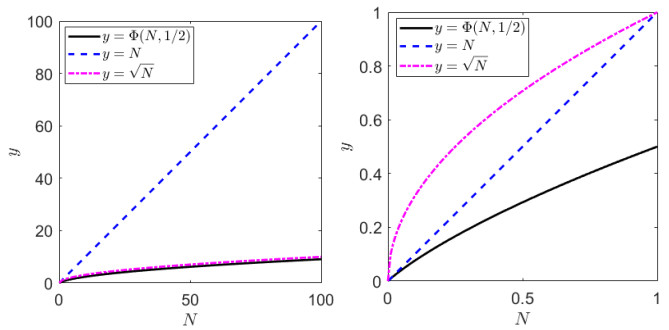
In this paper, focusing on individualistic generalist predators and prey living in herds which coexist in a common area, we propose a generalization of a previous model, namely, a two-population system that accounts for the prey response to predator attacks. In particular, we suggest a new prey-predator interaction term with a denominator of the Beddington-DeAngelis form and a function in the numerator that behaves as N for small values of N, and as Nα for large values of N, where N denotes the number of prey. We can take the savanna biome as a reference example, concentrating on large herbivores inhabiting it and some predators that feed on them. Only two conditionally stable equilibrium points have emerged from the model analysis: the predator-only equilibrium and the coexistence one. Transcritical bifurcations from the former to the latter type of equilibrium, as well as saddle-node bifurcations of the coexistence equilibrium have been identified numerically by using MATLAB. In addition, the model was found to exhibit bistability. Bistability is studied by using the MATLAB toolbox bSTAB, paying particular attention to the basin stability values. Comparison of coexistence equilibria with other prey-predator models in the literature essentially shows that, in this case, prey thrive in greater numbers and predators in smaller numbers. The population changes due to parameter variations were found to be significantly less pronounced.
Citation: Francesca Acotto, Ezio Venturino. How do predator interference, prey herding and their possible retaliation affect prey-predator coexistence?[J]. AIMS Mathematics, 2024, 9(7): 17122-17145. doi: 10.3934/math.2024831
[1] | Kwadwo Antwi-Fordjour, Rana D. Parshad, Hannah E. Thompson, Stephanie B. Westaway . Fear-driven extinction and (de)stabilization in a predator-prey model incorporating prey herd behavior and mutual interference. AIMS Mathematics, 2023, 8(2): 3353-3377. doi: 10.3934/math.2023173 |
[2] | Weili Kong, Yuanfu Shao . The effects of fear and delay on a predator-prey model with Crowley-Martin functional response and stage structure for predator. AIMS Mathematics, 2023, 8(12): 29260-29289. doi: 10.3934/math.20231498 |
[3] | Xuyang Cao, Qinglong Wang, Jie Liu . Hopf bifurcation in a predator-prey model under fuzzy parameters involving prey refuge and fear effects. AIMS Mathematics, 2024, 9(9): 23945-23970. doi: 10.3934/math.20241164 |
[4] | Binfeng Xie, Na Zhang . Influence of fear effect on a Holling type III prey-predator system with the prey refuge. AIMS Mathematics, 2022, 7(2): 1811-1830. doi: 10.3934/math.2022104 |
[5] | Chenxuan Nie, Dan Jin, Ruizhi Yang . Hopf bifurcation analysis in a delayed diffusive predator-prey system with nonlocal competition and generalist predator. AIMS Mathematics, 2022, 7(7): 13344-13360. doi: 10.3934/math.2022737 |
[6] | Reshma K P, Ankit Kumar . Stability and bifurcation in a predator-prey system with effect of fear and additional food. AIMS Mathematics, 2024, 9(2): 4211-4240. doi: 10.3934/math.2024208 |
[7] | Ahmad Suleman, Rizwan Ahmed, Fehaid Salem Alshammari, Nehad Ali Shah . Dynamic complexity of a slow-fast predator-prey model with herd behavior. AIMS Mathematics, 2023, 8(10): 24446-24472. doi: 10.3934/math.20231247 |
[8] | Ruizhi Yang, Dan Jin, Wenlong Wang . A diffusive predator-prey model with generalist predator and time delay. AIMS Mathematics, 2022, 7(3): 4574-4591. doi: 10.3934/math.2022255 |
[9] | Lingyu Liu, Alexander Wires . A free boundary problem with a Stefan condition for a ratio-dependent predator-prey model. AIMS Mathematics, 2021, 6(11): 12279-12297. doi: 10.3934/math.2021711 |
[10] | Teekam Singh, Ramu Dubey, Vishnu Narayan Mishra . Spatial dynamics of predator-prey system with hunting cooperation in predators and type I functional response. AIMS Mathematics, 2020, 5(1): 673-684. doi: 10.3934/math.2020045 |
In this paper, focusing on individualistic generalist predators and prey living in herds which coexist in a common area, we propose a generalization of a previous model, namely, a two-population system that accounts for the prey response to predator attacks. In particular, we suggest a new prey-predator interaction term with a denominator of the Beddington-DeAngelis form and a function in the numerator that behaves as N for small values of N, and as Nα for large values of N, where N denotes the number of prey. We can take the savanna biome as a reference example, concentrating on large herbivores inhabiting it and some predators that feed on them. Only two conditionally stable equilibrium points have emerged from the model analysis: the predator-only equilibrium and the coexistence one. Transcritical bifurcations from the former to the latter type of equilibrium, as well as saddle-node bifurcations of the coexistence equilibrium have been identified numerically by using MATLAB. In addition, the model was found to exhibit bistability. Bistability is studied by using the MATLAB toolbox bSTAB, paying particular attention to the basin stability values. Comparison of coexistence equilibria with other prey-predator models in the literature essentially shows that, in this case, prey thrive in greater numbers and predators in smaller numbers. The population changes due to parameter variations were found to be significantly less pronounced.
Different dynamics of prey-predator interaction have been considered over the years, starting with the classical Lotka-Volterra model. Of interest to us is the case in which prey with herd behavior and individualistic generalist predators coexist in a common area. For example, we can refer to a savanna, where large herbivores live alongside some predators that feed on them. A savanna is a mainly subtropical and tropical terrestrial biome that is mainly characterized by predominantly grassy vegetation with fairly widely spaced shrubs and trees; it is found in many transition zones between rainforest and desert or steppe regions in central Africa, Central and South America, India, Indochina, and Australia [1]. In this context, the choice to live in groups is one of the strategies that herbivores use to defend themselves against predator attacks and is therefore classified as a behavioral defense mechanism [2,3,4].
