I.A; methodology, S.P.A., M.R.I., and R.M.; software, S.P.A., M.R.I., A.I.A., and R.M.; validation, S.P.A., M.R.I., R.M., S.S.A., and A.I.A.; formal analysis, M.R.I., S.P.A., R.M., and A.I.A; writing-original draft preparation, M.R.I., S.P.A., R.M., A.I.A., and S.S.A.; writing-review and editing, M.R.I., S.P.A., R.M., A.I.A., and S.S.A.; visualization, M.R.I., S.P.A., R.M., A.I.A., and S.S.A. All authors have read and approved the final version of the manuscript for publication.
The authors declare they have not used Artificial Intelligence (AI) tools in the creation of this article.
There is no conflict of interest with the publication of this work.
[1] | A. M. Abd-Elrahman, Utilizing ordered statistics in lifetime distributions production: a new lifetime distribution and applications, J. Probab. Stat. Sci., 11 (2013), 153–164. |
[2] |
D. Hinkley, On quick choice of power transformation, Appl. Stat., 26 (1977), 67–69. https://doi.org/10.2307/2346869 doi: 10.2307/2346869
![]() |
[3] |
M. E. Ghitany, B. Atieh, S. Nadarajah, Lindley distribution and its application, Math. comput. simul., 78 (2008), 493–506. https://doi.org/10.1016/j.matcom.2007.06.007 doi: 10.1016/j.matcom.2007.06.007
![]() |
[4] |
A. M. Abd-Elrahman, S. F. Niazi, Approximate Bayes estimators applied to the Bilal model, J. Egypt. Math. Soc., 25 (2017), 65–70. https://doi.org/10.1016/j.joems.2016.05.001 doi: 10.1016/j.joems.2016.05.001
![]() |
[5] |
A. M. Abd-Elrahman, A new two-parameter lifetime distribution with decreasing, increasing or upside-down bathtub-shaped failure rate, Commun. Stat.-Theory Methods, 46 (2017), 8865–8880. https://doi.org/10.1080/03610926.2016.1193198 doi: 10.1080/03610926.2016.1193198
![]() |
[6] |
R. Maya, M. R. Irshad, M. Ahammed, C. Chesneau, The Harris extended Bilal distribution with applications in hydrology and quality control, AppliedMath, 3 (2023), 221–242. https://doi.org/10.3390/appliedmath3010013 doi: 10.3390/appliedmath3010013
![]() |
[7] |
M. R. Irshad, M. Ahammed, R. Maya, A. I. Al-Omari, Marshal-Olkin Bilal distribution with associated minification process and accptance sampling plans, Hacet. J. Math. Stat., 53 (2023), 201–229. https://doi.org/10.15672/hujms.1143156 doi: 10.15672/hujms.1143156
![]() |
[8] | R. Maya, M. R. Irshad, S. P. Arun, Farlie–Gumbel–Morgenstern bivariate Bilal distribution and its inferential aspects using concomitants of order statistics, J. Probab. Stat. Sci., 19 (2021), 1–20. |
[9] |
S. P. Arun, C. Chesneau, R. Maya, M. R. Irshad, Farlie–Gumbel–Morgenstern bivariate moment exponential distribution and its inferences based on concomitants of order statistics, Stats, 6 (2023), 253–267. https://doi.org/10.3390/stats6010015 doi: 10.3390/stats6010015
![]() |
[10] | R. A. Johnson, D. W. Wichern, Applied multivariate statistical analysis, Pearson College Div, Subsequent edition, 2002. |
[11] |
G. A. McIntyre, A method for unbiased selective sampling using ranked sets, Australian J. Agr. Res., 3 (1952), 385–390. https://doi.org/10.1071/AR9520385 doi: 10.1071/AR9520385
![]() |
[12] | K. Takahasi, K. Wakimoto, On unbiased estimates of the population mean based on the sample stratified by means of ordering, Ann. Inst. Stat. Math., 20 (1968), 1–31. |
