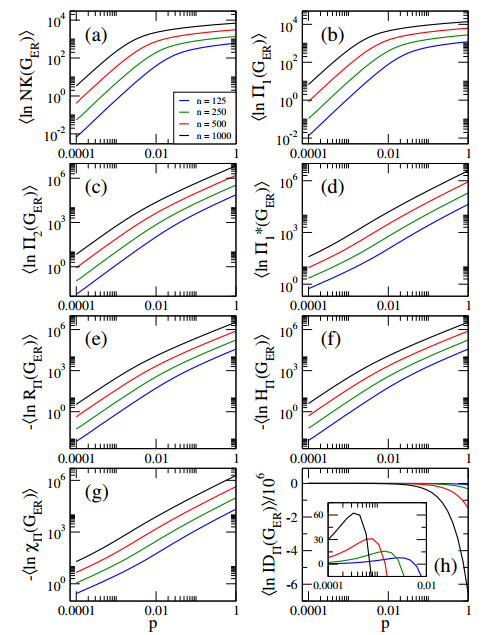
Securing Unmanned Aerial Vehicle (UAV) systems is vital to safeguard the processes involved in operating the drones. This involves the execution of robust communication encryption processes to defend the data exchanged between the UAVs and ground control stations. Intrusion detection, powered by Deep Learning (DL) techniques such as Convolutional Neural Networks (CNN), allows the classification and identification of potential attacks or illegal objects in the operational region of the drone, thus distinguishing them from the routine basics. The current research work offers a new Hybrid Arithmetic Optimizer Algorithm with DL method for Secure Unmanned Aerial Vehicle Network (HAOADL-UAVN) model. The purpose of the proposed HAOADL-UAVN technique is to secure the communication that occurs in UAV networks via threat detection. At the primary level, the network data is normalized through min-max normalization approach in order to scale the input dataset into a useful format. The HAOA is used to select a set of optimal features. Next, the security is attained via Deep Belief Network Autoencoder (DBN-AE)-based threat detection. At last, the hyperparameter choice of the DBN-AE method is implemented using the Seagull Optimization Algorithm (SOA). A huge array of simulations was conducted using the benchmark datasets to demonstrate the improved performance of the proposed HAOADL-UAVN algorithm. The comprehensive results underline the supremacy of the HAOADL-UAVN methodology under distinct evaluation metrics.
Citation: Sultanah M. Alshammari, Nofe A. Alganmi, Mohammed H. Ba-Aoum, Sami Saeed Binyamin, Abdullah AL-Malaise AL-Ghamdi, Mahmoud Ragab. Hybrid arithmetic optimization algorithm with deep learning model for secure Unmanned Aerial Vehicle networks[J]. AIMS Mathematics, 2024, 9(3): 7131-7151. doi: 10.3934/math.2024348
[1] | Usman Babar, Haidar Ali, Shahid Hussain Arshad, Umber Sheikh . Multiplicative topological properties of graphs derived from honeycomb structure. AIMS Mathematics, 2020, 5(2): 1562-1587. doi: 10.3934/math.2020107 |
[2] | Ali Al Khabyah . Mathematical aspects and topological properties of two chemical networks. AIMS Mathematics, 2023, 8(2): 4666-4681. doi: 10.3934/math.2023230 |
[3] | Ali N. A. Koam, Ali Ahmad, Azeem Haider, Moin A. Ansari . Computation of eccentric topological indices of zero-divisor graphs based on their edges. AIMS Mathematics, 2022, 7(7): 11509-11518. doi: 10.3934/math.2022641 |
[4] | Edil D. Molina, José M. Rodríguez-García, José M. Sigarreta, Sergio J. Torralbas Fitz . On the Gutman-Milovanović index and chemical applications. AIMS Mathematics, 2025, 10(2): 1998-2020. doi: 10.3934/math.2025094 |
[5] | Shama Liaqat, Zeeshan Saleem Mufti, Yilun Shang . Newly defined fuzzy Misbalance Prodeg Index with application in multi-criteria decision-making. AIMS Mathematics, 2024, 9(8): 20193-20220. doi: 10.3934/math.2024984 |
[6] | Sumiya Nasir, Nadeem ul Hassan Awan, Fozia Bashir Farooq, Saima Parveen . Topological indices of novel drugs used in blood cancer treatment and its QSPR modeling. AIMS Mathematics, 2022, 7(7): 11829-11850. doi: 10.3934/math.2022660 |
[7] | Chenxu Yang, Meng Ji, Kinkar Chandra Das, Yaping Mao . Extreme graphs on the Sombor indices. AIMS Mathematics, 2022, 7(10): 19126-19146. doi: 10.3934/math.20221050 |
[8] | Zeeshan Saleem Mufti, Ali Tabraiz, Qin Xin, Bander Almutairi, Rukhshanda Anjum . Fuzzy topological analysis of pizza graph. AIMS Mathematics, 2023, 8(6): 12841-12856. doi: 10.3934/math.2023647 |
[9] | Sumaira Hafeez, Rashid Farooq . On generalized inverse sum indeg index and energy of graphs. AIMS Mathematics, 2020, 5(3): 2388-2411. doi: 10.3934/math.2020158 |
[10] | Wei Gao, Zahid Iqbal, Shehnaz Akhter, Muhammad Ishaq, Adnan Aslam . On irregularity descriptors of derived graphs. AIMS Mathematics, 2020, 5(5): 4085-4107. doi: 10.3934/math.2020262 |
Securing Unmanned Aerial Vehicle (UAV) systems is vital to safeguard the processes involved in operating the drones. This involves the execution of robust communication encryption processes to defend the data exchanged between the UAVs and ground control stations. Intrusion detection, powered by Deep Learning (DL) techniques such as Convolutional Neural Networks (CNN), allows the classification and identification of potential attacks or illegal objects in the operational region of the drone, thus distinguishing them from the routine basics. The current research work offers a new Hybrid Arithmetic Optimizer Algorithm with DL method for Secure Unmanned Aerial Vehicle Network (HAOADL-UAVN) model. The purpose of the proposed HAOADL-UAVN technique is to secure the communication that occurs in UAV networks via threat detection. At the primary level, the network data is normalized through min-max normalization approach in order to scale the input dataset into a useful format. The HAOA is used to select a set of optimal features. Next, the security is attained via Deep Belief Network Autoencoder (DBN-AE)-based threat detection. At last, the hyperparameter choice of the DBN-AE method is implemented using the Seagull Optimization Algorithm (SOA). A huge array of simulations was conducted using the benchmark datasets to demonstrate the improved performance of the proposed HAOADL-UAVN algorithm. The comprehensive results underline the supremacy of the HAOADL-UAVN methodology under distinct evaluation metrics.