Predator hunting on prey herds can be modeled by observing that the individuals most likely to be affected by the predator attack are those on the herd perimeter. Therefore, denoting by N the number of prey per spatial unit as a function of time, the number of possible prey caught should be proportional to √N; see [5]; for more general case, it should be proportional to Nα, as described in [6], with the herd shape index being constrained as follows: 12≤α<1. Based on this assumption, several models that also incorporate the predator satiation phenomenon, [7,8,9,10,11], and the presence of disease, e.g., those detailed in[12,13,14], have been proposed. In addition, the prey response to predator attacks was considered in [15]. This is in agreement with the fact that, not only is aggregation advantageous for prey in terms of defense, the benefits tend to increase with growing herd size. Indeed, a large herd of herbivores can discourage hunting attempts by predators, who can become frightened, fearing that they in turn will be attacked in defense and injured, especially if the first ones have passive defense structural elements such as being large in size or having strong horns [16]. This prey-induced fear of predators is not to be confused with predator-induced fear of prey, in [17,18,19,20,21].
In this paper, we propose a generalization of the two-population model presented in [15]. In particular, still focusing on individualistic generalist predators and prey that live in herds, we propose a new predator-prey interaction term. This new term has a denominator of the Beddington-DeAngelis form, [22,23], which contains a binary-value parameter that allows to take into account the prey response to predator attacks or not, depending on the species considered. In addition, this term presents a function Φ(N,α) in the numerator, instead of Nα, that behaves as N for small values of N, and as Nα for large values of N. This function has been carefully constructed in such a way as to prevent singularity problems, similar to what was done in [24,25].
The article is organized as follows. The next section contains the details of the new model and its analytical study. In particular, the possible equilibrium points are derived with their feasibility and local stability conditions. In Section 3, on the other hand, the possible equilibrium bifurcations and the bistability of the model are numerically analyzed. We used MATLAB to identify the transcritical bifurcations from the predator-only equilibrium to the coexistence equilibrium and saddle-node bifurcations of the coexistence equilibrium. In addition, by using the MATLAB toolbox bSTAB, introduced in [26], bistability was identified and analyzed, as presented here with particular emphasis on the basin stability values; see [27,28] for further details. The paper ends with a discussion in which the new system is compared with some models in the literature. Of particular importance are Figures 10, 11, 12, and 13 which compare the population values at coexistence in the N-P plane all of these models.
We propose here a simple model, particularly, a generalization of the two-population system presented in [15], to describe the interaction dynamics of generalist predators, which behave individualistically, and prey herds. In this case, prey may or may not be able to respond to predator attacks. This depends on the particular prey species that is being considered.
Herd behavior is generally represented by a power function, as described in [5,6], that can possibly be modified to account for the predator satiation phenomenon. This function can be modeled, as in [15], by the classical Holling type Ⅱ (HTⅡ) functional response [30], with the prey power function modified in the denominator to account for the prey response to predator attacks.
Here, at first, we choose to generalize this fraction by replacing the prey power function in the numerator with a function Φ(N,α), with 12≤α<1, such that
Φ(N,α)≈N, when N→0, |
and
Φ(N,α)≈Nα, when N→+∞. |
Specifically, the function we select is given by
Φ(N,α)=N1+N1−α. | (2.1) |
Since Φ(0,α)=0 and
∂Φ(N,α)∂N=1+αN1−α(1+N1−α)2>0, |
Φ(N,α) is a nonnegative increasing function. Its behavior with α=12 is shown in Figure 1. Using the function Φ(N,α) instead of Nα presents advantages from a mathematical point of view because it avoids the singularity problems associated with Nα. These problems incur in the study of local stability as a result of evaluating the Jacobian at equilibria under the condition that the first component is equal to zero. Moreover, from a modeling point of view, this function, Φ(N,α), better describes the real situation than does Nα. Indeed, for large values of N, it behaves as Nα. For small values of N, Φ(N,α) behaves like N, which is in agreement with the fact that as the members of a herd decrease, they each tend to interact individually with the predator population. The function proposed here is not the only function that exhibits the desired features; see [24,25], in which similar considerations for α=12 are introduced.
Secondly, we chose to use a fraction denominator of the Beddington-DeAngelis form; see [22,23]. In addition, it contains a binary-valued parameter q which, for q=1, may account, or, for q=0, may not account for prey response to predator attacks.
In summary, the model equations we propose are as follows:
dNdt=rN(1−NK)−aΦ(N,α)P1+bNα+q+cP, | (2.2) |
dPdt=sP(1−PH)+eaΦ(N,α)P1+bNα+q+cP, | (2.3) |
where N and P respectively represent prey and predators, with the function Φ(N,α) as defined in (2.1) and the parameter restrictions as shown below:
12≤α<1,0<e≤1 and q∈{0,1}. | (2.4) |
In this system, the predator's individualistic behavior is represented by the classical unit exponent in the numerator and the denominator of the fractional interaction terms. Furthermore, it is assumed that prey grow logistically with a reproduction rate r and carrying capacity K, while predators behave similarly, with a growth rate s and carrying capacity H. In particular, the logistic growth in (2.3) is due to the assumption that the predators are generalists. Hence, they have additional available resources that are not explicitly modeled. The remaining parameters indicate the predation rate a, the assimilation coefficient e, the herd shape index α, the prey defense parameter q, and the Beddington-DeAngelis coefficients for prey and predators, b and c, respectively. Table 1 summarizes the model parameters. We have assumed the restrictions stated in (2.4) and that all other parameters are positive.
Parameters | Descriptions | Dimensions |
a | predation rate | 1[t] |
b | prey Beddington–DeAngelis coefficient | - |
c | predator Beddington–DeAngelis coefficient | - |
e∈(0,1] | assimilation coefficient | - |
r | prey growth rate | 1[t] |
s | predator growth rate | 1[t] |
K | prey carrying capacity | - |
H | predator carrying capacity | - |
α∈[12,1) | herd shape index | - |
q∈{0,1} | prey defense parameter | - |
Note that, if q=0 as the number of prey increases, keeping fixed the number of predators P, the second term of (2.2) tends to −ab−1P, and that of (2.3) tends to eab−1P. Instead, if q=1, both terms in (2.2) and (2.3) go to zero. This follows from the fact that, if P is fixed, the function
g(N,P)=Φ(N,α)P1+bNα+q+cP |
has the following limit:
limN→+∞g(N,P)=limN→+∞PNq(b+cPNα+q)={b−1P, if q=00, if q=1. |
On the other hand, if the numbers of prey and predators increase simultaneously, g(N,P) diverges positively if q=0, and it tends to b−1 if q=1. The behavior of the function g(N,P), for q=0 and q=1, is shown in Figure 2, for fixed parameter values α=12 and b=c=1. Thus, if q=1, the model accounts for the prey response to predator attacks since, as the number of individuals in the prey herd increases, predation decreases, whereas, if q=0, this is not the case.
