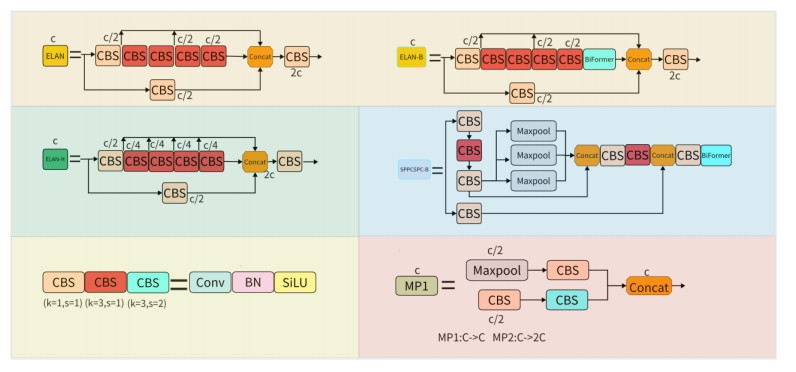
Fire is a common but serious disaster, which poses a great threat to human life and property. Therefore, fire-smoke detection technology is of great significance in various fields. In order to improve the detection ability of tiny-fire, so as to realize the prediction and suppression of fire as soon as possible, we proposed an efficient and accurate tiny-fire detection method based on the optimized YOLOv7, and we named the improved model YOLOv7-FIRE. First, we introduced the BiFormer into YOLOv7 to make the network pay more attention to the fire-smoke area. Second, we introduced the NWD technique to enhance the perception of the algorithm for small targets, and provided richer semantic information by modeling the context information around the target. Finally, CARAFE was applied for content-aware feature reorganization, which preserved the details and texture information in the image and improved the quality of fire-smoke detection. Furthermore, in order to improve the robustness of the improved algorithm, we expanded the fire-smoke dataset. The experimental results showed that YOLOv7-FIRE as significantly better than the previous algorithm in detection accuracy and recall rate, the Precision increased from 75.83% to 82.31%, and the Recall increased from 66.43% to 74.02%.
Citation: Baoshan Sun, Kaiyu Bi, Qiuyan Wang. YOLOv7-FIRE: A tiny-fire identification and detection method applied on UAV[J]. AIMS Mathematics, 2024, 9(5): 10775-10801. doi: 10.3934/math.2024526
[1] | Sultanah M. Alshammari, Nofe A. Alganmi, Mohammed H. Ba-Aoum, Sami Saeed Binyamin, Abdullah AL-Malaise AL-Ghamdi, Mahmoud Ragab . Hybrid arithmetic optimization algorithm with deep learning model for secure Unmanned Aerial Vehicle networks. AIMS Mathematics, 2024, 9(3): 7131-7151. doi: 10.3934/math.2024348 |
[2] | Xiaoxiao Liu, Chunrui Zhang . Forest model dynamics analysis and optimal control based on disease and fire interactions. AIMS Mathematics, 2024, 9(2): 3174-3194. doi: 10.3934/math.2024154 |
[3] | Yahia Said, Amjad A. Alsuwaylimi . AI-based outdoor moving object detection for smart city surveillance. AIMS Mathematics, 2024, 9(6): 16015-16030. doi: 10.3934/math.2024776 |
[4] | Muhammad Suleman, Muhammad Ilyas, M. Ikram Ullah Lali, Hafiz Tayyab Rauf, Seifedine Kadry . A review of different deep learning techniques for sperm fertility prediction. AIMS Mathematics, 2023, 8(7): 16360-16416. doi: 10.3934/math.2023838 |
[5] | Alexander Musaev, Dmitry Grigoriev, Maxim Kolosov . Adaptive algorithms for change point detection in financial time series. AIMS Mathematics, 2024, 9(12): 35238-35263. doi: 10.3934/math.20241674 |
[6] | Sang Gil Kang, Woo Dong Lee, Yongku Kim . Bayesian multiple changing-points detection. AIMS Mathematics, 2025, 10(3): 4662-4708. doi: 10.3934/math.2025216 |
[7] | Thavavel Vaiyapuri, M. Sivakumar, Shridevi S, Velmurugan Subbiah Parvathy, Janjhyam Venkata Naga Ramesh, Khasim Syed, Sachi Nandan Mohanty . An intelligent water drop algorithm with deep learning driven vehicle detection and classification. AIMS Mathematics, 2024, 9(5): 11352-11371. doi: 10.3934/math.2024557 |
[8] | Muhammad Danish Zia, Esmail Hassan Abdullatif Al-Sabri, Faisal Yousafzai, Murad-ul-Islam Khan, Rashad Ismail, Mohammed M. Khalaf . A study of quadratic Diophantine fuzzy sets with structural properties and their application in face mask detection during COVID-19. AIMS Mathematics, 2023, 8(6): 14449-14474. doi: 10.3934/math.2023738 |
[9] | E Laxmi Lydia, Chukka Santhaiah, Mohammed Altaf Ahmed, K. Vijaya Kumar, Gyanendra Prasad Joshi, Woong Cho . An equilibrium optimizer with deep recurrent neural networks enabled intrusion detection in secure cyber-physical systems. AIMS Mathematics, 2024, 9(5): 11718-11734. doi: 10.3934/math.2024574 |
[10] | Nuha Alruwais, Hayam Alamro, Majdy M. Eltahir, Ahmed S. Salama, Mohammed Assiri, Noura Abdelaziz Ahmed . Modified arithmetic optimization algorithm with Deep Learning based data analytics for depression detection. AIMS Mathematics, 2023, 8(12): 30335-30352. doi: 10.3934/math.20231549 |
Fire is a common but serious disaster, which poses a great threat to human life and property. Therefore, fire-smoke detection technology is of great significance in various fields. In order to improve the detection ability of tiny-fire, so as to realize the prediction and suppression of fire as soon as possible, we proposed an efficient and accurate tiny-fire detection method based on the optimized YOLOv7, and we named the improved model YOLOv7-FIRE. First, we introduced the BiFormer into YOLOv7 to make the network pay more attention to the fire-smoke area. Second, we introduced the NWD technique to enhance the perception of the algorithm for small targets, and provided richer semantic information by modeling the context information around the target. Finally, CARAFE was applied for content-aware feature reorganization, which preserved the details and texture information in the image and improved the quality of fire-smoke detection. Furthermore, in order to improve the robustness of the improved algorithm, we expanded the fire-smoke dataset. The experimental results showed that YOLOv7-FIRE as significantly better than the previous algorithm in detection accuracy and recall rate, the Precision increased from 75.83% to 82.31%, and the Recall increased from 66.43% to 74.02%.
In recent years, as a common but very serious disaster, fire has caused a great threat to human life and property. Therefore, fire-smoke detection technology is of great significance in various fields, such as building safety, intelligent transportation, industrial monitoring, etc. Our main purpose of this research is to improve the detection accuracy and detection speed of tiny-fire. The method adopted is to improve the detection ability of small targets. Tiny-fire detection can quickly detect the distance of even faint fire-smoke, allowing the fire to be detected at the initial stage. This provides valuable time for timely fire suppression measures, which greatly reduces the spread and loss caused by the fire. Traditional fire-smoke detection methods often have problems such as low detection rates, high computational complexity, and poor adaptability to environmental changes, so they need to be optimized. At present, the technology of sensor-based pyrotechnics detection devices is becoming more and more mature, but it faces a problem that when the pyrotechnics are small, they cannot generate an alarm in time, so they cannot effectively suppress the spread of the fire in time [1,2]. With the development of deep learning, fire-smoke detection methods based on deep learning have been widely studied and applied in recent years. Among them, YOLO (You Only Look Once) series algorithms [3,4,5,6,7,8,9] are a kind of real-time object detection algorithms, which have attracted much attention for their efficiency and accuracy. As an improved version of YOLO series, YOLOv7 [9] has achieved remarkable results in the field of object detection. However, the original YOLOv7 algorithm has certain limitations in tiny-fire detection scenes, such as low detection accuracy and missed detection. Therefore, it is urgent to propose an efficient and accurate improved algorithm to realize tiny-fire detection.