We can identify two types of graph invariants which are currently being studied in chemical graph theory. Namely,
XΣ(G)=XΣ,FV(G)=∑u∈V(G)FV(du)orXΣ(G)=XΣ,FE(G)=∑uv∈E(G)FE(du,dv) | (1.1) |
and
XΠ(G)=XΠ,FV(G)=∏u∈V(G)FV(du)orXΠ(G)=XΠ,FE(G)=∏uv∈E(G)FE(du,dv). | (1.2) |
Here, uv denotes the edge of the graph G=(V(G),E(G)) connecting the vertices u and v, du is the degree of the vertex u, and FV(x) and FE(x,y) are appropriately defined functions (see e.g., [1]). Since XΣ(G) and XΠ(G) are usually known as topological indices in the literature, here we will refer to XΠ(G) as multiplicative topological indices (MTIs) to make a clear difference between both types of indices.
Among the vast amount of topological indices of the form XΣ(G), the first and second Zagreb indices [2] stand out, and they are defined as
M1(G)=∑u∈V(G)d2u=∑uv∈E(G)(du+dv) | (1.3) |
and
M2(G)=∑uv∈E(G)dudv, | (1.4) |
respectively. Also, the Randić connectivity index [3]
R(G)=∑uv∈E(G)1√dudv, | (1.5) |
the harmonic index [4]
H(G)=∑uv∈E(G)2du+dv, | (1.6) |
the sum-connectivity index [5]
χ(G)=∑uv∈E(G)1√du+dv, | (1.7) |
and the inverse degree index [4]
ID(G)=∑u∈V(G)1du=∑uv∈E(G)(1d2u+1d2v). | (1.8) |
The topological indices mentioned above and many others of the form XΣ(G) have been widely studied during recent decades.
More recently, MTIs have attracted a lot of attention, see e.g., [6,7,8,9,10,11,12]. Indeed, several MTIs have already been deeply analyzed in the literature (see e.g., the work of V. R. Kulli). Among the most relevant ones, the Narumi-Katayama index [13] should be mentioned:
NK(G)=∏u∈V(G)du. | (1.9) |
Additionally, multiplicative versions of the Zagreb indices [14]
Π1(G)=∏u∈V(G)d2u, | (1.10) |
Π2(G)=∏uv∈E(G)dudv | (1.11) |
and
Π∗1(G)=∏uv∈E(G)(du+dv). | (1.12) |
In addition to the MTIs of Eqs (1.9)–(1.12), in this work we also consider multiplicative versions of the indices in Eqs (1.5)–(1.8): the multiplicative Randić connectivity index
RΠ(G)=∏uv∈E(G)1√dudv, | (1.13) |
the multiplicative harmonic index
HΠ(G)=∏uv∈E(G)2du+dv, | (1.14) |
the multiplicative sum-connectivity index
χΠ(G)=∏uv∈E(G)1√du+dv, | (1.15) |
and the multiplicative inverse degree index
IDΠ(G)=∏uv∈E(G)(1d2u+1d2v). | (1.16) |
Therefore, motivated by their potential applications, in this work we perform an analytical as well as computational study of MTIs. Specifically, the purpose of this work is threefold:
First, we follow an analytical viewpoint to find several new inequalities that relate two MTIs indices between them as well as to their additive versions in Section 2. Note that the search for inequalities between different indices is a classical topic in the study of topological indices. See e.g., [6,7,8,9,10,11,12] for previous results relating MTIs.
Second, we want to establish the statistical analysis of MTIs as a generic tool for the study of average properties of random networks in Section 3. For some previous works dealing with the statistical analysis of (non-multiplicative) topological indices, see, e.g., [15,16,17,18,19].
Finally, in this paper we perform for the first time (to our knowledge) a scaling study of MTIs on random networks which let us state a scaling law that relates different random graph models in Section 4.
There are many works studying particular MTIs; see, e.g., [20,21] and the references therein. However, in this section we obtain several analytical inequalities involving the general MTIs XΠ,FV(G) and XΠ,FE(G).
The following equalities are direct:
XΠ,FV(G)=∏u∈V(G)FV(du)=e∑u∈V(G)logFV(du)=eXΣ,logFV(G),XΠ,FE(G)=∏uv∈E(G)FE(du,dv)=e∑uv∈E(G)logFE(du,dv)=eXΣ,logFE(G). |
Since the geometric mean is at most the arithmetic mean (by Jensen's inequality), we have the following inequalities.
Proposition 2.1. Let G be a graph with n vertices and m edges. Then,
XΠ,FV(G)1/n≤1nXΣ,FV(G),XΠ,FE(G)1/m≤1mXΣ,FE(G). |
This general result has as a particular consequence the following known inequality, see [9, Theorem 2.1].
Corollary 2.1. Let G be a graph with n vertices and m edges. Then,
Π1(G)≤(2mn)2n. |
Proof. If we take FV(du)=du, then
XΠ,FV(G)=Π1(G)1/2,XΣ,FV(G)=2m, |
and Proposition 2.1 gives
Π1(G)1/(2n)≤2mn, |
which implies the desired inequality.
Proposition 2.1 has several consequences:
Proposition 2.2. Let G be a graph with n vertices and m edges.
(1) If FE(du,dv)=h(du)+h(dv) and FV(du)=duh(du) for some function h, then
XΠ,FV(G)1/n≤1nXΣ,FE(G),XΠ,FE(G)1/m≤1mXΣ,FV(G). |
(2) If FE(du,dv)=h(du)h(dv) and FV(du)=h(du)du for some function h, then
XΠ,FE(G)1/n≤1nXΣ,FV(G),XΠ,FV(G)1/m≤1mXΣ,FE(G). |
Proof. Let G be a graph with n vertices and m edges.
If FE(du,dv)=h(du)+h(dv) and FV(du)=duh(du) for some function h, then
XΣ,FE(G)=∑uv∈E(G)FE(du,dv)=∑uv∈E(G)(h(du)+h(dv))=∑u∈V(G)duh(du)=XΣ,FV(G). |
Consequently, Proposition 2.1 implies
XΠ,FV(G)1/n≤1nXΣ,FV(G)=1nXΣ,FE(G),XΠ,FE(G)1/m≤1mXΣ,FE(G)=1mXΣ,FV(G). |
If FE(du,dv)=h(du)h(dv) and FV(du)=h(du)du for some function h, then
XΠ,FE(G)=∏uv∈E(G)FE(du,dv)=∏uv∈E(G)h(du)h(dv)=∏u∈V(G)h(du)du=XΠ,FV(G). |
Hence, Proposition 2.1 gives
XΠ,FE(G)1/n=XΠ,FV(G)1/n≤1nXΣ,FV(G),XΠ,FV(G)1/m=XΠ,FE(G)1/m≤1mXΣ,FE(G). |
Theorem 2.1. Let G be a graph with m edges. Assume that F1(du,dv)=h(du)+h(dv) and F2(du,dv)=h(du)h(dv) for some function h. Then,
XΠ,F2(G)≤(12mXΣ,F1(G))2m. |
Proof. Since the (weighted) geometric mean is at most the (weighted) arithmetic mean (by Jensen's inequality), the following inequality holds for every xi,wi>0:
(n∏i=1xwii)1/∑ni=1wi≤1∑ni=1win∑i=1xiwi. |
If we take xi=h(du) and wi=du, then we get
(∏u∈V(G)h(du)du)1/∑u∈E(G)du≤1∑u∈V(G)du∑u∈V(G)duh(du),(∏uv∈E(G)h(du)h(dv))1/(2m)≤12m∑uv∈E(G)(h(du)+h(dv)),XΠ,F2(G)≤(12mXΣ,F1(G))2m. |
This general result has as a particular consequence the following known inequality, see [9, Theorem 3.1].
