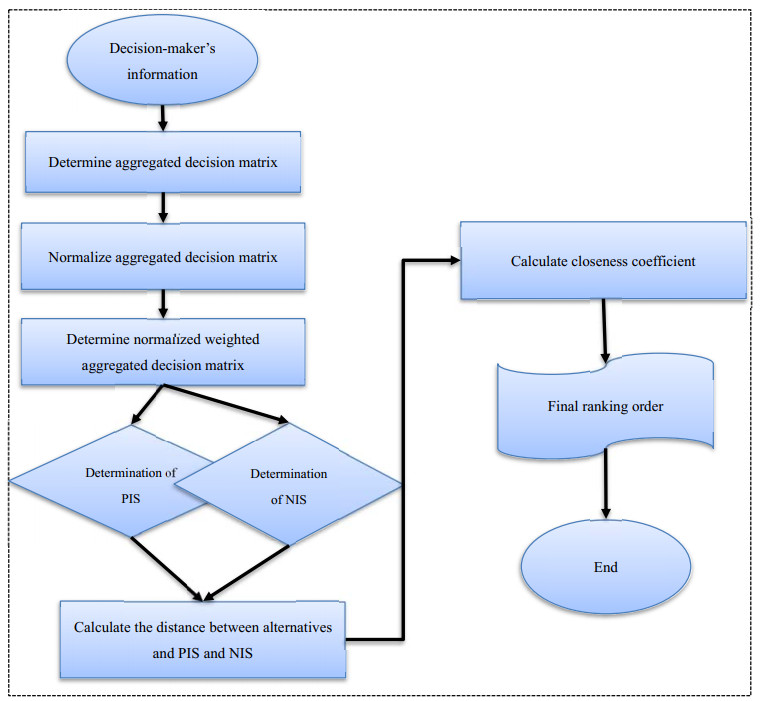
We delved into a category of output feedback nonlinear systems that are distinguished by unmodeled dynamics, quantized input delays, and dynamic uncertainties. We introduce a novel finite-time adaptive dynamic surface control scheme developed through the construction of a first-order nonlinear filter. This approach integrates Young's inequality with neural network technologies. Then, to address unmodeled dynamics, the scheme incorporates a dynamic signal and utilizes Radial Basis Function (RBF) neural networks to approximate unknown smooth functions. Furthermore, an auxiliary function is devised to mitigate the impact of input quantization delays on the system's performance. The new controller design is both simple and effective, addressing the "hasingularity" problems typically associated with traditional finite-time controls. Theoretical analyses and simulation outcomes confirm the effectiveness of this approach, guaranteeing that all signals in the system are confined within a finite period.
Citation: Changgui Wu, Liang Zhao. Finite-time adaptive dynamic surface control for output feedback nonlinear systems with unmodeled dynamics and quantized input delays[J]. AIMS Mathematics, 2024, 9(11): 31553-31580. doi: 10.3934/math.20241518
[1] | Muhammad Akram, Sumera Naz, Feng Feng, Ghada Ali, Aqsa Shafiq . Extended MABAC method based on 2-tuple linguistic T-spherical fuzzy sets and Heronian mean operators: An application to alternative fuel selection. AIMS Mathematics, 2023, 8(5): 10619-10653. doi: 10.3934/math.2023539 |
[2] | Muhammad Akram, Sumera Naz, Gustavo Santos-García, Muhammad Ramzan Saeed . Extended CODAS method for MAGDM with 2-tuple linguistic T-spherical fuzzy sets. AIMS Mathematics, 2023, 8(2): 3428-3468. doi: 10.3934/math.2023176 |
[3] | Muhammad Naeem, Aziz Khan, Shahzaib Ashraf, Saleem Abdullah, Muhammad Ayaz, Nejib Ghanmi . A novel decision making technique based on spherical hesitant fuzzy Yager aggregation information: application to treat Parkinson's disease. AIMS Mathematics, 2022, 7(2): 1678-1706. doi: 10.3934/math.2022097 |
[4] | Muhammad Qiyas, Muhammad Naeem, Saleem Abdullah, Neelam Khan . Decision support system based on complex T-Spherical fuzzy power aggregation operators. AIMS Mathematics, 2022, 7(9): 16171-16207. doi: 10.3934/math.2022884 |
[5] | Hafiz Muhammad Athar Farid, Muhammad Riaz, Gustavo Santos Garcia . T-spherical fuzzy information aggregation with multi-criteria decision-making. AIMS Mathematics, 2023, 8(5): 10113-10145. doi: 10.3934/math.2023512 |
[6] | Ashraf Al-Quran . T-spherical linear Diophantine fuzzy aggregation operators for multiple attribute decision-making. AIMS Mathematics, 2023, 8(5): 12257-12286. doi: 10.3934/math.2023618 |
[7] | Muhammad Naeem, Muhammad Qiyas, Lazim Abdullah, Neelam Khan, Salman Khan . Spherical fuzzy rough Hamacher aggregation operators and their application in decision making problem. AIMS Mathematics, 2023, 8(7): 17112-17141. doi: 10.3934/math.2023874 |
[8] | Muhammad Ali Khan, Saleem Abdullah, Alaa O. Almagrabi . Analysis of deep learning technique using a complex spherical fuzzy rough decision support model. AIMS Mathematics, 2023, 8(10): 23372-23402. doi: 10.3934/math.20231188 |
[9] | Muhammad Qiyas, Muhammad Naeem, Neelam Khan . Fractional orthotriple fuzzy Choquet-Frank aggregation operators and their application in optimal selection for EEG of depression patients. AIMS Mathematics, 2023, 8(3): 6323-6355. doi: 10.3934/math.2023320 |
[10] | Khawlah Alhulwah, Muhammad Azeem, Mehwish Sarfraz, Nasreen Almohanna, Ali Ahmad . Prioritized aggregation operators for Schweizer-Sklar multi-attribute decision-making for complex spherical fuzzy information in mobile e-tourism applications. AIMS Mathematics, 2024, 9(12): 34753-34784. doi: 10.3934/math.20241655 |
We delved into a category of output feedback nonlinear systems that are distinguished by unmodeled dynamics, quantized input delays, and dynamic uncertainties. We introduce a novel finite-time adaptive dynamic surface control scheme developed through the construction of a first-order nonlinear filter. This approach integrates Young's inequality with neural network technologies. Then, to address unmodeled dynamics, the scheme incorporates a dynamic signal and utilizes Radial Basis Function (RBF) neural networks to approximate unknown smooth functions. Furthermore, an auxiliary function is devised to mitigate the impact of input quantization delays on the system's performance. The new controller design is both simple and effective, addressing the "hasingularity" problems typically associated with traditional finite-time controls. Theoretical analyses and simulation outcomes confirm the effectiveness of this approach, guaranteeing that all signals in the system are confined within a finite period.
Cloud storage is the practice of storing digital data on remote servers accessed via the internet instead of local physical devices. In comparison to conventional storage techniques, it has many benefits. It allows users to store, access, and manage their data from any location with an internet connection [1]. The selection of a cloud storage provider is crucial. It guarantees the safety, dependability and accessibility of data that has been saved. Strong data security measures are provided by a reliable source, lowering the possibility of breaches or illegal access [2]. When several decision-makers (DMs) or stakeholders with varied preferences and opinions are participating in the selection process, the cloud storage provider selection problem changes into a multi-attribute group decision-making (MAGDM) problem. Multiple attributes or criteria must be taken into account in these circumstances in order to reach a wise and unified choice [3].
Multiple decision-makers (DMs) evaluate and rank alternatives using a variety of attributes or criteria in a decision-making process known as MAGDM. The attributes or criteria might be qualitative or quantitative, such as cost, quality, efficiency or subjective aspects. MAGDM aims to incorporate the preferences of several DMs into a collective decision or rating while taking into account each one's unique priorities, opinions and areas of skills. It entails using strategies and tactics to compile and evaluate the DMs' ideas, sometimes using mathematical or statistical methods, in order to facilitate group decision-making and find common ground or a compromise between opposing points of view [4,5,6,7,8,9,10]. MAGDM is frequently utilized in numerous fields, including business [11], management [12,13,14], engineering [15,16] and social sciences [17], where decisions often require input from multiple stakeholders with different perspectives. For the MAGDM process to be successful, the DMs must communicate, work together and coordinate effectively. However, it can also be challenging due to potential conflicts, differing opinions and the need for effective decision-making processes to account for varying perspectives. Properly executed, MAGDM can lead to better decision-making outcomes that reflect a more comprehensive consideration of various attributes and perspectives.
MAGDM problems often involve uncertain or ambiguous information, such as subjective preferences or incomplete data. Due to its capacity to handle uncertainty, linguistic modeling and assist group decision-making, DMs use fuzzy set (FS) theory proposed by Zadeeh [18] in the context of MAGDM. Classical FSs allow for the representation of uncertainty using membership degree (MD) and are capable of handling vague or ambiguous information. However, it does not explicitly capture the hesitation or uncertainty in making decisions or assigning non-membership degree (NMD) to elements. The development of an intuitionistic fuzzy set (IFS) [19] has been motivated by the need for a more comprehensive and nuanced approach to decision-making, especially in situations where DMs may have varying levels of confidence or hesitation in their judgments. It uses separate MD and NMD to represent uncertainty more precisely, with the restriction that the sum of MD and NMD must lie between zero and one. Yager [20,21] introduced Pythagorean fuzzy set (PyFS) by expanding the condition that the square sum of the MD and NMD must be within [0, 1]. Later, he generalized this idea by presenting a parameter 'q' such that the sum of the qth power of MD and qth power of NMD must lie between zero and one [22]. This kind of set was named as q-rung orthopair fuzzy set (q-ROFs). q-ROF is a more general and flexible approach to modeling uncertainty and decision-making problems.
Although, many developments have been made in providing freedom to the DMs by introducing various extended and hybrid fuzzy sets. However, there are instances in which the decision-making process becomes complicated by the involvement of abstinence, and then the DMs have no tool to tackle this type of issues. For example, in the voting system, some individuals refuse to give their opinions. Cuong and Kreinovich developed the concept of the picture fuzzy set (PFS) to address this kind of situation [23]. The authors introduced abstinence degree (AD) together with MD and NMD and defined the requirement that MD, AD and NMD added together must be less than or equal to one. PFS-based regret theory was proposed by He and Wang [24] for the evaluation of new energy vehicles from an online review. Frank t-norm and t-conform-based new operations for PFS were offered by Seikh et al. in [25]. PFS can describe AD information that IFS, PyFS and q-ROFS cannot, but it has the limitation of failing if the sum of 3 degrees exceeds one. Mahmood et al. [26] expanded the idea of the spherical fuzzy set (SFS) in this regard. Then they promoted it to the generalized form, i.e., T-spherical fuzzy set (t-SFS), in order to free DMs from the constraints of MD, AD, and ND allocation with a larger decision space and allow them to express their preferences and opinions more freely. The extended fuzzy sets discussed above are all special instances of t-SFS, which has received much attention from academics due to its generalized t-SFS without any restrictions. t-SFS have been used in several research areas, such as decision-making, pattern recognition, image processing and more. For example, in [27] the proposed method considers both the economic and environmental aspects of supplier selection. In [28], the authors proposed Archimedean aggregation operators using t-SF information and developed an MAGM technique to use it for the selection of surgical instruments. Interval-valued T-SFS-based decision-making framework was utilized by Pirbalouti et al. [29] to manage hydrogen refueling station leakage.
However, DMs frequently deal with difficult issues including ambiguous and inaccurate information. This type of information is frequently expressed in natural language, but linguistic expressions are difficult for traditional mathematical models to represent and handle. By emergence of the concept of a linguistic term set (LTS) [30,31,32] in fuzzy set theory, DMs have a tool that allows them to represent and manipulate linguistic variables in a flexible and accurate way. This implies that professionals can use linguistic terms to deal with ambiguous and imprecise information in a more natural and intuitive way and to make better decisions based on the available data. For instance, in a medical diagnosis system, the expert might want to use linguistic terms like "mild", "moderate", and "severe" to indicate the severity of a patient's symptoms. The expert can use fuzzy set operations to combine the various symptoms and make a diagnosis by associating each term with a fuzzy set, modeling the uncertainty in each term's meaning. Various fuzzy sets have been combined with LTSs. For example, Yazdi [33] explained system safety and reliability analysis using different linguistic methods under a fuzzy environment. Chen et al. [34] suggested the linguistic IFS (LIFS), which combines the IFS and LTS. Extensions of the LIFS have been suggested for some cases where the LIFS fails, such as the linguistic PYFS (LPYFS) [35], linguistic PFS [36], linguistic SFSs [37], linguistic t-SFS and linguistic interval valued t-SFSs [38,39].
Fuzzy set theory is a mathematical framework that allows for the representation of uncertainty and imprecision in data. It does this by allowing for partial membership in a set, meaning that an element can belong to a set to a degree or with a certain level of certainty, rather than simply being a member or not. Probability theory, on the other hand, is a mathematical framework for dealing with uncertainty and randomness. It allows for the quantification of the likelihood of a particular event occurring, based on available evidence or prior knowledge. By combining probability and fuzzy set theory, researchers have created more powerful models for dealing with uncertainty in complex systems. For example, in [40], the authors have assigned probability to the occurrence of each event in t-SFSs. Luo and Liu applied the probabilistic interval-valued hesitant PyFS for the selection process of project private partners [41]. Liu and Huang [42] introduced the idea of probabilistic linguistic q-rung orthopair fuzzy set and used it to construct a consensus reaching process. Xu et al. [43] extended the TOPSIS method under interval-valued probabilistic Lq-ROF information. In [44], Pang et al. introduced the concept of probabilistic linguistic term set (PLTS).
Furthermore, MAGDM techniques have been thoroughly studied using aggregation operators, similarity and distance measures, as well as some other well-known traditional decision-making methods, such as the TOPSIS method [45], VIKOR method [46], AHP method [47], multi-attributive border approximation area comparison (MABAC) method [48] and TODIM model [49,50]. These approaches indicated above have been extended by numerous researchers employing various types of fuzzy information. For example, Gurmani et al [51] extended TOPSIS method under q-rung orthopair hypersoft set. In [52], Tahir et al. designed an aggregation operator based MADM methodology. Wątróbski et al. [53] prolonged the DARIA-TOPSIS method and used it for the assessment of sustainable cities and communities. The TOPSIS technique is being extended in our study to the IVPLt-SF scenario. The TOPSIS methodology is a quick and efficient method for making decisions that aims to find the best option that is the closest to the positive ideal solution (PIS) and farthest from the negative ideal solution (NIS). Numerous academics have proposed TOPSIS adaptations for various fuzzy environments during the last few decades [54,55].
