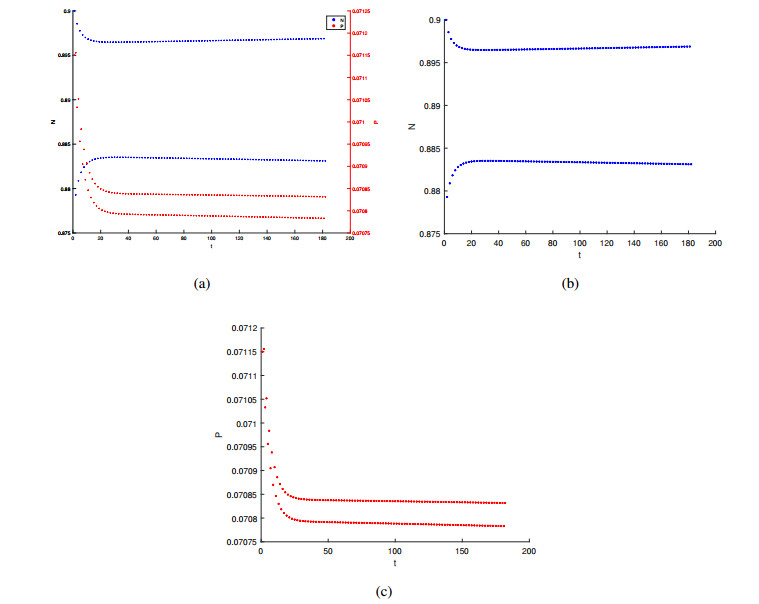
In this study, we introduce a novel reaction-diffusion epidemic model to analyze the transmission dynamics of the hepatitis B virus (HBV). The model captured the interactions between five population groups: Susceptible individuals, those in the latent stage, acutely infected individuals, chronically infected individuals, and those who have recovered, while considering the spatial movement of these groups. Chronic HBV infection contributes to severe liver diseases such as cirrhosis and hepatocellular carcinoma. It is also a major cause of long-term disability due to complications that impair daily functioning. The stability conditions for the model were derived, and the basic reproductive number, R0, was calculated using the next-generation matrix approach. Numerical simulations were performed using the Crank-Nicolson operator splitting method and the Unconditionally Positivity Preserving technique to solve the model under scenarios with and without diffusion. The stability of the endemic equilibrium point was analyzed comprehensively. Detailed simulation results are presented, highlighting a comparative analysis of the numerical findings in cases where exact solutions were unavailable. The reliability of the numerical results was validated by their alignment with theoretical expectations.
Citation: Kamel Guedri, Rahat Zarin, Ashfaq Khan, Amir Khan, Basim M. Makhdoum, Hatoon A. Niyazi. Modeling hepatitis B transmission dynamics with spatial diffusion and disability potential in the chronic stage[J]. AIMS Mathematics, 2025, 10(1): 1322-1349. doi: 10.3934/math.2025061
[1] | A. Q. Khan, Ibraheem M. Alsulami . Complicate dynamical analysis of a discrete predator-prey model with a prey refuge. AIMS Mathematics, 2023, 8(7): 15035-15057. doi: 10.3934/math.2023768 |
[2] | Figen Kangalgil, Seval Isșık . Effect of immigration in a predator-prey system: Stability, bifurcation and chaos. AIMS Mathematics, 2022, 7(8): 14354-14375. doi: 10.3934/math.2022791 |
[3] | Xiaoming Su, Jiahui Wang, Adiya Bao . Stability analysis and chaos control in a discrete predator-prey system with Allee effect, fear effect, and refuge. AIMS Mathematics, 2024, 9(5): 13462-13491. doi: 10.3934/math.2024656 |
[4] | Xiongxiong Du, Xiaoling Han, Ceyu Lei . Dynamics of a nonlinear discrete predator-prey system with fear effect. AIMS Mathematics, 2023, 8(10): 23953-23973. doi: 10.3934/math.20231221 |
[5] | Wei Li, Qingkai Xu, Xingjian Wang, Chunrui Zhang . Dynamics analysis of spatiotemporal discrete predator-prey model based on coupled map lattices. AIMS Mathematics, 2025, 10(1): 1248-1299. doi: 10.3934/math.2025059 |
[6] | A. Q. Khan, Ibraheem M. Alsulami, S. K. A. Hamdani . Controlling the chaos and bifurcations of a discrete prey-predator model. AIMS Mathematics, 2024, 9(1): 1783-1818. doi: 10.3934/math.2024087 |
[7] | Yingyan Zhao, Changjin Xu, Yiya Xu, Jinting Lin, Yicheng Pang, Zixin Liu, Jianwei Shen . Mathematical exploration on control of bifurcation for a 3D predator-prey model with delay. AIMS Mathematics, 2024, 9(11): 29883-29915. doi: 10.3934/math.20241445 |
[8] | Jie Liu, Qinglong Wang, Xuyang Cao, Ting Yu . Bifurcation and optimal harvesting analysis of a discrete-time predator–prey model with fear and prey refuge effects. AIMS Mathematics, 2024, 9(10): 26283-26306. doi: 10.3934/math.20241281 |
[9] | Qinghui Liu, Xin Zhang . Chaos detection in predator-prey dynamics with delayed interactions and Ivlev-type functional response. AIMS Mathematics, 2024, 9(9): 24555-24575. doi: 10.3934/math.20241196 |
[10] | Aeshah A. Raezah, Jahangir Chowdhury, Fahad Al Basir . Global stability of the interior equilibrium and the stability of Hopf bifurcating limit cycle in a model for crop pest control. AIMS Mathematics, 2024, 9(9): 24229-24246. doi: 10.3934/math.20241179 |
In this study, we introduce a novel reaction-diffusion epidemic model to analyze the transmission dynamics of the hepatitis B virus (HBV). The model captured the interactions between five population groups: Susceptible individuals, those in the latent stage, acutely infected individuals, chronically infected individuals, and those who have recovered, while considering the spatial movement of these groups. Chronic HBV infection contributes to severe liver diseases such as cirrhosis and hepatocellular carcinoma. It is also a major cause of long-term disability due to complications that impair daily functioning. The stability conditions for the model were derived, and the basic reproductive number, R0, was calculated using the next-generation matrix approach. Numerical simulations were performed using the Crank-Nicolson operator splitting method and the Unconditionally Positivity Preserving technique to solve the model under scenarios with and without diffusion. The stability of the endemic equilibrium point was analyzed comprehensively. Detailed simulation results are presented, highlighting a comparative analysis of the numerical findings in cases where exact solutions were unavailable. The reliability of the numerical results was validated by their alignment with theoretical expectations.