Corollary 2.2. Let G be a graph with m edges. Then,
Π2(G)≤(12mM1(G))2m. |
Proof. If we take h(x)=x, then F1(du,dv)=du+dv, F2(du,dv)=dudv, XΠ,F2(G)=Π2(G), XΣ,F1(G)=M1(G), and Theorem 2.1 gives the desired inequality.
In [22] appears the following Jensen-type inequality.
Theorem 2.2. Let μ be a probability measure on the space X and a≤b be real constants. If f:X→[a,b] is a measurable function and φ is a convex function on [a,b], then f and φ∘f are μ-integrable functions, and
φ(a+b−∫Xfdμ)≤φ(a)+φ(b)−∫Xφ∘fdμ. |
Theorem 2.2 allows one to find the following converse of Proposition 2.1, if we consider the normalized counting measure as μ.
Theorem 2.3. Let G be a graph with n vertices and m edges, aV≤bV, aE≤bE be real constants, and FV, FE be functions satisfying
aV≤FV(du)≤bV∀u∈V(G),aE≤FE(du,dv)≤bE∀uv∈E(G). |
Then,
1nXΣ,FV(G)≤eaV+ebV−eaV+bVXΠ,FV(G)−1/n,1mXΣ,FE(G)≤eaE+ebE−eaE+bEXΠ,FE(G)−1/m. |
Proof. Since φ(x)=ex is a convex function on R, Theorem 2.2 gives
eaV+bV−1n∑u∈V(G)logFV(du)≤eaV+ebV−1n∑u∈V(G)elogFV(du),eaV+bVelog(Πu∈V(G)FV(du))−1/n≤eaV+ebV−1n∑u∈V(G)FV(du),eaV+bVXΠ,FV(G)−1/n≤eaV+ebV−1nXΣ,FV(G). |
In a similar way,
eaE+bE−1m∑uv∈E(G)logFE(du,dv)≤eaE+ebE−1m∑uv∈E(G)elogFE(du,dv),eaE+bEelog(Πuv∈E(G)FE(du,dv))−1/m≤eaE+ebE−1m∑uv∈E(G)FE(du,dv),eaE+bEXΠ,FE(G)−1/m≤eaE+ebE−1mXΣ,FE(G). |
Theorem 2.3 provides the following new lower bounds of Π1 and Π∗1.
Corollary 2.3. Let G be a graph with n vertices and m edges, minimum degree δ, and maximum degree Δ. Then,
Π1(G)≥(e−δ2+e−Δ2−e−δ2−Δ2nM1(G))−n,Π∗1(G)≥(e−2δ+e−2Δ−e−2δ−2ΔmM1(G))−m. |
Proof. Since δ2≤d2u≤Δ2 and 2δ≤du+dv≤2Δ, Theorem 2.3 implies
1nM1(G)≤eδ2+eΔ2−eδ2+Δ2Π1(G)−1/n,Π1(G)≥(e−δ2+e−Δ2−e−δ2−Δ2nM1(G))−n,1mM1(G)≤e2δ+e2Δ−e2δ+2ΔΠ∗1(G)−1/m,Π∗1(G)≥(e−2δ+e−2Δ−e−2δ−2ΔmM1(G))−m. |
The following Kober's inequalities in [23] (see also [24, Lemma 1]) are useful.
Lemma 2.1. If aj>0 for 1≤j≤k, then
k∑j=1aj+k(k−1)(k∏j=1aj)1/k≤(k∑j=1√aj)2≤(k−1)k∑j=1aj+k(k∏j=1aj)1/k. |
Lemma 2.1 has the following consequence.
Theorem 2.4. Let G be a graph with n vertices and m edges. Then,
XΣ,F2V(G)+n(n−1)XΠ,FV(G)2/n≤XΣ,FV(G)2≤(n−1)XΣ,F2V(G)+nXΠ,FV(G)2/n,XΣ,F2E(G)+m(m−1)XΠ,FE(G)2/m≤XΣ,FE(G)2≤(m−1)XΣ,F2E(G)+mXΠ,FE(G)2/m. |
Proof. Lemma 2.1 gives
∑u∈V(G)FV(du)2+n(n−1)(∏u∈V(G)FV(du)2)1/n≤(∑u∈V(G)FV(du))2,XΣ,F2V(G)+n(n−1)XΠ,FV(G)2/n≤XΣ,FV(G)2, |
and
(∑u∈V(G)FV(du))2≤(n−1)∑u∈V(G)FV(du)2+n(∏u∈V(G)FV(du)2)1/n,XΣ,FV(G)2≤(n−1)XΣ,F2V(G)+nXΠ,FV(G)2/n. |
In a similar way, replacing FV(du) and n with FE(du,dv) and m, respectively, we obtain
∑uv∈E(G)FE(du,dv)2+m(m−1)(∏uv∈E(G)FE(du,dv)2)1/m≤(∑uv∈E(G)FE(du,dv))2,XΣ,F2E(G)+m(m−1)XΠ,FE(G)2/m≤XΣ,FE(G)2, |
and
(∑uv∈E(G)FE(du,dv))2≤(m−1)∑uv∈E(G)FE(du,dv)2+m(∏uv∈E(G)FE(du,dv)2)1/m,XΣ,FE(G)2≤(m−1)XΣ,F2E(G)+mXΠ,FE(G)2/m. |
Let us recall the following known Petrović inequality [25].
Theorem 2.5. Let φ be a convex function on [0,a], and w1,…,wn≥0. If t1,…,tn∈[0,a] satisfy ∑nk=1tkwk∈(0,a], and
n∑k=1tkwk≥tj,j=1,…,n, |
then
n∑k=1φ(tk)wk≤φ(n∑k=1tkwk)+(n∑k=1wk−1)φ(0). |
Theorem 2.5 has the following consequence.
Proposition 2.3. Let φ be a convex function on [0,a]. If t1,…,tn∈[0,a] satisfy ∑nk=1tk∈(0,a], then
n∑k=1φ(tk)≤φ(n∑k=1tk)+(n−1)φ(0). |
Proposition 2.3 allows for the proof of the following inequalities.