We aim to study and propose an efficient and accurate tiny-fire detection method based on the improved YOLOv7 [9] algorithm. By optimizing the network structure and loss function, and expanding and upgrading the dataset, the detection accuracy and robustness of the algorithm are improved. Specifically, we introduce the BiFormer [10] attention mechanism, integrate the NWD [11] loss function to the model, and also apply the CARAFE [12] technique. Through the improvement of the above three aspects, YOLOv7 is specifically optimized to enhance the detection ability of the algorithm in tiny-fire scenes. The BiFormer technique utilizes Bi-Level Routing Attention mechanism, which helps to improve the accuracy of object localization and improve the detection ability of small objects. Furthermore, it can better capture the global and semantic information of the image in the feature extraction process, improve the model's ability to perceive the image and global feature extraction ability, and can more accurately identify the characteristics of fire-smoke, such as color, shape, and texture. Therefore, by introducing BiFormer in fire-smoke detection, we can make the network pay more attention to the pyrotechnical area and be able to capture the global and semantic features of pyrotechnical more accurately. Figure 1 is the annotation of the key modules in the model of YOLOv7-FIRE, from which we can see that after adding the BiFormer module to the ELAN module, we named it ELAN-B. Furthermore, after a large number of experiments to verify, the BiFormer module can also be added to the SPPCSPC module and become the SPPCSPC-B module. The overall structure of YOLOv7-FIRE will be shown and explained in detail in Chapter 3. The NWD technique is specifically improved for tiny-object detection. Tiny-fire usually have the characteristics of low resolution, low contrast and large spatial variation, which brings great challenges to fire-smoke detection. The normalized Gaussian Wasserstein distance is used to model the distribution between the predicted bounding box and the real bounding box, and to correlate and match the target features, which can effectively overcome the above problems and improve the detection accuracy and recall rate of tiny-fire. We also applied the CARAFE technique for content-aware feature reorganization. Traditional object detection algorithms may lose details and texture information when dealing with small objects. By introducing the CARAFE layer, we are able to preserve the detail and texture information in the image during the feature reorganization process, thus improving the quality of pyrotechnics detection. Especially for the detail enhancement and reconstruction of small targets, CARAFE technology shows significant advantages in fire-smoke detection. The structure diagram of the improved model is shown in Figure 1. In order to make YOLOv7-FIRE more targeted for detection, we have also specially optimized the fire-smoke dataset, selected more images containing tiny-fire, covering a variety of different environments, such as indoor, outdoor, strong light, etc., and accurately labeled the location of fires and smokes. Moreover, we also retain a certain proportion of images containing medium and large fires and smokes, in order to make the algorithm not only be able to identify tiny-fire, but also be able to detect medium and large fires and smokes, and enhance the accuracy of fire-smoke recognition. In order to improve the robustness of the algorithm, the dataset also contains fire-smoke images under different lighting conditions and different viewing angles. On our dataset, we evaluate the performance of the improved algorithm in fire-smoke detection through a large number of experiments. The improved algorithm is superior to the unimproved algorithm in terms of detection accuracy and calculation speed, the Precision rises from 75.83% to 82.31%, an increase of about 7 percentage points, and the Recall rises from 66.43% to 74.02%, an increase of about 8 percentage points. Because the detection of tiny-fire is difficult, such an improvement effect has been very difficult.We also compare with other object detection algorithms, and the experimental results show that our improved algorithm has excellent performance.
The contributions of this paper mostly include two aspects. First, we integrate YOLOv7 with three technologies of BiFormer, NWD, and CARAFE and carry out special optimization to form a new target detection model YOLOv7-FIRE, which improves the detection accuracy of tiny-fire. Second, we propose a fire-smoke detection method based on the improved YOLOv7 algorithm, which improves the robustness of tiny-fire and is of great significance for tiny-fire detection.
The remaining part of this paper is organized as follows: Section 2 introduces the related work in detail, including a brief introduction of YOLOv7, and describes some representative results of current fire-smoke detection and small object detection. Section 3 will detail the improved method for tiny pyrotechnics. Section 4 will describe the experimental method, the evaluation index of the model and the comparison and analysis of the experimental results. Section 5 will conclude this paper and propose future research directions. It is believed that the results of this study will be of great significance to realize a more accurate and efficient fire-smoke detection system, improve fire safety, and reduce casualties and property losses. Future research can further explore the problem of pyrotechnical detection in more complex scenarios and further improve the algorithm to improve its performance.
YOLOv7 [9] is a new real-time target detection model. The model can quickly and accurately detect multiple types of targets, such as people, cars, animals and other common targets, and can provide more fine detection details for objects. The main optimization work of YOLOv7 includes the following aspects:
1) The backbone network structure is optimized: the lightweight network structure is adopted to reduce the complexity and redundant calculation of the network, so as to improve the operation speed and detection accuracy of the network.
2) An efficient feature extraction method is adopted: YOLOv7 extracts the features of the target image by means of feature pyramid network (FPN) [13] and attention mechanism [14], thereby reducing the computational complexity of target detection.
3) A new data enhancement method is introduced: YOLOv7 improves the generalization ability and robustness of the model by increasing the diversity and amount of data, thereby improving the performance of the model.
4) The model training process is optimized: the cumulative gradient correction, weight adjustment and multi-scale training techniques are used to optimize the model training process, thereby improving the training efficiency and accuracy of the model.
In short, YOLOv7 is an efficient, accurate and fast object detection model, which is one of the more excellent real-time object detection models at present. It has been widely used and promoted. However, in some special scenarios, the detection performance is unsatisfactory, and there is room for improvement. For example, the detection accuracy of small targets is not high, and the detection performance of infrequent targets such as fire-smoke is poor. The purpose of this paper is to improve YOLOv7 and improve the detection performance of fire-smoke, especially tiny-fire and tiny-smoke.
Through reading the literatures, it can be seen that deep learning and convolutional neural network (CNN) have caused extensive research in the academic community in fire detection, and have achieved good results in practical applications. Take the study of Zhao et al. [15] in 2018 as an example, they proposed a forest fire detection system based on visual camera, which can be applied in UAV deployment. Due to the possible instability in the images captured by the UAV during flight, the detection and localization of fires become very difficult. To solve this problem, their research team proposed a saliency detection method. The method first identifies the location of the fire by locating the core of the fire region, and then divides the fire region into multiple sub-images to avoid the loss of features due to the change of image size. The application of this method can improve the accuracy and efficiency of forest fire detection. In addition, in 2020, Li et al. [16] tested the fire detection ability of the current most advanced object detection convolutional neural network model in different scenarios. It includes the two-stage Faster R-CNN [17], the fully convolutional network model R-FCN [18], the single-stage SSD [19], and the much-concerned YOLOv3 [5] image fire detection algorithm. Moreover, in 2021, Xu et al. [20] adopted an ensemble learning approach and developed a new model for forest fire detection. The model integrates three deep learning algorithms, YOLOv5 [7] and EfficientDet [21] to detect fire locations and generate candidate boxes, while EfficientNet [22] is used to learn global information to determine whether an image contains a fire object and reduce the possibility of false positives. Despite the improvement to some extent in all the above studies, there are some limitations and deficiencies.