By solving the system of equilibrium equations obtained by setting to zero the right-hand sides of (2.2) and (2.3), three unconditionally feasible equilibria can be immediately identified. These are the origin, E0=(0,0), and two equilibria with only one vanishing population, i.e., EN=(K,0) and EP=(0,H).
The process of analytically determining the coexistence equilibrium of the form ENP=(N∗,P∗), with N∗>0 and P∗>0, as based on the equilibrium equations, turns out to be less straightforward and far more difficult. This amounts to solving a highly nonlinear algebraic system. Thus, we can only numerically determine the explicit coexistence equilibrium coordinates. However, we can also rely on a graphic interpretation that will provide conditions for feasibility. The general idea is to interpret the two equilibrium equations as curves in the phase plane and look for sufficient conditions that ensure their possible intersections, which represent the coexistence equilibria. We now proceed to detail the construction of these curves, which, in what follows, will be studied analytically to determine when they may have intersection points in the first quadrant.
By summing the two equilibrium equations, after multiplying the first equation by e, we obtain
Γ(N,P)=erKN2+sHP2−erN−sP=0, | (2.5) |
which is a conic section. Moreover, from the first equilibrium equation, it is possible to explicitly get P as a function of N, which is P=ψ(N), with
P=ψ(N)=rN(K−N)(1+bNα+q)aKΦ(N,α)−crN(K−N)=N(ψ)(N)D(ψ)(N). | (2.6) |
The first curve, Γ(N,P)=0, is given implicity, whereas the second one, P=ψ(N), turns out to be an explicit function. If intersections between Γ, from (2.5), and ψ, from (2.6), exist inside of the first quadrant of the N-P plane, these are coexistence equilibria of the model. We now seek to indentify these intersections or, at least, the conditions guaranteeing them, so as to ensure the feasibility of the coexistence point ENP.
Note that the choice to consider the conic Γ and the first nullcline, i.e, P=ψ(N), that is instead of the two nullclines, is dictated by the difficulty of the problem and simplifies the analysis. Indeed, both nullclines have highly nonlinear algebraic expressions. In the first nullcline, however, it is possible to obtain P as a function of N, which is not true for the second nullcline, nor can one find N as a function of P. Consequently, the second nullcline turns out to be particularly problematic. Determining its qualitative behavior analytically is very complicated. Moreover, numerically obtaining its graphical representation is also difficult. The MATLAB built-in functions for graphical representation of its implicit expression also fail in this case. For these reasons, we found that it is possible to get the simpler conic Γ from the sum of the two equilibrium equations and consider it in place of the second nullcline.
We begin by studying the conic Γ. To assess its nature, we need to determine its invariants.
Note that for each conic in non-homogeneous coordinates of the general form
C:a11x2+a22y2+2a12xy+2a13x+2a23y+a33=0, |
two relevant matrices can be considered: the matrix of coefficients, MC=(aij), which is a symmetric matrix of order 3, and the matrix of quadratic terms, which is the submatrix SC of MC that is obtained by deleting the third row and third column. See, for example, [31,32]. Further, det(MC) and det(SC) are respectively called cubic and quadratic invariants of C.
The two matrices associated with the conic (2.5) are given by
MΓ=[erK0−er20sH−s2−er2−s20]andSΓ=[erK00sH]. |
They have the following determinants, respectively:
det(MΓ)=−ers4(sK+erH)≠0anddet(SΓ)=ersKH>0. |
Consequently, using the classification scheme of conics in non-homogeneous coordinates [31,32], we can say that the conic is non-degenerate and it is an ellipse. The axes of this ellipse are the two perpendicular lines N=K2 and P=H2. Then, its center is C=(K2,H2) and its vertices are given by
V1,2=(K(er±√ΔN)2er,H2)andV3,4=(K2,H(s±√ΔP)2s), |
with
ΔN=er(er+sHK)>0andΔP=s(s+erKH)>0. |
Furthermore, the conic intersects the coordinate axes at the origin and two other points, i.e., W1=(K,0) and W2=(0,H). Note also that the vertices of Γ lie outside of the rectangle formed by the points W1 and W2, the origin, and (K,H).
As for the function P=ψ(N), note that it goes through W1, as does Γ. Now, ψ(N) is defined only for the values N>0 such that
Φ(N,α)≠craKN(K−N). | (2.7) |
Observe also that
limN→0+ψ(N)=ra−cr. |
Assume that (2.7) holds. In view of the fact that ψ(N) does not have zeros other than N=K, in the interval [0,K], it is positive only if
cr<a. | (2.8) |
Further, ψ(N) itself will intersect Γ(N,P), if at some point is lower than Γ(N,P) and at other points is greater. Since both vanish at N=K, it is enough to require that the height of ψ at the origin is above W2, i.e., the condition ψ(0)>H must be satisfied. Hence, the first set of sufficient conditions to ensure at least one coexistence equilibrium is defined by (2.8), together with
cr<a<cr+rH. | (2.9) |
If the above condition (2.9) is not satisfied, ψ is negative in [0,K] and no feasible EN,P exists.
We now look into the situation in which (2.7) fails to hold. In such case, ψ admits vertical asymptotes.
Note that, if they exist, they must lie to the left of W1, i.e., for 0<N<K. Indeed, Φ(N,α)>0 and the function ψ must be positive on the right-hand side of (2.7) to have an equality. In the same interval, we also have N(ψ)(N)>0. Moreover, since, for N>K, the numerator and denominator of ψ(N) have constant signs, N(ψ)(N)<0 and D(ψ)(N)>0, it follows that ψ(N)<0 for N>K, that is, it is negative to the right of W1, with
limN→+∞ψ(N)=−∞. |
In addition, we can observe that
ψ′(K)=−r(1+bKα+q+bKq+1+K1−α)aK<0. |
Let us consider separately the two functions in (2.7). The former is the increasing nonnegative function Φ(N,α), while the latter, i.e.,
θ(N)=craKN(K−N), |
is a concave parabola through the origin, and W1. Their graphs certainly intersect at the origin and possibly at other points within the first quadrant, which correspond to as many vertical asymptotes of ψ(N). Since
∂Φ(0,α)∂N=1andθ′(0)=cra, |
a sufficient condition to have at least one vertical asymptote of ψ(N) is the opposite one of (2.8), i.e.,
a<cr. | (2.10) |
Let us say that the vertical asymptote is located at some 0<N1<K. Since ψ′(K)<0, the function ψ in (N1,K] has a positive branch descending from +∞ at N=N1 down to zero at N=K. Considering the continuity of both ψ and Γ in (N1,K] and the fact that Γ(N1,α) is finite, there must be an intersection in the positive stripe (N1,K]×R+, ensuring a coexistence equilibrium ENP for the system.