[13] |
S. L. Stokes, Ranked set sampling with concomitant variables, Commun. Stat.-Theory Methods, 6 (1977), 1207–1211. https://doi.org/10.1080/03610927708827563 doi: 10.1080/03610927708827563
![]() |
[14] | H. A. David, H. N. Nagaraja, Order statistics, 3 Eds., John Wiley and Sons, New York, 2003. |
[15] | M. R. Irshad, R. Maya, A. I. Al-Omari, A. A. Hanadeh, S. P. Arun, Estimation of a parameter of Farlie–Gumbel–Morgenstern bivariate Bilal distribution by ranked set sampling, Reliability: Theory Appl., 18 (2023), 129–140. |
[16] | M. F. Al-Saleh, M. Al-Kadiri, Double-ranked set sampling, Stat. Probabil. Lett., 48 (2000), 205–212. https://doi.org/10.1016/S0167-7152(99)00206-0 |
[17] |
M. F. Al-Saleh, A. I. Al-Omari, Multistage ranked set sampling, J. Stat. plan. Infer., 102 (2002), 273–286. https://doi.org/10.1016/S0378-3758(01)00086-6 doi: 10.1016/S0378-3758(01)00086-6
![]() |
[18] |
M. F. Al-Saleh, Steady-state ranked set sampling and parametric estimation, J. Stat. plan. Infer., 123 (2004), 83–95. https://doi.org/10.1016/S0378-3758(03)00139-3 doi: 10.1016/S0378-3758(03)00139-3
![]() |
[19] | A. A. Jemain, A. I. Al-Omari, Multistage percentile ranked set samples, Adv. Appl. Stat., 7 (2007), 127–139. |
[20] | A. A. Jemain, A. I. Al-Omari, Multistage quartile ranked set samples, Pakistan J. Stat., 23 (2007), 11–22. |
[21] | A. A. Jemain, A. I. Al-Omari, K. Ibrahim, Multistage median ranked set sampling for estimating the population median, J. Math. Stat., 3 (2007), 58–64. |
[22] | A. I. Al-Omari, C. N. Bouza, Review of ranked set sampling: modifications and applications, Revista Investigacion Operacional, 35 (2014), 215–240. |
[23] |
A. Haq, J. Brown, E. Moltchanova, A. I. Al-Omari, Varied L ranked set sampling scheme, J. Stat. Theory Pract., 9 (2015), 741–767. https://doi.org/10.1080/15598608.2015.1008606 doi: 10.1080/15598608.2015.1008606
![]() |
[24] |
N. Koyuncu, A. I. Al-Omari, Generalized robust-regression-type estimators under different ranked set sampling, Math. Sci., 15 (2021), 29–40. https://doi.org/10.1007/s40096-020-00360-7 doi: 10.1007/s40096-020-00360-7
![]() |
[25] |
E. Zamanzade, E. Zamanzade, A. Parvardeh, Estimation of a decreasing mean residual life based on ranked set sampling with an application to survival analysis, Int. J. Biostat., 20 (2024), 571–583. https://doi.org/10.1515/ijb-2023-0051 doi: 10.1515/ijb-2023-0051
![]() |
[26] |
E. Zamanzade, M. Mahdizadeh, H. M. Samawi, Nonparametric estimation of mean residual lifetime in ranked set sampling with a concomitant variable, J. Appl. Stat., 51 (2024), 2512–2528. https://doi.org/10.1080/02664763.2023.2301334 doi: 10.1080/02664763.2023.2301334
![]() |
[27] |
E. Zamanzade, M. Asadi, A. Parvardeh, E. Zamanzade, A ranked-based estimator of the mean past lifetime with an application, Stat. Papers, 64 (2023), 161–177. https://doi.org/10.1007/s00362-022-01314-y doi: 10.1007/s00362-022-01314-y
![]() |
n | ϕ | e(˜σn(1)2|ˆσ2) | ϕ | e(˜σ1(1)2|ˆσ2) |
0.25 | 1.0302 | -0.25 | 1.0302 | |
2 | 0.50 | 1.0596 | -0.50 | 1.0596 |
0.75 | 1.0863 | -0.75 | 1.0863 | |