BiFormer [10] is an improved vision Transformer model that improves the performance of image understanding tasks by introducing Bi-Level Routing Attention. In the traditional Transformer model, the attention mechanism is widely used to capture relevant information in the input data. However, when dealing with visual data such as images, traditional Transformer models may face some challenges. Image data has high dimensionality and rich spatial structure, which may not be fully utilized by traditional attention mechanisms.
To solve this problem, the BiFormer model is proposed, which introduces Bi-Level Routing Attention. This mechanism better captures important features in the image by introducing two levels of routing in the attention computation. This Bi-Level Routing Attention mechanism enables the BiFormer model to better understand the contextual relationships and interactions between local features in images, thus improving the performance of image understanding tasks. Specifically, the Bi-Level Routing Attention consists of two phases. Initially, the first level routes the attention weights learned from the global context. These weights are then used to compute the routing attention at the second level to further capture important features in local regions. This mechanism enables the BiFormer model to simultaneously capture the global context and local features in the image [10].
In the task of tiny-object detection, the BiFormer [10] model has some advantages. The Bi-Level Routing Attention enables the BiFormer model to better capture the local features of tiny objects in the image, especially for those tiny objects that occupy a small area in the image. The traditional attention mechanism may not be able to effectively focus on these tiny target areas, while the BiFormer model shows better results in detection performance by introducing dual-level routing attention.
Literature [11] proposed an innovative tiny-object detection evaluation method based on Wasserstein distance. In this method, the bounding box (BBox) is represented as a two-dimensional Gaussian distribution, and the Normalized Wasserstein Distance (NWD) is introduced as a new metric to evaluate the quality of object detection results. The NWD metric is measured by calculating the similarity between the Gaussian distribution in the object detection result and the Gaussian distribution in the true bounding box. This metric can be easily embedded into the assignment, non-maximum suppression, and loss functions of anchor-based detectors to replace the traditional IoU metric. Wang et al. [11] evaluated the metric on AI-TOD, a new dataset dedicated to tiny-object detection. Compared with the existing object detection datasets, the average size of the objects in the AI-TOD dataset is smaller. Extensive experimental results demonstrate the potential application of the proposed method in improving the accuracy of tiny-object detection when using the NWD metric.
The traditional tiny-object detection method has the problem of inaccurate detection, which is caused by the small size and weak characteristics of the small object. To solve this problem, we propose a new loss function called NWD. Specifically, the method first uses a pre-trained detector to detect small objects in the image and obtains the detection results. Then, the normalized Gaussian tile loss distance is calculated as the loss function based on the difference between the detection results and the true labels.
Wang et al. [11] also pointed out that this method can effectively cope with the size, shape and distribution changes of small objects, so as to improve the stability and accuracy of small object detection. Overall, we provide an innovative way to evaluate algorithm improvements in the field of tiny-object detection and demonstrates the potential of the method to improve accuracy.
The main goal of CARAFE is to solve the blurring and distortion problems faced by traditional super-resolution methods when dealing with details and textures. Traditional methods usually employ techniques such as interpolation or inverse convolution to perform image enlargement, but these techniques often introduce artifacts and blur. To this end, the CARAFE layer proposes a content-aware feature reorganization method to improve the quality and detail preservation ability of the reconstructed image. The key idea is to improve the spatial resolution of the image by regrouping and reconstructing the input feature map. Specifically, the CARAFE layer uses trainable operation units to reorganize the input features into different patches, so that each patch can interact and reorganize information with the surrounding features pixel by pixel. In this content-aware way, CARAFE is able to better preserve the texture details of the image. To achieve efficient computation, the CARAFE layer employs a design based on differentiable parameterization to reduce computation and memory consumption. In addition, in order to better guide the grouping and interaction process, an adaptive context-aware filter is proposed, and an attention mechanism is introduced to further enhance the reconstruction effect [12].
The CARAFE [12] layer can improve the spatial resolution of the image through the content-aware feature reorganization method, so as to better retain the texture and detail information of the image in the small target detection, thereby enhancing the feature expression of the small target and improving the quality of the reconstructed image. Improving the resolution and contrast of the target region by means of reorganizing features helps to improve the detection precision and accuracy of small targets. The feature reorganization method of CARAFE layer can better retain details and textures, and then enhance the features of the target area, which has obvious advantages in the detection and recognition tasks of small targets.
In reference [10], there has been a detailed introduction to BiFormer. In order to better understand the working mechanism of BiFormer, I will first briefly introduce the principle of BiFormer.
BiFormer [10] introduced Bi-Level Routing Attention (BRA), an approach to achieve content-aware sparsity patterns through adaptive queries. First, the feature map is divided into S×S non-overlapping regions to obtain Q, K and V. Next, Qr and Kr are obtained by averaging Q and K, and the affinity adjacency matrix Ar between regions is obtained by transpose multiplication. The region routing index matrix Ir is obtained using the top k operator to retain only the top k regions most closely related to the region. Then, fine-grained token-to-token attention can be applied, as shown in Figure 2. First, all the routing regions indexed by Ir are clustered, and all their key sum values are collected to obtain Kg and Vg, and then Kg and Vg are applied to the attention calculation. Finally, BiFormer use O=Attention(Q,Kg,Vg)+LCE(V) structure, including LCE is a local context [23].
BiFormer uses BRA as the basic building block and the overall structure can be viewed as a four-stage pyramid structure (Figure 3 left). Overlapping patch embedding is used in the first stage, patch merging module is used in the second to fourth stages, and finally Ni consecutive BiFormer blocks are used to transform features [10].
According to the above principles, we first consider the whole BiFormer module as the backbone network of YOLOv7. However, doing so results in a substantial increase in the amount of FLOPs and parameters. To solve this problem, we tried other ways of adding. We try to use only the attention mechanism in the BiFormer module. Experiments show that adding BiFormer attention to a location in backbone can improve the detection ability of tiny-fire, while increasing the FLOPs, parameters, and training time is not much. Therefore, we decided that only adding BiFormer attention to YOLOv7 would achieve good results. Theoretically, the amount of BiFormer attention is positively correlated with the detection effect. Therefore, we add multiple BiFormer attention to the two ELAN modules of backbone and the SPPCSPC module of head. After experiments, when the accuracy reaches a certain level, adding more BiFormer attention is difficult to further improve the accuracy, but it will lead to a rapid increase in the amount of parameters and FLOPs. Therefore, after repeated experiments, we finally determined that adding BiFormer attention to the two ELAN modules of the backbone network and the SPPCSPC module of the head works best. Thus, ELAN becomes ELAN-B and SPPCSPC becomes SPPCSPC-B, where the letter "B" indicates that BiFormer attention has been added. Please refer to Figures 4 and 5 for the modification method of the two modules.
Before we talk about how to add NWD, let us first explain the principle and limitations of YOLOv7's original coordinate Loss function CIoU (Complete IoU Loss).
