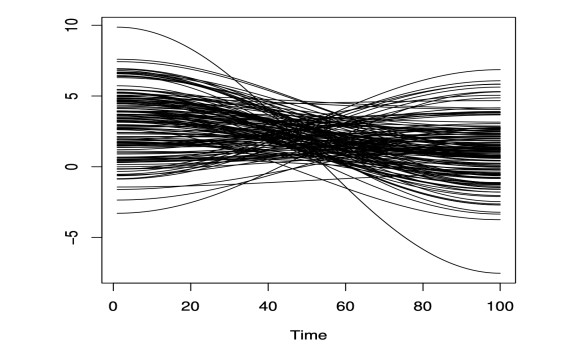
In the present study, we address the nonparametric estimation challenge related to the regression function within the Single Functional Index Model in the random censoring framework. The principal achievement of this investigation lies in the establishment of the asymptotic characteristics of the estimator, including rates of almost complete convergence. Moreover, we establish the asymptotic normality of the constructed estimator under mild conditions. Subsequently, we provide the application of our findings towards the construction of confidence intervals. Lastly, we illuminate the finite-sample performance of both the model and the estimation methodology through the analysis of simulated data and a real-world data example.
Citation: Said Attaoui, Billal Bentata, Salim Bouzebda, Ali Laksaci. The strong consistency and asymptotic normality of the kernel estimator type in functional single index model in presence of censored data[J]. AIMS Mathematics, 2024, 9(3): 7340-7371. doi: 10.3934/math.2024356
[1] | Fatimah Alshahrani, Wahiba Bouabsa, Ibrahim M. Almanjahie, Mohammed Kadi Attouch . Robust kernel regression function with uncertain scale parameter for high dimensional ergodic data using $ k $-nearest neighbor estimation. AIMS Mathematics, 2023, 8(6): 13000-13023. doi: 10.3934/math.2023655 |
[2] | Yue Li, Yunyan Wang . Strong consistency of the nonparametric kernel estimator of the transition density for the second-order diffusion process. AIMS Mathematics, 2024, 9(7): 19015-19030. doi: 10.3934/math.2024925 |
[3] | Breix Michael Agua, Salim Bouzebda . Single index regression for locally stationary functional time series. AIMS Mathematics, 2024, 9(12): 36202-36258. doi: 10.3934/math.20241719 |
[4] | Zouaoui Chikr Elmezouar, Fatimah Alshahrani, Ibrahim M. Almanjahie, Salim Bouzebda, Zoulikha Kaid, Ali Laksaci . Strong consistency rate in functional single index expectile model for spatial data. AIMS Mathematics, 2024, 9(3): 5550-5581. doi: 10.3934/math.2024269 |
[5] | Salim Bouzebda, Amel Nezzal, Issam Elhattab . Limit theorems for nonparametric conditional U-statistics smoothed by asymmetric kernels. AIMS Mathematics, 2024, 9(9): 26195-26282. doi: 10.3934/math.20241280 |
[6] | Sangwan Kim, Yongku Kim, Jung-In Seo . Nonparametric Bayesian modeling for non-normal data through a transformation. AIMS Mathematics, 2024, 9(7): 18103-18116. doi: 10.3934/math.2024883 |
[7] | Oussama Bouanani, Salim Bouzebda . Limit theorems for local polynomial estimation of regression for functional dependent data. AIMS Mathematics, 2024, 9(9): 23651-23691. doi: 10.3934/math.20241150 |
[8] | Xueping Hu, Jingya Wang . A Berry-Ess$\acute{e}$n bound of wavelet estimation for a nonparametric regression model under linear process errors based on LNQD sequence. AIMS Mathematics, 2020, 5(6): 6985-6995. doi: 10.3934/math.2020448 |
[9] | Salim Bouzebda, Amel Nezzal . Uniform in number of neighbors consistency and weak convergence of $ k $NN empirical conditional processes and $ k $NN conditional $ U $-processes involving functional mixing data. AIMS Mathematics, 2024, 9(2): 4427-4550. doi: 10.3934/math.2024218 |
[10] | Fatimah A Almulhim, Torkia Merouan, Mohammed B. Alamari, Boubaker Mechab . Local linear estimation for the censored functional regression. AIMS Mathematics, 2024, 9(6): 13980-13997. doi: 10.3934/math.2024679 |
In the present study, we address the nonparametric estimation challenge related to the regression function within the Single Functional Index Model in the random censoring framework. The principal achievement of this investigation lies in the establishment of the asymptotic characteristics of the estimator, including rates of almost complete convergence. Moreover, we establish the asymptotic normality of the constructed estimator under mild conditions. Subsequently, we provide the application of our findings towards the construction of confidence intervals. Lastly, we illuminate the finite-sample performance of both the model and the estimation methodology through the analysis of simulated data and a real-world data example.
Regression analysis has proven to be versatile and has offered a strong statistical modeling framework in a wide range of practical and theoretical situations where the goal is to model the predictive relationship between related responses and predictors. Parametric regression models are valuable tools for analysing real-world data, but they can be subject to significant modeling biases if the model structures are poorly described. This is a common issue in many practical problems. Nonparametric smoothing techniques can be used as an option to address concerns about modeling bias. This article will specifically examine the analysis of estimators that belong to the kernel type. For x∈Rd, the regression function, whenever it exists, is defined to be
r(x):=E(Y∣X=x). | (1.1) |
A well-known estimator for the regression function r(⋅), often used in nonparametric statistics, is the kernel regression function estimator. This estimator is, under suitable conditions, strongly consistent, i.e., it converges almost surely to the unknown regression function at x. Because of numerous applications and their important role in mathematical statistics, the problem of estimating m(⋅,φ) and fX(⋅) has been a subject of considerable interest during recent decades. For good sources of references to the research literature in this area along with statistical applications, consult [11,14,19,20,24,25,26,60,65,67,71] and the references therein. The increased dimensionality of X can provide challenges when attempting to move beyond standard multiple linear models and pursue nonparametric estimation of r(x). This strongly motivates the consideration of regression models that offer dimension reduction. Single index models are widely used to achieve this by assuming that the predictors' influence on the response can be simplified to a single index. This index represents a projection on a specified direction and is combined with a nonparametric link function. This simplifies the predictors to a single-variable index while still including important characteristics. Additionally, because the nonparametric link function only operates on a one-dimensional index, these models are not affected by the problem of having a high number of dimensions, known as the curse of dimensionality. The single index model extends the concept of linear regression by incorporating a link function that is equivalent to the identity function; the interested reader may refer to [6,35,41,50,69]. The statistical challenges associated with the analysis of functional random variables, which are variables that take values in an infinite-dimensional space, have gained increasing attention in the statistics literature in recent decades. The motivation behind the development of this research issue stems from the abundant data collected on a progressively more precise temporal/spatial grids. This is evident in fields such as meteorology, medicine, satellite images, and various other research domains. Consequently, the statistical analysis of this data, viewed as unpredictable functions, gave rise to numerous complex theoretical and computational research inquiries. To gain a comprehensive understanding of both the theoretical and practical aspects of functional data analysis, readers are encouraged to consult the monographs by [8] for linear models involving random variables in a Hilbert space, and by [64] for scalar-on-function and function-on-function linear models, functional principal component analysis, and parametric discriminant analysis. The work of [28] primarily emphasises nonparametric techniques, particularly kernel-based estimation, for scalar-on-function nonlinear regression models. They expanded the application of these technologies to include classification and discrimination analysis. The book by [42] explores the extension of many statistical concepts, including goodness-of-fit tests, portmanteau tests, and change detection, to the framework of functional data. Recent advances in functional data analysis have highlighted the necessity of developing models aiming to reduce dimensionality effects (see [22,34,51] recent surveys), see also [2,12,13], and semiparametric ideas are natural candidates for that purpose. In that way, [29] and [1] studied the Functional Single-Index Model (FSIM). [43] proposed functional single index composite quantile regression and estimated the unknown slope function and link function by using B-spline basis functions. [61] proposed a new compact functional single index model, in which the coefficient function is only nonzero in a subregion. [76] investigated the estimation of a general functional single index model, in which the conditional distribution of the response depends on the functional predictor via a functional single index structure. [70] developed a new estimation procedure that combines a functional principal component analysis of the functional predictors, B-spline model for the parameters, and profile estimation of the unknown parameters and functions in the model. [53,54] investigated the estimation of the functional single index regression model with missing responses at random for strong mixing time series data. [27] introduced a new functional single-index varying coefficient model with the functional predictor being single-index part. By means of functional principal components analysis and basis function approximation, they obtain the estimators of slope function and coefficient functions, and propose an iterative estimating procedure. [62] developed a new automatic and location-adaptive procedure for estimating regression in FSIM that is based on k-Nearest Neighbours (kNN) ideas. Motivated by the analysis of imaging data, [47] proposed a novel functional varying-coefficient single-index model to carry out the regression analysis of functional response data on a set of covariates of interest. [4] investigated a functional Hilbertian regressor for a nonparametric estimation of the conditional cumulative distribution with a scalar response variable in the single index structure. [21] developed an alternative approach, where their methodology includes the multi-index case and does not anchor the true parameter on a prespecified sieve, and they provide a detailed theoretical analysis of a direct kernel-based estimation scheme which establishes a polynomial convergence rate. [3] considered the nonparametric estimation of the conditional density of a scalar response variable given a random variable taking values in separable Hilbert space in the single-index structure. [52] investigated the estimation of conditional density function based on the single-index model for functional time series data. [49] proposed a model that accommodates the existence of interaction between a single functional predictor and other covariates, which has usually been ignored in previous works. For that, the authors consider a semiparametric single-index structure to model the potential interaction between the functional predictor and other covariates, which provides a feasible two-stage procedure to address estimation and inference issues. To explore recent progress and relevant sources in semiparametric and/or functional data analysis, we refer to [39,40,46,58,75] which considered the kNN method in a single index regression model when the explanatory variable is valued in functional space in the setting of the quasi-association dependence condition. It should be noted that most of the contributions involved above are in the case of the samples being observed completely. Therefore, it is imperative to thoroughly examine the functioning statistical models within the context of censored data. From a pragmatic perspective, this type of data holds significance. Clinical trials commonly involve survival statistics or failure time data, which are frequently affected by censoring. More precisely, numerous statistical experiments provide incomplete samples, even when conducted under carefully regulated circumstances. For instance, clinical data pertaining to the survival of various diseases is typically obscured by other life-threatening dangers that ultimately lead to mortality. To be more precise, let (Y0i)1≤i≤n be the life (or survival) times supposed independent identically distributed (i.i.d.) sequence of random variables (r.v.s) with common unknown continuous distribution function (d.f.) F(⋅) and density f(⋅). In many practical situations, instead observing the lifetimes Y0 we observe only censored lifetimes of the items. Further, we assume that (Ci)1≤i≤n is an i.i.d. sequence of censoring r.v.s with common continuous d.f. G(⋅) which is independent of (Y0i)1≤i≤n, and we observe the n pairs (Yi,δi)1≤i≤n with Yi=min(Y0i,Ci)=:Y0i∧Ci and δi=1{Y0i≤Ci}, where 1A is the indicator function of the set A (if δi=1, this means that Y0i≤Ci and Yi=Y0i, and the observed value is the true lifetime of subject i; otherwise, Ci<Y0i and hence Yi=Ci and so the actual lifetime Y0i is not observed). Following the convention usually assumed, in right censored model, we suppose that (Ci)1≤i≤n and (Xi,Y0i)1≤i≤n are independent, for instance, see [14,15,45,63].
