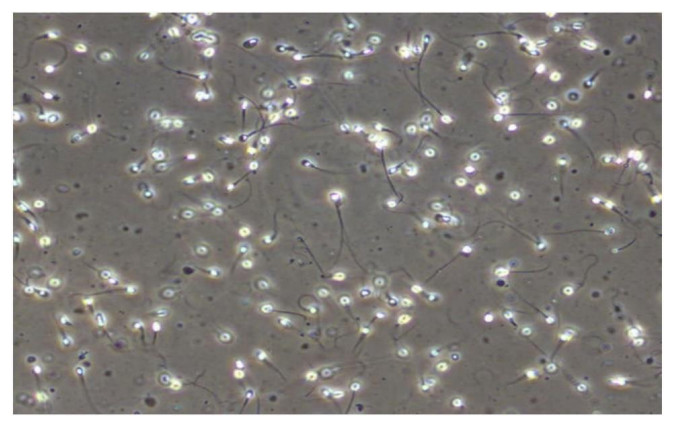
Sperm morphology analysis (SMA) is a significant factor in diagnosing male infertility. Therefore, healthy sperm detection is of great significance in this process. However, the traditional manual microscopic sperm detection methods have the disadvantages of a long detection cycle, low detection accuracy in large orders, and very complex fertility prediction. Therefore, it is meaningful to apply computer image analysis technology to the field of fertility prediction. Computer image analysis can give high precision and high efficiency in detecting sperm cells. In this article, first, we analyze the existing sperm detection techniques in chronological order, from traditional image processing and machine learning to deep learning methods in segmentation and classification. Then, we analyze and summarize these existing methods and introduce some potential methods, including visual transformers. Finally, the future development direction and challenges of sperm cell detection are discussed. We have summarized 44 related technical papers from 2012 to the present. This review will help researchers have a more comprehensive understanding of the development process, research status, and future trends in the field of fertility prediction and provide a reference for researchers in other fields.
Citation: Muhammad Suleman, Muhammad Ilyas, M. Ikram Ullah Lali, Hafiz Tayyab Rauf, Seifedine Kadry. A review of different deep learning techniques for sperm fertility prediction[J]. AIMS Mathematics, 2023, 8(7): 16360-16416. doi: 10.3934/math.2023838
[1] | Alaa O. Khadidos . Advancements in remote sensing: Harnessing the power of artificial intelligence for scene image classification. AIMS Mathematics, 2024, 9(4): 10235-10254. doi: 10.3934/math.2024500 |
[2] | Mesut GUVEN . Leveraging deep learning and image conversion of executable files for effective malware detection: A static malware analysis approach. AIMS Mathematics, 2024, 9(6): 15223-15245. doi: 10.3934/math.2024739 |
[3] | Mahmoud M. Abdelwahab, Khamis A. Al-Karawi, H. E. Semary . Integrating gene selection and deep learning for enhanced Autisms' disease prediction: a comparative study using microarray data. AIMS Mathematics, 2024, 9(7): 17827-17846. doi: 10.3934/math.2024867 |
[4] | Ibrahim M. Hezam, Osama Abdul-Raof, Abdelaziz Foul, Faisal Aqlan . A Quantum-Inspired Sperm Motility Algorithm. AIMS Mathematics, 2022, 7(5): 9057-9088. doi: 10.3934/math.2022504 |
[5] | Abdelwahed Motwake, Aisha Hassan Abdalla Hashim, Marwa Obayya, Majdy M. Eltahir . Enhancing land cover classification in remote sensing imagery using an optimal deep learning model. AIMS Mathematics, 2024, 9(1): 140-159. doi: 10.3934/math.2024009 |
[6] | Eman A. Al-Shahari, Marwa Obayya, Faiz Abdullah Alotaibi, Safa Alsafari, Ahmed S. Salama, Mohammed Assiri . Accelerating biomedical image segmentation using equilibrium optimization with a deep learning approach. AIMS Mathematics, 2024, 9(3): 5905-5924. doi: 10.3934/math.2024288 |
[7] | Mashael Maashi, Mohammed Abdullah Al-Hagery, Mohammed Rizwanullah, Azza Elneil Osman . Deep convolutional neural network-based Leveraging Lion Swarm Optimizer for gesture recognition and classification. AIMS Mathematics, 2024, 9(4): 9380-9393. doi: 10.3934/math.2024457 |
[8] | Álvaro Abucide, Koldo Portal, Unai Fernandez-Gamiz, Ekaitz Zulueta, Iker Azurmendi . Unsteady-state turbulent flow field predictions with a convolutional autoencoder architecture. AIMS Mathematics, 2023, 8(12): 29734-29758. doi: 10.3934/math.20231522 |
[9] | Youseef Alotaibi, Veera Ankalu. Vuyyuru . Electroencephalogram based face emotion recognition using multimodal fusion and 1-D convolution neural network (ID-CNN) classifier. AIMS Mathematics, 2023, 8(10): 22984-23002. doi: 10.3934/math.20231169 |
[10] | Ghazanfar Latif, Jaafar Alghazo, Majid Ali Khan, Ghassen Ben Brahim, Khaled Fawagreh, Nazeeruddin Mohammad . Deep convolutional neural network (CNN) model optimization techniques—Review for medical imaging. AIMS Mathematics, 2024, 9(8): 20539-20571. doi: 10.3934/math.2024998 |
Sperm morphology analysis (SMA) is a significant factor in diagnosing male infertility. Therefore, healthy sperm detection is of great significance in this process. However, the traditional manual microscopic sperm detection methods have the disadvantages of a long detection cycle, low detection accuracy in large orders, and very complex fertility prediction. Therefore, it is meaningful to apply computer image analysis technology to the field of fertility prediction. Computer image analysis can give high precision and high efficiency in detecting sperm cells. In this article, first, we analyze the existing sperm detection techniques in chronological order, from traditional image processing and machine learning to deep learning methods in segmentation and classification. Then, we analyze and summarize these existing methods and introduce some potential methods, including visual transformers. Finally, the future development direction and challenges of sperm cell detection are discussed. We have summarized 44 related technical papers from 2012 to the present. This review will help researchers have a more comprehensive understanding of the development process, research status, and future trends in the field of fertility prediction and provide a reference for researchers in other fields.
Human semen is a very complex biological fluid, a mixture of seminal plasma and sperm [1]. It is retrieved from multiple organs of a reproductive system. This fluid is different from other body fluids and contains peptides and proteins. These proteins play a vital role in fertilization, hence showing the overall quality of sperm.
Researchers have observed that human semen quality has declined gradually [2]. To seek the reason behind this, scientists perform semen analysis according to the rules given by World Health Organization (WHO), like assessing sperm concentration, semen volume, total sperm count, sperm morphology, sperm vitality and sperm motility [3]. Research shows that semen quality in men is decreasing gradually with time, the authors in [2,4] reviewed more than 60 papers and found out seminal fluid quantity and number of sperms in a given sample are declining in the past 50 years [2]. Many researchers compare a sample of different geographical areas, and a few studies different characteristics of sperm motility in their geographical regions. Swan et al studied 101 papers and figured out that reduced sperm count was due to different reasons in past years [4]. Huang et al examined more than 115 papers and tested about 23000 young Chinese men, concluding that there is a remarkable decrease in seminal plasma (SP) and concentration of complex biological fluid composed of sperms [5]. Another reason for human semen decline observed is environmental contaminants, also known as persistent organic pollutants (POPs) and polychlorinated biphenyls (PCBs). These are highly toxic industrial compounds and shows high health risk to humanity. PCBs are found in almost all parts of the world in fishery and dairy products, even in women's breast milk. Recent studies show that PCBs also affect the reproductive system in men and women, like miscarriage and infertility in men [6] One more factor of men's infertility or loss in sperm quality is the sexual abstinence period. The ejaculatory abstinence period may increase the motility and viability of sperms but damaged DNA due to increased RSO levels [7].
There are several machine learning techniques that can be used for sperm fertility prediction are Convolutional Neural Networks (CNNs): Due to their capacity to automatically extract characteristics from images, CNNs are frequently employed in image processing and recognition tasks [8].
Long Short-Term Memory Networks: In order to analyze time series data, recurrent neural networks of the LSTM variety have been employed. To simulate the evolution of sperm motility over time and forecast future sperm motility values, LSTMs can be employed in the context of sperm fertility prediction [9].
Generative Adversarial Networks: GANs are a kind of deep learning model that can create fresh samples by studying the distribution of the input data. GANs can be utilized to create artificial samples of sperm pictures for deep learning model training in the area of sperm fertility prediction [10].
Autoencoders: A particular kind of unsupervised deep learning model called an autoencoder is capable of learning a compressed representation of the input data. Autoencoders can be utilized in the context of sperm fertility prediction to reduce the dimensionality of the sperm image data and extract practical characteristics for classification [11].
Semen analysis is vital in predicting male fertility [12]. WHO sets some standards for semen analysis which include sperm motility, morphology and vitality etc. However, with the help of these guidelines, we cannot differentiate between fertile samples with unfertile samples. On the other hand, manual semen analysis is challenging, clinical results for male fertility prediction are uncertain, and a complex procedure requires trained medical staff and special equipment [13].
Therefore, researchers have developed automatic systems for semen analysis for several decades. After the digitization of images, Computer Aided Sperm Analysis (CASA) was introduced in the 1980s and made it possible to analyze the image using computer systems [14]. CASA provide a fast and objective assessment of sperm motility and concentration, but with low accuracy due to the presence of other particles in sperm samples, CASA is not recommended for clinical use. So, to improve methodology, Steven et al apply classical and modern machine learning techniques to the dataset of 85 videos and try to predict sperm motility automatically. Figure 1 shows extracted frame from a video dataset.
Urbano et al propose an automatic sperm tracking technique called a fully automated multi-sperm Tracking Algorithm. It can track hundreds of spermatozoa simultaneously [16]. Dewan et al. also proposes a technique that uses Convolutional Neural Network (CNN) to classify into sperm or non-sperm. Their results were evaluated on a close dataset, so they compared it with other approaches [17].
Graph Neural Networks (GNNs) [18] is an important tool for investigating graphs, which have become global in various applications, including recommendation systems, social networks, and computational biology [19]. Due to their effectiveness in handling a variety of graph-structured datasets, such as social networks, molecular structures, and 3D models, Graph Neural Networks (GNNs), a class of deep learning models, have gained increasing amounts of popularity in recent years. B. Jiang et al [20] introduce a new learning framework, termed Multiple Graph Learning Neural Networks (MGLNN), for performing multi-graph learning and semi-supervised classification across multiple views. The primary aim of MGLNN is to acquire the most optimum graph structure that can facilitate both the learning of GNNs and the integration of multiple graph structures in representation.
In previous research methodologies, CASA tool was used with classic image processing and machine learning. But in recent years, deep learning has played a vital role in many computer vision applications. For example, deep learning techniques became more capable of handling human behavior. Just like Robots, because they are now more capable of handling human behaviour with the help of deep learning techniques. Singh et al introduce Tinku, a socially intelligent robot with low operating costs that was created to teach special need children. Tinku is inexpensive, has a ton of features, and can make expressions akin to those of a human. The well-known animated character "WALL-E" served as inspiration for its design. Its features include offline speech processing and computer vision—for obstacle avoidance, non-verbal communication, anthropomorphic emotion expression, etc., author also employed light object detection models like Yolo v3-tiny and single shot detector (SSD). It localizes the items in the scene using an inbuilt deep learning approach and uses the data for semantic perception.
In our research, we evaluate the efficiency of modern machine learning and deep learning techniques over sperm microscopic videos of human sperm microscopic datasets/videos and related parameters to automate the prediction of human sperm fertility. The current study looks on the possibilities of deep learning approaches for predicting sperm fertility. The goal of this research is to create a model that is more accurate and trustworthy than current state-of-the-art models. The current work provides an in-depth examination of many deep learning approaches utilized in sperm fertility prediction. The review focuses on each technique's strengths and weaknesses, as well as potential for improvement.
Infertility is public health issue around the world that affect 15% of all men at the age of reproduction [21]. In male infertility, semen quality is a critical parameter that reduces daily. When couples are diagnosed with infertility, women undergo ovulation and tubal patency tests, and men need semen analysis. Semen analysis includes many factors that need to be examined, like sperm morphology, density and motility.
Semen quality is an essential parameter in determining male fertility and infertility. A fertile sample could provide us data from which researcher can classify a given sample as Normozoospermic (A normal sperm that can be fertile). Semen sample was taken from 4 continents, 14 countries and 4500 men and selected men as normozoospermic. Those men have selected whose wives have time to pregnancy (TTP) is 12 months.
According to WHO a fertile sample volume must be greater than 1.5 ml, total sperm count in one ejaculation near 39 million, sperm concentration 15 million per ml, energy 58%, progressive motility 32%, and total progressive and non-progressive motility must be 40% and morphology 4%. Research shows that human semen quality varies from time to time. According to WHO, three reasons are essential in sample variation [3].
a) Delay in sexual activity
b) Human error during testing
c) Inheritance
To make this process up to standard, researchers have to follow the guidelines by WHO. Global demographic population studies give us a better understanding of semen quality. Before experimenting in the laboratory, research needs to preserve semen sample; this process is called Cryopreservation [22]. But this process damage spermatozoa which cause reduces in semen quality. So, the researcher introduces an additional method called (sil-select density gradient centrifugation) before cryopreservation. The new method gives us more sperm count and motile count and less DNA fragmentation than conventional methods.
Minh Tam Le compares vitrification and conventional freezing procedure for preserving semen samples in terms of vitality, motility and morphology of a semen sample. After evaluating the results, they conclude that the conventional freezing preservation method is more effective than the vitrification method [23].
Another reason that affects semen quality is the sexual abstinence period. Delay in sexual activity increases sperm motility and viability but damages DNA due to an increase in reactive oxygen species (RSO) level [7].
Huang et al examined more than 115 papers and tested about 23000 young Chinese men, concluding that there is a remarkable decrease in the concentration of semen samples [5]. G Anifrandis et al also divide 13892 infertile men of different nationalities into two regions. One is called mena and the other is non-mena regions. This experiment is carried out in health care provider in Qatar. Results shows persons belonging to Mena region are more infertile than non-mena region [24].
Infertility also causes due to different diseases. Luca Boeri finds out Papillomavirus is one of them. In this research work, he tested 729 samples of infertile European men. He found that 15.5% of men are effect by Papillomavirus, and their sperm motility is lower than the of an average person that is not have Papillomavirus [25]. Avi Harlev uses Ritalinic Acid (RA), a drug to treat attention-deficit hyperactivity disorder. The author concludes that ritalinic acid increases sample concentration and sperm motility in the infertile semen sample. He also considers the effect of the drug on other parameters of a sample [26].
Fabiana Faja discusses the consequence of mitochondrial DNA on sperm motility. If they increase the quantity of mitochondrial genome, it helps to restore damages in the semen sample. The quality of the semen sample is based on the sperm count, which is an essential factor for predicting a person's fertility and infertility [27].
Based on these infertility factors, motion analysis of sperm is very important for determining male fertility. As the detection and tracking of sperms be performed more accurately, it results in a more accurate diagnosis of infertility problems. Usually, sperm analysis is a complex procedure done by experts by spotting numbers on sperm through a microscope, detecting their motion quality and morphology etc. [28].
Besides the manual way, Computer-aided sperm analysis (CASA) was introduced in 1980s for sperm analysis. It was a very successful software for measuring sperm characteristics like sperm concentration, and progressive and non-progressive motility in many animal species. But in the case of human semen analysis CASA tool does not show good results due to complications present in human seminal fluid [29].
So, researchers need more advanced methods for semen analysis, Hamza et al propose smart phone base hybrid system for automatic analysis of sperm morphology, as sperm morphology is an essential factor of male fertility. The author divided this procedure into two steps, ⅰ) classification of normal and abnormal sperm and ⅱ) automatic segmentation of sperm shape. Machine learning models have been used for the sake of classification. Moreover, deep neural network architectures were used for high-level feature extraction from raw images. Similarly, Mobile-Net, a very efficient network for smartphones that gave 87% accuracy. On the other hand, wave-let and descriptor-base features gave 80.5 % and 83.8% accuracy with the support vector machine, respectively [30].
Soroush et al also worked on sperm morphology analysis by malformation detection of sperm morphology in sperm image dataset and applied the deep learning algorithms. They performed their analysis on the images from MHSMA dataset were out of 1540 sperm images, and 235 infertile patients. Deformities were detected in different parts of human sperm using trained deep neural network architecture. Results show F0.5 scores of 83.86%, 84.74%, and 94.65% in head, acrosome and vacuole abnormality detection, respectively [31]. Furthermore, they used deep learning for the classification of sperms. They apply VGG16, a deep CNN architecture, to train on ImageNet and apply to freely available sperm image datasets. This technique shows efficient results against previously proposed techniques in head-to-head comparison and classify sperms with high accuracy [32].
Steven takes a dataset of 85 videos and applies modern and classical machine learning techniques to automate the prediction of sperm motility. He adopts convolutional neural networks with simple linear regression to do so. Results show that deep learning gives very quick and consistent output, and this analysis may become a valuable tool in male fertility prediction [33].
In this latest research work, the author uses RetinaNet, a deep, fully convolutional neural network, as an object detector. Sperms are microscopic objects with few characteristics, and it is challenging to identify millions of sperm with other particles in semen samples. One main characteristic of sperms is their movement. Still, only one image is not enough to detect the movement of sperm and consecutive images were needed to identify the movement and motility of sperm, as sperm motility is an essential factor for predicting male fertility. For sperm tracking, a modified CSR-DCF algorithm was used. This technique works very well even in high density of sperms, occlusions, sperm colliding, and when sperms exit from a frame and re-enter in the subsequent frames. Results show F1 score of the tracking method is 96.61% and the detection phase is 99.1% [34].
In the next section, we will discuss different techniques used by the researchers regarding dataset collection. These datasets and techniques are selected from different scientific databases published from 2012 to 2022, including ACM, Google Scholar, IEEE, Xplore, Springer etc. The selection procedure for datasets is explained in Figure 2.
Moreover, in Figure 3, we also discuss pre-processing techniques, feature extraction techniques, segmentation techniques, classification techniques and their evaluation matrices from the above-selected articles. For better understanding, we create different tables respectively. We further divided feature extraction and segmentation techniques into two tables, one for machine learning techniques and the second for deep learning techniques. But pre-processing, classification and evaluation metrics are discussed in one table.
In this section we discuss shorty about dataset used in above selected articles. Table 1 shows dataset name, its source, type either it is in image form or in video form, their number of instances, resolution and target class.
Ref | Dataset name | Source Link attach | Type | No. of instance | Color space | Resolution | classes |
[35] | - | - | Images | 10 to 15 | RGB | 576 × 764 | - |
[12] | Original images | http://dx.doi.org/10.1155/2013/687607 | Images | 160 | Gray Scale | - | 2 |
[36] | Public dataset | UCI data repository [14] | Images | 100 | - | - | 2 |
[37] | HSMA-DS | Images | 1457 | RGB | 576 × 764 | ||
[38] | Video dataset | Hospital Universiti Sains Malaysia (HUSM) | Video | 80 | |||
[39] | - | Images | 100 | 7 | 2 | ||
[40] | Video dataset | Andrology lab of the Academic Unit of Reproductive and Developmental Medicine (University of Sheffield) | video | 2040 × 1086 | |||
[41] | Video dataset | Hospital Universiti Sains Malaysia (HUSM) | Video | 20 | RGB | 480 × 640 | |
[42] | Gold-stadard dataset | Images | 19 | RGB | 780 × 580 | ||
[43] | - | Images | 886 | 2 | |||
[44] | Video dataset | Academic Unit of Reproductive and Develop mental Medicine (AURDM) | Video | 940 | 2040 × 1086 | 4 | |
[45] | SCIAN-MorphoSpermGS | Laboratory of Spermiogram, (ICBM), Faculty of Medicine, University of Chile. | Images | 7 × 7 | 5 | ||
[46] | HuSHeM | https://data.mendeley.com/datasets/tt3yj2pf38/1. | Images | 216 sperm head | RGB | 131×131 | 4 |
[47] | Isfahan Fertility and Infertility Center (IFIC) | Images | 170 sperm head | RGB | 720×576 | 4 | |
[48] | Images | 765 | Gray Scale | 1920×1440 | 2 | ||
[49] | Fertility Dataset |
UCI repository | Images | 100 | |||
[50] | Ocular images | Images | 13 | ||||
[51] | 3D+t | Images | 512 × 512 | ||||
[52] | Gold-standard dataset | Images | 20 | 780 × 580 | |||
[17] | Video dataset | Video | 90 frames at 30fps (3600 images) |
3200 × 2400 | 2 | ||
[33] | VISEM | [https://datasets.simula.no/viem/] | Video | 85 | 3 | ||
[53] | In-house dataset | https://figshare.com/articles/Deep_learningbased_selection_of_human_sperm_with_high_DNA_integrity/8124932 | Images | 1064 | |||
[32] | HuSHeM and SCIAN. | Images | 216 and 1132 |
Gray Scale and RGB | 5 | ||
[54] | Gold-standard dataset | Images | 20 | 780 × 580 | |||
[55] | Video dataset | Shenzhen Sixth People's Hospital, | Video | 54 | 720×540 | ||
[31] | MHSMA | [35] | Images | 1540 | RGB | 1280×1024 | 2 |
[56] | Video dataset | Royan institute Research Lab | Video | 36 | 768 × 576 | ||
[57] | - | SUMC | Images | 119 | 18 | ||
[58] | - | Avicenna Research Institute (Tehran, Iran) | 41 | 7 | |||
[59] | HuSHeM and SCIAN. | Images | 1154 and 1132 | Gray Scale | 5 | ||
[60] | Sperm Morphology Image Data Set (SMIDS) | Infertility centre of Istanbul University | Images | 200 | |||
[61] | Video dataset | Video | 288 × 352 | ||||
[62] | VISEM | https://datasets.simula.no/visem/ | Video | 85 | 640 × 480 | ||
[63] | SCIAN-SpermSegGS | Faculty of Medicine, University of Chile, Santiago, Chile. | Images | 19 | |||
[8] | VISEM | Simula Research Laboratory | Video | 85 | 640 × 480 | ||
[64] | VISEM | Simula Research Laboratory | Video | 85 | 640 × 480 | ||
[65] | MHSMA | Images | 1540 | Gray Scale | |||
[66] | Testicular biopsies | Images | 702 | ||||
[67] | Sperm image dataset | http://morfologia.cedai.cl/publi c/) | Images | 20 | Gray Scale | ||
[68] | SVIA dataset | https://github.com/Demozsj/Detection-Sperm. | Images and video | 101 and 130042 | 698528 | ||
[69] | MHSMA | Images | 1540 | Gray Scale | |||
[70] | MHSMA | Images | 1540 | Gray Scale | |||
[71] | VISEM | Simula Research Laboratory | Video | 85 | 640 × 480 |
In the first dataset, 100 slides were used, containing 1 to 5 sperms. Each slide contains 10 to 25 images. Image resolution was 576 × 764 pixels in RGB color space [36]. The article by Tseng et al. took datasets from the hospital and named them original images. The dataset is based upon 160 sperms, 80 normal and 80 abnormal sperms. The images are provided on https://code.google.com/p/support-vector-machine-for-sperm-morphology diagnosis/ as an Additional material [12]. Wang et al used a public dataset in their research work. This dataset contains 100 university students in good health, from 18 to 36 years old. Every case of the dataset consists of 9 different attributes like health, life habit, semen quality and concentration etc. [37]. Another famous dataset is the human sperm morphology analysis dataset (HSMA-DS). This dataset contains 1457 sperms cells with normal and abnormal sperms with different deformities in the head, midpiece, and tail. Images were captured at ×400 and ×600 magnification using IX70 microscope and DP71 CCD camera with chromatic infinity objective lenses and a resolution of 576 × 764 pixels in RGB color space [35]. Some researchers also consider video sample for sperm fertility prediction, in the same year Tan et al recorded video samples for semen analysis in Hospital University Sains Malaysia (HUSM). 80 samples were recorded using x40 magnification [38]. In the same year, another researcher selected 100 images with 7 attributes including age, alcohol consumption, smoking habits, accident/trauma, surgical intervention, number of hours spent sitting per day, childish disease and high fever with two target classes [39]. Another research took a video dataset for sperm analysis. The Videos were recorded in the andrology lab of the Academic Unit of Reproductive and Developmental Medicine (University of Sheffield). A healthy volunteer was ready to give his sperm sample for research purposes. Image resolution was set to 2040 × 1086 pixels. University Research Ethics Committee approved all procedures for experiment [40]. In 2016 tan et al performed another experiment on a video dataset recorded in the same university i.e (Hospital University Sains Malaysia (HUSM)). This time age of donors was at least 15 years and 50 microliters of fresh semen samples were collected but the equipment and parameters for recording the video were the same as used by the author in 2015. The dataset consists of 20 original images; each frame shows 60 sperm cells. So total 1200 (60 × 20) sperm cells were used for segmentation [41]. In same year Shaker et al use the Gold-standard dataset for semen analysis. This dataset consists of 19 stained sperm samples, total of 210 consider valid out of 264 sperm cells. Images in this dataset are in RGB format with 780 × 580 pixels [42]. In 2017 Mirsky et al took semen sample from 8 healthy volunteers, after one week of the sexual absence period. Total 886 images were selected for this experiment [43]. Garcia et al used video dataset for experiment. Images were divided into different classes based on orientation and morphology of sperm cell [44]. Similarly, Chang et al introduced human sperm head classification gold-standard SCIAN-MorphoSpermGS, introduced in [45]. Sample were collected in the Laboratory of Spermiogram, Program of Anatomy and Developmental Biology (ICBM) from young volunteers ages from 28 to 35-year-old [45]. The Human Sperm Head Morphology dataset (HuSHeM) is very famous, freely available online dataset, mostly researchers used this dataset for sperm analysis. It is consisting of 216 sperm heads (57 pyriform, 53 tapered, and 52 amorphous and 54 normal). Images are in RGD format with 131 × 131 pixels size [46]. Shaker et al also work on another dataset in the same year 2017. Healthy donors collected samples in Isfahan Fertility and Infertility center (IFIC). Slides were dried then fixed and stained using Diff-Quik method as described in WHO 2010 manual [3]. Image resolution was 720×576 pixels and stored in bitmap format. Then sperm head was classified by expert staff available at IFIC. Final dataset consists of 170 sperm heads (39 pyriform, 23 tapered, and 42 amorphous and 66 normal) [47]. Nissen et al constructed a dataset of 35 samples and 765 grayscale images. These 35 samples were diluted using Bicarbonate-Formalin solution as recommended by WHO [3], to get specific number of cells in each image i.e. 2 to 290 cells per image. The image resolution was 1920 × 1440 pixels [48]. Engy et al perform Experiments on the fertility-dataset, which is available at UCI repository. This dataset is based on 100 instances with 9 attributes like age, the rate of alcohol, diseases in childhood and surgeries etc. [49]. Author use Ocular images of the microscope through a smartphone-based data acquisition technique. In this dataset total 13 stained images of 13 persons were collected and analyse in this study(Ilhan et al., 2018). In next paper author captured images using inverted microscope (Olympus IX71) with camera (Optronis 5000) with speed of 3000 images per second. Image resolution was 512 × 512 pixels [51]. Movahed et al introduce Gold-standard Dataset. This dataset consists of 20 stained normal and abnormal sperm images with resolution of 780 × 580 pixels. The author divides this dataset into 14 training and 6 testing sets for the sake of the experiment [52].
In last paper of 2018 author use video dataset for semen analysis. In this digital experiment microscope was used at 400× magnification with 3200 × 2400 digital resolution. The slides of semen sample consist of 10µL volume and videos captured by automated microscope at the rate or 90 frames with 30 frames per second. These images were categorized by expert pathologist into sperm and non-sperm [17].
Hicks et al used online available video dataset (VISEM) from 85 persons for experiment. These videos were recorded at 400× magnification and saved as AVI file format. The videos were between 2 to 7 minutes with 50 frames per second. Expert manually assessed this sample for sperm motility and concentration. Author also stores donor's data like BMI, age, sexual absence period etc. (Hicks et al., 2019). In the next paper, the author employed an in-house dataset of fluorescence images and bright-field with the confocal microscope at ×100 objective magnification, 488 nm wavelength and 598–660 nm emission filter for red and 500–550 nm emission filter for green [53]. Riordon et al used 2 datasets to evaluate VGG16-based approach; first one is (HuSHeM) and second (SCIAN) dataset. These two datasets are freely available online for research purposes. HuSHeM dataset contains 216 RGB images with 131 pixels × 131 pixels at ×100 magnification. All images were labelled and classified by three experts as 57 Pyriform, 52 Amorphous, 53 Tapered and 54 Normal. SCIAN dataset contains 1132 greyscale images with 35 pixels × 35 pixels at × 63 magnification. Similarly, all images were labelled and classified by expert as 7 Pyriform, 262 Amorphous, 69 Tapered and 35 Normal [32]. In 2018 Movahed et al again work on public Gold-standard dataset in 2019. This dataset consists of 20 images with a resolution of 780×580 pixels and is divided into normal and abnormal sperm cells. However, handmade ground truths were publicly available, but some errors should be corrected [54].
In next study, the dataset contains a semen sample of 18 persons from Shenzhen Sixth People's Hospital. The videos were recorded by BA210 contrast microscope handed with 20× phase contrast objective and a Monochrome CCD Camera. The videos were captured at 25 frames per seconds and duration was 3 seconds with resolution of 720 × 540 pixels. Author recorded 3 videos per sample, total dataset contains 54 videos [55]. Javedi et al use new dataset named as Modified Human Sperm Morphology Analysis dataset (MHSMA); this dataset is an extended form of the Human Sperm Morphology Analysis dataset (HSMA-DS) introduced in [35]. This dataset consists of 1,540 RGB images with a pixel size of 1280 × 1024 [31]. In 2020 Mohammadi et al introduce dataset that contains 36 different videos that were recorded in the Royan institute Research Lab. at Tehran. The recorded videos are 8bit grayscale with 50 frames per second frame rate and 768 × 576 pixels resolution. Each video consists of 25 frames. The number of sperms in the videos is in the range of 4 to 95 in each video, and in total, all the videos contain 1628 sperms [56].