The selection of a cloud storage provider is a highly intricate matter characterized by considerable uncertainty. It should be noted that different companies offer distinct facilities based on a variety of terms and conditions. As an authority in the field, decision-making relies heavily on personal experience and skill. Given the presence of numerous uncertain factors, the evaluator faces multiple values when assessing a company's attributes, each having a different level of significance. To capture these differences in decision-making information provided by DMs, the introduction of probability serves the purpose of quantifying the significance levels. In this context, the data is presented using the IVPLt-SFS format, which incorporates interval-valued probability values that account for membership, abstinence and non-membership degrees. Even though the studies mentioned above have been proven to be useful and adaptable in a variety of situations, some DMs could choose to use interval values to convey their uncertainty and irresolution in complicated systems [56,57,58,59]. In this paper, we introduce interval values into the probability and propose the idea of interval-valued probabilistic linguistic T-spherical fuzzy set (IVPLt-SFS). As an extension of Lt-SFS, the IVPLt-SFS significantly broadens the field of information description. The IVPLt-SFS has a strong capacity to manage heterogeneous information concurrently, which makes it more versatile, adaptive and accurate. DMs have more freedom to communicate their assessment values and clearly articulate their perspectives regarding potential candidate alternatives when using IVPLt-SFSs.
The primary contributions of this study are as follows:
(1) The interval-valued probabilistic linguistic T-spherical fuzzy set (IVPLt-SFS) notion is one we put out.
(2) For IVPLt-SF information, several fundamental operations, comparison rules, Euclidean distance and two aggregation operators are defined. Additionally, some of their characteristics are also investigated.
(3) An extended IVPt-SF TOPSIS method-based MAGDM technique is designed for the selection of cloud storage provider.
(4) Finally, the comparison and sensitivity analysis is carried out, which shows the robustness and effectiveness of the proposed model.
The organization of this paper is as follow: Section 2 presents the fundamental concepts related to this paper. Section 3 is devoted to the inception of IVPLt-SFS. In Section 4, two aggregation operators for IVPLt-SFNs are presented. Section 5 is about the development of TOPSIS method-based MAGDM technique under IVPLt-SF environment. Practical example and comparison analysis is provided in Section 6. Finally, Section 7 provides a brief conclusion of the study.
In this section, some basic concept related to t-SFSs have been reviewed.
Definition 2.1. [38] Let X be a universe of discourse and S={st|t=0,1,...,τ} be a continuous linguistic term set; then a Lt-SFS is defined as,
T={⟨x,sμ(x),sη(x),sν(x)⟩|x∈X} |
where sμ(x),sη(x),sν(x)∈S are knows as linguistic membership degree, linguistic abstinence degree and linguistic non-membership degrees of the element x∈X to the set T, respectively, satisfying the constraint 0⩽μq(x)+ηq(x)+νq(x)⩽tq for a positive number q⩾1 and πT=sq√tq−(μq(x)+ηq(x)+νq(x)) is known as linguistic refusal degree of x in T. For simplicity, the triplet T=(sμ,sη,sν) is known as an Lt-SF number (Lt-SFN).
Definition 2.2. [38] Let T=(sμ,sη,sν), T1=(sμ1,sη1,sν1) and T2=(sμ2,sη2,sν2) be three Lt-SFNs and λ>0. Then, following are the Dombi operations for Lt-SFNs:
(ⅰ) T1⊕T2=(sq√tq−tq1+{(μq1tq−μq1)ξ+(μq2tq−μq2)ξ}1/1ξξ,sq√tq1+{(tq−ηq1ηq1)ξ+(tq−ηq2ηq2)ξ}1/1ξξ,sq√tq1+{(tq−νq1νq1)ξ+(tq−νq2νq2)ξ}1/1ξξ)
(ⅱ) T1⊗T2=(sq√tq1+{(tq−μq1μq1)ξ+(tq−μq2μq2)ξ}1/1ξξ,sq√tq−tq1+{(ηq1tq−ηq1)ξ+(ηq2tq−ηq2)ξ}1/1ξξ,sq√tq−tq1+{(νq1tq−νq1)ξ+(νq2tq−νq2)ξ}1/1ξξ)
(ⅲ) λ.T=(sq√tq−tq1+{λ(μqtq−μq)ξ}1/1ξξ,sq√tq1+{λ(tq−ηqηq)ξ}1/1ξξ,sq√tq1+{λ(tq−νqνq)ξ}1/1ξξ)
(ⅳ) Tλ=(sq√tq1+{λ(tq−μqμq)ξ}1/1ξξ,sq√tq−tq1+{λ(ηqtq−ηq)ξ}1/1ξξ,sq√tq−tq1+{λ(νqtq−νq)ξ}1/1ξξ)
This section presents the concept of IVPLt-SFS, beginning with its definition. It also includes algebraic operations, a comparison rule for IVLPLt-SF numbers (IVLPLt-SF) and a distance measure between them.
Definition 3.1. Let X be a universal set and S={sα|s0⩽sα⩽st,α∈[0,t]} continuous linguistic term set. An interval-valued probabilistic linguistic T-SFS (IVPLt-SFS) ˜A defined on X is expressed as
˜A={⟨x,μ˜A(x)|p˜A(x),η˜A(x)|h˜A(x),v˜A(x)|d˜A(x),⟩|x∈X} | (3.1) |
where μ˜A(x), η˜A(x), v˜A(x)∈S are three sets of some linguistic terms, which are called as LMD, LAD and LNMD of the element x∈X to ˜A respectively. p˜A(x)=[pL˜A(x),pU˜A(x)], pL˜A(x)=infp˜A(x) and pU˜A(x)=supp˜A(x); h˜A(x)=[hL˜A(x),hU˜A(x)], hL˜A(x)=infh˜A(x) and hU˜A(x)=suph˜A(x); d˜A(x)=[dL˜A(x),dU˜A(x)], dL˜A(x)=infd˜A(x) and dU˜A(x)=supd˜A(x) are three interval values, denoting the interval-valued probabilistic information of p˜A(x), h˜A(x) and d˜A(x) respectively.
Additionally, sφ,sϕ,sγ∈S, (φ+)q+(ϕ+)q+(γ+)q⩽tq for a positive integer (q⩾1), where sφ∈μ˜A(x),sϕ∈η˜A(x),sγ∈v˜A(x) and sφ+=∪sφ∈μ˜A(x)max{sφ}, sϕ+=∪sϕ∈μ˜A(x)max{sϕ} and sγ+=∪sγ∈μ˜A(x)max{sγ}, #μ,#η,#v denote the number of values in μ,η and v respectively. Here, p˜A(x),h˜A(x),d˜A(x)⊆[0,1] such that #μ∑i=1pU˜A(x)i⩽1, #η∑j=1hU˜A(x)j⩽1 and #v∑k=1dU˜A(x)k⩽1. We call the triplet ˜A={μ˜A(x)|p˜A(x),η˜A(x)|h˜A(x),v˜A(x)|d˜A(x),} an IVPLt-SF number (IVPLt-SFN) and is simply denoted as ˜a={μ|pμ,η|hη,v|dv}.
Example 1. Let S={sα|s0⩽sα⩽s7,α∈[0,7]} be LTS. Suppose that two DMs are asked to evaluate whether a healthcare provider is a good investment in terms of its potential value, they will need to conduct a comprehensive assessment of the provider's financial and operational performance. To do this, they might review financial statements, analyze operational metrics, evaluate the competitive landscape, consider future growth potential and estimate the provider's overall value based on projected cash flows. Ultimately, the DMs will draw on their knowledge and expertise to determine whether the healthcare provider is a wise investment choice. For that, the first expert considers 30–50% for a positive outcome, 10–20% for abstinence, and 20–40% for a negative outcome. To express his opinion, the decision maker uses linguistic variables, specifically "s5" to indicate a positive opinion, "s2" to indicate abstinence, and "s3" to indicate a negative opinion. In other words, the expert has some degree of uncertainty about the investment opportunity but feels confident enough to express an opinion. Using linguistic variables may reflect the decision maker's preference for qualitative or subjective assessments rather than strictly numerical or quantitative approaches. The second expert has more nuanced opinions about the investment. He considers 20–50% for a positive outcome and uses the linguistic expression "s4" to express his opinion. He also believes that there is a 30–50% chance that the investment is worth it, and he uses the linguistic expression "s6" to express this opinion. To express the abstinence degree, he chooses 10–15% by using the linguistic expression "s3" However, he still has some doubts about the investment, as they believe there is a 30–45% chance that it is not worth it, and use the linguistic expression "s2" to express this opinion. The evaluation given by the two individuals can then be described as
˜a1={{s5|[0.3,0.5]},{s2|[0.1,0.2]},{s3|[0.2,0.4]}}, and
˜a1={{s4|[0.2,0.5],s6|[0.3,0.5]},{s3|[0.1,0.15]},{s4|[0.3,0.45]}}.
We can see that the value of linguistic variable in this example is '7' and if we consider q>3 then it is easy to spot that 53+23+33=160<t3=343 and 63+33+43=307<t3=343. Which means that the constraint holds and meets the definition of IVPLt-SFS.
Remark 1. From Definition 3.2, it can be seen that
(1) When q=1, then ˜A is reduced to interval-valued probabilistic linguistic picture fuzzy set.
(2) When q=2, then ˜A is reduced to interval-valued probabilistic linguistic spherical fuzzy set.
(3) When q=1, η˜A(x)=0 then ˜A is reduced to interval-valued probabilistic linguistic intuitionistic fuzzy set.
(4) When q=2, η˜A(x)=0 then ˜A is reduced to interval-valued probabilistic linguistic Pythagorean fuzzy set.
(5) When pL˜A(x)=pU˜A(x), hL˜A(x)=hU˜A(x), and dL˜A(x)=dU˜A(x), then ˜A is reduced to probabilistic linguistic t-SFS.
Definition 3.2. Let ˜a={μ|pμ,η|hη,v|dv}, ˜a1={μ1|pμ1,η1|hη1,v1|dv1}
and ˜a2={μ2|pμ2,η2|hη2,v2|dv2} be any three IVPLt-SFNs and λ>0, then
(1)˜a1⊕˜a2=⋃sφ1∈μ1,sϕ1∈η1,sγ1∈v1sφ2∈μ2,sϕ2∈η2,sγ2∈v2{{s(φq1+φq2+φq1φq2/φq1+φq2+φq1φq2tqtq)1/1qq|[pLφ1pLφ2,pUφ1pUφ2]},{s(ϕ1ϕ2/ϕ1ϕ2tt)|[hLϕ1hLϕ2,hUϕ1hUϕ2]},(s(γ1γ2/γ1γ2tt)|[dLγ1dLγ2,dUγ1dUγ2])} | (3.2) |
(2)˜a1⊗˜a2=⋃sφ1∈μ1,sϕ1∈η1,sγ1∈v1sφ2∈μ2,sϕ2∈η2,sγ2∈v2{{s(φ1φ2/φ1φ2tt)|[pLφ2pLφ2,pUφ1pUφ2]},(s(ϕ1ϕ2/ϕ1ϕ2tt)|[dLϕ1dLϕ2,dUϕ1dUϕ2]),{s(γq1+γq2+γq1γq2/γq1+γq2+γq1γq2tqtq)1/1qq|[dLγ1dLγ2,dUγ1dUγ2]}} | (3.3) |
(3)λ˜a=⋃sφ∈μ,sϕ∈η,sγ∈v{{st(1−(1−φq/1−φqtqtq)λ)1/1qq|[pLφ,pUφ]},{st(ϕ/ϕtt)λ|[hLϕ,hUϕ]},{st(γ/γtt)λ|[dLγ,dUγ]}} | (3.4) |
(4)(˜a)λ=⋃sφ∈μ,sϕ∈η,sγ∈v{{st(φ/φtt)λ|[pLφ,pUφ]},{st(1−(1−ϕq/1−ϕqtqtq)λ)1/1qq|[hLϕ,hUϕ]},{st(1−(1−γq/1−γqtqtq)λ)1/1qq|[dLγ,dUγ]}} | (3.5) |
Theorem 3.1. Let ˜a={μ|pμ,η|hη,v|dv}, ˜a1={μ1|pμ1,η1|hη1,v1|dv1} and
˜a2={μ2|pμ2,η2|hη2,v2|dv2} be any three IVPLt-SFNs and λ,λ1,λ2>0, then we have
1) ˜a1⊕˜a2=˜a2⊕˜a1
2) ˜a1⊗˜a2=˜a2⊗˜a1
3) λ˜a2⊕λ˜a1=λ(˜a1⊕˜a2)
4) {\lambda }_{1}ã\oplus {\lambda }_{2}ã=\left({\lambda }_{1}\oplus {\lambda }_{2}\right)ã
5) {\left( {{{\tilde {\mathsf{a}}} _1} \otimes {{\tilde {\mathsf{a}}} _2}} \right)^\lambda } = {\tilde {\mathsf{a}}} _1^\lambda \otimes {\tilde {\mathsf{a}}} _2^\lambda
6) {\left( {{{\tilde {\mathsf{a}}} _1}} \right)^c} \oplus {\left( {{{\tilde {\mathsf{a}}} _2}} \right)^c} = {\left( {{{\tilde {\mathsf{a}}} _1} \oplus {{\tilde {\mathsf{a}}} _2}} \right)^c} .
Proof. The proof of (3) and (5) will be discussed in this section and the other proofs are similar.