In biology, discrete models of interspecific relationships in populations without generation-level overlap are more generalizable than continuous ones, which has attracted many scholars in recent years (see [1,2,3,4,5,6,7,8,9,10,11,12]). Obviously, all these works indicate that discrete systems have more complex dynamic behavior. The predator-prey model is a class of classical biomathematical model that has been studied by different scholars in terms of the evolutionary patterns of populations over time [13,14] and the effects of different functional responses on the stability of populations [15,16].
For the predator-prey system, external factors such as food supply, climate change and population migration can affect predation and population reproduction [17,18]. However, the effects of changes in predators themselves are often overlooked, such as predator fear [19]. Frightened predators tend to eat less, which leads to reduced birth rates and increased mortality [19]. Therefore, predator fear, which cannot be quantitatively described, is more difficult to study.
Sasmal [20] studied a predator-prey model with Allee effect and reduced reproduction of prey due to cost of fear. They discussed the effects of time scale separation between prey and predator.
{dudt=ru(1−uk)(u−θ)11+fv−auv(0<θ<k)dvdt=aαuv−mv | (1.1) |
where u and v are the prey and predator population densities, respectively. r is the maximum growth rate of the population, k is the carrying capacity, and θ represents the Allee threshold (0<θ<k), below which the population becomes extinct. f refers to the level of fear, which is due to the anti-predator response of prey. a is the predation rate, α is the conversion efficiency of predator by consuming prey, and m represents the predator's natural mortality rate.
To simplify parameters, define N=uk, P=arkv, ϵ=aαr, θ=θk, m=maαk, f=rkfa, t=aαkt. Then, the system is given by
{dNdt=1ϵ[N(1−N)(N−θ)11+fP−NP]dPdt=NP−mP |
At present, there are some studies on predator-prey systems with Flip bifurcation and Hopf bifurcation [21] in continuous models, where, however, the chaotic properties have not been mentioned. For example, Khan studied a discrete-time Nicholson-Bailey host-parasitoid model and obtained the local dynamics and supercritical Neimark-Sacker bifurcation in 2017 [22]. In 2019, Li et al. investigated the discrete time predator-prey model undergoing Flip and Neimark Sacker bifurcation using the center manifold theorem and bifurcation theory [23]. In 2022, Yu et al. gave a description of the bifurcations in theory for a symmetrically coupled period-doubling system, including the Transcritical bifurcation, Pitchfork bifurcation, Flip bifurcation and Neimark-Sacker bifurcation [24]. In 2022, Chen et al. studied a discrete predator-prey system with Allee effect, which undergoes Fold bifurcation and Flip bifurcation. They obtained cascades of period-doubling bifurcation in orbits of period-2, 4, 8 and chaotic properties [25].
Based on the above analysis and discussion, we obtain the following discrete-time model:
{Nn+1=Nn+h{1ϵ[Nn(1−Nn)(Nn−θ)11+fPn−NnPn]}Pn+1=Pn+h[NnPn−mPn] | (1.2) |
where h>0 refers to the step length of (1.2), 0<θ<1.
In this paper, we study the existence and stability of four equilibria of model (1.2) and focus on local dynamics of the unique positive equilibrium point in Section 2. In Sections 3 and 4, we study Flip bifurcation and Hopf bifurcation when bifurcation parameter h varies in a small neighborhood of the unique positive equilibrium point. In Section 5, we study chaos scenarios of Flip bifurcation. Meanwhile, some numerical simulations about bifurcations are given by maximum Lyapunov exponent and phase diagrams to verify our results. Finally, brief conclusions are given in Section 6.
Initially, the existence and stability of the equilibrium point of model (1.2) are analyzed.
By calculating model (1.2), obviously, the trivial equilibrium point E1=(0,0) and boundary equilibria E2=(1,0), E3=(θ,0) are obtained. Since the negative equilibrium point has no practical meaning in biology, the unique positive internal equilibrium point E4=(m,−1+√1+4f(1−m)(m−θ)2f) is chosen.
The Jacobi matrix of the linear system of (1.2) at any equilibrium point (N∗,P∗) is given by:
J=(1+hϵ[−3N2+2(1+θ)N−θ1+fP−P]hϵ[(N3−(1+θ)N2+θN)f(1+fP)2−N]hP1+hN−mh)|(N∗,P∗) |
Now, we give some dynamical properties of four equilibria.
Theorem 2.1. For the trivial equilibrium E1, with eigenvalues λ1=1−hθϵ and λ2=1−hm.
(i) E1 is a sink if 0<h<min{2ϵθ,2m}, with eigenvalues |λ1|<1 and |λ2|<1.
(ii) E1 is a source if h>max{2ϵθ,2m}, with eigenvalues |λ1|>1 and |λ2|>1.
(iii) E1 is a saddle point if 2ϵθ<h<2m with eigenvalues |λ1|>1 and |λ2|<1, or 2m<h<2ϵθ with eigenvalues |λ1|<1 and |λ2|>1.
(iv) Flip bifurcation occurs at E1 if h=2ϵθ<2m, with eigenvalues λ1=−1 and |λ2|<1, or h=2m<2ϵθ, with eigenvalues λ2=−1 and |λ1|<1.
Theorem 2.2. For the boundary equilibrium E2, with eigenvalues λ1=1+hϵ(θ−1) and λ2=1+h(1−m).
(i) E2 is a sink if {m>10<h<min(2ϵ1−θ,2m−1), with eigenvalues |λ1|<1 and |λ2|<1.
(ii) E2 is a source if {m<1h>2ϵ1−θ or {m>1h>max{2ϵ1−θ,2m−1}, with eigenvalues |λ1|>1 and |λ2|>1.
(iii) E2 is a saddle point if {m>12ϵ1−θ<h<2m−1 or {m>12m−1<h<2ϵ1−θ with eigenvalues |λ1|>1 and |λ2|<1, or {m<1h<2ϵ1−θ with eigenvalues |λ1|<1 and |λ2|>1.
(iv) Flip bifurcation occurs at E2 if {m>1h=2ϵ1−θ<2m−1 with eigenvalues λ1=−1 and |λ2|<1, or h=2m−1<2ϵ1−θ with eigenvalues λ2=−1 and |λ1|<1.
(v) Transcritical bifurcation occurs at E2 if {m=1h<2ϵ1−θ, with eigenvalues λ2=1 and |λ1|<1.
Theorem 2.3. For the equilibrium E3, with eigenvalues λ1=1+hϵθ(1−θ) and λ2=1+h(θ−m).
(i) E3 is a source if θ>m or {θ<mh>2m−θ, with eigenvalues |λ1|>1 and |λ2|>1.
(ii) E3 is a saddle point if {θ<m0<h<2m−θ, with eigenvalues |λ1|>1 and |λ2|<1.