The CIoU loss [27] takes into account three important geometric factors, namely the overlap area, the distance of the center point, and the aspect ratio. Given a prediction box B and a target box Bgt, the CIOU loss is defined as follows:
LCIoU=1−IoU+ρ2(b,bgt)c2+αv. | (1) |
Here, b and bgt denote the center points of B and Bgt, respectively ρ(⋅)=∥b−bgt∥2 denotes the Euclidean distance. c is the diagonal length of the smallest closed box covering both boxes. The α is a balancing parameter (this coefficient is not involved in the gradient calculation), where the priority is given based on the IoU value. The higher the IoU of the prediction and target boxes, the higher the coefficient. It is calculated as follows:
α=v(1−IoU)+v. | (2) |
The v is used to calculate the consistency of the aspect ratio of the predicted and target boxes, which is measured by the tan Angle, and it is calculated as follows:
v=4π2(arctanwgthgt−arctanwh)2. | (3) |
The gradient of v with respect to w and h is computed as follows:
∂v∂w=8π2(arctanwgthgt−arctanwh)∗hw2+h2, | (4) |
∂v∂h=−8π2(arctanwgthgt−arctanwh)∗ww2+h2. | (5) |
Although CIoU is based on DIoU, it adds the loss of detection box scale, and increases the loss of length and width, so that the predicted box will be more in line with the real box. However, there are four problems as follows: (Ⅰ) v reflects only the difference of aspect ratio, rather than the actual relationship between w and wgt or h and hgt. That is, all with attributes {(w=kwgt,h=khgt)∣k∈R+} have v=0, so the CIoU loss may optimize the similarity in an unreasonable way, which is not consistent with reality. (Ⅱ) CIoU loss function is sensitive to the size of the bounding box. When calculating the CIoU loss, the width and height of the bounding box need to be divided, which makes the CIoU loss function very sensitive to the size change of the bounding box. This means that when the size of the bounding box changes a lot, the value of the CIoU loss function also changes a lot. This sensitivity may cause the model to focus more on the size of the bounding box while ignoring other important features during training. (Ⅲ) CIoU loss function is not sensitive to the error of the center point. When calculating the CIoU loss, only the overlap degree of the bounding boxes and the distance of the center point are considered, and the error of the center point is ignored. However, in the object detection task, the accuracy of the center point is very important for locating the object. Therefore, the insensitivity of the CIoU loss function may cause the model to ignore the accuracy of the center point during the training process, which affects the positioning accuracy of the object. (Ⅳ) The CIoU loss function has difficulties in dealing with objects of different scales. Since the CIoU loss function is sensitive to the size of the bounding box, difficulties arise when dealing with objects of different scales. When the target scale difference is large, the CIoU loss function may lead to a large loss value, which affects the training effect of the model [27].
Then, we will briefly describe the implementation process of NWD.
It was mentioned in reference [11] that for small objects, there tend to be some background pixels in their bounding boxes, since most real objects are not strictly rectangular. In these bounding boxes, foreground and background pixels are concentrated on the center and boundary of the bounding box, respectively. To better describe the weights of different pixels in the bounding box, the bounding box can be modeled as a two-dimensional Gaussian distribution, where the center pixel of the bounding box has the highest weight and the importance of the pixel gradually decreases from the center to the boundary. Specifically, for the horizontal bounding box R = (cx, cy, w, h), where (cx, cy), w and h denote the center coordinates, width and height, respectively. The equation for its inscribed ellipse can be expressed as
(x−μx)2σ2x+(y−μy)2σ2y=1. | (6) |
Where (μx,μy) represents the center coordinates of the ellipse, and σx,σy represent the lengths along the X-axis and the Y-axis. Therefore, μx=cx,μy=cy,σx=w2,σy=h2. The probability density function of a two-dimensional Gaussian distribution can be expressed as follows.
f(x∣μ,Σ)=exp(−12(x−μ)⊤Σ−1(x−μ))2π|Σ|12. | (7) |
Where x,μ,Σ denote the center point (x, y), μ denote the mean of the Gaussian distribution, and Σ denote the covariance of the Gaussian distribution. When (x−μ)⊤Σ−1(x−μ)=1, the ellipse in Eq (6) is the density profile of a two-dimensional Gaussian distribution. Thus, the horizontal bounding box can be represented as a two-dimensional Gaussian distribution N(μ,Σ), obeying
μ=[cxcy],Σ=[w2400h24]. | (8) |
Thus, the similarity of two bounding boxes is transformed into the distance distribution of two Gaussians.
Wasserstein distance [11] is derived from the optimal transport theory and is used to calculate the distance between two distributions. For two gaussian distribution μ1=N(m1,Σ1) and μ2=N(m2,Σ2), the second-order Wasserstein distance between μ1 and μ2 can be defined as:
W22(μ1,μ2)=∥m1−m2∥22+Tr(Σ1+Σ2−2(Σ122Σ1Σ122)12). | (9) |
It can be simplified to:
W22(μ1,μ2)=∥m1−m2∥22+∥Σ121−Σ122∥2F. | (10) |
For two bounding boxes:
W22(Na,Nb)=∥([cxa,cya,wa2,ha2]T,[cxb,cyb,wb2⋅hb2]T)∥22. | (11) |
However, this is a distance metric and cannot be used directly for similarity. The normalized exponent is used to obtain a new metric called the normalized Wasserstein distance [11].
NWD(Na,Nb)=exp(−√W22(Na,Nb)C). | (12) |
Here, C is a constant, depending on the dataset. The best results are achieved by setting C to the average absolute size of the objects in the dataset. C is stable with respect to the results within a certain range.
Since NWD [11] is not sensitive to objects of different scales, it is more suitable for measuring the similarity between tiny objects. However, NWD can also be used when the objects to be detected in the dataset are not entirely small objects. In this paper, both IoU loss and NWD loss are used. In order to better deal with small targets, we introduce the coefficient iou_ratio. This factor is critical to balance the weight of IoU loss and NWD loss in the total loss. Suppose we set iou_ratio = 0.5, meaning that IoU and NWD each account for 50% of the total loss.
First, let us consider the case when the dataset is full of small objects. Since small targets tend to have small regions, the intersection and union ratio between the predicted box and the true target box may be relatively low. Therefore, we can set the iou_ratio to 0 so that the NWD loss will be more important. By paying more attention to the distance between the predicted box and the true target box, the algorithm will locate the small target more accurately and improve the detection accuracy.
However, the number of tiny targets in my dataset is about 80%. This means that a certain percentage of medium and large targets exist in the dataset. If we completely ignore the importance of the IoU metric, we may not be able to take full advantage of the overlap between the predicted box and the actual target box. Therefore, in order to balance the performance of the object detection algorithm considering different object sizes, I set iou_ratio to 0.8. With this setting, we add the losses of IoU and NWD to the total loss with equal weight. This practice allows the algorithm to achieve a reasonable balance between small objectives and other objectives. It makes the algorithm more accurate in locating small targets, and can make full use of the intersection and union ratio information of medium and large targets.
Using iou_ratio to balance the loss of IoU and NWD is very important when dealing with datasets containing small targets. By properly setting the value of iou_ratio, we are able to optimize the performance of the object detection algorithm on different object sizes. This method takes full account of the characteristics of small targets, but also retains the accuracy requirements for other targets, so as to improve the overall object detection performance. In practical applications, we can dynamically adjust the value of iou_ratio according to the characteristics and requirements of the dataset to further improve the effect of the algorithm. Compared with IoU, NWD has the advantages of scale invariance in tiny-object detection, smoothness to position deviations, and the ability to measure the similarity between bounding boxes that do not overlap or contain each other.