(3) By Eq (3.4), we have
\lambda {{\tilde {\mathsf{a}}} _1} = \bigcup\limits_{{s_{{\varphi _1}}} \in {\mu _1}, {s_{{\phi _1}}} \in {\eta _1}, {s_{{\gamma _1}}} \in {v_1}} {\left\{ {\left\{ {{s_{t{{\left( {1 - {{\left( {{{1 - \varphi _1^q} \mathord{\left/ {\vphantom {{1 - \varphi _1^q} {{t^q}}}} \right. } {{t^q}}}} \right)}^\lambda }} \right)}^{{1 \mathord{\left/ {\vphantom {1 q}} \right. } q}}}}}\left| {\left[ {p_{{\varphi _1}}^L, p_{{\varphi _1}}^U} \right]} \right.} \right\}, \left\{ {{s_{t{{\left( {{{{\phi _1}} \mathord{\left/ {\vphantom {{{\phi _1}} t}} \right. } t}} \right)}^\lambda }}}\left| {\left[ {h_{{\phi _1}}^L, h_{{\phi _1}}^U} \right]} \right.} \right\}, \left\{ {{s_{t{{\left( {{{{\gamma _1}} \mathord{\left/ {\vphantom {{{\gamma _1}} t}} \right. } t}} \right)}^\lambda }}}\left| {\left[ {d_{{\gamma _1}}^L, d_{{\gamma _1}}^U} \right]} \right.} \right\}} \right\}} | (3.6) |
\begin{array}{l} \lambda {{\tilde {\mathsf{a}}} _2} = \bigcup\limits_{{s_{{\varphi _2}}} \in {\mu _2}, {s_{\phi 2}} \in {\eta _2}, {s_{{\gamma _2}}} \in {v_2}} {\left\{ {\left\{ {{s_{t{{\left( {1 - {{\left( {{{1 - \varphi _2^q} \mathord{\left/ {\vphantom {{1 - \varphi _2^q} {{t^q}}}} \right. } {{t^q}}}} \right)}^\lambda }} \right)}^{{1 \mathord{\left/ {\vphantom {1 q}} \right. } q}}}}}\left| {\left[ {p_{{\varphi _2}}^L, p_{{\varphi _2}}^U} \right]} \right.} \right\}, \left\{ {{s_{t{{\left( {{{{\phi _2}} \mathord{\left/ {\vphantom {{{\phi _2}} t}} \right. } t}} \right)}^\lambda }}}\left| {\left[ {h_{{\phi _2}}^L, h_{{\phi _2}}^U} \right]} \right.} \right\}, \left\{ {{s_{t{{\left( {{{{\gamma _2}} \mathord{\left/ {\vphantom {{{\gamma _2}} t}} \right. } t}} \right)}^\lambda }}}\left| {\left[ {d_{{\gamma _2}}^L, d_{{\gamma _2}}^U} \right]} \right.} \right\}} \right\}}\\ \lambda {{\tilde {\mathsf{a}}} _1} \oplus \lambda {{\tilde {\mathsf{a}}} _2} = \bigcup\limits_{{s_{{\varphi _1}}} \in {\mu _1}, {s_{{\phi _1}}} \in {\eta _1}, {s_{{\gamma _1}}} \in {v_1}} {\left\{ {\left\{ {{s_{t{{\left( {1 - {{\left( {{{1 - \varphi _1^q} \mathord{\left/ {\vphantom {{1 - \varphi _1^q} {{t^q}}}} \right. } {{t^q}}}} \right)}^\lambda }} \right)}^{{1 \mathord{\left/ {\vphantom {1 q}} \right. } q}}}}}\left| {\left[ {p_{{\varphi _1}}^L, p_{{\varphi _1}}^U} \right]} \right.} \right\}, \left\{ {{s_{t{{\left( {{{{\phi _1}} \mathord{\left/ {\vphantom {{{\phi _1}} t}} \right. } t}} \right)}^\lambda }}}\left| {\left[ {h_{{\phi _1}}^L, h_{{\phi _1}}^U} \right]} \right.} \right\}, \left\{ {{s_{t{{\left( {{{{\gamma _1}} \mathord{\left/ {\vphantom {{{\gamma _1}} t}} \right. } t}} \right)}^\lambda }}}\left| {\left[ {d_{{\gamma _1}}^L, d_{{\gamma _1}}^U} \right]} \right.} \right\}} \right\} \oplus } \\ \, \, \, \, \, \, \, \, \, \, \, \, \, \, \, \, \, \, \, \, \, \bigcup\limits_{{s_{{\varphi _2}}} \in {\mu _2}, {s_{\phi 2}} \in {\eta _2}, {s_{{\gamma _2}}} \in {v_2}} {\left\{ {\left\{ {{s_{t{{\left( {1 - {{\left( {{{1 - \varphi _2^q} \mathord{\left/ {\vphantom {{1 - \varphi _2^q} {{t^q}}}} \right. } {{t^q}}}} \right)}^\lambda }} \right)}^{{1 \mathord{\left/ {\vphantom {1 q}} \right. } q}}}}}\left| {\left[ {p_{{\varphi _2}}^L, p_{{\varphi _2}}^U} \right]} \right.} \right\}, \left\{ {{s_{t{{\left( {{{{\phi _2}} \mathord{\left/ {\vphantom {{{\phi _2}} t}} \right. } t}} \right)}^\lambda }}}\left| {\left[ {h_{{\phi _2}}^L, h_{{\phi _2}}^U} \right]} \right.} \right\}, \left\{ {{s_{t{{\left( {{{{\gamma _2}} \mathord{\left/ {\vphantom {{{\gamma _2}} t}} \right. } t}} \right)}^\lambda }}}\left| {\left[ {d_{{\gamma _2}}^L, d_{{\gamma _2}}^U} \right]} \right.} \right\}} \right\}} \end{array} | (3.7) |
Then, by Eq (3.2), we obtain
\lambda {{\tilde {\mathsf{a}}} _1} \oplus \lambda {{\tilde {\mathsf{a}}} _2} = \bigcup\limits_{{s_{{\varphi _i}}} \in {\mu _i}, {s_{{\phi _i}}} \in {\eta _i}, {s_{{\gamma _i}}} \in {v_i};\, \left( {i = 1, 2} \right)} {\left\{ \begin{array}{l} \left\{ {{s_{t{{\left( {1 - \prod\limits_{i = 1}^2 {{{\left( {{{1 - \varphi _i^q} \mathord{\left/ {\vphantom {{1 - \varphi _i^q} {{t^q}}}} \right. } {{t^q}}}} \right)}^\lambda }} } \right)}^{{1 \mathord{\left/ {\vphantom {1 q}} \right. } q}}}}}\left| {\left[ {\prod\limits_{i = 1}^2 {p_{{\varphi _i}}^L, \prod\limits_{i = 1}^2 {p_{{\varphi _i}}^U} } } \right]} \right.} \right\}, \\ \left\{ {{s_{t\prod\limits_{i = 1}^n {{{\left( {{{{\phi _i}} \mathord{\left/ {\vphantom {{{\phi _i}} t}} \right. } t}} \right)}^\lambda }} }}\left| {\left[ {\prod\limits_{i = 1}^2 {h_{{\phi _i}}^L, \prod\limits_{i = 1}^2 {h_{{\phi _i}}^U} } } \right]} \right.} \right\}, \left\{ {\left\{ {{s_{t\prod\limits_{i = 1}^n {{{\left( {{{{\gamma _i}} \mathord{\left/ {\vphantom {{{\gamma _i}} t}} \right. } t}} \right)}^\lambda }} }}\left| {\left[ {\prod\limits_{i = 1}^2 {d_{{\gamma _i}}^L, \prod\limits_{i = 1}^2 {d_{{\gamma _i}}^U} } } \right]} \right.} \right\}} \right\} \end{array} \right\}} |
(5) For any IVPLt-SFN {\lambda }_{1}ã\oplus {\lambda }_{2}ã=\left({\lambda }_{1}\oplus {\lambda }_{2}\right)ã , by Eq (3.4) we have
{\lambda _i}{\tilde {\mathsf{a}}} = \bigcup\limits_{{s_\varphi } \in \mu , {s_\phi } \in \eta , {s_\gamma } \in v} {\left\{ {\left\{ {{s_{t{{\left( {1 - {{\left( {{{1 - {\varphi ^q}} \mathord{\left/ {\vphantom {{1 - {\varphi ^q}} {{t^q}}}} \right. } {{t^q}}}} \right)}^{{\lambda _i}}}} \right)}^{{1 \mathord{\left/ {\vphantom {1 q}} \right. } q}}}}}\left| {\left[ {p_\varphi ^L, p_\varphi ^U} \right]} \right.} \right\}, \left\{ {{s_{t{{\left( {{\phi \mathord{\left/ {\vphantom {\phi t}} \right. } t}} \right)}^{{\lambda _i}}}}}\left| {\left[ {h_\phi ^L, h_\phi ^U} \right]} \right.} \right\}, \left\{ {{s_{t{{\left( {{\gamma \mathord{\left/ {\vphantom {\gamma t}} \right. } t}} \right)}^{{\lambda _i}}}}}\left| {\left[ {d_\gamma ^L, d_\gamma ^U} \right]} \right.} \right\}} \right\}} |
where i = 1, 2 .
Then, according to Eqs (3.2), (3.6) and (3.7)
\begin{array}{l} {\lambda _1}{\tilde {\mathsf{a}}} \oplus {\lambda _2}{\tilde {\mathsf{a}}} = \bigcup\limits_{{s_\varphi } \in \mu , {s_\phi } \in \eta , {s_\gamma } \in v} {\left\{ \begin{array}{l} \left\{ {{s_{t{{\left( {1 - \prod\limits_{i = 1}^2 {{{\left( {{{1 - \varphi _i^q} \mathord{\left/ {\vphantom {{1 - \varphi _i^q} {{t^q}}}} \right. } {{t^q}}}} \right)}^{{\lambda _i}}}} } \right)}^{{1 \mathord{\left/ {\vphantom {1 q}} \right. } q}}}}}\left| {\left[ {\prod\limits_{i = 1}^2 {p_{{\varphi _i}}^L, \prod\limits_{i = 1}^2 {p_{{\varphi _i}}^U} } } \right]} \right.} \right\}, \\ \left\{ {{s_{t\prod\limits_{i = 1}^n {{{\left( {{{{\phi _i}} \mathord{\left/ {\vphantom {{{\phi _i}} t}} \right. } t}} \right)}^{{\lambda _i}}}} }}\left| {\left[ {\prod\limits_{i = 1}^2 {h_{{\phi _i}}^L, \prod\limits_{i = 1}^2 {h_{{\phi _i}}^U} } } \right]} \right.} \right\}, \left\{ {\left\{ {{s_{t\prod\limits_{i = 1}^n {{{\left( {{{{\gamma _i}} \mathord{\left/ {\vphantom {{{\gamma _i}} t}} \right. } t}} \right)}^{{\lambda _i}}}} }}\left| {\left[ {\prod\limits_{i = 1}^2 {d_{{\gamma _i}}^L, \prod\limits_{i = 1}^2 {d_{{\gamma _i}}^U} } } \right]} \right.} \right\}} \right\} \end{array} \right\}} \\ \, \, \, \, \, \, \, \, \, \, \, \, \, \, \, \, \, \, \, \, \, = \bigcup\limits_{{s_\varphi } \in \mu , {s_\phi } \in \eta , {s_\gamma } \in v} {\left\{ {\left\{ {{s_{t{{\left( {1 - {{\left( {{{1 - {\varphi ^q}} \mathord{\left/ {\vphantom {{1 - {\varphi ^q}} {{t^q}}}} \right. } {{t^q}}}} \right)}^{{\lambda _1} + {\lambda _2}}}} \right)}^{{1 \mathord{\left/ {\vphantom {1 q}} \right. } q}}}}}\left| {\left[ {p_\varphi ^L, p_\varphi ^U} \right]} \right.} \right\}, \left\{ {{s_{t{{\left( {{\phi \mathord{\left/ {\vphantom {\phi t}} \right. } t}} \right)}^{{\lambda _1} + {\lambda _2}}}}}\left| {\left[ {h_\phi ^L, h_\phi ^U} \right]} \right.} \right\}, \left\{ {{s_{t{{\left( {{\gamma \mathord{\left/ {\vphantom {\gamma t}} \right. } t}} \right)}^{{\lambda _1} + {\lambda _2}}}}}\left| {\left[ {d_\gamma ^L, d_\gamma ^U} \right]} \right.} \right\}} \right\}}\\ \, \, \, \, \, \, \, \, \, \, \, \, \, \, \, \, \, \, \, \, \, = \left( {{\lambda _1} \oplus {\lambda _2}} \right){\tilde {\mathsf{a}}} . \end{array} |
Thus, we have shown that {\lambda }_{1}ã\oplus {\lambda }_{2}ã=\left({\lambda }_{1}\oplus {\lambda }_{2}\right)ã holds.
Definition 3.3. Let {\tilde {\mathsf{a}}} = \left\{ {\mu \left| {{p_\mu }, \eta \left| {{h_\eta }, v\left| {{d_v}} \right.} \right.} \right.} \right\} be any IVPLt-SFN defined on LTS S = \left\{ {{s_\alpha }\left| {{s_0} \leqslant {s_\alpha } \leqslant {s_t}, \alpha \in \left[ {0, t} \right]} \right.} \right\} , where {s_\varphi } \in {\mu _{\tilde {\mathsf{A}}}}\left( x \right), {s_\phi } \in {\eta _{\tilde {\mathsf{A}}}}\left( x \right), {s_\gamma } \in {v_{\tilde {\mathsf{A}}}}\left( x \right) . Then the definitions of score function SF\left( {\tilde {\mathsf{a}}} \right) and AF\left( {\tilde {\mathsf{a}}} \right) of {\tilde {\mathsf{a}}} are given as follows:
SF\left( {\tilde {\mathsf{a}}} \right) = \left( {\frac{1}{{\# \mu }}{{\sum\limits_{i = 1, {\varphi _i} \in \mu }^{\# \mu } {\left( {{\varphi _i}p_i^L} \right)} }^q} + \frac{1}{{\# \mu }}{{\sum\limits_{i = 1, {\varphi _i} \in \mu }^{\# \mu } {\left( {{\varphi _i}p_i^U} \right)} }^q}} \right) - \left( {\frac{1}{{\# v}}{{\sum\limits_{i = 1, {\gamma _i} \in v}^{\# v} {\left( {{\gamma _i}d_i^L} \right)} }^q} + \frac{1}{{\# v}}{{\sum\limits_{i = 1, {\gamma _i} \in v}^{\# v} {\left( {{\gamma _i}d_i^U} \right)} }^q}} \right) | (3.8) |
and
AF\left( {\tilde {\mathsf{a}}} \right) = \left( {\frac{1}{{\# \mu }}{{\sum\limits_{i = 1, {\varphi _i} \in \mu }^{\# \mu } {\left( {{\varphi _i}p_i^L} \right)} }^q} + \frac{1}{{\# \mu }}{{\sum\limits_{i = 1, {\varphi _i} \in \mu }^{\# \mu } {\left( {{\varphi _i}p_i^U} \right)} }^q}} \right) + \left( {\frac{1}{{\# v}}{{\sum\limits_{i = 1, {\gamma _i} \in v}^{\# v} {\left( {{\gamma _i}d_i^L} \right)} }^q} + \frac{1}{{\# v}}{{\sum\limits_{i = 1, {\gamma _i} \in v}^{\# v} {\left( {{\gamma _i}d_i^U} \right)} }^q}} \right) . | (3.9) |
Definition 3.4. Let {{\tilde {\mathsf{a}}} _1} = \left\{ {{\mu _1}\left| {{p_{{\mu _1}}}, {\eta _1}\left| {{h_{{\eta _1}}}, {v_1}\left| {{d_{{v_1}}}} \right.} \right.} \right.} \right\} and {{\tilde {\mathsf{a}}} _2} = \left\{ {{\mu _2}\left| {{p_{{\mu _2}}}, {\eta _2}\left| {{h_{{\eta _2}}}, {v_2}\left| {{d_{{v_2}}}} \right.} \right.} \right.} \right\} be any two IVPLt-SFNs, then
(1) If SF\left( {{{\tilde {\mathsf{a}}} _1}} \right) > SF\left( {{{\tilde {\mathsf{a}}} _2}} \right) , then {{\tilde {\mathsf{a}}} _1} > {{\tilde {\mathsf{a}}} _2} ;
(2) If SF\left( {{{\tilde {\mathsf{a}}} _1}} \right) < SF\left( {{{\tilde {\mathsf{a}}} _2}} \right) , then {ã}_{1}<{ã}_{2} ;
(3) If SF\left( {{{\tilde {\mathsf{a}}} _1}} \right) = SF\left( {{{\tilde {\mathsf{a}}} _2}} \right) , then there are two possible situations:
(ⅰ) If AF\left( {{{\tilde {\mathsf{a}}} _1}} \right) > AF\left( {{{\tilde {\mathsf{a}}} _2}} \right) , then {{\tilde {\mathsf{a}}} _1} > {{\tilde {\mathsf{a}}} _2} ;
(ⅱ) If AF\left( {{{\tilde {\mathsf{a}}} _1}} \right) = AF\left( {{{\tilde {\mathsf{a}}} _2}} \right) , then {ã}_{1}={ã}_{2} .