In next study 119 non-obstructive azoospermia (NOA) patients were referred to the IVF unit at SUMC for conventional testicular sperm extraction (cTESE) between 1995 and 2017. Azoospermia means absence of sperm after ×400 magnification and 3000g centrifugation. According to WHO criteria, all patients submit their semen sample on 4 different occasions [3]. None of these patients underwent TESE before [57]. Lesani et al collected semen samples from 41 patient's ages between 30 and 45 years old. These patients were referred to Avicenna Infertility Clinic for male infertility treatment [58].
SCIAN is a gold-standard dataset for the morphological classification of human sperm heads with five categories: Normal, Tapered, Pyriform, Amorphous, and Small. The images in this dataset are of greyscale with stained sperm heads, taken at 63 × magnification and their height and width are both 35 pixels and 7 µm. Domain experts have three separate agreement settings: no agreement, partial agreement, and total agreement. The first set consists of 1854 sperm head images (175 Normal, 420 Tapered, 188 Pyriform, 919 Amorphous, and 152 Small). Still, an image in this set can be labelled manually into three different classes by three domain experts. The second set comprises 1132 images (100 Normal, 228 Tapered, 76 Pyriform, 656 Amorphous, and 72 Small), but an image can be labelled into two different sperm head classes. The third set includes 384 images (35 Normal, 69 Tapered, 7 Pyriform, 262 Amorphous, and 11 Small). All three experts assigned the same class label to a sperm head image. From the number of images in these three sets, we can appreciate the difficulty of the morphological classification of human sperm heads even by human experts. For illustration (Figure 4), we show typical samples of human sperm heads of microscopic images of the five classes in the partial agreement setting of the SCIAN dataset and the four classes of the HuSHeM dataset [59].
Ilhan et al used Sperm Morphology Image Data Set (SMIDS) for sperm detection and classification. Sample were collected from 17 different persons from ages 19 to 39 years. They had no sexual activity (no ejaculation) for at least 2 days but not more than 7 days. Software-based image stabilizer was used for better image acquisition. Figure 5 shows sample capturing procedure [30]In next study author collect semen sample and make sure that human must not ejaculate from 4 to 7 days. For recording videos of semen. Videos were record at 30 frames per second with 288 × 352 resolution. Figure 6 shows procedure of microscopic semen analysis [60].
Marin et al used the human sperm segmentation gold-standard SCIAN-SpermSegGS dataset. Semen samples were collected at the Laboratory of Spermiogram, Program of Anatomy and Developmental Biology (ICBM), Faculty of Medicine, University of Chile, ages 28 to 35. This dataset contains total of 19 images with 264 sperm cells. The image resolution was 780 × 580 pixels. Field experts designed hand-made segmentation masks for every image in the dataset. Figure 7 shows a detailed image from SCIAN-SpermSegGS dataset. (a) Number of sperm cells in original RGB colour space (780 × 580 pixels) (b)–(d) Nucleus, acrosome and head of valid sperm in (a), Handmade segmentation mask respectively [61].
Ottl et al also used a visem dataset from the Simula Research Laboratory. This dataset consists of 85 videos of live spermatozoa from men aged 18. Figure 8 shows still image from the sperm sample videos [62]. In next paper, according to the best of the author's knowledge, there are no existing large-scale image datasets of testicular biopsies. After institutional review board (IRB) approval at Stanford University, Stanford, CA, USA (Approval No. 41652), they collected a novel dataset of 702 de-identified images from testicular biopsy samples of 30 patients [63].
Next dataset is based on 20 images with 15 to 20 sperms cells in each image. The author uses morphological analysis for edge detection and filtering. Figure 9 shows sperm input images, after applying the morphological process, image representation and segmentation will be performed [64].
In the year 2022 SVIA dataset was introduced by [65]. This dataset was obtained from JingHua Hospital of Shenyang, and the preparation of the SVIA dataset production began in 2017. After about four years of work, more than 278000 objects were annotated, as shown in Figure 10. Table 2 shows three subsets of given dataset.
Subset-A | Video | Number 101 |
No. of annotated object 125,000 | Format .mp4 |
Image | Number 3590 |
Size 698 × 528 × 3 |
Format .png |
|
Subset-B | Video | Number 10 |
No. of annotated object 26,000 | Format .mp4 |
Image | Number 451 |
Size 698 × 528 × 3 |
Format .png |
|
Subset-C | Image | Class Sperm impurity |
Number 121,401 4,479 |
Format .png |
Subset-A is mainly used for object detection tasks, providing more than 125000 objects' location and category information in 101 videos [66], their formats were.mp4, and the frame rates were 30 frames per second. Moreover, as object detection datasets, 3590 images and annotation files are obtained from 19 microscopic videos. The formats of the images are.png, and the sizes were 698 × 528 × 3. The annotation files contain the object categories and locations.
Subset-B was prepared for image segmentation and object tracking tasks, providing more than 26,000 sperms in 10 videos as ground truth (GT) for tiny object tracking and 451 images as GT for segmentation. Each GT contains the location of each sperm in different videos and frames, their formats are.png, and the sizes are 698 × 528 × 3.
Subset-C was developed for image denoising and classification tasks, which provides more than 125; 000 independent images of sperms and impurities, including 121; 401 sperm images and 4; 479 impurity images. Meanwhile, this large-scale dataset can also test the robustness of various deep learning models to noises. To highlight the advantages of this dataset, author compare the SVIA dataset with three publicly available datasets. HuSHeM [46], Visem [8] and MHSMA [31] (as shown in Table 3). However, for all the above datasets, the dataset size is relatively small and quickly bottlenecked for cases where large datasets need to be used. This dataset contains 101 videos, 130042 images, and 127600 object information, which perfectly solves the problem of too little information and quantity in the above three datasets.
Dataset | Video | Image | Size | Number of annotated objects |
HuSHeM | 0 | 216 | 131 × 131 | 0 |
Visem | 85 | 0 | 640 × 480 | 0 |
MHSMA | 0 | 1540 | 128 × 128 or 64 × 64 | 0 |
SIVA | 101 | 130042 | 698 × 528 | 127600 |
In this section we discuss pre-processing techniques that were applied on each dataset one by one. Table 4 demonstrate pre-processing techniques with their respective outcomes. In first artical Bijar et al scaled the image as a pre-processing technique using Red component of RGB. M was the range between zero to maximum intensity, and it was 12-byte data I initially, then scaled to a new intensity between 0 to 255 (8-bit). It was formulated as I s = I/M × 255 [36]. Wang el al. Introduced Joint Rank Difference and Gray Level Method as a pre-processing step to identify between normal and abnormal sperms. They also pre-process the dataset by only removing the majority Tomek Links and balance data with the help of SMOTE technique [37]. Ghasemian et al apply Gaussian filter for noise reduction on very famous dataset (HSMA-DS); this algorithm converts RGB color values to YcbCr Y-channel sharpening. By applying Gaussian Filter on Y channel Noise Reduction by wavelet transform, the noise percentage decreased. However, this percentage was not acceptable for further processing [35]. Duggal et al has proposed an optional attribute selection process technique that helps to filter attributes in dataset. The cumulative data was pre-processed using an optional attribute selection process. This intermediate step was used to filter only the relevant and important attributes from the bulk data. Attribute selection can affect the overall results derived from the data [39].
Ref | Dataset name | Pre-processing techniques | Outcomes |
[36] | - | Red component of RGB | Author first scaled image as a pre-processing technique using Red component of RGB. M is the range between zero to maximum intensity, and it was 12-byte data I initially, then scaled to a new intensity Is between 0 to 255 (8-bit). It was formulated as I s = I/M × 255. |
[37] | Public dataset | SMOTE | Removing majority Tomek Links and balance data with the help of SMOTE technique. |
[35] | HSMA-DS | Gaussian filter | By applying Gaussian Filter on Y channel Noise Reduction by wavelet transform, the percentage of noises was decreases. However, this percentage was not acceptable for further processing |
[39] | - | optional attribute selection process | The cumulative data was pre-processed using an optional attribute selection process. This intermediate step was used to filter only the relevant and important attributes from the bulk data. Attribute selection can affect the overall results derived from the data. |
[41] | Video dataset | Laplacian of Gaussian (LoG) filter |
First, they convert images into gray scale from RGB. Because colour image required more processing time as compare to gray scale images. In next step Laplacian of Gaussian (LoG) filter was deployed in kernel as a pre-processing step so that it automatically detect and segment human spermatozoa. |
[67] | SCIAN-MorphoSpermGS | Anisotropic diffusion | Anisotropic diffusion method was used for noise reduction. Moreover, this method also preserves the border of images. |
[50] | Ocular images | Modified Overlapping Groups Shrinkage (MOGS) | In MOGS, convex function is used to detect the correct region of sperm, therefore denoising problem was accepted. In this regard non-convex regularization was used and desired group sparsity property were verified |
[51] | 3D+t | logarithm transform | To shrink the range of intensity values, log transformation (V log (~ x) = log (V (~ x) + 1)) was applied on image stack V. so it increases the contrast of low intensity values. |
[52] | Gold-standard dataset | Homomorphic Filtering Filling holes |
This method helps to highlight each sperm in the background by correct uneven illumination. Moreover, some post processing steps were also involved to improve segmentation procedure. |
[54] | Gold-standard dataset | Serialized | This method helps to highlight sperm cells in the image rather than other particles. It also restrains unnecessary characteristics of the image like low contrast between the sperms tails and its neighbouring region and non-uniform distribution of light |
[59] | HuSHeM and SCIAN. | Rotation Translation flipping |
Author used three common techniques as previously used in the training set of the SCIAN dataset. For rotation, they rotate the training image by 25 to 25 degrees. For translation, they shift ~6% of the original image to the left, the right, up, and down. For flipping, they vertically flip the image. Due to the same distribution of classes within this dataset, they apply equal augmentation options to each class |
[68] | Sperm Morphology Image Data Set (SMIDS) | Modified Overlapping Groups Shrinkage (MOGS) |
Ilhan et al again used same pre-processing technique as he had used in 2018 called MOGS. This technique helps to remove random noise without changing sperm shapes. |
[60] | Video dataset | median filter, elliptic curve | Median filter was also used for pre-processing, this technique was applied to each image. The edges of images were preserved when they pass through median filter. |
[8] | VISEM | Convolutional neural network (CNN) | CNN was a specialized type of network used that has a grid-like topology for processing data. They are most commonly used on image and video data |
[62] | VISEM | Spatial warping, Background removal, and Filtering |
Geometric distortion, non-uniform contrast, and noise are alleviated by spatial warping, background removal and filtering, respectively |
[63] | Testicular biopsies | Glare filters and diffraction correction, MobileNetV2 |
A single embryologist annotated each image with bounding boxes around each identified spermatozoon. At the time of collection, each image was normalized, passed through glare filters and diffraction correction (Hamilton Thorne, HT video and image capture software version 3, Hamilton Thorne, Inc., Beverly, MA, USA), and had microscopy artifacts removed. |
[64] | Sperm image dataset | Median filtering | It helps to reduce noise in the image and perform edge detection and improve image resolution. It also sorts pixel value by comparing mean filter. It does not create any unreal surrounding pixels, so it gave efficient result after pre-processing. |
In 2016 researcher used video dataset for sperm analysis. First, they convert images into gray scale from RGB. Because colour image required more processing time as compare to gray scale images. In next step Laplacian of Gaussian (LoG) filter was deployed in kernel as a pre-processing step so that it automatically detects and segment human spermatozoa [41].
In same year Shaker et al used Ostu method for sperm head detection. Moreover, they apply a histogram of the Hue channel of HSV color space along with size criterion for successful sperm head detection in the microscopic image [42]. Chang et al introduced Anisotropic diffusion method for noise reduction. Moreover, this method also preserves the border of images [45].
Ilhan et al use Modified Overlapping Groups Shrinkage (MOGS) as a pre-processing step to remove the noising data from the sperm images that is not required for image analysis. Generally, these signal (y) of length N is modelled as
(5.1) |
Where x was the noise free regions and w is the additive white Gaussian noise. In this technique, large magnitude pixels for an image tend not to be isolated. If noise found by using prior knowledge, then the standard deviation of the noise is applied and the noise free signal (the area of sperm and non-sperm particles) was known, the noise free groups can be separated from the noisy signal without knowing the group.
(5.2) |
Where R(x) was the penalty function, λ was the regularization parameter and satisfies λ > 0. In MOGS, convex function was used to detect the correct region of sperm, therefore denoising problem was accepted in which a non-convex regularization term was employed and this verify the desired group sparsity property. Figure 11 shows effect of MOGS on given image as a pre-processing technique [50].
Movahed et al has presented logarithm transform for pre-processing. To shrink the range of intensity values, log transformation (V log (~ x) = log (V (~ x) + 1)) was applied on image stack V. So, it increases the contrast of low intensity values [51]. Homomorphic filtering was another pre-processing method in the frequency domain. This method helps to highlight each sperm in the background by correcting uneven illumination. Moreover, some post-processing steps were also involved in improving segmentation procedures like removing unwanted objects with respect to size, filling holes, closing/opening morphology operations etc. [52]. Similarly, in 2019 author used serialized method of pre-processing on Gold-standard dataset. This method helps to highlight sperm cells in the image rather than other particles. It also restrains unnecessary image characteristics like low contrast between the sperm's tails and its neighbouring region and non-uniform distribution of light [54]. Iqbal et al adopt three common techniques as previously used in the training set of the SCIAN dataset. For rotation, they rotate the training image by 25 to 25 degrees. For translation, they shift ~6% of the original image to the left, the right, up, and down. For flipping, they vertically flip the image. Due to the same distribution of classes within this dataset, they apply equal augmentation options to each class [59]. Ilhan et al. again used the same pre-processing technique he used in 2018 called MOGS. This technique helps to remove random noise without changing sperm shapes [77].
Median filter was also used for pre-processing; this technique was applied to each image. The edges of images were preserved when they pass through the median filter [60]. Some researcher also employs CNN for pre-processing. CNN is a specialized type of network used that has a grid-like topology for processing data. They are most commonly used on image and video datasets [8]Ottl el al; remove Geometric distortion, non-uniform contrast, and noise by spatial warping, background removal and filtering, respectively [62]. Wu et al use glare filters and diffraction correction for the pre-processing dataset. A single embryologist annotated each image with bounding boxes around each identified spermatozoon. At the time of collection, each image was normalized, passed through glare filters and diffraction correction (Hamilton Thorne, HT video and image capture software version 3, Hamilton Thorne, Inc., Beverly, MA, USA), and had microscopy artefacts removed [63]. In last article, Prabaharan et al used Median filtering as a pre-processing step. It helps to reduce noise in the image, perform edge detection, and improve image resolution. It also sorts pixel value by comparing the mean filter. It does not create any unreal surrounding pixels, so it gives efficient result after pre-processing [64]
In this section, we discuss methods used by different researchers for feature extraction for their respective datasets. Table 5 represent state-of-the-art Systematic comparison of Feature Extraction approaches through Machine Learning. [12] emian et al apply Sobel algorithm as a feature extraction technique for edge detection [35]. (Van Raemdonck et al presented Otsu's threshold as a feature extraction technique. It converts the image to grayscale and then eliminates the background from the foreground [40]. Shaker et al use HSV color space for sperm head detection in a pre-processing step. But HSV was a typical perceptual color space, instead, they use GVF model and achieve 92% success rate which outperformed state-of-the-art techniques [42]. In 2017 Mirsky et al used Phase Map Acquisition (PMA) technique to extract 89 custom-design features from the dataset. These features included the acrosome area, the ratio of head length to width, mean OPD and the correlation of the OPD map of the head to a model of the ideal head etc [43]. In the same year Garcia et al used SURF (Speeded-Up Robust Features) method for feature extraction, it also detects key point from a given dataset. Hessian matrix was used to select the most demonstrative pixels found in low-level features [44]. Chang et al introduce a new method for feature extraction called, five shape-based descriptors. It easily recognizes human sperm head and also helps in sperm morphology analysis [67].
Ref | Dataset name | Method of Feature Extraction | Outcomes |
[12] | Original Images | Fourier Transform Infrared Spectroscopy (FTIRS), grey level feature | FTIRS and grey level feature was used to differentiate multiple sperms according to their characteristics. |
[35] | HSMA-DS | Sobel algorithm | Sobel algorithm was used for edge detection |
[40] | video frames | Otsu's threshold | Author use Otsu's threshold as a feature extraction technique. It converts image to gray scale then eliminate background from the foreground. |
[42] | Gold Standard | Gaussian filter, Ostu method, Gradient vector flow, curvature and edge energy | Author use ostu method for sperm head detection. Moreover, they use histogram of the Hue channel of HSV color space along with size criterion for successful sperm head detection in microscopic image |
[43] | - | Phase Map Acquisition | Phase Map Acquisition (PMA) technique was used to extract 89 custom-design features from dataset. These features included the acrosome area, mean OPD and the correlation of the OPD map of the head etc |
[44] | - | (Speeded-Up Robust Features) SURF | It detects key point from given dataset. Hessian matrix was used to select most demonstrative pixels founded in low-level features. |
[67] | SCIAN-MorphoSpermGS | five shape-based descriptors | Author proposes combine five shape-based descriptors It easily recognize human sperm head and also help in sperm morphology analysis. |
[50] | Ocular images | Pixel-Based Spatial analyses, Blob analyses | PBS analyses, Blob analyses was used for feature extraction (spatial-based features), over segmented parts. |
[33] | VISEM | Lucene Image Retrieval (LIRE) Tamura image features |
LIRE is a java library that use to extract features from images and photos with the help of texture and color characteristics. Author test more than 30 features with machine learning algorithms but Tamura image features work best with proposed algorithm in this study. |
[55] | Gaussian mixture model | This technique used statistical information of pixel for background representation, such that target pixel was figure out using statistical difference and judged probability density in long time period with large sample value. As result a complex dynamic background could be modelled. |
Ilhan et al apply Pixel-Based Spatial analyses, Blob analyses for feature extraction (spatial-based features), over segmented parts. Normally a sperm is 2.5–3.5 micrometers wide and 5–6 micrometers long and contain an oval head with a single long tail. But abnormal have different shapes and sizes depending on their abnormality type. Moreover, images also contain different clusters than normal/abnormal sperm due to the staining process, which is indicated as non-perm [50]. Hicks et al used Lucene Image Retrieval (LIRE) for feature extraction. LIRE is a java library used to extract features from images and photos with the help of texture and color characteristics. The author test more than 30 features with machine learning algorithms, but Tamura image features work best with the proposed algorithm in this study [33]. The gaussian mixture model is another feature extraction technique. This technique used statistical information of pixels for background representation, such that the target pixel was figured out using statistical difference and judged probability density in a long period with a large sample value. As a result, a complex dynamic background could be modelled [55].
In this section we discuss different techniques for segmentation using Machine Learning. These Traditional Segmentation approaches with Machine learning can be seen in Table 6. In 2012 Bijar et al used Markov random field (MRF) Model and Entropy based (EM) algorithm for segmentation. This segmentation includes sperms acrosome, mid piece, nucleus and sperms tail. They also place some estimated points on sperms tail for identification of morphological characteristics like length and shape. In first section Bayesian classifier was used to segment sperm's Nucleus, Mid-piece and Acrosome. In second section EM and MRF model was used to upgrade and obtain class conditional probability density function (CCPDF) and apriori probability for each class [36].
Ref | Dataset name | Method of Segmentation | Outcomes |
[36] | - | Markov random field (MRF) Model and Entropy based (EM) algorithm |
In first section Bayesian classifier was used to segment sperm's Nucleus, Mid-piece and Acrosome. In second section EM and MRF model was used to upgrade and obtain class conditional probability density function (CCPDF) and apriori probability for each class. |
[12] | Original images | Average Rank Difference | By using Average Rank Difference between abnormal and normal sperms the segmentation of accuracy 55% achieved. |
[37] | Public dataset | Clustering-Based Decision Forests (CBDF Algorithm) | Author used CBDF Algorithm to deal with unbalanced class learning problem for sperm analysis. Result shows that CBDF Algorithm outclass state of the art techniques like logistic regression, multilayer perceptron neural networks, random forests, Support Vector Machines and decision tree etc with great difference. |
[40] | Video dataset | The structural similarity measure (SSIM) The correlation (CORR) coefficient, and The Bhattacharyya distance (BHAT) |
Author used 3 different techniques for segmentation. The original image was labelled with 1 or 0 and count of sperm, non-sperm, immotile and motile cells. Only motile cells were kept for experiment and all other non-sperm and immotile cells were removed. |
[42] | Gold standard | GVF, curvature and edge energy (TDCEE), Dice Coefficient | For more refine segmentation, researcher used edge-based active contour method. Moreover, they also used a novel tail point detection technique that trace and eliminate midpiece from segmented head, for more efficient segmentation. Results shows 92% achievement against hand segmented ground truth that outperform famous techniques. |
[43] | - | Support Vector Machines (SVMs) |
The SVM classifier achieves receiver operating characteristic curve of 88.59%, precisions of 90% and precision-recall curve of 88.67%. Researcher believed that this automatic process of sperm analysis will become base of automatic and objective sperm cell collection in IVF |
[44] | Support Vector Machines (SVMs) |
Support Vector Machines (SVMs) was used for segmentation. Simplified feature vectors that extracted from dataset were used to classify sperm cells into normal, abnormal and non-cell categories. | |
[67] | SCIAN-MorphoSpermGS | SVM-based cascade classification | This two-stage classification scheme shows better results than monolithic classifiers and it also work like a human expert. |
[50] | Ocular images | Fuzzy C- Means (FCM) and K-Mean |
FCM segmented the gray scaled imaged more successfully than K-Means because of fuzzy technique. But there is room of improvement because only 50% sperms were correctly classified. |
[55] | Threshold segmentation | This technique was applied on background image for the sake of counting immotile sperm. |
In 2013 Tseng et al used Average Rank Difference method for segmentation between normal and abnormal sperms. By using Average Rank Difference between abnormal and normal sperms the segmentation accuracy of 55% was achieve [12]. Wang et al propose Clustering-Based Decision Forests (CBDF) Algorithm to deal with unbalanced class learning problem for sperm analysis. Result shows that CBDF Algorithm outclass state of the art techniques like logistic regression, multilayer perceptron neural networks, random forests, Support Vector Machines and decision tree etc. with great difference [37]. Van Raemdonck et al proposed 3 different techniques for segmentation i.e. the structural similarity measure (SSIM), correlation (CORR) coefficient and the Bhattacharyya distance (BHAT). The original image was labelled with 1 or 0 and count of sperm, non-sperm, immotile and motile cells. Only motile cells were kept for experiment and all other non-sperm and immotile cells were removed [40].
For more refine segmentation, Shaker et al used edge-based active contour technique. Moreover, they also used a novel tail point detection technique that trace and eliminate midpiece from segmented head, for more efficient segmentation. Results shows 92% achievement against hand segmented ground truth, that outperform famous techniques [42].
In previous section shaker et al used different pre-processing and feature extraction techniques for efficient segmentation process. In 2017 Mirsky et al use Support Vector Machines (SVM) classifier for segmentation. The SVM classifier achieves receiver operating characteristic curve of 88.59%, precisions of 90% and precision-recall curve of 88.67%. Researcher believed that this automatic process of sperm analysis will become base of automatic and objective sperm cell collection in IVF [43]. Similarly, Garcia et al also used Support Vector Machines (SVMs) for segmentation. Simplified feature vectors that extracted from dataset were used to classify sperm cells into normal, abnormal and non-cell categories [44]. Again, Chang et al also used SVM base classifier called SVM-based cascade classification. This two-stage classification scheme shows better results than monolithic classifiers and it also work like a human expert [67] Ilhan et al apply Fuzzy C- Means (FCM) and K-Mean for segmentation. FCM segmented the gray scaled imaged more successfully than K-Means because of fuzzy technique. But there is room of improvement because only 50% sperms were correctly classified [50]. In last paper of Machine Learning techniques, Qi et al used the commonly used threshold segmentation. This technique was applied on background image for the sake of counting immotile sperm [55].
In this section we discuss different techniques for segmentation using deep learning. Table 7 elaborate State of the art Segmentation approaches through deep learning with their respective results. Tan et al used Pulse Coupled Neural Network (PCNN) technique for segmentation. PCNN is very famous method for sperm image segmentation. It helps to solve inaccurate sperm head shape problems as well. It also uses to differentiate agglutinated sperms with high accuracy and precision. Moreover, PCNN model include eight parameters so, optimized PSO algorithm was used improve PCNN parameters [41]. In 2016 they also proposed ICM mode for segmentation of same video type dataset. For efficient sperm head segmentation ICM is derived from several visual cortex models. There is some parameter selection problem, so researcher optimize ICM network with PSO. New fitness function know as feature mutual information was introduced here. This model was more efficient and precise than other famous segmentation techniques [41]. In 2017 Shaker et al introduced Active contours method for segmentation. This method automatically segmented cropped sperm heads. Colour images were transformed to gray scale images and apply threshold using Otsu method for fast locating of sperm heads. Moreover, edges of the images were extracted by Canny edge detector method and Gradient Vector Flow (GVF) active contour method was employed to extract outer contour of sperm heads [47]. Nissen et al apply CNN for segmentation. CNN outperform classical image analysis approach by achieving 91.89 % recall and 93.87 % precision on a given dataset [48]. In 2018 Movahed et al also apply CNN for segmentation on Gold standard dataset. For segmentation of sperm head a deep convolutional neural network was used. For improve head segmentation, geometric constraints and filling holes operation were also employed. Then SVM helps to classify all pixel of segmented heads to acrosome and nucleus regions [52]. Similarly, in 2019 Movahed et al introduced two CNN networks with different technique for segmentation of head and axial filament of the sperms. Overlapping patches were extracted from RGB images at the end of background using CNN model 1. Moreover, at Histogram Stretching stage, patches were extracted from RGD images using CNN model 2 [54]. Javedi et al used CNN network to deal with morphological deformities present in human sperm like head, vacuole, and acrosome. It was first technique that focus on acrosome. It also works very efficient in low resolution and non-stained images [31].
Ref | Dataset name | Method of Segmentation | Outcomes |
[38] | Video dataset | Pulse Coupled Neural Network (PCNN) Particle Swarm Optimization (PSO) |
PCNN is very famous method for sperm image segmentation. It helps to solve inaccurate sperm head shape problems as well. It also uses to differentiate agglutinated sperms with high accuracy and precision. Moreover, PCNN model include eight parameters so, optimized PSO algorithm was used improve PCNN parameters. |
[41] | Video dataset | Intersecting cortical model (ICM) | For efficient sperm head segmentation ICM was derived from several visual cortex models. There was some parameter selection problem so researcher optimize ICM network with PSO. New fitness function know as feature mutual information was introduced here. This model was more efficient and precise than other famous segmentation techniques. |
[47] | - | Active contours. | Active contours method was used for segmentation. This method automatically segmented cropped sperm heads. Color images were transformed to gray scale images and apply threshold using Otsu method for fast locating of sperm heads. Edges of the images were extracted by Canny edge detector method and Gradient Vector Flow (GVF) active contour method was employed to extract outer contour of sperm heads. |
[48] | Convolutional Neural Network (CNN) | CNN outperform classical image analysis approach by achieving 91.89% recall and 93.87% precision on a given dataset. | |
[52] | Gold-standard dataset | Convolutional Neural Networks (CNN) | For segmentation of sperm head a deep convolutional neural network was used. For improve head segmentation, geometric constraints and filling holes operation were also employed. Then SVM helps to classify all pixel of segmented heads to acrosome and nucleus regions. |
[54] | Gold-standard dataset | CNN model 1 CNN model 2 |
Two CNN networks were used with different technique for segmentation of head and axial filament of the sperms. Overlapping patches were extracted from RGB images at the end of background using CNN model 1. Moreover, at Histogram Stretching stage, patches was extracted from RGD images using CNN model 2 |
[31] | MHSMA | Convolutional Neural Networks (CNN) |
CNN network was used to deal with morphological deformities present in human sperm like head, vacuole, and acrosome. It was first technique that focus on acrosome. It also works very efficient in low resolution and non-stained images |
[68] | Sperm Morphology Image Data Set (SMIDS) | K-Means clustering Fuzzy C-Means clustering |
MOGS technique was used as a pre-processing step and it helps to reduce noise present in sample images and improve the overall segmentation process. Similarly, Fuzzy C-Means clustering shows impressive results when MOGS was applied on images as a pre-processing method. Moreover, Fuzzy C-Means clustering apply fuzziness for the belongingness of each image pixels, which increase efficiency of segmentation process. |
[60] | Video dataset | Local Region Contour segmentation | Author separates tail region with binary images with the help of local region contour segmentation. In past may algorithm were unsuccessful to detect tail region from video dataset because of minor gradient in the tail region. Some researcher able to do so while denoising images. |
[61] | SCIAN-SpermSegGS | U-Net and Mask- RCNN |
Results show that U-net with transfer learning, outclass state of the art sperm segmentation method with 95% overlapping efficiency against hand-segmented masks for sperm head 0.96, acrosome 0.94 and nucleus 0.95. |
[69] | VISEM | Region Based Convolutional Neural Networks (R-CNN) architecture |
The Faster R-CNN-Inception_V2-COCO model was used to develop the sperm counting algorithm. Its advantage is that it is quite accurate to learn to distinguish objects even from a small database. The model takes about 50 ms to analyze the image. Because the model uses regions, it is easier to detect a larger number of objects in a single frame. Additionally, the R-CNN model can read information from frames of any size, so there is no need to crop frames before submitting them to the neural network. Achieved 91.77% (95% CI, 91.11–92.43%) accuracy of sperm head detection on the VISEM sperm sample video dataset. |
[62] | VISEM | Mean squared displacement of each particle (imsd) |
Segmentation can be done for each individual particle (mean squared displacement of each particle (imsd)) or again as an average for all of the particles (ensemble mean squared displacement of all particles (emsd)). These results show a relative improvement of 4.4% against state-of-the-art results. |
[70] | MHSMA | Deep Transfer Learning (DTL) technique and Deep Multi-task Transfer Learning (DMTL) |
DTL technique accurately label head, vacuole, and acrosome with accuracy of 84%, 94%, and 79% respectively. Similarly, DMTL technique accurately label head, vacuole, and acrosome with 82%, 92.33%, and 80.66% respectively. |
[63] | Testicular biopsies | VGG16 | Classifies each proposal as either a spermatozoon or background and regresses the bounding box shape to match identified component objects. |
[64] | Sperm image dataset | Enhanced Otsu's threshold method |
It reduces the computation complexity and sobel operator was used for edge reduction, which improve overall performance of segmentation. The abnormal regions were detected by convolution layer. |
[65] | SVIA dataset |
k-means, U-net, SegNet and DeepLabv3 | The effects of U-net and Deeplab_v3 are the best, and other segmentation methods have a certain gap compared with these two segmentation methods |
[71] | VISEM | Crocker-Grier Algorithm With CNN |
The mean absolute error (MAE) could be reduced from 8.83 to 7.31. |
[72] | VISEM | YOLOv4 algorithm | The results obtained were 90.31% AP (Average Precision) for sperm objects and 68.19% AP (Average Precision) for non-sperm objects, then for the results of the training obtained by the model 79.58% mAP (Mean Average Precision). |
[73] | VISEM | YOLOv5 algorithm | YOLOv5 deep learning model trained on the VISEM-Tracking dataset present baseline sperm detection performances. This dataset can be used to train complex deep learning models to analyze spermatozoa. |
[74] | VISEM | YOLOv5 algorithm | The best-performing model is yolov5l. This network achieves a precision of 88.6 per cent, recall of 52.6, and mAP is 72.1. Other networks are achieving lower results. The second-best network is nano, with a mAP of 69.6 and precision of 64.7 per cent |
As previously discussed, that MOGS technique was used as a pre-processing step, and it helps to reduce noise present in sample images and improve the overall segmentation process. Similarly, Fuzzy C-Means clustering shows impressive results when MOGS was applied on images as a pre-processing method. Moreover, Fuzzy C-Means clustering apply fuzziness for the belongingness of each image pixels, which increase efficiency of segmentation process [68].