We can elaborate more on this situation. No other intersection exists in [0,N1] in the case that (2.10) holds because ψ in such an interval is negative and it is negative at the origin. Indeed, we have
limN→N1−ψ(N)=−∞. |
If more asymptotes exist to the left of N1, for each of these asymptotes, N=Nk, we must have
limN→N±kψ(N)=−∞ |
because, once again, the function ψ does not have zeros in [0,K). Hence, no other feasible intersections can exist between ψ and Γ.
By combining the conditions (2.10) and (2.9), we can conclude that a sufficient condition for the existence of at least one coexistence equilibrium is given by
a<a∗=cr+rH, witha≠cr. | (2.11) |
We use the Jacobian of the system of equations (2.2) and (2.3) to study the local stability of its equilibria. This matrix is given by
J(N,P)=[r−2rKN−a∂D(N,P)∂N−a∂D(N,P)∂Pea∂D(N,P)∂Ns−2sHP+ea∂D(N,P)∂P], |
with
∂D(N,P)∂N=P((1+αN1−α)(1+bNα+q+cP)−b(α+q)Nα+q(1+N1−α))(1+N1−α)2(1+bNα+q+cP)2 |
and
∂D(N,P)∂P=N(1+bNα+q)(1+N1−α)(1+bNα+q+cP)2, |
where
D(N,P)=Φ(N,α)P1+bNα+q+cP. |
Evaluating J at the three equilibria with at least one nonvanishing population, we find that
J(0,0)=[r00s],J(0,H)=[r−aH1+cH0eaH1+cH−s],J(K,0)=[−r−aK(1+K1−α)(1+bKα+q)0s+eaK(1+K1−α)(1+bKα+q)]. |
The origin and EN are unconditionally unstable since both eigenvalues of J(0,0) are positive and the first and the second eigenvalues of J(K,0) are are respectively negative and positive. The second eigenvalue on the diagonal of the matrix J(0,H), instead, is always negative, while the first one is negative if and only if
a>a∗=cr+rH. | (2.12) |
The point EP is asymptotically stable if and only if this condition is verified.
Finally, to discuss the local stability of the coexistence equilibrium, whose coordinates are not known, we can calculate the trace and the determinant of the Jacobian. The trace is given by
trJ(N,P)=A(N,P)+B(N,P)−aN1(N,P) |
and the determinant is given by
detJ(N,P)=A(N,P)B(N,P)−aN2(N,P), |
with
N1(N,P)=∂D(N,P)∂N−e∂D(N,P)∂P,N2(N,P)=B(N,P)∂D(N,P)∂N−eA(N,P)∂D(N,P)∂P, |
A(N,P)=r(1−2KN) and B(N,P)=s(1−2HP). |
Then, using the Routh-Hurwitz criterion, we can say that the equilibria of the form ENP, when they are feasible, are asymptotically stable if and only if
A(N∗,P∗)+B(N∗,P∗)<aN1(N∗,P∗),A(N∗,P∗)B(N∗,P∗)>aN2(N∗,P∗). | (2.13) |
The fact that these two stability conditions can be simultaneously satisfied for a feasible coexistence equilibrium has been verified through numerical simulations. For instance, by setting the parameter values
a=0.8,b=0.3,c=0.5,e=0.6,r=0.4,s=0.3,K=40,H=50,α=0.5,q=1, | (2.14) |
we can obtain an asymptotically stable coexistence equilibrium point by applying the initial conditions N(0)=60 and P(0)=15; see the left panel of Figure 3.
Table 2 summarizes the identified information concerning the model equilibrium points with their feasibility and local stability conditions.
Equilibria | Feasibility | Local Stability |
E0=(0,0) | unconditionally feasible | unconditionally unstable |
EP=(0,H) | unconditionally feasible | asymptotically stable iff (2.12) |
EN=(K,0) | unconditionally feasible | unconditionally unstable |
ENP=(N∗,P∗) | feasible if (2.11) | asymptotically stable iff (2.13) |
From Table 2, the local stability condition (2.12) for the predator-only equilibrium and the feasibility condition (2.11) for coexistence are complementary. However, by performing numerical simulations, as the parameter a changes, transcritical bifurcations can be identified at a=a∗∗, with a∗∗≥a∗=cr+rH, whose value changes depending on the initial conditions. Sotomayor's theorem constitutes a useful tool to analytically prove the presence of transcritical, saddle-node, and pitchfork bifurcations, provided that the coordinates of one equilibrium point are explicitly known [33]. In addition, it is necessary to know the exact value of the threshold for the bifurcation parameter. Thus, in this case, since we cannot exactly identify the critical value a∗∗, this theorem cannot be used. Consequently, we can only perform numerical simulations to visualize such transcritical bifurcations and estimate the value of a∗∗. For example, in Figure 4, using the parameter values (2.14) and the initial conditions N(0)=P(0)=30, we observe a transcritical bifurcation from ENP to EP at a=a∗∗, with a∗∗≈1.08834>a∗=0.20800.
This is in agreement with the fact that the condition (2.11) is only sufficient for the feasibility of the coexistence point. Hence, ENP could be feasible even if (2.11) is not satisfied. Thus, there may exist regions in the parameter space for which the equilibria EP and ENP turn out to be simultaneously feasible and asymptotically stable. In these regions we have bistability. For instance, as illustrated in Figure 3, fixing the parameter values as detailed in (2.14), we can observe how the system can reach the two different equilibria under different initial conditions.
