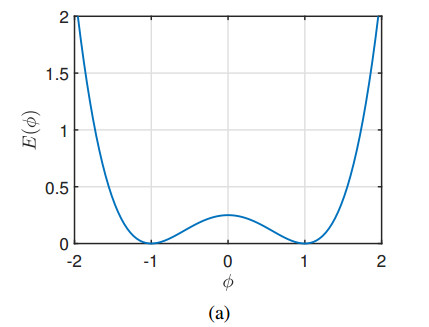
Electroencephalography (EEG) is essential for diagnosing neurological disorders such as epilepsy. This paper introduces a novel approach that employs the Allen-Cahn (AC) energy function for the extraction of nonlinear features. Drawing on the concept of multifractals, this method facilitates the acquisition of features across multi-scale. Features extracted by our method are combined with a support vector machine (SVM) to create the AC-SVM classifier. By incorporating additional measures such as Kolmogorov complexity, Shannon entropy, and Higuchi's Hurst exponent, we further developed the AC-MC-SVM classifier. Both classifiers demonstrate excellent performance in classifying epilepsy conditions. The AC-SVM classifier achieves 89.97% accuracy, 94.17% sensitivity, and 89.95% specificity, while the AC-MC-SVM reaches 97.19%, 97.96%, and 94.61%, respectively. Furthermore, our proposed method significantly reduces computational costs and demonstrates substantial potential as a tool for analyzing medical signals.
Citation: Ziling Lu, Jian Wang. A novel and efficient multi-scale feature extraction method for EEG classification[J]. AIMS Mathematics, 2024, 9(6): 16605-16622. doi: 10.3934/math.2024805
[1] | Nizar Alsharif, Mosleh Hmoud Al-Adhaileh, Mohammed Al-Yaari . Diagnosis of attention deficit hyperactivity disorder: A deep learning approach. AIMS Mathematics, 2024, 9(5): 10580-10608. doi: 10.3934/math.2024517 |
[2] | Youseef Alotaibi, Veera Ankalu. Vuyyuru . Electroencephalogram based face emotion recognition using multimodal fusion and 1-D convolution neural network (ID-CNN) classifier. AIMS Mathematics, 2023, 8(10): 22984-23002. doi: 10.3934/math.20231169 |
[3] | Hyun Geun Lee . A mass conservative and energy stable scheme for the conservative Allen–Cahn type Ohta–Kawasaki model for diblock copolymers. AIMS Mathematics, 2025, 10(3): 6719-6731. doi: 10.3934/math.2025307 |
[4] | Chaeyoung Lee, Seokjun Ham, Youngjin Hwang, Soobin Kwak, Junseok Kim . An explicit fourth-order accurate compact method for the Allen-Cahn equation. AIMS Mathematics, 2024, 9(1): 735-762. doi: 10.3934/math.2024038 |
[5] | Youngjin Hwang, Jyoti, Soobin Kwak, Hyundong Kim, Junseok Kim . An explicit numerical method for the conservative Allen–Cahn equation on a cubic surface. AIMS Mathematics, 2024, 9(12): 34447-34465. doi: 10.3934/math.20241641 |
[6] | Yuxin Luo, Yu Fang, Guofei Zeng, Yibin Lu, Li Du, Lisha Nie, Pu-Yeh Wu, Dechuan Zhang, Longling Fan . DAFNet: A dual attention-guided fuzzy network for cardiac MRI segmentation. AIMS Mathematics, 2024, 9(4): 8814-8833. doi: 10.3934/math.2024429 |
[7] | Tomoyuki Suzuki, Keisuke Takasao, Noriaki Yamazaki . New approximate method for the Allen–Cahn equation with double-obstacle constraint and stability criteria for numerical simulations. AIMS Mathematics, 2016, 1(3): 288-317. doi: 10.3934/Math.2016.3.288 |
[8] | Wei Xue, Pengcheng Wan, Qiao Li, Ping Zhong, Gaohang Yu, Tao Tao . An online conjugate gradient algorithm for large-scale data analysis in machine learning. AIMS Mathematics, 2021, 6(2): 1515-1537. doi: 10.3934/math.2021092 |
[9] | Yangfang Deng, Zhifeng Weng . Barycentric interpolation collocation method based on Crank-Nicolson scheme for the Allen-Cahn equation. AIMS Mathematics, 2021, 6(4): 3857-3873. doi: 10.3934/math.2021229 |
[10] | Yunan He, Jian Liu . Multi-scale Hochschild spectral analysis on graph data. AIMS Mathematics, 2025, 10(1): 1384-1406. doi: 10.3934/math.2025064 |
Electroencephalography (EEG) is essential for diagnosing neurological disorders such as epilepsy. This paper introduces a novel approach that employs the Allen-Cahn (AC) energy function for the extraction of nonlinear features. Drawing on the concept of multifractals, this method facilitates the acquisition of features across multi-scale. Features extracted by our method are combined with a support vector machine (SVM) to create the AC-SVM classifier. By incorporating additional measures such as Kolmogorov complexity, Shannon entropy, and Higuchi's Hurst exponent, we further developed the AC-MC-SVM classifier. Both classifiers demonstrate excellent performance in classifying epilepsy conditions. The AC-SVM classifier achieves 89.97% accuracy, 94.17% sensitivity, and 89.95% specificity, while the AC-MC-SVM reaches 97.19%, 97.96%, and 94.61%, respectively. Furthermore, our proposed method significantly reduces computational costs and demonstrates substantial potential as a tool for analyzing medical signals.
The electroencephalogram (EEG) stands as one of the most crucial non-invasive tools in neuroscience for imaging the brain, serving to measure its electrical activity [1]. Commonly employed for various purposes, EEG aids in the diagnosis and detection of signs indicating brain injuries [2,3], brain tumors [4,5], epilepsy, and related seizure types [6,7,8]. Furthermore, given the complexity of EEG data, it is challenging for physicians to make efficient and accurate judgments. Hence, a robust automatic classification method can aid physicians and mitigate the risk of misdiagnosis [9]. The World Health Organization released a report highlighting epilepsy as one of the most prevalent chronic neurological disorders globally, affecting approximately 50 million people worldwide [10]. The brain discharges abnormally during a seizure, resulting in differences in EEG signal presentation. In addition to this, recurrent and sudden seizures in epilepsy are very dangerous and can lead to life-threatening situations [11]. In addition to its pivotal role in medical diagnosis, EEG has emerged as an indispensable tool in the realm of brain-machine interface research and emotion recognition [12,13,14,15]. The rapid development of artificial intelligence combined with the advancement of medical device technology highlights the importance of using artificial intelligence for EEG signal classification. The analysis of voluminous EEG datasets to unearth novel features and patterns of cerebral activity is instrumental in unlocking latent knowledge. In recent epochs, a multitude of scholars have dedicated efforts towards the innovation of sophisticated classifiers for EEG signal processing. These endeavors can be broadly categorized into classifiers rooted in traditional machine learning techniques and those predicated on deep learning paradigms [16,17,18,19].