As mentioned in reference [12], CARAFE is divided into two major modules, which are called Kernel Prediction Module (Figure 6) and Content-aware Ressembly Module (Figure 7). Assuming an upsampling multiplier σ, we are given an input feature map of shape H×W×C. First, the upsampling kernel prediction module is used to predict the upsampling kernel, and then the upsampling is performed by the feature recombination module to obtain the output feature map with shape σH×σW×C.
For an input feature map of shape H×W×C, a 1×1 convolutional layer is first used to compress the number of channels to Cm, the main purpose of this step is to reduce the complexity of subsequent calculations.
Assuming that the upsampling kernel size is kup×kup (a larger upsampling kernel means a larger receptive field and more computation), if we want to use a different upsampling kernel for each position of the output feature map, we need to predict an upsampling kernel of shape σH×σW×kup×kup. To achieve this goal, for the compressed input feature map, we use a convolutional layer that utilizes a kencoder×kencoder to predict the upsampling kernel, where the number of input channels is Cm and the number of output channels is σ2k2up. Then, we expand the channel dimension in the spatial dimension to obtain an upsampling kernel of shape σH×σW×k2up [12].
The parameters of the encoder are kencoder×kencoder×Cm×Cup. Intuitively, increasing kencoder expands the acceptance range of the encoder and exploits a wider range of contextual information, which is important for predicting and reorganizing kernels. However, as the kernel size increases, the computational complexity also increases. The benefits of a larger kernel do not increase the computational complexity. Therefore, choosing kencoder=kup−2 can strike a good balance between performance and efficiency. Each kup×kup recombination kernel is spatially normalized using a softmax function. This normalization step enforces the sum of kernel values to be one, achieving soft selection across local regions. Thanks to the kernel normalizer, CARAFE does not do any scaling or change the mean of the feature maps [12].
Since only two Upsample modules are included in the original YOLOv7 [9] algorithm, adding CARAFE to YOLOv7 becomes very simple by simply replacing the Upsample module with CARAFE. The specific replacement method is shown in Figure 8. There are two key hyperparameters for tiny-object detection, kencoder and kup. The authors experimented with various Settings of these two hyperparameters, and the best detection performance was obtained when kencoder=5 and kup=7. To simplify the experiment, I directly adopted the setting of the authors to evaluate the performance of CARAFE in tiny-fire detection. However, we found that kencoder is set to 5 and kup is set to 6 to achieve the best tiny-fire detection.
After the above improvements on the three main aspects of YOLOv7, we finally formed an efficient detector that can detect tiny fire-smoke, namely YOLOv7-FIRE. As you can see from Figure 9, an image of size 640*640*3 is input into Backbone to process the image. In backbone, due to the addition of the BiFormer attention module (the improved ELAN module, its name is ELAN-B), Therefore, the detection will focus more on the area of the image where the fireworks are, so as to improve the feature extraction ability. Then, in the Head part, the BiFormer attention module is also applied (the improved SPPCSPC module, its name is SPPCSPC-B). In this part, we also replace the Upsample module with the CARAFE module, so that the feature extraction ability of YOLOv7-FIRE is further enhanced. Finally, the processed image is output. From the output results, no matter what kind of scene, fireworks can be accurately detected and the location of fireworks can be accurately located. The input image in the structure diagram is only used as an example, and more detailed detection results will be described in Section 4.
The operating system used in this experiment is Windows 10, and Python 3.8 and PyTorch 1.13 are used to build the network model. The image input size is 640×640×3, and a Nvidia GeForce RTX 3080 is utilized as computational resources. Considering the limited computing resources, we set the batch-size to 8 and the epoch to 200. During training, each epoch takes about 3 minutes, so each training session will last about 600 minutes, or 10 hours.
In the training process, some parameters are involved as shown in Table 1. First, let us explain the meaning of some parameters: lr0 stands for the initial value of the learning rate, and lrf stands for the final value of the learning rate.The momentum can be understood as the inertia of the parameter update. It does this by maintaining a momentum vector that records the weighted average of the previous gradient directions and uses it for parameter updates. Doing so can speed up training and improve model stability. Properly adjusting the magnitude of the momentum can make the parameter update direction smoother, and a larger momentum can accelerate the parameter update. Another parameter is weight_decay, which is a common regularization technique designed to reduce model complexity to prevent overfitting. Higher values of weight_decay leads to stronger regularization and better model generalization. However, too much weight_decay will cause the problem of underfitting the model. Now to warmup_epochs, when training a deep learning model, it is sometimes necessary to warmup with a smaller learning rate first to avoid unstable gradients or loss in the initial stage. The warmup_epochs control the number of warm-up epochs, that is, using a smaller learning rate for the first few epochs of training to allow the model to converge to a steady state faster. After the warm-up phase, the learning rate will gradually increase to the set initial learning rate, and the training will continue at the set learning rate. Finally, we have warmup_momentum, which, in a nutshell, represents the setting of the momentum during warmup.
Parameters | Values |
Epoch | 200 |
Batch size | 8 |
Lr0 | 0.01 |
Lrf | 0.1 |
Momentum | 0.937 |
Weight_Decay | 0.005 |
Warmup_Epochs | 3 |
warmup_momentum | 0.8 |
Number of images | 2885 |
Image size | 640 |
Layers | 503 |
Parameters | 71736790 |
FLOPS | 190.4G |
In this experiment, the dataset pictures we use are all from the public data in the network. The dataset contains two categories, namely fire-smoke and smoke. We first collect a large number of images containing fire-smoke and smoke from the Web. In order to verify the performance of the improved algorithm in the detection ability of tiny-fire, we screened from these pictures. We kept most of the images containing tiny-fire and a small number of images containing larger fire-smoke. Among them, tiny-fire account for about 80%, while large fire-smoke account for about 20%. The purpose of retaining large fire-smoke images is to evaluate whether the improved algorithm has an impact on the detection ability of large fire-smoke in addition to tiny-fire. Our goal is to improve the detection accuracy of tiny-fire without degrading the detection ability of large fire-smoke. In order to achieve the robustness of the algorithm, in addition to considering the size of the fire-smoke shape, we also collected fire-smoke pictures with different angles, different shapes, different colors, and different brightness. After the collection of images in the dataset, we carried out the data cleaning work. For the bad quality images, we deleted them or processed the blurry images clearly. Finally, we annotate the remaining pictures in detail to accurately locate the location of the fire-smoke to ensure the accuracy of the model during training. After labeling the images, we randomly split the dataset into training, validation, and test sets. The training set contains 2522 images, the validation set contains 240 images, and the test set contains 123 images.
The experimental results based on this dataset show that YOLOv7-FIRE achieves a significant improvement in fire-smoke detection, and does not have a negative impact on the detection effect of large fire-smoke. This indicates that the improved method can accurately detect tiny-fire and maintain a good detection effect for large fire-smoke. These experimental results further verify the effectiveness and robustness of YOLOv7-FIRE in dealing with the problem of fire-smoke detection. In general, we obtain a high equality fire-smoke image dataset containing diversity using public datasets in the web through screening and cleaning work. The improved algorithm shows a significant improvement in fire-smoke detection ability on this dataset, and also maintains a good effect on the detection of large fire-smoke. These experimental results provide further verification basis for the effectiveness and robustness of YOLOv7-FIRE.