The primary objective of this paper is to delineate the asymptotic properties of the estimator ˆrn(θ,⋅), defined in (2.7) below, in a censorship setting where the variables exhibit a functional form and adhere to a single index structure. This includes investigating the rates of almost complete convergence (a.co.*). Additionally, we derive the asymptotic distribution under certain mild conditions.
*Let (zn) for n∈N, be a sequence of real r.v.'s. We say that (zn) converges almost-completely (a.co.) toward zero if, and only if, for all
ϵ>0,∞∑n=1P(|zn|>ϵ)<∞. |
Moreover, we say that the rate of the almost-complete convergence of (zn) toward zero is of order un (with un→0) and we write zn=Oa.co.(un) if, and only if, there exists ϵ>0 such that
∞∑n=1P(|zn|>ϵun)<∞. |
This kind of convergence implies both the almost-sure convergence and the convergence in probability.
The rest of this work is organized as follows: In Section 2, we describe the single index regression model for functional data and in the censored framework. In Section 3, we will establish our main results of the uniform almost complete convergence of the kernel estimators (in § 3.1) and the asymptotic normality (in § 3.2) under non-restrictive conditions. In Section 4, we discuss the impact of our contribution in practical application of our results for the construction of the confidence interval. In Section 5, we perform a simulation study to show that our proposed model works well for finite samples of simulated data and a real data example. We conclude this paper with some remarks and future works in Section 6. To avoid interrupting the flow of the presentation, all mathematical developments are relegated to Section 7.
Let {(Xi,Ci,Y0i),1≤i≤n} be n independent identical copies of a triple of random variables (X,C,Y0), where X takes values in a separable real Hilbert space H endowed with the inner product ⟨⋅,⋅⟩ and its norm ‖⋅‖=⟨⋅,⋅⟩1/2, C is a censoring variable and Y0 is the variable of interest, typically called a life time variable. We consider the semi-metric dθ(⋅,⋅),† associated with the single-index θ, which is defined by dθ(χ1,χ2):=|⟨χ1−χ2,θ⟩|. In the given topological structure and for any θ, our focus is to estimate nonparametrically the regression function, whenever it exists, of Y0 given ⟨X,θ⟩, denoted by
†A semi-metric (sometimes called pseudo-metric) dθ(⋅,⋅) is a metric which allows dθ(x1,x2)=0 for some x1≠x2.
rθ(χ):=IE(Y0|⟨θ,X⟩=⟨θ,χ⟩). | (2.1) |
Since the single functional index θ plays a crucial role in such a model, the identifiability of this model will be important. Clearly, the relation in (2.1) can be reformulated as
Y0=r(⟨X,θ⟩)+ϵ, |
where IE(ϵ|X)=0. To ensure the identifiability of the model θ (i.e., θ1=θ2) we require that rj(⟨χ,θj⟩) is differentiable with respect to (w.r.t) the variable ⟨χ,θj⟩,j=1,2, respectively, and that ⟨θ,e1⟩=1, where e1 is the first element of an orthonormal basis of the space H. For more details on the proof of identifiability of the functional single model, see [29]. It is worth highlighting that when censored data is considered, the smoothing nonparametric regression estimator ˆr(θ,χ) of r(θ,χ) can be viewed as a local least squares estimator, that is
ˆr(θ,χ)=argmint∈IRn∑i=1Wi,n(θ,χ)(Yi−t)2, |
where Wi,n(θ,χ) are the Nadaraya-Watson weights given by
Wi,n(θ,χ)=K(h−1n|⟨χ−Xi,θ⟩|)n∑i=1K(h−1n|⟨χ−Xi,θ⟩|). |
The [59,73] type-estimator of r(θ,χ) is defined as
ˆr(θ,χ)=n∑i=1Y0iWi,n(θ,χ)=n∑i=1Y0iK(h−1n|⟨χ−Xi,θ⟩|)n∑i=1K(h−1n|⟨χ−Xi,θ⟩|), | (2.2) |
where K(⋅) is a kernel function, and hn:=hn,K is a sequence of bandwidths (positive real numbers) decreasing to zero as n goes to infinity. When working with censored data, the initial challenge is understanding and handling the censorship itself, which occurs when observations are incomplete or only partially obtained. One of the suggested approaches to tackle censored data is to apply the method of synthetic data, which allows to take into account the censoring effect on the lifetime distribution, see for instance: [16,17,18,45]. Theoretically, the synthetic data transformation provides equal expected values for both variables. In this case, synthetic response variable (transformed variable) is given by δiYi¯G(Yi),1≤i≤n, where ¯G(⋅)=1−G(⋅), and if we assume (Y0i,Xi) and (Ci) are independent (usually supposed for censored data), we get
IE[δiYi¯G(Yi)|Xi]=IE{IE[δiYi¯G(Yi)|Y0i,Xi]|Xi}=IE{IE[1{Y0i≤Ci}Yi¯G(Yi)|Y0i,Xi]|Xi}=IE{Y0i¯G(Y0i)IE[1{Y0i≤Ci}|Y0i]|Xi}=IE[Y0i|Xi]. | (2.3) |
In the same manner, we get by conditioning w.r.t ⟨θ,Xi⟩
IE[δiYi¯G(Yi)|⟨θ,Xi⟩]=IE[Y0i|⟨θ,Xi⟩]. | (2.4) |
Thus, for the infinite dimension case one can define the pseudo-estimator of rθ(χ) as:
˜r(θ,χ)=n∑i=1δiYi¯G(Yi)K(h−1n|⟨χ−Xi,θ⟩|)n∑i=1K(h−1n|⟨χ−Xi,θ⟩|)=˜rN(θ,χ)ˆrD(θ,χ), | (2.5) |
where
˜rN(θ,χ)=1nIE[K1(θ,χ)]n∑i=1δiYi¯G(Yi)Ki(θ,χ)ˆrD(θ,χ)=1nIE[K1(θ,χ)]n∑i=1Ki(θ,χ)Ki(θ,χ)=K(h−1n|⟨χ−Xi,θ⟩|)=K(h−1ndθ(χ,Xi)). |
In practice, since the values Y0 are censored observations, the survival function ¯G(⋅)=1−G(⋅) is unknown (because G(⋅) is too), so it is impossible to use the estimator (2.5). To complete the building of the good estimator of r(θ,χ), we may can replace the function ¯G(⋅) by its corresponding estimator [44], ¯Gn(⋅):=1−Gn(⋅) defined by
¯Gn(t)={n∏i=1(1−1−δ(i)n−i+1)1{Y(i)≤t},if t<Y(n);0,if t≥Y(n). | (2.6) |
Where Y(1)≤Y(2)≤⋯≤Y(n) are the order statistics of Yi and δ(i) is the indicator of no censoring associated with Y(i). [23] presented some probabilistic strong functional limit theorems extending the results known for completely observable data. More precisely, for a continuous G(⋅), as n→∞, we have
sup0≤t≤Y(n)|1Gn(t)−1G(t)|=O((log2nn)12), |
almost surely where log2u=log+(log+u),log+u=log(u∨e). Such a result will be useful to establish the main results of this paper. Now, plugging the estimator (2.6) into (2.5), one can define an estimator of r(θ,χ) as:
ˆrn(θ,χ)=1nIE[K1(θ,χ)]n∑i=1δiYi¯Gn(Yi)K(h−1n|⟨χ−Xi,θ⟩|)1nIE[K1(θ,χ)]n∑i=1K(h−1n|⟨χ−Xi,θ⟩|)=:ˆrN(θ,χ)ˆrD(θ,χ). | (2.7) |
We emphasize that this study represents the first exploration of semi-parametric regression for censored functional data. It is important to note that the model investigated here differs significantly from the conditional quantile examined in [38]. Additionally, the asymptotic findings presented in our paper diverge from those established in the aforementioned study. Specifically, our results not only confirm uniform consistency, but also demonstrate the asymptotic normality of the estimator we construct. Henceforth, let us consider Y0 as having bounded values. While the assumption of boundedness for Y0 can be substituted with a finite moment assumption, such a replacement would introduce significantly greater complexity into the proofs. Specifically, it necessitates the utilization of a truncation argument.
Throughout this paper, when no confusion will be possible, we will denote by C,C′ or/and Cθ,x some strictly positive generic constants which may be different in each appearance. For any sequences (un) and (vn) we write un=O(vn) if |un|≤C|vn|,∀n (if the property holds almost completely we use the symbol Oa.co.). We design by Nχ a fixed neighborhood of a fixed point χ in H, and by
Bθ(χ,r):={χ1∈H:|⟨χ−χ1,θ⟩|<r}, |
the ball centered at χ, with radius r. Let τF=sup{y:¯F(y)>0} and τG=sup{y:¯G(y)>0} be upper endpoints of F(⋅) and G(⋅), respectively. We assume that τF<∞, and ¯G(τF)>0, which implies that τF<τG. Let us consider the following coverings of the compacts SH and ΘH :
SH⊂dSHn⋃k=1B(χk,ln) and ΘH⊂dΘHn⋃j=1B(tj,ln), |
with χk,tj∈H and ∀χ∈H; ∀θ∈ΘH, one sets k(χ)=argmink∈{1,…dSHn}‖χ−χk‖ and j(θ)=argminj∈{1,…dΘHn} ‖θ−tj‖. Note that this cover of the compact subset is necessary to derive our uniform consistency, and it is a key point to ensure the geometric link between the number dSHn,dΘHn of balls and the sequence of radius ln. In abstract semi-metric spaces, it is usually assumed that dSHnln(dΘHnln) is bounded, see [1] for more discussion. We now list the assumptions needed to derive our first result.