There is no situation as |λ1|<1, i.e., stability does not exist.
Theorem 2.4. For the internal equilibrium E4,
(i) E4 is a sink if
{1+hϵ[m(1+θ−2m)1+√1+4f(1−m)(m−θ)]+h2ϵ[m(1−m)(m−θ)√1+4f(1−m)(m−θ)[1+√1+4f(1−m)(m−θ)]2]>0m(1−m)(m−θ)√1+4f(1−m)(m−θ)>01+θ−2m1+√1+4f(1−m)(m−θ)+h[2(1−m)(m−θ)√1+4f(1−m)(m−θ)[1+√1+4f(1−m)(m−θ)]2]<0 |
(ii) Transcritical bifurcation occurs at E4 if
{m=1 or m=θ−2ϵ<h[2m(1+θ−2m)1+√1+4f(1−m)(m−θ)]<0. |
(iii) Flip bifurcation occurs at E4 if
{1+hϵ[m(1+θ−2m)1+√1+4f(1−m)(m−θ)]+h2ϵ[m(1−m)(m−θ)√1+4f(1−m)(m−θ)[1+√1+4f(1−m)(m−θ)]2]=0−4<hϵ[m(1+θ−2m)1+√1+4f(1−m)(m−θ)]<−2. |
(iv) Hopf bifurcation occurs at E4 if
{h=(2m−θ−1)[1+√1+4f(1−m)(m−θ)]2(1−m)(m−θ)√1+4f(1−m)(m−θ)−4<hϵ[m(1+θ−2m)1+√1+4f(1−m)(m−θ)]<0. |
Proof. The Jacobi matrix of (1.2) at point E4=(N0,P0) is
J∣(N0,P0)=(1+hϵ[2m(1+θ−2m)1+√1+4f(1−m)(m−θ)] hϵ[−2m+√1+4f(1−m)(m−θ)1+√1+4f(1−m)(m−θ)]h(−1+√1+4f(1−m)(m−θ))2f 1) | (2.1) |
where n=1+√1+4f(1−m)(m−θ).
Meanwhile, the characteristic polynomial of J∣(N0,P0) is given by:
f(λ)=λ2−(A+1)λ+(A+BC)=0 | (2.2) |
where
A=1+hϵ[2m(1+θ−2m)1+√1+4f(1−m)(m−θ)], |
B=hϵ[−2m+√1+4f(1−m)(m−θ)1+√1+4f(1−m)(m−θ)],C=h(−1+√1+4f(1−m)(m−θ))2f. |
Then, eigenvalues of J∣(N0,P0) are λ1=A+1+√Δ2 and λ2=A+1−√Δ2, where Δ=(A−1)2−4BC.
(i) (N0,P0) is a sink, if |λ1|<1 and |λ2|<1. From the Jury Criterion [26], we obtain {1+(A+1)+(A+BC)>01−(A+1)+(A+BC)>01−(A+BC)>0.
(ii) If Δ>0, λ1=1 and |λ2|<1 or λ2=1 and |λ1|<1, then we have {BC=0|A|<1.
(iii) If Δ>0, λ1=−1 and |λ2|<1 or λ2=−1 and |λ1|<1, then we have {2A+BC+2=0|A+2|<1.
(iv) If Δ<0, |λ1|=|λ2|=1, which is a pair of conjugate complex roots, then we have {A+BC=10<|A+1|<2.
When λ1 and λ2 is a pair of conjugate complex roots, we assume λ1=a+bi and λ2=a−bi (a2+b2=1 b≠0), i.e., 0<|a|<1. Since (λ−λ1)(λ−λ2)=λ2−(λ1+λ2)λ+λ1λ2=0, obviously we have λ1+λ2=A+1=2a and λ1λ2=A+BC=1.
All of the expressions A,B,C can be brought into equations mentioned above to obtain the corresponding conclusions.
In this section, we discuss how system (1.2) undergoes Flip bifurcation around its internal equilibrium E4=(m,−1+√1+4f(1−m)(m−θ)2f) when h is chosen as bifurcation parameter. The necessary conditions for Flip bifurcation to occur is given by the following curve:
U={(m,n,θ,f,ϵ)∈R5+:h=h∗=2f(2m−1−θ)+2√f2(1+θ−2m)2+1m(n−1)(n−2)ϵfnm(n−1)(n−2) ,|D|<1} |
where D=hϵ(m(1+θ−2m)1+√1+4f(1−m)(m−θ))+3 and n=1+√1+4f(1−m)(m−θ).
The Jacobi matrix of the linear system of (1.2) at the equilibrium point (N0,P0) is given by:
J∣(N0,P0)=(1+hϵ[2m(1+θ−2m)n] hϵ[−2m(n−1)n]h(n−2)2f 1) | (3.1) |
where n=1+√1+4f(1−m)(m−θ).
In order to obtain the bifurcation properties, we consider translations ¯Nn+1=Nn+1−N0, ¯Pn+1=Pn+1−P0 for shifting (N0,P0) to the origin. Then, the model (1.2) is given by
{¯Nn+1=¯Nn+h{1ϵ[(¯Nn+N0)(1−¯Nn−N0)(¯Nn+N0−θ)11+f(¯Pn+P0)−(¯Nn+N0)(¯Pn+P0)]}¯Pn+1=¯Pn+h[(¯Nn+N0)(¯Pn+P0)−m(¯Pn+P0)] | (3.2) |
Using Taylor expansion at the point E4(N0,P0) yields the following expression :
(¯Nn+1¯Pn+1)=J∣(N0,P0)(¯Nn¯Pn)+h(1ϵψ1(¯Nn,¯Pn)ψ2(¯Nn,¯Pn)) | (3.3) |
where
ψ1(¯Nn,¯Pn)=b1¯Nn2+b2¯Nn¯Pn+b3¯Pn2+b4¯Nn3+b5¯Nn2¯Pn+b6¯Nn¯Pn2+b7¯Pn3+O((|¯Nn|+|¯Pn|)4),
ψ2(¯Nn,¯Pn)=¯Nn¯Pn,
b1=−3N0+1+θ1+fP0, b2=[3N20−2(1+θ)N0+θ]f(1+fP0)2−1, b3=[−N30+(1+θ)N20−θN0]f2(1+fP0)3, b4=−11+fP0, b5=(3N0−1−θ)f(1+fP0)2,
b6=[3N20−2(1+θ)N0+θ]f2(1+fP0)3, b7=[N30−(1+θ)N20+θN0]f3(1+fP0)4.
From the characteristic polynomial (2.2), we obtain f(−1)=h2ϵ[m(n−1)(n−2)fn]+hϵ[4m(1+θ−2m)n]+4.