This bistability has been explored by using the MATLAB toolbox bSTAB, as introduced in [26]. In the square Q=[0,50]×[0,50], n=104 uniformly distributed initial conditions were considered together with the relative tolerance of the numerical integration scheme RelTol=10−6. The basins of stability of the two equilibria mentioned above are illustrated in the right panel of the top row of Figure 5, while the left panel shows the basin stability values. Refer to [26,27,28] for their definitions. In particular, the basin stability value of an attractor A in a D-dimensional state space is defined as follows:
SB(A)=∫κB(y)ρ(y)dy,y∈RD, |
where κ(y) is an indicator function
κ(y)={1,if y∈B(A)0,otherwise |
which determines whether y belongs to the attraction basin B(A) and ρ is a density distribution of states such that the system may be perturbed with ∫Rρ(y)dy=1. The MATLAB toolbox bSTAB was used to estimate the basin stability values by performing Monte Carlo sampling from the density distribution ρ. Specifically, the two basin stability values found were SB(EP)≃0.13 and SB(ENP)≃0.87. By increasing the side of the square Q, SB(EP) was found to decrease and, consequently, SB(ENP) increased, and vice versa.
In the bottom row of Figure 5, we can see how the values SB(EP) and SB(ENP) vary as the parameter a moves from 0.8 to 1.3. At first, we have that SB(EP)≠0 and SB(ENP)≠0 such that SB(EP)+SB(ENP)=1; in this case, there is bistability of EP and ENP. Then, we have that SB(EP)=1 and SB(ENP)=0; in this second case, only EP is feasible and asymptotically stable at the same time.
Figures 6 and 7 show how SB(EP) and SB(ENP) change as the c value varies. This parameter is of great interest since it controls the interference of predators. Recall that it appears in the denominator of the predator-prey interaction terms of the equations (2.2) and (2.3). To obtain the results shown in Figure 6, we did not consider the response of prey to predator attacks; hence, we set q=0. Alternatively, Figure 7 shows the results of considering the prey response, which means that we set q=1. Further, to see if there is a significant variation as the shape of the prey herd changes within the range of the shape index, we considered a low value, α=0.5, and a higher value, α=0.9, because technical constraints of bSTAB prevent to generate a figure for a continuous range of values of α. These variations appear to be most significant in the case in which there is no prey response to predator attacks. Indeed, in Figure 6, we see that, for the lowest value of α, the transition from having EP as the only attractor to having only ENP occurs earlier than in the case in which α=0.9. As can be seen in the right frame of the top row of Figure 6, for α=0.5, bistability occurs in the range of 0.95⪅c<1.4. As can be seen in the right frame of the bottom row of Figure 6, for α=0.9, either no bistability occurs, suggesting perhaps a transcritical bifurcation, or, there is a very tiny bistability region that is difficult to locate numerically given the very long processing time of bSTAB. Alternatively as shown in Figure 7, although different values of SB(EP) and SB(ENP) can be observed in the intermediate phase, as α varies, the transitions from only stability of EP to bistability, as well as from bistability to only stability of ENP, occur for very close values of c.
Finally, we can easily identify other bifurcations by examining the expressions (2.5) and (2.6). Indeed as e, s, and H vary, the graphical representation of P=ψ(N) remains fixed in the N-P plane while Γ(N,P)=0 moves; this is because only the expression of the ellipse contains these three parameters. Conversely, varying α, a, b, c, and q was found to keep the ellipse fixed and shift the graph of the function ψ(N), whose expression is the only one that contains these five parameters. In both cases, the intersections between the two curves in the first quadrant of the N-P plane can change, giving rise to bifurcations. For example, as shown in Figures 8 and 9, under the conditions of the fixed parameter values
a=8.5,b=3.5,c=3.3,e=0.7,r=1.2,s=0.1,K=6,H=5,α=0.7,q=1, | (3.1) |
we were able to observe saddle-node bifurcations of the coexistence equilibrium by varying e, s, and H in the first case and α, a, b, and c in the second one. Tipically Sotomayor's theorem is also employed to analytically prove the presence of saddle-node bifurcations. However, in this case, since we cannot exactly identify the threshold values of the bifurcation parameters, the theorem is of no use. Thus, we investigated the occurrence of such bifurcations only through numerical simulations.
This new model exhibits just two possible steady states, i.e., the coexistence and the prey-free equilibrium. They can be linked by a transcritical bifurcation, as shown in Figure 4.
Figures 8 and 9 show that, in the interior of the first quadrant, there are two, one, or no intersections among Γ(N,P)=0, from (2.5), and P=ψ(N), from (2.6). For no intersection, the equilibrium attained by the system is EP, i.e., the intersection of the conic section with the vertical axis. When there are two, these are coexistence equilibria, where the right one is stable and the left one is unstable, because it must separate the basins of attractions of EP and the stable branch of ENP. Indeed, the bistability situation (the right intersection of the conic Γ with P=ψ(N) and the intersection of the conic section with the vertical axis) translates into the fact that EP remains stable and, topologically, that the left of the two intersections of (2.5) with (2.6) must be a saddle, while the right one must be an attractor. When the two curves are tangent to each other, a saddle-node bifurcation occurs.
Note that, for an increasing hunting rate, the predators wipe out the prey and continue to thrive because they are not specialists on N. Furthermore, Figure 3 shows that different initial conditions lead to different system outcomes with the same choice of model parameters, i.e., a bistability situation. In Figure 5, we show the basin stability values. In particular, the bottom frame shows that, if a<ˆa, for a certain 1<ˆa<1.1, the size of the basin of attraction of the coexistence equilibrium is much larger than that of the prey-free equilibrium. For a>ˆa, no prey survive and the basin of attraction of the prey-free equilibrium is the whole domain. This means that, in this case, for a>ˆa, EP is a 100% attractor. For the results shown in Figures 6 and 7, on the other hand, we consider variations of the basin stability values for EP and ENP as the parameters controlling predator interference, prey herding shape, and prey retaliation change.
A direct analytical comparison with the two-population model in [15], of which the model proposed here is a generalization, is possible. The two systems have the same equilibrium points, i.e., the coexistence equilibrium with different components. However, for the model in [15], the unconditionally feasible equilibrium EP turns out to be always unstable. In that case, since coexistence is the only conditionally feasible and locally asymptotically stable equilibrium, there can be no transcritical bifurcations, but saddle-node bifurcations of the coexistence equilibrium have been numerically identified. A similar comparison, however, is not possible with many other previously mentioned prey-predator models, that have appeared in the literature because of different assumptions. In particular, here, we are considering generalist predators, while many of these models focus on specialist predators.