Accuracy refers to the proportion of detected pyrotechnical targets that are truly pyrotechnical. It measures the accuracy of the model in predicting fire-smoke. The accuracy is calculated as follows:
Precision=TPTP+FP. | (13) |
Where TP is a true positive (bounding box correctly detected as a firework) and FP is a false positive (bounding box incorrectly detected as a fire-smoke). High accuracy indicates that the model produces fewer false positives when predicting fire-smoke, that is, incorrectly labeling non-fire-smoke targets as fire-smoke.
Recall is the proportion of targets that are actually fire-smoke that are correctly detected. It measures the ability of the model in identifying all the pyrotechnical targets. Recall is calculated as follows:
Recall=TPTP+FN. | (14) |
Where TP is a true positive and FN is a false negative (a real firework bounding box that was not detected). A high recall indicates that the model produces fewer false negatives when identifying fire-smoke, that is, the targets that are actually fire-smoke are correctly labeled as fire-smoke.
Mean Average Precision (mAP) is a widely used object detection evaluation metric to comprehensively evaluate the performance of an algorithm on multiple object categories. It is measured by calculating the average accuracy of the model between the detection result and the true label under different accuracy thresholds. The mAP value is between 0 and 1, and a higher mAP value indicates that the model has better performance on multiple categories and can accurately detect and locate the object. This metric yield results by calculating the area under the precision-recall curve for each category. For each class, the mAP is computed as follows:
1) Rank the detection results according to the confidence of the prediction.
2) Calculate the precision and recall of the model according to different confidence thresholds.
3) The final mAP is obtained by calculating the area under the precision-recall curve and averaging the results across all classes.
The formula is expressed as:
mAP=1c∑cj=1APj. | (15) |
AP=∫10Precision(Recall)d(Recall). | (16) |
After training, YOLOv7-FIRE has good performance. We use YOLOv7-FIRE to detect tiny-fire, and the results can meet the expected requirements. Figure 10 shows the confusion matrix of YOLOv7-fire after training. The confusion matrix summarizes the records in the dataset in the form of a matrix according to the two criteria of the true category and the category judgment predicted by YOLOv7-FIRE. The rows of the matrix represent the actual values and the columns represent the predicted values.
In the Figure 11, we can see that YOLOv7-FIRE can accurately detect tiny fire-smokes in the image. Furthermore, the detection effect of slightly larger fire-smokes is not affected at all. The detected images cover almost all scenarios of fire-smokes. From the detection results, our YOLOv7-FIRE is robust and accurate, it can accurately detect fire-smokes in different scenarios and accurately locate the position of fire-smokes. Moreover, the brightness of the illumination, the change of the background and even the occlusion of the foreground object do not have a great impact on the detection of fire-smokes. Note that different classes are labeled with different bounding boxes, with the orange box for smoke and the blue box for fire.
The first thing we need to compare is the change in accuracy between YOLOv7 [9] and optimized YOLOv7-FIRE. On our dataset, the accuracy of YOLOv7 is 75.83%, and the accuracy of optimized YOLOv7-FIRE is improved to 82.31%, which is about 7 percentage points higher. This is not an easy achievement because objects with irregular shapes are often involved in fire detection, which makes the detection work difficult. From Figure 12, we can intuitively see the detection performance improvement of YOLOv7-FIRE.
Second, we compare the improvement of the two in terms of Recall. Compared with YOLOv7, YOLOv7-FIRE improves the Recall by about 8 percentage points. From Figure 13, we can observe the improvement in Recall.
Next, we look at how the mAP value changes. mAP values have two forms: mAP@.5 and mAP@.5:.95. Here, mAP@.5 represents the average mAP at a threshold greater than 0.5, and mAP@.5:.95 represents the average mAP at different IoU thresholds (0.5 to 0.95 in steps of 0.05) (0.5, 0.55, 0.6, 0.65, 0.7, 0.75, 0.8, 0.85, 0.9, 0.95). From Figure 14, we can observe the lifting of both mAP values.
Finally, we analyze the change of loss. As can be seen in Figure 15, the optimization effect for loss is not particularly obvious. However, through our experiments, we can conclude that it is feasible to optimize the loss function with our improved method. In the following, we will continue to study the optimization of the loss function on this basis, in order to obtain more significant improvement.
In order to enhance the authority of YOLOv7-FIRE model in fire-smoke detection, we conduct a comparison with other mainstream object detection models, including YOLOv4 [6], YOLOv5s [7], and Faster R-CNN [17]. Same as YOLOv7-FIRE, we use the same hyperparameter setting, experimental environment and dataset in the experiment. We recorded the precision, recall, mAP@0.5, number of parameters, and FLOPs of these models to evaluate their performance.
It can be seen from Table 2 that compared with other mainstream object detection models, our YOLOv7-FIRE has good performance and achieves the best results in the three indicators of precision, recall rate and mAP@0.5. Therefore, it can be further verified that our improved YOLOv7-FIRE method is feasible in the detection of tiny fire-smokes. Figure 16 shows the precision, recall and mAP@0.5 of different object detection models in a more clear and intuitive form.
Model | Precision | Recall | mAP@0.5 | #Param. (M) | FLOPs (G) |
YOLOv4 | 0.6952 | 0.6085 | 0.5027 | 64.40 | 142.8 |
YOLOv5s | 0.7336 | 0.6525 | 0.5403 | 21.26 | 49.2 |
Faster R-CNN | 0.7583 | 0.6643 | 0.5314 | 37.62 | 116.5 |
YOLOv7 | 0.8154 | 0.7176 | 0.6018 | 60.13 | 245.7 |
YOLOv-FIRE | 0.8231 | 0.7402 | 0.6159 | 71.74 | 190.4 |
It is worth noting that although Faster R-CNN is almost the same as YOLOv7-FIRE in the detection of tiny fire-smokes, or even can ignore the difference between them, it can be seen from Figure 17 that the FLOPs of Faster R-CNN is much higher than that of YOLOv7-FIRE. Thus, it can be concluded that our YOLOv7- FIRE performs better.
We aim to study and propose an efficient and accurate fire-smoke detection method based on the improved YOLOv7 algorithm. By optimizing the network structure and loss function, and augmenting the dataset, we improve the detection accuracy and robustness of the algorithm. The experimental results verify the superior performance of the improved algorithm in fire-smoke detection. In the following, the research of this paper is summarized and future research directions are proposed.
We first analyze the importance of fire detection and the problems of existing methods, and point out the research significance of the improved YOLOv7 algorithm. By introducing the BiFormer attention mechanism and introducing the NWD loss function, and integrating the CARAFE technology, we improve the YOLOv7 algorithm and achieve higher detection accuracy in tiny-fire scenes. The attention mechanism makes the network pay more attention to detecting fire-smoke areas and improves the accuracy of the algorithm. NWD and CARAFE improve the accuracy of the algorithm by focusing on the details of the fire-smoke. In addition, through the optimization of the dataset, we increase the robustness of the algorithm in diverse environments, so that it can better adapt to different fire-smoke detection tasks. After experimental evaluation, we verified the superior performance of the improved algorithm in pyrotechnical detection. Compared with the traditional YOLOv7 algorithm and other fire-smoke detection algorithms, the improved algorithm has achieved significant advantages in detection accuracy and calculation speed. Furthermore, the robustness test shows that the improved algorithm has strong ability to detect fire-smoke in different environments. These experimental evidences fully demonstrate the effectiveness and feasibility of the proposed method.
However, the work of this paper has some limitations and room for improvement. To this end, we propose the following outlook and future research directions:
First, although we optimized the algorithm and achieved good results, it is possible to further improve the network structure and algorithm design to further improve the accuracy and robustness of fire-smoke detection. For example, more complex attention mechanisms and fusion strategies can be considered to further improve the algorithm's ability to perceive fire-smoke areas. In addition, different loss function [24,25,26] designs and training strategies can be explored to further improve the performance of the algorithm.