In past many algorithms were unsuccessful to detect tail region from video dataset because of minor gradient in the tail region. Some researchers able to do so while denoising images. Somasundaram et al successfully separate tail region with binary images with the help of local region contour segmentation [60]. Two well-known deep learning techniques U-Net and Mask-RCNN were proposed for sperm cell segmentation, using cross validation, hyper parameter tuning, transfer learning and data augmentation technique. Figure 12 shows that U-net with transfer learning, outclass state of the art sperm segmentation method with 95% overlapping efficiency against hand-segmented masks for sperm head 0.96, acrosome 0.94 and nucleus 0.95 [61].
In 2021 Valiuškaitė et al develop the sperm counting algorithm using the Faster R-CNN-Inception_V2-COCO model. Its advantage is that it is quite accurate to learn to distinguish objects even from a small database. The model takes about 50 ms to analyze the image. Because the model uses regions, it is easier to detect a larger number of objects in a single frame. Additionally, the R-CNN model can read information from frames of any size, so there is no need to crop frames before submitting them to the neural network. This approach achieved 91.77% accuracy of sperm head detection on the VISEM sperm sample video dataset, which is largest available video dataset (32 GB) that is available online [69]. Ottl et al introduced new segmentation process for each individual particle (mean squared displacement of each particle (imsd)) or again as an average for all of the particles (ensemble mean squared displacement of all particles (emsd)). These results show a relative improvement of 4.4% against state-of-the-art results [62]. Abbasi et al used Deep Transfer Learning (DTL) technique and Deep Multi-task Transfer Learning (DMTL) for segmentation. DTL technique accurately label head, vacuole, and acrosome with accuracy of 84%, 94%, and 79% respectively. Similarly, DMTL technique accurately label head, vacuole, and acrosome with 82%, 92.33%, and 80.66% respectively. Figure 13 shows visual representation of both models [70].
A new CASA system was developed with the help of deep learning that gave proximate human level performance on testicular sperm extraction (TESE). VGG16 convolution network employed or segmentation and trained on custom dataset. It classifies each proposal as either a spermatozoon or background and regresses the bounding box shape to match identified component objects [63]. Enhanced Otsu's threshold method reduces the computation complexity for segmentation. Sobel operator was introduced by Prabaharan et al for-edge reduction, which improve overall performance of segmentation. The abnormal regions were detected by convolution layer [64]. Chen at al used subset – B of SVIA dataset for segmentation. To evaluate the effectiveness of SVIA dataset author employ four traditional image segmentation techniques and four deep leering techniques, among the classical image segmentation methods, OT has the best effect. But it is lower than the four image segmentation methods based on deep learning. In general, the effects of U-net and Deeplab_v3 were the best, and other segmentation methods have a certain gap compared with these two segmentation methods [65]. In next study Ottl et al shows best results by unsupervised tracking of sperm cells with the Crocker-Grier algorithm, extracting imsd features for each detected track and aggregating those features into a histogram representation using BoW. With this feature representation, a linear SVR improved the mean (3-fold) MAE from 8.83 to 7.31, a decrease of over 17%. The results further show that the unsupervised feature quantization helps to achieve more consistent and robust results, regardless of which feature representation is chosen as input [71]. Aristoteles et al use YOLOv4 architecture for sperm detection from famous online available video dataset called VISEM. In this experiment dataset was available in the form of video and then converted into 1330 images. The training process was carried out with 3 different learning rate experiments, namely 0.002, 0.0002, 0.00002. In each of these experiments, 3 data divisions were made for each of the reading rates being tested. The best accuracy results are found in experiments with a learning rate of 0.0002 which has an accuracy value of 79.58% mAP on 70% train data distribution, 25% validation and 5% test. Each trial process for training uses 6000 iterations to create the training data. The test in this study uses video, the results of which are that all objects can be detected properly and have been labelled with a bounding box. In this study there were cases where the model was not able to detect optimally because the video data used contained blurred objects and sperm objects that were cut off by the frame [72] Thambawita et al present YOLOv5 deep learning model that is trained on the VISEM-Tracking dataset present baseline sperm detection performances. YOLOv5l performed best among all other versions of yolov5. This network achieves a precision of 43 per cent, recall of 25, and mAP is 22.3. Other networks are achieving lower results. The second-best network is medium, with a mAP of 22.1 and precision of 57.1 per cent. This dataset can be used to train complex deep learning models to analyze spermatozoa [75] Dobrovolny et al also use yolov5 for sperm cell detection and achieved very efficient results. The best-performing model is yolov5l. This network achieves a precision of 88.6 per cent, recall of 52.6, and mAP is 72.1. Other networks are achieving lower results. The second-best network is nano, with a mAP of 69.6 and precision of 64.7 percent [74].
Future research directions and challenges
In this section we discussed Systematic comparison of state-of-the-art Segmentation approaches through deep learning as well as we also suggest future research directions and challenges regarding these segmentation approaches [48]. Moreover, this process needs clinical testing for verification if we put this technique in lab practice for fast and quick semen analysis. Table 8 summarized Systematic comparison of state-of-the-art Segmentation approaches through deep learning. In first paper MOGS technique was used as a pre-processing step and it helps to reduce noise present in sample images and improve the overall segmentation process. Similarly, Fuzzy C-Means clustering shows impressive results when MOGS was applied on images as a pre-processing method. Moreover, Fuzzy C-Means clustering apply fuzziness for the belongingness of each image pixels, which increase efficiency of segmentation process. Author used K-Fold Cross Validation, with K = 5, Result shows Precision 83%, recall 82%, f-measure 83% and accuracy 88% respectively [68]. If Android-based embedded system will be deployed in clinical environment with real time working version of this proposed technique, we may get fast and robust diagnosis easily. In past many algorithms were unsuccessful to detect tail region from video dataset because of minor gradient in the tail region. Some researchers able to do so while denoising images. Somasundaram et al successfully separate tail region with binary images with the help of local region contour segmentation. Other methods that were used in literature measured with different densities of fluid. But in this study precision will not drop below 91%, even density was increased. So overall sperm detection process will be improved. Overall accuracy of this system is 97.37%. [60]. Minimum execution time was 1.12s and will be improved in future. Two well-known deep learning techniques U-Net and Mask-RCNN were proposed for sperm cell segmentation, using cross validation, hyper parameter tuning, transfer learning and data augmentation technique. Results shows that U-net with transfer learning, outclass state of the art sperm segmentation method with 95% overlapping efficiency against hand-segmented masks for sperm head 0.96, acrosome 0.94 and nucleus 0.95 using Dice coefficient as the evaluation metric [61]. These techniques show several opportunities for future research. First one, only focus on sperm head segmentation using U-net architecture rather than whole architecture. Second, computer-based sperm morphological analysis is hot research area and we plan to continue researching this area, and finally try to improve segmentation and classification result for fast and accurate analysis.
Ref | Dataset name | Method of Segmentation | Network | Evaluation metrics |
[48] | Convolutional Neural Network (CNN) | 3-conv-full-up-inc | Precision 93.87% Recall 91.89% |
|
[68] | Sperm Morphology Image Data Set (SMIDS) | K-Means clustering Fuzzy C-Means clustering |
Mobile-Net | Precision 83%, recall 82%, F-measure 83% and accuracy 88%. |
[60] | Video dataset | Local Region Contour segmentation | CNN network | Precision 97.37% |
[61] | SCIAN-SpermSegGS | U-Net and Mask- RCNN |
U-Net and MaskRCNN | 95% overlapping efficiency against hand-segmented masks for sperm head 0.96, acrosome 0.94 and nucleus 0.95. |
[69] | VISEM | Region Based Convolutional Neural Networks (R-CNN) architecture |
R-CNN | Accuracy 91.77 %. |
[62] | VISEM | Mean squared displacement of each particle (imsd) |
CNN RNN |
4.4% improvement against state-of-the-art results. |
[70] | MHSMA | Deep Transfer Learning (DTL) technique and Deep Multi-task Transfer Learning (DMTL) |
VGG19 | DTL technique accurately label head, vacuole, and acrosome with accuracy of 84%, 94%, and 79% respectively. Similarly, DMTL technique accurately label head, vacuole, and acrosome with 82%, 92.33%, and 80.66% respectively. |
[63] | Testicular biopsies | VGG16 | MobileNetV2 | mean average precision mAp 0.741 Average recall AR 0.376. |
[64] | Sperm image dataset | Enhanced Otsu's threshold method |
Deep CNN | Accuracy 98.99% |
[65] | SVIA dataset |
k-means, U-net, SegNet and DeepLabv3 | Deep CNN | The effects of U-net and Deeplab_v3 are the best, and other segmentation methods have a certain gap compared with these two segmentation methods |
[71] | VISEM | Crocker-Grier Algorithm With CNN |
RNN | The mean absolute error (MAE) could be reduced from 8.83 to 7.31. |
[72] | VISEM | YOLOv4 algorithm | YOLO Network | The results obtained were 90.31% AP (Average Precision) for sperm objects and 68.19% AP (Average Precision) for non-sperm objects, then for the results of the training obtained by the model 79.58% mAP (Mean Average Precision). |
The sperm counting algorithm using the Faster R-CNN-Inception_V2-COCO model, also gave us impressive result on sperm head segmentation. Its advantage is that it is quite accurate to learn to distinguish objects even from a small database. The model takes about 50 ms to analyse the image. Because the model uses regions, it is easier to detect a larger number of objects in a single frame. Additionally, the R-CNN model can read information from frames of any size, so there is no need to crop frames before submitting them to the neural network. This approach achieved 91.77% accuracy of sperm head detection on the VISEM sperm sample video dataset, which is largest available video dataset (32 GB) that is available online [69]. As a future work there is great option available to apply this modern technique on another online available standard dataset like MHSMA, SMIDS, HUSHEM AND SCIAN etc. Ottle et al predicted motility of a given sperm sample by using machine learning framework, embedded with unsupervised technique of feature extraction using downstream regression models. The results show a relative improvement of 4.4% against state-of-the-art techniques [62]. Author shows interest to provide this solution to everyone at low cost by integrating this method into digital microscope in future. Abbasi et al used Deep Transfer Learning (DTL) technique and Deep Multi-task Transfer Learning (DMTL) for segmentation. DTL technique accurately label head, vacuole, and acrosome with accuracy of 84%, 94%, and 79% respectively. Similarly, DMTL technique accurately label head, vacuole, and acrosome with 82%, 92.33%, and 80.66% respectively. Figure 10 shows visual representation of both models. We suggest this deep transfer learning approach should be applied on other online available datasets [70]. Regardless of outperforming all state-of-the-art techniques, this proposed method cannot give perfect results and f 0.5 score on all labels of the MHSMA dataset. So, there is room to improve results and accuracy. A new CASA system was developed with the help of deep learning that gave proximate human level performance on testicular sperm extraction (TESE). VGG16 convolution network employed or segmentation and trained on custom dataset. It classifies each proposal as either a spermatozoon or background and regresses the bounding box shape to match identified component objects. This deep CASA system gave mean average precision (mAP) of 0.741, with an average recall (AR) of 0.376 on custom dataset [63]. Researchers were focused on creating hand on tool for real-time sperm identification. Yet there is no accurate enough tool is available in market to embryologist on the number of spermatozoa found in each microscopy image but must be fast and accurate enough to outperform an embryologist in the amount of time it takes to find spermatozoa in a sample. We expect that future work will focus on locating and identifying sperm in TESE samples in tandem with embryologists. Enhanced Otsu's threshold method reduces the computation complexity for segmentation. Sobel operator was used for edge reduction, which improve overall performance of segmentation. The abnormal regions were detected by convolution layer. After comparing with other techniques author concluded that this method gave 98.99% accuracy [64]. Results may be improved if in future with the help of embedded genetic algorithms using deep CNN and multiple datasets will be chosen for comparative analysis.
In year 2022 Chen et al used subset–B of SVIA dataset for segmentation. To evaluate the effectiveness of SVIA dataset author employ four traditional image segmentation techniques and four deep leering techniques, among the classical image segmentation methods, OT has the best effect. But it is lower than the four image segmentation methods based on deep learning. In general, the effects of U-net and Deeplab_v3 were the best, and other segmentation methods have a certain gap compared with these two segmentation methods [65]. In the future, Author plans to increase the number of sperm microscopy videos and images, expand the number of GTs, add more sperm and impurity images information, and improve the quality of the images. Ottle et al continue their research work in 2022 by reducing the mean absolute error (MAE) from 8.83 to 7.31. Researcher focused on unsupervised tracking of sperm cells with the Crocker-Grier algorithm, extracting imsd features for each detected track and aggregating those features into a histogram representation by using BoW. With this feature representation, a linear SVR improved the mean (3-fold) MAE from 8.83 to 7.31, a decrease of over 17%. The results further show that the unsupervised feature quantization helps to achieve more consistent and robust results, regardless of which feature representation is chosen as input [71]. For future work, the presented framework can be extended and improved upon by pursuing a number of additional research directions. In last article of Systematic comparison. Aristoteles et al also use VISEM dataset for experiments. This dataset was available in the form of video and then converted into 1330 images. The training process was carried out with 3 different learning rate experiments, namely 0.002, 0.0002, 0.00002. In each of these experiments, 3 data divisions were made for each of the reading rates being tested. The best accuracy results are found in experiments with a learning rate of 0.0002 which has an accuracy value of 79.58% mAP on 70% train data distribution, 25% validation and 5% test. Each trial process for training uses 6000 iterations to create the training data. The test in this study uses video, the results of which are that all objects can be detected properly and have been labelled with a bounding box [72]. In this study there were cases where the model was not able to detect optimally because the video data used contained blurred objects and sperm objects that were cut off by the frame. In future author will try to reduce this problem and achieve better results by using new model of yolo architecture like yolov7 and yolov8.
In this section we discuss different classification method used in different researches and their outcomes. Ghasemain et al used SMA algorithm for classification and results shows above 90% of accuracy for sperm abnormality detection. It also shows high rates of True positive and True negative [35]. Duggal et al has presented Particle Swarm Optimization PSO algorithm for classification. PSO gives the highest accuracy then both MLP and SVM methods, PSO obtains superior specificity values at 88%. Therefore, PSO seems to be the preferred method for predicting the quality of fertility data with respect to the environmental factors and lifestyle, this method seems to be useful with the new data also as it can handle generalization potential [39]. In 2017 Shaker et al introduced Linear Discriminant Analysis (LDA) method for classification. To do so LDA minimize variance within class and maximize between classes, as a result maximum separation between classes was achieved [47].
Engy et al introduced Sperm Whale Optimization algorithm (SWA) with Artificial Neural Networks (ANN-SWA) on fertility dataset for classification. ANN-SWA was based on four phases. First one was trained by fertility diseases. In second multilayer perceptron (MLP) was employed to get maximum fitness function. In third phase parameter were optimized like biases and weights to get rapid conjunction with high accuracy. In final step ANN-SWA was employed to get sperm fertility prediction [49]. In next article researcher used 3D+t Human Sperm Flagellum Tracing in low SNR Fluorescence Images. Sperms were assigned by an ID for the sake of tracking. Proposed algorithm extract similar traces as compared with ground truth. Results shows that this algorithm was more accurate and robust than multi-branch algorithms. This enhanced 3D image permits guiding an iterative center line algorithm towards the flagellum's centerline. Each sperm is assigned an ID to keep track of it in 3D+t, this algorithm was extracting similar traces as compared with ground truth and it was more robust and accurate to trace the flagellum's centerline than multi-branch algorithms [51]. Dewan et al also employ CNN for classification. This CNN contain 5-layer network with 3 convolution layers with 16, 32 and 64 3 × 3 filters respectively. These were followed by 2 fully connected layers with 256 neurons in each layer and finally two node soft max layer that classify input into sperm and non-sperm [17]. Hicks et al also use CNN for classification. CNN was trained that learned temporal and spatial combined features of sperm motility. That was based on image preparation and representation of single or stacking frame. Then participant data and output of CNN model was combined. Before performing multivariate prediction on the three motility variables, this combined vector was passed through two fully-connected layers [33].
In 2019 McCallum et al presented CNN base VGG16 (CNN) technique for classification. Results shows that moderate correlation (bivariate correlation ~0.43) between DNA quality and sperm cell image, and ability to identify higher DNA integrity cells was related to median. This deep learning model will help clinicians for the microscopy-based sperm selection, it predicts fast DNA quality with in 10ms per cell and up to 86 % successful sperm selection for fertility analysis [53]. Movahed el al also introduce SVM classifier for classification. Multi-channel image generation and SFFS algorithm was optimized with SVM classifier to compete with other classifiers. As a result, axial filament pixels show improved performance and accurate classification [54]. Javadi et al employed CNN for classification of sperm image dataset into normal and abnormal sperms with the help of multiple morphological characteristics. CNN achieved F 0.5 score of 94.65%, 83.86% and 84.74% in vacuole, head and acrosome abnormality revealing, respectively. Figure 14 shows the proposed architecture [31].
Riordon et al used deep learning technique for sperm head classification. This Deep Neural Network architecture gave high accuracy in head-to-head comparison against other famous methods with identical dataset. Result shows better true positive rate 94.1% vs 92.3% on HuSHeM dataset, over cascade ensemble of SVM (CE-SVM) [32]. In 2020 Mohammadi et al used CSR-DCF tracker algorithm for classification. CSR-DCF algorithm was a robust multi-sperm tracker that works accurately, even when sperms collide or cross each other pass. This technique Obtained 96.61% F1 score from evaluation (Mohammadi et al., 2020). Zeadna et al proposed GBT and random forest method for classification and compare it with multivariate logistic regression model (MvLRM). GBT technique produced improved prediction results of successful and unsuccessful TESE against MvLRM, with little bit lower sensitivity. This model efficiently deals with missing values of several variables by automatically self-learning based on training loss reduction [57]. Lesani et al introduced two models, Full Spectrum and Selected Peak Neural Network for classification. To achieve low root, mean square error these models were boosted for transfer function, structure of hidden layer and different combination of training techniques. FSNN shows 93% prediction accuracy for sperm concentration and gave 100% assessments for differentiating the samples of patients and healthy donors [58]. Ilhan et al shows that SVM model with RBF kernel achieved highest accuracy that is 83.82% and tested by KAZE descriptor-based features. On the other hand, k-NN over DWT-based features shows worst classifier result with 47.23% efficiency. In terms of sperm morphology representation problem, KAZE features were the most informative features for RusBoost, Polynomial, Bagging and RBF kernel SVM classifiers (Ilhan et al., 2020). Iqbal et al used Morphological Classification of Human Sperm Heads MC-HSH for classification. In this deep learning architecture, there are a total of 53 convolutional layers. Before each convolutional layer, the batch normalization and LeakyReLU were used. They also apply the channel-wise concatenation and element-wise addition to make this model more efficient classification of human sperm heads in terms of morphology [59].
For classification of human sperm author introduced a novel Faster Region Convolutional Neural Network (FRCNN) with Elliptic Scanning Algorithm (ESA). More over for tracking and motility analysis Novel Tail to Head movement algorithm (THMA) was used. These proposed techniques improve the efficiency of computer assisted semen analysis (CASA) [60]. Priyansi et al use ResNet18 model for classification. The first ResNet18 model reached 100% accuracy on both validation and test sets. The second ResNet18 + Tabular Data model correctly predicted with an accuracy of 100% on the validation set and an average accuracy 88.89% on the test set. The third ResNet34 + Tabular Data model correctly predicted with an accuracy 87.5% on the validation set and 77.78% on the test set [8]. Figure 13 shows proposed framework by Ottl et al for-motility predictions. This framework shows best results for motility prediction. Figure 15 shows the proposed framework for motility prediction. The lower the Mean Absolute Error (MAE) the better, showing that SVR models achieve superior results than the other models for every type of features. BoWs outperform models trained on emsd features. Considering the best validation result, a minimum MAE of 8.60 was obtained on evaluation with the SVR trained on emsd features. Measured against the state-of-the-art, this result shows a relative improvement of 2.6% [62].
Abbasi et al used Deep Transfer Learning (DTL) technique and Deep Multi-task Transfer Learning (DMTL) for classification. The proposed algorithm achieved accuracy of vacuole, acrosome and head by 94%, 80.66% and 84% respectively by gaining improvement of 1.33%, 3% and 6.66% on these labels respectively. Therefore, this algorithm can be used in fertility institutes and make work easier for hospital staff [70]. Again, convolutional layers of VGG16 and GoogleNet used for classification. Deep learning CASA system achieved a mean average precision (mAP) of 0.741, with an average recall (AR) of 0.376 on given dataset [63].
Similarly, Prabaharan et al also use CNN for classification. MATLAB 2018a was employed to perform overall procedure. Classification process was improved with Deep CNN statistical training and morphology of sperm images. After comparison results shows efficient performance of proposed technique by providing neck and tail accuracy 97.99%, head utilize 98.22% and vacuole achieve 99.66% accuracy [64]. Chen et al applied 18 deep learning techniques on SVIA dataset. The precision, recall, F1-score and accuracy of Inception-v3 and DenseNet-121 were among the top. However, the precision, recall F1-score and accuracy of ViT were the lowest. So, for the image classification task of subset-C, Inception-v3 and DenseNet have the best classification effect, and ViT has the worst classification effect [65]. In next experiment author used all famous pre-trained deep learning models for classification like VGG16, VGG19, ResNet50, InceptionV3, InceptionResNetV2, MobileNet, MobileNetV2, DenseNet, NASNetMobile, NASNetLarge and Xception. VGG19 and ResNet50 shows best accuracy of 87.33%, 71% and 73% for vacuole, acrosome, and head label, respectively [76]. In last article of classification Miahi et al also use MHSMA dataset for Sperm morphology analysis. Author introduce a novel automatic SMA technique that is based on the neural architecture search algorithm, named Genetic Neural Architecture Search (GeNAS). This Neural Architecture method shows accuracy of 91.66%, 77.66%, and 77.33% in the vacuole, acrosome, and head detection, respectively [77] Mashaal et al work on a dataset consists of 1200 images of human sperm heads divided into healthy and unhealthy. Here, the VGG16 model is fine-tuned and achieved an accuracy of 97.92% and a sensitivity of 98.82%. Moreover, it achieved an F1 score of 98.53%. this is effective and real time system model for detecting healthy sperms that can be injected into eggs for achieving successful fertilization (Mashaal et al., 2022). In year 2023 shahzad et al introduced a new Sequential Deep Neural Network architecture (SDNN) that detected abnormalities in the acrosome, head, and vacuole with an accuracy of 89%, 90%, and 92%, respectively.
We evaluate the efficiency of SDNN technique, against 11 pre-trained state-of-the-art deep learning models mentioned in [78] tested with the same dataset. We found that SDNN model outperforms all modern deep-learning models by a significant margin. Table 9 presents the big picture of these models, besides our model with respect to Accuracy, F1-score, Recall and Precision in different parts of sperm cells.
Model | Accuarcy | Preceision | Recall | F1-score |
SDNN | 89.00 | 96.18 | 91.63 | 95.23 |
VGGI16 | 70.67 | 84.15 | 72.30 | 77.78 |
VGG19 | 71.00 | 83.87 | 73.24 | 78.20 |
ResNet.50 | 71.00 | 84.24 | 72.77 | 78.09 |
Inception V3 | 61.00 | 78.57 | 61.97 | 69.29 |
InceptipnResNet | 70.33 | 84.44 | 71.36 | 77.35 |
MobileNet | 71.00 | 85.00 | 71.83 | 77.86 |
MobileNetV2 | 69.00 | 81.58 | 72.77 | 76.92 |
DenseNaet | 66.00 | 81.71 | 67.14 | 73.71 |
NASNetMobile | 71.00 | 83.51 | 73.71 | 78.30 |
NASNetLarge | 63.00 | 80.36 | 63.38 | 70.87 |
Xception | 55.00 | 73.78 | 56.81 | 64.19 |
Model | Accuarcy | Preceision | Recall | F1-score |
SDNN | 92.00 | 97.70 | 93.43 | 95.52 |
VGGI16 | 87.33 | 96.28 | 88.93 | 92.46 |
VGG19 | 87.33 | 95.90 | 89.31 | 92.49 |
ResNet.50 | 87.33 | 95.53 | 89.69 | 92.52 |
Inception V3 | 82.67 | 94.49 | 85.11 | 89.56 |
InceptipnResNet | 84.33 | 95.36 | 86.26 | 90.58 |
MobileNet | 77.67 | 94.12 | 79.39 | 86.13 |
MobileNetV2 | 86.67 | 95.87 | 88.55 | 92.06 |
DenseNaet | 85.67 | 95.44 | 87.79 | 91.45 |
NASNetMobile | 86.00 | 95.45 | 88.17 | 91.67 |
NASNetLarge | 28.00 | 73.47 | 27.48 | 40.00 |
Xception | 48.00 | 86.30 | 48.09 | 61.76 |
Model | Accuarcy | Preceision | Recall | F1-score |
SDNN | 90.00 | 96.94 | 92.02 | 95.92 |
VGGI16 | 73.00 | 86.70 | 74.43 | 80.10 |
VGG19 | 73.33 | 87.17 | 74.43 | 80.30 |
ResNet.50 | 73.67 | 87.23 | 74.89 | 80.59 |
Inception V3 | 70.00 | 84.49 | 72.15 | 77.83 |
InceptipnResNet | 73.00 | 87.10 | 73.97 | 80.00 |
MobileNet | 73.00 | 86.32 | 74.89 | 80.20 |
MobileNetV2 | 62.67 | 81.29 | 63.47 | 71.28 |
DenseNaet | 72.33 | 86.56 | 73.52 | 79.51 |
NASNetMobile | 71.67 | 86.02 | 73.06 | 79.01 |
NASNetLarge | 69.67 | 84.41 | 71.69 | 77.53 |
Xception | 66.00 | 81.97 | 68.49 | 74.63 |
Neural Networks also used to solve multiple graph learning problem. In many machine learning applications, data are coming with multiple graphs, which is known as the multiple graph learning problem. Jiang et al proposed a novel learning framework, called Multiple Graph Learning Neural Networks (MGLNN), for multiple graph learning and multi-view semi-supervised classification. The objective of MGLNN is to simultaneously integrate multiple graph learning and GNN representation to learn an ideal graph structure from a variety of graph topologies that best supports GNN learning. The suggested MGLNN is an all-encompassing framework that can include any particular GNN model to deal with multiple graphs. To enhance/train the suggested MGLNN model, a general algorithm has also been created. MGLNN outperforms various other related approaches on semi-supervised classification tasks, according to experimental results on a number of datasets [20].
Similarly, after comparing with pre-trained modern deep learning models, author also compare this result with the previously proposed technique by different researchers with the same dataset. Eventually, as shown in Table 10, the proposed model shows higher accuracy, precision, recall and 𝑓0.5 on the test set for all three labels. Moreover, they demonstrated that, when applied to all three head, vacuole and acrosome labels of the MHSMA dataset, SDNN model can identify better than modern architectures than previously proposed model by different research, for instance manually designed CNN architectures, random search and image processing approaches, in terms of accuracy, precision, and f0.5 [77]. In Table 11 we elaborate all different classification Techniques and their respective outcomes.