Since step size h>0, the bifurcation parameter is chosen as
h∗=2f(2m−1−θ)+2√f2(1+θ−2m)2+1m(n−1)(n−2)ϵfnm(n−1)(n−2) |
where parameter m satisfies 1+θ2<m<1.
Consider parameter h with a small perturbation δ, i.e., h=h∗+δ, |δ|≪1, and the system (3.3) becomes
(¯Nn+1¯Pn+1)=(1+(h∗+δ)ϵ[2m(1+θ−2m)n](h∗+δ)ϵ[−2m(n−1)n](h∗+δ)(n−2)2f1)(¯Nn¯Pn)+(h∗+δ)(1ϵψ1(¯Nn,¯Pn)ψ2(¯Nn,¯Pn)) | (3.4) |
The characteristic polynomial of (3.4) is given by
g(λ)=λ2−[2+h∗+δϵ[2m(1+θ−2m)n]]λ+1+h∗+δϵ[2m(1+θ−2m)n]+(h∗+δ)2ϵ[m(n−1)(n−2)fn] |
The transversal condition at (N0,P0) is
dg(λ)dδ∣λ=−1,δ=0=−4−2mh∗(1+θ−2m)ϵn |
If dg(λ)dδ∣λ=−1,δ=0≠0, then Flip bifurcation will occur at E4.
To facilitate discussion, define A=(1+h∗ϵ[2m(1+θ−2m)n]h∗ϵ[−2m(n−1)n]h∗(n−2)2f1).
If the eigenvalue of A goes for λ=−1, the corresponding eigenvector is given as:
T1=(h∗ϵ[−2m(n−1)n]−2−h∗ϵ[2m(1+θ−2m)n]) |
If the eigenvalue of A goes for λ=λ2, the corresponding eigenvector is given as:
T2=(h∗ϵ[−2m(n−1)n]λ2−1−h∗ϵ[2m(1+θ−2m)n]) |
Then, we have the invertible matrix
T=(T1,T2)=(h∗ϵ[−2m(n−1)n]h∗ϵ[−2m(n−1)n]−2−h∗ϵ[2m(1+θ−2m)n] λ2−1−h∗ϵ[2m(1+θ−2m)n]) |
Using translation (xnyn)=T−1(¯Nn¯Pn), system (3.4) turns into
(xn+1yn+1)=(−100λ2)(xnyn)+(f1(¯Nn,¯Pn,δ)g1(¯Nn,¯Pn,δ)) | (3.5) |
where
f1(¯Nn,¯Pn,δ)=a11¯Nnδ+a12¯Pnδ+b11¯Nn2+b12¯Nn¯Pn+b13¯Pn2+b14¯Nn3+b15¯Nn2¯Pn+b16¯Nn¯Pn2+b17¯Pn3+c11¯Nn2δ+c12¯Nn¯Pnδ+c13¯Pn2δ+c14¯Nn3δ+c15¯Nn2¯Pnδ+c16¯Nn¯Pn2δ+c17¯Pn3δ |
g1(¯Nn,¯Pn,δ)=a21¯Nnδ+a22¯Pnδ+b21¯Nn2+b22¯Nn¯Pn+b23¯Pn2+b24¯Nn3+b25¯Nn2¯Pn+b26¯Nn¯Pn2+b27¯Pn3+c21¯Nn2δ+c22¯Nn¯Pnδ+c23¯Pn2δ+c24¯Nn3δ+c25¯Nn2¯Pnδ+c26¯Nn¯Pn2δ+c27¯Pn3δ |
a11=1h∗(λ2+1)[2m−1−θn−1](λ2−1−h∗ϵ[2m(1+θ−2m)n])−n−22f(λ2+1)a12=1h∗(λ2+1)(λ2−1−h∗ϵ[2m(1+θ−2m)n])a21=1h∗(λ2+1)[2m−1−θn−1](2+h∗ϵ[2m(1+θ−2m)n])+n−22f(λ2+1)a22=1h∗(λ2+1)(2+h∗ϵ[2m(1+θ−2m)n])b11=1λ2+1[n−2m(n−1)](λ2−1−h∗ϵ[2m(1+θ−2m)n])b1b12=1λ2+1[n−2m(n−1)](λ2−1−h∗ϵ[2m(1+θ−2m)n])b2−h∗λ2+1b13=1λ2+1[n−2m(n−1)](λ2−1−h∗ϵ[2m(1+θ−2m)n])b3⋮b17=1λ2+1[n−2m(n−1)](λ2−1−h∗ϵ[2m(1+θ−2m)n])b7cij=h∗bij(i=1,2 ; j=1,2,⋯,7) |
Using translation (¯Nn+1¯Pn+1)=T(xn+1yn+1), system (3.5) becomes
(xn+1yn+1)=(−xnλ2yn)+(f2(xn,yn,δ)g2(xn,yn,δ)) | (3.6) |
where
f2(xn,yn,δ)=d11xnδ+d12ynδ+e11x2n+e12xnyn+e13y2n+e14x3n+e15x2nyn+e16xny2n+e17y3n+f11x2nδ+f12xnynδ+f13y2nδ+f14x3nδ+f15x2nynδ+f16xny2nδ+f17y3nδ |
g2(xn,yn,δ)=d21xnδ+d22ynδ+e21x2n+e22xnyn+e23y2n+e24x3n+e25x2nyn+e26xny2n+e27y3n+f21x2nδ+f22xnynδ+f23y2nδ+f24x3nδ+f25x2nynδ+f26xny2nδ+f27y3nδ |
k11=k12=h∗ϵ[−2m(n−1)n], k21=−2−h∗ϵ[2m(1+θ−2m)n], k22=λ2−1−h∗ϵ[2m(1+θ−2m)n]
d11=a11k11+a12k21,d12=a11k12+a12k22,e11=b11k211+b12k11k21+b13k221,e12=2b11k11k12+b12(k11k22+k12k21)+2b13k21k22,e13=b11k212+b12k12k22+b13k222,e14=b14k311+b15k211k21+b16k11k221+b17k321,e15=3b14k211k12+b15(k211k22+2k11k12k21)+b16(2k11k21k22+k12k221)+3b17k221k22,e16=3b14k11k212+b15(2k11k12k22+k212k21)+b16(k11k222+2k12k21k22)+3b17k21k222,e17=b14k312+b15k212k22+b16k12k222+b17k322,d21=a21k11+a22k21,d22=a21k12+a22k22,e21=b21k211+b22k11k21+b23k221,e22=2b21k11k12+b22(k11k22+k12k21)+2b23k21k22,e23=b21k212+b22k12k22+b23k222,e24=b24k311+b25k211k21+b26k11k221+b27k321,e25=3b24k211k12+b25(k211k22+2k11k12k21)+b26(2k11k21k22+k12k221)+3b27k221k22,e26=3b24k11k212+b25(2k11k12k22+k212k21)+b26(k11k222+2k12k21k22)+3b27k21k222,e27=b24k312+b25k212k22+b26k12k222+b27k322,fij=heij(i=1,2 ; j=1,2,⋯,7). |
Next, by using the center manifold theorem and normal form theories, the direction of Flip bifurcation at E4 is given.