In order to make a direct comparison by focusing only on predation terms, we chose to rewrite the models chosen for comparison, by inserting logistic growth terms into both differential equations we assume generalist predators in all cases. The general reference form is given by
dNdt=rN(1−NK)−af(N,P), | (4.1) |
dPdt=sP(1−PH)+aef(N,P), | (4.2) |
where the function f(N,P) is characteristic of each model. In particular, we were interested in comparing coexistence equilibria. As in [15] we choose to illustrate the differences graphically. We work for simplicity with α=0.5. There would be no substantial differences with a generic herd shape index 0.5≤α<1. The four functions we consider concern predators that hunt prey that gather in herds, with or without predator satiation, predator interference and prey retaliation to predator attacks. These are respectively defined as follows:
f1(N,P)=√NP,f2(N,P)=√NP1+b√N,f3(N,P)=√NP1+bN,f4(N,P)=Φ(N,12)P1+bN12+q+cP. |
The first one of these, as introduced in [5], is the simplest possible function in the case of individualistic predators and grouped prey. The second one, which appears, for example, in [7,8,9,10,11,13,14], combines the HTⅡ functional response with prey herd behavior. The third one, proposed in [15], is a variant of the second function that takes into account the prey response to predator attacks. We observe that f1(N,P) is a special case of both f2(N,P) and f3(N,P) with b=0. Finally, the fourth function, which is the one proposed in this paper, applies a denominator of Beddington-DeAngelis type [22,23], and has the function Φ(N,α) in the numerator, is a generalization of the previous three. In particular, we are interested in both the case q=0, in which there is no response of prey to predator attacks, and the case q=1, in which this response is present.
Note that the comparison with models that apply
f5(N,P)=NP and f6(N,P)=NP1+bN |
instead of f(N,P) in the equations (4.1) and (4.2), i.e., models considering prey and predators both individualistic, particularly, the former with the classical quadratic or Holling type Ⅰ term and the latter with the Holling type Ⅱ functional response, has been already investigated. We refer the reader to the results in [15].
The graphical results obtained are shown in Figures 10, 11, 12, and 13. The parameters used were as follows:
a=0.01,b=0.05,c=0.1,e=0.6,r=0.5,s=0.8,H=80,K=100. | (4.3) |
To obtained the results shown in each subfigure, one of the parameters in (4.3) were varied within an interval, while all of the other ones remained fixed to see how the coordinates of the achieved coexistence equilibria change in the N-P plane. The chosen initial conditions were applied as follows:
N(0)=P(0)=50. | (4.4) |
The model proposed here, with or without prey response to predator attacks, shows that, at each coexistence equilibrium, prey thrive in greater numbers and predators in smaller numbers than in the other three models considered. In particular, it can be observed that, by fixing all parameter values, the number of prey increases and the number of predators decreases from the first to the fifth case, i.e., the coexistence equilibrium moves rightward and downward. Note that, although there are four functional forms fi(N,P), i=1,…,4, we split the analysis of f4 into two, obtaining five cases. The fourth case corresponds to taking the function f(N,P) in (4.1) and (4.2) equal to f4(N,P) with q=0, i.e., without prey response to predator attacks. The fifth case corresponds to considering f4(N,P) with q=1, indicating prey retaliation. This variation is more significant over the course of the first case to the third one and less so from the third case to the last one. The changes obtained for each model as the parameter values were varied also became significantly less pronounced over the course of the first to the fifth case, except for the variations of the parameters H and K. In addition, we observed that, as b and c varied, the coordinates of the achieved coexistence equilibrium did not change in all systems because not all functions fi(N,P), for i=1,2,3,4, contained them. In particular, f1(N,P) does not contain b and only f4(N,P) contains c. Consequently, in Figure 13, we did not observe a shifting of the coexistence equilibrium, as b changed, in the left panel, and a variation in the position of this equilibrium was observed only for the last two cases, as c value changed, in the right one.
The new model has the following characteristics: the equilibrium points that it admits are the origin, the predator-only equilibrium, the predator-free equilibrium, and two, one, or no coexistence points. The first three are unconditionally feasible, while coexistence is conditionally feasible. Moreover, only the equilibrium EP and the coexistence points can be locally asymptotically stable. Consequently, these equilibria are the only two steady states to which the system can converge, regardless of the parameter values and the initial conditions.
Transcritical bifurcations from the predator-only equilibrium to the coexistence point are possible. In addition, saddle-node bifurcations of coexistence have also been numerically identified. Furthermore, there are combinations of parameter values for which, simultaneously, EP and ENP are admissible and locally asymptotically stable. In these cases, by fixing certain parameter values, the equilibrium toward which the system converges depends only on the initial conditions.
Situations of bistability, or, more generally, of multistability, have already been identified in the literature for different predator-prey dynamics; some examples can be found in [19,34,35,36,37,38].
A direct analytical comparison with the two-population model in [15], of which this model is a generalization, has been done. Finally, the new model has been also compared graphically, in terms of coexistence equilibria, with several other prey-predator models that have appeared in the literature, considering generalist predators. The main evidence is that the new model always shows that prey and predators thrive in respectively larger and smaller numbers than in the other models considered for comparison. In particular, we have observed that by fixing all parameter values and refining the model (i.e., changing from the model that only takes into account grouped prey to the model that also takes into account the predator satiation, predator interference, and the possible prey response to predator attacks) the coexistence equilibrium moves rightward and downward. This is in agreement with the fact that the phenomenon of predator satiation and prey retaliation are advantageous for prey population growth and disadvantageous for predators. On the other hand, predator interference by itself should promote predator growth. However, in the new model, it is offset by the other two factors. Thus, the model seems to suggest that predator interference in the case of prey herds with or without response to predator attacks, does not significantly help the predator population to increase in size because the grouping of prey turns out to have a stronger influence.
Moreover, by combining the results of this study with those obtained in [15], we can generally remark prey that gather in herds suffer fewer losses from attacks of the predators than individualistic ones. This is also in agreement with the results of previous investigations, e.g., [38]. Similar results hold for herds of competing population. On the other hand, the advantages for symbiotic populations are reduced because only the outermost individuals can exploit the other populations for additional resources. A secondary but important result highlighted in [38] is the occurrence of tristability in the case of herd competition.
Overall, models with herds in which the response function takes different forms, among which the one presented in this paper is a further example, appear to exhibit novel, interesting features that are not observed with the classical quadratic population models and therefore should be given an appropriate amount of attention by the theoretical biologists, despite their simple conceptual formulations.
The authors declare that they have not used artificial intelligence tools in the creation of this article.
The research work has been partially supported by the project "Approximation methods and models for the life sciences" of the Department of Mathematics of the University of Turin. The authors are members of the INdAM research group GNCS and the Research ITalian network on Approximation (RITA).