Label | Method | Accuracy | Precision | Recall | f0.5 score |
Acrosome | |||||
SDNN | 89.00 | 96.18 | 91.63 | 95.23 | |
GeNAS | 77.66 | 84.76 | 83.56 | 84.42 | |
Random Search | 69.66 | 74.5 | 86.8 | 76.67 | |
Javadi et al. | 76.67 | 85.93 | 80.02 | 84.74 | |
Ghasemian et al. | N/A | N/A | N/A | N/A | |
Head | SDNN | 90.00 | 96.94 | 92.02 | 95.92 |
GeNAS | 77.33 | 84.47 | 84.47 | 84.47 | |
Random Search | 76.00 | 80.49 | 88.58 | 81.98 | |
Javadi et al. | 77.00 | 83.48 | 85.93 | 83.86 | |
Ghasemian et al. | 61.00 | 76.71 | 71.79 | 75.68 | |
Vacuole | SDNN | 92.00 | 97.70 | 93.43 | 96.82 |
GeNAS | 91.66 | 95.40 | 95.03 | 95.32 | |
Random Search | 89.00 | 94.20 | 93.12 | 93.98 | |
Javadi et al. | 91.33 | 94.36 | 95.80 | 94.65 | |
Ghasemian et al. | 80.33 | 83.21 | 93.56 | 85.09 |
Ref | Dataset name | Method of classification | Outcomes |
[35] | HSMA-DS | SMA algorithm | SMA algorithm was used for classification and results shows above 90% of accuracy for sperm abnormality detection. It also shows high rates of True positive and True negative |
[39] | - | Particle Swarm Optimization (PSO) algorithm |
PSO gives the highest accuracy then both MLP and SVM methods, PSO obtains superior specificity values at 88 %. Therefore, PSO seems to be the preferred method for predicting the quality of fertility data with respect to the environmental factors and lifestyle, this method seems to be useful with the new data also as it can handle generalization potential |
[47] | Linear Discriminant Analysis (LDA) | LDA was used for classification. To do so LDA minimize variance within class and maximize between classes, as a result maximum separation between classes was achieved. | |
[49] | Fertility Dataset | Artificial Neural Networks (ANN) Sperm Whale Optimization algorithm (SWA) |
ANN-SWA was based on four phases. First one was trained by fertility diseases. In second multilayer perceptron (MLP) was employed to get maximum fitness function. In third phase parameter were optimized like biases and weights to get rapid conjunction with high accuracy. In final step ANN-SWA was employed to get sperm fertility prediction. |
[51] | 3D+t | Algorithm based on one-class classification | Sperms were assigned by an ID for the sake of tracking. Proposed algorithm extract similar traces as compared with ground truth. Results shows that this algorithm is more accurate and robust than multi-branch algorithms |
[17] | Video dataset | CNN | This CNN contain 5-layer network with 3 convolution layers with 16, 32 and 64 3 × 3 filters respectively. These were followed by 2 fully connected layers with 256 neurons in each layer and finally two node soft max layer that classify input into sperm and non-sperm |
[33] | VISEM | CNN | CNN was trained that learned temporal and spatial combined features of sperm motility. That was based on image preparation and representation of single or stacking frame. Then participant data and output of CNN model was combined. Before performing multivariate prediction on the three motility variables, this combined vector was passed through two fully-connected layers. |
[53] | In-House dataset | VGG16 (CNN) | This deep learning model will help clinicians for the microscopy-based sperm selection, it predicts fast DNA quality with in 10ms per cell and up to 86 % successful sperm selection for fertility analysis. |
[54] | Gold-standard dataset | SVM classifier | Multi-channel image generation and SFFS algorithm was optimized with SVM classifier to compete with other classifiers. As a result, axial filament pixels show improved performance and accurate classification. |
[31] | MHSMA | Deep CNN | CNN was used for classification of sperm image dataset into normal and abnormal sperms with the help of multiple morphological characteristics. CNN achieved F 0.5 score of 94.65%, 83.86% and 84.74% in vacuole, head and acrosome abnormality revealing, respectively. |
[32] | HuSHeM and SCIAN | Deep Neural Network architecture | This Deep Neural Network architecture gave high accuracy in head-to-head comparison against other famous methods with identical dataset. Result shows better true positive rate 94.1% vs 92.3% on HuSHeM dataset, over cascade ensemble of SVM (CE-SVM). |
[56] | Video dataset | CSR-DCF tracker algorithm | CSR-DCF algorithm is a robust multi-sperm tracker that works accurately, even when sperms collide or cross each other pass. This technique Obtained 96.61% F1 score from evaluation. |
[57] | Gradient-Boosted Trees (GBT) and random forest, Logistic regression | GBT technique produced improved prediction results of successful and unsuccessful TESE against MvLRM, with little bit lower sensitivity. This model efficiently deals with missing values of several variables by automatically self-learning based on training loss reduction. | |
[58] | Full spectrum neural network (FSNN) Selected peak neural network (SPNN) |
To achieve low root mean square error these models were boosted for transfer function, structure of hidden layer and different combination of training techniques. FSNN shows 93% prediction accuracy for sperm concentration and gave 100% assessments for differentiating the samples of patients and healthy donors. | |
[68] | Sperm Morphology Image Data Set (SMIDS) | InceptionV3, VGG19 and MobileNet | Results shows that SVM model with RBF kernel achieved highest accuracy that is 83.82% and tested by KAZE descriptor-based features. On the other hand, k-NN over DWT-based features shows worst classifier result with 47.23% efficiency. In terms of sperm morphology representation problem, KAZE features were the most informative features for RusBoost, Polynomial, Bagging and RBF kernel SVM classifiers |
[59] | HuSHeM and SCIAN | Morphological Classification of Human Sperm Heads MC-HSH |
In this deep learning architecture, there are a total of 53 convolutional layers. Before each convolutional layer, the batch normalization and LeakyReLU were used. They also apply the channel-wise concatenation and element-wise addition to make this model more efficient classification of human sperm heads in terms of morphology. |
[60] | Video dataset | Elliptic Scanning Algorithm (ESA) Novel Faster Region Convolutional Neural Network (FRCNN), |
A novel FRCNN with ESA detection algorithm was introduced classification and analysis of human sperm. Results shows accuracy of 97.37%. Moreover, for tracking and motility analysis a Tail head movement-based (THMA) algorithm was used. |
[8] | VISEM | ResNet18 model | The first ResNet18 model reached 100% accuracy on both validation and test sets. The second ResNet18 + Tabular Data model correctly predicted with an accuracy of 100% on the validation set and an average accuracy 88.89 on the test set. The third ResNet34 + Tabular Data model correctly predicted with an accuracy 87.5% on the validation set and 77.78% on the test set. |
[62] | VISEM | Linear Support vector Regressor, MultiLayer, perceptron, CNN, RNN |
This technique shows best results for motility prediction and state-of-the-art. The lower the Mean Absolute Error (MAE) the better, showing that SVR models achieve better results than the other models for every type of features. BoWs outperform models trained on emsd features. Considering the best validation result, a minimum MAE of 8.60 is obtained on evaluation with the SVR trained on emsd features. Measured against the state-of-the-art, this result shows a relative improvement of 2.6% |
[70] | MHSMA | Deep Transfer Learning (DTL) technique Deep Multi-task Transfer Learning (DMTL) |
The proposed algorithm achieved accuracy of vacuole, acrosome and head by 94%, 80.66% and 84% respectively by gaining improvement of 1.33%, 3% and 6.66% on these labels respectively. Therefore, this algorithm can be used in fertility institutes and make work easier for hospital staff. |
[63] | Testicular biopsies | Convolutional layers of VGG16, GoogleNet | Deep learning CASA system achieved a mean average precision (MAP) of 0.741, with an average recall (AR) of 0.376 on given dataset. |
[64] | Sperm image dataset | Deep Convolutional Neural Network | MATLAB 2018a was employed to perform overall procedure. Classification process was improved with Deep CNN statistical training and morphology of sperm images. After comparison results shoes efficient performance of proposed technique by providing neck and tail accuracy 97.99%, head utilize 98.22% and vacuole achieve 99.66 accuracy. |
[65] | SVIA dataset |
AlexNet, VGG, ResNet, GoogleNet, DenseNet, Inception-v3, Mobilenet-v2, ShuffleNet-v2, Xception, Vision Transformer ViT, BotNet, DeiT, T2T-ViT. | Inception-v3 and DenseNet-121 shows maximum accuracy 98.32 % and 98.06 % respectively. While ViT shows lowest result i.e 93 % |
[76] | MHSMA | VGG19 and ResNet50 | VGG19 and ResNet50 shows best accuracy of 87.33%, 71% and 73% for vacuole, acrosome, and head label, respectively. |
[77] | MHSMA | Genetic Neural Architecture Search | This Neural Architecture method shows accuracy of 91.66%, 77.66%, and 77.33% in the vacuole, acrosome, and head detection, respectively |
[79] | In-House dataset | VGG16 (CNN) | The VGG16 model is fine-tuned and achieved an accuracy of 97.92% and a sensitivity of 98.82%. Moreover, it achieved an F1 score of 98.53%. |
[78] | MHSMA | Sequential Deep Neural Network architecture (SDNN) | Results show that Sequential Deep Neural Network architecture successfully detected abnormalities in the acrosome, head, and vacuole with an accuracy of 89%, 90%, and 92%, respectively. Moreover, it also out class all state-of-the-art model as well as GeNAS algorithm proposed by Miahi et al. |
Future research directions and challenges
In this section we discussed Systematic comparison of state-of-the-art classification approaches through deep learning as well as we also suggest future research directions and challenges regarding these segmentation approaches. Table 12 represent method of classification with proposed neural network and their evaluation metrics. In first paper author use CSR-DCF algorithm with a robust multi-sperm tracker that works accurately, even when sperms collide or cross each other pass. Result shows precision 99.1% and achieve 96.61% F1 score from evaluation of their proposed tracker method(Mohammadi et al., 2020). This experiment was performed on small dataset of 25 frames per video, so there is a space of research available and this technique will be applied on other larger dataset available online and compare results with previous ones. Zeadna et al proposed GBT and random forest method for classification and compare it with multivariate logistic regression model (MvLRM). GBT technique produced improved prediction results of successful and unsuccessful TESE against MvLRM, with little bit lower sensitivity. This model efficiently deals with missing values of several variables by automatically self-learning based on training loss reduction. GBT technique shows sensitivity of 91% and MvLRM shows sensitivity of 97% [57]. Machine-learning models may lay the foundation for a decision support system for clinicians together with their NOA patients concerning TESE. The findings of this study should be confirmed with further larger and prospective studies. Well trained Full Spectrum and Selected Peak Neural Network was used for sperm classification. To achieve low root, mean square error these models were boosted for transfer function, structure of hidden layer and different combination of training techniques. FSNN shows 93% prediction accuracy for sperm concentration and gave 100% assessments for differentiating the samples of patients and healthy donors [58]. However, by increasing sperm concentration by increasing sample size, must improve prediction accuracy of this method and increase chance of clinical adoption. Ilhan et al shows that SVM model with RBF kernel achieved highest accuracy that is 83.82% and tested by KAZE descriptor-based features. On the other hand, k-NN over DWT-based features shows worst classifier result with 47.23% efficiency. In terms of sperm morphology representation problem, KAZE features were the most informative features for RusBoost, Polynomial, Bagging and RBF kernel SVM classifiers (Ilhan et al., 2020). If Android-based embedded system will be deployed in clinical environment with real time working version of this proposed technique, we may get fast and robust diagnosis easily. Similarly, a novel Faster Region Convolutional Neural Network (FRCNN) was used for efficient sperm classification with Elliptic Scanning Algorithm (ESA). These proposed techniques improve the efficiency of computer assisted semen analysis (CASA). Results shows accuracy of 97.37%. Moreover, for tracking and motility analysis a Tail head movement-based (THMA) algorithm was used [60]. Researcher were satisfied with results of proposed architecture and plan to implement this technique in infertility centres in near future for healthy sperm detection in semen sample. Priyansi et al use ResNet18 model for classification. The first ResNet18 model reached 100% accuracy on both validation and test sets. The second ResNet18 + Tabular Data model correctly predicted with an accuracy of 100% on the validation set and an average accuracy 88.89% on the test set. The third ResNet34 + Tabular Data model correctly predicted with an accuracy 87.5% on the validation set and 77.78% on the test set [8]. This experiment concluded that the addition of tabular data decreased the accuracy of the model, which isn't necessarily an architecture limitation as the accuracy decreased moreover when a heavier 3D ResNet34 was applied, they found that these features were redundant for the training and classification of spermatozoa motility as they cause data leakage. The proposed models can be applied to automating the semen analysis process in the future which currently uses images instead of video and can even extend to predicting other quality parameters. Figure 12 shows proposed framework by Ottl et al for-motility predictions. This framework shows best results for motility prediction. The lower the Mean Absolute Error (MAE) the better, showing that SVR models achieve superior results than the other models for every type of features. BoWs outperform models trained on emsd features. Considering the best validation result, a minimum MAE of 8.60 was obtained on evaluation with the SVR trained on emsd features. Measured against the state-of-the-art, this result shows a relative improvement of 2.6% [62]. Author shows interest to provide this solution to everyone at low cost by integrating this method into digital microscope. Abbasi et al used Deep Transfer Learning (DTL) technique and Deep Multi-task Transfer Learning (DMTL) for classification. The proposed algorithm achieved accuracy of vacuole, acrosome and head by 94%, 80.66% and 84% respectively by gaining improvement of 1.33%, 3% and 6.66% on these labels respectively. Therefore, this algorithm can be used in fertility institutes and make work easier for hospital staff [70]. Regardless of outperforming all state-of-the-art techniques, this proposed method cannot give perfect results and f 0.5 score on all labels of the MHSMA dataset. So, there is room to improve results and accuracy. A new CASA system was developed with the help of deep learning that gave proximate human level performance on testicular sperm extraction (TESE). VGG16 convolution network employed or segmentation and trained on custom dataset. It classifies each proposal as either a spermatozoon or background and regresses the bounding box shape to match identified component objects. This deep CASA system gave mean average precision (mAP) of 0.741, with an average recall (AR) of 0.376 on custom dataset. Again, convolutional layers of VGG16 and GoogleNet used for classification. Deep learning CASA system achieved a mean average precision (mAP) of 0.741, with an average recall (AR) of 0.376 on given dataset [63]. After initial testing of the model, it will be deployed in an academic in vitro fertilization centre as a research tool for further testing. they intend to deploy the model as a real-time video classification pipeline, which automatically identifies sperm in testicular tissue. This novel tool will improve the efficiency of searching for sperm in testicular biopsy samples, which is currently a labour-intensive process dependent on the skill of the embryologist. Similarly, Prabaharan et al also use CNN for classification. MATLAB 2018a was employed to perform overall procedure. Classification process was improved with Deep CNN statistical training and morphology of sperm images. After comparison results shows efficient performance of proposed technique by providing neck and tail accuracy 97.99%, head utilize 98.22% and vacuole achieve 99.66% accuracy [64]. Results may be improved if in future through embedded genetic algorithms with deep CNN and multiple datasets will be chosen for comparative analysis. In year 2022 Chen et al applied 18 deep learning techniques on SVIA dataset. The precision, recall, F1-score and accuracy of Inception-v3 and DenseNet-121 were among the top. However, the precision, recall F1-score and accuracy of ViT were the lowest. So, for the image classification task of subset-C, Inception-v3 and DenseNet have the best classification effect, and ViT has the worst classification effect [65]. In the future, Author plans to increase the number of sperm microscopy videos and images, expand the number of GTs, add more sperm and impurity images information, and improve the quality of the images. Similarly in same year Chandra et al used pre-trained deep learning models as a feature extraction like VGG16, VGG19, ResNet50, InceptionV3, InceptionResNetV2, MobileNet, MobileNetV2, DenseNet, NASNetMobile, NASNetLarge and Xception. VGG19 and ResNet50 shows best accuracy of 87.33%, 71% and 73% for vacuole, acrosome, and head label, respectively [76]. Moreover, the size of the current dataset is one of the enormous existing available datasets, but it needs to be enlarged for further experimental assessment of the algorithms in future. In last article of classification Miahi et al also use MHSMA dataset for Sperm morphology analysis. Author introduce a novel automatic SMA technique that is based on the neural architecture search algorithm, named Genetic Neural Architecture Search (GeNAS). This Neural Architecture method shows accuracy of 91.66%, 77.66%, and 77.33% in the vacuole, acrosome, and head detection, respectively [77]. Author proved that GeNAS can ascertain better state-of-the-art architectures – in terms of accuracy, precision, and 𝑓0.5 measure–compared to previously proposed methods, such as hand designed CNN architectures, image processing approaches, and random search, with less amount of computational power and human effort on all three acrosome, head, and vacuole labels of MHSMA dataset. Moreover, the architectures discovered by GeNAS have exceptionally fewer parameters on the head and vacuole labels. Finally, concerning the lack of NAS research to address the challenges of real-world datasets, author recommend that further research should be done in this area of research. In last paper shahzad et al evaluate the efficiency of SDNN technique, against 11 pre-trained state-of-the-art deep learning models mentioned in [78] tested with the same dataset. We found that SDNN model outperforms all modern deep-learning models by a significant margin. Table 9 presents the big picture of these models, besides our model with respect to Accuracy, F1-score, Recall and Precision in different parts of sperm cells. In future we suggest to compare this technique with other dataset as well as provide a mobile application to test sperm samples in real time environment.
Ref | Dataset name | Method of Classification | Network | Evaluation metrics |
[56] | Video dataset | CSR-DCF tracker algorithm | RetinaNet | Precision 99.1 % F1 score 96.61% |
[57] | Machine-learning model |
Gradient-Boosted Trees (GBT) and random forest, Logistic regression | GBT sensitivity 91% MvLRM sensitivity 97% |
|
[58] | ANN | Full spectrum neural network (FSNN) Selected peak neural network (SPNN) |
Accuracy 93% | |
[80] | Sperm Morphology Image Data Set (SMIDS) | Wavelet Descriptor-based features with SVM |
MobileNet | Accuracy 80.5% and 83.8% |
[60] | Video dataset | Elliptic Scanning Algorithm (ESA) Novel Faster Region Convolutional Neural Network (FRCNN), |
FRCNN | Accuracy 97.37%. |
[8] | VISEM | CNN | ResNet18 ResNet34 |
Accuracy 88.89% Accuracy 77.78% |
[62] | VISEM | Four Machine-learning models |
SVR, MLP, CNN, RNN |
Mean Absolute Error 7.31 |
[70] | MHSMA | Deep CNN | Deep Transfer Learning (DTL) technique Deep Multi-task Transfer Learning (DMTL) |
DTL technique accurately label head, vacuole, and acrosome with accuracy of 84%, 94%, and 79% respectively. Similarly, DMTL technique accurately label head, vacuole, and acrosome with 82%, 92.33%, and 80.66% respectively. |
[63] | Testicular biopsies | CNN | VGG GoogleNet 16 |
mean average precision (mAP) of 0.741 average recall (AR) of 0.376 |
[64] | Sperm image dataset | CNN | Deep CNN | Neck and tail accuracy 97.99%, head utilize 98.22% and vacuole achieve 99.66% accuracy |
[65] | SVIA dataset |
18 Deep Learning models | AlexNet, VGG, ResNet, GoogleNet, DenseNet, Inception-v3, Mobilenet-v2, ShuffleNet-v2, Xception, Vision Transformer ViT, BotNet, DeiT, T2T-ViT. | Inception-v3 and DenseNet-121 shows maximum accuracy 98.32 % and 98.06 % respectively. While ViT shows lowest result i.e 93 % |
[76] | MHSMA | Deep CNN | VGG19 and ResNet50 | VGG19 and ResNet50 shows best accuracy of 87.33%, 71% and 73% for vacuole, acrosome, and head label, respectively. |
[77] | MHSMA | CNN | Genetic Neural Architecture Search | This Neural Architecture method shows accuracy of 91.66%, 77.66%, and 77.33% in the vacuole, acrosome, and head detection, respectively |
[78] | MHSMA | Deep CNN | Sequential Deep Neural Network architecture (SDNN) | Results show that Sequential Deep Neural Network architecture successfully detected abnormalities in the acrosome, head, and vacuole with an accuracy of 89%, 90%, and 92%, respectively. |
In this section we discuss Evaluation metrics techniques used by different authors. Table 13 explain different Evaluation metrics used by different researcher and their respective results. In first paper Bijar et al used expectation–maximization (EM) algorithm and Markov random field (MRF) model for segmentation. Results shows 97.6% overall success rate of this model. Moreover, Accuracy of sperm's head, Accuracy (A c) 96.82%, Sensitivity (S c) 97.52% and Specificity (S p) 95.27% respectively [36]. Ghasemain et al uses confusion metrics for evaluation. SMA algorithm shows its performance in less than 9 seconds. True negative rate was 100% for tail malformation detection. However, there was small number of false negative due to high rate of noise in the image [35]. Tan et al used Confusion metrics for evaluation in term of Accuracy, sensitivity, specificity and precision. Results show Accuracy 0.97, sensitivity 0.97, specificity 0.71 and precision 0.99 respectively [41]. In next paper the presented methods and their improvements can at least replace the initial tests for checking the fertility of a population or an individual as well as in selecting the donors for data collection. Stating and testing environmental and lifestyle factors trough the mentioned techniques were able to give an accuracy of up-to 90%, these results support the argument [39]. Similarly, Tan et al apply Intersecting cortical model (ICM) technique on video dataset in 2016. Results shows that this model was more robust and accurate than four state of the art segmentation models, in terms of accuracy, sensitivity, specificity and precision with success rate of 98.14%, 98.82%, 86.46% and 99.81% respectively [41]. In same year shaker et al apply different techniques for segmentation and achieve remarkable results. They use Dice coefficient to evaluate segmentation performance. Dice coefficient was 3% more than Chang method. Total performance was 88% [42]. The proposed method of Chang at al shows 73% efficiency as well as human experts were fully satisfied with the classification of sperm heads [67]. Shaker et al used machine learning based approach for classification of sperm head into 4 different classes. This method achieved average F-score of 92.9%, average recall of 92.3%, average precision of 93.5%, and average accuracy of 92.2%. This method shows very impressive result in abnormalities recognizing for all above mentioned four classes [81]. In next paper results shows the average accuracy of overall classes reached the 78% by using confusion metrics measurements [44]. Engy et al used 10-fold cross validation for evaluation metric and show that artificial neural network based on SWA achieved more than 99.96% of the accuracy [49]. Movahed et al apply 3 techniques for evaluation metric, namely Dice, Jaccard, and F1-score metrics respectively. The CNN was validated with 20 images of sperm sample. Results shows 0.94, 0.87, and 0.88 of Dice Similarity Coefficient for the head, the acrosome, and the nucleus segments, respectively [52]. In last paper of 2018 dewan et al use SQA-V Gold for evaluation. They conclude that SQA-V Gold obtain a B0 value of 1.91 and confidence interval of (−12.6 to −0.57) and B1 value of 1.665 with 95% confidence interval of (1.5 to 1.8) [17]. Javadi et al used CCN for segmentation. The proposed technique attains F0.5 Score of 83.86%, 84.74% and 94.65% in head, acrosome and vacuole abnormality detection, respectively [31].
Ref | Dataset name | Evaluation metrics | Outcomes |
[36] | Automated Sperm Morphology Analyzer (ASMA) |
Accuracy (A c) 96.829% Sensitivity (S c) 97.522% Specificity (S p) 95.275% |
|
[35] | HSMA-DS | Confusion metrics | SMA algorithm shows its performance in less than 9 seconds. True negative rate was 100% for tail malformation detection. However, there was small number of False negative due to high rate of noise in the image. |
[82] | Video dataset | Confusion metrics | Accuracy 0.97, sensitivity 0.97, specificity 0.71 and precision 0.99 |
[39] | - | confusion metrics, 12 parameters used | The presented methods and their improvements can at least replace the initial tests for checking the fertility of a population or an individual as well as in selecting the donors for data collection. Stating and testing environmental and lifestyle factors trough the mentioned techniques are able to give an accuracy of up-to 90%, these results support the argument. |
[41] | Video dataset | Intersecting cortical model (ICM) | Results shows that this model is more robust and accurate than four state of the art segmentation models, in terms of accuracy, sensitivity, specificity and precision with success rate of 98.14%, 98.82%, 86.46% and 99.81% respectively. |
[42] | Gold-standard | Dice Coefficient | Dice coefficient was used to evaluate segmentation performance. Dice coefficient was 3% more than Chang method. Total performance was 88%. |
[45] | SCIAN-MorphoSpermGS | Two-stage classification | Results shows 73% efficiency as well as human experts were fully satisfied with the classification of sperm heads. |
[47] | Confusion metrics | This method achieved average F-score of 92.9 %, average recall of 92.3 %, average precision of 93.5%, and average accuracy of 92.2%. This method shows very impressive result in abnormalities recognizing for all above mentioned four classes. | |
[44] | Confusion metrics | The average accuracy overall classes reached the 78% by using confusion metrics measurements. | |
[49] | Fertility Dataset | 10-fold cross validation | 99.96% of the accuracy. |
[52] | Gold-standard dataset | Dice, Jaccard, and F1-score metrics | The CNN was validated with 20 images of sperm sample. Results shows 0.94, 0.87, and 0.88 of Dice Similarity Coefficient for the head, the acrosome, and the nucleus segments, respectively |
[17] | Video dataset | SQA-V Gold | SQA-V Gold obtain a B0 value of 1.91 and confidence interval of (−12.6 to −0.57) and B1 value of 1.665 with 95% confidence interval of (1.5 to 1.8) |
[31] | MHSMA | Confusion metrics | The proposed technique attains F0.5 Score of 83.86%, 84.74% and 94.65% in head, acrosome and vacuole abnormality detection, respectively. |
[56] | Video dataset | Average precision (AP) metric | Precision 99.1 % and obtained 96.61% F1 score from evaluation of their proposed tracker method. |
[58] | 13-fold cross validation | 13-fold cross validation shows average classification accuracy of 92% was achieved by the system | |
[68] | Sperm Morphology Image Data Set (SMIDS) | K-Fold Cross Validation, with K=5 |
Precision 83%, recall 82%, f-measure 83% and accuracy 88%. |
[60] | Video dataset | Confusion metrics | The Faster Region Convolutional Neural Network (FRCNN) was used for classification and improve the efficiency of computer assisted semen analysis (CASA). Other methods that were used in literature measured with different densities of fluid. But in this study precision will not drop below 91%, even density was increased. So overall sperm detection process will be improved. |
[69] | VISEM | Region Based Convolutional Neural Networks (R-CNN) |
The motility of the semen sample calculated by the algorithm differs from the experimental result by only 2.92% on average, while the accuracy of sperm head detection was 91.77%. |
[61] | SCIAN-SpermSegGS | cross validation, Dice coefficient | Results show that U-net with transfer learning, outclass state of the art sperm segmentation method with 95% overlapping efficiency against hand-segmented masks for sperm head 0.96, acrosome 0.94 and nucleus 0.95. |
[65] | SVIA dataset |
Confusion metrics | Inception-v3 and DenseNet-121 shows maximum accuracy 98.32 % and 98.06 % respectively. While ViT shows lowest result i.e 93 % |
[71] | VISEM | 3-fold cross-validation | The mean absolute error (MAE) could be reduced from 8.83 to 7.31. |
[72] | VISEM | Confusion metrics | The results obtained were 90.31% AP (Average Precision) for sperm objects and 68.19% AP (Average Precision) for non-sperm objects, then for the results of the training obtained by the model 79.58% mAP (Mean Average Precision). |
[77] | MHSMA | Confusion metrics | This Neural Architecture method shows accuracy of 91.66%, 77.66%, and 77.33% in the vacuole, acrosome, and head detection, respectively |
[75] | MHSMA | Confusion metrics | VGG19 and ResNet50 shows best accuracy of 87.33%, 71% and 73% for vacuole, acrosome, and head label, respectively. |
[79] | In-House dataset | Confusion metrics | The VGG16 model is fine-tuned and achieved an accuracy of 97.92% and a sensitivity of 98.82%, precision equals 98.25%, specificity equals 95.71% and F1 Score equals 98.53% Moreover, it achieved an F1 score of 98.53%. |
[75] | VISEM | Different evaluation metrics | YOLOv5 deep learning model trained on the VISEM-Tracking dataset present baseline sperm detection performances. This dataset can be used to train complex deep learning models to analyze spermatozoa. |
[74] | VISEM | detailed quantitative investigation | The best-performing model is yolov5l. This network achieves a precision of 88.6 per cent, recall of 52.6, and mAP is 72.1. Other networks are achieving lower results. The second-best network is nano, with a mAP of 69.6 and precision of 64.7 per cent |
[78] | MHSMA | Confusion metrics | Results show that Sequential Deep Neural Network architecture successfully detected abnormalities in the acrosome, head, and vacuole with an accuracy of 89%, 90%, and 92%, respectively. |
In 2020 Mohammadi et al used Average precision (AP) metric for evaluation. Result shows precision 99.1% and achieve 96.61% F1 score from evaluation of their proposed tracker method (Mohammadi et al., 2020). Zeadna et al propose leave-one-out cross-validation for evaluation. The leave-one-out cross-validation procedure allows us to use the largest available training set and achieve an unbiased estimate for the expected accuracy [57]. To validate the performance of two model, 13-fold cross validation was employed by Lesani et al. Results shows average classification accuracy of 92% was achieved by the system [58]. Ilhan et al used K-Fold Cross Validation, with K = 5, Result shows Precision 83%, recall 82%, f-measure 83% and accuracy 88% respectively [80].