Second, although the dataset used in this paper has been expanded, there may be certain data bias and shortcomings. Future research could consider collecting more diverse and realistic pyrotechnical data covering more fire scenarios and environmental conditions to increase the adaptability and robustness of the algorithm. In addition, the introduction of Uavs or other sensor devices for pyrotechnical detection using multi-source data can be considered to enhance the reliability and accuracy of the algorithm.
In addition, the research in this paper focuses on the static scene of fire-smoke detection, and future research can extend the focus to the detection of fire-smoke in dynamic scenes. Considering the characteristics of fire movement in the actual scene, the detection and tracking of mobile fire-smoke is also a challenging problem. Future research can explore the application of the improved YOLOv7 algorithm in dynamic scenarios to improve the accuracy and real-time performance of mobile fire-smoke.
Finally, our focus of this paper is on pyrotechnical detection; however, fire safety also involves a wider range of applications and challenges, such as fire prevention, suppression strategies, and rescue operations. Future research can further explore other issues related to fire safety, such as smoke detection and combustion process analysis, on the basis of pyrotechnics detection, in order to comprehensively improve the ability of fire safety.
In summary, we propose an efficient and accurate fire-smoke detection method based on the improved YOLOv7 algorithm, and verifies its superior performance through experiments. However, there are many aspects that can be further improved and studied. We believe that in future work, these perspectives and research directions will further promote the development of pyrotechnic detection technology and provide more reliable and efficient solutions for fire safety.
The authors declare they have not used Artificial Intelligence (AI) tools in the creation of this article.
This work is partically supported by Natural Science Foundation of China Grants (61972456, 61173032) and Tianjin Natural Science Foundation (20JCYBJC00140). Also, we would like to thank the School of Computer Science and Technology, Tiangong University for supporting our work.
The authors declare no conflicts of interest.
[1] |
F. Q. Zhang, P. C. Zhao, S. W. Xu, Y. Wu, X. B. Yang, Y. Zhang, Integrating multiple factors to optimize watchtower deployment for wildfire detection, Sci. Total Environ., 737 (2020), 139561. https://doi.org/10.1016/j.scitotenv.2020.139561 doi: 10.1016/j.scitotenv.2020.139561
![]() |
[2] | M. Karthi, R. Priscilla, S. G, N. Infantia C, A. G. R, V. J, Forest fire detection: A comparative analysis of deep learning algorithms, 2023 International Conference on Artificial Intelligence and Knowledge Discovery in Concurrent Engineering (ICECONF), Chennai, India, 2023, 1–6. https://doi.org/10.1109/ICECONF57129.2023.10084329 |
[3] | J. Redmon, S. Divvala, R. Girshick, A. Farhadi, You only look once: Unified, real-time object detection, 2016 IEEE Conference on Computer Vision and Pattern Recognition (CVPR), Las Vegas, NV, USA, 2016,779–788. https://doi.org/10.1109/CVPR.2016.91 |
[4] | J. Redmon, A. Farhadi, Yolo9000: better, faster, stronger, 2017 IEEE Conference on Computer Vision and Pattern Recognition (CVPR), Honolulu, HI, USA, 2017, 6517–6525. https://doi.org/10.1109/CVPR.2017.690 |
[5] |
Z. Liu, X. Y. Gu, H. L. Yang, L. T. Wang, Y. H. Chen, D. Y. Wang, Novel YOLOv3 model with structure and hyperparameter optimization for detection of pavement concealed cracks in GPR images, IEEE T. Intell. Transp., 23 (2022), 22258–22268. https://doi.org/10.1109/TITS.2022.3174626 doi: 10.1109/TITS.2022.3174626
![]() |
[6] | A. Bochkovskiy, C. Y. Wang, H. Y. M. Liao, YOLOv4: Optimal speed and accuracy of object detection, 2020, arXiv: 2004.10934. https://doi.org/10.48550/arXiv.2004.10934 |
[7] |
Q. Q. Ding, P. Li, X. F. Yan, D. Shi, L. M. Liang, W. M. Wang, et al., CF-YOLO: Cross fusion YOLO for object detection in adverse weather with a high-quality real snow dataset, IEEE T. Intell. Transp., 24 (2023), 10749–10759. https://doi.org/10.1109/TITS.2023.3285035 doi: 10.1109/TITS.2023.3285035
![]() |
[8] |
K. C. Song, X. K. Sun, S. Ma, Y. H. Yan, Surface defect detection of aeroengine blades based on cross-layer semantic guidance, IEEE T. Instrum. Meas., 72 (2023), 2514411. https://doi.org/10.1109/TIM.2023.3276026 doi: 10.1109/TIM.2023.3276026
![]() |
[9] | C. Y. Wang, A. Bochkovskiy, H. Y. M. Liao, YOLOv7: Trainable bag-of-freebies sets new state-of-the-art for real-time object detectors, 2023 IEEE/CVF Conference on Computer Vision and Pattern Recognition (CVPR), Vancouver, BC, Canada, 2023, 7464–7475. https://doi.org/10.1109/CVPR52729.2023.00721 |
[10] | L. Zhu, X. J. Wang, Z. H. Ke, W. Zhang, R. Lau, BiFormer: Vision transformer with Bi-level routing attention, 2023 IEEE/CVF Conference on Computer Vision and Pattern Recognition (CVPR), Vancouver, BC, Canada, 2023, 10323–10333. https://doi.org/10.1109/CVPR52729.2023.00995 |
[11] | J. W. Wang, C. Xu, W. Yang, L. Yu, A normalized Gaussian Wasserstein distance for tiny object detection, 2021, arXiv: 2110.13389. https://doi.org/10.48550/arXiv.2110.13389 |
[12] | J. Q. Wang, K. Chen, R. Xu, Z. W. Liu, C. C. Loy, D. H. Lin, CARAFE: Content-aware ReAssembly of Features, 2019 IEEE/CVF International Conference on Computer Vision (ICCV), Seoul, Korea (South), 2019, 3007–3016. https://doi.org/10.1109/ICCV.2019.00310 |
[13] | A. Kirillov, R. Girshick, K. He, P. Dollár, Panoptic Feature Pyramid Networks, 2019 IEEE/CVF Conference on Computer Vision and Pattern Recognition (CVPR), Long Beach, CA, USA, 2019, 6392–6401. https://doi.org/10.1109/CVPR.2019.00656 |