Francesca Acotto: Conceptualization, Data curation, Formal Analysis, Funding acquisition, Investigation, Methodology, Resources, Software, Validation, Visualization, Writing – original draft, Writing – review & editing; Ezio Venturino: Conceptualization, Formal Analysis, Funding acquisition, Investigation, Methodology, Project administration, Resources, Supervision, Validation, Writing – original draft, Writing – review & editing. All authors have read and approved the final version of the manuscript for publication.
All authors declare no conflicts of interest in this paper.
[1] | L. B. Hutley, S. A. Setterfield, Savanna, Encyclopedia of Ecology, Academic Press, (2008), 3143–3154. https://doi.org/10.1016/B978-008045405-4.00358-X |
[2] |
S. L. Lima, Back to the basics of anti-predatory vigilance: the group-size effect, Anim. Behav., 49 (1995), 11–20. https://doi.org/10.1016/0003-3472(95)80149-9 doi: 10.1016/0003-3472(95)80149-9
![]() |
[3] |
G. Roberts, Why individual vigilance declines as group size increase, Anim. Behav., 51 (1996), 1077–1086. https://doi.org/10.1006/anbe.1996.0109 doi: 10.1006/anbe.1996.0109
![]() |
[4] | T. M. Caro, Antipredator defenses in birds and mammals, University of Chicago Press, 2005. |
[5] |
V. Ajraldi, M. Pittavino, E. Venturino, Modeling herd behavior in population systems, Nonlinear Anal. Real World Appl., 12 (2011), 2319–2338. https://doi.org/10.1016/j.nonrwa.2011.02.002 doi: 10.1016/j.nonrwa.2011.02.002
![]() |
[6] |
I. M. Bulai, E. Venturino, Shape effects on herd behavior in ecological interacting population models, Math. Comput., 141 (2017), 40–55. https://doi.org/10.1016/j.matcom.2017.04.009 doi: 10.1016/j.matcom.2017.04.009
![]() |
[7] | S. P. Bera, A. Maiti, G. Samanta, Modelling herd behavior of prey: analysis of a prey-predator model, WJMS, 11 (2015), 3–14. |
[8] |
C. Berardo, I. M. Bulai, E. Venturino, Interactions obtained from basic mechanistic principles: prey herds and predators, Mathematics, 9 (2021), 2555. https://doi.org/10.3390/math9202555 doi: 10.3390/math9202555
![]() |
[9] |
P. A. Braza, Predator–prey dynamics with square root functional responses, Nonlinear Anal. Real World Appl., 13 (2012), 1837–1843. https://doi.org/10.1016/j.nonrwa.2011.12.014 doi: 10.1016/j.nonrwa.2011.12.014
![]() |
[10] |
S. Djilali, C. Cattani, L. N. Guin, Delayed predator-prey model with prey social behavior, Eur. Phys. J. Plus, 136 (2021), 940. https://doi.org/10.1140/epjp/s13360-021-01940-9 doi: 10.1140/epjp/s13360-021-01940-9
![]() |
[11] |
J. Tan, W. Wang, J. Feng, Transient dynamics analysis of a predator-prey system with square root functional responses and random perturbation, Mathematics, 10 (2022), 4087. https://doi.org/10.3390/math10214087 doi: 10.3390/math10214087
![]() |
[12] |
S. Belvisi, E. Venturino, An ecoepidemic model with diseased predators and prey group defense, Simul. Model. Pract. Theory, 34 (2013), 144–155. https://doi.org/10.1016/j.simpat.2013.02.004 doi: 10.1016/j.simpat.2013.02.004
![]() |
[13] |
G. Gimmelli, B. W. Kooi, E. Venturino, Ecoepidemic models with prey group defense and feeding saturation, Ecol. Complex., 22 (2015), 50–58. https://doi.org/10.1016/j.ecocom.2015.02.004 doi: 10.1016/j.ecocom.2015.02.004
![]() |
[14] |
S. Saha, G. P. Samanta, Analysis of a predator-prey model with herd behavior and disease in prey incorporating prey refuge, Int. J. Biomath., 12 (2019), 1950007. https://doi.org/10.1142/S1793524519500074 doi: 10.1142/S1793524519500074
![]() |
[15] |
F. Acotto, E. Venturino, Modeling the herd prey response to individualistic predators attacks, Math. Meth. Appl. Sci., 46 (2023), 13436–13456. https://doi.org/10.1002/mma.9262 doi: 10.1002/mma.9262
![]() |
[16] |
S. C. Hayley, J. T. Craig, I. H. K. Graham, Prey morphology and predator sociality drive predator-prey preferences, J. Mammal., 97 (2016), 919–927. https://doi.org/10.1093/jmammal/gyw017 doi: 10.1093/jmammal/gyw017
![]() |
[17] |
M. Chen, Y. Takeuchi, J. F. Zhang, Dynamic complexity of a modified Leslie-Gower predator-prey system with fear effect, Commun. Nonlinear Sci. Numer. Simul., 119 (2023), 107109. https://doi.org/10.1016/j.cnsns.2023.107109 doi: 10.1016/j.cnsns.2023.107109
![]() |
[18] |
M. Das, G. P. Samanta, A delayed fractional order food chain model with fear effect and prey refuge, Math. Comput., 178 (2020), 218–245. https://doi.org/10.1016/j.matcom.2020.06.015 doi: 10.1016/j.matcom.2020.06.015
![]() |
[19] |
S. Garai, N. C. Pati, N. Pal, G. C. Layek, Organized periodic structures and coexistence of triple attractors in a predator-prey model with fear and refuge, Chaos Solit., 165 (2022), 112833. https://doi.org/10.1016/j.chaos.2022.112833 doi: 10.1016/j.chaos.2022.112833
![]() |
[20] |
S. Kim, K. Antwi-Fordjour, Prey group defense to predator aggregated induced fear, Eur. Phys. J. Plus, 137 (2022), 704. https://doi.org/10.1140/epjp/s13360-022-02926-x doi: 10.1140/epjp/s13360-022-02926-x
![]() |
[21] |
S. K. Sasmal, Y. Takeuchi, Dynamics of a predator-prey system with fear and group defense, J. Math. Anal. Appl., 481 (2020), 123471. https://doi.org/10.1016/j.jmaa.2019.123471 doi: 10.1016/j.jmaa.2019.123471
![]() |
[22] |
J. R. Beddington, Mutual interference between parasites or predators and its effect on searching efficiency, J. Anim. Ecol., 44 (1975), 331–340. https://doi.org/10.2307/3866 doi: 10.2307/3866