This section aims to meticulously review the literature to elucidate the research advancements in EEG signal classifiers, focusing on both traditional machine learning and deep learning approaches. Through this examination, we endeavor to highlight the distinct advantages and inherent challenges of these methodologies. The advancement of deep learning techniques has greatly propelled the field of EEG signal classification due to their remarkable capacity for automatic feature extraction and processing large-scale EEG datasets. Acharya et al. [20] pioneered the use of convolutional neural networks (CNN) for EEG classification for epilepsy diagnosis. Xin et al. [21] utilized multi-scale wavelet analysis for decomposing EEG signal into frequency components before classification. Author Roy employed CNN to extract discriminative features from multiple scales across several non-overlapping standardized frequency bands for the classification of motor imagery in the EEG [22]. Following this, the scholar seamlessly integrated an adaptive transfer learning model while synergistically fusing multi-scale features to craft a top-tier classifier. Dalin et al. [23] proposed a graph-based convolutional Recurrent Attention Model for motor imagery classification from EEG signal, aiming to directly extend pre-trained models to new users without subject-specific adaptation. In the realm of epilepsy diagnosis, certain researchers have innovatively merged brainwave signals with two-dimensional imagery. Dissanayake et al. [24] proposed a subject-independent seizure prediction model based on Geometric Deep Learning and discussed its potential contribution to epilepsy localization using scalp EEG.
Although deep learning techniques have made significant advancements in the field of EEG signal processing, some researchers still emphasize the potential advantages of traditional machine learning methods in data interpretation and explicit feature characterization of EEG signal. Therefore, these scholars have invested a considerable amount of research effort in the feature extraction of EEG signal to explore feature sets that can enhance the interpretability and accuracy of models. Yuan et al. [30] extracted approximate entropy, Hurst exponent, and scaling index obtained from Multifractal Detrended Fluctuation Analysis (MF-DFA) and classified them using an extreme learning machine with a satisfactory recognition accuracy of 96.5%. Zhang et al. [31] utilized common spatial patterns for feature extraction, followed by implementing a CNN to distinguish seizure periods and make predictions for epilepsy seizures. Tuncer [32] used the Substitution Box of the Hamsi Hash Function to generate multi-level features and combined iterative domain component analysis to establish an automatic EEG classifier. Sethy et al. [33] evaluated the classification performance of machine learning for EEG signal and concluded that the best classifier is a fine KNN. Varlı and Yılmaz [34] created a combined deep learning model that utilizes both time-frequency component images of EEG signal and raw EEG signal for automatic classification. To reduce dimensionality and enhance classification accuracy and generalization, many scholars have researched feature extraction [35,36].
Multifractals function as powerful tools for data analysis and pattern recognition across diverse fields, adept at capturing the multifractal structure of data and extracting valuable features to address complex and irregular problems. One specific realization of multifractals is multifractal detrended fluctuation analysis (MF-DFA), a proven effective technique for assessing multifractal scale in non-stationary data [37]. Researchers have successfully applied MF-DFA to analyze EEG signal with promising outcomes [38,39]. Zorick and Mandelkern [40] conducted an analysis of EEG in different sleep states, demonstrating that MF-DFA serves as a useful pattern classification technique capable of distinguishing various brain functional states. Additionally, Zhang et al. [41] constructed a classification model based on MF-DFA and SVM, utilizing a genetic algorithm to determine SVM parameters. They applied this model to the automatic detection of seizures. Furthermore, Wang et al. [42] integrated fractal features extracted by MF-DFA with features based on other indicators to develop a multi-index classifier.
Despite the availability of various classification models for EEG signal classification, many of them suffer from issues such as excessive complexity or low accuracy. Inspired by the work in [43], we observe a similarity between the energy dissipation curve during the phase separation process of the Alle-Cahn (AC) model and the Hurst exponent curve 2−H(2) calculated using MF-DFA. Therefore, we propose a classification framework that leverages the AC energy function. We compute energy features using the AC energy function and achieve multi-scale feature extraction by varying the exponent value in the double-well potential energy function. Then, we combine energy features extracted at multi-scale into a single vector, which is then applied to the SVM for classification. Finally, we compare the classifier proposed in this paper with the classifier based on the multifractal method, and find that our classifier shows better performance.
The contents of this paper are as follows: In Section 2, we describe the methodology. Section 3 presents the data information. Section 4 contains the computational experiments. In Section 5, we juxtapose the methodology with both conventional and state-of-the-art approaches in epilepsy automatic classification. The conclusion is put forward in Section 6.
Building upon the insights presented in the article by Wang et al. [43], it is observed that the energy dissipation curve, corresponding to the energy function in the AC model, closely aligns with the 2−H(2) curve in multifractal analysis. Here, 2−H(2) serves as a measure describing the fractal features at a specific scale. In the multifractal approach, the generalized Hurst exponent is extracted at multiple scales by varying the q value, and these features are amalgamated to form the feature vectors of the data. Following this conceptual framework, the current study initially computes the energy of the data using the AC function as the standard energy feature. Subsequently, multi-scale feature extraction is achieved by modifying the exponent of the double-well potential energy function. A novel feature vector is then constructed by amalgamating the energy features at different scales, effectively portraying the self-similarity and complexity of the data. Ultimately, this vector is utilized as input for classification in an SVM.