Theorem 2.6. Let G be a graph with n vertices and m edges. Then,
XΣ,FV(G)≤XΠ,FV(G)+n−1,XΣ,FE(G)≤XΠ,FE(G)+m−1. |
Proof. Let us consider the convex function φ(x)=ex. Proposition 2.3 gives
XΣ,FV(G)=∑u∈V(G)FV(du)=∑u∈V(G)elogFV(du)≤e∑u∈V(G)logFV(du)+n−1=elogΠu∈V(G)FV(du)+n−1=Πu∈V(G)FV(du)+n−1=XΠ,FV(G)+n−1. |
In a similar way,
XΣ,FE(G)=∑uv∈E(G)FE(du,dv)=∑uv∈E(G)elogFE(du,dv)≤e∑uv∈E(G)logFE(du,dv)+m−1=elogΠuv∈E(G)FE(du,dv)+m−1=Πuv∈E(G)FE(du,dv)+m−1=XΠ,FE(G)+m−1. |
Theorem 2.7. Let G be a graph. Then,
XΠ,FV(G)≥XΣ,logFV(G)+1,XΠ,FE(G)≥XΣ,logFE(G)+1. |
Proof. The equality ex≥x+1 holds for every x∈R. This inequality gives
XΠ,FV(G)=elogXΠ,FV(G)≥logXΠ,FV(G)+1=log(Πu∈E(G)FV(du))+1=∑u∈E(G)logFV(du)+1=XΣ,logFV(G)+1. |
The same argument gives the second inequality.
In [26], the Gutman-Milovanović index is defined as
Mα,β(G)=∑uv∈E(G)(dudv)α(du+dv)β, |
which is a natural generalization of Zagreb indices.
Next, we present an inequality relating some multiplicative indices and the Gutman-Milovanović index.
Theorem 2.8. Let G be a graph with m edges, and α,β∈R. Then,
Mα,β(G)≥mΠ2(G)α/mΠ∗1(G)β/m. |
The equality is attained for any α,β if G is regular.
Proof. Since the geometric mean is at most the arithmetic mean, we get
1mMα,β(G)=1m∑uv∈E(G)(dudv)α(du+dv)β≥(∏uv∈E(G)(dudv)α(du+dv)β)1/m=Π2(G)α/mΠ∗1(G)β/m. |
Thus,
Mα,β(G)≥mΠ2(G)α/mΠ∗1(G)β/m. |
If the graph is δ-regular, then Mα,β(G)=2βδ2α+βm, Π2(G)α/m=(δ2m)α/m=δ2α, Π∗1(G)β/m=((2δ)m)β/m=2βδβ, and the equality holds.
We have some direct formulas for some multiplicative indices, e.g.,
Π1(G)=NK(G)2,Π2(G)=RΠ(G)−2,Π∗1(G)=2−mHΠ(G)−1,Π∗1(G)=SΠ(G)−2,HΠ(G)=2mSΠ(G)2, |
for every graph G with m edges.
Let us show some inequalities relating different multiplicative indices.
Theorem 2.9. Let G be a graph with m edges, maximum degree Δ and minimum degree δ. Then,
2mΔmΠ2(G)≤Π∗1(G)≤2mδmΠ2(G),(2√ΔδΔ+δ)mRΠ(G)≤HΠ(G)≤RΠ(G),(2δ2)mΠ2(G)−2≤IDΠ(G)≤(2Δ2)mΠ2(G)−2,2mΠ2(G)−1≤IDΠ(G)≤(Δ2+δ2Δδ)mΠ2(G)−1. |
Furthermore, the equality in each inequality is attained for every regular graph.
Proof. First of all, note that if
c≤FE(x,y)αF′E(x,y)β≤C |
for every δ≤x,y≤Δ, then we have for every uv∈E(G),
cF′E(du,dv)β≤FE(du,dv)α≤CF′E(du,dv)β,cm(∏uv∈E(G)F′E(du,dv))β≤(∏uv∈E(G)FE(du,dv))α≤Cm(∏uv∈E(G)F′E(du,dv))β,cmXΠ,F′E(G)β≤XΠ,FE(G)α≤CmXΠ,F′E(G)β, |
for every graph G with m edges, maximum degree Δ, and minimum degree δ.
Since
2Δ≤1x+1y=x+yxy≤2δ, |
we have
2mΔmΠ2(G)≤Π∗1(G)≤2mδmΠ2(G). |
Since
2√ΔδΔ+δ≤2√xyx+y=2x+y1√xy≤1, |
we have
(2√ΔδΔ+δ)mRΠ(G)≤HΠ(G)≤RΠ(G). |
Since
2δ2≤x2+y2=1x2+1y2(xy)−2≤2Δ2, |
we have
(2δ2)mΠ2(G)−2≤IDΠ(G)≤(2Δ2)mΠ2(G)−2. |
Let us consider the function
A(x,y)=1x2+1y21xy=xy+yx=f(t), |
where
t=xy,f(t)=t+1t. |
Since
f′(t)=1−1t2=t2−1t2 |
f is decreasing on (0,1] and increasing on [1,∞). Since f(1)=2, we conclude
2≤A(x,y)≤Δ2+δ2Δδ. |
Hence,
2mΠ2(G)−1≤IDΠ(G)≤(Δ2+δ2Δδ)mΠ2(G)−1. |
Let G be a δ-regular graph. Hence,
Π2(G)=δ2m,Π∗1(G)=(2δ)m,RΠ(G)=δ−m,HΠ(G)=δ−m,IDΠ(G)=2mδ−2m,2√ΔδΔ+δ=1,Δ2+δ2Δδ=2. |
Therefore, the equality in each inequality is attained for every regular graph.
Recall that a biregular graph is a bipartite graph for which any vertex in one side of the given bipartition has degree Δ and any vertex in the other side of the bipartition has degree δ. We say that a graph is (Δ,δ)-biregular if we want to write explicitly the maximum and minimum degrees.
Theorem 2.10. If G is a graph with m edges, minimum degree δ, and maximum degree Δ, then
23mΠ∗1(G)−2≤IDΠ(G)≤(Δ+δ)2m(Δ−2+δ−2)mΠ∗1(G)−2. |
The equality in the lower bound is attained if and only if each connected component of G is a regular graph. The equality in the upper bound is attained if and only if G is a regular or biregular graph.
Proof. Define
A(x,y):=x−2+y−2(x+y)−2=(x+y)2(x−2+y−2), |
for x,y∈[δ,Δ]. Note that, in order to find the bounds of A, it suffices to consider the case y≥x. We have
∂A∂y(x,y)=2(x+y)(x−2+y−2)−2(x+y)2y−3=2x−2y−3(x+y)(y3+x2y−x3−x2y)=2x−2y−3(x+y)(y3−x3)>0, |
for every y∈(x,Δ]. Hence, A(x,x)≤A(x,y)≤A(x,Δ). Note that A(x,x)=8.