1.00 | 1.1026 | -1.00 | 1.1030 | |
0.25 | 1.0588 | -0.25 | 1.0588 | |
4 | 0.50 | 1.1259 | -0.50 | 1.1259 |
0.75 | 1.1989 | -0.75 | 1.1989 | |
1.00 | 1.2702 | -1.00 | 1.2710 | |
0.25 | 1.0720 | -0.25 | 1.0720 | |
6 | 0.50 | 1.1577 | -0.50 | 1.1577 |
0.75 | 1.2563 | -0.75 | 1.2563 | |
1.00 | 1.3580 | -1.00 | 1.3600 | |
0.25 | 1.0800 | -0.25 | 1.0800 | |
8 | 0.50 | 1.1773 | -0.50 | 1.1773 |
0.75 | 1.2901 | -0.75 | 1.2901 | |
1.00 | 1.4123 | -1.00 | 1.4150 | |
0.25 | 1.0858 | -0.25 | 1.0858 | |
10 | 0.50 | 1.3273 | -0.50 | 1.3273 |
0.75 | 1.3119 | -0.75 | 1.3119 | |
1.00 | 1.4461 | -1.00 | 1.4490 | |
0.25 | 1.0882 | -0.25 | 1.0882 | |
12 | 0.50 | 1.1950 | -0.50 | 1.1950 |
0.75 | 1.3292 | -0.75 | 1.3292 | |
1.00 | 1.4733 | -1.00 | 1.4733 | |
0.25 | 1.0882 | -0.25 | 1.0882 | |
14 | 0.50 | 1.2026 | -0.50 | 1.2026 |
0.75 | 1.3395 | -0.75 | 1.3395 | |
1.00 | 1.4979 | -1.00 | 1.4979 | |
0.25 | 1.0909 | -0.25 | 1.0909 | |
16 | 0.50 | 1.2105 | -0.50 | 1.2105 |
0.75 | 1.3475 | -0.75 | 1.3475 | |
1.00 | 1.5122 | -1.00 | 1.5122 | |
0.25 | 1.0951 | -0.25 | 1.0951 | |
18 | 0.50 | 1.2119 | -0.50 | 1.2119 |
0.75 | 1.3606 | -0.75 | 1.3606 | |
1.00 | 1.5249 | -1.00 | 1.5249 | |
0.25 | 1.0928 | -0.25 | 1.0928 | |
20 | 0.50 | 1.2170 | -0.50 | 1.2170 |
0.75 | 1.3583 | -0.75 | 1.3583 | |
1.00 | 1.5309 | -1.00 | 1.5309 |
ϕ | e(˜σn(∞)2|^σ2) | ϕ | e(˜σ1(∞)2|^σ2) |
0.25 | 1.1073 | -0.25 | 1.1073 |
0.50 | 1.2472 | -0.50 | 1.2472 |
0.75 | 1.4223 | -0.75 | 1.4226 |
1.00 | 1.6352 | -1.00 | 1.6365 |
n | ϕ | e(˜σn(1)2|ˆσ2) | ϕ | e(˜σ1(1)2|ˆσ2) |
0.25 | 1.0302 | -0.25 | 1.0302 | |
2 | 0.50 | 1.0596 | -0.50 | 1.0596 |
0.75 | 1.0863 | -0.75 | 1.0863 | |
1.00 | 1.1026 | -1.00 | 1.1030 | |
0.25 | 1.0588 | -0.25 | 1.0588 | |
4 | 0.50 | 1.1259 | -0.50 | 1.1259 |
0.75 | 1.1989 | -0.75 | 1.1989 | |
1.00 | 1.2702 | -1.00 | 1.2710 | |
0.25 | 1.0720 | -0.25 | 1.0720 | |
6 | 0.50 | 1.1577 | -0.50 | 1.1577 |
0.75 | 1.2563 | -0.75 | 1.2563 | |
1.00 | 1.3580 | -1.00 | 1.3600 | |
0.25 | 1.0800 | -0.25 | 1.0800 | |
8 | 0.50 | 1.1773 | -0.50 | 1.1773 |
0.75 | 1.2901 | -0.75 | 1.2901 | |
1.00 | 1.4123 | -1.00 | 1.4150 | |
0.25 | 1.0858 | -0.25 | 1.0858 | |
10 | 0.50 | 1.3273 | -0.50 | 1.3273 |
0.75 | 1.3119 | -0.75 | 1.3119 | |
1.00 | 1.4461 | -1.00 | 1.4490 | |
0.25 | 1.0882 | -0.25 | 1.0882 | |
12 | 0.50 | 1.1950 | -0.50 | 1.1950 |
0.75 | 1.3292 | -0.75 | 1.3292 | |
1.00 | 1.4733 | -1.00 | 1.4733 | |
0.25 | 1.0882 | -0.25 | 1.0882 | |
14 | 0.50 | 1.2026 | -0.50 | 1.2026 |
0.75 | 1.3395 | -0.75 | 1.3395 | |
1.00 | 1.4979 | -1.00 | 1.4979 | |
0.25 | 1.0909 | -0.25 | 1.0909 | |
16 | 0.50 | 1.2105 | -0.50 | 1.2105 |
0.75 | 1.3475 | -0.75 | 1.3475 | |
1.00 | 1.5122 | -1.00 | 1.5122 | |
0.25 | 1.0951 | -0.25 | 1.0951 | |
18 | 0.50 | 1.2119 | -0.50 | 1.2119 |
0.75 | 1.3606 | -0.75 | 1.3606 | |
1.00 | 1.5249 | -1.00 | 1.5249 | |
0.25 | 1.0928 | -0.25 | 1.0928 | |
20 | 0.50 | 1.2170 | -0.50 | 1.2170 |
0.75 | 1.3583 | -0.75 | 1.3583 | |
1.00 | 1.5309 | -1.00 | 1.5309 |
ϕ | e(˜σn(∞)2|^σ2) | ϕ | e(˜σ1(∞)2|^σ2) |
0.25 | 1.1073 | -0.25 | 1.1073 |
0.50 | 1.2472 | -0.50 | 1.2472 |
0.75 | 1.4223 | -0.75 | 1.4226 |
1.00 | 1.6352 | -1.00 | 1.6365 |