(A0) (X,Y0) and C are independent;
(A1) (i) IP(X∈Bθ(χ,ϵ))=:ϕθ,χ(ϵ)>0,and ∀ϵ>0,limϵ→0ϕθ,χ(ϵ)=0,
(ii) There exists a differentiable function ϕ(⋅), ∀χ∈SH,θ∈ΘH,0<Cϕ(hn)≤ϕθ,χ(hn)≤C′ϕ(hn)<∞, and ∃ξ0>0,∀ξ<ξ0,ϕ′(ξ)<C;
(A2) The function r(⋅,⋅) satisfies the following Hölder condition: ∀θ∈H,∀(χ1,χ2)∈Nχ×Nχ:
|r(θ,χ1)−r(θ,χ2)|≤Cθ,χdθ(χ1,χ2)γ,γ>0; |
(A3) K(⋅) is a bounded continuous function such that
(i) 0<C1[0,1](t)<K(t)<C′1[0,1](t)<∞,
(ii) ∀t1,t2∈IR,|K(t1)−K(t2)|≤C|t1−t2|;
(A4) For ln=O(lognn), the sequences dSHn and dΘHn satisfy:
(i) (logn)2nϕ(hn)<logdSHn+logdΘHn<nϕ(hn)logn,
(ii) ∞∑n=1(dSHndΘHn)1−β<∞,for someβ>2,
(iii) nϕ(hn)=O((logn)2) and limn→∞nϕ(hn)=∞.
Comments on the assumptions
We first recall that our assumptions are not restrictive and may be considered as standard in the present context. Assumption (A0) was introduced in [18] and used in [10,36,45] which is plausible whenever the censoring is independent to the characteristics of the patients under study. This condition can be relaxed to conditional independence, but this will add extra complexity to the proofs. Note also that the assumption τF<τG (implying ¯G(Y0)>¯G(τF)) is classical for asymptotic normality results in the censorship framework. Assumption (A1) controls the behavior of the small ball probability around zero and is the usual condition on the small ball probability. Assumption (A2) imposes some smoothness of the regression operator required to control the convergence rate of bias. Assumption (A3) pertains to the choice of the kernel K(⋅), a common practice in nonparametric functional estimation. It is important to observe that the Parzen symmetric kernel is not suitable in this particular situation due to the fact that the random process |⟨χ−Xi,θ⟩| is always positive. As a result, we will instead use K(⋅) with a support range of [0,1]. This is an extension of the often assumed condition on the kernel in the situation of multiple variables, where K(⋅) is expected to be a density function that exhibits spherical symmetry. Assumption (A4) serves a technical purpose, allowing for the evaluation of the asymptotic variance component. This assumption pertains to the Kolmogorov entropy of the functional subsets ΘH and SH. Such assumptions in functional data analysis were originally introduced by [9] and have since found application in functional single index models, as demonstrated by [5] and [38].
In the following theorem, we first present the uniform almost complete convergence rate of the estimator ˆrn(θ,χ).
Theorem 3.1. Assume that (A0)–(A4) hold. We then have, as n→∞,
supχ∈SHsupθ∈ΘH|ˆrn(θ,χ)−r(θ,χ)|=O(hγn)+Oa.co.(√logdSHn+logdΘHnnϕ(hn)). |
In the particular case where the functional single index is fixed we get the following result.
Corollary 3.2. Under the Assumptions (A0)–(A4), we have, as n→∞,
supχ∈SH|ˆrn(θ,χ)−r(θ,χ)|=O(hγn)+Oa.co.(√logdSHnnϕ(hn)). |
Proof of Theorem 3.1. We may consider the following decomposition
ˆrn(θ,χ)−r(θ,χ)=ˆrN(θ,χ)−r(θ,χ)ˆrD(θ,χ)ˆrD(θ,χ)=ˆrN(θ,χ)−˜rN(θ,χ)ˆrD(θ,χ)+˜rN(θ,χ)−IE[˜rN(θ,χ)]ˆrD(θ,χ)+IE[˜rN(θ,χ)]−r(θ,χ)ˆrD(θ,χ)+r(θ,χ)(1−ˆrD(θ,χ))ˆrD(θ,χ). | (3.1) |
Through this decomposition, the proof of Theorem 3.1 will rely on the following lemmas, the proofs of which are deferred to Section 7.
Lemma 3.3. Under the Assumptions (A1), (A3), and (A4), we have, as n→∞,
supχ∈SHsupθ∈ΘH|ˆrD(θ,χ)−1|=Oa.co.(√logdSHn+logdΘHnnϕ(hn)). |
Corollary 3.4. Under the Assumptions (A1), (A3), and (A4), we have, as n→∞,
∞∑n=1IP(infθ∈ΘHinfχ∈H|ˆrD(θ,χ)|<1/2)<∞. | (3.2) |
Lemma 3.5. Under the Assumptions (A0), (A1), (A3), and (A4), we have, as n→∞,
supχ∈SHsupθ∈ΘH|ˆrN(θ,χ)−˜rN(θ,χ)|=Oa.co.(√logdSHn+logdΘHnnϕ(hn)). |
Lemma 3.6. Under the Assumptions (A0), (A1-(i)), and (A2), we have, as n→∞,
supχ∈SHsupθ∈ΘH|IE[˜rN(θ,χ)]−r(θ,χ)|=O(hγn). |
Lemma 3.7. Under the Assumptions (A0), (A1), (A3), and (A4), we have, as n→∞,
supχ∈SHsupθ∈ΘH|˜rN(θ,χ)−IE[˜rN(θ,χ)]|=Oa.co.(√logdSHn+logdΘHnnϕ(hn)). |
Our second result consists of establishing the asymptotic normality of the estimator ˆrn(θ,χ). We fix a point χ in H, and we introduce the following assumptions:
(A1') The concentration property in (A1-(i)) holds. Moreover, there exists a function βθ,χ(⋅) satisfying:
∀s∈[0,1],limn→∞ϕθ,χ(shn)ϕθ,χ(hn)=βθ,χ(s); |
(A3') The kernel K(⋅) is a bounded continuous function with support [0,1], differentiable, its derivative K′(⋅) exists, and is such that there exist two constants C and C′ with −∞<C<K′(t)<C′<0, for all t∈[0,1];
(A4') The bandwidth hn satisfies :
(i) limn→∞hn=0, and limn→∞1nϕθ,χ(hn)=0,
(ii) limn→∞nϕθ,χ(hn)h2γn=0.
Comments on the assumptions.
Again assumption (A1') controls the behavior of the small ball probability around zero and is the usual condition on the small ball probability. This approximately shows that the small ball probability can be written approximately as the product of two independent functions, refer to [56] for the diffusion process, [7] for a Gaussian measure, and [48] for a general Gaussian process, and these assumptions have been employed by [55] for strongly mixing processes. For example, the function ϕ(⋅) can be expressed as ϕ(ϵ)=ϵδexp(−C/ϵa) with δ≥0 and a≥0, and it corresponds to the Ornstein-Uhlenbeck and general diffusion processes (for such processes, a=2 and δ=0) and the fractal processes (for such processes, δ>0 and a=0). We refer to the paper of [30] and for other examples [68]. As was discussed in [55], the assumption (A1') is consistent with the assumptions made by [33] in the context of density estimation for functional data. When H is a separable Hilbert space and is infinite dimensional, ϕ(h) could decrease to zero as h→0 exponentially fast, for instance, see [33]. This condition also permits us to present an explicitly asymptotic variance term. The condition (A3') is essential in the variance calculation as in [55]. The assumption (A4'-ii) will be used to remove the bias term in the asymptotic normality results.
Below, we write ZD→N(μ,σ2) whenever the random variable Z follows a normal law with expectation μ and variance σ2. Our main result of this section is summarized in the following theorem.
Theorem 3.8. Assume that (A0)-(A1'), (A2), and (A3')-(A4') hold. We have, as n→∞,
(nϕθ,χ(hn))1/2(ˆrn(θ,χ)−r(θ,χ))D⟶N(0,σ2r(θ,χ)), |
where
σ2r(θ,χ):=C2(r(θ,χ))2C21withCl=Kl(1)−∫10(Kl)′(s)βθ,χ(s)ds,forl=1,2. |
Proof of Theorem 3.8. Considering the decomposition:
ˆrn(θ,χ)−r(θ,χ)=ˆrN(θ,χ)−r(θ,χ)ˆrD(θ,χ)ˆrD(θ,χ)=ˆrN(θ,χ)−IE[ˆrN(θ,χ)]+IE[ˆrN(θ,χ)]−r(θ,χ)ˆrD(θ,χ)ˆrD(θ,χ). | (3.3) |
Using Eq (3.3), we conclude that the remaining task is to demonstrate the convergence in probability of the numerator to 1, the vanishing of the last term of the denominator, and the asymptotic normality of the first term of the denominator.
Finally, we will need the following lemmas to state Theorem 3.8:
Lemma 3.9. Under Assumptions (A1'), (A3'), (A4'-(i)), we have, as n→∞,
ˆrD(θ,χ)−1→0,in probability. |
Lemma 3.10. Under Assumptions (A0)-(A1'), (A2) and (A3')-(A4'), we have, as n→∞,
(nϕθ,χ(hn))1/2(IE[ˆrN(θ,χ)]−r(θ,χ)ˆrD(θ,χ))→0. |
Lemma 3.11. Under Assumptions (A0)-(A1') and (A3')-(A4'), we have, as n→∞,
(nϕθ,χ(hn))1/2(ˆrN(θ,χ)−IE[ˆrN(θ,χ)])D⟶N(0,σ2r(θ,χ)). |
Remark 3.12. We allow C to be defective, that is, such that P(C=∞) is possibly positive, to cover the uncensored case corresponding to the particular case where P(C=∞)=1. It is to be noted that the uncensored case corresponds to the case G(x)=0 for all x∈R.