Definition 3.5. Let {{\tilde {\mathsf{a}}} _1} = \left\{ {{\mu _1}\left| {{p_{{\mu _1}}}, {\eta _1}\left| {{h_{{\eta _1}}}, {v_1}\left| {{d_{{v_1}}}} \right.} \right.} \right.} \right\} and {{\tilde {\mathsf{a}}} _2} = \left\{ {{\mu _2}\left| {{p_{{\mu _2}}}, {\eta _2}\left| {{h_{{\eta _2}}}, {v_2}\left| {{d_{{v_2}}}} \right.} \right.} \right.} \right\} be any two IVPLt-SFNs, then the Euclidean distance measure for two IVPLt-SFNs is defined as follows:
dis\left( {{{\tilde {\mathsf{a}}} _1}, {{\tilde {\mathsf{a}}} _2}} \right) = \frac{1}{{4t}}{\left( \begin{array}{l} \sum\limits_{i = 1}^{\# {\mu _1}} {\sum\limits_{j = 1}^{\# {\mu _2}} {{{\left| {\frac{{{\varphi _i}p_i^L - {\varphi _j}p_j^L}}{{\# {\mu _1}\# {\mu _2}}}} \right|}^q} + \sum\limits_{i = 1}^{\# {\mu _1}} {\sum\limits_{j = 1}^{\# {\mu _2}} {{{\left| {\frac{{{\varphi _i}p_i^U - {\varphi _j}p_j^U}}{{\# {\mu _1}\# {\mu _2}}}} \right|}^q}} } } } \\ + \sum\limits_{i = 1}^{\# {\gamma _1}} {\sum\limits_{j = 1}^{\# {\gamma _2}} {{{\left| {\frac{{{\gamma _i}d_i^L - {\gamma _j}d_j^L}}{{\# {\gamma _1}\# {\gamma _2}}}} \right|}^q} + \sum\limits_{i = 1}^{\# {\gamma _1}} {\sum\limits_{j = 1}^{\# {\gamma _2}} {{{\left| {\frac{{{\gamma _i}d_i^U - {\gamma _j}d_j^U}}{{\# {\gamma _1}\# {\gamma _2}}}} \right|}^q}} } } } \end{array} \right)^{{1 \mathord{\left/ {\vphantom {1 q}} \right. } q}}} . | (3.10) |
Aggregation operators refer to mathematical functions that are utilized to summarize or combine multiple values into a single value. They are commonly used in data analysis, decision-making and database management to compute summary statistics like counts, averages, minimum and maximum values, sums and more. These operators are useful for analyzing large datasets and can provide valuable insights into the data. Therefore, in this section, two aggregation operators have been developed, namely; IVPLt-SF weighted averaging (IVPLt-SFWA) operator and IVPLt-SF weighted geometric (IVPLt-SFGA) operator to fuse the DMs assessment information.
Definition 4.1. Let {{\tilde {\mathsf{a}}} _1} = \left\{ {{\mu _i}\left| {{p_{{\mu _i}}}, {\eta _i}\left| {{h_{{\eta _i}}}, {v_i}\left| {{d_{{v_i}}}} \right.} \right.} \right.} \right\}\left( {i = 1, 2, ..., n} \right) be a collection of IVPLt-SFNs and w = {\left( {{w_1}, {w_2}, ..., {w_n}} \right)^T} be the corresponding weight vector, such that \sum\limits_{i = 1}^n {{w_i}} = 1 and 0 \leqslant {w_i} \leqslant 1 . Then, the IVPLt-SFWA operator of dimension is a mapping IVPLt - SFWA:{\Omega ^n} \to \Omega such that
IVPLt - SFWA\left( {{{\tilde {\mathsf{a}}} _1}, {{\tilde {\mathsf{a}}} _2}, ..., {{\tilde {\mathsf{a}}} _n}} \right) = \mathop \oplus \limits_{i = 1}^n \left( {{w_i}{\alpha _i}} \right) . | (4.1) |
Theorem 4.1. Let {{\tilde {\mathsf{a}}} _1} = \left\{ {{\mu _i}\left| {{p_{{\mu _i}}}, {\eta _i}\left| {{h_{{\eta _i}}}, {v_i}\left| {{d_{{v_i}}}} \right.} \right.} \right.} \right\}\left( {i = 1, 2, ..., n} \right) be a collection of IVPLt-SFNs. Then, the aggregation results obtained by IVPLt-SFWA operator is still an IVPLt-SFNs and is expressed as,
IVPLt - SFWA\left( {{{\tilde {\mathsf{a}}} _1}, {{\tilde {\mathsf{a}}} _2}, ..., {{\tilde {\mathsf{a}}} _n}} \right) = \bigcup\limits_{{s_{{\varphi _i}}} \in {\mu _i}, {s_{{\phi _i}}} \in {\eta _i}, {s_{{\gamma _i}}} \in {v_i}} {\left\{ \begin{array}{l} \left\{ {{s_{t{{\left( {1 - \prod\limits_{i = 1}^n {{{\left( {{{1 - \varphi _i^q} \mathord{\left/ {\vphantom {{1 - \varphi _i^q} {{t^q}}}} \right. } {{t^q}}}} \right)}^{{w_i}}}} } \right)}^{{1 \mathord{\left/ {\vphantom {1 q}} \right. } q}}}}}\left| {\left[ {\prod\limits_{i = 1}^n {p_{{\varphi _i}}^L, \prod\limits_{i = 1}^n {p_{{\varphi _i}}^U} } } \right]} \right.} \right\}, \\ \left\{ {{s_{t\prod\limits_{i = 1}^n {{{\left( {{{{\phi _i}} \mathord{\left/ {\vphantom {{{\phi _i}} t}} \right. } t}} \right)}^{{w_i}}}} }}\left| {\left[ {\prod\limits_{i = 1}^n {h_{{\phi _i}}^L, \prod\limits_{i = 1}^n {h_{{\phi _i}}^U} } } \right]} \right.} \right\}, \left\{ {\left\{ {{s_{t\prod\limits_{i = 1}^n {{{\left( {{{{\gamma _i}} \mathord{\left/ {\vphantom {{{\gamma _i}} t}} \right. } t}} \right)}^{{w_i}}}} }}\left| {\left[ {\prod\limits_{i = 1}^n {d_{{\gamma _i}}^L, \prod\limits_{i = 1}^n {d_{{\gamma _i}}^U} } } \right]} \right.} \right\}} \right\} \end{array} \right\}} . | (4.2) |
Proof. According to Eq (3.4), we can get
{w_i}{\alpha _i} = \bigcup\limits_{{s_{{\varphi _i}}} \in {\mu _i}, {s_{{\phi _i}}} \in {\eta _i}, {s_{{\gamma _i}}} \in {v_i}} {\left\{ {\left\{ {{s_{t{{\left( {1 - {{\left( {{{1 - \varphi _i^q} \mathord{\left/ {\vphantom {{1 - \varphi _i^q} {{t^q}}}} \right. } {{t^q}}}} \right)}^{{w_i}}}} \right)}^{{1 \mathord{\left/ {\vphantom {1 q}} \right. } q}}}}}\left| {\left[ {p_{{\varphi _i}}^L, p_{{\varphi _i}}^U} \right]} \right.} \right\}, \left\{ {{s_{t{{\left( {{{{\phi _i}} \mathord{\left/ {\vphantom {{{\phi _i}} t}} \right. } t}} \right)}^{{w_i}}}}}\left| {\left[ {h_{{\phi _i}}^L, h_{{\phi _i}}^U} \right]} \right.} \right\}, \left\{ {{s_{t{{\left( {{{{\gamma _i}} \mathord{\left/ {\vphantom {{{\gamma _i}} t}} \right. } t}} \right)}^{{w_i}}}}}\left| {\left[ {d_{{\gamma _i}}^L, d_{{\gamma _i}}^U} \right]} \right.} \right\}} \right\}} |
then we can obtain,
\mathop \oplus \limits_{i = 1}^n \left( {{w_i}{\alpha _i}} \right) = \bigcup\limits_{{s_{{\varphi _i}}} \in {\mu _i}, {s_{{\phi _i}}} \in {\eta _i}, {s_{{\gamma _i}}} \in {v_i}} {\left\{ \begin{array}{l} \left\{ {{s_{t{{\left( {1 - \prod\limits_{i = 1}^n {{{\left( {{{1 - \varphi _i^q} \mathord{\left/ {\vphantom {{1 - \varphi _i^q} {{t^q}}}} \right. } {{t^q}}}} \right)}^{{w_i}}}} } \right)}^{{1 \mathord{\left/ {\vphantom {1 q}} \right. } q}}}}}\left| {\left[ {\prod\limits_{i = 1}^n {p_{{\varphi _i}}^L, \prod\limits_{i = 1}^n {p_{{\varphi _i}}^U} } } \right]} \right.} \right\}, \\ \left\{ {{s_{t\prod\limits_{i = 1}^n {{{\left( {{{{\phi _i}} \mathord{\left/ {\vphantom {{{\phi _i}} t}} \right. } t}} \right)}^{{w_i}}}} }}\left| {\left[ {\prod\limits_{i = 1}^n {h_{{\phi _i}}^L, \prod\limits_{i = 1}^n {h_{{\phi _i}}^U} } } \right]} \right.} \right\}, \left\{ {\left\{ {{s_{t\prod\limits_{i = 1}^n {{{\left( {{{{\gamma _i}} \mathord{\left/ {\vphantom {{{\gamma _i}} t}} \right. } t}} \right)}^{{w_i}}}} }}\left| {\left[ {\prod\limits_{i = 1}^n {d_{{\gamma _i}}^L, \prod\limits_{i = 1}^n {d_{{\gamma _i}}^U} } } \right]} \right.} \right\}} \right\} \end{array} \right\}} |
which completes the proof of Theorem 4.1.
Definition 4.2. Let {{\tilde {\mathsf{a}}} _1} = \left\{ {{\mu _i}\left| {{p_{{\mu _i}}}, {\eta _i}\left| {{h_{{\eta _i}}}, {v_i}\left| {{d_{{v_i}}}} \right.} \right.} \right.} \right\}\left( {i = 1, 2, ..., n} \right) be a collection of IVPLt-SFNs and w = {\left( {{w_1}, {w_2}, ..., {w_n}} \right)^T} be the corresponding weight vector, such that \sum\limits_{i = 1}^n {{w_i}} = 1 and 0 \leqslant {w_i} \leqslant 1 . Then, the IVPLt-SFWG operator of dimension is a mapping IVPLt - SFWG:{\Omega ^n} \to \Omega such that
IVPLt - SFWG\left( {{{\tilde {\mathsf{a}}} _1}, {{\tilde {\mathsf{a}}} _2}, ..., {{\tilde {\mathsf{a}}} _n}} \right) = \mathop \otimes \limits_{i = 1}^n {\left( {{\alpha _i}} \right)^{{w_i}}} | (4.3) |
Theorem 4.2. Let {{\tilde {\mathsf{a}}} _1} = \left\{ {{\mu _i}\left| {{p_{{\mu _i}}}, {\eta _i}\left| {{h_{{\eta _i}}}, {v_i}\left| {{d_{{v_i}}}} \right.} \right.} \right.} \right\}\left( {i = 1, 2, ..., n} \right) be a collection of IVPLt-SFNs. Then, the aggregation results obtained by the IVPLt-SFWG operator is still an IVPLt-SFNs and is expressed as,
IVPLt - SFWG\left( {{{\tilde {\mathsf{a}}} _1}, {{\tilde {\mathsf{a}}} _2}, ..., {{\tilde {\mathsf{a}}} _n}} \right) = \bigcup\limits_{{s_{{\varphi _i}}} \in {\mu _i}, {s_{{\phi _i}}} \in {\eta _i}, {s_{{\gamma _i}}} \in {v_i}} {\left\{ \begin{array}{l} \left\{ {{s_{t\prod\limits_{i = 1}^n {{{\left( {{{{\varphi _i}} \mathord{\left/ {\vphantom {{{\varphi _i}} t}} \right. } t}} \right)}^{{w_i}}}} }}\left| {\left[ {\prod\limits_{i = 1}^n {p_{{\varphi _i}}^L, \prod\limits_{i = 1}^n {p_{{\varphi _i}}^U} } } \right]} \right.} \right\}, \left\{ {{s_{t{{\left( {1 - \prod\limits_{i = 1}^n {{{\left( {{{1 - \phi _i^q} \mathord{\left/ {\vphantom {{1 - \phi _i^q} {{t^q}}}} \right. } {{t^q}}}} \right)}^{{w_i}}}} } \right)}^{{1 \mathord{\left/ {\vphantom {1 q}} \right. } q}}}}}\left| {\left[ {\prod\limits_{i = 1}^n {h_{{\phi _i}}^L, \prod\limits_{i = 1}^n {h_{{\phi _i}}^U} } } \right]} \right.} \right\}, \\ \left\{ {{s_{t{{\left( {1 - \prod\limits_{i = 1}^n {{{\left( {{{1 - \gamma _i^q} \mathord{\left/ {\vphantom {{1 - \gamma _i^q} {{t^q}}}} \right. } {{t^q}}}} \right)}^{{w_i}}}} } \right)}^{{1 \mathord{\left/ {\vphantom {1 q}} \right. } q}}}}}\left| {\left[ {\prod\limits_{i = 1}^n {d_{{\gamma _i}}^L, \prod\limits_{i = 1}^n {d_{{\gamma _i}}^U} } } \right]} \right.} \right\} \end{array} \right\}} | (4.4) |
Proof of Theorem 4.1 is similar to the Theorem 4.1, which is omitted here.
Multi-attribute group decision-making with interval valued probabilistic linguistic T-spherical fuzzy information is a complex process used to evaluate and rank multiple options based on various criteria. With this approach, DMs communicate their ideas and preferences by providing IVPLt-SF information. Decision maker's information is a representation of uncertainty that takes into consideration their level of confidence in their evaluation of the available options. Compared to conventional fuzzy sets, the IVPLt-SFS is a kind of fuzzy set that may represent information in a more adaptable and sophisticated manner. The procedure entails establishing the standards and potential solutions, gathering and combining the IVPLt-SF data and rating the potential solutions according to their overall effectiveness. To address this issue, a variety of tools and procedures are available, such as the TOPSIS, VIKOR and ELECTRE methods.
The TOPSIS (Technique for Order of Preference by Similarity to Ideal Solution) method is a decision-making approach used to rank options based on their similarity to the ideal solution and dissimilarity to the negative ideal solution. This method is commonly used for multi-criteria decision-making and can be adapted to handle the complexity of MAGDM with interval valued probabilistic linguistic T-spherical fuzzy information. In this paper, we will extend the TOPSIS method using IVPLt-SF information.
Extended TOPSIS method based methodology for MAGDM under IVPLt-SFSs
In this section, we aim to provide an MAGDM methodology based on extend TOPSIS model where the evaluation information of DMs take the form of IVPLt-SFNs.