Define
B(x)=A(x,Δ)=(x+Δ)2(x−2+Δ−2), |
for x∈[δ,Δ]. We get
B′(x)=2Δ−2x−3(x+Δ)(x3−Δ3)<0, |
for every x∈[δ,Δ). Therefore,
A(x,Δ)=B(x)≤B(δ)=(Δ+δ)2(Δ−2+δ−2), |
for x∈[δ,Δ]. Hence,
8≤A(x,y)≤(Δ+δ)2(Δ−2+δ−2), |
and so,
23mΠ∗1(G)−2≤IDΠ(G)≤(Δ+δ)2m(Δ−2+δ−2)mΠ∗1(G)−2. |
The previous argument gives that the equality in the lower bound is attained if and only if du=dv for every uv∈E(G), and this happens if and only if each connected component of G is a regular graph.
Also, the equality in the upper bound is attained if and only if {du,dv}={Δ,δ} for every uv∈E(G), and this happens if and only if G is a regular (if Δ=δ) or (Δ,δ)-biregular (if Δ≠δ) graph.
The statistical properties of topological indices in random networks have been intensively studied, see, e.g., [27,28]. Within this statistical approach, it has been recently shown that the average values of indices of the type XΣ(G), normalized to the order of the network n, scale with the average degree ⟨d⟩, see, e.g., [15,16,17,18]. That is, ⟨XΣ(G)⟩/n is a function of ⟨d⟩ only. Moreover, it was also found that ⟨XΣ(G)⟩, for indices like R(G) and H(G), is highly correlated with the Shannon entropy of the eigenvectors of the adjacency matrix of random networks [19]. This is a notable result because it puts forward the application of topological indices beyond mathematical chemistry. Specifically, given the equivalence of the Hamiltonian of a tight-binding network (in the proper setup) and the corresponding network adjacency matrix, either ⟨R(G)⟩ or ⟨H(G)⟩ could be used to determine the eigenvector localization and delocalization regimes which in turn determine the insulator and metallic regimes of quantum transport.
Below, for the first time to our knowledge, we apply the MTIs of Eqs (1.9)–(1.16) on three models of random networks: Erdös-Rényi (ER) networks, random geometric (RG) graphs, and bipartite random (BR) networks.
ER networks [29,30,31] GER(n,p) are formed by n vertices connected independently with probability p∈[0,1]. While RG graphs [32,33] GRG(n,r) consist of n vertices uniformly and independently distributed on the unit square, where two vertices are connected by an edge if their Euclidean distance is less than or equal to the connection radius r∈[0,√2]. In addition, we examine BR networks GBR(n1,n2,p) composed of two disjoint sets, set 1 and set 2, with n1 and n2 vertices each such that there are no adjacent vertices within the same set, being n=n1+n2 the total number of vertices in the bipartite network. The vertices of the two sets are connected randomly with probability p∈[0,1].
Before computing MTIs on ER random networks, we note that in the dense limit, i.e., when ⟨d⟩≫1, we can approximate du≈dv≈⟨d⟩ in Eqs (1.9)–(1.16), with
⟨d⟩=(n−1)p. | (3.1) |
Thus, for example, when np≫1, we can approximate NKΠ(GER) as
NK(GER)=∏u∈V(G)du≈∏u∈V(G)⟨d⟩≈⟨d⟩n, |
which leads us to
lnNK(GER)≈nln⟨d⟩ |
or
lnNK(GER)n≈ln⟨d⟩. | (3.2) |
A similar approximation gives
lnΠ1(GER)n≈2ln⟨d⟩. | (3.3) |
Also, for Π2(GER), we have that
Π2(GER)=∏uv∈E(G)dudv≈∏uv∈E(G)⟨d⟩⟨d⟩=∏uv∈E(G)⟨d⟩2≈⟨d⟩n⟨d⟩ |
where we have used ∣E(GER)∣=n⟨d⟩/2. Therefore,
lnΠ2(GER)≈n⟨d⟩ln⟨d⟩ |
or
lnΠ2(GER)n≈⟨d⟩ln⟨d⟩. | (3.4) |
Following this procedure, for the rest of the MTIs of Eqs (1.12)–(1.16) we get:
lnΠ∗1(GER)n≈12⟨d⟩ln(2⟨d⟩), | (3.5) |
lnRΠ(GER)n≈−12⟨d⟩ln⟨d⟩, | (3.6) |
lnHΠ(GER)n≈−12⟨d⟩ln⟨d⟩, | (3.7) |
lnχΠ(GER)n≈−ln24⟨d⟩−14⟨d⟩ln⟨d⟩, | (3.8) |
and
lnIDΠ(GER)n≈ln22⟨d⟩−⟨d⟩ln⟨d⟩. | (3.9) |
From the approximate expressions above we note that the logarithm of the MTIs on ER random networks, normalized to the network size n, does not depend on n. That is, in the dense limit, we expect lnXΠ(GER)/n to depend on ⟨d⟩ only; here, XΠ represents all the MTIs of Eqs (1.9)–(1.16).
With Eqs (3.2)–(3.9) as a guide, in what follows we compute the average values of the logarithm of the MTIs listed in Eqs (1.9)–(1.16). All averages are computed over ensembles of 107/n ER networks characterized by the parameter pair (n,p).
In Figure 1 we present the average logarithm of the eight MTIs of Eqs (1.9)–(1.16) as a function of the probability p of ER networks of sizes n={125,250,500,1000}. Since ⟨lnXΠ(GER)⟩<0, for RΠ, HΠ, and χΠ we conveniently plotted −⟨lnXΠ(GER)⟩ in log scale to have a detailed view of the data for small p, see Figure 1(e–g). Thus, we observe that ⟨lnXΠ(GER)⟩ for NK, Π1,2, and Π∗1 [for RΠ, HΠ, and χΠ] is a monotonically increasing [monotonically decreasing] function of p. In contrast, ⟨lnIDΠ(GER)⟩ is a nonmonotonic function of p, see Figure 1(h).
Then, following Eqs (3.2)–(3.9), in Figure 2 we show again the average logarithm of the MTIs, but now normalized to the network size, ⟨lnXΠ(GER)⟩/n, as a function of ⟨d⟩. As can be clearly observed in this figure, the curves ⟨lnXΠ(GER)⟩/n versus ⟨d⟩ fall one on top of the other for different network sizes; so, these indices are properly scaled where ⟨d⟩ works as the scaling parameter. That is, ⟨d⟩ is the parameter that fixes the average values of the normalized MTIs on ER networks. It is remarkable that the scaling of ⟨lnXΠ(GER)⟩/n with ⟨d⟩, expected for ⟨d⟩≫1 according to Eqs (3.2)–(3.9), works perfectly well for all values of ⟨d⟩; even for ⟨d⟩≪1. Since the topological properties of random graphs and networks, when characterized by indices of the form XΣ(G), have been shown to scale with ⟨d⟩ for all values of ⟨d⟩ (see e.g., [15,16,17,18,19]), we should expect other topological measures to also scale with ⟨d⟩, such as the MTIs studied here. Also, in Figure 2, we show that Eqs (3.2)–(3.9) (orange-dashed lines) indeed describe well the data (thick full curves) for ⟨d⟩≥10, which can be regarded as the dense limit of the ER model.