The AC model was first proposed by Allen and Cahn in 1979 [44]. This model was used to describe the process of phase separation and interface evolution in material phase transition, and it is one of the important models in the fields of solid-state physics and material science. However, in this paper, we just focus on AC energy function. This function is mainly used to describe the energy distribution of the phase field separation system. The AC energy function usually consists of two main terms, the gradient term and the free energy density term, and this function is shown in Eq (2.1):
ε(ϕ(x))=∫baE(ϕ(x))ϵ2+12(|∇ϕ(x)|)2dx. | (2.1) |
Among them, where [a,b]⊂R. ϕ(x) is the value of the sequence at point x. ∇ϕ is a gradient term, which represents the spatial variation of the phase field variable ϕ. ϵ is a positive parameter related to the interfacial transition thickness. E(ϕ(x))ϵ2 as an energy term in the energy function. Where E(ϕ(x)) represents a potential energy function, typically a smooth function of ϕ(x), used to describe the interaction potential energy. The double-well potential energy function is one of the most useful energy potentials in the phase field model [45]. Figure 1 shows the energy curve of the double-well potential energy function. When ϕ takes 1 or −1, it is the minimum energy state. In this paper, the function with a double-well potential form is selected,
E(ϕ)=14(ϕ2−1)2. | (2.2) |
Our novel model delineates multiple scales by introducing variability into the exponential value of the original double-well potential energy function, represented as a variable exponent denoted by M.
EM(ϕ)=14(ϕ2−1)M. | (2.3) |
In this model, the parameter M represents the concept of multi-scale analysis. Assume that energy features are extracted at n different scales. To clarify, we designate the values of M across n scales as {mj}, where j=1,2,…,n, corresponding to the j-th scale. Based on different datasets, adjusting the value of M to achieve the best performance is necessary. After numerous numerical experiments, we have observed that the choice of scale significantly impacts the quality of feature extraction. Typically, selecting n evenly spaced numbers within the range [10,15] or [1,3] yields satisfactory results. Therefore, the multi-scale double-well potential energy function is represented by Eq (2.3). Accordingly, we have refined Eq (2.1) to derive the multi-scale energy equation as follows:
εM(ϕ(x))=∫baEM(ϕ(x))ϵ2+12(|∇ϕ(x)|)2dx. | (2.4) |
In numerical calculations, we discretize the equation in one dimension. Assuming there are N EEG signal in a sample set, we denote ϕ(xi) as the EEG signal at the i−th position, where i=1,2,...N. Let ϕi=ϕ(xi). Subsequently, we discretize the gradient term in a central difference format. Then, the AC energy value of the EEG signal at the i−th position at scale M can be calculated as follows:
εM(ϕi)=EM(ϕi)ϵ2+12(ϕi+1−ϕi−1)24h2. | (2.5) |
The ultimate AC energy expression is shown in Eq (2.6). By means of this function, the energy features of the sample set across n different scales can be computed.
εM(ϕ(x))=n−1∑i=2εM(ϕi). | (2.6) |
SVM was originally conceived and developed by Vapnik and Cortes in the late 1960s and early 1970s [46]. SVM is a common tool for data classification and image classification [47,48]. When dealing with nonlinear multi-scale features for EEG classification, SVM can leverage kernel functions to map the data into a high-dimensional space. This transformation effectively converts the nonlinear problem into a linear one, ensuring the linear separability of the data in the high-dimensional space. Moreover, employing the kernel trick to compute kernel function values eliminates the necessity of explicitly calculating feature vectors and their corresponding inner products in the high-dimensional feature space. This strategic approach significantly mitigates computational complexity.
Drawing from the aforementioned considerations, this study opts for SVM, leveraging its performance advantages, to conduct binary classification on nonlinear EEG signal for the purpose of automated seizure detection and classification. Employing the Gaussian kernel function facilitates the mapping of data into an infinite-dimensional feature space, ensuring relative robustness while concurrently enhancing computational efficiency. First, build a training data set containing n samples with m features each and denote the dataset as (x1,y1),(x2,y2),...,(xn,yn), where xi⊂Rm is an m-dimensional feature vector. yi is the corresponding label, which takes the value of +1 or −1 to denote positive and negative categories, respectively. Define the decision function f(x) and denote it as:
f(x)=sign(w⋅K(xi,xj)+b), | (2.7) |
where w is a weight vector, K(xi,xj) is the kernel function, and b denotes the intercept. The Gaussian kernel function was chosen for this study in the following form:
K(xi,xj)=exp(−‖xi−xj‖22σ2). | (2.8) |
Let C be a regularization parameter that controls the trade-off between the interval and the classification error. ζi is a slack variable that is used to handle the sample points in the nonlinear case. The objective function of SVM can be expressed as:
minimize12(‖w‖)2+Cn∑i=1ζi, | (2.9) |
s.t.{yi(w⋅K(xi,xj)+b)≥1−ζi,ζi≥0,i=1,2,...,n. | (2.10) |
We obtained five sets of EEG signal related to epilepsy from the publicly available database at the University of Bonn, Germany. This database comprises EEG data from five healthy individuals and five epilepsy patients. We collect 200 sets of normal EEG signal and 300 sets of abnormal EEG signal. This is a single-channel database, with each sub-dataset containing 100 data fragments. Each data fragment has a duration of 23.6 seconds and consists of 4097 data points. The signal has a resolution of 12 bits and is sampled at a frequency of 173.61 Hz.
In the numerical experiment, we calculated the energy features of EEG signal at six different scales using the proposed new model for feature extraction. These features were then tested for classification performance using SVM. Additionally, we extracted six features from the same scale using MF-DFA and combined them with SVM to build a classifier. All computations were performed on MATLAB, running on an Intel(R) Core(TM) i7-10750H CPU@2.60GHz.
Now, we conduct numerical experiments on AC-SVM using a database comprising 500 EEG sequences. Firstly, we set the parameter ϵ to 1 and set n=6 as the number of multi-scale and we choose six evenly spaced values for mj from the interval [1,3]. Subsequently, for each scale, we compute the AC energy features εM for 300 abnormal EEG signal and 200 normal EEG signal. The features extracted across these scales, corresponding to each column of data, are illustrated in Figure 2. Notably, the range of features extracted from normal EEG signal differs from that of abnormal EEG signal. Overall, the energy features of normal EEG signal are significantly lower than those of abnormal EEG signal. Specifically, the energy feature range for normal EEG signal spans from −0.20 to 0.15, whereas the range for abnormal EEG signal spans from −0.24 to 0.19. Additionally, the energy features at different scales can be roughly delineated into distinct regions, with each color region in Figure 2(b) being larger than its counterpart in Figure 2(a).