The Faster Region Convolutional Neural Network (FRCNN) was used for classification and improve the efficiency of computer assisted semen analysis (CASA). Other methods that were used in literature measured with different densities of fluid. But in this study precision will not drop below 91%, even density was increased. So overall sperm detection process will be improved(Somasundaram et al., 2021). Author use Region Based Convolutional Neural Networks (R-CNN) for segmentation. The motility of the semen sample calculated by the algorithm differs from the experimental result by only 2.92% on average, while the accuracy of sperm head detection was 91.77% [69]. In this article author use dice confident as an evaluation metrics. Results show that U-net with transfer learning, outclass state of the art sperm segmentation method with 95% overlapping efficiency against hand-segmented masks for sperm head 0.96, acrosome 0.94 and nucleus 0.95 [61]. Abbasi et al used Confusion metrics and Gradient-weighted Class Activation Mapping (Grad-CAM) for evaluation. To classify the vacuole part of a sperm image, our model should pay more attention to the vacuole of the sperm during the classification process. Chen et al applied 18 deep learning techniques on SVIA dataset. The precision, recall, F1-score and accuracy of Inception-v3 and DenseNet-121 were among the top. However, the precision, recall F1-score and accuracy of ViT were the lowest. So for the image classification task of subset-C, Inception-v3 and DenseNet have the best classification effect, and ViT has the worst classification effect [65]. In next study Ottl et al shows best results by unsupervised tracking of sperm cells with the Crocker-Grier algorithm, extracting imsd features for each detected track and aggregating those features into a histogram representation using BoW. With this feature representation, a linear SVR improved the mean (3-fold) MAE from 8.83 to 7.31, a decrease of over 17%. The results further show that the unsupervised feature quantization helps to achieve more consistent and robust results, regardless of which feature representation is chosen as input [71]. In this experiment dataset was available in the form of video and then converted into 1330 images. The training process was carried out with 3 different learning rate experiments, namely 0.002, 0.0002, 0.00002. In each of these experiments, 3 data divisions were made for each of the reading rates being tested. The best accuracy results are found in experiments with a learning rate of 0.0002 which has an accuracy value of 79.58% mAP on 70% train data distribution, 25% validation and 5% test. Each trial process for training uses 6000 iterations to create the training data. The test in this study uses video, the results of which are that all objects can be detected properly and have been labelled with a bounding box. In this study there were cases where the model was not able to detect optimally because the video data used contained blurred objects and sperm objects that were cut off by the frame [72]. Miahi et al also use MHSMA dataset for Sperm morphology analysis. Author introduce a novel automatic SMA technique that is based on the neural architecture search algorithm, named Genetic Neural Architecture Search (GeNAS). This Neural Architecture method shows accuracy of 91.66%, 77.66%, and 77.33% in the vacuole, acrosome, and head detection, respectively [77]. In last article author used pre-trained deep learning models as a feature extraction like VGG16, VGG19, ResNet50, InceptionV3, InceptionResNetV2, MobileNet, MobileNetV2, DenseNet, NASNetMobile, NASNetLarge and Xception. VGG19 and ResNet50 shows best accuracy of 87.33%, 71% and 73% for vacuole, acrosome, and head label, respectively [76]. Thambawita et al present YOLOv5 deep learning model that is trained on the VISEM-Tracking dataset present baseline sperm detection performances. YOLOv5l performed best among all other versions of yolov5. This network achieves a precision of 43 per cent, recall of 25, and mAP is 22.3. Other networks are achieving lower results. The second-best network is medium, with a mAP of 22.1 and precision of 57.1 per cent. This dataset can be used to train complex deep learning models to analyze spermatozoa [75]. Similarly, Dobrovolny et al aslo use yolov5 for sperm cell detection and achieved very efficient results. The best-performing model is yolov5l. This network achieves a precision of 88.6 per cent, recall of 52.6, and mAP is 72.1. Other networks are achieving lower results. The second-best network is nano, with a mAP of 69.6 and precision of 64.7 per cent [74]. Mashaal et al work on a dataset consists of 1200 images of human sperm heads divided into healthy and unhealthy. Here, the VGG16 model is fine-tuned and achieved an accuracy of 97.92% and a sensitivity of 98.82%. Moreover, it achieved an F1 score of 98.53%. this is effective and real time system model for detecting healthy sperms that can be injected into eggs for achieving successful fertilization [79]. In year 2023 shahzad et al introduced a new Sequential Deep Neural Network architecture (SDNN) that detected abnormalities in the acrosome, head, and vacuole with an accuracy of 89%, 90%, and 92%, respectively.
In this study we consider 29 image dataset and 15 video datasets from latest published articles. We also mention source of dataset, no of images or videos included, method of recording dataset, their color space, resolution and target class. In next section we discussed pre-processing technique applied by different researchers. Total 17 techniques were applied on their respective datasets. Famous pre-processing technique was MOGS. Ilhan et al used these techniques in 2018 and 2020, In MOGS, convex function was used to detect the correct region of sperm, therefore denoising problem was accepted in which a non-convex regularization term was employed and this verify the desired group sparsity property. This technique helps to remove random noise without changing sperm shapes. Other techniques included CNN, Median filter, MobileNetV2, Rotation Translation flipping and elliptic curve were also used in literature for pre-processing. In next segment feature extraction techniques was discussed after pre-processing, that were based on machine Learning. Ten techniques were mention in this study included Phase Map Acquisition, five shape-based descriptors, Pixel-Based Spatial analyses, Blob analyses, Lucene Image Retrieval (LIRE) Tamura image features and Gaussian mixture model etc. similarly we also discussed feature extraction techniques based on deep learning. Thirteen deep learning technique were considered like DNA Fragmentation Index (DFI), CNN, VGG16 CNN, Feature Pyramid Network (FPN), 3D ResNet 18, RPN network, Inception, Resnet, MobileNet, MobileNetV2, Single-Shot Detector (SSD), ImageNet etc. Similarly, we also discuss 10 segmentation techniques with machine learning. Most researcher use Support Vector Machines for segmentation but some researcher also used Fuzzy C-Means (FCM) and K-Mean, Threshold segmentation, Clustering-Based Decision Forests (CBDF Algorithm) etc. on the other hand we also discuss eighteen deep learning techniques for segmentation, because capable of automatically extracting high-dimensional features from the input raw data. Marin et al used U-Net and Mask-RCNN for segmentation. Results show that U-net with transfer learning, outclass state of the art sperm segmentation method with 95% overlapping efficiency against hand-segmented masks for sperm head 0.96, acrosome 0.94 and nucleus 0.95. Other methods also show remarkable results like CNN, VGG16, DTL and DMTL etc. In next section we combine discuss machine learning and deep learning methods for classification. Most researcher used CNN, VGG19 and SVM classifier for classification process. Another network included Full Spectrum and Selected Peak Neural Network. To achieve low root, mean square error these models were boosted for transfer function, structure of hidden layer and different combination of training techniques. FSNN shows 93% prediction accuracy for sperm concentration and gave 100% assessments for differentiating the samples of patients and healthy donors. Similarly, the first ResNet18 model reached 100% accuracy on both validation and test sets. The second ResNet18 + Tabular Data model correctly predicted with an accuracy of 100% on the validation set and an average accuracy 88.89 on the test set. The third ResNet34 + Tabular Data model correctly predicted with an accuracy 87.5% on the validation set and 77.78% on the test set. Moreover, different version of YOLO algorithm also plays an important role in sperm cell detection with great efficient and accuracy. Like Dobrovolny et al also use yolov5 for sperm cell detection and achieved very efficient results. The best-performing model was yolov5l. This network achieves a precision of 88.6 per cent, recall of 52.6, and mAP is 72.1. Other networks are achieving lower results. The second-best network is nano, with a mAP of 69.6 and precision of 64.7 per cent. Similarly Sequential deep learning approach significantly outperformed existing Sperm Morphology Analysis (SMA) methods regarding the accuracy, precision, recall, f0.5, and f1 score. Notably, the accuracy of the head, acrosome and vacuole labels using SDNN technique was 90%, 92%, and 89%, respectively. Significantly, Sequential deep learning has never been used to examine sperm morphology, mainly when combined stack network-based deep learning is used. Furthermore, we hypothesize that the ground-breaking SDNN technique can also be applied to address issues related to the SMA in the real world, like fertility clinics, etc.
This research received no external funding.
The authors declare no conflict of interest.
[1] |
V. Kumar, P. G. Kumar, J. K. Yadav, Impact of semen-derived amyloid (SEVI) on sperm viability and motility: Its implication in male reproductive fitness, Eur. Biophys. J., 48 (2019), 659–671. https://doi.org/10.1007/s00249-019-01391-2 doi: 10.1007/s00249-019-01391-2
![]() |
[2] |
E. Carlsen, A. Giwercman, N. Keiding, N. E. Skakkebaek, Evidence for decreasing quality of semen during past 50 years, BMJ: B. Med. J., 305 (1992), 609. https://doi.org/10.1136/BMJ.305.6854.609 doi: 10.1136/BMJ.305.6854.609
![]() |
[3] |
T. G. Cooper, E. Noonan, S. von Eckardstein, J. Auger, H. W. G. Baker, H. M. Behre, et al., World Health Organization reference values for human semen characteristics, Hum Reprod Update, 16 (2010), 231–245. https://doi.org/10.1093/HUMUPD/DMP048 doi: 10.1093/HUMUPD/DMP048
![]() |
[4] |
S. H. Swan, E. P. Elkin, L. Fenster, The question of declining sperm density revisited: An analysis of 101 studies published 1934–1996, Environ. Health Perspect., 108 (2000), 961. https://doi.org/10.1289/EHP.00108961 doi: 10.1289/EHP.00108961
![]() |
[5] |
C. Huang, B. Li, K. Xu, D. Liu, J. Hu, Y. Yang, et al., Decline in semen quality among 30,636 young Chinese men from 2001 to 2015, Fertil. Steril., 107 (2017), 83–88.e2. https://doi.org/10.1016/j.fertnstert.2016.09.035 doi: 10.1016/j.fertnstert.2016.09.035
![]() |
[6] |
L. G. Jiang, L. Y. Cheng, S. H. Kong, Y. Yang, Y. J. Shen, C. Chen, et al., Toxic effects of polychlorinated biphenyls (Aroclor 1254) on human sperm motility, Asian J. Androl., 19 (2017), 561. https://doi.org/10.4103/1008-682X.186876 doi: 10.4103/1008-682X.186876
![]() |
[7] |
B. M. Ayad, G. van der Horst, S. S. du Plessis, Revisiting the relationship between the Ejaculatory abstinence period and semen characteristics, Int. J. Fertil. Steril., 11 (2018), 238. https://doi.org/10.22074/IJFS.2018.5192 doi: 10.22074/IJFS.2018.5192
![]() |
[8] | Priyansi, B. Bhattacharjee, J. Rahim, Predicting Semen Motility using three-dimensional Convolutional Neural Networks, (2021). https://doi.org/10.48550/arXiv.2101.02888 |
[9] |
C. Dai, Z. Zhang, G. Shan, L. T. Chu, Z. Huang, S. Moskovstev, et al., Advances in sperm analysis: Techniques, discoveries and applications, Nat. Rev. Urol., 18 (2021), 447–467. https://doi.org/10.1038/s41585-021-00472-2 doi: 10.1038/s41585-021-00472-2
![]() |
[10] | V. Thambawita, T. B. Haugen, M. H. Stensen, O. Witczak, H. L. Hammer, P. Halvorsen, et al., P-029 Identification of spermatozoa by unsupervised learning from video data. |
[11] |
M. Sewak, S. K. Sahay, H. Rathore, An overview of deep learning architecture of deep neural networks and autoencoders, J. Comput. Theor. Nanosci., 17 (2020), 182–188. https://doi.org/10.1166/jctn.2020.8648 doi: 10.1166/jctn.2020.8648
![]() |
[12] |
K. K. Tseng, Y. Li, C. Y. Hsu, H. N. Huang, M. Zhao, M. Ding, Computer-assisted system with multiple feature fused support vector machine for sperm morphology diagnosis, Biomed. Res. Int., 2013 (2013). https://doi.org/10.1155/2013/687607 doi: 10.1155/2013/687607
![]() |
[13] |
M. J. Tomlinson, Uncertainty of measurement and clinical value of semen analysis: Has standardisation through professional guidelines helped or hindered progress? Andrology, 4 (2016), 763–770. https://doi.org/10.1111/ANDR.12209 doi: 10.1111/ANDR.12209
![]() |
[14] |
R. P. Amann, D. Waberski, Computer-assisted sperm analysis (CASA): Capabilities and potential developments, Theriogenology, 81 (2014), 5–17.e3. https://doi.org/10.1016/j.theriogenology.2013.09.004 doi: 10.1016/j.theriogenology.2013.09.004
![]() |
[15] |
S. A. Hicks, J. M. Andersen, O. Witczak, V. Thambawita, P. Halvorsen, H. L. Hammer, et al., Machine learning-based analysis of sperm videos and participant data for male fertility prediction, Sci. Rep., 9 (2019). https://doi.org/10.1038/s41598-019-53217-y doi: 10.1038/s41598-019-53217-y
![]() |
[16] |
L. F. Urbano, P. Masson, M. Vermilyea, M. Kam, Automatic tracking and motility analysis of human sperm in Time-Lapse images, IEEE Trans. Med. Imaging, 36 (2017), 792–801. https://doi.org/10.1109/TMI.2016.2630720 doi: 10.1109/TMI.2016.2630720
![]() |
[17] |
K. Dewan, T. R. Dastidar, M. Ahmad, Estimation of sperm concentration and total motility from microscopic videos of human semen samples, IEEE Computer Society Conference on Computer Vision and Pattern Recognition Workshops, (2018) 2380–2387. https://doi.org/10.1109/CVPRW.2018.00307 doi: 10.1109/CVPRW.2018.00307
![]() |
[18] | L. Wu, P. Cui, J. Pei, L. Zhao, L. Song, Graph Neural Networks. |
[19] | K. Xu, W. Hu, J. Leskovec, S. Jegelka, How powerful are graph neural networks? |
[20] | B. Jiang, S. Chen, B. Luo, MGLNN: Semi-supervised learning via multiple graph cooperative learning neural networks, Neural Networks, 143 (2022), 204–214. |
[21] |
H. Elbardisi, A. Majzoub, S. al Said, K. al Rumaihi, W. el Ansari, A. Alattar, et al., Geographical differences in semen characteristics of 13 892 infertile men, Arab. J. Urol., 16 (2018), 3–9. https://doi.org/10.1016/j.aju.2017.11.018 doi: 10.1016/j.aju.2017.11.018
![]() |
[22] | R. Jeamanukoolkit, C. Treetampinich, M. Sukprasert, S. Rattanasiri, W. Choktanasiri, C. Satirapod, Comparison of the Motility, Morphology, and DNA Integrity of Cryopreserved Human Spermatozoa from Processing Semen before and after Cryopreservation, J. Medical Association Thailand, 100 (2017), 1255. |
[23] |
M. T. Le, T. T. T. Nguyen, T. T. Nguyen, V. T. Nguyen, T. T. A. Nguyen, V. Q. H. Nguyen, et al., Cryopreservation of human spermatozoa by vitrification versus conventional rapid freezing: Effects on motility, viability, morphology and cellular defects, Eur. J. Obstet. Gyn. R. B.., 234 (2019), 14–20. https://doi.org/10.1016/j.ejogrb.2019.01.001 doi: 10.1016/j.ejogrb.2019.01.001
![]() |
[24] |
G. Anifandis, K. Katsanaki, G. Lagodonti, C. Messini, M. Simopoulou, K. Dafopoulos, et al., The effect of glyphosate on human sperm motility and sperm DNA fragmentation, Int. J. Environ. Res. Public Health, 15 (2018). https://doi.org/10.3390/ijerph15061117 doi: 10.3390/ijerph15061117
![]() |
[25] |
L. Boeri, P. Capogrosso, E. Ventimiglia, F. Pederzoli, W. Cazzaniga, F. Chierigo, et al., High-risk human papillomavirus in semen is associated with poor sperm progressive motility and a high sperm DNA fragmentation index in infertile men, Hum. Reprod., 34 (2019), 209–217. https://doi.org/10.1093/humrep/dey348 doi: 10.1093/humrep/dey348
![]() |
[26] |
A. Harlev, R. Henkel, L. Samanta, A. Agarwal, Ritalinic acid stimulates human sperm motility and maintains vitality in vitro, World J. Mens. Health, 38 (2020), 61–67. https://doi.org/10.5534/WJMH.180127 doi: 10.5534/WJMH.180127
![]() |
[27] |
F. Faja, T. Carlini, G. Coltrinari, F. Finocchi, M. Nespoli, F. Pallotti, et al., Human sperm motility: A molecular study of mitochondrial DNA, mitochondrial transcription factor A gene and DNA fragmentation, Mol. Biol. Rep., 46 (2019), 4113–4121. https://doi.org/10.1007/s11033-019-04861-0 doi: 10.1007/s11033-019-04861-0
![]() |
[28] |
R. P. Amann, D. F. Katz, Andrology Lab Corner*: Reflections on CASA After 25 Years, J. Androl., 25 (2004), 317–325. https://doi.org/10.1002/J.1939-4640.2004.TB02793.X doi: 10.1002/J.1939-4640.2004.TB02793.X
![]() |
[29] |
S. T. Mortimer, G. van der Horst, D. Mortimer, The future of computer-aided sperm analysis, Asian J. Androl., 17 (2015), 545–553. https://doi.org/10.4103/1008-682X.154312 doi: 10.4103/1008-682X.154312
![]() |
[30] |
H. O. Ilhan, G. Serbes, N. Aydin, Automated sperm morphology analysis approach using a directional masking technique, Comput. Biol. Med., 122 (2020), 103845. https://doi.org/10.1016/j.compbiomed.2020.103845 doi: 10.1016/j.compbiomed.2020.103845
![]() |
[31] |
S. Javadi, S. A. Mirroshandel, A novel deep learning method for automatic assessment of human sperm images, Comput. Biol. Med., 109 (2019), 182–194. https://doi.org/10.1016/j.compbiomed.2019.04.030 doi: 10.1016/j.compbiomed.2019.04.030
![]() |
[32] |
J. Riordon, C. McCallum, D. Sinton, Deep learning for the classification of human sperm, Comput. Biol. Med., 111 (2019). https://doi.org/10.1016/j.compbiomed.2019.103342 doi: 10.1016/j.compbiomed.2019.103342
![]() |
[33] |
S. A. Hicks, J. M. Andersen, O. Witczak, V. Thambawita, P. Halvorsen, H. L. Hammer, et al., Machine learning-based analysis of sperm videos and participant data for male fertility prediction. Sci. Rep., 9 (2019), 1–10. https://doi.org/10.1038/s41598-019-53217-y doi: 10.1038/s41598-019-53217-y
![]() |
[34] | M. reza Mohammadi, M. Rahimzadeh, A. Attar, Sperm detection and tracking in Phase-Contrast microscopy image sequences using deep learning and modified CSR-DCF. (2020). |
[35] |
F. Ghasemian, S. A. Mirroshandel, S. Monji-Azad, M. Azarnia, Z. Zahiri, An efficient method for automatic morphological abnormality detection from human sperm images, Comput. Meth. Prog. Bio., 122 (2015), 409–420. https://doi.org/10.1016/J.CMPB.2015.08.013 doi: 10.1016/J.CMPB.2015.08.013
![]() |
[36] |
A. Bijar, A. P. Benavent, M. Mikaeili, R. khayati, Fully automatic identification and discrimination of sperm's parts in microscopic images of stained human semen smear, J. Biomed. Sci. Eng., 05 (2012), 384–395. https://doi.org/10.4236/jbise.2012.57049 doi: 10.4236/jbise.2012.57049
![]() |
[37] |
C. Wang, R. S. Swerdloff, Limitations of semen analysis as a test of male fertility and anticipated needs from newer tests, Fertil. Steril., 102 (2014), 1502–1507. https://doi.org/10.1016/j.fertnstert.2014.10.021 doi: 10.1016/j.fertnstert.2014.10.021
![]() |
[38] | W. C. Tan, N. A. M. Isa, Segmentation and detection of human spermatozoa using modified Pulse Coupled Neural Network optimized by Particle Swarm Optimization with Mutual Information, In: 2015 IEEE 10th Conference on Industrial Electronics and Applications (ICIEA), (2015), 192–197. https://doi.org/10.1109/ICIEA.2015.7334109 |
[39] |
P. S. Duggal, S. Paul, P. Tiwari, Analytics for the quality of fertility data using particle swarm optimization, Int. J. Bio-Sci. Bio-Technol., 7 (2015), 39–50. https://doi.org/10.14257/IJBSBT.2015.7.1.05 doi: 10.14257/IJBSBT.2015.7.1.05
![]() |
[40] | L. E. M. van Raemdonck, Ata-ur-rehman, M. L. Davila-garcia, L. Mihaylova, R. F. Harrison, A. Pacey, An algorithm for Morphological classification of motile human sperm, 2015 Sensor Data Fusion: Trends, Solutions, Applications (SDF) (IEEE, 2015), 1–6. https://doi.org/10.1109/SDF.2015.7347714 |
[41] |
W. C. Tan, N. A. Mat Isa, Automated sperm head detection using intersecting cortical model optimised by particle swarm optimization, PLoS One, 11 (2016), e0162985. https://doi.org/10.1371/journal.pone.0162985 doi: 10.1371/journal.pone.0162985
![]() |
[42] |
F. Shaker, S. A. Monadjemi, A. R. Naghsh-Nilchi, Automatic detection and segmentation of sperm head, acrosome and nucleus in microscopic images of human semen smears, Comput. Meth. Prog. Bio., 132 (2016), 11–20. https://doi.org/10.1016/j.cmpb.2016.04.026 doi: 10.1016/j.cmpb.2016.04.026
![]() |
[43] |
S. K. Mirsky, I. Barnea, M. Levi, H. Greenspan, N. T. Shaked, Automated analysis of individual sperm cells using stain-free interferometric phase microscopy and machine learning, Cytom. A, 91 (2017), 893–900. https://doi.org/10.1002/CYTO.A.23189 doi: 10.1002/CYTO.A.23189
![]() |
[44] | M. L. D. Garcia, D. A. P. Soto, L. S. Mihaylova, A Bag of Features Based Approach for Classification of Motile Sperm Cells, 2017 IEEE International Conference on Internet of Things (iThings) and IEEE Green Computing and Communications (GreenCom) and IEEE Cyber, Physical and Social Computing (CPSCom) and IEEE Smart Data (SmartData) (IEEE, 2017), 104–109. https://doi.org/10.1109/iThings-GreenCom-CPSCom-SmartData.2017.21 |
[45] |
V. Chang, A. Garcia, N. Hitschfeld, S. Härtel, Gold-standard for computer-assisted morphological sperm analysis, Comput. Biol. Med., 83 (2017), 143–150. https://doi.org/10.1016/J.COMPBIOMED.2017.03.004 doi: 10.1016/J.COMPBIOMED.2017.03.004
![]() |
[46] |
F. Shaker, S. A. Monadjemi, J. Alirezaie, A. R. Naghsh-Nilchi, A dictionary learning approach for human sperm heads classification, Comput. Biol. Med., 91 (2017), 181–190. https://doi.org/10.1016/j.compbiomed.2017.10.009 doi: 10.1016/j.compbiomed.2017.10.009
![]() |
[47] | F. Shaker, S. A. Monadjemi, J. Alirezaie, Classification of human sperm heads using elliptic features and LDA, 3rd International Conference on Pattern Analysis and Image Analysis, IPRIA 2017 (Institute of Electrical and Electronics Engineers Inc., 2017), 151–155. https://doi.org/10.1109/PRIA.2017.7983036 |
[48] |
M. S. Nissen, O. Krause, K. Almstrup, S. Kjærulff, T. T. Nielsen, M. Nielsen, Convolutional neural networks for segmentation and object detection of human semen, Lect. Notes Comput. Sc., 10269 LNCS (2017), 397–406. https://doi.org/10.48550/arXiv.1704.00498 doi: 10.48550/arXiv.1704.00498
![]() |
[49] |
E. El-Shafeiy, A. El-Desouky, S. El-Ghamrawy, An optimized artificial neural network approach based on sperm whale optimization algorithm for predicting fertility quality, Stud. Inform. Control, 27 (2018), 349–358. https://doi.org/10.24846/V27I3Y201810 doi: 10.24846/V27I3Y201810
![]() |
[50] | H. O. Ilhan, G. Serbes, N. Aydin, The Effects of the Modified Overlapping Group Shrinkage Technique on the Sperm Segmentation in the Stained Images, 2018 41st International Conference on Telecommunications and Signal Processing, TSP 2018, 1–4. https://doi.org/10.1109/TSP.2018.8441271 |
[51] |
P. Hernandez-Herrera, F. Montoya, J. M. Rendon-Mancha, A. Darszon, G. Corkidi, 3-D + t human sperm flagellum tracing in low SNR fluorescence images, IEEE T. Med. Imaging, 37 (2018), 2236–2247. https://doi.org/10.1109/TMI.2018.2840047 doi: 10.1109/TMI.2018.2840047
![]() |
[52] |
R. A. Movahed, M. Orooji, A Learning-Based Framework for the Automatic Segmentation of Human Sperm Head, Acrosome and Nucleus, 2018 25th Iranian Conference on Biomedical Engineering and 2018 3rd International Iranian Conference on Biomedical Engineering, ICBME 2018, (2018). https://doi.org/10.1109/ICBME.2018.8703544 doi: 10.1109/ICBME.2018.8703544
![]() |
[53] |
C. McCallum, J. Riordon, Y. Wang, T. Kong, J. B. You, S. Sanner, et al., Deep learning-based selection of human sperm with high DNA integrity, Commun. Biol., 2 (2019). https://doi.org/10.1038/s42003-019-0491-6 doi: 10.1038/s42003-019-0491-6
![]() |
[54] |
R. A. Movahed, E. Mohammadi, M. Orooji, Automatic segmentation of Sperm's parts in microscopic images of human semen smears using concatenated learning approaches, Comput. Biol. Med., 109 (2019), 242–253. https://doi.org/10.1016/j.compbiomed.2019.04.032 doi: 10.1016/j.compbiomed.2019.04.032
![]() |
[55] | S. Qi, T. Nie, Q. Li, Z. He, D. Xu, Q. Chen, A Sperm Cell Tracking Recognition and Classification Method, 2019 International Conference on Systems, Signals and Image Processing (IWSSIP), 2019-June (2019), 163–167. https://doi.org/10.1109/IWSSIP.2019.8787312 |
[56] | M. reza Mohammadi, M. Rahimzadeh, A. Attar, Sperm Detection and Tracking in Phase-Contrast Microscopy Image Sequences using Deep Learning and Modified CSR-DCF. (2020). https://doi.org/10.48550/arXiv.2002.04034 |
[57] |
A. Zeadna, N. Khateeb, L. Rokach, Y. Lior, I. Har-Vardi, A. Harlev, et al., Prediction of sperm extraction in non-obstructive azoospermia patients: a machine-learning perspective, Hum. Reprod., 35 (2020), 1505–1514. https://doi.org/10.1093/HUMREP/DEAA109 doi: 10.1093/HUMREP/DEAA109
![]() |
[58] |
A. Lesani, S. Kazemnejad, M. Moghimi Zand, M. Azadi, H. Jafari, M. R. K. Mofrad, et al., Quantification of human sperm concentration using machine learning-based spectrophotometry, Comput. Biol. Med., 127 (2020), 104061. https://doi.org/10.1016/j.compbiomed.2020.104061 doi: 10.1016/j.compbiomed.2020.104061
![]() |
[59] |
I. Iqbal, G. Mustafa, J. Ma, Deep learning-based morphological classification of human sperm heads, Diagnostics (Basel), 10 (2020). https://doi.org/10.3390/diagnostics10050325 doi: 10.3390/diagnostics10050325
![]() |
[60] |
D. Somasundaram, M. Nirmala, Faster region convolutional neural network and semen tracking algorithm for sperm analysis, Comput. Meth. Prog. Bio., 200 (2021), 105918. https://doi.org/10.1016/J.CMPB.2020.105918 doi: 10.1016/J.CMPB.2020.105918
![]() |
[61] |
R. Marín, V. Chang, Impact of transfer learning for human sperm segmentation using deep learning, Comput. Biol. Med., 136 (2021), 104687. https://doi.org/10.1016/J.COMPBIOMED.2021.104687 doi: 10.1016/J.COMPBIOMED.2021.104687
![]() |
[62] | S. Ottl, S. Amiriparian, M. Gerczuk, B. Schuller, A machine learning framework for automatic prediction of human semen motility, (2021). https://doi.org/10.48550/arXiv.2109.08049 |
[63] |
D. Wu, O. Badamjav, V. Reddy, M. Eisenberg, B. Behr, A preliminary study of sperm identification in microdissection testicular sperm extraction samples with deep convolutional neural networks, Asian J. Androl., 23 (2021), 135–139. https://doi.org/10.4103/AJA.AJA_66_20 doi: 10.4103/AJA.AJA_66_20
![]() |
[64] |
L. Prabaharan, A. Raghunathan, An improved convolutional neural network for abnormality detection and segmentation from human sperm images, J. Amb. Intell. Hum. Comp., 12 (2021), 3341–3352. https://doi.org/10.1007/S12652-020-02773-7 doi: 10.1007/S12652-020-02773-7
![]() |
[65] |
A. Chen, C. Li, S. Zou, M. M. Rahaman, Y. Yao, H. Chen, et al., SVIA dataset: A new dataset of microscopic videos and images for computer-aided sperm analysis, Biocybern. Biomed. Eng., 42 (2022), 204–214. https://doi.org/10.1016/J.BBE.2021.12.010 doi: 10.1016/J.BBE.2021.12.010
![]() |
[66] |
Y. A. Hu, J. C. Lu, Y. Shao, Y. F. Huang, N. Q. Lü, Comparison of the semen analysis results obtained from two branded computer-aided sperm analysis systems, Andrologia, 45 (2013), 315–318. https://doi.org/10.1111/and.12010 doi: 10.1111/and.12010
![]() |
[67] |
V. Chang, L. Heutte, C. Petitjean, S. Härtel, N. Hitschfeld, Automatic classification of human sperm head morphology, Comput. Biol. Med., 84 (2017), 205–216. https://doi.org/10.1016/j.compbiomed.2017.03.029 doi: 10.1016/j.compbiomed.2017.03.029
![]() |
[68] |
H. O. Ilhan, I. O. Sigirci, G. Serbes, N. Aydin, A fully automated hybrid human sperm detection and classification system based on mobile-net and the performance comparison with conventional methods, Med. Biol. Eng. Comput., 58 (2020), 1047–1068. https://doi.org/10.1007/s11517-019-02101-y doi: 10.1007/s11517-019-02101-y
![]() |
[69] |
V. Valiuškaitė, V. Raudonis, R. Maskeliūnas, R. Damaševičius, T. Krilavičius, Deep learning based evaluation of spermatozoid motility for artificial insemination, Sensors, 21 (2020), 72. https://doi.org/10.3390/s21010072 doi: 10.3390/s21010072
![]() |
[70] |
A. Abbasi, E. Miahi, S. A. Mirroshandel, Effect of deep transfer and multi-task learning on sperm abnormality detection, Comput. Biol. Med., 128 (2021). https://doi.org/10.1016/j.compbiomed.2020.104121 doi: 10.1016/j.compbiomed.2020.104121
![]() |
[71] |
S. Ottl, S. Amiriparian, M. Gerczuk, B. W. Schuller, motilitAI: A machine learning framework for automatic prediction of human sperm motility, iScience, 25 (2022), 104644. https://doi.org/10.1016/j.isci.2022.104644 doi: 10.1016/j.isci.2022.104644
![]() |
[72] |
A. Aristoteles, A. Syarif, S. F. R. Lumbanraja, A. Hidayatullah, Identification of human sperm based on morphology using the you only look once version 4 algorithm, Int. J. Adv. Comput. Sci. Appl., 13 (2022). https://doi.org/10.14569/IJACSA.2022.0130752 doi: 10.14569/IJACSA.2022.0130752
![]() |
[73] | V. Thambawita, S. A. Hicks, A. M. Storås, T. Nguyen, J. M. Andersen, O. Witczak, et al., VISEM-Tracking: Human Spermatozoa Tracking Dataset. (2022). |