As in the previous Subsection, here we start by exploring the dense limit. Indeed, for RG graphs in the dense limit, i.e., when ⟨d⟩≫1, we can approximate du≈dv≈⟨d⟩, where
⟨d⟩=(n−1)g(r) | (3.10) |
and [34]
g(r)={r2[π−83r+12r2],0≤r≤1,13−2r2[1−arcsin(1/r)+arccos(1/r)]+43(2r2+1)√r2−1−12r4,1≤r≤√2. | (3.11) |
Thus, we obtain
lnNK(GRG)n≈ln⟨d⟩, | (3.12) |
lnΠ1(GRG)n≈2ln⟨d⟩, | (3.13) |
lnΠ2(GRG)n≈⟨d⟩ln⟨d⟩, | (3.14) |
lnΠ∗1(GRG)n≈12⟨d⟩ln(2⟨d⟩), | (3.15) |
lnRΠ(GRG)n≈−12⟨d⟩ln⟨d⟩, | (3.16) |
lnHΠ(GRG)n≈−12⟨d⟩ln⟨d⟩, | (3.17) |
lnχΠ(GRG)n≈−ln24⟨d⟩−14⟨d⟩ln⟨d⟩, | (3.18) |
and
lnIDΠ(GRG)n≈ln22⟨d⟩−⟨d⟩ln⟨d⟩. | (3.19) |
Remarkably, the approximate Eqs (3.12)–(3.19) for RG graphs are exactly the same as the corresponding equations for ER graphs, see Eqs (3.2)–(3.9); however, note that the definition of ⟨d⟩ is different for both models, i.e., compare Eqs (3.1), (3.10), and (3.11).
Then, in Figure 3 we present the average logarithm of the eight MTIs of Eqs (1.9)–(1.16) as a function of the connection radius r of RG graphs of sizes n={125,250,500,1000}. All averages are computed over ensembles of 107/n random graphs, and each ensemble is characterized by a fixed parameter pair (n,r).
For comparison purposes, Figure 1 for ER networks and Figure 3 for RG graphs have the same format and contents. In fact, all the observations made in the previous Subsection for ER networks are also valid for RG graphs by replacing GER→GRG and p→g(r). Therefore, in Figure 4 we plot ⟨lnXΠ(GRG)⟩/n as a function of ⟨d⟩ showing that all curves are properly scaled; that is, curves for different graph sizes fall on top of each other. Also, in Figure 4 we show that Eqs (3.12)–(3.19) (orange-dashed lines) indeed describe the data well (thick full curves) for ⟨d⟩≥10, which can be regarded as the dense limit of RG graphs.
We start by writing approximate expressions for the MTIs on BR networks in the dense limit. Moreover, since edges in a bipartite network join vertices of different sets, and we are labeling here the sets as set 1 and set 2, we replace du by d1 and dv by d2 in the expression for the MTIs. Thus, when n1p≫1 and n2p≫1, we can approximate du=d1≈⟨d1⟩ and dv=d2≈⟨d2⟩ in Eqs (1.9)–(1.16), with
⟨d1,2⟩=n2,1p. | (3.20) |
Therefore, in the dense limit, the MTIs of Eqs (1.11)–(1.16) on BR networks are well approximated by
lnΠ2(GBR)n1,2≈⟨d1,2⟩ln(⟨d1⟩⟨d2⟩), | (3.21) |
lnΠ∗1(GBR)n1,2≈⟨d1,2⟩ln(⟨d1⟩+⟨d2⟩), | (3.22) |
lnRΠ(GBR)n1,2≈−12⟨d1,2⟩ln(⟨d1⟩⟨d2⟩), | (3.23) |
lnHΠ(GBR)n1,2≈⟨d1,2⟩[ln2−ln(⟨d1⟩+⟨d2⟩)], | (3.24) |
lnχΠ(GBR)n1,2≈−12⟨d1,2⟩ln(⟨d1⟩+⟨d2⟩), | (3.25) |
and
lnIDΠ(GBR)n1,2≈⟨d1,2⟩ln(1⟨d1⟩2+1⟨d2⟩2). | (3.26) |
Above we used ∣E(GBR)∣=n1n2p=n1,2⟨d1,2⟩. We note that we are not providing approximate expressions for neither NK(GBR) nor Π1(GBR).
Now we compute MTIs on ensembles of 107/n BR networks. In contrast to ER and RG networks, now the BR network ensembles are characterized by three parameters: n1, n2, and p. For simplicity, but without lost of generality, in our numerical calculations we consider n1=n2. It is remarkable to notice that, in the case of n1=n2=n/2, where ⟨d1⟩=⟨d2⟩=⟨d⟩=np/2, Eqs (3.21)–(3.26) reproduce Eqs (3.4)–(3.9).
Then, in Figure 5 we present the average logarithm of the eight MTIs of Eqs (1.9)–(1.16) as a function of the probability p of BR networks of sizes n1=n2={125,250,500,1000}. Therefore, by plotting ⟨lnXΠ(GBR)⟩/n as a function of ⟨d⟩ (see Figure 6), we confirm that the curves of the MTIs on BR networks are properly scaled, as predicted by Eqs (3.21)–(3.26); see the orange dashed lines in Figure 6 (c–h). Here, we can also say that ⟨d⟩≥10 can be regarded as de dense limit of BR networks. We have verified (not shown here) that we arrive at equivalent conclusions when n1≠n2.
Note that in panels (a, b) of Figure 6 we included Eqs (3.2) and (3.3) as orange dashed lines. Those equations were obtained for ER networks, however they reproduce perfectly well both NK(GBR) and Π1(GBR) when ⟨d⟩≥10 since we are considering n1=n2=n/2.
In this work we have performed a thorough analytical and statistical study of multiplicative topological indices (MTIs) XΠ.
From an analytical viewpoint, we found several inequalities that relate MTIs among themselves as well as to their additive versions XΣ. To find inequalities between different indices is a classical topic in the study of topological indices.