Suppose that there are ' m ' alternatives of the form {X_i}\left( {i = 1, 2, ..., m} \right) which are to be evaluated based on ' n ' attributes {G_j}\left( {j = 1, 2, ..., n} \right) , whose weighting vector is given as \omega = {\left( {{\omega _1}, {\omega _2}, ..., {\omega _n}} \right)^T} , satisfying the constraint that \sum\limits_{j = 1}^n {{\omega _j} = 1} and 0 \leqslant {\omega _j} \leqslant 1 . Consider that there are ' K ' DMs \left\{ {{E^{\left( 1 \right)}}, {E^{\left( 2 \right)}}, ..., {E^{\left( k \right)}}} \right\} with weighting vector \left( {{\rho _1}, {\rho _2}, ..., {\rho _k}} \right) . Assume that the ' K ' DMs have evaluated the alternatives {X_i} under the attributes {G_j} and give their evaluation information in the form IVPLt-SFNs {Y^{\left( \lambda \right)}} = \left( {y_{ij}^{\left( \lambda \right)}} \right) , \lambda = 1, 2, ..., k where y_{ij}^{\left( \lambda \right)} = \left\{ {\mu _{ij}^k\left| {{p_{\mu _{ij}^k}}} \right., \eta _{ij}^k{{\left| h \right.}_{\eta _{ij}^k}}, v_{ij}^k\left| {{d_{v_{ij}^k}}} \right.} \right\} . The TOPSIS technique is expanded to the IVPLt-SF scenario in order to determine the best option in the decision-making issue (Figure 1). With all of the given information, the computation procedure can be shown in the following.
Step 1. Get the individual assessments of the DMs in the form of IVPLt-SF setting.
{Y^{\left( \lambda \right)}} = \left( {y_{ij}^{\left( \lambda \right)}} \right) , \lambda = 1, 2, ..., k | (5.1) |
where
y_{ij}^{\left( \lambda \right)} = \left\{ {\mu _{ij}^k\left| {{p_{\mu _{ij}^k}}} \right., \eta _{ij}^k{{\left| h \right.}_{\eta _{ij}^k}}, v_{ij}^k\left| {{d_{v_{ij}^k}}} \right.} \right\} . |
Step 2. The second step involves aggregating all the information the DMs provided into a collective one using an IVPLt-SFWA operator. According to Eq (4.2):
{y_{ij}} = IVPLt - SFWA\left( {y_{ij}^{\left( 1 \right)}, y_{ij}^{\left( 2 \right)}, ..., y_{ij}^{\left( k \right)}} \right) = \mathop { \oplus \, \, }\limits_{\lambda = 1}^k {\rho _\lambda }y_{ij}^{\left( \lambda \right)} . | (5.2) |
Step 3. Normalize the group IVPLt-SF decision matrix. If all the criteri are of the same type, then do nothing; if the criterion is cost type, then the cost type criterion should be converted into a benefit type. The normalized group decision matrix is shown as follow:
Y = {y_{ij}} = \left\{ {\mu _{ij}^k\left| {{p_{\mu _{ij}^k}}} \right., \eta _{ij}^k{{\left| h \right.}_{\eta _{ij}^k}}, v_{ij}^k\left| {{d_{v_{ij}^k}}} \right.} \right\} = \left\{ \begin{array}{l} \left\{ {\mu _{ij}^k\left| {{p_{\mu _{ij}^k}}} \right., \eta _{ij}^k{{\left| h \right.}_{\eta _{ij}^k}}, v_{ij}^k\left| {{d_{v_{ij}^k}}} \right.} \right\}, \, \, for\, \, benefit\, \, type\, \, {G_j} \\ \left\{ {v_{ij}^k\left| {{d_{v_{ij}^k}}} \right., \eta _{ij}^k{{\left| h \right.}_{\eta _{ij}^k}}, \mu _{ij}^k\left| {{p_{\mu _{ij}^k}}} \right.} \right\}, \, \, for\, \, \cos t\, \, type\, \, {G_j} \end{array} \right. . | (5.3) |
Step 4. The weighted collective decision matrix should be computed in the third stage utilizing the attribute weights. According to Eq (3.7):
\overline Y = {\left( {\overline {{y_{ij}}} } \right)_{m \times n}} = {\left( {{\omega _j}{y_{ij}}} \right)_{m \times n}} | (5.4) |
where \left( {i = 1, 2, ..., m\, ;\, j = 1, 2, ..., n} \right) .
Step 5. In the third step, we must use the following equations to get the IVPLt-SF positive ideal solution (IVPLt-SF-PIS) y_j^ + and IVPLt-SF negative ideal solution (IVPLt-SF-NIS) y_j^ - by using the following equations:
\begin{array}{l} y_j^ + = \left\{ {{\tilde {\mathsf{a}}} _1^ + , {\tilde {\mathsf{a}}} _2^ + , ...{\tilde {\mathsf{a}}} _n^ + } \right\} \\ = \left\{ {\left\{ {\max SF\left( {{{\tilde {\mathsf{a}}} _{i1}}} \right)} \right\}, \left\{ {\max SF\left( {{{\tilde {\mathsf{a}}} _{i2}}} \right)} \right\}, ..., \left\{ {\max SF\left( {{{\tilde {\mathsf{a}}} _{in}}} \right)} \right\}} \right\} \end{array} | (5.5) |
\begin{array}{l} y_j^ - = \left\{ {{\tilde {\mathsf{a}}} _1^ - , {\tilde {\mathsf{a}}} _2^ - , ...{\tilde {\mathsf{a}}} _n^ - } \right\} \\ = \left\{ {\left\{ {\min SF\left( {{{\tilde {\mathsf{a}}} _{i1}}} \right)} \right\}, \left\{ {\min SF\left( {{{\tilde {\mathsf{a}}} _{i2}}} \right)} \right\}, ..., \left\{ {\min SF\left( {{{\tilde {\mathsf{a}}} _{in}}} \right)} \right\}} \right\} \end{array} | (5.6) |
where \left( {i = 1, 2, ..., m\, ;\, j = 1, 2, ..., n} \right) .
Step 6. The distances between each alternative with PIS and NIS are calculated based on Euclidean distance measure by using Eq (3.10):
\beta _i^ + = \sum\limits_{j = 1}^n {d\left( {\overline {{y_{ij}}} , y_j^ + } \right)} ; | (5.7) |
\beta _i^ - = \sum\limits_{j = 1}^n {d\left( {\overline {{y_{ij}}} , y_j^ - } \right)} | (5.8) |
where \left( {i = 1, 2, ...m} \right) and \left( {j = 1, 2, ...n} \right)
Step 7. Now, we will calculate the closeness ratio {\Xi _i}\left( {i = 1, 2, ...m} \right) of the alternatives by using the following equation:
{\Xi _i} = \frac{{\beta _i^ - }}{{\beta _i^ + + \beta _i^ - }} . | (5.9) |
Step 8. Rank all the alternatives in descending order. The best alternative will be the one with greater values.
In the following part, a numerical example and a comparison with existing techniques are provided to further elucidate the applicability of IVPLt-SFSs:
Cloud storage providers offer a third-party service that allows companies to store their digital data, such as files, documents, images and videos on remote servers that are hosted by the provider. This type of storage enables companies to store and access their data over the internet from anywhere, using various devices such as computers, smartphones and tablets. Using cloud storage can provide several benefits to companies, including scalability, accessibility, cost savings, security and collaboration features. Cloud storage providers offer scalable storage capacity, which means that companies can adjust their storage needs based on their business requirements. They also offer easy access to data from anywhere and anytime, which is convenient for remote teams or employees. Using cloud storage can also save companies money, as they do not have to invest in expensive hardware or maintenance. Cloud storage providers offer several security features to protect data from unauthorized access or loss, including data encryption, access controls and disaster recovery mechanisms. Finally, cloud storage providers offer collaboration features that enable teams to work together more effectively, regardless of their location.
When choosing a cloud storage provider, there are several criteria that companies should consider. Let us consider that a company invited three DMs D = \left\{ {{D^{\left( 1 \right)}}, {D^{\left( 2 \right)}}, {D^{\left( 3 \right)}}} \right\} with different knowledge and experiences having weighting vector \rho = \left( {0.36, 0.24, 0.4} \right) to review some world-renown cloud storage providers. These DMs short-listed three alternatives:
{X_1} - Amazon\;Web\;Services ; {X_2} - Alibaba\;Cloud ; and {X_3} - Tencent\;Cloud
The four effective attributes they settled on and used to the company's selection procedure are explained as follows:
Storage capacity and scalability \left( {{Z_1}} \right) : The quantity of storage space, the business needs, and the provider's ability to grow storage capacity are two key factors to consider when choosing a cloud storage provider. This implies that companies should evaluate their current and future demands for data storage and select a supplier that can satisfy those needs. It is crucial to choose a supplier that will allow the organization to increase its storage capacity without moving providers or buying new gear. By only paying for the storage space they actually use, scalability may help businesses save money. It is also vital to consider the provider's pricing strategy because some use pay-as-you-go or tiered pricing. Ultimately, choosing a cloud storage provider with the appropriate scalability and storage capacity helps ensure that the business has the space to retain its data and can quickly adjust its storage requirements as it expands.
Accessibility \left( {{Z_2}} \right) : The second aspect to consider when selecting a cloud storage provider is accessibility. A provider who offers easy accessibility may boost productivity by enabling customers to access data from any place and on any device. Businesses should look for service providers who offer durable connections, nimble online and mobile applications and reliable data transfer methods. Consideration must be given to the provider's ability to integrate applications while maintaining ease of access.
Security \left( {{Z_3}} \right) : The third aspect to consider when selecting a cloud storage provider is security. Businesses should seek service providers with robust security processes, such as encryption, access controls, data backups and disaster recovery plans. Additionally, service providers must adhere to rules particular to their business and have a transparent data privacy policy. The security, integrity and accessibility of data stored on the cloud platform must all be guaranteed.
Vendor Stability \left( {{Z_4}} \right) : Vendor stability is a term used to describe a cloud storage provider's financial stability, standing and durability. When choosing a cloud storage service, it's crucial to consider stability. This entails evaluating their financial standing, performance history and standing within their sector. A recognized, financially secure supplier is more likely to provide dependable services and has the funds to make changes. The likelihood of unexpected disruptions or the requirement for data migration is decreased by longevity in the market, which also shows their dedication. Assessing vendor stability aids in ensuring your firm receives dependable and trustworthy cloud storage services.
The decision hierarchy of the cloud storage provider selection problem is further explained in Figure 2.
These four attributes explained above have weighting vector w = \left( {0.10, 0.30, 0.15, 0.45} \right) , and the evaluation matrices provided by DMs are {R^{\left( 1 \right)}} , {R^{\left( 2 \right)}} and {R^{\left( 3 \right)}} , which are expressed in the form of IVPLt-SFS with t = 7 and q = 3 , are given in Tables 1–3. In Section 5, the MAGDM technique is proposed as a way to determine the optimal alternative by aggregating the opinions of multiple DMs. The technique involves various computational processes, and the findings and outcomes are outlined and discussed below.
{Z_1} | |
{X_1} | \left\{ {\left\{ {{s_4}\left| {\left[ {.3, .5} \right]} \right.} \right\}, \left\{ {{s_5}\left| {\left[ {.1, .2} \right]} \right.} \right\}, \left\{ {{s_2}\left| {\left[ {.2, .4} \right]} \right.} \right\}} \right\} |
{X_2} | \left\{ {\left\{ {{s_3}\left| {\left[ {.1, .5} \right]} \right., {s_6}\left| {\left[ {.4, .5} \right]} \right.} \right\}, \left\{ {{s_1}\left| {\left[ {.1, .3} \right]} \right.} \right\}, \left\{ {{s_4}\left| {\left[ {.2, .6} \right]} \right.} \right\}} \right\} |
{X_3} | \left\{ {\left\{ {{s_4}\left| {\left[ {.1, .8} \right]} \right.} \right\}, \left\{ {{s_3}\left| {\left[ {.3, .4} \right]} \right.} \right\}, \left\{ {{s_5}\left| {\left[ {.2, .8} \right]} \right.} \right\}} \right\} |
{Z_2} | |
{X_1} | \left\{ {\left\{ {{s_2}\left| {\left[ {.2, .6} \right]} \right.} \right\}, \left\{ {{s_3}\left| {\left[ {.5, .7} \right]} \right.} \right\}, \left\{ {{s_1}\left| {\left[ {.1, .5} \right]} \right.} \right\}} \right\} |
{X_2} | \left\{ {\left\{ {{s_4}\left| {\left[ {.5, .7} \right]} \right.} \right\}, \left\{ {{s_1}\left| {\left[ {.2, .4} \right]} \right.} \right\}, \left\{ {{s_3}\left| {\left[ {.5, .7} \right]} \right.} \right\}} \right\} |
{X_3} | \left\{ {\left\{ {{s_2}\left| {\left[ {.4, .7} \right]} \right.} \right\}, \left\{ {{s_1}\left| {\left[ {.2, .4} \right]} \right., {s_6}\left| {\left[ {.3, .6} \right]} \right.} \right\}, \left\{ {{s_4}\left| {\left[ {.1, .3} \right]} \right.} \right\}} \right\} |
{Z_3} | |