α1=(∂2f∂˜xnδ+12∂f∂δ∂2f∂˜x2n)∣(0,0),α2=(16∂3f∂˜x3n+(12∂2f∂˜x2n)2)∣(0,0), |
where the coefficients of α1 and α2 are derived from (3.6).
Theorem 3.1. If α1≠0, α2≠0, then the system undergoes Flip bifurcation at the immobile point (N0,P0), and if α2>0(<0), then the two-cycle point is stable (unstable).
For sufficiently small neighborhoods of the parameter δ=0, there exists a central manifold at (0,0):
Wc(0,0)={(˜x,˜y):˜yn+1=m1˜x2n+1+m2˜xn+1δ} | (3.7) |
Substituting (3.7) into (3.6), the solution is given as
m1=e211−λ2=b21k211+b22k11k21+b23k2211−λ2,m2=−d211+λ2=−(a21k11+a22k21) 1+λ2 |
Restricting the system of equations on Wc(0,0), the results are as follows.
˜xn+1=−˜xn+l1˜x2n+l2˜xnδ+l3˜x2nδ+l4˜xnδ2+l5˜x3n+O((|˜xn|+|δ|)4) |
where l1=e11=b11k211+b12k11k21+b13k221, l2=d11=a11k11+a12k21, l3=d12m1+f11+e12m2, l4=d12m2, l5=e12m1+e14.
According to the normal form theories related to bifurcation analysis, we require the following quantity at (x,y,δ)=(0,0,0).
α1=(∂2f∂˜xnδ+12∂f∂δ∂2f∂˜x2n)∣(0,0)=l2+l3,α2=(16∂3f∂˜x3n+(12∂2f∂˜x2n)2)∣(0,0)=l5+l21 |
For numerical simulations, we choose the following parameters to prove our theoretical discussion. The unique positive equilibrium point (N0,P0)≈(0.89,0.0708765) of the model can be obtained by giving the parameters h=0.1, e=0.023933614, θ=0.2, f=1, m=0.89. By choosing the initial value of (0.9,0.07115), the result can be obtained by numerical simulation as Figure 1.
It is obvious from the diagrams that variables N and P appear as two-point solutions with a period for a given parameter condition and gradually stabilize near the equilibrium point, which verifies Flip bifurcation occurs at this point.
In this section, we still choose h as the bifurcation parameter to discuss Hopf bifurcation around its positive equilibrium (N0,P0). The necessary conditions for Hopf bifurcation to occur is given by the following curve:
S={(m,n,θ,f,ϵ)∈R5+:h=h∗1=(2m−1−θ)[1+√1+4f(1−m)(m−θ)]2(1−m)(m−θ)√1+4f(1−m)(m−θ), |E|<2} |
where E=hϵ(m(1+θ−2m)1+√1+4f(1−m)(m−θ))+2.
For emergence of Hopf bifurcation around positive equilibrium (N0,P0), two roots of the characteristic polynomial (2.2) must be complex conjugate with unit modulus. Therefore, it is easy to obtain the bifurcation parameter h∗1=(2m−θ−1)(1+√1+4f(1−m)(m−θ))2(1−m)(m−θ)√1+4f(1−m)(m−θ)>0, i.e., 0<m<θ or 1+θ2<m<1.
We still consider parameter h with a small perturbation δ, and then characteristic equation (2.2) can be rewritten as:
λ2+p(δ)λ+q(δ)=0, |
where
p(δ)=−[2+(h∗1+δ)ϵ[2m(1+θ−2m)n]], |
q(δ)=1+(h∗1+δ)ϵ[2m(1+θ−2m)n]+(h∗1+δ)2ϵ[m(n−1)(n−2)fn] |
The roots of the characteristic equation of J∣(N0,P0) are
λ1=p(δ)+i√4q(δ)−p(δ)22 , λ2=p(δ)−i√4q(δ)−p(δ)22 |
Also,
|λ1,2|=√q(δ),d|λ1,2|dδ∣δ=0=[1−4f(1+θ−2m)2ϵn(n−1)(n−2)+2(2m−1−θ)ϵmn]−12(1+θ−2m)(m−2)ϵn |
where d|λ1,2|dδ∣δ=0>0 if and only if (1+θ−2m)(m−2)ϵn>0.
If p(0)≠0,1, we have −4f(2m−1−θ)2ϵn(n−1)(n−2)≠2,3, which means λn1,2≠1 n=1,2,3,4.
The transversal condition at (N0,P0) is given by
d|λ1|2dδ∣δ=0=(λ1dλ2dδ+λ2dλ1dδ)∣δ=0=m(1+θ−2m)ϵn[3+2mh∗1(1+θ−2m)ϵn]+mh∗1(n−1)(n−2)ϵfn |
If 1+θ2<m<1, we have d|λ1|2dδ∣δ=0>0. Then, Hopf bifurcation will occur at (N0,P0).
Now, let
α=1+h∗1ϵ[m(1+θ−2m)n],β=h∗1√m(n−1)(n−2)ϵfn−m2(1+θ−2m)2ϵ2n2 |
The invertible matrix T is given by
T=( hϵ[−2m(n−1)n]0 α−1−hϵ[2m(1+θ−2m)n]−β ) |
Using the following translation
(¯Nn+1¯Pn+1)=T(¯xn+1¯yn+1) |
Then, the Eq (3.4) transforms to
(¯xn+1¯yn+1)=(α−ββα)(ˉxnˉyn)+(f(ˉxn,ˉyn)g(ˉxn,ˉyn)) |
where
f(ˉxn,ˉyn)=l11ˉx2n+l12ˉxnˉyn+l13ˉy2n+l14ˉx3n+l15ˉxn2ˉyn+l16ˉxnˉy2n+l17ˉy3n,g(ˉxn,ˉyn)=l21ˉx2n+l22ˉxnˉyn+l23ˉy2n+l24ˉx3n+l25ˉxn2ˉyn+l26ˉxnˉy2n+l27ˉy3n,l11=b1α3+b2α2β+αβ2+b3αβ2,l12=−2b1α2β+b2α3+βα2−b2αβ2−β3+2b3α2β,l13=b1αβ2−b2α2β−αβ2+b3α3,l14=b4α4+b5α3β+b6α2β2+b7αβ3,l15=−3b4α3β+b5α4−2b5α2β2+2b6α3β−b6αβ3+3b7α2β2,l16=3b4α2β2+b5αβ3−2b5α3β+b6α4−2b6α2β2+3b7α3β,l17=−b4αβ3+b5α2β2−b6α3β+b7α4,l21=b1α2β+b2αβ2−α2β+b3β3,l22=−2b1αβ2+b2α2β−b2β3−α3+αβ2+2b3αβ2,l23=b1β3−b2αβ2+α2β+b3α2β,l24=b4α3β+b5α2β2+b6αβ3+b7β4,l25=−3b4α2β2+b5α3β−2b5αβ3+2b6α2β2−b6β4+3b7αβ3,l26=3b4αβ3+b5β4−2b5α2β2+b6α3β−2b6αβ3+3b7α2β2,l27=−b4β4+b5αβ3−b6α2β2+b7α3β |
Next, by using the center manifold theorem and normal form theories, the direction of Hopf bifurcation at E4 is given. According to the normal form theories related to bifurcation analysis, we require the following quantity at (x,y,δ)=(0,0,0).