Within a statistical approach we computed MTIs on random networks. Some previous works deal with the statistical analysis of (non-multiplicative) topological indices. As models of random networks we have used Erdös-Rényi (ER) networks, random geometric (RG) graphs, and bipartite random (BR) networks. We showed that the average logarithm of the MTIs, ⟨lnXΠ(G)⟩, normalized to the order of the network, scale with the network average degree ⟨d⟩. Thus, we conclude that ⟨d⟩ is the parameter that fixes the average values of the logarithm of the MTIs on random networks. Moreover, the equivalence among Eqs (3.2)–(3.9) for ER networks, Eqs (3.12)–(3.19) for RG graphs, and Eqs (3.21)–(3.26) for BR networks (when the subsets are equal in size) allows us to state a scaling law that connects the three graph models. That is, given the MTI XΠ, the average of its logarithm divided by the network size is the same function of the average degree regardless the network model:
⟨lnXΠ(GER)⟩n≈⟨lnXΠ(GRG)⟩n≈⟨lnXΠ(GBR)⟩n≈f(⟨d⟩). | (4.1) |
To validate Eq (4.1), without loss of generality we choose three MTIs: the Narumi-Katayama index, the multiplicative sum-connectivity index, and the multiplicative inverse degree index. We apply these indices to ER networks, RG graphs, and BR networks and plot ⟨lnNKΠ(G)⟩/n, −⟨lnχΠ(G)⟩/n and ⟨lnIDΠ(G)⟩/n in Figure 7. There, for each index, we can clearly see that the curves corresponding to the three network models fall one on top of the other, thus validating Eq (4.1). Also, notice that we are using networks of different sizes to stress the scaling law in Eq (4.1). We observe the same scaling behavior in all the other MTIs of Eqs (1.10)–(1.14) (not shown here).
Finally, it is fair to mention that we found that the multiplicative version of the geometric-arithmetic index GAΠ(G) on random networks does not scale with the average degree. This situation is quite unexpected for us since all other MTIs we study here scale with ⟨d⟩. In addition, our previous statistical and theoretical studies of topological indices of the form XΣ(G) (see Eq (1.1)), showed that the normalized geometric-arithmetic index GAΣ(G) scales with ⟨d⟩ [16]. Thus, we believe that the multiplicative index GAΠ(G) on random graphs requires further analysis.
Augmented Reality (AR) algorithms have shown rapid development in recent years when applied to the study of different networks, such as neural and communication networks (see e.g., [35,36]). Therefore, it would be interesting to apply AR techniques in the analysis of random networks.
We hope that our work may motivate further analytical and computational studies of MTIs.
The authors declare they have not used Artificial Intelligence (AI) tools in the creation of this article.
This publication is part of the grant PID2019-106433GB-I00 funded by Agencia Estatal de Investigación MCIN/AEI/ 10.13039/501100011033. J.A.M.-B. thanks support from CONACyT (Grant No. 286633), CONACyT-Fronteras (Grant No. 425854), and VIEP-BUAP (Grant No. 100405811-VIEP2023), Mexico. J.M.R. was supported by the Madrid Government (Comunidad de Madrid-Spain) under the Multiannual Agreement with UC3M in the line of Excellence of University Professors (EPUC3M23), and in the context of the V PRICIT (Regional Programme of Research and Technological Innovation).
We would like to thank the referees for their comments which have improved the presentation of the paper.
Prof. Jose M. Rodriguez is the Guest Editor of special issue "Graph theory and its applications" for AIMS Mathematics. Prof. Jose M. Rodriguez was not involved in the editorial review and the decision to publish this article.
All authors declare no conflicts of interest in this paper.
[1] |
T. Alladi, V. Kohli, V. Chamola, F. R. Yu, A deep learning-based misbehavior classification scheme for intrusion detection in cooperative intelligent transportation systems, Digit. Commun. Netw., 9 (2023), 1113–1122. https://doi.org/10.1016/j.dcan.2022.06.018 doi: 10.1016/j.dcan.2022.06.018
![]() |
[2] | K. H. Park, E. Park, H. K. Kim, Unsupervised intrusion detection system for unmanned aerial vehicle with less labeling effort. In: Information security applications: 21st international conference, Springer, Cham. 2020, 45–58. https://doi.org/10.1007/978-3-030-65299-9_4 |
[3] | S. Jeong, E. Park, K. U. Seo, J. Do Yoo, H. K. Kim, MUVIDS: false MAVLink injection attack detection in communication for unmanned vehicles. In: Workshop on automotive and autonomous vehicle security (AutoSec), 2021. https://doi.org/10.14722/autosec.2021.23036 |
[4] |
D. Basavaraj, S. Tayeb, Towards a lightweight intrusion detection framework for in-vehicle networks, J. Sensor Actuator Netw., 11 (2022), 6. https://doi.org/10.3390/jsan11010006 doi: 10.3390/jsan11010006
![]() |
[5] |
P. Mansourian, N. Zhang, A. Jaekel, M. Kneppers, Deep learning-based anomaly detection for connected autonomous vehicles using spatiotemporal information, IEEE T. Intell. Transp. Syst., 24 (2023), 16006–16017. https://doi.org/10.1109/TITS.2023.3286611 doi: 10.1109/TITS.2023.3286611
![]() |
[6] |
L. Yang, A. Moubayed, A. Shami, MTH-IDS: A multitiered hybrid intrusion detection system for internet of vehicles, IEEE Internet Things J., 9 (2022), 616–632. https://doi.org/10.1109/JIOT.2021.3084796 doi: 10.1109/JIOT.2021.3084796
![]() |
[7] | N. Vanitha, P. Ganapathi, Traffic analysis of UAV networks using enhanced deep feed forward neural networks (EDFFNN). In: Handbook of research on machine and deep learning applications for cyber security. 2020. https://doi.org/10.4018/978-1-5225-9611-0.ch011 |
[8] |
S. Aziz, M. T. Faiz, A. M. Adeniyi, K. H. Loo, K. N. Hasan, L. Xu, et al., Anomaly detection in the internet of vehicular networks using explainable neural networks (xnn), Mathematics, 10 (2022), 1267. https://doi.org/10.3390/math10081267 doi: 10.3390/math10081267
![]() |
[9] |