{X_1} | \left\{ {\left\{ {{s_6}\left| {\left[ {.3, .4} \right]} \right.} \right\}, \left\{ {{s_4}\left| {\left[ {.2, .5} \right]} \right.} \right\}, \left\{ {{s_1}\left| {\left[ {.1, .9} \right]} \right.} \right\}} \right\} |
{X_2} | \left\{ {\left\{ {{s_3}\left| {\left[ {.2, .5} \right]} \right.} \right\}, \left\{ {{s_4}\left| {\left[ {.4, .6} \right]} \right.} \right\}, \left\{ {{s_4}\left| {\left[ {.3, .4} \right]} \right., {s_5}\left| {\left[ {.3, .6} \right]} \right.} \right\}} \right\} |
{X_3} | \left\{ {\left\{ {{s_2}\left| {\left[ {.6, .7} \right]} \right.} \right\}, \left\{ {{s_1}\left| {\left[ {.3, .6} \right]} \right.} \right\}, \left\{ {{s_6}\left| {\left[ {.2, .4} \right]} \right.} \right\}} \right\} |
{Z_4} | |
{X_1} | \left\{ {\left\{ {{s_3}\left| {\left[ {.2, .5} \right]} \right.} \right\}, \left\{ {{s_4}\left| {\left[ {.1, .6} \right], {s_6}\left| {\left[ {.2, .4} \right]} \right.} \right.} \right\}, \left\{ {{s_1}\left| {\left[ {.1, .3} \right]} \right., {s_2}\left| {\left[ {.1, .4} \right]} \right.} \right\}} \right\} |
{X_2} | \left\{ {\left\{ {{s_4}\left| {\left[ {.4, .6} \right]} \right.} \right\}, \left\{ {{s_2}\left| {\left[ {.4, .7} \right], } \right.} \right\}, \left\{ {{s_3}\left| {\left[ {.3, .8} \right]} \right.} \right\}} \right\} |
{X_3} | \left\{ {\left\{ {{s_4}\left| {\left[ {.4, .7} \right]} \right.} \right\}, \left\{ {{s_3}\left| {\left[ {.1, .4} \right]} \right.} \right\}, \left\{ {{s_5}\left| {\left[ {.2, .6} \right]} \right.} \right\}} \right\} |
{Z_1} | |
{X_1} | \left\{ {\left\{ {{s_5}\left| {\left[ {.3, .5} \right]} \right.} \right\}, \left\{ {{s_4}\left| {\left[ {.2, .3} \right]} \right.} \right\}, \left\{ {{s_2}\left| {\left[ {.4, .5} \right]} \right.} \right\}} \right\} |
{X_2} | \left\{ {\left\{ {{s_4}\left| {\left[ {.4, .5} \right]} \right.} \right\}, \left\{ {{s_2}\left| {\left[ {.1, .3} \right]} \right.} \right\}, \left\{ {{s_5}\left| {\left[ {.5, .6} \right]} \right.} \right\}} \right\} |
{X_3} | \left\{ {\left\{ {{s_4}\left| {\left[ {.2, .7} \right]} \right.} \right\}, \left\{ {{s_3}\left| {\left[ {.5, .8} \right]} \right.} \right\}, \left\{ {{s_2}\left| {\left[ {.4, .5} \right]} \right.} \right\}} \right\} |
{Z_2} | |
{X_1} | \left\{ {\left\{ {{s_3}\left| {\left[ {.2, .6} \right]} \right., {s_4}\left| {\left[ {.2, .6} \right]} \right.} \right\}, \left\{ {{s_3}\left| {\left[ {.2, .4} \right]} \right.} \right\}, \left\{ {{s_4}\left| {\left[ {.3, .5} \right]} \right.} \right\}} \right\} |
{X_2} | \left\{ {\left\{ {{s_4}\left| {\left[ {.2, .45} \right]} \right.} \right\}, \left\{ {{s_2}\left| {\left[ {.2, .25} \right]} \right.} \right\}, \left\{ {{s_5}\left| {\left[ {.1, .2} \right]} \right.} \right\}} \right\} |
{X_3} | \left\{ {\left\{ {{s_6}\left| {\left[ {.3, .6} \right]} \right.} \right\}, \left\{ {{s_2}\left| {\left[ {.2, .4} \right]} \right.} \right\}, \left\{ {{s_4}\left| {\left[ {.3, .6} \right]} \right.} \right\}} \right\} |
{Z_3} | |
{X_1} | \left\{ {\left\{ {{s_6}\left| {\left[ {.3, .4} \right]} \right.} \right\}, \left\{ {{s_4}\left| {\left[ {.2, .5} \right]} \right.} \right\}, \left\{ {{s_1}\left| {\left[ {.1, .9} \right]} \right.} \right\}} \right\} |
{X_2} | \left\{ {\left\{ {{s_5}\left| {\left[ {.3, .4} \right]} \right.} \right\}, \left\{ {{s_1}\left| {\left[ {.1, .3} \right]} \right.} \right\}, \left\{ {{s_4}\left| {\left[ {.3, .4} \right]} \right.} \right\}} \right\} |
{X_3} | \left\{ {\left\{ {{s_5}\left| {\left[ {.3, .5} \right]} \right.} \right\}, \left\{ {{s_3}\left| {\left[ {.4, .5} \right]} \right.} \right\}, \left\{ {{s_3}\left| {\left[ {.1, .6} \right]} \right.} \right\}} \right\} |
{Z_4} | |
{X_1} | \left\{ {\left\{ {{s_4}\left| {\left[ {.4, .7} \right]} \right.} \right\}, \left\{ {{s_3}\left| {\left[ {.1, .4} \right]} \right.} \right\}, \left\{ {{s_5}\left| {\left[ {.2, .6} \right]} \right.} \right\}} \right\} |
{X_2} | \left\{ {\left\{ {{s_1}\left| {\left[ {0.4, 0.5} \right], {s_4}\left| {\left[ {0.2, 0.5} \right]} \right.} \right.} \right\}, \left\{ {{s_2}\left| {\left[ {0.1, 0.3} \right]} \right.} \right\}, \left\{ {{s_5}\left| {\left[ {0.5, 0.6} \right]} \right.} \right\}} \right\} |
{X_3} | \left\{ {\left\{ {{s_2}\left| {\left[ {.1, .5} \right]} \right.} \right\}, \left\{ {{s_3}\left| {\left[ {.2, .4} \right]} \right.} \right\}, \left\{ {{s_2}\left| {\left[ {.3, .8} \right]} \right.} \right\}} \right\} |
{Z_1} | |
{X_1} | \left\{ {\left\{ {{s_5}\left| {\left[ {.4, .5} \right]} \right., {s_6}\left| {\left[ {.3, .5} \right]} \right.} \right\}, \left\{ {{s_2}\left| {\left[ {.3, .9} \right]} \right.} \right\}, \left\{ {{s_6}\left| {\left[ {.3, .6} \right]} \right.} \right\}} \right\} |
{X_2} | \left\{ {\left\{ {{s_4}\left| {\left[ {.2, .45} \right]} \right.} \right\}, \left\{ {{s_4}\left| {\left[ {.2, .25} \right]} \right.} \right\}, \left\{ {{s_2}\left| {\left[ {.1, 0.7} \right], {s_5}\left| {\left[ {.2, 0.3} \right]} \right.} \right.} \right\}} \right\} |
{X_3} | \left\{ {\left\{ {{s_5}\left| {\left[ {.1, .2} \right]} \right.} \right\}, \left\{ {{s_3}\left| {\left[ {.4, .5} \right]} \right.} \right\}, \left\{ {{s_6}\left| {\left[ {.6, .9} \right]} \right.} \right\}} \right\} |
{Z_2} | |
{X_1} | \left\{ {\left\{ {{s_3}\left| {\left[ {.2, .45} \right], {s_5}\left| {\left[ {.4, .55} \right]} \right.} \right.} \right\}, \left\{ {{s_3}\left| {\left[ {.2, .6} \right]} \right.} \right\}, \left\{ {{s_6}\left| {\left[ {.7, .9} \right]} \right.} \right\}} \right\} |
{X_2} | \left\{ {\left\{ {{s_2}\left| {\left[ {.3, .6} \right]} \right.} \right\}, \left\{ {{s_6}\left| {\left[ {.4, .5} \right]} \right.} \right\}, \left\{ {{s_3}\left| {\left[ {.3, .9} \right]} \right.} \right\}} \right\} |
{X_3} | \left\{ {\left\{ {{s_5}\left| {\left[ {.2, .4} \right]} \right.} \right\}, \left\{ {{s_3}\left| {\left[ {.3, .2} \right], {s_4}\left| {\left[ {.1, .8} \right]} \right.} \right.} \right\}, \left\{ {{s_6}\left| {\left[ {.3, .6} \right]} \right.} \right\}} \right\} |
{Z_3} | |
{X_1} | \left\{ {\left\{ {{s_3}\left| {\left[ {.3, .6} \right]} \right., {s_5}\left| {\left[ {.2, .4} \right]} \right.} \right\}, \left\{ {{s_2}\left| {\left[ {.2, .5} \right]} \right.} \right\}, \left\{ {{s_1}\left| {\left[ {.4, .7} \right]} \right.} \right\}} \right\} |
{X_2} | \left\{ {\left\{ {{s_3}\left| {\left[ {.3, .5} \right]} \right.} \right\}, \left\{ {{s_3}\left| {\left[ {.1, .4} \right]} \right.} \right\}, \left\{ {{s_4}\left| {\left[ {.4, .6} \right]} \right.} \right\}} \right\} |
{X_3} | \left\{ {\left\{ {{s_6}\left| {\left[ {.3, .6} \right]} \right.} \right\}, \left\{ {{s_2}\left| {\left[ {.2, .4} \right]} \right.} \right\}, \left\{ {{s_4}\left| {\left[ {.3, .6} \right]} \right.} \right\}} \right\} |
{Z_4} | |
{X_1} | \left\{ {\left\{ {{s_3}\left| {\left[ {.5, .6} \right]} \right.} \right\}, \left\{ {{s_1}\left| {\left[ {.3, .4} \right]} \right.} \right\}, \left\{ {{s_4}\left| {\left[ {.4, .6} \right]} \right.} \right\}} \right\} |
{X_2} | \left\{ {\left\{ {{s_5}\left| {\left[ {.3, .5} \right], {s_6}\left| {\left[ {.3, .4} \right]} \right.} \right.} \right\}, \left\{ {{s_3}\left| {\left[ {.1, .4} \right]} \right.} \right\}, \left\{ {{s_4}\left| {\left[ {.3, .5} \right]} \right.} \right\}} \right\} |
{X_3} | \left\{ {\left\{ {{s_3}\left| {\left[ {.4, .5} \right]} \right.} \right\}, \left\{ {{s_5}\left| {\left[ {.2, .5} \right]} \right.} \right\}, \left\{ {{s_4}\left| {\left[ {.5, .6} \right]} \right.} \right\}} \right\} |
Step 1. The three DMs evaluate the short-listed alternatives \left\{ {{X_1}, {X_2}, {X_3}} \right\} and gave their assessment in the form of IVPLt-SFN, shown in Tables 1–3.
Step 2. By using the weights of DMs and Eqs (4.2) and (5.2), the collective decision matrix is given in Table 4.
{Z_1} | |
{X_1} | \left\{ {\left\{ {{s_{4.714}}\left| {\left[ {0.036, 0.125} \right], {s_{5.3258}}\left| {\left[ {0.027, 0.125} \right]} \right.} \right.} \right\}, \left\{ {{s_{3.285}}\left| {\left[ {0.006, 0.054} \right]} \right.} \right\}, \left\{ {{s_{3.1037}}\left| {\left[ {0.024, 0.12} \right]} \right.} \right\}} \right\} |
{X_2} | \left\{ \begin{array}{l} \left\{ {{s_{3.7135}}\left| {\left[ {0.008, 0.1125} \right], {s_{5.1024}}\left| {\left[ {0.032, 0.1125} \right]} \right.} \right.} \right\}, \left\{ {{s_{3.9193}}\left| {\left[ {0.001, 0.063} \right]} \right.} \right\}, \\ \left\{ {{s_{3.1982}}\left| {\left[ {0.01, 0.252} \right], {s_{4.6141}}\left| {\left[ {0.02, 0.108} \right]} \right.} \right.} \right\} \\ \end{array} \right\} |
{X_3} | \left\{ {\left\{ {{s_{4.485}}\left| {\left[ {0.002, 0.112} \right]} \right.} \right\}, \left\{ {{s_3}\left| {\left[ {0.06, 0.16} \right]} \right.} \right\}, \left\{ {{s_{4.3166}}\left| {\left[ {0.048, 0.36} \right]} \right.} \right\}} \right\} |
{Z_2} | |
{X_1} | \left\{ \begin{array}{l} \left\{ {{s_{2.7274}}\left| {\left[ {0.008, 0.162} \right], {s_{3.1}}\left| {\left[ {0.008, 0.162} \right]} \right., } \right.{s_{4.017}}\left| {\left[ {0.016, 0.198} \right], {s_{4.1796}}\left| {\left[ {0.016, 0.198} \right]} \right.} \right.} \right\} \\ \left\{ {{s_3}\left| {\left[ {0.02, 0.168} \right]} \right.} \right\}, \left\{ {{s_{2.856}}\left| {\left[ {0.021, 0.225} \right]} \right.} \right\} \\ \end{array} \right\} |
{X_2} | \left\{ {\left\{ {{s_{3.4985}}\left| {\left[ {0.03, 0.189} \right]} \right.} \right\}, \left\{ {{s_{2.4183}}\left| {\left[ {0.016, 0.05} \right]} \right.} \right\}, \left\{ {{s_{3.3913}}\left| {\left[ {0.015, 0.126} \right]} \right.} \right\}} \right\} |
{X_3} | \left\{ {\left\{ {{s_{4.9253}}\left| {\left[ {0.024, 0.168} \right]} \right.} \right\}, \left\{ \begin{array}{l} {s_{1.8327}}\left| {\left[ {0.012, 0.032} \right], {s_{3.4933}}\left| {\left[ {0.018, 0.048} \right]} \right., } \right. \\ {s_{2.0562}}\left| {\left[ {0.004, 0.128} \right], {s_{3.9193}}\left| {\left[ {0.006, 0.192} \right]} \right.} \right. \\ \end{array} \right\}, \left\{ {{s_{4.7043}}\left| {\left[ {0.009, 0.108} \right]} \right.} \right\}} \right\} |
{Z_3} | |
{X_1} | \left\{ {\left\{ {{s_{5.4302}}\left| {\left[ {0.027, 0.096} \right], {s_{5.7017}}\left| {\left[ {0.018, 0.064} \right]} \right.} \right.} \right\}, \left\{ {{s_{3.0314}}\left| {\left[ {0.008, 0.125} \right]} \right.} \right\}, \left\{ {{s_1}\left| {\left[ {0.004, 0.567} \right]} \right.} \right\}} \right\} |
{X_2} | \left\{ {\left\{ {{s_{3.7783}}\left| {\left[ {0.018, 0.1} \right]} \right.} \right\}, \left\{ {{s_{2.5562}}\left| {\left[ {0.004, 0.072} \right]} \right.} \right\}, \left\{ {{s_4}\left| {\left[ {0.036, 0.096} \right], {s_{4.3346}}\left| {\left[ {0.036, 0.144} \right]} \right.} \right.} \right\}} \right\} |
{X_3} | \left\{ {\left\{ {{s_{5.1676}}\left| {\left[ {0.054, 0.21} \right]} \right.} \right\}, \left\{ {{s_{1.7176}}\left| {\left[ {0.024, 0.12} \right]} \right.} \right\}, \left\{ {{s_{4.3198}}\left| {\left[ {0.006, 0.144} \right]} \right.} \right\}} \right\} |
{Z_4} | |
{X_1} | \left\{ \begin{array}{l} \left\{ {{s_{3.3112}}\left| {\left[ {0.04, 0.21} \right]} \right.} \right\}, \left\{ {{s_{2.1441}}\left| {\left[ {0.003, 0.096} \right], {s_{2.4811}}\left| {\left[ {0.006, 0.064} \right]} \right.} \right.} \right\}, \\ \left\{ {{s_{2.562}}\left| {\left[ {0.008, 0.108} \right], {s_{3.2881}}\left| {\left[ {0.008, 0.144} \right]} \right.} \right.} \right\} \\ \end{array} \right\} |
{X_2} | \left\{ \begin{array}{l} \left\{ {{s_{4.2646}}\left| {\left[ {0.048, 0.15} \right], {s_{4.485}}\left| {\left[ {0.024, 0.15} \right]} \right.} \right., {s_{5.0548}}\left| {\left[ {0.048, 0.12} \right]} \right., {s_{5.1844}}\left| {\left[ {0.024, 0.12} \right]} \right.} \right\}, \\ \left\{ {{s_{2.3522}}\left| {\left[ {0.004, 0.084} \right]} \right.} \right\}, \left\{ {{s_{3.8049}}\left| {\left[ {0.045, 0.24} \right]} \right.} \right\} \\ \end{array} \right\} |
{X_3} | \left\{ {\left\{ {{s_{3.3199}}\left| {\left[ {0.016, 0.175} \right]} \right.} \right\}, \left\{ {{s_{3.6801}}\left| {\left[ {0.004, 0.08} \right]} \right.} \right\}, \left\{ {{s_{3.6703}}\left| {\left[ {0.03, 0.288} \right]} \right.} \right\}} \right\} |
Step 3. All the attributes are of the same type. Therefore, there is no need for normalization. We will proceed to the next step.