Recall that in practical applications, the functional index is typically unknown in practice and needs to be estimated. In this context, two important rules exist for carrying out the estimation. The initial one is the Least Squares Cross-Validation (LSCV) rule defined by
ˆθ=argminθ∈Θ1nn∑i=1(Yi−ˆr−in(θ,Xi))2, | (4.1) |
where ˆr−in(θ,χ) is the leave-one-out estimator of ˆrn(θ,χ). The second one is the Maximum Likelihood Cross-Validation (MLCV) rule expressed by
ˆθ=argminθ∈Θ1nn∑i=1logˆf(Yi∣ˆr−in(θ,Xi)), | (4.2) |
where ˆf(⋅∣⋅) is the estimator of the conditional density of Y given ⟨θ,X⟩. Of course, in practice we must optimize these rule over finite subset Θ of indices. According to [1], we propose to select the optimal index from the following subset
Θ=Θn={θ∈H,θ=k∑i=1ciei,‖θ‖=1,and ∃j∈[1,k]such that ⟨θ,ej⟩>0}, | (4.3) |
where (ei)i=1,…,k are finite basis functions of the Hilbert subspace spanned by the covariates (Xi)i, and (ci)i some real calibrated constants allowing to ensure the model's identifiability. The common way is to choose the (ci)i with calibration from the subset {−1,0,1}. In practice, it is shown that one can estimate any parameter by the cross-validation method. Another alternative, which will be adopted in this section, consists of selecting θ(t) among the eigenfunctions of the covariance operator E[(X′−E(X′))⟨X′,⋅⟩H], where X(t) is, for instance, a diffusion-type process defined on a real interval [a,b] and X′(t) its first derivative see, for instance, [4]. Given a training sample L, the covariance operator can be estimated by its empirical version (1/|L|)∑i∈L(X′i−EX′)t(X′i−EX′). Consequently, one can obtain a discretized version of the eigenfunctions θi(t) by applying the principle component analysis method, as in [37]. Let θ∗ be the first eigenfunction corresponding to the highest eigenvalue of the empirical covariance operator, which will replace θ in the simulation steps to calculate the regression estimator. {Lastly, it should be noted that there are numerous more concepts in vectorial statistics that can be extended to the functional case. We refer to the local linear approach and jump-preserving approaches proposed by [39]. The primary characteristic of this latest version is the concurrent estimate of the link function and the single index through the utilization of distinct scoring functions. Therefore, implementing this latest approach in functional data analysis has great promise in the current study. In addition to this approach, we can also utilize the concepts proposed by [72] to estimate the linear component of this model. We refer back to [74] for other alternative estimating methods in the linear model. Nevertheless, in our work we have concentrated on the estimation of the nonparametric function and have established the uniform consistency over a single index. We point out that the obtained uniform consistency constitutes a mathematical support for the convergence for any estimator of a single index (see, [1]).
As previously mentioned, the asymptotic variance σ2r(θ,χ) given in Theorem 3.8 depends on the functions C1(θ,χ),C2(θ,χ), and r(θ,χ). So, the estimation of σ2r(θ,χ) will be basically obtained by estimating these functions, which are precisely based on the estimation of the functional parameter θ. Let us denote by θ∗ the first eigenfunction corresponding to the first higher eigenvalue, and the estimator ^Cl(θ∗,χ) defined as in [28,p. 44] by
^Cl(θ∗,χ)=1n^ϕθ∗(χ,hn)n∑i=1Kli(θ∗,χ),l=1,2, | (4.4) |
where
^ϕθ∗(χ,hn)=1nn∑i=11{|⟨χ−Xi,θ∗⟩|<hn}. |
By applying the kernel estimator of r(θ∗,χ) given above, the quantity σ2r(θ∗,χ) can be estimated finally by:
ˆσ2r(θ∗,χ)=^C2(θ∗,χ)(ˆr(θ∗,χ))2^C12(θ∗,χ). | (4.5) |
Corollary 4.1. Under the Assumptions of Theorem 3.8, we have
√n^ϕθ∗(χ,hn)ˆσ2r(θ∗,χ)(ˆrn(θ∗,χ)−r(θ∗,χ))→DN(0,1). | (4.6) |
Thus, following Corollary 4.1, we can approximate (1−ζ) confidence interval of r(θ∗,χ) by
ˆrn(θ∗,χ)±tζ/2׈σ(θ∗,χ)√n^ϕθ∗(χ,hn), | (4.7) |
where tζ/2 is the upper ζ/2 quantile of the standard normal variable N(0,1). Using approximated constants directly from the limiting law to generate confidence bands might sometimes result in inadequate convergence qualities. It should be noted that this drawback can be circumvented by employing the functional variant of the conventional wild bootstrap technique. Within the context of censored functional data, the existing methods in the literature for regression estimations on functional data, such as the one proposed by [31], can be regarded in a similar manner. Nevertheless, it is necessary to provide the theoretical justification.
In this section, we show the feasibility and the efficiency of the constructed estimator through a Monte Carlo experiment. Specifically, through this simulated data analysis we aim to:
(1) Examine the easy implementation of the constructed estimator;
(2) Evaluate the effect of the censorship as well as the regularity of the conditional distribution on the performance of the estimator.
For this empirical analysis, we generate a functional random variable by taking
Xi(t)=bicos(πt)+ηi for all t∈(0,1) and for i=1,2,…,n=150, |
where bi is generated according to the normal distribution N(1,2) and ηi is drawn from the standard normal distribution N(2,1). The curves are discretized on a grid generated from 100 equispaced measurements in (0,1).
Next, the scalar response Y is generated by a single index model as follows:
Yi=r(⟨θ,Xi⟩)+ϵi,r(z)=11+z2, for i=1,…,n=150, | (5.1) |
where the errors (ϵi) are assumed to be independent of (Xi). As the conditional distribution of Y given X=x is explicitly given by the law of the error ϵ shifted by r(⟨θ,Xi⟩), we consider four types of errors which are generated from the following normal mixture distributions (cf. [57] for more details on the normal mixture distributions).
Law | Distribution function |
Standard normal distribution (S.N.D.) | N(0,1) |
Skewed bimodal distribution (C.B.D.) | 34N(0,1)+14N(32,19) |
Now, concerning the functional index scalar θ, we put θ=e1 as the first element of the {K}arhunen-{L}oève basis functions. Explicitly, θ is the eigenfunction associated to the first eigenvalue of the covariance operator of the process (Xi)i. Clearly this index is an eligible functionals index because it belongs in the same Hilbert subspace of the functional variable and is an element of Θn (see the previous section). In practice, if this index is unknown, then we estimated it by using the rule (4.1 for J=5. The second feature of our study concerns the censorship aspect. The latter is modeled by considering a censoring variable C distributed as an Exponential distribution Exp(1/λ). Typically, the censoring rate is evaluated by the parameter λ. We choose three values of λ that are λ=2,0.5. Such values allowing to generate data with two censoring percentage 60% and 10%. Now the computationablity of the estimator as well as the effect of different parameters involved in its efficiency, such as the conditional model, the choice single index, the choice of the smoothing parameter, and the censorship are examined by drawing m independent n-samples of random pairs (Xi,Yi) and we compute, for each sample, empirical values of the quantities
NT(χ0,θ)=√n^ϕθ(χ0,hn)ˆσ2r(ˆθ,χ0)(ˆrn(ˆθ,χ0)−r(θ,χ0)), |
where χ0 is an arbitrary conditioning curve Xi0 and ˆσ2r(θ,χ0) is the plug-in estimator estimating the asymptotic variance expressed by (4.5). In this quantity, we select the optimal smoothing parameter by the cross-validation rule based on the Asymmetric Least Squares Error defined by
hCV=argminhn∈Hn1nn∑i=1(Yi−ˆr−in(θ,Xi))2, |
where Hn is the set of positive real numbers, hn, such that, the ball centered at x with radius hn contains exactly k neighbors of x. k is selected from the subset Hn={5,15,25,…,55}. Moreover, we point out that the quantity NT(χ0,θ) is obtained by using the β-kernel. Finally, we highlight the impact of the choice of the single index in the estimation quality, we compare this result to an arbitrary choice of θ. Indeed, We repeat the same algorithm where ˆθ=e3 the third, of element of the Karhunen-{L}oève basis functions. After computing the m independent values of (Zi=NTi(χ0,ˆθopt,hopt))i=1,…,m, for all these situations we compare in Figures 2–5 the Q-Q plot of these quantities.
Finally, we see clearly that the asymptotic behavior of the distribution of NT(χ0,θ) is strongly affected by the choice of θ, the censorship rate, and the conditional distribution type even if the effect of the last is not significant compared to the the censoring character and the index choice.
The objective of this section is to assess the performance of the censored functional version of the single index structure. This analysis complements the discussion in the previous section, where we evaluated the ease of implementing the constructed estimator for different levels of censoring. In this section, we return to emphasizing the importance of the functional single index model in practical applications. It is well-documented that the econometrics field is the most suitable domain for this type of semiparametric model (refer to [1] for further discussion). To illustrate the practical relevance, we consider financial data in the form of the intraday return of the Nikkei stock index during the period from January 1, 1984, to December 31, 2021. Given the nature of time-varying data, it can be treated as censored data, as trading in stock markets typically halts whenever the index falls below a certain threshold, especially during crisis periods like the Covid-19 pandemic. Considering the numerous stoppages during this period, the censoring rate of this data is highly significant. The data under study was obtained from the website https://fred.stlouisfed.org/series/NIKKEI225. To ensure a representative sample of independent functional data, we selected three separate months: January, May, and September. This selection resulted in a total of 111 functional observations. To address the heteroscedastic nature of the data, we opted for a transformed version obtained through logarithmic differencing. Typically, we proceed with
For each selected monthXi(d)=−100log(s(d)s(d−1)),dis the days of the month, |
where s(t) is the daily return. Therefore, our objective is to forecast the variation of X(t) one month ahead based on the historical trajectory of the preceding month. Typically, we frame this forecasting problem by defining Y as X(d0)−X(d30). We then assess our prediction approach in comparison to the nonparametric regression model defined by
˜r(x)=n∑i=1∑ni=1K(h−1d(x,Xi))Yi∑nj=1K(h−1d(x,Xj)). |
In conclusion, it is worth noting that we maintain consistent selection methods from the previous section, employing the same kernel. Nevertheless, due to the discontinuity of the functional variable, the selection of the PCA-metric becomes imperative. Additionally, the feasibility of both methods is assessed using the Mean Squared Error (MSE) defined by
MSE=1nn∑i=1(Yi−ˆR−in(Xi))2, |
where ˆR−in(Xi) means either ˆr−in(θ,Xi) or ˜r(x). Ultimately, we partition the data into two sets: a learning sample comprising 80 observations, and a testing sample with 31 observations. This observation split is repeated multiple times (50 times), and for each iteration, we compute the Mean Squared Error (MSE) for both estimators. The resulting errors are depicted in the boxplot shown in Figure 6.
As expected, the semiparametric approach outperforms the nonparametric one, a conclusion supported by the lower MSE values.