To comprehensively assess the efficacy of the novel classifier, we employ MF-DFA to extract fractal features at six distinct scales and use multifractal features combined with SVM and Gaussian kernal to form a classifier named MF-SVM. The resulting multifractal features corresponding to each data column are visually depicted in Figure 3. The graphical representation distinctly illustrates that multifractal features in abnormal EEG signal have a broader range compared to their normal counterparts. Nevertheless, in contrast to the discernible delineation of energy features, multifractal features lack the clarity to demarcate a specific region, underscoring their diminished independence across different scales. Furthermore, upon scrutinizing Figure 3(b), a notable dissimilarity in the multifractal feature range between the latter 100 signals and the preceding 200 signals is apparent, a contrast not evident in the energy features. This observation elucidates the superior stability exhibited by energy features relative to multifractal features.
In addition, we summarize the features at different scales and show them in Figures 4 and 5. Both features show that the features of abnormal EEG signal exhibit greater dispersion, whereas the features of normal EEG signal are comparatively concentrated. Comparing the two graphs vertically, it is found that the distribution of multifractal features is more dispersed than that of energy features. In addition, it can be intuitively reflected that the independence of fractal features between different scales is lower than that of energy features.
In Section 4.1, we use the AC energy function to extract the energy features at six scales. Next, we combine the corresponding features of each set of data into a feature vector, and finally form a 500*6 matrix for SVM for classification. Considering the shortage of EEG signal, this paper uses the Leave One Out Cross Validation (LOOCV) to evaluate the performance of the new classifier. We randomly divide the sample into k subsets, of which (k−1) subsets are used as the training set and the rest are used as the test set. Therefore, the data set is tested k times; that is, each sample is used for testing. In brief, LOOCV has a low estimation bias when evaluating the generalization ability of the model, and LOOCV usually provides the most accurate performance evaluation. After that, we use SVM to calculate the following accuracy, sensitivity, and specificity:
accuracy=TP+TNTP+FN+FP+TN,sensitivity=TPTP+FN,specificity=TNFP+TN. | (4.1) |
Among them, TP, TN, FP, and FN are true positive, true negative, false positive, and false negative, respectively. Accuracy, sensitivity, and specificity are used to evaluate the probability, missed diagnosis rate, and misdiagnosis rate of SVM correctly identifying samples, respectively. The performance of AC-SVM and MF-SVM is tested by the method of LOOCV. We partitioned the samples into 10 subsets, setting k = 10 for cross-validation. The classification accuracy, sensitivity, and specificity of AC-SVM and MF-SVM are shown in Table 1. Obviously, AC-SVM draws lessons from the idea of extracting features at multi-scales; it shows obvious advantages in accuracy, sensitivity, and specificity. In general, it is difficult to improve the accuracy, but AC-SVM can improve the accuracy by nearly 3% compared with MF-SVM. Of particular importance is the notable efficiency gained in extracting multi-scale features through the AC energy function compared to MF-DFA. The temporal footprint for feature extraction per signal unit is meticulously documented in Table 1, where each sample set comprises a total of 4,097 data points. Additionally, we found that classification using only the Hurst exponent and Approximate entropy both achieved an accuracy of 88% respectively, while classification using only DFA just achieved an accuracy of 81%.
Methods | Accuracy | Sensitivity | Specificity | Time(s) |
MF-SVM | 87.40% | 89.28% | 88.11% | 0.356934 |
AC-SVM | 89.97% | 94.17% | 89.95% | 0.007738 |
To further optimize classification performance, we will integrate the features extracted from AC energy with Kolmogorov complexity, Shannon entropy, and Higuchi's Hurst exponent into a new feature set. This combination results in a classifier named AC-MC-SVM. The conceptual framework for this approach draws inspiration from prior literature that utilizes SVM for multivariate classification of EEG signal, forming the MC-SVM classifier [42]. Introduced by Claude Shannon in 1948, Shannon entropy measures dataset uncertainty and guides data compression and cryptography [50]. Kolmogorov complexity, proposed by Andrei Nikolaevich Kolmogorov in 1963 and formally developed by Lempel and Ziv in 1976 through LZ78, quantifies the minimal bit-length required for sequence generation and reflects sequence complexity [51,52]. Higuchi's Hurst exponent, which measures long-range dependencies in time series, indicates persistence with values over 0.5, playing a crucial role in analyzing data patterns [53].
We employed the AC-MC-SVM classifier for testing on the same database and contrasted the results with those obtained using MC-SVM. The specific outcomes are delineated in Table 2. The comparison reveals that our method not only diminishes computational expenses but also enhances accuracy by approximately 2% compared to the MC-SVM.
Methods | Accuracy | Sensitivity | Specificity | Time(s) |
MC-SVM | 95.29% | 96.28% | 94.55% | 0.996189 |
AC-MC-SVM | 97.19% | 97.96% | 94.61% | 0.735338 |
Firstly, we assess our method against other traditional machine learning approaches by conducting a comprehensive comparison across three key metrics: Accuracy, sensitivity, and specificity. The results of this evaluation are presented in Table 3. Acharya et al. [54] utilized continuous wavelet transform (CWT), higher-order spectra (HOS), and texture features, combined with SVM (CWT-HOS-SVM), for automatic classification of EEG signal. Brari et al. [55] proposed a method based on the A. Wolf method for determining the maximum lyapunov exponent (LLE) algorithm, used for analyzing chaotic signals disturbed by noise. This method achieved a classification accuracy of 96.48% for classes AB-CDE in the Bonn dataset. Subsequently, we conducted a survey of cutting-edge EEG classification methods based on DL and summarized the statistical overview in rows 4–6 of Table 3. Acharya et al. [20] pioneered the application of CNN in analyzing EEG signal. They developed a deep convolutional neural network algorithm comprising 13 layers to automatically identify EEG signal corresponding to normal, pre-ictal, and ictal states. In the study by Türk and Özerdem [56], two-dimensional frequency-time scale diagrams were generated by applying a continuous wavelet transform to EEG records. Subsequently, they employed CNN to analyze these scale diagrams for feature extraction and classification. We demonstrated the effectiveness of this approach in classifying classes A-C in the Bonn dataset. Bajpai et al. [57] employed time-frequency spectrograms to convert EEG signal into the image domain. Subsequently, they conducted model fusion, integrating three prominent CNN models (MC-CNN) for the classification task on EEG datasets sourced from Temple University Hospital.