[74] | V. Thambawita, S. A. Hicks, A. M. Storås, T. Nguyen, J. M. Andersen, et al., VISEM-Tracking: Human Spermatozoa Tracking Dataset. (2022). |
[75] |
M. Dobrovolny, J. Benes, J. Langer, O. Krejcar, A. Selamat, Study on Sperm-Cell detection using YOLOv5 architecture with labaled dataset, Genes (Basel), 14 (2023). https://doi.org/10.3390/genes14020451 doi: 10.3390/genes14020451
![]() |
[76] |
S. Chandra, M. K. Gourisaria, H. Gm, D. Konar, X. Gao, T. Wang, et al., Prolificacy assessment of spermatozoan via State-of-the-Art deep learning frameworks, IEEE Access, 10 (2022), 13715–13727. https://doi.org/10.1109/ACCESS.2022.3146334 doi: 10.1109/ACCESS.2022.3146334
![]() |
[77] |
E. Miahi, S. A. Mirroshandel, A. Nasr, Genetic neural architecture search for automatic assessment of human sperm images, Expert Syst. Appl., 188 (2022). https://doi.org/10.1016/J.ESWA.2021.115937 doi: 10.1016/J.ESWA.2021.115937
![]() |
[78] |
S. Shahzad, M. Ilyas, M. I. U. Lali, H. T. Rauf, S. Kadry, E. A. Nasr, Sperm abnormality detection using sequential deep neural network, Mathematics, 11 (2023). https://doi.org/10.3390/math11030515 doi: 10.3390/math11030515
![]() |
[79] | A. Abdelaziz Mashaal, M. A. A Eldosoky, L. Nabil Mahdy, K. Ali Ezzat, Automatic Healthy Sperm Head Detection using Deep Learning. |
[80] |
H. O. Ilhan, I. O. Sigirci, G. Serbes, N. Aydin, A fully automated hybrid human sperm detection and classification system based on mobile-net and the performance comparison with conventional methods, Med. Biol. Eng. Comput., 58 (2020), 1047–1068. https://doi.org/10.1007/s11517-019-02101-y doi: 10.1007/s11517-019-02101-y
![]() |
[81] | F. Shaker, S. A. Monadjemi, J. Alirezaie, Classification of human sperm heads using elliptic features and LDA, 2017 3rd International Conference on Pattern Recognition and Image Analysis (IPRIA) (IEEE, 2017), 151–155. https://doi.org/10.1109/PRIA.2017.7983036 |
[82] |
W. C. Tan, N. A. M. Isa, Segmentation and detection of human spermatozoa using modified Pulse Coupled Neural Network optimized by Particle Swarm Optimization with Mutual Information, 2015 IEEE 10th Conference on Industrial Electronics and Applications (ICIEA) (2015), 192–197. https://doi.org/10.1109/ICIEA.2015.7334109 doi: 10.1109/ICIEA.2015.7334109
![]() |
1. | Alireza Sadeghi Hesar, Mahboobeh Houshmand, A memetic quantum-inspired genetic algorithm based on tabu search, 2024, 17, 1864-5909, 1837, 10.1007/s12065-023-00866-8 | |
2. | Chuanjiang Li, Wenjing Xia, Haozhi Han, Ang Li, Ziming Qi, Chongming Zhang, Zhuming Wang, Yanfei Zhu, Erlei Zhi, Zheng Li, A novel approach for one-stage sperm detection using advanced multi-scale feature pyramid networks, 2024, 93, 17468094, 106152, 10.1016/j.bspc.2024.106152 | |
3. | Alexandra Keller, McKenna Maus, Emma Keller, Karl Kerns, Deep learning classification method for boar sperm morphology analysis, 2024, 2047-2919, 10.1111/andr.13758 | |
4. | Rania Maalej, Olfa Abdelkefi, Salima Daoud, Advancements in automated sperm morphology analysis: a deep learning approach with comprehensive classification and model evaluation, 2024, 1573-7721, 10.1007/s11042-024-20188-w | |
5. | Shahid Naseem, Tariq Mahmood, Tanzila Saba, Faten S. Alamri, Saeed Ali Omer Bahaj, Hammad Ateeq, Umer Farooq, DeepFert: An Intelligent Fertility Rate Prediction Approach for Men Based on Deep Learning Neural Networks, 2023, 11, 2169-3536, 75006, 10.1109/ACCESS.2023.3290554 | |
6. | Pål Andreas Hoven Bentsen, Steven Hicks, Eric Jul, Pål Halvorsen, Vajira Thambawita, 2024, SAM in the Pipeline: Transforming Axis-Aligned to Oriented Bounding Boxes for Superior Sperm Detection, 979-8-3503-7844-3, 1, 10.1109/CBMI62980.2024.10859223 |
Ref | Dataset name | Source Link attach | Type | No. of instance | Color space | Resolution | classes |
[35] | - | - | Images | 10 to 15 | RGB | 576 × 764 | - |
[12] | Original images | http://dx.doi.org/10.1155/2013/687607 | Images | 160 | Gray Scale | - | 2 |
[36] | Public dataset | UCI data repository [14] | Images | 100 | - | - | 2 |
[37] | HSMA-DS | Images | 1457 | RGB | 576 × 764 | ||
[38] | Video dataset | Hospital Universiti Sains Malaysia (HUSM) | Video | 80 | |||
[39] | - | Images | 100 | 7 | 2 | ||
[40] | Video dataset | Andrology lab of the Academic Unit of Reproductive and Developmental Medicine (University of Sheffield) | video | 2040 × 1086 | |||
[41] | Video dataset | Hospital Universiti Sains Malaysia (HUSM) | Video | 20 | RGB | 480 × 640 | |
[42] | Gold-stadard dataset | Images | 19 | RGB | 780 × 580 | ||
[43] | - | Images | 886 | 2 | |||
[44] | Video dataset | Academic Unit of Reproductive and Develop mental Medicine (AURDM) | Video | 940 | 2040 × 1086 | 4 | |
[45] | SCIAN-MorphoSpermGS | Laboratory of Spermiogram, (ICBM), Faculty of Medicine, University of Chile. | Images | 7 × 7 | 5 | ||
[46] | HuSHeM | https://data.mendeley.com/datasets/tt3yj2pf38/1. | Images | 216 sperm head | RGB | 131×131 | 4 |
[47] | Isfahan Fertility and Infertility Center (IFIC) | Images | 170 sperm head | RGB | 720×576 | 4 | |
[48] | Images | 765 | Gray Scale | 1920×1440 | 2 | ||
[49] | Fertility Dataset |
UCI repository | Images | 100 | |||
[50] | Ocular images | Images | 13 | ||||
[51] | 3D+t | Images | 512 × 512 | ||||
[52] | Gold-standard dataset | Images | 20 | 780 × 580 | |||
[17] | Video dataset | Video | 90 frames at 30fps (3600 images) |
3200 × 2400 | 2 | ||
[33] | VISEM | [https://datasets.simula.no/viem/] | Video | 85 | 3 | ||
[53] | In-house dataset | https://figshare.com/articles/Deep_learningbased_selection_of_human_sperm_with_high_DNA_integrity/8124932 | Images | 1064 | |||
[32] | HuSHeM and SCIAN. | Images | 216 and 1132 |
Gray Scale and RGB | 5 | ||
[54] | Gold-standard dataset | Images | 20 | 780 × 580 | |||
[55] | Video dataset | Shenzhen Sixth People's Hospital, | Video | 54 | 720×540 | ||
[31] | MHSMA | [35] | Images | 1540 | RGB | 1280×1024 | 2 |
[56] | Video dataset | Royan institute Research Lab | Video | 36 | 768 × 576 | ||
[57] | - | SUMC | Images | 119 | 18 | ||
[58] | - | Avicenna Research Institute (Tehran, Iran) | 41 | 7 | |||
[59] | HuSHeM and SCIAN. | Images | 1154 and 1132 | Gray Scale | 5 | ||
[60] | Sperm Morphology Image Data Set (SMIDS) | Infertility centre of Istanbul University | Images | 200 | |||
[61] | Video dataset | Video | 288 × 352 | ||||
[62] | VISEM | https://datasets.simula.no/visem/ | Video | 85 | 640 × 480 | ||
[63] | SCIAN-SpermSegGS | Faculty of Medicine, University of Chile, Santiago, Chile. | Images | 19 | |||
[8] | VISEM | Simula Research Laboratory | Video | 85 | 640 × 480 | ||
[64] | VISEM | Simula Research Laboratory | Video | 85 | 640 × 480 | ||
[65] | MHSMA | Images | 1540 | Gray Scale | |||
[66] | Testicular biopsies | Images | 702 | ||||
[67] | Sperm image dataset | http://morfologia.cedai.cl/publi c/) | Images | 20 | Gray Scale | ||
[68] | SVIA dataset | https://github.com/Demozsj/Detection-Sperm. | Images and video | 101 and 130042 | 698528 | ||
[69] | MHSMA | Images | 1540 | Gray Scale | |||
[70] | MHSMA | Images | 1540 | Gray Scale | |||
[71] | VISEM | Simula Research Laboratory | Video | 85 | 640 × 480 |
Subset-A | Video | Number 101 |
No. of annotated object 125,000 | Format .mp4 |
Image | Number 3590 |
Size 698 × 528 × 3 |
Format .png |
|
Subset-B | Video | Number 10 |
No. of annotated object 26,000 | Format .mp4 |
Image | Number 451 |
Size 698 × 528 × 3 |
Format .png |
|
Subset-C | Image | Class Sperm impurity |
Number 121,401 4,479 |
Format .png |
Dataset | Video | Image | Size | Number of annotated objects |
HuSHeM | 0 | 216 | 131 × 131 | 0 |
Visem | 85 | 0 | 640 × 480 | 0 |
MHSMA | 0 | 1540 | 128 × 128 or 64 × 64 | 0 |
SIVA | 101 | 130042 | 698 × 528 | 127600 |
Ref | Dataset name | Pre-processing techniques | Outcomes |
[36] | - | Red component of RGB | Author first scaled image as a pre-processing technique using Red component of RGB. M is the range between zero to maximum intensity, and it was 12-byte data I initially, then scaled to a new intensity Is between 0 to 255 (8-bit). It was formulated as I s = I/M × 255. |
[37] | Public dataset | SMOTE | Removing majority Tomek Links and balance data with the help of SMOTE technique. |
[35] | HSMA-DS | Gaussian filter | By applying Gaussian Filter on Y channel Noise Reduction by wavelet transform, the percentage of noises was decreases. However, this percentage was not acceptable for further processing |
[39] | - | optional attribute selection process | The cumulative data was pre-processed using an optional attribute selection process. This intermediate step was used to filter only the relevant and important attributes from the bulk data. Attribute selection can affect the overall results derived from the data. |
[41] | Video dataset | Laplacian of Gaussian (LoG) filter |
First, they convert images into gray scale from RGB. Because colour image required more processing time as compare to gray scale images. In next step Laplacian of Gaussian (LoG) filter was deployed in kernel as a pre-processing step so that it automatically detect and segment human spermatozoa. |
[67] | SCIAN-MorphoSpermGS | Anisotropic diffusion | Anisotropic diffusion method was used for noise reduction. Moreover, this method also preserves the border of images. |
[50] | Ocular images | Modified Overlapping Groups Shrinkage (MOGS) | In MOGS, convex function is used to detect the correct region of sperm, therefore denoising problem was accepted. In this regard non-convex regularization was used and desired group sparsity property were verified |
[51] | 3D+t | logarithm transform | To shrink the range of intensity values, log transformation (V log (~ x) = log (V (~ x) + 1)) was applied on image stack V. so it increases the contrast of low intensity values. |
[52] | Gold-standard dataset | Homomorphic Filtering Filling holes |
This method helps to highlight each sperm in the background by correct uneven illumination. Moreover, some post processing steps were also involved to improve segmentation procedure. |
[54] | Gold-standard dataset | Serialized | This method helps to highlight sperm cells in the image rather than other particles. It also restrains unnecessary characteristics of the image like low contrast between the sperms tails and its neighbouring region and non-uniform distribution of light |
[59] | HuSHeM and SCIAN. | Rotation Translation flipping |
Author used three common techniques as previously used in the training set of the SCIAN dataset. For rotation, they rotate the training image by 25 to 25 degrees. For translation, they shift ~6% of the original image to the left, the right, up, and down. For flipping, they vertically flip the image. Due to the same distribution of classes within this dataset, they apply equal augmentation options to each class |
[68] | Sperm Morphology Image Data Set (SMIDS) | Modified Overlapping Groups Shrinkage (MOGS) |
Ilhan et al again used same pre-processing technique as he had used in 2018 called MOGS. This technique helps to remove random noise without changing sperm shapes. |
[60] | Video dataset | median filter, elliptic curve | Median filter was also used for pre-processing, this technique was applied to each image. The edges of images were preserved when they pass through median filter. |
[8] | VISEM | Convolutional neural network (CNN) | CNN was a specialized type of network used that has a grid-like topology for processing data. They are most commonly used on image and video data |
[62] | VISEM | Spatial warping, Background removal, and Filtering |
Geometric distortion, non-uniform contrast, and noise are alleviated by spatial warping, background removal and filtering, respectively |
[63] | Testicular biopsies | Glare filters and diffraction correction, MobileNetV2 |
A single embryologist annotated each image with bounding boxes around each identified spermatozoon. At the time of collection, each image was normalized, passed through glare filters and diffraction correction (Hamilton Thorne, HT video and image capture software version 3, Hamilton Thorne, Inc., Beverly, MA, USA), and had microscopy artifacts removed. |
[64] | Sperm image dataset | Median filtering | It helps to reduce noise in the image and perform edge detection and improve image resolution. It also sorts pixel value by comparing mean filter. It does not create any unreal surrounding pixels, so it gave efficient result after pre-processing. |
Ref | Dataset name | Method of Feature Extraction | Outcomes |
[12] | Original Images | Fourier Transform Infrared Spectroscopy (FTIRS), grey level feature | FTIRS and grey level feature was used to differentiate multiple sperms according to their characteristics. |
[35] | HSMA-DS | Sobel algorithm | Sobel algorithm was used for edge detection |
[40] | video frames | Otsu's threshold | Author use Otsu's threshold as a feature extraction technique. It converts image to gray scale then eliminate background from the foreground. |
[42] | Gold Standard | Gaussian filter, Ostu method, Gradient vector flow, curvature and edge energy | Author use ostu method for sperm head detection. Moreover, they use histogram of the Hue channel of HSV color space along with size criterion for successful sperm head detection in microscopic image |
[43] | - | Phase Map Acquisition | Phase Map Acquisition (PMA) technique was used to extract 89 custom-design features from dataset. These features included the acrosome area, mean OPD and the correlation of the OPD map of the head etc |
[44] | - | (Speeded-Up Robust Features) SURF | It detects key point from given dataset. Hessian matrix was used to select most demonstrative pixels founded in low-level features. |
[67] | SCIAN-MorphoSpermGS | five shape-based descriptors | Author proposes combine five shape-based descriptors It easily recognize human sperm head and also help in sperm morphology analysis. |
[50] | Ocular images | Pixel-Based Spatial analyses, Blob analyses | PBS analyses, Blob analyses was used for feature extraction (spatial-based features), over segmented parts. |
[33] | VISEM | Lucene Image Retrieval (LIRE) Tamura image features |
LIRE is a java library that use to extract features from images and photos with the help of texture and color characteristics. Author test more than 30 features with machine learning algorithms but Tamura image features work best with proposed algorithm in this study. |
[55] | Gaussian mixture model | This technique used statistical information of pixel for background representation, such that target pixel was figure out using statistical difference and judged probability density in long time period with large sample value. As result a complex dynamic background could be modelled. |
Ref | Dataset name | Method of Segmentation | Outcomes |
[36] | - | Markov random field (MRF) Model and Entropy based (EM) algorithm |
In first section Bayesian classifier was used to segment sperm's Nucleus, Mid-piece and Acrosome. In second section EM and MRF model was used to upgrade and obtain class conditional probability density function (CCPDF) and apriori probability for each class. |
[12] | Original images | Average Rank Difference | By using Average Rank Difference between abnormal and normal sperms the segmentation of accuracy 55% achieved. |
[37] | Public dataset | Clustering-Based Decision Forests (CBDF Algorithm) | Author used CBDF Algorithm to deal with unbalanced class learning problem for sperm analysis. Result shows that CBDF Algorithm outclass state of the art techniques like logistic regression, multilayer perceptron neural networks, random forests, Support Vector Machines and decision tree etc with great difference. |
[40] | Video dataset | The structural similarity measure (SSIM) The correlation (CORR) coefficient, and The Bhattacharyya distance (BHAT) |
Author used 3 different techniques for segmentation. The original image was labelled with 1 or 0 and count of sperm, non-sperm, immotile and motile cells. Only motile cells were kept for experiment and all other non-sperm and immotile cells were removed. |
[42] | Gold standard | GVF, curvature and edge energy (TDCEE), Dice Coefficient | For more refine segmentation, researcher used edge-based active contour method. Moreover, they also used a novel tail point detection technique that trace and eliminate midpiece from segmented head, for more efficient segmentation. Results shows 92% achievement against hand segmented ground truth that outperform famous techniques. |
[43] | - | Support Vector Machines (SVMs) |
The SVM classifier achieves receiver operating characteristic curve of 88.59%, precisions of 90% and precision-recall curve of 88.67%. Researcher believed that this automatic process of sperm analysis will become base of automatic and objective sperm cell collection in IVF |
[44] | Support Vector Machines (SVMs) |
Support Vector Machines (SVMs) was used for segmentation. Simplified feature vectors that extracted from dataset were used to classify sperm cells into normal, abnormal and non-cell categories. | |
[67] | SCIAN-MorphoSpermGS | SVM-based cascade classification | This two-stage classification scheme shows better results than monolithic classifiers and it also work like a human expert. |
[50] | Ocular images | Fuzzy C- Means (FCM) and K-Mean |
FCM segmented the gray scaled imaged more successfully than K-Means because of fuzzy technique. But there is room of improvement because only 50% sperms were correctly classified. |
[55] | Threshold segmentation | This technique was applied on background image for the sake of counting immotile sperm. |
Ref | Dataset name | Method of Segmentation | Outcomes |
[38] | Video dataset | Pulse Coupled Neural Network (PCNN) Particle Swarm Optimization (PSO) |
PCNN is very famous method for sperm image segmentation. It helps to solve inaccurate sperm head shape problems as well. It also uses to differentiate agglutinated sperms with high accuracy and precision. Moreover, PCNN model include eight parameters so, optimized PSO algorithm was used improve PCNN parameters. |
[41] | Video dataset | Intersecting cortical model (ICM) | For efficient sperm head segmentation ICM was derived from several visual cortex models. There was some parameter selection problem so researcher optimize ICM network with PSO. New fitness function know as feature mutual information was introduced here. This model was more efficient and precise than other famous segmentation techniques. |
[47] | - | Active contours. | Active contours method was used for segmentation. This method automatically segmented cropped sperm heads. Color images were transformed to gray scale images and apply threshold using Otsu method for fast locating of sperm heads. Edges of the images were extracted by Canny edge detector method and Gradient Vector Flow (GVF) active contour method was employed to extract outer contour of sperm heads. |
[48] | Convolutional Neural Network (CNN) | CNN outperform classical image analysis approach by achieving 91.89% recall and 93.87% precision on a given dataset. | |
[52] | Gold-standard dataset | Convolutional Neural Networks (CNN) | For segmentation of sperm head a deep convolutional neural network was used. For improve head segmentation, geometric constraints and filling holes operation were also employed. Then SVM helps to classify all pixel of segmented heads to acrosome and nucleus regions. |
[54] | Gold-standard dataset | CNN model 1 CNN model 2 |
Two CNN networks were used with different technique for segmentation of head and axial filament of the sperms. Overlapping patches were extracted from RGB images at the end of background using CNN model 1. Moreover, at Histogram Stretching stage, patches was extracted from RGD images using CNN model 2 |
[31] | MHSMA | Convolutional Neural Networks (CNN) |
CNN network was used to deal with morphological deformities present in human sperm like head, vacuole, and acrosome. It was first technique that focus on acrosome. It also works very efficient in low resolution and non-stained images |
[68] | Sperm Morphology Image Data Set (SMIDS) | K-Means clustering Fuzzy C-Means clustering |
MOGS technique was used as a pre-processing step and it helps to reduce noise present in sample images and improve the overall segmentation process. Similarly, Fuzzy C-Means clustering shows impressive results when MOGS was applied on images as a pre-processing method. Moreover, Fuzzy C-Means clustering apply fuzziness for the belongingness of each image pixels, which increase efficiency of segmentation process. |
[60] | Video dataset | Local Region Contour segmentation | Author separates tail region with binary images with the help of local region contour segmentation. In past may algorithm were unsuccessful to detect tail region from video dataset because of minor gradient in the tail region. Some researcher able to do so while denoising images. |
[61] | SCIAN-SpermSegGS | U-Net and Mask- RCNN |
Results show that U-net with transfer learning, outclass state of the art sperm segmentation method with 95% overlapping efficiency against hand-segmented masks for sperm head 0.96, acrosome 0.94 and nucleus 0.95. |
[69] | VISEM | Region Based Convolutional Neural Networks (R-CNN) architecture |
The Faster R-CNN-Inception_V2-COCO model was used to develop the sperm counting algorithm. Its advantage is that it is quite accurate to learn to distinguish objects even from a small database. The model takes about 50 ms to analyze the image. Because the model uses regions, it is easier to detect a larger number of objects in a single frame. Additionally, the R-CNN model can read information from frames of any size, so there is no need to crop frames before submitting them to the neural network. Achieved 91.77% (95% CI, 91.11–92.43%) accuracy of sperm head detection on the VISEM sperm sample video dataset. |
[62] | VISEM | Mean squared displacement of each particle (imsd) |
Segmentation can be done for each individual particle (mean squared displacement of each particle (imsd)) or again as an average for all of the particles (ensemble mean squared displacement of all particles (emsd)). These results show a relative improvement of 4.4% against state-of-the-art results. |
[70] | MHSMA | Deep Transfer Learning (DTL) technique and Deep Multi-task Transfer Learning (DMTL) |
DTL technique accurately label head, vacuole, and acrosome with accuracy of 84%, 94%, and 79% respectively. Similarly, DMTL technique accurately label head, vacuole, and acrosome with 82%, 92.33%, and 80.66% respectively. |
[63] | Testicular biopsies | VGG16 | Classifies each proposal as either a spermatozoon or background and regresses the bounding box shape to match identified component objects. |
[64] | Sperm image dataset | Enhanced Otsu's threshold method |
It reduces the computation complexity and sobel operator was used for edge reduction, which improve overall performance of segmentation. The abnormal regions were detected by convolution layer. |
[65] | SVIA dataset |
k-means, U-net, SegNet and DeepLabv3 | The effects of U-net and Deeplab_v3 are the best, and other segmentation methods have a certain gap compared with these two segmentation methods |
[71] | VISEM | Crocker-Grier Algorithm With CNN |
The mean absolute error (MAE) could be reduced from 8.83 to 7.31. |
[72] | VISEM | YOLOv4 algorithm | The results obtained were 90.31% AP (Average Precision) for sperm objects and 68.19% AP (Average Precision) for non-sperm objects, then for the results of the training obtained by the model 79.58% mAP (Mean Average Precision). |
[73] | VISEM | YOLOv5 algorithm | YOLOv5 deep learning model trained on the VISEM-Tracking dataset present baseline sperm detection performances. This dataset can be used to train complex deep learning models to analyze spermatozoa. |
[74] | VISEM | YOLOv5 algorithm | The best-performing model is yolov5l. This network achieves a precision of 88.6 per cent, recall of 52.6, and mAP is 72.1. Other networks are achieving lower results. The second-best network is nano, with a mAP of 69.6 and precision of 64.7 per cent |
Ref | Dataset name | Method of Segmentation | Network | Evaluation metrics |
[48] | Convolutional Neural Network (CNN) | 3-conv-full-up-inc | Precision 93.87% Recall 91.89% |
|
[68] | Sperm Morphology Image Data Set (SMIDS) | K-Means clustering Fuzzy C-Means clustering |
Mobile-Net | Precision 83%, recall 82%, F-measure 83% and accuracy 88%. |
[60] | Video dataset | Local Region Contour segmentation | CNN network | Precision 97.37% |
[61] | SCIAN-SpermSegGS | U-Net and Mask- RCNN |
U-Net and MaskRCNN | 95% overlapping efficiency against hand-segmented masks for sperm head 0.96, acrosome 0.94 and nucleus 0.95. |
[69] | VISEM | Region Based Convolutional Neural Networks (R-CNN) architecture |
R-CNN | Accuracy 91.77 %. |
[62] | VISEM | Mean squared displacement of each particle (imsd) |
CNN RNN |
4.4% improvement against state-of-the-art results. |
[70] | MHSMA | Deep Transfer Learning (DTL) technique and Deep Multi-task Transfer Learning (DMTL) |
VGG19 | DTL technique accurately label head, vacuole, and acrosome with accuracy of 84%, 94%, and 79% respectively. Similarly, DMTL technique accurately label head, vacuole, and acrosome with 82%, 92.33%, and 80.66% respectively. |
[63] | Testicular biopsies | VGG16 | MobileNetV2 | mean average precision mAp 0.741 Average recall AR 0.376. |
[64] | Sperm image dataset | Enhanced Otsu's threshold method |
Deep CNN | Accuracy 98.99% |
[65] | SVIA dataset |
k-means, U-net, SegNet and DeepLabv3 | Deep CNN | The effects of U-net and Deeplab_v3 are the best, and other segmentation methods have a certain gap compared with these two segmentation methods |
[71] | VISEM | Crocker-Grier Algorithm With CNN |
RNN | The mean absolute error (MAE) could be reduced from 8.83 to 7.31. |
[72] | VISEM | YOLOv4 algorithm | YOLO Network | The results obtained were 90.31% AP (Average Precision) for sperm objects and 68.19% AP (Average Precision) for non-sperm objects, then for the results of the training obtained by the model 79.58% mAP (Mean Average Precision). |
Model | Accuarcy | Preceision | Recall | F1-score |
SDNN | 89.00 | 96.18 | 91.63 | 95.23 |
VGGI16 | 70.67 | 84.15 | 72.30 | 77.78 |
VGG19 | 71.00 | 83.87 | 73.24 | 78.20 |
ResNet.50 | 71.00 | 84.24 | 72.77 | 78.09 |
Inception V3 | 61.00 | 78.57 | 61.97 | 69.29 |
InceptipnResNet | 70.33 | 84.44 | 71.36 | 77.35 |
MobileNet | 71.00 | 85.00 | 71.83 | 77.86 |
MobileNetV2 | 69.00 | 81.58 | 72.77 | 76.92 |
DenseNaet | 66.00 | 81.71 | 67.14 | 73.71 |
NASNetMobile | 71.00 | 83.51 | 73.71 | 78.30 |
NASNetLarge | 63.00 | 80.36 | 63.38 | 70.87 |
Xception | 55.00 | 73.78 | 56.81 | 64.19 |
Model | Accuarcy | Preceision | Recall | F1-score |
SDNN | 92.00 | 97.70 | 93.43 | 95.52 |
VGGI16 | 87.33 | 96.28 | 88.93 | 92.46 |
VGG19 | 87.33 | 95.90 | 89.31 | 92.49 |
ResNet.50 | 87.33 | 95.53 | 89.69 | 92.52 |
Inception V3 | 82.67 | 94.49 | 85.11 | 89.56 |
InceptipnResNet | 84.33 | 95.36 | 86.26 | 90.58 |
MobileNet | 77.67 | 94.12 | 79.39 | 86.13 |
MobileNetV2 | 86.67 | 95.87 | 88.55 | 92.06 |
DenseNaet | 85.67 | 95.44 | 87.79 | 91.45 |
NASNetMobile | 86.00 | 95.45 | 88.17 | 91.67 |
NASNetLarge | 28.00 | 73.47 | 27.48 | 40.00 |
Xception | 48.00 | 86.30 | 48.09 | 61.76 |
Model | Accuarcy | Preceision | Recall | F1-score |
SDNN | 90.00 | 96.94 | 92.02 | 95.92 |
VGGI16 | 73.00 | 86.70 | 74.43 | 80.10 |
VGG19 | 73.33 | 87.17 | 74.43 | 80.30 |
ResNet.50 | 73.67 | 87.23 | 74.89 | 80.59 |
Inception V3 | 70.00 | 84.49 | 72.15 | 77.83 |
InceptipnResNet | 73.00 | 87.10 | 73.97 | 80.00 |
MobileNet | 73.00 | 86.32 | 74.89 | 80.20 |
MobileNetV2 | 62.67 | 81.29 | 63.47 | 71.28 |
DenseNaet | 72.33 | 86.56 | 73.52 | 79.51 |
NASNetMobile | 71.67 | 86.02 | 73.06 | 79.01 |
NASNetLarge | 69.67 | 84.41 | 71.69 | 77.53 |
Xception | 66.00 | 81.97 | 68.49 | 74.63 |
Label | Method | Accuracy | Precision | Recall | f0.5 score |
Acrosome | |||||
SDNN | 89.00 | 96.18 | 91.63 | 95.23 | |
GeNAS | 77.66 | 84.76 | 83.56 | 84.42 | |
Random Search | 69.66 | 74.5 | 86.8 | 76.67 | |
Javadi et al. | 76.67 | 85.93 | 80.02 | 84.74 | |
Ghasemian et al. | N/A | N/A | N/A | N/A | |
Head | SDNN | 90.00 | 96.94 | 92.02 | 95.92 |
GeNAS | 77.33 | 84.47 | 84.47 | 84.47 | |
Random Search | 76.00 | 80.49 | 88.58 | 81.98 | |
Javadi et al. | 77.00 | 83.48 | 85.93 | 83.86 | |
Ghasemian et al. | 61.00 | 76.71 | 71.79 | 75.68 | |
Vacuole | SDNN | 92.00 | 97.70 | 93.43 | 96.82 |
GeNAS | 91.66 | 95.40 | 95.03 | 95.32 | |
Random Search | 89.00 | 94.20 | 93.12 | 93.98 | |
Javadi et al. | 91.33 | 94.36 | 95.80 | 94.65 | |
Ghasemian et al. | 80.33 | 83.21 | 93.56 | 85.09 |
Ref | Dataset name | Method of classification | Outcomes |
[35] | HSMA-DS | SMA algorithm | SMA algorithm was used for classification and results shows above 90% of accuracy for sperm abnormality detection. It also shows high rates of True positive and True negative |
[39] | - | Particle Swarm Optimization (PSO) algorithm |
PSO gives the highest accuracy then both MLP and SVM methods, PSO obtains superior specificity values at 88 %. Therefore, PSO seems to be the preferred method for predicting the quality of fertility data with respect to the environmental factors and lifestyle, this method seems to be useful with the new data also as it can handle generalization potential |