In this study, we investigated the nonparametric estimation challenge associated with the regression function in the Single Functional Index Model within the framework of random censoring. We focus on the kernel-type estimator for regression operator, achieving the strong almost-complete consistency along with its rate and the asymptotic normality. We apply our findings to construct confidence intervals. Finally, the usefulness of our proposed methodology is illustrated through both finite sample results and the analysis of real data. Multiple avenues exist for developing our method further. Observe that mixing is a type of asymptotic independence assumption that is commonly used in the pursuit of simplicity, but can be unrealistic in situations where the data are highly dependent. Extending non-parametric functional ideas to a general dependence structure is a discipline that is still in the beginning stages. Notably, the ergodic framework eschews the commonly employed strong mixing condition and its variants for measuring dependence, as well as the extremely involved probabilistic calculations that this condition necessitates. It would be intriguing to extend our work to the case of functional ergodic data, which requires nontrivial mathematics; however, this is well outside the scope of this paper. As a perspective on this work, one can relax the stationarity assumption we made in this paper and investigate similar limit theorems for local stationary functional processes. In this case, we allow the Single Functional Index estimator to change smoothly over time. It will be useful to establish similar results in this paper for data-driven bandwidth. The proof of such a statement, however, should require a different methodology than that used in the present paper, and we leave this problem open for future research. In a similar direction, it will be natural to consider in a future investigation the functional kNN local linear approach expectile regression estimators to obtain an alternative estimator which benefits from advantages of both methods, the local linear method and the kNN approach.
This section is dedicated to establishing the proof of our main result. The notation introduced earlier will persist throughout the subsequent discussion.
Proof of Lemma 3.3. Using the fact that
IE[ˆrD(θ,χ)]=IE[ˆrD(tj(θ),χk(χ))]=1, |
we can write
supχ∈SHsupθ∈ΘH|ˆrD(θ,χ)−IE[ˆrD(θ,χ)]|≤supχ∈SHsupθ∈ΘH|ˆrD(θ,χ)−ˆrD(θ,χk(χ))|+supχ∈SHsupθ∈ΘH|ˆrD(θ,χk(χ))−ˆrD(tj(θ),χk(χ))|+supχ∈SHsupθ∈ΘH|ˆrD(tj(θ),χk(χ))−1|. | (7.1) |
For the first and the second terms of the right-hand side of the inequality (7.1), we use the Hölder continuity condition of K(⋅) (in view of (A3-(ii))) and the Cauchy-Schwartz's inequality to get
supχ∈SHsupθ∈ΘH|ˆrD(θ,χ)−ˆrD(θ,χk(χ))|=O(√logdSHn+logdΘHnnϕ(hn)), | (7.2) |
and
supχ∈SHsupθ∈ΘH|ˆrD(θ,χk(χ))−ˆrD(tj(θ),χk(χ))|=O(√logdSHn+logdΘHnnϕ(hn)). | (7.3) |
Clearly, we have by the Lipschitz property of K(⋅) imposed in (A3-(ii)) and the Cauchy-Schwartz's inequality, we can write
supχ∈SHsupθ∈ΘH|ˆrD(θ,χ)−ˆrD(θ,χk(χ))|≤Cϕθ,χ(hn)supθ∈ΘH1nn∑i=1|K(dθ(χ,Xi)hn)−K(dθ(χk(χ),Xi)hn)|≤Chnϕθ,χ(hn)supθ∈ΘH1nn∑i=1||⟨θ,χ−Xi⟩|−|⟨θ,χk(χ)−Xi⟩||≤Csupθ∈ΘH‖θ‖⋅‖χ−χk(χ)‖hnϕ(hn)≤Clnϕ(hn). |
By the fact that ln=O(lognn), for n sufficiently large, we obtain
supχ∈SHsupθ∈ΘH|ˆrD(θ,χ)−ˆrD(θ,χk(χ))|=O(lognnϕ(hn))=o(√logdSHn+logdΘHnnϕ(hn)). | (7.4) |
Similarly, following the same steps leading to (7.4), we derive
supχ∈SHsupθ∈ΘH|ˆrD(θ,χk(χ))−ˆrD(tj(θ),χk(χ))|=O(lognnϕ(hn))=o(√logdSHn+logdΘHnnϕ(hn)). | (7.5) |
For the last term of the right-hand side of (7.1), we obtain
IP(supχ∈SHsupθ∈ΘH|ˆrD(tj(θ),χk(χ))−1|>η(logdSHn+logdΘHnnϕ(hn))1/2)≤IP(maxk=1,…,dSHnmaxj=1,…,dΘHn|ˆrD(tj(θ),χk(χ))−1|>η(logdSHn+logdΘHnnϕ(hn))1/2)≤CdSHndΘHnIP(|ˆrD(tj(θ),χk(χ))−1|>η(logdSHn+logdΘHnnϕ(hn))1/2). |
We highlight that
ˆrD(tj(θ),χk(χ))−1=1nn∑i=1Ki(tj(θ),χk(χ))−IE[K1(tj(θ),χk(χ))]IE[K1(tj(θ),χk(χ))]=1nn∑i=1Ψi, |
where
Ψi:=Ki(tj(θ),χk(χ))−IE[K1(tj(θ),χk(χ))]IE[K1(tj(θ),χk(χ))]. |
By combining (A1) and (A3-(i)), for all 1≤i≤n, we readily obtain
|Ψi|≤C/ϕ(hn) and IE[|Ψi|2]≤C′/ϕ(hn). | (7.6) |
Applying the Bernstein-type inequality, for instance, see [66], p. 855, we get
IP(1n|n∑i=1Ψi|>η√logdSHn+logdΘHnnϕ(hn))≤C′exp{−Cη2log(dSHndΘHn)}. |
This readily implies that
IP(supχ∈SHsupθ∈ΘH|ˆrD(tj(θ),χk(χ))−1|>η√logdSHn+logdΘHnnϕ(hn))≤C′(dSHndΘHn)1−Cη2. |
Choosing η large enough in such a way that β=Cη2>2 and using the condition (A5-iii) in combination with the Borel-Cantelli's lemma, we infer that
supχ∈SHsupθ∈ΘH|ˆrD(tj(θ),χk(χ))−1|=O(√logdSHn+logdΘHnnϕ(hn)). | (7.7) |
This last result completes the proof of the lemma together with results (7.2) and (7.3).
Proof of Corollary 3.4. For each χ∈SH and θ∈ΘH, we have
infχ∈SHinfθ∈ΘH|ˆrD(θ,χ)|≤12⇒∃χ∈SH∃θ∈ΘH such that supχ∈SHsupθ∈ΘH|1−ˆrD(θ,χ)|>12. |
Consequently, we have
IP(infχ∈SHinfθ∈ΘH|ˆrD(θ,χ)|≤12)≤IP(supχ∈SHsupθ∈ΘH|1−ˆrD(θ,χ)|>12). |
Lemma 3.3 conclude the proof of the corollary.
Proof of Lemma 3.5. We first notice that we have
|ˆrN(θ,χ)−˜rN(θ,χ)|=1nIE[K1(θ,χ)]n∑i=1|(δiYi¯Gn(Yi)−δiYi¯G(Yi))Ki(θ,χ)|=1nIE[K1(θ,χ)]n∑i=1|(1{Y0i≤Ci}Y0i¯Gn(Y0i)−1{Y0i≤Ci}Y0i¯G(Y0i))Ki(θ,χ)|≤1nIE[K1(θ,χ)]n∑i=1|Y0iKi(θ,χ)||(¯Gn(Y0i)−¯G(Y0i)¯Gn(Y0i)¯G(Y0i))|≤τF¯Gn(τF)¯G(τF)supt<τF|¯Gn(t)−¯G(t)|1nIE[K1(θ,χ)]n∑i=1Ki(θ,χ)≤ˆrD(θ,χ)τF¯Gn(τF)¯G(τF)supt<τF|¯Gn(t)−¯G(t)|≤(ˆrD(θ,χ)−1)τF¯Gn(τF)¯G(τF)supt<τF|¯Gn(t)−¯G(t)|+τF¯Gn(τF)¯G(τF)supt<τF|¯Gn(t)−¯G(t)|. |
This readily implies that
supχ∈SHsupθ∈ΘH|ˆrN(θ,χ)−˜rN(θ,χ)|≤supχ∈SHsupθ∈ΘH|ˆrD(θ,χ)−1|τF¯Gn(τF)¯G(τF)supt<τF|¯Gn(t)−¯G(t)|+τF¯Gn(τF)¯G(τF)supt<τF|¯Gn(t)−¯G(t)|. |
By Lemma 3.3 in conjunction with result of [32] or [23], that is :
supt<τF|¯Gn(t)−¯G(t)|=O((log2nn)1/2), |
which implies as n→∞,¯Gn(τF)¯G(τF)∼(¯G(τF))2. Since
log2nn=o(lognnϕ(hn)), |
by (A4-(ii)), we get
supχ∈SHsupθ∈ΘH|ˆrN(θ,χ)−˜rN(θ,χ)|=o(√logdSHn+logdΘHnnϕ(hn)). |
Hence, the proof is complete.
Proof of Lemma 3.3. By stationarity, conditioning w.r.t ⟨θ,X1⟩ and the fact IE[1{Y01≤C1}|Y01]=¯G(Y01), we readily infer
IE[˜rN(θ,χ)]−r(θ,χ)=1IE[K1(θ,χ)]IE[δ1Y1¯G(Y1)K1(θ,χ)]−r(θ,χ)=IE[IE[δ1Y1¯G(Y1)|⟨θ,X1⟩]K1(θ,χ)−r(θ,χ)IE[K1(θ,χ)]IE[K1(θ,χ)]]=IE[IE[Y01¯G(Y01)IE{1{Y01≤C1}|Y01}|⟨θ,X1⟩]K1(θ,χ)−r(θ,χ)IE[K1(θ,χ)]IE[K1(θ,χ)]]=IE[r(θ,X1)K1(θ,χ)−r(θ,χ)IE[K1(θ,χ)]IE[K1(θ,χ)]]=1IE[K1(θ,χ)]IE[K1(θ,χ)1{Bθ(χ,hn)}(X1)(r(θ,X1)−r(θ,χ))]. |
Due to the Lipschitz condition of the function r(⋅,⋅) given in assumption (A2), we get
1Bθ(χ,hn)(X1)|r(θ,X1)−r(θ,χ)|≤Cdθ(χ,X1)γ≤Chγn. |
Finally, uniformly on χ and θ, one can write
supχ∈SHsupθ∈ΘH|IE[˜rN(θ,χ)]−r(θ,χ)|=O(hγn). | (7.8) |
This completes the proof.