S. Anbalagan, G. Raja, S. Gurumoorthy, R. D. Suresh, K. Dev, ⅡDS: Intelligent intrusion detection system for sustainable development in autonomous vehicles, IEEE T. Intell. Transp. Syst., 24 (2023), 15866–15875. https://doi.org/10.1109/TITS.2023.3271768 doi: 10.1109/TITS.2023.3271768
![]() |
[10] |
R. Fotohi, E. Nazemi, F. S. Aliee, An agent-based self-protective method to secure communication between UAVs in unmanned aerial vehicle networks, Veh. Commun., 26 (2020), 100267. https://doi.org/10.1016/j.vehcom.2020.100267 doi: 10.1016/j.vehcom.2020.100267
![]() |
[11] |
F. Kateb, M. Ragab, Archimedes optimization with deep learning based aerial image classification for cybersecurity enabled UAV networks, Comput. Syst. Sci. Eng., 47 (2023), 2171–2185. https://doi.org/10.32604/csse.2023.039931 doi: 10.32604/csse.2023.039931
![]() |
[12] |
M. A. Sayeed, R. Kumar, V. Sharma, Safeguarding unmanned aerial systems: An approach for identifying malicious aerial nodes, IET Commun., 14 (2020), 3000–3012. https://doi.org/10.1049/iet-com.2020.0073 doi: 10.1049/iet-com.2020.0073
![]() |
[13] |
J. Tao, T. Han, R. Li, Deep-reinforcement-learning-based intrusion detection in aerial computing networks, IEEE Netw., 35 (2021), 66–72. https://doi.org/10.1109/MNET.011.2100068 doi: 10.1109/MNET.011.2100068
![]() |
[14] |
A. Masadeh, M. Alhafnawi, H. A. B. Salameh, A. Musa, Y. Jararweh, Reinforcement learning-based security/safety uav system for intrusion detection under dynamic and uncertain target movement, IEEE T. Eng. Manage., 2022, 1–11. https://doi.org/10.1109/TEM.2022.3165375 doi: 10.1109/TEM.2022.3165375
![]() |
[15] |
R. Kumar, P. Kumar, R. Tripathi, G. P. Gupta, T. R. Gadekallu, G. Srivastava, SP2F: A secured privacy-preserving framework for smart agricultural Unmanned Aerial Vehicles, Comput. Netw., 187 (2021), 107819. https://doi.org/10.1016/j.comnet.2021.107819 doi: 10.1016/j.comnet.2021.107819
![]() |
[16] |
L. Almutairi, R. Daniel, S. Khasimbee, E. L. Lydia, S. Acharya, H. Kim, Quantum dwarf mongoose optimization with ensemble deep learning based intrusion detection in cyber-physical systems, IEEE Access, 11 (2023), 66828–66837. https://doi.org/10.1109/ACCESS.2023.3287896 doi: 10.1109/ACCESS.2023.3287896
![]() |
[17] | I. U. Khan, A. Abdollahi, M. A. Khan, I. Uddin, I. Ullah, Securing against DoS/DDoS attacks in internet of flying things using experience-based deep learning algorithm, 2021. (Preprint) https://doi.org/10.21203/rs.3.rs-271920/v1 |
[18] |
Q. Abu Al-Haija, A. Al Badawi, High-performance intrusion detection system for networked UAVs via deep learning, Neural Comput. Appl., 34 (2022), 10885–10900. https://doi.org/10.1007/s00521-022-07015-9 doi: 10.1007/s00521-022-07015-9
![]() |
[19] |
M. S. Minu, R. Aroul Canessane, S. S. Subashka Ramesh, Optimal squeeze net with deep neural network-based arial image classification model in Unmanned Aerial Vehicles, Traitement du Signal, 39 (2022), 275–281. https://doi.org/10.18280/ts.390128 doi: 10.18280/ts.390128
![]() |
[20] |
J. Tian, B Wang, R. Guo, Z. Wang, K. Cao, X. Wang, Adversarial attacks and defenses for deep-learning-based unmanned aerial vehicles, IEEE Internet Things J., 9 (2021), 22399–22409. https://doi.org/10.1109/JIOT.2021.3111024 doi: 10.1109/JIOT.2021.3111024
![]() |
[21] |
V. F. S. Francelin, J. Daniel, S. Velliangiri, Intelligent agent and optimization‐based deep residual network to secure communication in UAV network, Int. J. Intell. Syst., 37 (2022), 5508–5529. https://doi.org/10.1002/int.22800 doi: 10.1002/int.22800
![]() |
[22] |
H. Zhang, J. Xue, Q. Wang, Y. Li, A security optimization scheme for data security transmission in UAV-assisted edge networks based on federal learning, Ad Hoc Netw., 150 (2023), 103277. https://doi.org/10.1016/j.adhoc.2023.103277 doi: 10.1016/j.adhoc.2023.103277
![]() |
[23] |
A. M. Elshewey, M. Y. Shams, N. El-Rashidy, A. M. Elhady, S. M. Shohieb, Z. Tarek, Bayesian optimization with support vector machine model for Parkinson disease classification, Sensors, 23 (2023), 2085. https://doi.org/10.3390/s23042085 doi: 10.3390/s23042085
![]() |
[24] |
M. Stankovic, J. Gavrilovic, D. Jovanovic, M. Zivkovic, M. Antonijevic, N. Bacanin, et al., Tuning multi-layer perceptron by hybridized arithmetic optimization algorithm for healthcare 4.0, Procedia Comput. Sci., 215 (2022), 51–60. https://doi.org/10.1016/j.procs.2022.12.006 doi: 10.1016/j.procs.2022.12.006
![]() |
[25] |
H. Al-Khazraji, A. R. Nasser, A. M. Hasan, A. K. Al Mhdawi, H. Al-Raweshidy, A. J. Humaidi, Aircraft engines remaining useful life prediction based on a hybrid model of autoencoder and deep belief network, IEEE Access, 10 (2022), 82156–82163. https://doi.org/10.1109/ACCESS.2022.3188681 doi: 10.1109/ACCESS.2022.3188681
![]() |
[26] |
P. Krishnadoss, V. K. Poornachary, P. Krishnamoorthy, L. Shanmugam, Improvised seagull optimization algorithm for scheduling tasks in heterogeneous cloud environment, Comput. Mater. Con., 74 (2023), 2461–2478. https://doi.org/10.32604/cmc.2023.031614 doi: 10.32604/cmc.2023.031614
![]() |
[27] |
N. Moustafa, A new distributed architecture for evaluating AI-based security systems at the edge: Network TON_IoT datasets, Sustain. Cities Soc., 72 (2021), 102994. https://doi.org/10.1016/j.scs.2021.102994 doi: 10.1016/j.scs.2021.102994
![]() |
[28] |
R. A. Ramadan, A. H. Emara, M. Al-Sarem, M. Elhamahmy, Internet of drones intrusion detection using deep learning, Electronics, 10 (2021), 2633. https://doi.org/10.3390/electronics10212633 doi: 10.3390/electronics10212633
![]() |
[29] |
L. Kou, S. Ding, T. Wu, W. Dong, Y. Yin, An intrusion detection model for drone communication network in SDN environment, Drones, 6 (2022), 342. https://doi.org/10.3390/drones6110342 doi: 10.3390/drones6110342
![]() |
1. | Sadia Noureen, Akbar Ali, Akhlaq A. Bhatti, Abdulaziz M. Alanazi, Yilun Shang, Predicting enthalpy of formation of benzenoid hydrocarbons and ordering molecular trees using general multiplicative Zagreb indices, 2024, 10, 24058440, e30913, 10.1016/j.heliyon.2024.e30913 |