Step 3. By using attributes weight and Eqs (3.7) and (5.4), we compute a weighted aggregated decision matrix which is given in Table 5.
{Z_1} | |
{X_1} | \left\{ {\left\{ {{s_{2.3068}}\left| {\left[ {0.036, 0.125} \right], {s_{2.6845}}\left| {\left[ {0.027, 0.125} \right]} \right.} \right.} \right\}, \left\{ {{s_{6.49}}\left| {\left[ {0.006, 0.054} \right]} \right.} \right\}, \left\{ {{s_{6.4532}}\left| {\left[ {0.024, 0.12} \right]} \right.} \right\}} \right\} |
{X_2} | \left\{ \begin{array}{l} \left\{ {{s_{1.7653}}\left| {\left[ {0.008, 0.1125} \right], {s_{2.5405}}\left| {\left[ {0.032, 0.1125} \right]} \right.} \right.} \right\}, \left\{ {{s_{6.6055}}\left| {\left[ {0.001, 0.063} \right]} \right.} \right\}, \\ \left\{ {{s_{6.4726}}\left| {\left[ {0.01, 0.252} \right], {s_{6.7142}}\left| {\left[ {0.02, 0.108} \right]} \right.} \right.} \right\} \\ \end{array} \right\} |
{X_3} | \left\{ {\left\{ {{s_{2.1765}}\left| {\left[ {0.002, 0.112} \right]} \right.} \right\}, \left\{ {{s_{6.4313}}\left| {\left[ {0.06, 0.16} \right]} \right.} \right\}, \left\{ {{s_{6.6696}}\left| {\left[ {0.048, 0.36} \right]} \right.} \right\}} \right\} |
{Z_2} | |
{X_1} | \left\{ \begin{array}{l} \left\{ {{s_{1.8387}}\left| {\left[ {0.008, 0.162} \right], {s_{2.0971}}\left| {\left[ {0.008, 0.162} \right]} \right., } \right.{s_{2.7541}}\left| {\left[ {0.016, 0.198} \right], {s_{2.8751}}\left| {\left[ {0.016, 0.198} \right]} \right.} \right.} \right\} \\ \left\{ {{s_{5.4288}}\left| {\left[ {0.02, 0.168} \right]} \right.} \right\}, \left\{ {{s_{5.3493}}\left| {\left[ {0.021, 0.225} \right]} \right.} \right\} \\ \end{array} \right\} |
{X_2} | \left\{ {\left\{ {{s_{2.3782}}\left| {\left[ {0.03, 0.189} \right]} \right.} \right\}, \left\{ {{s_{5.0889}}\left| {\left[ {0.016, 0.05} \right]} \right.} \right\}, \left\{ {{s_{5.6322}}\left| {\left[ {0.015, 0.126} \right]} \right.} \right\}} \right\} |
{X_3} | \left\{ {\left\{ {{s_{3.4581}}\left| {\left[ {0.024, 0.168} \right]} \right.} \right\}, \left\{ \begin{array}{l} {s_{4.6827}}\left| {\left[ {0.012, 0.032} \right], {s_{5.6825}}\left| {\left[ {0.018, 0.048} \right]} \right., } \right. \\ {s_{4.8472}}\left| {\left[ {0.004, 0.128} \right], {s_{5.8821}}\left| {\left[ {0.006, 0.192} \right]} \right.} \right. \\ \end{array} \right\}, \left\{ {{s_{6.2132}}\left| {\left[ {0.009, 0.108} \right]} \right.} \right\}} \right\} |
{Z_3} | |
{X_1} | \left\{ {\left\{ {{s_{3.1373}}\left| {\left[ {0.027, 0.096} \right], {s_{3.3547}}\left| {\left[ {0.018, 0.064} \right]} \right.} \right.} \right\}, \left\{ {{s_{6.1742}}\left| {\left[ {0.008, 0.125} \right]} \right.} \right\}, \left\{ {{s_{5.228}}\left| {\left[ {0.004, 0.567} \right]} \right.} \right\}} \right\} |
{X_2} | \left\{ {\left\{ {{s_{2.056}}\left| {\left[ {0.018, 0.1} \right]} \right.} \right\}, \left\{ {{s_{6.0183}}\left| {\left[ {0.004, 0.072} \right]} \right.} \right\}, \left\{ {{s_{6.4364}}\left| {\left[ {0.036, 0.096} \right], {s_{6.5144}}\left| {\left[ {0.036, 0.144} \right]} \right.} \right.} \right\}} \right\} |
{X_3} | \left\{ {\left\{ {{s_{2.9428}}\left| {\left[ {0.054, 0.21} \right]} \right.} \right\}, \left\{ {{s_{5.6698}}\left| {\left[ {0.024, 0.12} \right]} \right.} \right\}, \left\{ {{s_{6.5111}}\left| {\left[ {0.006, 0.144} \right]} \right.} \right\}} \right\} |
{Z_4} | |
{X_1} | \left\{ \begin{array}{l} \left\{ {{s_{2.5632}}\left| {\left[ {0.04, 0.21} \right]} \right.} \right\}, \left\{ {{s_{4.1102}}\left| {\left[ {0.003, 0.096} \right], {s_{4.3893}}\left| {\left[ {0.006, 0.064} \right]} \right.} \right.} \right\}, \\ \left\{ {{s_{4.4531}}\left| {\left[ {0.008, 0.108} \right], {s_{4.9823}}\left| {\left[ {0.008, 0.144} \right]} \right.} \right.} \right\} \\ \end{array} \right\} |
{X_2} | \left\{ \begin{array}{l} \left\{ {{s_{3.3433}}\left| {\left[ {0.048, 0.15} \right], {s_{3.5308}}\left| {\left[ {0.024, 0.15} \right]} \right.} \right., {s_{4.035}}\left| {\left[ {0.048, 0.12} \right]} \right., {s_{4.1549}}\left| {\left[ {0.024, 0.12} \right]} \right.} \right\}, \\ \left\{ {{s_{4.2851}}\left| {\left[ {0.004, 0.084} \right]} \right.} \right\}, \left\{ {{s_{5.3206}}\left| {\left[ {0.045, 0.24} \right]} \right.} \right\} \\ \end{array} \right\} |
{X_3} | \left\{ {\left\{ {{s_{2.5701}}\left| {\left[ {0.016, 0.175} \right]} \right.} \right\}, \left\{ {{s_{5.2413}}\left| {\left[ {0.004, 0.08} \right]} \right.} \right\}, \left\{ {{s_{5.235}}\left| {\left[ {0.03, 0.288} \right]} \right.} \right\}} \right\} |
Step 4. According to Table 5 and Eqs (5.5) and (5.6), we can compute the IVPLt-SF-PIS y_j^ + \left( {j = 1, 2, 3, 4} \right) and IVPLt-SF-NIS y_j^ - \left( {j = 1, 2, 3, 4} \right) , which are given below.
\begin{array}{l} y_1^ + = \left\{ {\left\{ {{s_{2.3068}}\left| {\left[ {0.036, 0.125} \right], {s_{2.6845}}\left| {\left[ {0.027, 0.125} \right]} \right.} \right.} \right\}, \left\{ {{s_{6.49}}\left| {\left[ {0.006, 0.054} \right]} \right.} \right\}, \left\{ {{s_{6.4532}}\left| {\left[ {0.024, 0.12} \right]} \right.} \right\}} \right\} \\ y_2^ + = \left\{ {\left\{ {{s_{3.4581}}\left| {\left[ {0.024, 0.168} \right]} \right.} \right\}, \left\{ \begin{array}{l} {s_{4.6827}}\left| {\left[ {0.012, 0.032} \right], {s_{5.6825}}\left| {\left[ {0.018, 0.048} \right]} \right., } \right. \\ {s_{4.8472}}\left| {\left[ {0.004, 0.128} \right], {s_{5.8821}}\left| {\left[ {0.006, 0.192} \right]} \right.} \right. \end{array} \right\}, \left\{ {{s_{6.2132}}\left| {\left[ {0.009, 0.108} \right]} \right.} \right\}} \right\} \end{array} |
y_3^ + = \left\{ {\left\{ {{s_{2.056}}\left| {\left[ {0.018, 0.1} \right]} \right.} \right\}, \left\{ {{s_{6.0183}}\left| {\left[ {0.004, 0.072} \right]} \right.} \right\}, \left\{ {{s_{6.4364}}\left| {\left[ {0.036, 0.096} \right], {s_{6.5144}}\left| {\left[ {0.036, 0.144} \right]} \right.} \right.} \right\}} \right\} |
y_4^ + = \left\{ \begin{array}{l} \left\{ {{s_{2.5632}}\left| {\left[ {0.04, 0.21} \right]} \right.} \right\}, \left\{ {{s_{4.1102}}\left| {\left[ {0.003, 0.096} \right], {s_{4.3893}}\left| {\left[ {0.006, 0.064} \right]} \right.} \right.} \right\}, \\ \left\{ {{s_{4.4531}}\left| {\left[ {0.008, 0.108} \right], {s_{4.9823}}\left| {\left[ {0.008, 0.144} \right]} \right.} \right.} \right\} \end{array} \right\} |
y_1^ - = \left\{ {\left\{ {{s_{2.1765}}\left| {\left[ {0.002, 0.112} \right]} \right.} \right\}, \left\{ {{s_{6.4313}}\left| {\left[ {0.06, 0.16} \right]} \right.} \right\}, \left\{ {{s_{6.6696}}\left| {\left[ {0.048, 0.36} \right]} \right.} \right\}} \right\} |
y_2^ - = \left\{ \begin{array}{l} \left\{ {{s_{1.8387}}\left| {\left[ {0.008, 0.162} \right], {s_{2.0971}}\left| {\left[ {0.008, 0.162} \right]} \right., } \right.{s_{2.7541}}\left| {\left[ {0.016, 0.198} \right], {s_{2.8751}}\left| {\left[ {0.016, 0.198} \right]} \right.} \right.} \right\} \\ \left\{ {{s_{5.4288}}\left| {\left[ {0.02, 0.168} \right]} \right.} \right\}, \left\{ {{s_{5.3493}}\left| {\left[ {0.021, 0.225} \right]} \right.} \right\} \end{array} \right\} |
y_3^ - = \left\{ {\left\{ {{s_{3.1373}}\left| {\left[ {0.027, 0.096} \right], {s_{3.3547}}\left| {\left[ {0.018, 0.064} \right]} \right.} \right.} \right\}, \left\{ {{s_{6.1742}}\left| {\left[ {0.008, 0.125} \right]} \right.} \right\}, \left\{ {{s_{5.228}}\left| {\left[ {0.004, 0.567} \right]} \right.} \right\}} \right\} |
y_4^ - = \left\{ {\left\{ {{s_{2.5701}}\left| {\left[ {0.016, 0.175} \right]} \right.} \right\}, \left\{ {{s_{5.2413}}\left| {\left[ {0.004, 0.08} \right]} \right.} \right\}, \left\{ {{s_{5.235}}\left| {\left[ {0.03, 0.288} \right]} \right.} \right\}} \right\} |
Step 5. The distances between each alternative with PIS and NIS are computed by using Eqs (3.8), (5.7) and (5.8), which are given below:
\beta _1^ + = 0.0410 , v \beta _2^ + = 0.0405 , \;\; \beta _3^ + = 0.0735 |
\beta _1^ - = 0.0539 , \;\; \beta _2^ - = 0.0640 , \;\; \beta _3^ - = 0.0466 |
Step 6. The closeness ratio {\Xi _i}\left( {i = 1, 2, 3} \right) of the alternatives is computed by using Eq (5.9).
{\Xi _1} = 0.5679 , \;\; {\Xi _2} = 0.6124 , \;\; {\Xi _3} = 0.3880 |
Step 7. The value of the closeness ratio indicates the overall ranking order of the alternatives which is {X_2} > {X_1} > {X_3} . We conclude that ' {X_2} - Alibaba\;Cloud ' is the best suitable cloud storage provider among all.
Sensitivity analysis
This part examines the effect of the parameter ' q '. Here, we take 3 \leqslant q \leqslant 10 and the outcomes are given in Table 6 and illustrated graphically in Figure 3 as well. According to the closeness ratio obtained from using different values of parameter ' 3 \leqslant q \leqslant 10 ' and again using each stage of the suggested process, it was discovered that the alternatives' ranking did not change, which is ' {X_2} > {X_1} > {X_3} , with the best alternative being {X_2} and worst alternative {X_3} .
Parameter | Closeness ratio | Ranking order | Best alternative |
q = 3 | {\Xi _1} = 0.5679 , {\Xi _2} = 0.6124 , {\Xi _3} = 0.3880 | {X_2} > {X_1} > {X_3} | {X_2} |
q = 4 | {\Xi _1} = 0.5526 , {\Xi _2} = 0.6080 , {\Xi _3} = 0.3804 | {X_2} > {X_1} > {X_3} | {X_2} |
q = 5 | {\Xi _1} = 0.5314 , {\Xi _2} = 0.6027 , {\Xi _3} = 0.3740 | {X_2} > {X_1} > {X_3} | {X_2} |
q = 6 | {\Xi _1} = 0.5213 , {\Xi _2} = 0.5880 , {\Xi _3} = 0.3710 | {X_2} > {X_1} > {X_3} | {X_2} |
q = 7 | {\Xi _1} = 0.5160 , {\Xi _2} = 0.5769 , {\Xi _3} = 0.3660 | {X_2} > {X_1} > {X_3} | {X_2} |
q = 8 | {\Xi _1} = 0.5100 , {\Xi _2} = 0.5700 , {\Xi _3} = 0.3590 | {X_2} > {X_1} > {X_3} | {X_2} |
q = 9 | {\Xi _1} = 0.5055 , {\Xi _2} = 0.5650 , {\Xi _3} = 0.3509 | {X_2} > {X_1} > {X_3} | {X_2} |
q = 10 | {\Xi _1} = 0.4970 , {\Xi _2} = 0.5560 , {\Xi _3} = 0.3459 | {X_2} > {X_1} > {X_3} | {X_2} |
One more thing is also worth noting: The closeness ratios decrease as the parameter value increases. The value of ' q ' in this study represents the complexity of the decision environment and conditions. The complexity is higher when the value of ' q ' means is higher. The final ranking order and best alternative obtained by using the different values of parameter ' q ' on our proposed method are the same. However, when the data provided by DMs is more complicated and ambiguous, the results can differ. In real-world decision problems, the DMs may change the value of ' q ' depending on the situation to achieve appropriate decision results.