Proof of Lemma 3.5. Consider the following decomposition:
supχ∈SHsupθ∈ΘH|˜rN(θ,χ)−IE[˜rN(θ,χ)]|≤supχ∈SHsupθ∈ΘH|˜rN(θ,χ)−˜rN(θ,χk(χ))|⏟F1+supχ∈SHsupθ∈ΘH|˜rN(θ,χk(χ))−˜rN(tj(θ),χk(χ))|⏟F2+supχ∈SHsupθ∈ΘH|˜rN(tj(θ),χk(χ))−IE[˜rN(tj(θ),χk(χ))]|⏟F3+supχ∈SHsupθ∈ΘH|IE[˜rN(tj(θ),χk(χ))]−IE[˜rN(θ,χk(χ))]|⏟F4+supχ∈SHsupθ∈ΘH|IE[˜rN(θ,χk(χ))]−IE[˜rN(θ,χ)]|⏟F5. |
As both F1 and F5 can be treated in a similar way, we only deal with F1. Indeed, by (A3-(ii)) and the Cauchy-Schwartz inequality, we have
supχ∈SHsupθ∈ΘH|˜rN(θ,χ)−˜rN(θ,χk(χ))|≤1nsupχ∈SHsupθ∈ΘHn∑i=1δi|Yi|¯G(Yi)|Ki(θ,χ)IE[K1(θ,χ)]−Ki(θ,χk(χ))IE[K1(θ,χk(χ))]|≤τFnϕ(hn)supχ∈SHsupθ∈ΘHn∑i=11{Y0i≤Ci}|Y0i|¯G(Y0i)|Ki(θ,χ)−Ki(θ,χk(χ))|≤CτF¯G(τF)supχ∈SHsupθ∈ΘH‖θ‖⋅‖χ−χk(χ)‖hnϕ(hn)≤CτF¯G(τF)lnϕ(hn). |
Thus, by assumption (A4-(ii)), since ln=O(lognn), by Jensen's inequality, as n tends to infinity, we get
F5≤F1=O(√logdSHn+logdΘHnnϕ(hn)). | (7.9) |
Analogously, dealing with F2 and F4 in the same way, one may easily obtain
supχ∈SHsupθ∈ΘH|˜rN(θ,χ)−˜rN(θ,χk(χ))|≤Cnϕ(hn)supχ∈SHsupθ∈ΘHn∑i=11{Y0i≤Ci}|Y0i|¯G(Y0i)|Ki(θ,χ)−Ki(tj(θ),χ)|≤CτF¯G(τF)supχ∈SHsupθ∈ΘH‖χ‖⋅‖θ−tj(θ)‖hnϕ(hn)≤CτF¯G(τF)lnϕ(hn)=O(lognnϕ(hn)). |
Hence, we conclude that
F4≤F2=O(√logdSHn+logdΘHnnϕ(hn)). | (7.10) |
Now, our focus turns to the upper bound of the term F3. On the one hand, we express
IP(F3>ϵ)≤dSHndΘHnmax1≤k≤dSHnmax1≤j≤dΘHnIP(|˜rN(tj(θ),χk(χ))−IE[˜rN(tj(θ),χk(χ))]|>ϵ)≤dSHndΘHnmax1≤k≤dSHnmax1≤j≤dΘHnIP(|n∑i=1˜Ψi|>nϵ), |
with
˜Ψi:=δiYiKi(tj(θ),χk(χ))−IE[δiYiKi(tj(θ),χk(χ))]¯G(Yi)IE[K1(tj(θ),χk(χ))]. |
On the other hand, in order to apply the Bernstein-type exponential inequality we need to bound the two quantities |˜Ψi| and IE|˜Ψi|2, for each i=1,…,n. Indeed, because of (A1) and (A3-(i)), by the fact that
IE[1{Y0i≤Ci}|Y0i]=¯G(Y0i), |
we have
|˜Ψi|=|1{Y0i≤Ci}Y0iKi(tj(θ),χk(χ))−IE[1{Y0i≤Ci}Y0iKi(tj(θ),χk(χ))]¯G(Y0i)IE[K1(tj(θ),χk(χ))]|=|Y0iKi(tj(θ),χk(χ))−IE[Y0iKi(tj(θ),χk(χ))]IE[K1(tj(θ),χk(χ))]|≤τF(|Ki(tj(θ),χk(χ))IE[K1(tj(θ),χk(χ))]|+1)=O(1ϕ(hn)). |
By assumption (A1-(ii)) and the fact that, for each θ,χ and i, as n goes to ∞ ,
IE[Kli(θ,χ)]→Clϕθ,χ(hn),l∈{1,2}, | (7.11) |
for details see [30]. This readily implies that
IE|˜Ψi|2=IE|1{Y0i≤Ci}Y0iKi(tj(θ),χk(χ))−IE[1{Y0i≤Ci}Y0iKi(tj(θ),χk(χ))]¯G(Y0i)IE[K1(tj(θ),χk(χ))]|2≤IE[(1{Y0i≤Ci}Y0i¯G(Y0i))2K2i(tj(θ),χk(χ))IE2[K1(tj(θ),χk(χ))]]≤IE[(IE[1{Y0i≤Ci}|Y0i]Y0i¯G(Y0i))2K2i(tj(θ),χk(χ))IE2[K1(tj(θ),χk(χ))]]≤(Y0iIE[K1(tj(θ),χk(χ))])2IE[K21(tj(θ),χk(χ))]≤(τFC1ϕθ,χ(hn))2(C2ϕθ,χ(hn))=O(1ϕ(hn)). |
Now, letting ϵ=η√logdSHn+logdΘHnnϕ(hn), and applying Bernstein's inequality, we get
IP(F3>η√logdSHn+logdΘHnnϕ(hn))≤C′dSHndΘHnexp{−Cη2log(dSHndΘHn)}≤C′(dSHndΘHn)1−Cη2. |
By choosing β=Cη2 and using (A4-(ii)), we get
F3=Oa.co.(√logdSHn+logdΘHnnϕ(hn)). | (7.12) |
Therefore, Lemma 3.5 follows from results (7.9), (7.10) and (7.12).
Proof of Lemma 3.9. It is clear that the result of Lemma 3.7 allows us to write
ˆrD(θ,χ)−1=O((lognnϕθ,χ(hn))1/2), |
almost completely, which implies that ˆrD(θ,χ) converges to 1, in probability as n goes to ∞.
Proof of Lemma 3.10. By the result of Lemma 3.9, and the fact that ¯Gn(t) converges asymptotically to ¯G(t), for all t<τF, and thanks to the result (7.8), under assumption (A4'-ii), it follows that
(nϕθ,χ(hn))1/2(IE[ˆrn(θ,χ)]−ˆrD(θ,χ)r(θ,χ))=O(√nϕθ,χ(hn)h2γn)→0, as n→∞. |
This completes the proof of the lemma.
Proof of Lemma 3.11. For all θ,χ∈H, we define
Qi(θ,χ)=√ϕθ,χ(hn)√nIE[K1(θ,χ)](δiYi¯Gn(Yi)Ki(θ,χ)−IE[δ1Y1¯Gn(Y1)K1(θ,χ)]), |
and
Sn:=n∑i=1Qi(θ,χ). |
We have
Sn=√nϕθ,χ(hn)(ˆrN(θ,χ)−IE[ˆrN(θ,χ)]). |
So, our claimed result is now
Sn→N(0,σ2r(θ,χ)). | (7.13) |
Therefore, we have
Var(Sn)=nϕθ,χ(hn)Var(ˆrN(θ,χ)−IE[ˆrN(θ,χ)])=nϕθ,χ(hn)Var(ˆrN(θ,χ)). |
Now, we have by conditioning w.r.t ⟨θ,X1⟩, and taking into account the stationarity property and the fact that ¯Gn(t)→¯G(t), we have
Var(ˆrN(θ,χ))=1(nIE[K1(θ,χ)])2Var(n∑i=1δiYi¯Gn(Yi)Ki(θ,χ))=(IE[δ1Y1¯G(Y1)|⟨θ,X1⟩])2(nIE[K1(θ,χ)])2Var(n∑i=1Ki(θ,χ))=(r(θ,χ))2{IE[K21(θ,χ)]n(IE[K1(θ,χ)])2−1n}. |
Thanks to the result (7.11), we have
Var(ˆrN(θ,χ))=(r(θ,χ))2[C2ϕθ,χ(hn)n(C1ϕθ,χ(hn))2−1n]=(r(θ,χ))2[C2nC21ϕθ,χ(hn)+o(1nϕθ,χ(hn))]=(r(θ,χ))2[C2C21+o(1)]1nϕθ,χ(hn), | (7.14) |
and finally
Var(Sn)=nϕθ,χ(hn)(r(θ,χ))2[C2C21+o(1)]1nϕθ,χ(hn)=(r(θ,χ))2[C2C21+o(1)]→C2/C21(r(θ,χ))2=:σ2r(θ,χ),as n→∞. |
Now, with Zi(θ,χ)=1nIE[K1(θ,χ)]δiYi¯Gn(Yi)Ki(θ,χ), the only remaining task is, for some l>0, to prove
n∑i=1IE[|Zi(θ,χ)−IEZi(θ,χ)|2+l](Var[n∑i=1Zi(θ,χ)])(2+l)/2⟶0, as n→∞. | (7.15) |
Thanks to result (7.14), it is clear that
nϕθ,χ(hn)Var[n∑i=1Zi(θ,χ)]=nϕθ,χ(hn)Var(ˆrN(θ,χ)), |
converges to C2C21(r(θ,χ))2=σ2r(θ,χ), as n→∞. So, it remains to prove that, as n→∞ :
(nϕθ,χ(hn))(2+l)/2n∑i=1IE[|Zi(θ,χ)−IEZi(θ,χ)|2+l]→0. |
Since |δiYi¯Gn(Yi)|<τF, and by the use of the elementary inequality : (a+b)p≤2p−1(ap+bp),∀a,b≥0,∀p≥1, we arrive at
(nϕθ,χ(hn))(2+l)/2n∑i=1IE[|Zi(θ,χ)−IEZi(θ,χ)|2+l]≤(nϕθ,χ(hn))(2+l)/2(τFC1nϕθ,χ(hn))2+ln∑i=1IE[(|Ki(θ,χ)|+|IE[Ki(θ,χ)]|)2+l]≤(τFC1)2+l1(nϕθ,χ(hn))(2+l)/2n∑i=1IE[(|Ki(θ,χ)|+|IE[Ki(θ,χ)]|)2+l]≤21+l(τFC1)2+l1(nϕθ,χ(hn))(2+l)/2n∑i=1IE[(|Ki(θ,χ)|2+l+|IE[Ki(θ,χ)]|2+l)]≤21+l(τFC1)2+l1(nϕθ,χ(hn))(2+l)/2n(IE[|K1(θ,χ)|2+l]+|IE[K1(θ,χ)]|2+l)≤21+l(τFC1)2+l1(nϕθ,χ(hn))(2+l)/2n(Cϕθ,χ(hn)+(ϕθ,χ(hn))2+l)≤21+l(τFC1)2+l1(nϕθ,χ(hn))(2+l)/2nϕθ,χ(hn)(C+o(1))=O(1(nϕθ,χ(hn))l/2)⟶0. |
The last convergence is a consequence of A4'(i). This finishes the proof of the lemma.
The authors declare they have not used Artificial Intelligence (AI) tools in the creation of this article.