L=−Re[(1−2λ)ˉλ21−λξ11ξ20]−12|ξ11|2−|ξ02|2+Re(ˉλξ21) |
where
ξ20=18[fˉxˉx+fˉyˉy+2gˉxˉy+i(gˉxˉx−gˉyˉy−2fˉxˉy)],ξ11=14[fˉxˉx+fˉyˉy+i(gˉxˉx+gˉyˉy)],ξ02=18[fˉxˉx−fˉyˉy+2gˉxˉy+i(gˉxˉx−gˉxˉy+2fˉxˉy)],ξ21=116[fˉxˉxˉx+fˉxˉyˉy+gˉxˉxˉy+gˉyˉyˉy+i(gˉxˉxˉx+gˉxˉyˉy−fˉxˉxˉy−fˉyˉyˉy)],fˉxˉx=2l11 , fˉxˉy=l12 , fˉxˉxˉx=6l14 , fˉxˉxˉy=2l15 , fˉxˉyˉy=2l16 , fˉyˉy=2l13 , fˉyˉyˉy=6l17,gˉxˉx=2l21 , gˉxˉy=l22 , gˉxˉxˉx=6l24 , gˉxˉxˉy=2l25 , gˉxˉyˉy=2l26 , gˉyˉy=2l23 , gˉyˉyˉy=6l27 . |
Theorem 4.1. If the above conditions hold, and L≠0, the Hopf bifurcation occurs at the point (N0,P0). When δ>0, if L<0, then it attracts at that point; when δ<0, if L>0, then it repels at that point.
To verify whether the condition is correct, we select the following parameters for numerical simulations and obtain the following conclusions. By selecting the first set of parameters h≈0.062163, e=1, θ=0.1, f=1, m=0.554, the unique positive equilibrium point (N0,P0)≈(0.554,0.004932) of the model can be obtained. By choosing the initial value K1=(0.5,0.005), the following results can be obtained by numerical simulation.
Figure 2 reflects that variable N gradually tends to oscillate steadily at the equilibrium point N=0.554 with the increase of the number of iterations n, while variable P also gradually oscillates steadily with the increase of the number of iterations N, but the equilibrium point is attracted to P≈0.157.
By choosing the second set of parameters h≈1.120901, e=1, θ=0.61, f=1, m=0.823 the only internal equilibrium point (N0,P0)≈(0.823,0.020442) of the model can be obtained. Choosing the initial value K2=(0.8,0.02), the following results can be obtained by numerical simulation.
Figure 3 shows that as the number of iterations n increases, eventually, the variable P will also be attracted to stabilize at P≈0.035.
In this section, chaotic cases at bifurcating points are analyzed using numerical simulations. We give maximum Lyapunov exponents and phase diagrams for different perturbation parameters to prove our results. The chaos theory analysis of Flip bifurcation and Hopf bifurcation is given as follows.
Definition 5.1. [27] The formula for the maximum Lyapunov index is given by
λ=limn→∞1nn−1∑n=0ln|df(xn,μ)dx| |
Theorem 5.1. [27] If λ<0, the neighboring points eventually come together and merge into a single point, which corresponds to stable immobile points and periodic motion. If λ>0, the neighboring points eventually separate, which corresponds to local instability of the orbit generating chaotic situations.
In the following, we choose two different equilibria to consider the Flip bifurcation with chaotic cases. Initially, we select the parameters h=0.95, e≈0.1841359, θ=0.5, f=1, m=0.961, and obviously the model comes to
{Nn+1=Nn+0.95{10.1841359[Nn(1−Nn)(Nn−0.5)11+Pn−NnPn]}Pn+1=Pn+0.95[NnPn−0.961Pn] |
where the internal equilibrium point is (N1,P1)≈(0.961,0.01807).
After selecting the perturbation δ∈(0,0.2) and analyzing the trend of N with δ by numerical simulation, we obtain the chaotic bifurcating cases (see Figure 4).
Next, choosing the parameters h=0.95, e≈0.184136, θ=0.5, f=1, m=0.9601, we obtain the model
{Nn+1=Nn+0.95{10.184136[Nn(1−Nn)(Nn−0.5)11+Pn−NnPn]}Pn+1=Pn+0.95[NnPn−0.9601Pn] |
Obviously, the internal equilibrium point (N2,P2)≈(0.9601,0.0180734). We still choose the perturbation δ∈(0,0.2) to analyze the trend of N with δ. The chaotic bifurcating case is obtained (see Figure 5). By calculating the bifurcating diagrams and the maximum Lyapunov exponent for two bifurcating points with small differences, it can be shown that the small change of the initial value near the equilibrium point has a great influence on the stability of the initial state. Although they are different perturbed bifurcating cases, the two cases tend to go the same during the process of δ gradually increasing.
In the following, we choose two different equilibria to consider Hopf bifurcation with chaotic cases. By picking the first set of parameters h≈0.062163, e=1, θ=0.1, f=1, m=0.554, the unique internal equilibrium point (N3,P3)≈(0.554,0.004932) of the model can be obtained.
After choosing perturbation δ∈(0,0.5), we obtain phase diagrams (see Figure 6).
The analysis mentioned above shows that with the perturbation δ increasing, the asymptotic stability of variable N gradually becomes weaker, and the range of periodic solutions gradually becomes larger, but the convergence speed comes faster increases (see Figure 6).
By choosing the second set of parameters h≈1.120901, e=1, θ=0.61, f=1, m=0.823, we can obtain the unique internal equilibrium point (N4,P4)≈(0.823,0.020442) of the model.