Author | Methods | Accuracy | Sensitivity | Specificity |
Wang et al. (2023) [42] | MC-SVM | 95.29% | 96.28% | 94.55% |
Acharya et al. (2013) [54] | CWT-HOS-SVM | 96.00% | 96.90% | 97.00% |
Brari et al. (2022)[55] | LLE-SVM | 96.48% | / | / |
Acharya et al. (2018) [20] | CNN | 88.67% | 90.00% | 95.00% |
Türk et al. (2019) [56] | CNN-Scalogram | 96.50% | 98.94% | 94.28% |
Bajpai et al. (2021) [57] | MC-CNN | 96.65% | 90.48% | 100.00% |
The method proposed in this paper | AC-MC-SVM | 97.19% | 97.96% | 94.61% |
Remarkably, the AC-SVM classifier exhibits a substantial advantage in processing speed, significantly enhancing classification efficiency. This improvement markedly accelerates the diagnostic process in practical applications. The extraction of complex nonlinear eigenvalues, such as the largest lyapunov exponent (LLE), approximate entropy (ApEn), and fuzzy entropy (FuzzyEn), typically demands extensive computational resources. For a detailed comparison of classification times across various nonlinear features, we refer the reader to the comprehensive summary presented in Table 4, which refers to the article [58].
Features | Classifier | Time (s) |
ApEn | SVM | 741.540 |
PuzzyEn | KNN | 21.390 |
LLE | RFC | 534.360 |
AC | SVM | 0.007 |
AC-MC | SVM | 0.735 |
In our research, we introduced AC-SVM, an innovative method that significantly improves the feature extraction process for medical signal analysis, thereby enhancing the efficiency of classification tasks. By integrating the AC energy function with a sophisticated approach for eigenvalue computation, this method achieves multi-scale feature extraction. The AC energy function is capable of addressing the non-linear characteristics of medical signals, achieving a certain level of accuracy while reducing computational costs. It has been proven that, especially in diagnosing epilepsy, this method, when combined with other linear features, surpasses traditional classification techniques in terms of accuracy, sensitivity, and specificity. This holds the potential to provide a promising new tool for medical diagnostics, offering broad prospects for future application.
Our approach has demonstrably optimized computational efficiency, substantially curtailing both computational expenses and duration. In terms of accuracy, it surpasses the majority of traditional machine learning methodologies, though it necessitates further refinement to align with the forefront of contemporary deep learning paradigms. In our further investigation into the automatic classification of epilepsy syndromes, we will integrate other neurologically relevant features to optimize classification performance. In addition, we will apply the method proposed in this paper to the classification of other medical signals, such as electrocardiograms. Moreover, we can further improve the accuracy of feature extraction in our method by leveraging additional phase field equations, aiming to furnish the biomedical sector with classifiers of enhanced caliber.
![]() |
Ziling Lu: Writing-original draft, Writing-review & editing; Jian Wang: Supervision, Methodology. All authors have read and approved the final version of the manuscript for publication.
The authors declare they have not used Artificial Intelligence (AI) tools in the creation of this article.
The corresponding author, Jian Wang, expresses thanks for the Natural Science Foundation of the Jiangsu Higher Education Institutions of China (Grant Nos. 22KJB110020) and supported by the Open Project of the Center for Applied Mathematics of Jiangsu Province (Nanjing University of Information Science and Technology).
The authors declare that there is no conflict of interest regarding the publication of this article.
[1] |
M. X. Cohen, Where does EEG come from and what does it mean, Trends Neurosci., 40 (2017), 208–218. https://doi.org/10.1016/j.tins.2017.02.004 doi: 10.1016/j.tins.2017.02.004
![]() |
[2] |
M. R. Nuwer, D. A. Hovda, L. M. Schrader, P. M. Vespa, Routine and quantitative EEG in mild traumatic brain injury, Clin. Neurophysiol, 116 (2005), 2001–2025. https://doi.org/10.1016/j.clinph.2005.05.008 doi: 10.1016/j.clinph.2005.05.008
![]() |
[3] |
R. W. Thatcher, D. M. North, R. T. Curtin, R. A. Walker, C. J. Biver, J. F. Gomez, et al., An EEG severity index of traumatic brain injury, J. Neuropsych. Clin. N., 13 (2001), 77–87. https://doi.org/10.1176/appi.neuropsych.13.1.77 doi: 10.1176/appi.neuropsych.13.1.77
![]() |
[4] |
R. Silipo, G. Deco, H. Bartsch, Brain tumor classification based on EEG hidden dynamics, Intell. Data Anal., 3 (1999), 287–306. https://doi.org/10.3233/IDA-1999-3404 doi: 10.3233/IDA-1999-3404
![]() |
[5] |
V. S. Selvam, S. S. Devi, Analysis of spectral features of EEG signal in brain tumor condition, Meas. Sci. Rev., 15 (2015), 219–225. https://doi.org/10.1515/msr-2015-0030 doi: 10.1515/msr-2015-0030
![]() |
[6] |
S. J. M. Smith, EEG in the diagnosis, classification and management of patients with epilepsy, J. Neuro. Neurosur. Ps., 76 (2005), ii2–ii7. https://doi.org/10.1136/jnnp.2005.069245 doi: 10.1136/jnnp.2005.069245
![]() |