The authors would like to thank the Associate-Editor and the four anonymous reviewer for their valuable comments and suggestions which improved substantially the quality of an earlier version of this paper.
The authors thank and extend their appreciation to the funders of this project: The Deanship of Scientific Research at King Khalid University through the Research Groups Program under grant number R.G.P. 1/366/44 and The Ministry of Higher Education and Scientific Research Algeria (MESRS), University of Sciences and Technology Oran Mohamed Boudiaf through PRFU program, under code C00L03UN310220230005.
The authors declare that they have no known competing financial interests or personal relationships that could have appeared to influence the work reported in this paper.
[1] |
A. Ait-Saïdi, F. Ferraty, R. Kassa, P. Vieu, Cross-validated estimations in the single-functional index model, Statistics, 42 (2008), 475–494. https://doi.org/10.1080/02331880801980377 doi: 10.1080/02331880801980377
![]() |
[2] |
I. M. Almanjahie, S. Bouzebda, Z. Kaid, A. Laksaci, Nonparametric estimation of expectile regression in functional dependent data, J. Nonparametr. Stat., 34 (2022), 250–281. https://doi.org/10.1080/10485252.2022.2027412 doi: 10.1080/10485252.2022.2027412
![]() |
[3] |
S. Attaoui, On the nonparametric conditional density and mode estimates in the single functional index model with strongly mixing data, Sankhya A, 76 (2014), 356–378. https://doi.org/10.1007/s13171-014-0051-6 doi: 10.1007/s13171-014-0051-6
![]() |
[4] |
S. Attaoui, N. Ling, Asymptotic results of a nonparametric conditional cumulative distribution estimator in the single functional index modeling for time series data with applications, Metrika, 79 (2016), 485–511. https://doi.org/10.1007/s00184-015-0564-6 doi: 10.1007/s00184-015-0564-6
![]() |
[5] |
S. Attaoui, A. Laksaci, E. Ould Said, A note on the conditional density estimate in the single functional index model, Statist. Probab. Lett., 81 (2011), 45–53. https://doi.org/10.1016/j.spl.2010.09.017 doi: 10.1016/j.spl.2010.09.017
![]() |
[6] | S. Bhattacharjee, H. G. Müller, Single index Fréchet regression, Ann. Statist., 51 (2023), 1770–1798. https://doi.org/110.1214/23-aos2307 |
[7] | V. I. Bogachev, Gaussian Measures, Providence: American Mathematical Society, 1998. |
[8] | D. Bosq, Linear Processes in Function Spaces, New York: Springer-Verlag, 2000. https://doi.org/10.1007/978-1-4612-1154-9 |
[9] | M. Bouraine, A. A. Saidi, F. Ferraty, P. Vieu, Choix optimal de l'indice multi-fonctionnel: méthode de validation croisée, Rev. Roumaine Math. Pures Appl., 55 (2010), 355–367. |
[10] | S. Bouzebda, On the weak convergence and the uniform-in-bandwidth consistency of the general conditional U-processes based on the copula representation: multivariate setting, Hacet. J. Math. Stat., 52 (2023), 1303–1348. |
[11] |
S. Bouzebda, General tests of conditional independence based on empirical processes indexed by functions, Jpn. J. Stat. Data Sci., 6 (2023), 115–177. https://doi.org/10.1007/s42081-023-00193-3 doi: 10.1007/s42081-023-00193-3
![]() |
[12] |
S. Bouzebda, M. Chaouch, Uniform limit theorems for a class of conditional Z-estimators when covariates are functions, J. Multivariate Anal., 189 (2022), 104872. https://doi.org/10.1016/j.jmva.2021.104872. doi: 10.1016/j.jmva.2021.104872
![]() |
[13] |
S. Bouzebda, B. Nemouchi, Weak-convergence of empirical conditional processes and conditional U-processes involving functional mixing data, Stat. Inference Stoch. Process., 26 (2023), 33–88. https://doi.org/10.1007/s11203-022-09276-6 doi: 10.1007/s11203-022-09276-6
![]() |
[14] |
S. Bouzebda, N. Taachouche, Rates of the strong uniform consistency for the kernel-type regression function estimators with general kernels on manifolds, Math. Methods Statist., 32 (2023), 27–80. https://doi.org/10.3103/s1066530723010027 doi: 10.3103/s1066530723010027
![]() |
[15] |
S. Bouzebda, N. Taachouche, On the variable bandwidth kernel estimation of conditional U-statistics at optimal rates in sup-norm, Phys. A, 625 (2023), 129000. https://doi.org/10.1016/j.physa.2023.129000 doi: 10.1016/j.physa.2023.129000
![]() |
[16] |
S. Bouzebda, I. Elhattab, A. Abdeldjaoued Ferfache, General M-estimator processes and their m out of n bootstrap with functional nuisance parameters, Methodol. Comput. Appl. Probab., 24 (2022), 2961–3005. https://doi.org/10.1007/s11009-022-09965-y doi: 10.1007/s11009-022-09965-y
![]() |
[17] |
S. Bouzebda, A. Abdeldjaoued Ferfache, T. El-hadjali, Uniform in bandwidth consistency of conditional U-statistics adaptive to intrinsic dimension in presence of censored data, Sankhya A, 85 (2023), 1548–1606. https://doi.org/10.1007/s13171-022-00301-7 doi: 10.1007/s13171-022-00301-7
![]() |
[18] | A. Carbonez, L. Györfi, E. C. van der Meulen, Partitioning-estimates of a regression function under random censoring, Statist. Decisions, 13 (1995), 21–37. |
[19] | L. Györfi, M. Kohler, A. Krzyżak, H. Walk, A Distribution-Free Theory of Nonparametric Regression, New York: Springer, 2002. https://doi.org/10.1007/b97848 |
[20] | J. E. Chacón, T. Duong, Multivariate Kernel Smoothing and Its Applications, Boca Raton: CRC Press, 2018. https://doi.org/10.1201/9780429485572. |
[21] |
D. Chen, P. Hall, H. G. Müller, Single and multiple index functional regression models with nonparametric link, Ann. Statist., 39 (2011), 1720–1747. https://doi.org/10.1214/11-AOS882 doi: 10.1214/11-AOS882
![]() |
[22] |
A. Cuevas, A partial overview of the theory of statistics with functional data, J. Statist. Plann. Inference, 147 (2014), 1–23. https://doi.org/10.1016/j.jspi.2013.04.002 doi: 10.1016/j.jspi.2013.04.002
![]() |
[23] |
P. Deheuvels, J. H. J. Einmahl, Functional limit laws for the increments of Kaplan-Meier product-limit processes and applications, Ann. Prob., 28 (2000), 1301–1335. https://doi.org/10.1214/aop/1019160336 doi: 10.1214/aop/1019160336
![]() |
[24] | L. Devroye, A Course in Density Estimation, Boston: Birkhäuser Boston, 1987. |
[25] | L. Devroye, G. Lugosi, Combinatorial Methods in Density Estimation, New York: Springer-Verlag, 2001. https://doi.org/10.1007/978-1-4613-0125-7 |
[26] | P. P. B. Eggermont, V. N. LaRiccia, Maximum Penalized Likelihood Estimation. Volume II. Regression, Dordrecht: Springer, 2009. https://doi.org/10.1007/b12285 |
[27] |
S. Feng, P. Tian, Y. Hu, G. Li, Estimation in functional single-index varying coefficient model, J. Statist. Plann. Inference, 214 (2021), 62–75. https://doi.org/10.1016/j.jspi.2021.01.003 doi: 10.1016/j.jspi.2021.01.003
![]() |
[28] | F. Ferraty, P. Vieu, Nonparametric Functional Data Analysis, New York: Springer, 2006. |
[29] |
F. Ferraty, A. Peuch, P. Vieu, Modèle à indice fonctionnel simple, C. R. Math. Acad. Sci. Paris, 336 (2003), 1025–1028. https://doi.org/10.1016/S1631-073X(03)00239-5 doi: 10.1016/S1631-073X(03)00239-5
![]() |
[30] |
F. Ferraty, A. Mas, P. Vieu, Nonparametric regression on functional data: inference and practical aspects, Aust. N. Z. J. Stat., 49 (2007), 267–286. https://doi.org/10.1111/j.1467-842X.2007.00480.x doi: 10.1111/j.1467-842X.2007.00480.x
![]() |
[31] |
F. Ferraty, I. Van Keilegom, P. Vieu, On the validity of the bootstrap in non-parametric functional regression, Scand. J. Stat., 37 (2010), 286–306. https://doi.org/10.1111/j.1467-9469.2009.00662.x doi: 10.1111/j.1467-9469.2009.00662.x
![]() |
[32] |
A. Földes, L. Rejtő, A LIL type result for the product limit estimator, Z. Wahrsch. Verw. Gebiete, 56 (1981), 75–86. https://doi.org/10.1007/BF00531975 doi: 10.1007/BF00531975
![]() |
[33] | T. Gasser, P. Hall, B. Presnell, Nonparametric estimation of the mode of a distribution of random curves, J. R. Stat. Soc. Ser. B Stat. Methodol., 60 (1998), 681–691. |
[34] |
A. Goia, P. Vieu, An introduction to recent advances in high/infinite dimensional statistics, J. Multivariate Anal., 146 (2016), 1–6. https://doi.org/10.1016/j.jmva.2015.12.001 doi: 10.1016/j.jmva.2015.12.001
![]() |
[35] |
L. Gu, L. Yang, Oracally efficient estimation for single-index link function with simultaneous confidence band, Electron. J. Stat., 9 (2015), 1540–1561. https://doi.org/10.1214/15-EJS1051 doi: 10.1214/15-EJS1051
![]() |
[36] |
Z. Guessoum, E. Ould-Saïd, On nonparametric estimation of the regression function under random censorship model, Statist. Decis., 26 (2008), 159–177. https://doi.org/10.1524/stnd.2008.0919 doi: 10.1524/stnd.2008.0919
![]() |
[37] |
P. Hall, M. Hosseini-Nasab, On properties of functional principal components analysis, J. R. Stat. Soc. Ser. B Stat. Methodol., 68 (2006), 109–126. https://doi.org/10.1111/j.1467-9868.2005.00535.x doi: 10.1111/j.1467-9868.2005.00535.x
![]() |
[38] | M. M. Hamri, S. D. Mekki, A. Rabhi, N. Kadiri, Single functional index quantile regression for independent functional data under right-censoring, Economet. Ekonometria. Adv. Appl. Data Anal., 26 (2022), 31–62. |
[39] |