![]() |
[23] |
D. L. DeAngelis, R. A. Goldstein, R. V. O'Neill, A model for tropic interaction, Ecology, 56 (1975), 881–892. https://doi.org/10.2307/1936298 doi: 10.2307/1936298
![]() |
[24] | D. Borgogni, L. Losero, E. Venturino, A more realistic formulation of herd behavior for interacting populations, R.P. Mondaini (eds) Trends in Biomathematics: Modeling Cells, Flows, Epidemics, and the Environment, BIOMAT 2019 (2020), Springer, Cham., Chapter 2, 9–21. https://doi.org/10.1007/978-3-030-46306-9_2 |
[25] |
E. Venturino, Y. Caridi, V. Dos Anjos, G. D'Ancona, On some methodological issues in mathematical modeling of interacting populations, J. Biol. Syst., 31 (2023), 169–184. https://doi.org/10.1142/S0218339023500080 doi: 10.1142/S0218339023500080
![]() |
[26] |
M. Stender, N. Hoffmann, bSTAB: an open-source software for computing the basin stability of multi-stable dynamical systems, Nonlinear Dyn., 107 (2022), 1451–1468. https://doi.org/10.1007/s11071-021-06786-5 doi: 10.1007/s11071-021-06786-5
![]() |
[27] |
P. J. Menck, J. Heitzig, N. Marwan, J. Kurths, How basin stability complements the linear-stability paradigm, Nat. Phys., 9 (2013), 89–92. https://doi.org/10.1038/nphys2516 doi: 10.1038/nphys2516
![]() |
[28] |
P. J. Menck, J. Heitzig, J. Kurths, H. J. Schellnhuber, How dead ends undermine power grid stability, Nat. Commun., 5 (2014), 3969. https://doi.org/10.1038/ncomms4969 doi: 10.1038/ncomms4969
![]() |
[29] |
K. A. Johnson, R. S. Goody, The original Michaelis constant: translation of the 1913 Michaelis-Menten paper, Biochem., 50 (2011), 8264–8269. https://doi.org/10.1021/bi201284u doi: 10.1021/bi201284u
![]() |
[30] |
C. S. Holling, The functional response of predators to prey density and its role in mimicry and population regulation, Mem. Ent. Soc. Can., 97 (1965), 5–60. https://doi.org/10.4039/entm9745fv doi: 10.4039/entm9745fv
![]() |
[31] | B. Noble, Applied Linear Algebra, Englewood Cliffs: Prentice-Hall, 1969. |
[32] | R. Woods, Analytic Geometry, New York: Mac Millan, 1939. |
[33] | L. Perko, Differential Equations and Dynamical Systems, New York: Springer, 2001. https://doi.org/10.1007/978-1-4613-0003-8 |
[34] |
A. Erbach, F. Lutscher, G. Seo, Bistability and limit cycles in generalist predator-prey dynamics, Ecol. Complex., 14 (2013), 48–55. https://doi.org/10.1016/j.ecocom.2013.02.005 doi: 10.1016/j.ecocom.2013.02.005
![]() |
[35] |
S. Garai, S. Karmakar, S. Jafari, N. Pal, Coexistence of triple, quadruple attractors and Wada basin boundaries in a predator-prey model with additional food for predators, Commun. Nonlinear Sci. Numer. Simul., 121 (2023), 107208. https://doi.org/10.1016/j.cnsns.2023.107208 doi: 10.1016/j.cnsns.2023.107208
![]() |
[36] |
R. López-Ruiz, D. Fournier-Prunaret, Indirect Allee effect, bistability and chaotic oscillations in a predator-prey discrete model of logistic type, Chaos Soliton. Fract., 24 (2005), 85–101. https://doi.org/10.1016/j.chaos.2004.07.018 doi: 10.1016/j.chaos.2004.07.018
![]() |
[37] |
Rajni, B. Ghosh, Multistability, chaos and mean population density in a discrete-time predator-prey system, Chaos Soliton. Fract., 162 (2022), 112497. https://doi.org/10.1016/j.chaos.2022.112497 doi: 10.1016/j.chaos.2022.112497
![]() |
[38] |
D. Melchionda, E. Pastacaldi, C. Perri, M. Banerjee, E. Venturino, Social behavior-induced multistability in minimal competitive ecosystems, J. Theor. Biol., 439 (2018), 24–38. https://doi.org/10.1016/j.jtbi.2017.11.016 doi: 10.1016/j.jtbi.2017.11.016
![]() |
1. | Ezio Venturino, The usefulness of mathematics in agriculture, for the environment and in contrasting diseases: insights from a wide range of simple models, 2024, 15, 2038-0909, 27, 10.2478/caim-2024-0002 |
Parameters | Descriptions | Dimensions |
a | predation rate | 1[t] |
b | prey Beddington–DeAngelis coefficient | - |
c | predator Beddington–DeAngelis coefficient | - |
e∈(0,1] | assimilation coefficient | - |
r | prey growth rate | 1[t] |
s | predator growth rate | 1[t] |
K | prey carrying capacity | - |
H | predator carrying capacity | - |
α∈[12,1) | herd shape index | - |
q∈{0,1} | prey defense parameter | - |
Equilibria | Feasibility | Local Stability |
E0=(0,0) | unconditionally feasible | unconditionally unstable |
EP=(0,H) | unconditionally feasible | asymptotically stable iff (2.12) |
EN=(K,0) | unconditionally feasible | unconditionally unstable |
ENP=(N∗,P∗) | feasible if (2.11) | asymptotically stable iff (2.13) |
Parameters | Descriptions | Dimensions |
a | predation rate | 1[t] |
b | prey Beddington–DeAngelis coefficient | - |
c | predator Beddington–DeAngelis coefficient | - |
e∈(0,1] | assimilation coefficient | - |
r | prey growth rate | 1[t] |
s | predator growth rate | 1[t] |
K | prey carrying capacity | - |
H | predator carrying capacity | - |
α∈[12,1) | herd shape index | - |
q∈{0,1} | prey defense parameter | - |
Equilibria | Feasibility | Local Stability |
E0=(0,0) | unconditionally feasible | unconditionally unstable |
EP=(0,H) | unconditionally feasible | asymptotically stable iff (2.12) |
EN=(K,0) | unconditionally feasible | unconditionally unstable |
ENP=(N∗,P∗) | feasible if (2.11) | asymptotically stable iff (2.13) |