[14] | M. Hu, Y. L. Li, L. Fang, S. J. Wang, A2-FPN: Attention aggregation based feature pyramid network for instance segmentation, 2021 IEEE/CVF Conference on Computer Vision and Pattern Recognition (CVPR), Nashville, TN, USA, 2021, 15338–15347. https://doi.org/10.1109/CVPR46437.2021.01509 |
[15] |
Z. Yi, J. Ma, X. H. Li, J. Zhang, Saliency detection and deep learning-based wildfire identification in UAV imagery, Sensors, 18 (2018), 712. https://doi.org/10.3390/s18030712 doi: 10.3390/s18030712
![]() |
[16] |
X. Q. Li, Z. X. Chen, Q. M. J. Wu, C. Y. Liu, 3D parallel fully convolutional networks for real-time video wildfire smoke detection, IEEE T. Circ. Syst. Vid., 30 (2020), 89–103. https://doi.org/10.1109/TCSVT.2018.2889193 doi: 10.1109/TCSVT.2018.2889193
![]() |
[17] |
S. Q. Ren, K. M. He, R. Girshick, J. Sun, Faster R-CNN: Towards real-time object detection with region proposal networks, IEEE T. Pattern Anal., 39 (2017), 1137–1149. https://doi.org/10.1109/TPAMI.2016.2577031 doi: 10.1109/TPAMI.2016.2577031
![]() |
[18] | J. L. Zhang, S. X. Chen, Y. W. Hou, Accurate object detection with relation module on improved R-FCN, 2020 Chinese Automation Congress (CAC), Shanghai, China, 2020, 7131–7135. https://doi.org/10.1109/CAC51589.2020.9326543 |
[19] |
J. J. Ni, K. Shen, Y. Chen, S. X. Yang, An improved SSD-like deep network-based object detection method for indoor scenes, IEEE T. Instrum. Meas., 72 (2023), 5006915. https://doi.org/10.1109/TIM.2023.3244819 doi: 10.1109/TIM.2023.3244819
![]() |
[20] |
R. J. Xu, H. F. Lin, K. J. Lu, L. Cao, Y. F. Liu, A forest fire detection system based on ensemble learning, Forests, 12 (2021), 217. https://doi.org/10.3390/f12020217 doi: 10.3390/f12020217
![]() |
[21] | M. X. Tan, R. M. Pang, Q. V. Le, EfficientDet: Scalable and efficient object detection, 2020 IEEE/CVF Conference on Computer Vision and Pattern Recognition (CVPR), Seattle, WA, USA, 2020, 10778–10787. https://doi.org/10.1109/CVPR42600.2020.01079 |
[22] |
K. Ramamurthy, A. R. Varikuti, B. Gupta, N. Aswani, A deep learning network for Gleason grading of prostate biopsies using EfficientNet, Biomed. Tech., 68 (2023), 187–198. https://doi.org/10.1515/bmt-2022-0201 doi: 10.1515/bmt-2022-0201
![]() |
[23] | S. C. Ren, D. Q. Zhou, S. F. He, J. S. Feng, X. C. Wang, Shunted Self-Attention via Multi-Scale Token Aggregation, 2022 IEEE/CVF Conference on Computer Vision and Pattern Recognition (CVPR), New Orleans, LA, USA, 2022, 10843–10852. https://doi.org/10.1109/CVPR52688.2022.01058 |
[24] | Z. J. Tong, Y. H. Chen, Z. Xu, R. Yu, Wise-IoU: Bounding box regression loss with dynamic focusing mechanism, 2023, arXiv: 2301.10051. https://doi.org/10.48550/arXiv.2301.10051 |
[25] |
H. Y. Peng, S. Q. Yu, A systematic IoU-Related method: Beyond simplified regression for better localization, IEEE T. Image Process., 30 (2021), 5032–5044. https://doi.org/10.1109/TIP.2021.3077144 doi: 10.1109/TIP.2021.3077144
![]() |
[26] | Z. Gevorgyan, SIoU loss: More powerful learning for bounding box regression, 2022, arXiv: 2205.12740. https://doi.org/10.48550/arXiv.2205.12740 |
[27] |
Z. H. Zheng, P. Wang, D. W. Ren, W. Liu, R. G. Ye, Q. H. Hu, et al., Enhancing geometric factors in model learning and inference for object detection and instance segmentation, IEEE T. Cybernetics, 52 (2022), 8574–8586. https://doi.org/10.1109/TCYB.2021.3095305 doi: 10.1109/TCYB.2021.3095305
![]() |
1. | Huihui Yan, Zhihua Cui, Haotian Zhao, Jingbo Zhang, Juanjuan Qin, Qian Guo, The Multi-Scale Depth-Separable Convolution Network for Fire and Smoke Detection, 2024, 0010-2202, 1, 10.1080/00102202.2024.2372689 | |
2. | Guanyi Li, Pengle Cheng, Yong Li, Ying Huang, Lightweight wildfire smoke monitoring algorithm based on unmanned aerial vehicle vision, 2024, 18, 1863-1703, 7079, 10.1007/s11760-024-03377-w | |
3. | Ruoyu Peng, Chaoyuan Cui, Yun Wu, Real-time fire detection algorithm on low-power endpoint device, 2025, 22, 1861-8200, 10.1007/s11554-024-01605-7 | |
4. | Dan Shao, Yu Liu, Guoxing Liu, Ning Wang, Pu Chen, Jiaxun Yu, Guangmin Liang, YOLOv7scb: A Small-Target Object Detection Method for Fire Smoke Inspection, 2025, 8, 2571-6255, 62, 10.3390/fire8020062 | |
5. | Yanjuan Wang, Jiayue Liu, Jun Zhao, Zhibin Li, Yuxian Yan, Xiaohong Yan, Fengqiang Xu, Fengqi Li, LCSC-UAVNet: A High-Precision and Lightweight Model for Small-Object Identification and Detection in Maritime UAV Perspective, 2025, 9, 2504-446X, 100, 10.3390/drones9020100 | |
6. | Wen-Tsai Sung, Indra Griha Tofik Isa, Ching-Hsiang Tseng, Sung-Jung Hsiao, Constructing a Lightweight Fire and Smoke Detection Through the Improved GhostNet Architecture and Attention Module Mechanism, 2025, 13, 2169-3536, 46794, 10.1109/ACCESS.2025.3550539 |
Parameters | Values |
Epoch | 200 |
Batch size | 8 |
Lr0 | 0.01 |
Lrf | 0.1 |
Momentum | 0.937 |
Weight_Decay | 0.005 |
Warmup_Epochs | 3 |
warmup_momentum | 0.8 |
Number of images | 2885 |
Image size | 640 |
Layers | 503 |
Parameters | 71736790 |
FLOPS | 190.4G |
Model | Precision | Recall | mAP@0.5 | #Param. (M) | FLOPs (G) |
YOLOv4 | 0.6952 | 0.6085 | 0.5027 | 64.40 | 142.8 |
YOLOv5s | 0.7336 | 0.6525 | 0.5403 | 21.26 | 49.2 |
Faster R-CNN | 0.7583 | 0.6643 | 0.5314 | 37.62 | 116.5 |
YOLOv7 | 0.8154 | 0.7176 | 0.6018 | 60.13 | 245.7 |
YOLOv-FIRE | 0.8231 | 0.7402 | 0.6159 | 71.74 | 190.4 |
Parameters | Values |
Epoch | 200 |
Batch size | 8 |
Lr0 | 0.01 |
Lrf | 0.1 |
Momentum | 0.937 |
Weight_Decay | 0.005 |
Warmup_Epochs | 3 |
warmup_momentum | 0.8 |
Number of images | 2885 |
Image size | 640 |
Layers | 503 |
Parameters | 71736790 |
FLOPS | 190.4G |
Model | Precision | Recall | mAP@0.5 | #Param. (M) | FLOPs (G) |
YOLOv4 | 0.6952 | 0.6085 | 0.5027 | 64.40 | 142.8 |
YOLOv5s | 0.7336 | 0.6525 | 0.5403 | 21.26 | 49.2 |
Faster R-CNN | 0.7583 | 0.6643 | 0.5314 | 37.62 | 116.5 |
YOLOv7 | 0.8154 | 0.7176 | 0.6018 | 60.13 | 245.7 |
YOLOv-FIRE | 0.8231 | 0.7402 | 0.6159 | 71.74 | 190.4 |