[47] | Linear Discriminant Analysis (LDA) | LDA was used for classification. To do so LDA minimize variance within class and maximize between classes, as a result maximum separation between classes was achieved. | |
[49] | Fertility Dataset | Artificial Neural Networks (ANN) Sperm Whale Optimization algorithm (SWA) |
ANN-SWA was based on four phases. First one was trained by fertility diseases. In second multilayer perceptron (MLP) was employed to get maximum fitness function. In third phase parameter were optimized like biases and weights to get rapid conjunction with high accuracy. In final step ANN-SWA was employed to get sperm fertility prediction. |
[51] | 3D+t | Algorithm based on one-class classification | Sperms were assigned by an ID for the sake of tracking. Proposed algorithm extract similar traces as compared with ground truth. Results shows that this algorithm is more accurate and robust than multi-branch algorithms |
[17] | Video dataset | CNN | This CNN contain 5-layer network with 3 convolution layers with 16, 32 and 64 3 × 3 filters respectively. These were followed by 2 fully connected layers with 256 neurons in each layer and finally two node soft max layer that classify input into sperm and non-sperm |
[33] | VISEM | CNN | CNN was trained that learned temporal and spatial combined features of sperm motility. That was based on image preparation and representation of single or stacking frame. Then participant data and output of CNN model was combined. Before performing multivariate prediction on the three motility variables, this combined vector was passed through two fully-connected layers. |
[53] | In-House dataset | VGG16 (CNN) | This deep learning model will help clinicians for the microscopy-based sperm selection, it predicts fast DNA quality with in 10ms per cell and up to 86 % successful sperm selection for fertility analysis. |
[54] | Gold-standard dataset | SVM classifier | Multi-channel image generation and SFFS algorithm was optimized with SVM classifier to compete with other classifiers. As a result, axial filament pixels show improved performance and accurate classification. |
[31] | MHSMA | Deep CNN | CNN was used for classification of sperm image dataset into normal and abnormal sperms with the help of multiple morphological characteristics. CNN achieved F 0.5 score of 94.65%, 83.86% and 84.74% in vacuole, head and acrosome abnormality revealing, respectively. |
[32] | HuSHeM and SCIAN | Deep Neural Network architecture | This Deep Neural Network architecture gave high accuracy in head-to-head comparison against other famous methods with identical dataset. Result shows better true positive rate 94.1% vs 92.3% on HuSHeM dataset, over cascade ensemble of SVM (CE-SVM). |
[56] | Video dataset | CSR-DCF tracker algorithm | CSR-DCF algorithm is a robust multi-sperm tracker that works accurately, even when sperms collide or cross each other pass. This technique Obtained 96.61% F1 score from evaluation. |
[57] | Gradient-Boosted Trees (GBT) and random forest, Logistic regression | GBT technique produced improved prediction results of successful and unsuccessful TESE against MvLRM, with little bit lower sensitivity. This model efficiently deals with missing values of several variables by automatically self-learning based on training loss reduction. | |
[58] | Full spectrum neural network (FSNN) Selected peak neural network (SPNN) |
To achieve low root mean square error these models were boosted for transfer function, structure of hidden layer and different combination of training techniques. FSNN shows 93% prediction accuracy for sperm concentration and gave 100% assessments for differentiating the samples of patients and healthy donors. | |
[68] | Sperm Morphology Image Data Set (SMIDS) | InceptionV3, VGG19 and MobileNet | Results shows that SVM model with RBF kernel achieved highest accuracy that is 83.82% and tested by KAZE descriptor-based features. On the other hand, k-NN over DWT-based features shows worst classifier result with 47.23% efficiency. In terms of sperm morphology representation problem, KAZE features were the most informative features for RusBoost, Polynomial, Bagging and RBF kernel SVM classifiers |
[59] | HuSHeM and SCIAN | Morphological Classification of Human Sperm Heads MC-HSH |
In this deep learning architecture, there are a total of 53 convolutional layers. Before each convolutional layer, the batch normalization and LeakyReLU were used. They also apply the channel-wise concatenation and element-wise addition to make this model more efficient classification of human sperm heads in terms of morphology. |
[60] | Video dataset | Elliptic Scanning Algorithm (ESA) Novel Faster Region Convolutional Neural Network (FRCNN), |
A novel FRCNN with ESA detection algorithm was introduced classification and analysis of human sperm. Results shows accuracy of 97.37%. Moreover, for tracking and motility analysis a Tail head movement-based (THMA) algorithm was used. |
[8] | VISEM | ResNet18 model | The first ResNet18 model reached 100% accuracy on both validation and test sets. The second ResNet18 + Tabular Data model correctly predicted with an accuracy of 100% on the validation set and an average accuracy 88.89 on the test set. The third ResNet34 + Tabular Data model correctly predicted with an accuracy 87.5% on the validation set and 77.78% on the test set. |
[62] | VISEM | Linear Support vector Regressor, MultiLayer, perceptron, CNN, RNN |
This technique shows best results for motility prediction and state-of-the-art. The lower the Mean Absolute Error (MAE) the better, showing that SVR models achieve better results than the other models for every type of features. BoWs outperform models trained on emsd features. Considering the best validation result, a minimum MAE of 8.60 is obtained on evaluation with the SVR trained on emsd features. Measured against the state-of-the-art, this result shows a relative improvement of 2.6% |
[70] | MHSMA | Deep Transfer Learning (DTL) technique Deep Multi-task Transfer Learning (DMTL) |
The proposed algorithm achieved accuracy of vacuole, acrosome and head by 94%, 80.66% and 84% respectively by gaining improvement of 1.33%, 3% and 6.66% on these labels respectively. Therefore, this algorithm can be used in fertility institutes and make work easier for hospital staff. |
[63] | Testicular biopsies | Convolutional layers of VGG16, GoogleNet | Deep learning CASA system achieved a mean average precision (MAP) of 0.741, with an average recall (AR) of 0.376 on given dataset. |
[64] | Sperm image dataset | Deep Convolutional Neural Network | MATLAB 2018a was employed to perform overall procedure. Classification process was improved with Deep CNN statistical training and morphology of sperm images. After comparison results shoes efficient performance of proposed technique by providing neck and tail accuracy 97.99%, head utilize 98.22% and vacuole achieve 99.66 accuracy. |
[65] | SVIA dataset |
AlexNet, VGG, ResNet, GoogleNet, DenseNet, Inception-v3, Mobilenet-v2, ShuffleNet-v2, Xception, Vision Transformer ViT, BotNet, DeiT, T2T-ViT. | Inception-v3 and DenseNet-121 shows maximum accuracy 98.32 % and 98.06 % respectively. While ViT shows lowest result i.e 93 % |
[76] | MHSMA | VGG19 and ResNet50 | VGG19 and ResNet50 shows best accuracy of 87.33%, 71% and 73% for vacuole, acrosome, and head label, respectively. |
[77] | MHSMA | Genetic Neural Architecture Search | This Neural Architecture method shows accuracy of 91.66%, 77.66%, and 77.33% in the vacuole, acrosome, and head detection, respectively |
[79] | In-House dataset | VGG16 (CNN) | The VGG16 model is fine-tuned and achieved an accuracy of 97.92% and a sensitivity of 98.82%. Moreover, it achieved an F1 score of 98.53%. |
[78] | MHSMA | Sequential Deep Neural Network architecture (SDNN) | Results show that Sequential Deep Neural Network architecture successfully detected abnormalities in the acrosome, head, and vacuole with an accuracy of 89%, 90%, and 92%, respectively. Moreover, it also out class all state-of-the-art model as well as GeNAS algorithm proposed by Miahi et al. |
Ref | Dataset name | Method of Classification | Network | Evaluation metrics |
[56] | Video dataset | CSR-DCF tracker algorithm | RetinaNet | Precision 99.1 % F1 score 96.61% |
[57] | Machine-learning model |
Gradient-Boosted Trees (GBT) and random forest, Logistic regression | GBT sensitivity 91% MvLRM sensitivity 97% |
|
[58] | ANN | Full spectrum neural network (FSNN) Selected peak neural network (SPNN) |
Accuracy 93% | |
[80] | Sperm Morphology Image Data Set (SMIDS) | Wavelet Descriptor-based features with SVM |
MobileNet | Accuracy 80.5% and 83.8% |
[60] | Video dataset | Elliptic Scanning Algorithm (ESA) Novel Faster Region Convolutional Neural Network (FRCNN), |
FRCNN | Accuracy 97.37%. |
[8] | VISEM | CNN | ResNet18 ResNet34 |
Accuracy 88.89% Accuracy 77.78% |
[62] | VISEM | Four Machine-learning models |
SVR, MLP, CNN, RNN |
Mean Absolute Error 7.31 |
[70] | MHSMA | Deep CNN | Deep Transfer Learning (DTL) technique Deep Multi-task Transfer Learning (DMTL) |
DTL technique accurately label head, vacuole, and acrosome with accuracy of 84%, 94%, and 79% respectively. Similarly, DMTL technique accurately label head, vacuole, and acrosome with 82%, 92.33%, and 80.66% respectively. |
[63] | Testicular biopsies | CNN | VGG GoogleNet 16 |
mean average precision (mAP) of 0.741 average recall (AR) of 0.376 |
[64] | Sperm image dataset | CNN | Deep CNN | Neck and tail accuracy 97.99%, head utilize 98.22% and vacuole achieve 99.66% accuracy |
[65] | SVIA dataset |
18 Deep Learning models | AlexNet, VGG, ResNet, GoogleNet, DenseNet, Inception-v3, Mobilenet-v2, ShuffleNet-v2, Xception, Vision Transformer ViT, BotNet, DeiT, T2T-ViT. | Inception-v3 and DenseNet-121 shows maximum accuracy 98.32 % and 98.06 % respectively. While ViT shows lowest result i.e 93 % |
[76] | MHSMA | Deep CNN | VGG19 and ResNet50 | VGG19 and ResNet50 shows best accuracy of 87.33%, 71% and 73% for vacuole, acrosome, and head label, respectively. |
[77] | MHSMA | CNN | Genetic Neural Architecture Search | This Neural Architecture method shows accuracy of 91.66%, 77.66%, and 77.33% in the vacuole, acrosome, and head detection, respectively |
[78] | MHSMA | Deep CNN | Sequential Deep Neural Network architecture (SDNN) | Results show that Sequential Deep Neural Network architecture successfully detected abnormalities in the acrosome, head, and vacuole with an accuracy of 89%, 90%, and 92%, respectively. |
Ref | Dataset name | Evaluation metrics | Outcomes |
[36] | Automated Sperm Morphology Analyzer (ASMA) |
Accuracy (A c) 96.829% Sensitivity (S c) 97.522% Specificity (S p) 95.275% |
|
[35] | HSMA-DS | Confusion metrics | SMA algorithm shows its performance in less than 9 seconds. True negative rate was 100% for tail malformation detection. However, there was small number of False negative due to high rate of noise in the image. |
[82] | Video dataset | Confusion metrics | Accuracy 0.97, sensitivity 0.97, specificity 0.71 and precision 0.99 |
[39] | - | confusion metrics, 12 parameters used | The presented methods and their improvements can at least replace the initial tests for checking the fertility of a population or an individual as well as in selecting the donors for data collection. Stating and testing environmental and lifestyle factors trough the mentioned techniques are able to give an accuracy of up-to 90%, these results support the argument. |
[41] | Video dataset | Intersecting cortical model (ICM) | Results shows that this model is more robust and accurate than four state of the art segmentation models, in terms of accuracy, sensitivity, specificity and precision with success rate of 98.14%, 98.82%, 86.46% and 99.81% respectively. |
[42] | Gold-standard | Dice Coefficient | Dice coefficient was used to evaluate segmentation performance. Dice coefficient was 3% more than Chang method. Total performance was 88%. |
[45] | SCIAN-MorphoSpermGS | Two-stage classification | Results shows 73% efficiency as well as human experts were fully satisfied with the classification of sperm heads. |
[47] | Confusion metrics | This method achieved average F-score of 92.9 %, average recall of 92.3 %, average precision of 93.5%, and average accuracy of 92.2%. This method shows very impressive result in abnormalities recognizing for all above mentioned four classes. | |
[44] | Confusion metrics | The average accuracy overall classes reached the 78% by using confusion metrics measurements. | |
[49] | Fertility Dataset | 10-fold cross validation | 99.96% of the accuracy. |
[52] | Gold-standard dataset | Dice, Jaccard, and F1-score metrics | The CNN was validated with 20 images of sperm sample. Results shows 0.94, 0.87, and 0.88 of Dice Similarity Coefficient for the head, the acrosome, and the nucleus segments, respectively |
[17] | Video dataset | SQA-V Gold | SQA-V Gold obtain a B0 value of 1.91 and confidence interval of (−12.6 to −0.57) and B1 value of 1.665 with 95% confidence interval of (1.5 to 1.8) |
[31] | MHSMA | Confusion metrics | The proposed technique attains F0.5 Score of 83.86%, 84.74% and 94.65% in head, acrosome and vacuole abnormality detection, respectively. |
[56] | Video dataset | Average precision (AP) metric | Precision 99.1 % and obtained 96.61% F1 score from evaluation of their proposed tracker method. |
[58] | 13-fold cross validation | 13-fold cross validation shows average classification accuracy of 92% was achieved by the system | |
[68] | Sperm Morphology Image Data Set (SMIDS) | K-Fold Cross Validation, with K=5 |
Precision 83%, recall 82%, f-measure 83% and accuracy 88%. |
[60] | Video dataset | Confusion metrics | The Faster Region Convolutional Neural Network (FRCNN) was used for classification and improve the efficiency of computer assisted semen analysis (CASA). Other methods that were used in literature measured with different densities of fluid. But in this study precision will not drop below 91%, even density was increased. So overall sperm detection process will be improved. |
[69] | VISEM | Region Based Convolutional Neural Networks (R-CNN) |
The motility of the semen sample calculated by the algorithm differs from the experimental result by only 2.92% on average, while the accuracy of sperm head detection was 91.77%. |
[61] | SCIAN-SpermSegGS | cross validation, Dice coefficient | Results show that U-net with transfer learning, outclass state of the art sperm segmentation method with 95% overlapping efficiency against hand-segmented masks for sperm head 0.96, acrosome 0.94 and nucleus 0.95. |
[65] | SVIA dataset |
Confusion metrics | Inception-v3 and DenseNet-121 shows maximum accuracy 98.32 % and 98.06 % respectively. While ViT shows lowest result i.e 93 % |
[71] | VISEM | 3-fold cross-validation | The mean absolute error (MAE) could be reduced from 8.83 to 7.31. |
[72] | VISEM | Confusion metrics | The results obtained were 90.31% AP (Average Precision) for sperm objects and 68.19% AP (Average Precision) for non-sperm objects, then for the results of the training obtained by the model 79.58% mAP (Mean Average Precision). |
[77] | MHSMA | Confusion metrics | This Neural Architecture method shows accuracy of 91.66%, 77.66%, and 77.33% in the vacuole, acrosome, and head detection, respectively |
[75] | MHSMA | Confusion metrics | VGG19 and ResNet50 shows best accuracy of 87.33%, 71% and 73% for vacuole, acrosome, and head label, respectively. |
[79] | In-House dataset | Confusion metrics | The VGG16 model is fine-tuned and achieved an accuracy of 97.92% and a sensitivity of 98.82%, precision equals 98.25%, specificity equals 95.71% and F1 Score equals 98.53% Moreover, it achieved an F1 score of 98.53%. |
[75] | VISEM | Different evaluation metrics | YOLOv5 deep learning model trained on the VISEM-Tracking dataset present baseline sperm detection performances. This dataset can be used to train complex deep learning models to analyze spermatozoa. |
[74] | VISEM | detailed quantitative investigation | The best-performing model is yolov5l. This network achieves a precision of 88.6 per cent, recall of 52.6, and mAP is 72.1. Other networks are achieving lower results. The second-best network is nano, with a mAP of 69.6 and precision of 64.7 per cent |
[78] | MHSMA | Confusion metrics | Results show that Sequential Deep Neural Network architecture successfully detected abnormalities in the acrosome, head, and vacuole with an accuracy of 89%, 90%, and 92%, respectively. |
Ref | Dataset name | Source Link attach | Type | No. of instance | Color space | Resolution | classes |
[35] | - | - | Images | 10 to 15 | RGB | 576 × 764 | - |
[12] | Original images | http://dx.doi.org/10.1155/2013/687607 | Images | 160 | Gray Scale | - | 2 |
[36] | Public dataset | UCI data repository [14] | Images | 100 | - | - | 2 |
[37] | HSMA-DS | Images | 1457 | RGB | 576 × 764 | ||
[38] | Video dataset | Hospital Universiti Sains Malaysia (HUSM) | Video | 80 | |||
[39] | - | Images | 100 | 7 | 2 | ||
[40] | Video dataset | Andrology lab of the Academic Unit of Reproductive and Developmental Medicine (University of Sheffield) | video | 2040 × 1086 | |||
[41] | Video dataset | Hospital Universiti Sains Malaysia (HUSM) | Video | 20 | RGB | 480 × 640 | |
[42] | Gold-stadard dataset | Images | 19 | RGB | 780 × 580 | ||
[43] | - | Images | 886 | 2 | |||
[44] | Video dataset | Academic Unit of Reproductive and Develop mental Medicine (AURDM) | Video | 940 | 2040 × 1086 | 4 | |
[45] | SCIAN-MorphoSpermGS | Laboratory of Spermiogram, (ICBM), Faculty of Medicine, University of Chile. | Images | 7 × 7 | 5 | ||
[46] | HuSHeM | https://data.mendeley.com/datasets/tt3yj2pf38/1. | Images | 216 sperm head | RGB | 131×131 | 4 |
[47] | Isfahan Fertility and Infertility Center (IFIC) | Images | 170 sperm head | RGB | 720×576 | 4 | |
[48] | Images | 765 | Gray Scale | 1920×1440 | 2 | ||
[49] | Fertility Dataset |
UCI repository | Images | 100 | |||
[50] | Ocular images | Images | 13 | ||||
[51] | 3D+t | Images | 512 × 512 | ||||
[52] | Gold-standard dataset | Images | 20 | 780 × 580 | |||
[17] | Video dataset | Video | 90 frames at 30fps (3600 images) |
3200 × 2400 | 2 | ||
[33] | VISEM | [https://datasets.simula.no/viem/] | Video | 85 | 3 | ||
[53] | In-house dataset | https://figshare.com/articles/Deep_learningbased_selection_of_human_sperm_with_high_DNA_integrity/8124932 | Images | 1064 | |||
[32] | HuSHeM and SCIAN. | Images | 216 and 1132 |
Gray Scale and RGB | 5 | ||
[54] | Gold-standard dataset | Images | 20 | 780 × 580 | |||
[55] | Video dataset | Shenzhen Sixth People's Hospital, | Video | 54 | 720×540 | ||
[31] | MHSMA | [35] | Images | 1540 | RGB | 1280×1024 | 2 |
[56] | Video dataset | Royan institute Research Lab | Video | 36 | 768 × 576 | ||
[57] | - | SUMC | Images | 119 | 18 | ||
[58] | - | Avicenna Research Institute (Tehran, Iran) | 41 | 7 | |||
[59] | HuSHeM and SCIAN. | Images | 1154 and 1132 | Gray Scale | 5 | ||
[60] | Sperm Morphology Image Data Set (SMIDS) | Infertility centre of Istanbul University | Images | 200 | |||
[61] | Video dataset | Video | 288 × 352 | ||||
[62] | VISEM | https://datasets.simula.no/visem/ | Video | 85 | 640 × 480 | ||
[63] | SCIAN-SpermSegGS | Faculty of Medicine, University of Chile, Santiago, Chile. | Images | 19 | |||
[8] | VISEM | Simula Research Laboratory | Video | 85 | 640 × 480 | ||
[64] | VISEM | Simula Research Laboratory | Video | 85 | 640 × 480 | ||
[65] | MHSMA | Images | 1540 | Gray Scale | |||
[66] | Testicular biopsies | Images | 702 | ||||
[67] | Sperm image dataset | http://morfologia.cedai.cl/publi c/) | Images | 20 | Gray Scale | ||
[68] | SVIA dataset | https://github.com/Demozsj/Detection-Sperm. | Images and video | 101 and 130042 | 698528 | ||
[69] | MHSMA | Images | 1540 | Gray Scale | |||
[70] | MHSMA | Images | 1540 | Gray Scale | |||
[71] | VISEM | Simula Research Laboratory | Video | 85 | 640 × 480 |
Subset-A | Video | Number 101 |
No. of annotated object 125,000 | Format .mp4 |
Image | Number 3590 |
Size 698 × 528 × 3 |
Format .png |
|
Subset-B | Video | Number 10 |
No. of annotated object 26,000 | Format .mp4 |
Image | Number 451 |
Size 698 × 528 × 3 |
Format .png |
|
Subset-C | Image | Class Sperm impurity |
Number 121,401 4,479 |
Format .png |
Dataset | Video | Image | Size | Number of annotated objects |
HuSHeM | 0 | 216 | 131 × 131 | 0 |
Visem | 85 | 0 | 640 × 480 | 0 |
MHSMA | 0 | 1540 | 128 × 128 or 64 × 64 | 0 |
SIVA | 101 | 130042 | 698 × 528 | 127600 |
Ref | Dataset name | Pre-processing techniques | Outcomes |
[36] | - | Red component of RGB | Author first scaled image as a pre-processing technique using Red component of RGB. M is the range between zero to maximum intensity, and it was 12-byte data I initially, then scaled to a new intensity Is between 0 to 255 (8-bit). It was formulated as I s = I/M × 255. |
[37] | Public dataset | SMOTE | Removing majority Tomek Links and balance data with the help of SMOTE technique. |
[35] | HSMA-DS | Gaussian filter | By applying Gaussian Filter on Y channel Noise Reduction by wavelet transform, the percentage of noises was decreases. However, this percentage was not acceptable for further processing |
[39] | - | optional attribute selection process | The cumulative data was pre-processed using an optional attribute selection process. This intermediate step was used to filter only the relevant and important attributes from the bulk data. Attribute selection can affect the overall results derived from the data. |
[41] | Video dataset | Laplacian of Gaussian (LoG) filter |
First, they convert images into gray scale from RGB. Because colour image required more processing time as compare to gray scale images. In next step Laplacian of Gaussian (LoG) filter was deployed in kernel as a pre-processing step so that it automatically detect and segment human spermatozoa. |
[67] | SCIAN-MorphoSpermGS | Anisotropic diffusion | Anisotropic diffusion method was used for noise reduction. Moreover, this method also preserves the border of images. |
[50] | Ocular images | Modified Overlapping Groups Shrinkage (MOGS) | In MOGS, convex function is used to detect the correct region of sperm, therefore denoising problem was accepted. In this regard non-convex regularization was used and desired group sparsity property were verified |
[51] | 3D+t | logarithm transform | To shrink the range of intensity values, log transformation (V log (~ x) = log (V (~ x) + 1)) was applied on image stack V. so it increases the contrast of low intensity values. |
[52] | Gold-standard dataset | Homomorphic Filtering Filling holes |
This method helps to highlight each sperm in the background by correct uneven illumination. Moreover, some post processing steps were also involved to improve segmentation procedure. |
[54] | Gold-standard dataset | Serialized | This method helps to highlight sperm cells in the image rather than other particles. It also restrains unnecessary characteristics of the image like low contrast between the sperms tails and its neighbouring region and non-uniform distribution of light |
[59] | HuSHeM and SCIAN. | Rotation Translation flipping |
Author used three common techniques as previously used in the training set of the SCIAN dataset. For rotation, they rotate the training image by 25 to 25 degrees. For translation, they shift ~6% of the original image to the left, the right, up, and down. For flipping, they vertically flip the image. Due to the same distribution of classes within this dataset, they apply equal augmentation options to each class |
[68] | Sperm Morphology Image Data Set (SMIDS) | Modified Overlapping Groups Shrinkage (MOGS) |
Ilhan et al again used same pre-processing technique as he had used in 2018 called MOGS. This technique helps to remove random noise without changing sperm shapes. |
[60] | Video dataset | median filter, elliptic curve | Median filter was also used for pre-processing, this technique was applied to each image. The edges of images were preserved when they pass through median filter. |
[8] | VISEM | Convolutional neural network (CNN) | CNN was a specialized type of network used that has a grid-like topology for processing data. They are most commonly used on image and video data |
[62] | VISEM | Spatial warping, Background removal, and Filtering |
Geometric distortion, non-uniform contrast, and noise are alleviated by spatial warping, background removal and filtering, respectively |
[63] | Testicular biopsies | Glare filters and diffraction correction, MobileNetV2 |
A single embryologist annotated each image with bounding boxes around each identified spermatozoon. At the time of collection, each image was normalized, passed through glare filters and diffraction correction (Hamilton Thorne, HT video and image capture software version 3, Hamilton Thorne, Inc., Beverly, MA, USA), and had microscopy artifacts removed. |
[64] | Sperm image dataset | Median filtering | It helps to reduce noise in the image and perform edge detection and improve image resolution. It also sorts pixel value by comparing mean filter. It does not create any unreal surrounding pixels, so it gave efficient result after pre-processing. |
Ref | Dataset name | Method of Feature Extraction | Outcomes |
[12] | Original Images | Fourier Transform Infrared Spectroscopy (FTIRS), grey level feature | FTIRS and grey level feature was used to differentiate multiple sperms according to their characteristics. |
[35] | HSMA-DS | Sobel algorithm | Sobel algorithm was used for edge detection |
[40] | video frames | Otsu's threshold | Author use Otsu's threshold as a feature extraction technique. It converts image to gray scale then eliminate background from the foreground. |
[42] | Gold Standard | Gaussian filter, Ostu method, Gradient vector flow, curvature and edge energy | Author use ostu method for sperm head detection. Moreover, they use histogram of the Hue channel of HSV color space along with size criterion for successful sperm head detection in microscopic image |
[43] | - | Phase Map Acquisition | Phase Map Acquisition (PMA) technique was used to extract 89 custom-design features from dataset. These features included the acrosome area, mean OPD and the correlation of the OPD map of the head etc |
[44] | - | (Speeded-Up Robust Features) SURF | It detects key point from given dataset. Hessian matrix was used to select most demonstrative pixels founded in low-level features. |
[67] | SCIAN-MorphoSpermGS | five shape-based descriptors | Author proposes combine five shape-based descriptors It easily recognize human sperm head and also help in sperm morphology analysis. |
[50] | Ocular images | Pixel-Based Spatial analyses, Blob analyses | PBS analyses, Blob analyses was used for feature extraction (spatial-based features), over segmented parts. |
[33] | VISEM | Lucene Image Retrieval (LIRE) Tamura image features |
LIRE is a java library that use to extract features from images and photos with the help of texture and color characteristics. Author test more than 30 features with machine learning algorithms but Tamura image features work best with proposed algorithm in this study. |
[55] | Gaussian mixture model | This technique used statistical information of pixel for background representation, such that target pixel was figure out using statistical difference and judged probability density in long time period with large sample value. As result a complex dynamic background could be modelled. |
Ref | Dataset name | Method of Segmentation | Outcomes |
[36] | - | Markov random field (MRF) Model and Entropy based (EM) algorithm |
In first section Bayesian classifier was used to segment sperm's Nucleus, Mid-piece and Acrosome. In second section EM and MRF model was used to upgrade and obtain class conditional probability density function (CCPDF) and apriori probability for each class. |
[12] | Original images | Average Rank Difference | By using Average Rank Difference between abnormal and normal sperms the segmentation of accuracy 55% achieved. |
[37] | Public dataset | Clustering-Based Decision Forests (CBDF Algorithm) | Author used CBDF Algorithm to deal with unbalanced class learning problem for sperm analysis. Result shows that CBDF Algorithm outclass state of the art techniques like logistic regression, multilayer perceptron neural networks, random forests, Support Vector Machines and decision tree etc with great difference. |
[40] | Video dataset | The structural similarity measure (SSIM) The correlation (CORR) coefficient, and The Bhattacharyya distance (BHAT) |
Author used 3 different techniques for segmentation. The original image was labelled with 1 or 0 and count of sperm, non-sperm, immotile and motile cells. Only motile cells were kept for experiment and all other non-sperm and immotile cells were removed. |
[42] | Gold standard | GVF, curvature and edge energy (TDCEE), Dice Coefficient | For more refine segmentation, researcher used edge-based active contour method. Moreover, they also used a novel tail point detection technique that trace and eliminate midpiece from segmented head, for more efficient segmentation. Results shows 92% achievement against hand segmented ground truth that outperform famous techniques. |
[43] | - | Support Vector Machines (SVMs) |