After choosing perturbation δ∈(−1,1), we obtain phase diagrams (see Figure 7).
Our work deals with the study of local dynamical properties of a predator-prey model with discrete time (1.2), Flip bifurcation and Hopf bifurcation associated with the periodic solution, as well as their chaotic cases. We prove that the system (1.2) has four unique equilibria. In addition, their stability and instability conditions are given, which mark the final equilibrium state of both groups as fear, climate and other factors change. We focus on the unique positive equilibrium point E4=(m,−1+√1+4f(1−m)(m−θ)2f), with stability condition:
{1+hϵ[m(1+θ−2m)1+√1+4f(1−m)(m−θ)]+h2ϵ[m(1−m)(m−θ)√1+4f(1−m)(m−θ)[1+√1+4f(1−m)(m−θ)]2]>0 m(1−m)(m−θ)√1+4f(1−m)(m−θ)>01+θ−2m1+√1+4f(1−m)(m−θ)+h[2(1−m)(m−θ)√1+4f(1−m)(m−θ)[1+√1+4f(1−m)(m−θ)]2]<0 |
Significantly, after analyzing the formation conditions of Flip bifurcation and Hopf bifurcation at (N0,P0), we give their chaotic scenarios from both theoretical and numerical simulations. Moreover, it is found that Flip bifurcation is more prone to chaos scenarios, and Hopf bifurcation is closely related to periodic solutions.
Biologically, the occurrence of Flip bifurcation implies the number of predators and prey will alternate around a value instead of converging to a fixed value, eventually. The corresponding chaotic scenario suggests that small perturbations in the variable parameters will eventually have a significant impact on the population, ultimately leading to chaos. The appearance of Hopf bifurcation points indicates that the equilibrium point of the system loses its attraction and eventually produces a closed orbit, which implies the existence of periodic oscillations of predators and prey. Therefore, we can precisely change the biological density of predators and prey to achieve the desired goal by regulating the number of bifurcation parameters h, for the fact that the chaotic scenario does not exist. All the conclusions illustrate the effect of fear on populations. Finally, the accuracy of the theory is demonstrated using the maximum Lyapunov exponent and phase diagrams.
In future work, the model can be investigated using different discrete methods. In addition, to obtain specific biological properties, new bifurcation parameters can be selected for the study to obtain the desired conclusions.
The authors declare that they have no competing interests.
[1] | WHO, Hepatitis B, The World Health Organisation. Available from: http://www.who.int/mediacentre/factsheets/fs204/en/ |
[2] | Canadian Centre for Occupational Health and Safety, Hepatitis B. Available from: http://www.ccohs.ca/oshanswers/diseases/hepatitis_b.html |
[3] |
A. Schweitzer, J. Horn, R. T. Mikolajczyk, G. Krause, J. J. Ott, Estimations of worldwide prevalence of chronic hepatitis B virus infection: A systematic review of data published between 1965 and 2013, The Lancet, 386 (2015), 1546–1555. https://doi.org/10.1016/S0140-6736(15)61412-X doi: 10.1016/S0140-6736(15)61412-X
![]() |
[4] |
G. F. Medley, N. A. Lindop, W. J. Edmunds, D. J. Nokes, Hepatitis-B virus endemicity: Heterogeneity, catastrophic dynamics and control, Nat. Med., 7 (2001), 619–624. https://doi.org/10.1038/87953 doi: 10.1038/87953
![]() |
[5] |
A. Khan, R. Zarin, G. Hussain, A. H. Usman, U. W. Humphries, J. F. Gomez-Aguilar, Modeling and sensitivity analysis of HBV epidemic model with convex incidence rate, Results Phys., 22 (2021), 103836. https://doi.org/10.1016/j.rinp.2021.103836 doi: 10.1016/j.rinp.2021.103836
![]() |
[6] |
R. Zarin, Modeling and numerical analysis of fractional order hepatitis B virus model with harmonic mean type incidence rate, Comput. Method Biomech Biomed, 26 (2023), 1018–1033. https://doi.org/10.1080/10255842.2022.2103371 doi: 10.1080/10255842.2022.2103371
![]() |
[7] |
J. Mann, M. Roberts, Modelling the epidemiology of hepatitis B in New Zealand, J. Theor. Biol., 269 (2011), 266–272. https://doi.org/10.1016/j.jtbi.2010.10.028 doi: 10.1016/j.jtbi.2010.10.028
![]() |
[8] | MMWR Recommendations and Reports, Hepatitis B virus: A comprehensive strategy for eliminating transmission in the United States through universal childhood vaccination. Recommendations of the Immunization Practices Advisory Committee (ACIP), 1991, 1–25. |
[9] |
M. K. Libbus, L. M. Phillips, Public health management of perinatal hepatitis B virus, Public Health Nurs., 26 (2009), 353–361. https://doi.org/10.1111/j.1525-1446.2009.00790.x doi: 10.1111/j.1525-1446.2009.00790.x
![]() |
[10] |
F. B. Hollinger, D. T. Lau, Hepatitis B: The pathway to recovery through treatment, Gastroenterol. Clin. N., 35 (2006), 425–461. https://doi.org/10.1016/j.gtc.2006.03.002 doi: 10.1016/j.gtc.2006.03.002
![]() |
[11] |
C. L. Lai, M. F. Yuen, The natural history and treatment of chronic hepatitis B: A critical evaluation of standard treatment criteria and end points, Ann. Intern. Med., 147 (2007), 58–61. https://doi.org/10.7326/0003-4819-147-1-200707030-00010 doi: 10.7326/0003-4819-147-1-200707030-00010
![]() |
[12] |
R. Zarin, A. Raouf, A. Khan, U. W. Humphries, Modeling hepatitis B infection dynamics with a novel mathematical model incorporating convex incidence rate and real data, Eur. Phys. J. Plus, 138 (2023), 1056. https://doi.org/10.1140/epjp/s13360-023-04642-6 doi: 10.1140/epjp/s13360-023-04642-6
![]() |
[13] |
R. Zarin, U. W. Humphries, T. Saleewong, Advanced mathematical modeling of hepatitis B transmission dynamics with and without diffusion effect using real data from Thailand, Eur. Phys. J. Plus, 139 (2024), 385. https://doi.org/10.1140/epjp/s13360-024-05154-7 doi: 10.1140/epjp/s13360-024-05154-7