Z. C. Han, J. G. Lin, Y. Y. Zhao, Adaptive semiparametric estimation for single index models with jumps, Comput. Statist. Data Anal., 151 (2020), 107013. https://doi.org/10.1016/j.csda.2020.107013 doi: 10.1016/j.csda.2020.107013
![]() |
[40] | M. Hao, K. Liu, W. Su, X. Zhao, Semiparametric estimation for the functional additive hazards model, Canad. J. Statist., 2023. https://doi.org/10.1002/cjs.11805 |
[41] |
W. Härdle, V. Spokoiny, S. Sperlich, Semiparametric single index versus fixed link function modelling, Ann. Statist., 25 (1997), 212–243. https://doi.org/10.1214/aos/1034276627 doi: 10.1214/aos/1034276627
![]() |
[42] | L. Horváth, P. Kokoszka, Inference for Functional Data with Applications. New York: Springer, 2012. https://doi.org/10.1007/978-1-4614-3655-3 |
[43] |
Z. Jiang, Z. Huang, J. Zhang, Functional single-index composite quantile regression, Metrika, 86 (2023), 595–603. https://doi.org/10.1007/s00184-022-00887-w doi: 10.1007/s00184-022-00887-w
![]() |
[44] | E. L. Kaplan, P. Meier, Nonparametric estimation from incomplete observations, J. Amer. Statist. Assoc., 53 (1958), 457–481. |
[45] |
M. Kohler, K. Máthé, M. Pintér, Prediction from randomly right censored data, J. Multivariate Anal., 80 (2002), 73–100. https://doi.org/10.1006/jmva.2000.1973 doi: 10.1006/jmva.2000.1973
![]() |
[46] |
D. R. Kowal, A. Canale, Semiparametric functional factor models with bayesian rank selection, Bayesian Anal., 18 (2023), 1161–1189. https://doi.org/10.1214/23-ba1410 doi: 10.1214/23-ba1410
![]() |
[47] |
J. Li, C. Huang, H. Zhu, A functional varying-coefficient single-index model for functional response data, J. Amer. Statist. Assoc., 112 (2017), 1169–1181. https://doi.org/10.1080/01621459.2016.1195742 doi: 10.1080/01621459.2016.1195742
![]() |
[48] |
W. V. Li, Q. M. Shao, Gaussian processes: inequalities, small ball probabilities and applications, Handbook Statist., 19 (2001), 533–597. https://doi.org/10.1016/S0169-7161(01)19019-X doi: 10.1016/S0169-7161(01)19019-X
![]() |
[49] |
Y. Li, N. Wang, R. J. Carroll, Generalized functional linear models with semiparametric single-index interactions, J. Amer. Statist. Assoc., 105 (2010), 621–633. https://doi.org/10.1198/jasa.2010.tm09313 doi: 10.1198/jasa.2010.tm09313
![]() |
[50] |
H. Liang, X. Liu, R. Li, C. L. Tsai, Estimation and testing for partially linear single-index models, Ann. Statist., 38 (2010), 3811–3836. https://doi.org/10.1214/10-AOS835 doi: 10.1214/10-AOS835
![]() |
[51] |
N. Ling, P. Vieu, Nonparametric modelling for functional data: selected survey and tracks for future, Statistics, 52 (2018), 934–949. https://doi.org/10.1080/02331888.2018.1487120 doi: 10.1080/02331888.2018.1487120
![]() |
[52] |
N. Ling, Qian Xu, Asymptotic normality of conditional density estimation in the single index model for functional time series data, Statist. Probab. Lett., 82 (2012), 2235–2243. https://doi.org/10.1016/j.spl.2012.08.018 doi: 10.1016/j.spl.2012.08.018
![]() |
[53] | N. Ling, L. Cheng, P. Vieu, Single functional index model under responses MAR and dependent observations, In: Functional and high-dimensional statistics and related fields, 161–168, 2020. https://doi.org/10.1007/978-3-030-47756-1_22 |
[54] |
N. Ling, L. Cheng, P. Vieu, H. Ding, Missing responses at random in functional single index model for time series data, Statist. Papers, 63 (2022), 665–692. https://doi.org/10.1007/s00362-021-01251-2 doi: 10.1007/s00362-021-01251-2
![]() |
[55] |
E. Masry, Nonparametric regression estimation for dependent functional data: asymptotic normality, Stochast. Process. Appl., 115 (2005), 155–177. https://doi.org/10.1016/j.spa.2004.07.006 doi: 10.1016/j.spa.2004.07.006
![]() |
[56] | E. Mayer-Wolf, O. Zeitouni, The probability of small Gaussian ellipsoids and associated conditional moments, Ann. Probab., 21 (1993), 14–24. |
[57] | G. McLachlan, D. Peel, Finite Mixture Models, New York: Wiley-Interscience, 2000. https://doi.org/10.1002/0471721182 |
[58] | M. Mohammedi, S. Bouzebda, A. Laksaci, O. Bouanani, Asymptotic normality of the k-nn single index regression estimator for functional weak dependence data, Comm. Statist. Theory Meth., 2022. https://doi.org/10.1080/03610926.2022.2150823 |
[59] | È. A. Nadaraja, On a regression estimate, Teor. Verojatnost. Primenen., 9 (1964), 157–159. |
[60] | È. A. Nadaraya, Nonparametric Estimation of Probability Densities and Regression Curves, Dordrecht: Kluwer Academic Publishers, 1989. https://doi.org/10.1007/978-94-009-2583-0 |
[61] |
Y. Nie, L. Wang, J. Cao, Estimating functional single index models with compact support, Environmetrics, 34 (2023), e2784. https://doi.org/10.1002/env.2784 doi: 10.1002/env.2784
![]() |
[62] |
S. Novo, G. Aneiros, P. Vieu, Automatic and location-adaptive estimation in functional single-index regression, J. Nonparametr. Stat., 31 (2019), 364–392. https://doi.org/10.1080/10485252.2019.1567726 doi: 10.1080/10485252.2019.1567726
![]() |
[63] |
E. Ould-Saïd, A strong uniform convergence rate of kernel conditional quantile estimator under random censorship, Statist. Prob. Lett., 76 (2006), 579–586. https://doi.org/10.1016/j.spl.2005.09.002 doi: 10.1016/j.spl.2005.09.002
![]() |
[64] | J. O. Ramsay, B. W. Silverman, Functional Data Analysis, New York: Springer, 2005. |
[65] | D. W. Scott, Multivariate Density Estimation. Theory, Practice, and Visualization, WHoboken: John Wiley & Sons, 2015. |
[66] | G. R. Shorack, J. A. Wellner, Empirical Processes with Applications to Statistics, New York: John Wiley & Sons, 1986. |
[67] | B. W. Silverman, Density Estimation for Statistics and Data Analysis, London: Chapman & Hall, 1986. https://doi.org/10.1007/978-1-4899-3324-9 |
[68] | I. Soukarieh, S. Bouzebda, Weak convergence of the conditional U-statistics for locally stationary functional time series, Stat. Infer. Stoch. Process., 2022. |
[69] |
W. Stute, L. X. Zhu, Nonparametric checks for single-index models, Ann. Statist., 33 (2005), 1048–1083. https://doi.org/10.1214/009053605000000020 doi: 10.1214/009053605000000020
![]() |
[70] |
Q. Tang, L. Kong, D. Rupper, R. J. Karunamuni, Partial functional partially linear single-index models, Statist. Sinica, 31 (2021), 107–133. https://doi.org/10.5705/ss.20 doi: 10.5705/ss.20
![]() |
[71] | M. P. Wand, M. C. Jones, Kernel Smoothing, London: Chapman and Hall, 1995. https://doi.org/10.1007/978-1-4899-4493-1 |
[72] | Y. A. Wang, Q. Huang, Z. Yao, Y. Zhang, On a class of linear regression methods, J. Complexity, 2024, 101826. https://doi.org/10.1016/j.jco.2024.101826 |
[73] | G. S. Watson, Smooth regression analysis, Sankhy\ = {a} Ser. A, 26 (1964), 359–372. |
[74] |
Y. Zhang, B. Hofmann, On fractional asymptotical regularization of linear ill-posed problems in Hilbert spaces, Fract. Calc. Appl. Anal., 22 (2019), 699–721. https://doi.org/10.1515/fca-2019-0039 doi: 10.1515/fca-2019-0039
![]() |
[75] |
W. Zhou, J. Gao, D. Harris, H. Kew, Semi-parametric single-index predictive regression models with cointegrated regressors, J. Economet., 238 (2024), 105577. https://doi.org/10.1016/j.jeconom.2023.105577 doi: 10.1016/j.jeconom.2023.105577
![]() |
[76] |
H. Zhu, R. Zhang, Y. Liu, H. Ding, Robust estimation for a general functional single index model via quantile regression, J. Korean Statist. Soc., 51 (2022), 1041–1070. https://doi.org/10.1007/s42952-022-00174-4 doi: 10.1007/s42952-022-00174-4
![]() |
1. | Salim Bouzebda, Weak convergence of the conditional single index $ U $-statistics for locally stationary functional time series, 2024, 9, 2473-6988, 14807, 10.3934/math.2024720 | |
2. | Fatimah A Almulhim, Torkia Merouan, Mohammed B. Alamari, Boubaker Mechab, Local linear estimation for the censored functional regression, 2024, 9, 2473-6988, 13980, 10.3934/math.2024679 | |
3. | Oussama Bouanani, Salim Bouzebda, Limit theorems for local polynomial estimation of regression for functional dependent data, 2024, 9, 2473-6988, 23651, 10.3934/math.20241150 | |
4. | Salim Bouzebda, Uniform in Number of Neighbor Consistency and Weak Convergence of k-Nearest Neighbor Single Index Conditional Processes and k-Nearest Neighbor Single Index Conditional U-Processes Involving Functional Mixing Data, 2024, 16, 2073-8994, 1576, 10.3390/sym16121576 | |
5. | Breix Michael Agua, Salim Bouzebda, Single index regression for locally stationary functional time series, 2024, 9, 2473-6988, 36202, 10.3934/math.20241719 | |
6. | Fatimah A. Almulhim, Mohammed B. Alamari, Salim Bouzebda, Zoulikha Kaid, Ali Laksaci, Robust Estimation of L1-Modal Regression Under Functional Single-Index Models for Practical Applications, 2025, 13, 2227-7390, 602, 10.3390/math13040602 | |
7. | Khalid Chokri, Salim Bouzebda, Optimal Almost Sure Rate of Convergence for the Wavelets Estimator in the Partially Linear Additive Models, 2025, 17, 2073-8994, 394, 10.3390/sym17030394 | |
8. | Said Attaoui, Oum Elkheir Benouda, Salim Bouzebda, Ali Laksaci, Limit Theorems for Kernel Regression Estimator for Quasi-Associated Functional Censored Time Series Within Single Index Structure, 2025, 13, 2227-7390, 886, 10.3390/math13050886 |