The SVM classifier achieves receiver operating characteristic curve of 88.59%, precisions of 90% and precision-recall curve of 88.67%. Researcher believed that this automatic process of sperm analysis will become base of automatic and objective sperm cell collection in IVF |
[44] | Support Vector Machines (SVMs) |
Support Vector Machines (SVMs) was used for segmentation. Simplified feature vectors that extracted from dataset were used to classify sperm cells into normal, abnormal and non-cell categories. | |
[67] | SCIAN-MorphoSpermGS | SVM-based cascade classification | This two-stage classification scheme shows better results than monolithic classifiers and it also work like a human expert. |
[50] | Ocular images | Fuzzy C- Means (FCM) and K-Mean |
FCM segmented the gray scaled imaged more successfully than K-Means because of fuzzy technique. But there is room of improvement because only 50% sperms were correctly classified. |
[55] | Threshold segmentation | This technique was applied on background image for the sake of counting immotile sperm. |
Ref | Dataset name | Method of Segmentation | Outcomes |
[38] | Video dataset | Pulse Coupled Neural Network (PCNN) Particle Swarm Optimization (PSO) |
PCNN is very famous method for sperm image segmentation. It helps to solve inaccurate sperm head shape problems as well. It also uses to differentiate agglutinated sperms with high accuracy and precision. Moreover, PCNN model include eight parameters so, optimized PSO algorithm was used improve PCNN parameters. |
[41] | Video dataset | Intersecting cortical model (ICM) | For efficient sperm head segmentation ICM was derived from several visual cortex models. There was some parameter selection problem so researcher optimize ICM network with PSO. New fitness function know as feature mutual information was introduced here. This model was more efficient and precise than other famous segmentation techniques. |
[47] | - | Active contours. | Active contours method was used for segmentation. This method automatically segmented cropped sperm heads. Color images were transformed to gray scale images and apply threshold using Otsu method for fast locating of sperm heads. Edges of the images were extracted by Canny edge detector method and Gradient Vector Flow (GVF) active contour method was employed to extract outer contour of sperm heads. |
[48] | Convolutional Neural Network (CNN) | CNN outperform classical image analysis approach by achieving 91.89% recall and 93.87% precision on a given dataset. | |
[52] | Gold-standard dataset | Convolutional Neural Networks (CNN) | For segmentation of sperm head a deep convolutional neural network was used. For improve head segmentation, geometric constraints and filling holes operation were also employed. Then SVM helps to classify all pixel of segmented heads to acrosome and nucleus regions. |
[54] | Gold-standard dataset | CNN model 1 CNN model 2 |
Two CNN networks were used with different technique for segmentation of head and axial filament of the sperms. Overlapping patches were extracted from RGB images at the end of background using CNN model 1. Moreover, at Histogram Stretching stage, patches was extracted from RGD images using CNN model 2 |
[31] | MHSMA | Convolutional Neural Networks (CNN) |
CNN network was used to deal with morphological deformities present in human sperm like head, vacuole, and acrosome. It was first technique that focus on acrosome. It also works very efficient in low resolution and non-stained images |
[68] | Sperm Morphology Image Data Set (SMIDS) | K-Means clustering Fuzzy C-Means clustering |
MOGS technique was used as a pre-processing step and it helps to reduce noise present in sample images and improve the overall segmentation process. Similarly, Fuzzy C-Means clustering shows impressive results when MOGS was applied on images as a pre-processing method. Moreover, Fuzzy C-Means clustering apply fuzziness for the belongingness of each image pixels, which increase efficiency of segmentation process. |
[60] | Video dataset | Local Region Contour segmentation | Author separates tail region with binary images with the help of local region contour segmentation. In past may algorithm were unsuccessful to detect tail region from video dataset because of minor gradient in the tail region. Some researcher able to do so while denoising images. |
[61] | SCIAN-SpermSegGS | U-Net and Mask- RCNN |
Results show that U-net with transfer learning, outclass state of the art sperm segmentation method with 95% overlapping efficiency against hand-segmented masks for sperm head 0.96, acrosome 0.94 and nucleus 0.95. |
[69] | VISEM | Region Based Convolutional Neural Networks (R-CNN) architecture |
The Faster R-CNN-Inception_V2-COCO model was used to develop the sperm counting algorithm. Its advantage is that it is quite accurate to learn to distinguish objects even from a small database. The model takes about 50 ms to analyze the image. Because the model uses regions, it is easier to detect a larger number of objects in a single frame. Additionally, the R-CNN model can read information from frames of any size, so there is no need to crop frames before submitting them to the neural network. Achieved 91.77% (95% CI, 91.11–92.43%) accuracy of sperm head detection on the VISEM sperm sample video dataset. |
[62] | VISEM | Mean squared displacement of each particle (imsd) |
Segmentation can be done for each individual particle (mean squared displacement of each particle (imsd)) or again as an average for all of the particles (ensemble mean squared displacement of all particles (emsd)). These results show a relative improvement of 4.4% against state-of-the-art results. |
[70] | MHSMA | Deep Transfer Learning (DTL) technique and Deep Multi-task Transfer Learning (DMTL) |
DTL technique accurately label head, vacuole, and acrosome with accuracy of 84%, 94%, and 79% respectively. Similarly, DMTL technique accurately label head, vacuole, and acrosome with 82%, 92.33%, and 80.66% respectively. |
[63] | Testicular biopsies | VGG16 | Classifies each proposal as either a spermatozoon or background and regresses the bounding box shape to match identified component objects. |
[64] | Sperm image dataset | Enhanced Otsu's threshold method |
It reduces the computation complexity and sobel operator was used for edge reduction, which improve overall performance of segmentation. The abnormal regions were detected by convolution layer. |
[65] | SVIA dataset |
k-means, U-net, SegNet and DeepLabv3 | The effects of U-net and Deeplab_v3 are the best, and other segmentation methods have a certain gap compared with these two segmentation methods |
[71] | VISEM | Crocker-Grier Algorithm With CNN |
The mean absolute error (MAE) could be reduced from 8.83 to 7.31. |
[72] | VISEM | YOLOv4 algorithm | The results obtained were 90.31% AP (Average Precision) for sperm objects and 68.19% AP (Average Precision) for non-sperm objects, then for the results of the training obtained by the model 79.58% mAP (Mean Average Precision). |
[73] | VISEM | YOLOv5 algorithm | YOLOv5 deep learning model trained on the VISEM-Tracking dataset present baseline sperm detection performances. This dataset can be used to train complex deep learning models to analyze spermatozoa. |
[74] | VISEM | YOLOv5 algorithm | The best-performing model is yolov5l. This network achieves a precision of 88.6 per cent, recall of 52.6, and mAP is 72.1. Other networks are achieving lower results. The second-best network is nano, with a mAP of 69.6 and precision of 64.7 per cent |
Ref | Dataset name | Method of Segmentation | Network | Evaluation metrics |
[48] | Convolutional Neural Network (CNN) | 3-conv-full-up-inc | Precision 93.87% Recall 91.89% |
|
[68] | Sperm Morphology Image Data Set (SMIDS) | K-Means clustering Fuzzy C-Means clustering |
Mobile-Net | Precision 83%, recall 82%, F-measure 83% and accuracy 88%. |
[60] | Video dataset | Local Region Contour segmentation | CNN network | Precision 97.37% |
[61] | SCIAN-SpermSegGS | U-Net and Mask- RCNN |
U-Net and MaskRCNN | 95% overlapping efficiency against hand-segmented masks for sperm head 0.96, acrosome 0.94 and nucleus 0.95. |
[69] | VISEM | Region Based Convolutional Neural Networks (R-CNN) architecture |
R-CNN | Accuracy 91.77 %. |
[62] | VISEM | Mean squared displacement of each particle (imsd) |
CNN RNN |
4.4% improvement against state-of-the-art results. |
[70] | MHSMA | Deep Transfer Learning (DTL) technique and Deep Multi-task Transfer Learning (DMTL) |
VGG19 | DTL technique accurately label head, vacuole, and acrosome with accuracy of 84%, 94%, and 79% respectively. Similarly, DMTL technique accurately label head, vacuole, and acrosome with 82%, 92.33%, and 80.66% respectively. |
[63] | Testicular biopsies | VGG16 | MobileNetV2 | mean average precision mAp 0.741 Average recall AR 0.376. |
[64] | Sperm image dataset | Enhanced Otsu's threshold method |
Deep CNN | Accuracy 98.99% |
[65] | SVIA dataset |
k-means, U-net, SegNet and DeepLabv3 | Deep CNN | The effects of U-net and Deeplab_v3 are the best, and other segmentation methods have a certain gap compared with these two segmentation methods |
[71] | VISEM | Crocker-Grier Algorithm With CNN |
RNN | The mean absolute error (MAE) could be reduced from 8.83 to 7.31. |
[72] | VISEM | YOLOv4 algorithm | YOLO Network | The results obtained were 90.31% AP (Average Precision) for sperm objects and 68.19% AP (Average Precision) for non-sperm objects, then for the results of the training obtained by the model 79.58% mAP (Mean Average Precision). |
Model | Accuarcy | Preceision | Recall | F1-score |
SDNN | 89.00 | 96.18 | 91.63 | 95.23 |
VGGI16 | 70.67 | 84.15 | 72.30 | 77.78 |
VGG19 | 71.00 | 83.87 | 73.24 | 78.20 |
ResNet.50 | 71.00 | 84.24 | 72.77 | 78.09 |
Inception V3 | 61.00 | 78.57 | 61.97 | 69.29 |
InceptipnResNet | 70.33 | 84.44 | 71.36 | 77.35 |
MobileNet | 71.00 | 85.00 | 71.83 | 77.86 |
MobileNetV2 | 69.00 | 81.58 | 72.77 | 76.92 |
DenseNaet | 66.00 | 81.71 | 67.14 | 73.71 |
NASNetMobile | 71.00 | 83.51 | 73.71 | 78.30 |
NASNetLarge | 63.00 | 80.36 | 63.38 | 70.87 |
Xception | 55.00 | 73.78 | 56.81 | 64.19 |
Model | Accuarcy | Preceision | Recall | F1-score |
SDNN | 92.00 | 97.70 | 93.43 | 95.52 |
VGGI16 | 87.33 | 96.28 | 88.93 | 92.46 |
VGG19 | 87.33 | 95.90 | 89.31 | 92.49 |
ResNet.50 | 87.33 | 95.53 | 89.69 | 92.52 |
Inception V3 | 82.67 | 94.49 | 85.11 | 89.56 |
InceptipnResNet | 84.33 | 95.36 | 86.26 | 90.58 |
MobileNet | 77.67 | 94.12 | 79.39 | 86.13 |
MobileNetV2 | 86.67 | 95.87 | 88.55 | 92.06 |
DenseNaet | 85.67 | 95.44 | 87.79 | 91.45 |
NASNetMobile | 86.00 | 95.45 | 88.17 | 91.67 |
NASNetLarge | 28.00 | 73.47 | 27.48 | 40.00 |
Xception | 48.00 | 86.30 | 48.09 | 61.76 |
Model | Accuarcy | Preceision | Recall | F1-score |
SDNN | 90.00 | 96.94 | 92.02 | 95.92 |
VGGI16 | 73.00 | 86.70 | 74.43 | 80.10 |
VGG19 | 73.33 | 87.17 | 74.43 | 80.30 |
ResNet.50 | 73.67 | 87.23 | 74.89 | 80.59 |
Inception V3 | 70.00 | 84.49 | 72.15 | 77.83 |
InceptipnResNet | 73.00 | 87.10 | 73.97 | 80.00 |
MobileNet | 73.00 | 86.32 | 74.89 | 80.20 |
MobileNetV2 | 62.67 | 81.29 | 63.47 | 71.28 |
DenseNaet | 72.33 | 86.56 | 73.52 | 79.51 |
NASNetMobile | 71.67 | 86.02 | 73.06 | 79.01 |
NASNetLarge | 69.67 | 84.41 | 71.69 | 77.53 |
Xception | 66.00 | 81.97 | 68.49 | 74.63 |
Label | Method | Accuracy | Precision | Recall | f0.5 score |
Acrosome | |||||
SDNN | 89.00 | 96.18 | 91.63 | 95.23 | |
GeNAS | 77.66 | 84.76 | 83.56 | 84.42 | |
Random Search | 69.66 | 74.5 | 86.8 | 76.67 | |
Javadi et al. | 76.67 | 85.93 | 80.02 | 84.74 | |
Ghasemian et al. | N/A | N/A | N/A | N/A | |
Head | SDNN | 90.00 | 96.94 | 92.02 | 95.92 |
GeNAS | 77.33 | 84.47 | 84.47 | 84.47 | |
Random Search | 76.00 | 80.49 | 88.58 | 81.98 | |
Javadi et al. | 77.00 | 83.48 | 85.93 | 83.86 | |
Ghasemian et al. | 61.00 | 76.71 | 71.79 | 75.68 | |
Vacuole | SDNN | 92.00 | 97.70 | 93.43 | 96.82 |
GeNAS | 91.66 | 95.40 | 95.03 | 95.32 | |
Random Search | 89.00 | 94.20 | 93.12 | 93.98 | |
Javadi et al. | 91.33 | 94.36 | 95.80 | 94.65 | |
Ghasemian et al. | 80.33 | 83.21 | 93.56 | 85.09 |
Ref | Dataset name | Method of classification | Outcomes |
[35] | HSMA-DS | SMA algorithm | SMA algorithm was used for classification and results shows above 90% of accuracy for sperm abnormality detection. It also shows high rates of True positive and True negative |
[39] | - | Particle Swarm Optimization (PSO) algorithm |
PSO gives the highest accuracy then both MLP and SVM methods, PSO obtains superior specificity values at 88 %. Therefore, PSO seems to be the preferred method for predicting the quality of fertility data with respect to the environmental factors and lifestyle, this method seems to be useful with the new data also as it can handle generalization potential |
[47] | Linear Discriminant Analysis (LDA) | LDA was used for classification. To do so LDA minimize variance within class and maximize between classes, as a result maximum separation between classes was achieved. | |
[49] | Fertility Dataset | Artificial Neural Networks (ANN) Sperm Whale Optimization algorithm (SWA) |
ANN-SWA was based on four phases. First one was trained by fertility diseases. In second multilayer perceptron (MLP) was employed to get maximum fitness function. In third phase parameter were optimized like biases and weights to get rapid conjunction with high accuracy. In final step ANN-SWA was employed to get sperm fertility prediction. |
[51] | 3D+t | Algorithm based on one-class classification | Sperms were assigned by an ID for the sake of tracking. Proposed algorithm extract similar traces as compared with ground truth. Results shows that this algorithm is more accurate and robust than multi-branch algorithms |
[17] | Video dataset | CNN | This CNN contain 5-layer network with 3 convolution layers with 16, 32 and 64 3 × 3 filters respectively. These were followed by 2 fully connected layers with 256 neurons in each layer and finally two node soft max layer that classify input into sperm and non-sperm |
[33] | VISEM | CNN | CNN was trained that learned temporal and spatial combined features of sperm motility. That was based on image preparation and representation of single or stacking frame. Then participant data and output of CNN model was combined. Before performing multivariate prediction on the three motility variables, this combined vector was passed through two fully-connected layers. |
[53] | In-House dataset | VGG16 (CNN) | This deep learning model will help clinicians for the microscopy-based sperm selection, it predicts fast DNA quality with in 10ms per cell and up to 86 % successful sperm selection for fertility analysis. |
[54] | Gold-standard dataset | SVM classifier | Multi-channel image generation and SFFS algorithm was optimized with SVM classifier to compete with other classifiers. As a result, axial filament pixels show improved performance and accurate classification. |
[31] | MHSMA | Deep CNN | CNN was used for classification of sperm image dataset into normal and abnormal sperms with the help of multiple morphological characteristics. CNN achieved F 0.5 score of 94.65%, 83.86% and 84.74% in vacuole, head and acrosome abnormality revealing, respectively. |
[32] | HuSHeM and SCIAN | Deep Neural Network architecture | This Deep Neural Network architecture gave high accuracy in head-to-head comparison against other famous methods with identical dataset. Result shows better true positive rate 94.1% vs 92.3% on HuSHeM dataset, over cascade ensemble of SVM (CE-SVM). |
[56] | Video dataset | CSR-DCF tracker algorithm | CSR-DCF algorithm is a robust multi-sperm tracker that works accurately, even when sperms collide or cross each other pass. This technique Obtained 96.61% F1 score from evaluation. |
[57] | Gradient-Boosted Trees (GBT) and random forest, Logistic regression | GBT technique produced improved prediction results of successful and unsuccessful TESE against MvLRM, with little bit lower sensitivity. This model efficiently deals with missing values of several variables by automatically self-learning based on training loss reduction. | |
[58] | Full spectrum neural network (FSNN) Selected peak neural network (SPNN) |
To achieve low root mean square error these models were boosted for transfer function, structure of hidden layer and different combination of training techniques. FSNN shows 93% prediction accuracy for sperm concentration and gave 100% assessments for differentiating the samples of patients and healthy donors. | |
[68] | Sperm Morphology Image Data Set (SMIDS) | InceptionV3, VGG19 and MobileNet | Results shows that SVM model with RBF kernel achieved highest accuracy that is 83.82% and tested by KAZE descriptor-based features. On the other hand, k-NN over DWT-based features shows worst classifier result with 47.23% efficiency. In terms of sperm morphology representation problem, KAZE features were the most informative features for RusBoost, Polynomial, Bagging and RBF kernel SVM classifiers |
[59] | HuSHeM and SCIAN | Morphological Classification of Human Sperm Heads MC-HSH |
In this deep learning architecture, there are a total of 53 convolutional layers. Before each convolutional layer, the batch normalization and LeakyReLU were used. They also apply the channel-wise concatenation and element-wise addition to make this model more efficient classification of human sperm heads in terms of morphology. |
[60] | Video dataset | Elliptic Scanning Algorithm (ESA) Novel Faster Region Convolutional Neural Network (FRCNN), |
A novel FRCNN with ESA detection algorithm was introduced classification and analysis of human sperm. Results shows accuracy of 97.37%. Moreover, for tracking and motility analysis a Tail head movement-based (THMA) algorithm was used. |
[8] | VISEM | ResNet18 model | The first ResNet18 model reached 100% accuracy on both validation and test sets. The second ResNet18 + Tabular Data model correctly predicted with an accuracy of 100% on the validation set and an average accuracy 88.89 on the test set. The third ResNet34 + Tabular Data model correctly predicted with an accuracy 87.5% on the validation set and 77.78% on the test set. |
[62] | VISEM | Linear Support vector Regressor, MultiLayer, perceptron, CNN, RNN |
This technique shows best results for motility prediction and state-of-the-art. The lower the Mean Absolute Error (MAE) the better, showing that SVR models achieve better results than the other models for every type of features. BoWs outperform models trained on emsd features. Considering the best validation result, a minimum MAE of 8.60 is obtained on evaluation with the SVR trained on emsd features. Measured against the state-of-the-art, this result shows a relative improvement of 2.6% |
[70] | MHSMA | Deep Transfer Learning (DTL) technique Deep Multi-task Transfer Learning (DMTL) |
The proposed algorithm achieved accuracy of vacuole, acrosome and head by 94%, 80.66% and 84% respectively by gaining improvement of 1.33%, 3% and 6.66% on these labels respectively. Therefore, this algorithm can be used in fertility institutes and make work easier for hospital staff. |
[63] | Testicular biopsies | Convolutional layers of VGG16, GoogleNet | Deep learning CASA system achieved a mean average precision (MAP) of 0.741, with an average recall (AR) of 0.376 on given dataset. |
[64] | Sperm image dataset | Deep Convolutional Neural Network | MATLAB 2018a was employed to perform overall procedure. Classification process was improved with Deep CNN statistical training and morphology of sperm images. After comparison results shoes efficient performance of proposed technique by providing neck and tail accuracy 97.99%, head utilize 98.22% and vacuole achieve 99.66 accuracy. |
[65] | SVIA dataset |
AlexNet, VGG, ResNet, GoogleNet, DenseNet, Inception-v3, Mobilenet-v2, ShuffleNet-v2, Xception, Vision Transformer ViT, BotNet, DeiT, T2T-ViT. | Inception-v3 and DenseNet-121 shows maximum accuracy 98.32 % and 98.06 % respectively. While ViT shows lowest result i.e 93 % |
[76] | MHSMA | VGG19 and ResNet50 | VGG19 and ResNet50 shows best accuracy of 87.33%, 71% and 73% for vacuole, acrosome, and head label, respectively. |
[77] | MHSMA | Genetic Neural Architecture Search | This Neural Architecture method shows accuracy of 91.66%, 77.66%, and 77.33% in the vacuole, acrosome, and head detection, respectively |
[79] | In-House dataset | VGG16 (CNN) | The VGG16 model is fine-tuned and achieved an accuracy of 97.92% and a sensitivity of 98.82%. Moreover, it achieved an F1 score of 98.53%. |
[78] | MHSMA | Sequential Deep Neural Network architecture (SDNN) | Results show that Sequential Deep Neural Network architecture successfully detected abnormalities in the acrosome, head, and vacuole with an accuracy of 89%, 90%, and 92%, respectively. Moreover, it also out class all state-of-the-art model as well as GeNAS algorithm proposed by Miahi et al. |
Ref | Dataset name | Method of Classification | Network | Evaluation metrics |
[56] | Video dataset | CSR-DCF tracker algorithm | RetinaNet | Precision 99.1 % F1 score 96.61% |
[57] | Machine-learning model |
Gradient-Boosted Trees (GBT) and random forest, Logistic regression | GBT sensitivity 91% MvLRM sensitivity 97% |
|
[58] | ANN | Full spectrum neural network (FSNN) Selected peak neural network (SPNN) |
Accuracy 93% | |
[80] | Sperm Morphology Image Data Set (SMIDS) | Wavelet Descriptor-based features with SVM |
MobileNet | Accuracy 80.5% and 83.8% |
[60] | Video dataset | Elliptic Scanning Algorithm (ESA) Novel Faster Region Convolutional Neural Network (FRCNN), |
FRCNN | Accuracy 97.37%. |
[8] | VISEM | CNN | ResNet18 ResNet34 |
Accuracy 88.89% Accuracy 77.78% |
[62] | VISEM | Four Machine-learning models |
SVR, MLP, CNN, RNN |
Mean Absolute Error 7.31 |
[70] | MHSMA | Deep CNN | Deep Transfer Learning (DTL) technique Deep Multi-task Transfer Learning (DMTL) |
DTL technique accurately label head, vacuole, and acrosome with accuracy of 84%, 94%, and 79% respectively. Similarly, DMTL technique accurately label head, vacuole, and acrosome with 82%, 92.33%, and 80.66% respectively. |
[63] | Testicular biopsies | CNN | VGG GoogleNet 16 |
mean average precision (mAP) of 0.741 average recall (AR) of 0.376 |
[64] | Sperm image dataset | CNN | Deep CNN | Neck and tail accuracy 97.99%, head utilize 98.22% and vacuole achieve 99.66% accuracy |
[65] | SVIA dataset |
18 Deep Learning models | AlexNet, VGG, ResNet, GoogleNet, DenseNet, Inception-v3, Mobilenet-v2, ShuffleNet-v2, Xception, Vision Transformer ViT, BotNet, DeiT, T2T-ViT. | Inception-v3 and DenseNet-121 shows maximum accuracy 98.32 % and 98.06 % respectively. While ViT shows lowest result i.e 93 % |
[76] | MHSMA | Deep CNN | VGG19 and ResNet50 | VGG19 and ResNet50 shows best accuracy of 87.33%, 71% and 73% for vacuole, acrosome, and head label, respectively. |
[77] | MHSMA | CNN | Genetic Neural Architecture Search | This Neural Architecture method shows accuracy of 91.66%, 77.66%, and 77.33% in the vacuole, acrosome, and head detection, respectively |
[78] | MHSMA | Deep CNN | Sequential Deep Neural Network architecture (SDNN) | Results show that Sequential Deep Neural Network architecture successfully detected abnormalities in the acrosome, head, and vacuole with an accuracy of 89%, 90%, and 92%, respectively. |
Ref | Dataset name | Evaluation metrics | Outcomes |
[36] | Automated Sperm Morphology Analyzer (ASMA) |
Accuracy (A c) 96.829% Sensitivity (S c) 97.522% Specificity (S p) 95.275% |
|
[35] | HSMA-DS | Confusion metrics | SMA algorithm shows its performance in less than 9 seconds. True negative rate was 100% for tail malformation detection. However, there was small number of False negative due to high rate of noise in the image. |
[82] | Video dataset | Confusion metrics | Accuracy 0.97, sensitivity 0.97, specificity 0.71 and precision 0.99 |
[39] | - | confusion metrics, 12 parameters used | The presented methods and their improvements can at least replace the initial tests for checking the fertility of a population or an individual as well as in selecting the donors for data collection. Stating and testing environmental and lifestyle factors trough the mentioned techniques are able to give an accuracy of up-to 90%, these results support the argument. |
[41] | Video dataset | Intersecting cortical model (ICM) | Results shows that this model is more robust and accurate than four state of the art segmentation models, in terms of accuracy, sensitivity, specificity and precision with success rate of 98.14%, 98.82%, 86.46% and 99.81% respectively. |
[42] | Gold-standard | Dice Coefficient | Dice coefficient was used to evaluate segmentation performance. Dice coefficient was 3% more than Chang method. Total performance was 88%. |
[45] | SCIAN-MorphoSpermGS | Two-stage classification | Results shows 73% efficiency as well as human experts were fully satisfied with the classification of sperm heads. |
[47] | Confusion metrics | This method achieved average F-score of 92.9 %, average recall of 92.3 %, average precision of 93.5%, and average accuracy of 92.2%. This method shows very impressive result in abnormalities recognizing for all above mentioned four classes. | |
[44] | Confusion metrics | The average accuracy overall classes reached the 78% by using confusion metrics measurements. | |
[49] | Fertility Dataset | 10-fold cross validation | 99.96% of the accuracy. |
[52] | Gold-standard dataset | Dice, Jaccard, and F1-score metrics | The CNN was validated with 20 images of sperm sample. Results shows 0.94, 0.87, and 0.88 of Dice Similarity Coefficient for the head, the acrosome, and the nucleus segments, respectively |
[17] | Video dataset | SQA-V Gold | SQA-V Gold obtain a B0 value of 1.91 and confidence interval of (−12.6 to −0.57) and B1 value of 1.665 with 95% confidence interval of (1.5 to 1.8) |
[31] | MHSMA | Confusion metrics | The proposed technique attains F0.5 Score of 83.86%, 84.74% and 94.65% in head, acrosome and vacuole abnormality detection, respectively. |
[56] | Video dataset | Average precision (AP) metric | Precision 99.1 % and obtained 96.61% F1 score from evaluation of their proposed tracker method. |
[58] | 13-fold cross validation | 13-fold cross validation shows average classification accuracy of 92% was achieved by the system | |
[68] | Sperm Morphology Image Data Set (SMIDS) | K-Fold Cross Validation, with K=5 |
Precision 83%, recall 82%, f-measure 83% and accuracy 88%. |
[60] | Video dataset | Confusion metrics | The Faster Region Convolutional Neural Network (FRCNN) was used for classification and improve the efficiency of computer assisted semen analysis (CASA). Other methods that were used in literature measured with different densities of fluid. But in this study precision will not drop below 91%, even density was increased. So overall sperm detection process will be improved. |
[69] | VISEM | Region Based Convolutional Neural Networks (R-CNN) |
The motility of the semen sample calculated by the algorithm differs from the experimental result by only 2.92% on average, while the accuracy of sperm head detection was 91.77%. |
[61] | SCIAN-SpermSegGS | cross validation, Dice coefficient | Results show that U-net with transfer learning, outclass state of the art sperm segmentation method with 95% overlapping efficiency against hand-segmented masks for sperm head 0.96, acrosome 0.94 and nucleus 0.95. |
[65] | SVIA dataset |
Confusion metrics | Inception-v3 and DenseNet-121 shows maximum accuracy 98.32 % and 98.06 % respectively. While ViT shows lowest result i.e 93 % |
[71] | VISEM | 3-fold cross-validation | The mean absolute error (MAE) could be reduced from 8.83 to 7.31. |
[72] | VISEM | Confusion metrics | The results obtained were 90.31% AP (Average Precision) for sperm objects and 68.19% AP (Average Precision) for non-sperm objects, then for the results of the training obtained by the model 79.58% mAP (Mean Average Precision). |
[77] | MHSMA | Confusion metrics | This Neural Architecture method shows accuracy of 91.66%, 77.66%, and 77.33% in the vacuole, acrosome, and head detection, respectively |
[75] | MHSMA | Confusion metrics | VGG19 and ResNet50 shows best accuracy of 87.33%, 71% and 73% for vacuole, acrosome, and head label, respectively. |
[79] | In-House dataset | Confusion metrics | The VGG16 model is fine-tuned and achieved an accuracy of 97.92% and a sensitivity of 98.82%, precision equals 98.25%, specificity equals 95.71% and F1 Score equals 98.53% Moreover, it achieved an F1 score of 98.53%. |
[75] | VISEM | Different evaluation metrics | YOLOv5 deep learning model trained on the VISEM-Tracking dataset present baseline sperm detection performances. This dataset can be used to train complex deep learning models to analyze spermatozoa. |
[74] | VISEM | detailed quantitative investigation | The best-performing model is yolov5l. This network achieves a precision of 88.6 per cent, recall of 52.6, and mAP is 72.1. Other networks are achieving lower results. The second-best network is nano, with a mAP of 69.6 and precision of 64.7 per cent |
[78] | MHSMA | Confusion metrics | Results show that Sequential Deep Neural Network architecture successfully detected abnormalities in the acrosome, head, and vacuole with an accuracy of 89%, 90%, and 92%, respectively. |