[7] |
S. Kiranyaz, T. Ince, M. Zabihi, D. Ince, Automated patient-specific classification of long-term electroencephalography, J. Biomed. Inform., 49 (2014), 16–31. https://doi.org/10.1016/j.jbi.2014.02.005 doi: 10.1016/j.jbi.2014.02.005
![]() |
[8] |
L. Cui, S. S. Sahoo, S. D. Lhatoo, G. Garg, P. Rai, A. Bozorgi, et al., Complex epilepsy phenotype extraction from narrative clinical discharge summaries, J. Biomed. Inform., 51 (2014), 272–279. https://doi.org/10.1016/j.jbi.2014.06.006 doi: 10.1016/j.jbi.2014.06.006
![]() |
[9] |
R. G. Andrzejak, K. Lehnertz, F. Mormann, C. Rieke, P. David, C. E. Elger, Indications of nonlinear deterministic and finite-dimensional structures in time series of brain electrical activity: Dependence on recording region and brain state, Phys. Rev. E, 64 (2001), 061907. https://doi.org/10.1103/PhysRevE.64.061907 doi: 10.1103/PhysRevE.64.061907
![]() |
[10] | World health organization, 2023. Available from: https://www.who.int/en/news-room/fact-sheets/detail/epilepsy. |
[11] |
D. Buck, G. A. Baker, A. Jacoby, D. F. Smith, D. W. Chadwick, Patients' experiences of injury as a result of epilepsy, Epilepsia, 38 (1997), 439–444. https://doi.org/10.1111/j.1528-1157.1997.tb01733.x doi: 10.1111/j.1528-1157.1997.tb01733.x
![]() |
[12] |
Y. Alotaibi, V. A. Veera, Electroencephalogram based face emotion recognition using multimodal fusion and 1-D convolution neural network (ID-CNN) classifier, AIMS Math., 8 (2023), 22984–23002. https://doi.org/10.3934/math.20231169 doi: 10.3934/math.20231169
![]() |
[13] |
A. M. Roy, Adaptive transfer learning-based multiscale feature fused deep convolutional neural network for EEG MI multiclassification in brain-computer interface, Eng. Appl. Artif. Intel., 116 (2022), 105347. https://doi.org/10.1016/j.engappai.2022.105347 doi: 10.1016/j.engappai.2022.105347
![]() |
[14] | A. M. Roy, A multi-scale fusion CNN model based on adaptive transfer learning for multi-class MI-classification in BCI system, BioRxiv, 2022. https://doi.org/10.1101/2022.03.17.481909 |
[15] |
S. Liu, X. Wang, L. Zhao, J. Zhao, Q. Xin, S. H. Wang, Subject-independent emotion recognition of EEG signal based on dynamic empirical convolutional neural network, IEEE ACM T. Comput. Bi., 18 (2020), 1710–1721. https://doi.org/10.1109/TCBB.2020.3018137 doi: 10.1109/TCBB.2020.3018137
![]() |
[16] |
H. Altaheri, G. Muhammad, M. Alsulaiman, M. Amin, S. U. Altuwaijri, G. A. Abdul, et al., Deep learning techniques for classification of electroencephalogram (EEG) motor imagery (MI) signals: A review, Neural Comput. Appl., 35 (2023), 14681–14722. https://doi.org/10.1007/s00521-021-06352-5 doi: 10.1007/s00521-021-06352-5
![]() |
[17] |
V. Bajaj, R. B. Pachori, Classification of seizure and nonseizure EEG signal using empirical mode decomposition, IEEE T. Inf. Technol. B., 16 (2011), 1135–1142. https://doi.org/10.1109/TITB.2011.2181403 doi: 10.1109/TITB.2011.2181403
![]() |
[18] |
Q. Xin, S. Hu, S. Liu, L. Zhao, S. Wang, WTRPNet: An explainable graph feature convolutional neural network for epileptic EEG classification, ACM T. Multim. Comput., 17 (2021), 1–18. https://doi.org/10.1145/3460522 doi: 10.1145/3460522
![]() |
[19] | A. Akan, H. S. Ture, Classification of epileptic and psychogenic nonepileptic seizures via time-frequency features of EEG data, Int. J. Neural Syst., 2023. https://doi.org/10.1142/S0129065723500454 |
[20] |
U. R. Acharya, S. L. Oh, Y. Hagiwara, J. H. Tan, H. Adeli, Deep convolutional neural network for the automated detection and diagnosis of seizure using EEG signal, Comput. Biol. Med., 100 (2018), 270–278. https://doi.org/10.1016/j.cmpb.2018.04.012 doi: 10.1016/j.cmpb.2018.04.012
![]() |
[21] |
Q. Xin, S. Hu, S. Liu, L. Zhao, Y. D. Zhang, An attention-based wavelet convolution neural network for epilepsy EEG classification, IEEE T. Neur. Sys. Reh., 30 (2022), 957–966. https://doi.org/10.1016/j.cej.2019.123775 doi: 10.1016/j.cej.2019.123775
![]() |
[22] | A. M. Roy, An efficient multi-scale CNN model with intrinsic feature integration for motor imagery EEG subject classification in brain-machine interfaces, Biomed. Signal Proces., 74 (2022) 103496. https://doi.org/10.1016/j.bspc.2022.103496 |
[23] |
D. Zhang, L. Yao, K. Chen, J. Monaghan, A convolutional recurrent attention model for subject-independent EEG signal analysis, IEEE Signal Proc. Let., 26 (2019), 715–719. https://doi.org/10.1109/LSP.2019.2906824 doi: 10.1109/LSP.2019.2906824
![]() |
[24] |
T. Dissanayake, T. Fernando, S. Denman, S. Sridharan, C. Fookes, Geometric deep learning for subject independent epileptic seizure prediction using scalp EEG signal, IEEE J. Biomed. Health, 26 (2021), 527–538. https://doi.org/10.1109/JBHI.2021.3100297 doi: 10.1109/JBHI.2021.3100297
![]() |
[25] |
M. Qiyas, M. Naeem, N. Khan, Fractional orthotriple fuzzy Choquet-Frank aggregation operators and their application in optimal selection for EEG of depression patients, AIMS Math., 8 (2023), 6323–6355. https://doi.org/10.3934/math.2023320 doi: 10.3934/math.2023320
![]() |
[26] |