![]() |
[14] | R. M. Anderson, R. M. May, Infectious disease of humans: Dynamics and control, Oxford: Oxford University Press, 1991. https://doi.org/10.1093/oso/9780198545996.001.0001 |
[15] |
S. Thornley, C. Bullen, M. Roberts, Hepatitis B in a high prevalence New Zealand population: A mathematical model applied to infection control policy, J. Theor. Biol., 254 (2008), 599–603. https://doi.org/10.1016/j.jtbi.2008.06.022 doi: 10.1016/j.jtbi.2008.06.022
![]() |
[16] |
S. Zhao, Z. Xu, Y. Lu, A mathematical model of hepatitis B virus transmission and its application for vaccination strategy in China, Int. J. Epidemiol., 29 (2000), 744–752. https://doi.org/10.1093/ije/29.4.744 doi: 10.1093/ije/29.4.744
![]() |
[17] |
K. Wang, W. Wang, S. Song, Dynamics of an HBV model with diffusion and delay, J. Theor. Biol., 253 (2008), 36–44. https://doi.org/10.1016/j.jtbi.2007.11.007 doi: 10.1016/j.jtbi.2007.11.007
![]() |
[18] |
S. Zhang, Y. Zhou, The analysis and application of an HBV model, Appl. Math. Model., 36 (2012), 1302–1312. https://doi.org/10.1016/j.apm.2011.07.087 doi: 10.1016/j.apm.2011.07.087
![]() |
[19] |
T. Khan, G. Zaman, M. I. Chohan, The transmission dynamic and optimal control of acute and chronic hepatitis B, J. Biol. Dyn., 11 (2016), 172–189. https://doi.org/10.1080/17513758.2016.1256441 doi: 10.1080/17513758.2016.1256441
![]() |
[20] |
G. P. Samanta, S. Sharma, Analysis of a delayed Chlamydia epidemic model with pulse vaccination, Appl. Math. Comput., 230 (2014), 555–569. https://doi.org/10.1016/j.amc.2013.12.123 doi: 10.1016/j.amc.2013.12.123
![]() |
[21] | T. Khan, G. Zaman, O. Algahtani, Transmission dynamic and vaccination of hepatitis B epidemic model, Wulfenia J., 22 (2015), 230–241. |
[22] |
J. Danane, K. Allali, Mathematical analysis and treatment for a delayed hepatitis B viral infection model with the adaptive immune response and DNA-containing capsids, High-Throughput, 7 (2018), 35. https://doi.org/10.3390/ht7040035 doi: 10.3390/ht7040035
![]() |
[23] |
A. A. Raezah, A. Raouf, R. Zarin, A. Khan, Exploring the effectiveness of control measures and long-term behavior in Hepatitis B: An analysis of an endemic model with horizontal and vertical transmission, Results Phys., 53 (2023), 106966. https://doi.org/10.1016/j.rinp.2023.106966 doi: 10.1016/j.rinp.2023.106966
![]() |
[24] |
R. Zarin, U. W. Humphries, Modeling the dynamics of COVID-19 Epidemic with a reaction-diffusion framework: A case study from Thailand, Eur. Phys. J. Plus, 139 (2024), 1076. https://doi.org/10.1140/epjp/s13360-024-05870-0 doi: 10.1140/epjp/s13360-024-05870-0
![]() |
[25] |
I. Zada, M. N. Jan, N. Ali, D. Alrowail, K. S. Nisar, G. Zaman, Mathematical analysis of hepatitis B epidemic model with optimal control, Adv. Differ. Equ., 2021 (2021), 451. https://doi.org/10.1186/s13662-021-03607-2 doi: 10.1186/s13662-021-03607-2
![]() |
[26] |
O. Diekmann, J. A. P. Heesterbeek, J. A. J. Metz, On the definition and the computation of the basic reproduction ratio R0 in models for infectious diseases in heterogeneous populations, J. Math. Biol., 28 (1990), 365–382. https://doi.org/10.1007/BF00178324 doi: 10.1007/BF00178324
![]() |
[27] |
P. van den Driessche, Reproduction numbers of infectious disease models, Infect. Dis. Model., 2 (2017), 288–303. https://doi.org/10.1016/j.idm.2017.06.002 doi: 10.1016/j.idm.2017.06.002
![]() |
[28] |
A. Chakrabrty, M. Singh, B. Lucy, P. Ridland, Predator-prey model with prey-taxis and diffusion, Math. Comput. Model., 46 (2007), 482–498. https://doi.org/10.1016/j.mcm.2006.10.010 doi: 10.1016/j.mcm.2006.10.010
![]() |
[29] |
N. Ahmed, S. S. Tahira, M. Rafiq, M. A. Rehman, M. Ali, M. O. Ahmad, Positivity preserving operator splitting nonstandard finite difference methods for SEIR reaction diffusion model, Open Math., 17 (2019), 313–330. https://doi.org/10.1515/math-2019-0027 doi: 10.1515/math-2019-0027
![]() |
[30] | R. E. Mickens, Nonstandard finite difference models of differential equations, World Scientific, 1993. https://doi.org/10.1142/2081 |
[31] |
C. Basdevant, M. Devile, P. Haldenwang, J. M. Lacroix, J. Ouazzan, R. Peyret, et al., Spectral and finite difference solutions of the Burgers equation, Comput. Fluids, 14 (1986), 23–41. https://doi.org/10.1016/0045-7930(86)90036-8 doi: 10.1016/0045-7930(86)90036-8
![]() |
[32] | D. Gottlieb, S. A. Orszag, Numerical analysis of spectral methods: Theory and applications, SIAM, 1977. |
[33] |
N. Ahmed, M. Rafiq, M. A. Rehman, M. S. Iqbal, M. Ali, Numerical modelling of three-dimensional Brusselator reaction diffusion system, AIP Adv., 9 (2019), 015205. https://doi.org/10.1063/1.5070093 doi: 10.1063/1.5070093
![]() |
[34] | N. Ahmed, M. Rafiq, M. A. Rehman, M. Ali, M. O. Ahmad, Numerical modeling of SEIR measles dynamics with diffusion, Commun. Math. Appl., 9 (2018), 315–326. |
1. | Xiaoming Su, Jiahui Wang, Adiya Bao, Stability analysis and chaos control in a discrete predator-prey system with Allee effect, fear effect, and refuge, 2024, 9, 2473-6988, 13462, 10.3934/math.2024656 | |
2. | Wei Li, Chunrui Zhang, Mi Wang, Marek Galewski, Analysis of the Dynamical Properties of Discrete Predator-Prey Systems with Fear Effects and Refuges, 2024, 2024, 1607-887X, 1, 10.1155/2024/9185585 | |
3. | Halima Benali, Karima Mokni, Hajar Mouhsine, Mohamed Ch-Chaoui, Unveiling Complexity: A Discrete-Time Prey–Predator Model with Immigration Effects, 2024, 2731-8095, 10.1007/s40995-024-01742-5 |