S. Siuly, Y. Li, Y. Zhang, EEG signal analysis and classification, IEEE T. Neur. Sys. Reh., 11 (2016), 141–144. https://doi.org/10.1007/978-3-319-47653-7 doi: 10.1007/978-3-319-47653-7
![]() |
[27] | A. Craik, Y. He, J. L. C. Vidal, Deep learning for electroencephalogram (EEG), classification tasks: A review, J. Neural Eng., 16 (2019). https://doi.org/10.1088/1741-2552/ab0ab5 |
[28] |
T. Mondéjar, R. Hervás, E. Johnson, C. Gutierrez, J. M. Latorre, Correlation between videogame mechanics and executive functions through EEG analysis, J. Biomed. Inform., 63 (2016), 131–140. https://doi.org/10.1016/j.jbi.2016.08.006 doi: 10.1016/j.jbi.2016.08.006
![]() |
[29] | D. Zhang, K. Chen, D. Jian, L. Yao, Motor imagery classification via temporal attention cues of graph embedded EEG signals, IEEE J. Biomed. Health, 24 (2020). https://doi.org/10.1109/JBHI.2020.2967128 |
[30] |
Q. Yuan, W. Zhou, S. Li, D. Cai, Epileptic EEG classification based on extreme learning machine and nonlinear features, Epilepsy Res., 96 (2011), 29–38. https://doi.org/10.1016/j.eplepsyres.2011.04.013 doi: 10.1016/j.eplepsyres.2011.04.013
![]() |
[31] |
Y. Zhang, Y. Guo, P. Yang, W. Chen, B. Lo, Epilepsy seizure prediction on EEG using common spatial pattern and convolutional neural network, IEEE J. Biomed. Health, 24 (2019), 465–474. https://doi.org/10.1109/JBHI.2019.2933046 doi: 10.1109/JBHI.2019.2933046
![]() |
[32] |
T. Tuncer, A new stable nonlinear textural feature extraction method based EEG signal classification method using substitution Box of the Hamsi hash function: Hamsi pattern, Appl. Acoust., 172 (2021), 107607. https://doi.org/10.1016/j.apacoust.2020.107607 doi: 10.1016/j.apacoust.2020.107607
![]() |
[33] | P. K. Sethy, M. Panigrahi, K. Vijayakumar, S. K. Behera, Machine learning based classification of EEG signal for detection of child epileptic seizure without snipping, Int. J. Speech Technol., 26 (2023). https://doi.org/10.1007/s10772-021-09855-7 |
[34] |
M. Varlı, H. Yılmaz, Multiple classification of EEG signal and epileptic seizure diagnosis with combined deep learning, J. Comput. Sci.-Neth., 67 (2023), 101943. https://doi.org/10.1016/j.jocs.2023.101943 doi: 10.1016/j.jocs.2023.101943
![]() |
[35] |
W. Chen, Y. Wang, Y. Ren, H. Jiang, G. Du, J. Zhang, et al., An automated detection of epileptic seizures EEG using CNN classifier based on feature fusion with high accuracy, BMC. Med. Inform. Decis., 23 (2023), 96. https://doi.org/10.1186/s12911-023-02180-w doi: 10.1186/s12911-023-02180-w
![]() |
[36] |
L. Jiang, J. He, H. Pan, D. Wu, T. Jiang, J. Liu, Seizure detection algorithm based on improved functional brain network structure feature extraction, Biomed. Signal Proces., 79 (2023), 104053. https://doi.org/10.1016/j.bspc.2022.104053 doi: 10.1016/j.bspc.2022.104053
![]() |
[37] |
J. W. Kantelhardt, S. A. Zschiegner, E. K. Bunde, S. Havlin, A. Bunde, H. E. Stanley, Multifractal detrended fluctuation analysis of nonstationary time series, Physica A, 316 (2002), 81–91. https://doi.org/10.1016/S0378-4371(02)01383-3 doi: 10.1016/S0378-4371(02)01383-3
![]() |
[38] |
F. Wang, H. Wang, X. Zhou, R. Fu, A driving fatigue feature detection method based on multifractal theory, IEEE Sens. J., 22 (2022), 19046–19059. https://doi.org/10.1109/JSEN.2022.3201015 doi: 10.1109/JSEN.2022.3201015
![]() |
[39] |
V. Matic, P. J. Cherian, N. Koolen, A. H. Ansari, G. Naulaers, P. Govaert, et al., Objective differentiation of neonatal EEG background grades using detrended fluctuation analysis, Front. Hum. Neurosci., 9 (2015), 189. https://doi.org/10.3389/fnhum.2015.00189 doi: 10.3389/fnhum.2015.00189
![]() |
[40] |
T. Zorick, M. A. Mandelkern, Multifractal detrended fluctuation analysis of human EEG: Preliminary investigation and comparison with the wavelet transform modulus maxima technique, PLoS One, 8 (2013), e68360. https://doi.org/10.1371/journal.pone.0068360 doi: 10.1371/journal.pone.0068360
![]() |
[41] |
Z. Zhang, T. Wen, W. Huang, M. Wang, C. Li, Automatic epileptic seizure detection in EEGs using MF-DFA, SVM based on cloud computing, J. X-ray Sci. Technol., 25 (2017), 261–272. https://doi.org/10.3233/XST-17258 doi: 10.3233/XST-17258
![]() |
[42] |
J. Wang, W. Jiang, J. Kim, A novel ECG and EEG classification system based on nonlinear statistical features, Fractals, 31 (2023), 2350096. https://doi.org/10.1142/S0218348X23500962 doi: 10.1142/S0218348X23500962
![]() |
[43] |
J. Wang, H. Xu, J. Yang, J. Kim, Fractal feature analysis based on phase transitions of the Allen-Cahn and Cahn-Hilliard equations, J. Comput. Sci., 72 (2023), 102114. https://doi.org/10.1016/j.jocs.2023.102114 doi: 10.1016/j.jocs.2023.102114
![]() |
[44] |
S. M. Allen, J. W. Cahn, A microscopic theory for antiphase boundary motion and its application to antiphase domain coarsening, Acta. Metall., 27 (1979), 1085–1095. https://doi.org/10.1016/0001-6160(79)90196-2 doi: 10.1016/0001-6160(79)90196-2
![]() |
[45] |
J. Neumann, C. Schnörr, G. Steidl, Combined SVM-based feature selection and classification, Mach. Learn., 61 (2005), 129–150. https://doi.org/10.1007/s10994-005-1505-9 doi: 10.1007/s10994-005-1505-9
![]() |
[46] | C. Cortes, V. Vapnik, Support-vector networks, Mach. Learn., 20 (1995), 273–297. https://doi.org/10.1007/BF00994018 |
[47] |
A. Kampouraki, G. Manis, C. Nikou, Heartbeat time series classification with support vector machines, IEEE T. Inf. Technol. Biomed., 13 (2008), 512–518. https://doi.org/10.1109/TITB.2008.2003323 doi: 10.1109/TITB.2008.2003323
![]() |
[48] |
C. S. Lo, C. M. Wang, Support vector machine for breast MR image classification, Comput. Math. Appl., 64 (2012), 1153–1162. https://doi.org/10.1016/j.camwa.2012.03.033 doi: 10.1016/j.camwa.2012.03.033
![]() |
[49] |
M. Varlı, H. Yılmaz, Multiple classification of EEG signal and epileptic seizure diagnosis with combined deep learning, J. Comput. Sci., 67 (2023), 101943. https://doi.org/10.1016/j.jocs.2023.101943 doi: 10.1016/j.jocs.2023.101943
![]() |
[50] | C. E. Shannon, A mathematical theory of communication, Bell Syst. Tech. J., 27 (1948) 379–423. https://doi.org/10.1002/j.1538-7305.1948.tb01338.x |
[51] |
A. N. Kolmogorov, Combinatorial foundations of information theory and the calculus of probabilities, Russ. Math. Surv.+, 38 (1983), 29. https://doi.org/10.1070/RM1983v038n04ABEH004203 doi: 10.1070/RM1983v038n04ABEH004203
![]() |
[52] |
A. Lempel, J. Ziv, On the complexity of finite sequences, IEEE T. Inform. Theory, 22 (1976), 75–81. https://doi.org/10.1109/TIT.1976.1055501 doi: 10.1109/TIT.1976.1055501
![]() |
[53] |
T. Higuchi, Approach to an irregular time series on the basis of the fractal theory, Physica D, 31 (1988), 277–283. https://doi.org/10.1016/0167-2789(88)90081-4 doi: 10.1016/0167-2789(88)90081-4
![]() |
[54] |
U. R. Acharya, R. Yanti, J. W. Zheng, M. M. R. Krishnan, J. H. Tan, R. J. Martis, et al., Automated diagnosis of epilepsy using CWT, HOS and texture parameters, Int. J. Neural Syst., 23 (2013), 1350009. https://doi.org/10.1142/S0129065713500093 doi: 10.1142/S0129065713500093
![]() |
[55] |
Z. Brari, S. Belghith, A new algorithm for largest Lyapunov exponent determination for noisy chaotic signal studies with application to electroencephalographic signals analysis for epilepsy and epileptic seizures detection, Chaos Soliton. Fract., 165 (2022), 112757. https://doi.org/10.1016/j.chaos.2022.112757 doi: 10.1016/j.chaos.2022.112757
![]() |
[56] |
Ö. Türk, M. S. Özerdem, Epilepsy detection by using scalogram based convolutional neural network from EEG signal, Brain Sci., 9 (2019), 115. https://doi.org/10.3390/brainsci9050115 doi: 10.3390/brainsci9050115
![]() |
[57] |
R. Bajpai, R. Yuvaraj, A. A. Prince, Automated EEG pathology detection based on different convolutional neural network models: Deep learning approach, Comput. Biol. Med., 133 (2021), 104434. https://doi.org/10.1016/j.compbiomed.2021.104434 doi: 10.1016/j.compbiomed.2021.104434
![]() |
[58] |
M. Li, W. Chen, T. Zhang, Automatic epileptic EEG detection using DT-CWT-based non-linear features, Biomed. Signal Proces., 34 (2017), 114–125. https://doi.org/10.1016/j.bspc.2017.01.010 doi: 10.1016/j.bspc.2017.01.010
![]() |
1. | Shruti G. Taley, M. A. Pund, 2024, Multiscale Feature Extraction Techniques for Emotion Recognition from Physiological Signal, 979-8-3503-5681-6, 1867, 10.1109/ICAC2N63387.2024.10895032 |
Methods | Accuracy | Sensitivity | Specificity | Time(s) |
MF-SVM | 87.40% | 89.28% | 88.11% | 0.356934 |
AC-SVM | 89.97% | 94.17% | 89.95% | 0.007738 |
Methods | Accuracy | Sensitivity | Specificity | Time(s) |
MC-SVM | 95.29% | 96.28% | 94.55% | 0.996189 |
AC-MC-SVM | 97.19% | 97.96% | 94.61% | 0.735338 |
Author | Methods | Accuracy | Sensitivity | Specificity |
Wang et al. (2023) [42] | MC-SVM | 95.29% | 96.28% | 94.55% |
Acharya et al. (2013) [54] | CWT-HOS-SVM | 96.00% | 96.90% | 97.00% |
Brari et al. (2022)[55] | LLE-SVM | 96.48% | / | / |
Acharya et al. (2018) [20] | CNN | 88.67% | 90.00% | 95.00% |
Türk et al. (2019) [56] | CNN-Scalogram | 96.50% | 98.94% | 94.28% |
Bajpai et al. (2021) [57] | MC-CNN | 96.65% | 90.48% | 100.00% |
The method proposed in this paper | AC-MC-SVM | 97.19% | 97.96% | 94.61% |
Features | Classifier | Time (s) |
ApEn | SVM | 741.540 |
PuzzyEn | KNN | 21.390 |
LLE | RFC | 534.360 |
AC | SVM | 0.007 |
AC-MC | SVM | 0.735 |
Methods | Accuracy | Sensitivity | Specificity | Time(s) |
MF-SVM | 87.40% | 89.28% | 88.11% | 0.356934 |
AC-SVM | 89.97% | 94.17% | 89.95% | 0.007738 |
Methods | Accuracy | Sensitivity | Specificity | Time(s) |
MC-SVM | 95.29% | 96.28% | 94.55% | 0.996189 |
AC-MC-SVM | 97.19% | 97.96% | 94.61% | 0.735338 |
Author | Methods | Accuracy | Sensitivity | Specificity |
Wang et al. (2023) [42] | MC-SVM | 95.29% | 96.28% | 94.55% |
Acharya et al. (2013) [54] | CWT-HOS-SVM | 96.00% | 96.90% | 97.00% |
Brari et al. (2022)[55] | LLE-SVM | 96.48% | / | / |
Acharya et al. (2018) [20] | CNN | 88.67% | 90.00% | 95.00% |
Türk et al. (2019) [56] | CNN-Scalogram | 96.50% | 98.94% | 94.28% |
Bajpai et al. (2021) [57] | MC-CNN | 96.65% | 90.48% | 100.00% |
The method proposed in this paper | AC-MC-SVM | 97.19% | 97.96% | 94.61% |
Features | Classifier | Time (s) |
ApEn | SVM | 741.540 |
PuzzyEn | KNN | 21.390 |
LLE | RFC | 534.360 |
AC | SVM | 0.007 |
AC-MC | SVM | 0.735 |