A fuzzy set [18] can only handle situations when the MD of the elements is present and cannot handle circumstances where the NMD of the elements is also present. The MD and NMD scenarios can be handled by IFSs [19]. There are numerous applications of fuzzy set theory that can cope with MD, AD and NMD, and were made possible by the development of PFSs [23]. Later, Mahmood et al. [26] developed t-SFSs by improving the restrictions of PFS, which cannot handle the circumstances in which the sum of MD, AD and NMD exceeds one. Further, Lt-SFS [38] was offered by providing more freedom to the DMs so that they could give their assessments in a qualitative manner. Lt-SFSs thus enable professionals the freedom to present their analysis with the fewest limitations. Due to this, we employed IVPLt-SFSs to gather evaluation data from professionals for this study. As we discussed in the introduction, there is no literature available about the presentation of probability in Lt-SFS. However, probabilistic linguistic q‐rung orthopair fuzzy weighted averaging (PLq‐ROFWA) [42] and IVPLq-ROFS [43] are the special cases of the proposed approach. For example, when the linguistic abstinence degree is considered to be zero or the internal-valued probability is a single-valued probability in the above given practical example, the final results obtained by using the techniques proposed in [42] and [43] are compared with our suggested method which is shown in Table 7. We can see from Table 7 that the final ranking obtained is slightly different than our proposed approach, but the best option is still the same, which is {X_2} . Further, we have discussed the qualitative analysis of our designed methodology with some existing approaches. From Table 8 and Figure 4, It has come to light that the method suggested in this study is more comprehensive than the ones already in use.
Methods | Closeness ratio/Score values | Ranking of alternatives | Best option |
PLq‐ROFWA [42] |
SC\left( {{X_1}} \right) = 0.4671 , SC\left( {{X_2}} \right) = 0.5890 , SC\left( {{X_3}} \right) = 0.3910 |
{X_2} > {X_1} > {X_3} | {X_2} |
IVPLq-ROFS [43] |
SC\left( {{X_1}} \right) = 0.4279 , SC\left( {{X_2}} \right) = 0.6511 , SC\left( {{X_3}} \right) = 0.6345 |
{X_2} > {X_3} > {X_1} | {X_2} |
Proposed method | {\Xi _1} = 0.5679 , {\Xi _2} = 0.6124 , {\Xi _3} = 0.3880 | {X_2} > {X_1} > {X_3} | {X_2} |
Set | Parameter | Involvement of abstinence degree | Involvement of linguistic term | Involvement of probability | Involvement of interval-valued probability | Computational complexity |
Lt-SFS [38] | Yes | Yes | Yes | No | No | Relatively high |
PLTS [60] | No | No | Yes | Yes | No | Relatively high |
IVPLTS [61] | No | No | Yes | Yes | Yes | Relatively high |
PLT [62] | No | No | Yes | Yes | No | Relatively high |
TOPSIS-based PLTS [63] | No | No | Yes | Yes | No | Relatively high |
Pt-SHFS [40] | Yes | Yes | No | Yes | No | Relatively high |
PLq‐ROFWA) [42] | Yes | No | Yes | Yes | No | Relatively high |
IVPLq-ROFS [43] | Yes | No | Yes | Yes | Yes | Relatively high |
Proposed method | Yes | Yes | Yes | Yes | Yes | Low |
Through this investigation and comparison analysis, we have come to the conclusion that our suggested strategy provides the following advantages over other existing techniques. (1) Since they can handle different types of assessment information by employing the IVPLPFSs, the evaluation process is more flexible; (2) Information fusion is more accurate compared with IVPLt-SFWA and IVPLt-SFWG operators; (3) More freedom for DMs when offering their opinions because of the relaxed restrictions of the novel information expression method; (4) The calculation complexity is significantly reduced due to the innovative distance measurement method we have introduced. This means that evaluations can be directly utilized without the need for normalization. As a result, our proposed methods have a broader range of applications and provide more accurate calculation results compared to other existing methods.
This paper introduces a new way of information expression, based on which a novel MAGDM technique is established. We first gave a brief introduction to a few current and inspirational perspectives. Then, as a foundation for solving a real-world MAGDM problem, the definition of IVPLt-SFS and a few fundamental principles were provided, such as the basic operational laws, the comparison and ranking rules and the distance measurement method. In addition, the extension of the IVPLt-SFWA and IVPLt-SFWG operators and their mathematical properties were offered. These operators were employed in the following section to aggregate assessments from various levels. After that, we presented a comprehensive framework to define a typical MAGDM problem and thoroughly propose and exhibit the application procedures of the suggested approach. The IVPLt-SFWA-TOPSIS method's specific calculation steps were provided throughout the problem of choosing a cloud storage provider. In the end, the impact of the parameter on final decision results and comparison analysis revealed that our technique is more flexible, robust and efficient than the other existing methods in describing fuzzy information.
Limitations and future directions
However, the idea of large-scale group decision-making (LSGDM) has drawn much attention from scholars in today's information-driven cultivation. LSGDM is the term used to describe a decision-making procedure including a significant number of participants and requiring consideration of several opinions, preferences and criteria which inevitably enhances the intricacy involved in reaching a conclusive decision [64]. Furthermore, in real-world LSGDM situations, consensus checking and improving, clustering, a more comprehensive information expression approach and an automated consensus-reaching mechanism are typically necessary [65].
This paper allocates limited attention to the issues mentioned above, and future research endeavors could place greater emphasis on addressing these aspects. In the future, it may be investigated whether the suggested approach may be used to solve LSGDM issues. It should also be noted that the formula for calculating the closeness ratio in the TOPSIS approach used in our work is outdated. Future work may also be concentrated using the updated closeness ratio formula. In this paper, the proposed method is employed for the selection of cloud storage provider. In the future, our goal is to apply this developed method to other real-life decision problems, such as medical diagnosis problems [26], bio-medical waste management [66], risk assessment [29] and electric-vehicle site selection problems [67].
The authors declare they have not used Artificial Intelligence (AI) tools in the creation of this article.
This work was supported by the National Natural Science Foundation of China under Grant no (U20A2068).
The authors have no conflict of interest.
[1] |
S. D. Gu, J. G. Kuba, Y. P. Chen, Y. L. Du, L. Yang, A. Knoll, et al., Safe multi-agent reinforcement learning for multi-robot controly, Artif. Intell., 319 (2023), 103905. https://doi.org/10.1016/j.artint.2023.103905 doi: 10.1016/j.artint.2023.103905
![]() |
[2] |
Z. B. Liu, K. R. Peng, L. P. Han, S. C. Guan, Modeling and control of robotic manipulators based on artificial neural networks: A review, Iran. J. Sci. Technol. Trans. Mech. Eng., 47 (2023), 1307–1347. https://doi.org/10.1007/s40997-023-00596-3 doi: 10.1007/s40997-023-00596-3
![]() |
[3] |
K. S. Ratnam, K. Palanisamy, G. Y. Yang, Future low-inertia power systems: Requirements, issues, and solutions-A review, Renew. Sust. Energ. Rev., 124 (2020), 109773. https://doi.org/10.1016/j.rser.2020.109773 doi: 10.1016/j.rser.2020.109773
![]() |
[4] |
H. Q. Xie, Z. J. Qin, G. Y. Li, B. H. Juang, Deep learning enabled semantic communication systems, IEEE T. Signal Proces., 69 (2021), 2663–2675. https://doi.org/10.1109/TSP.2021.3071210 doi: 10.1109/TSP.2021.3071210
![]() |
[5] |
M. Hennink, B. N. Kaiser, Sample sizes for saturation in qualitative research: A systematic review of empirical tests, Soc. Sci. Med., 292 (2022), 114523. https://doi.org/10.1016/j.socscimed.2021.114523 doi: 10.1016/j.socscimed.2021.114523
![]() |
[6] |
L. You, C. D. Li, X. Y. Zhang, Z. L. He, Edge event-triggered control and state-constraint impulsive consensus for nonlinear multi-agent systems, AIMS Math., 5 (2022), 4151–4167. https://doi.org/10.3934/math.2020266 doi: 10.3934/math.2020266
![]() |
[7] |
D. H. He, B. Z. Bao, L. G. Xu, Robust stability and boundedness of uncertain conformable fractional-order delay systems under input saturation, AIMS Math., 8 (2023), 21123–21137. https://doi.org/10.3934/math.20231076 doi: 10.3934/math.20231076
![]() |
[8] |
X. W. Shao, L. Chen, J. L. Chen, D. X. Zhang, Prescribed-time control for spacecraft formation flying with uncertainties and disturbances, AIMS Math., 9 (2024), 1180–1198. https://doi.org/10.3934/math.2024058 doi: 10.3934/math.2024058
![]() |
[9] |
M. N. A. Parlakci, Robust static output feedback H_{\infty} controller design for linear parameter-varying time delay systems, Circuits Syst. Signal Process., 43 (2024), 843–864. https://doi.org/10.1007/s00034-023-02514-z doi: 10.1007/s00034-023-02514-z
![]() |
[10] |
D. Swaroop, J. K. Hedrick, P. P. Yip, J. C. Gerdes, Dynamic surface control for a class of nonlinear systems, IEEE T. Automat. Contr., 45 (2000), 1893–1899. https://doi.org/10.1109/TAC.2000.880994 doi: 10.1109/TAC.2000.880994
![]() |
[11] | I. Kanellakopoulos, P. V. Kokotovic, A. S. Morse, Systematic design of adaptive controllers for feedback linearizable systems, In: 1991 American Control Conference, 1991,649–654. https://doi.org/10.23919/ACC.1991.4791451 |
[12] |
X. F. Wang, M. Y. Sun, A new family of fourth-order Ostrowski-type iterative methods for solving nonlinear systems, AIMS Math., 9 (2024), 10255–10266. https://doi.org/10.3934/math.2024501 doi: 10.3934/math.2024501
![]() |
[13] |
H. Alhazmi, M. Kharrat, Echo state network-based adaptive control for nonstrict-feedback nonlinear systems with input dead-zone and external disturbance, AIMS Math., 9 (2024), 20742–20762. https://doi.org/10.3934/math.20241008 doi: 10.3934/math.20241008
![]() |
[14] |
H. R. Ren, H. Ma, H. Y. Li, R. Q. Lu, A disturbance observer based intelligent control for nonstrict-feedback nonlinear systems, Sci. China Tech. Sci., 66 (2023), 456–467. https://doi.org/10.1007/s11431-022-2126-7 doi: 10.1007/s11431-022-2126-7
![]() |
[15] |
Q. T. Meng, Q. Ma, Y. Shi, Adaptive fixed-time stabilization for a class of uncertain nonlinear systems, IEEE T. Automat. Contr., 68 (2023), 6929–6936. https://doi.org/10.1109/TAC.2023.3244151 doi: 10.1109/TAC.2023.3244151
![]() |
[16] |
D. Yang, Y. J. Liu, F. Ding, E. F. Yang, Hierarchical gradient-based iterative parameter estimation algorithms for a nonlinear feedback system based on the hierarchical identification principle, Circuits Syst. Signal Process., 43 (2024), 124–151. https://doi.org/10.1007/s00034-023-02477-1 doi: 10.1007/s00034-023-02477-1
![]() |
[17] |
J. B. Zhang, F. Ding, Y. Shi, Self-tuning control based on multi-innovation stochastic gradient parameter estimation, Syst. Control Lett., 58 (2009), 69–75. https://doi.org/10.1016/j.sysconle.2008.08.005 doi: 10.1016/j.sysconle.2008.08.005
![]() |
[18] |
Y. Sun, M. Chen, C. Gao, L. B. Wu, Output feedback command filtered fuzzy controller design for uncertain strict-feedback nonlinear systems with unmodeled dynamics and event-triggered strategy, Eng. Res. Express, 6 (2024), 025315. https://doi.org/10.1088/2631-8695/ad3f75 doi: 10.1088/2631-8695/ad3f75
![]() |
[19] |
G. Q. Ma, P. R. Pagilla, Periodic event-triggered dynamic output feedback control of switched systems, Nonlinear Anal-Hybri., 31 (2019), 247–264. https://doi.org/10.1016/j.nahs.2018.10.001 doi: 10.1016/j.nahs.2018.10.001
![]() |
[20] |
X. N. Xia, T. P. Zhang, Adaptive output feedback dynamic surface control of nonlinear systems with unmodeled dynamics and unknown high-frequency gain sign, Neurocomput, 143 (2014), 312–321. https://doi.org/10.1016/j.neucom.2014.05.061 doi: 10.1016/j.neucom.2014.05.061
![]() |
[21] |
T. P. Zhang, M. Z. Xia, J. M. Zhu, Adaptive backstepping neural control of state-delayed nonlinear systems with full-state constraints and unmodeled dynamics, Int. J. Adapt. Control Signal Process., 31 (2017), 1704–1722. https://doi.org/10.1002/acs.2795 doi: 10.1002/acs.2795
![]() |
[22] |
T. P. Zhang, X. N. Xia, Decentralized adaptive fuzzy output feedback control of stochastic nonlinear large-scale systems with dynamic uncertainties, Inform. Sci., 315 (2015), 17–38. https://doi.org/10.1016/j.ins.2015.04.002 doi: 10.1016/j.ins.2015.04.002
![]() |
[23] |
L. Xu, F. Ding, X. Zhang, Q. M. Zhu, Novel parameter estimation method for the systems with colored noises by using the filtering identification idea, Syst. Control Lett., 186 (2024), 105774. https://doi.org/10.1016/j.sysconle.2024.105774 doi: 10.1016/j.sysconle.2024.105774
![]() |
[24] |
L. T. Xing, C. Y. Wen, Y. Zhu, H. Y. Su, Z. T. Liu, Output feedback control for uncertain nonlinear systems with input quantization, Automatica, 65 (2016), 191–202. https://doi.org/10.1016/j.automatica.2015.11.028 doi: 10.1016/j.automatica.2015.11.028
![]() |
[25] |
K. Yu, X. H. Chang, Quantized output feedback resilient control of uncertain systems under hybrid cyber attacks, Int. J. Adapt. Control Signal Process., 36 (2022), 2954–2970. https://doi.org/10.1002/acs.3496 doi: 10.1002/acs.3496
![]() |
[26] |
N. Li, J. Feng, Quantized feedback adaptive reliable H_{\infty} control for linear time-varying delayed systems, Circuits Syst. Signal Process., 35 (2016), 851–874. https://doi.org/10.1007/s00034-015-0109-2 doi: 10.1007/s00034-015-0109-2
![]() |
[27] |
Y. X. Li, G. H. Yang, Adaptive asymptotic tracking control of uncertain nonlinear systems with input quantization and actuator faults, Automatica, 72 (2016), 177–185. https://doi.org/10.1016/j.automatica.2016.06.008 doi: 10.1016/j.automatica.2016.06.008
![]() |
[28] |
T. F. Liu, Z. P. Jiang, D. J. Hill, A sector bound approach to feedback control of nonlinear systems with state quantization, Automatica, 48 (2012), 142–152. https://doi.org/10.1016/j.automatica.2011.09.041 doi: 10.1016/j.automatica.2011.09.041
![]() |
[29] |
W. L. Chen, J. H. Wang, K. M. Ma, T. Wang, Adaptive event-triggered neural control for nonlinear uncertain system with input constraint, Int. J. Robust Nonlin., 30 (2020), 3801–3815. https://doi.org/10.1002/rnc.4965 doi: 10.1002/rnc.4965
![]() |
[30] |
J. L. Ma, S. Y. Xu, Y. M. Li, Y. M. Chu, Z. Q. Zhang, Neural networks-based adaptive output feedback control for a class of uncertain nonlinear systems with input delay and disturbances, J. Franklin I., 355 (2018), 5503–5519. https://doi.org/10.1016/j.jfranklin.2018.05.045 doi: 10.1016/j.jfranklin.2018.05.045
![]() |
[31] |
T. P. Zhang, X. N. Xia, J. M. Zhu, Adaptive neural control of state delayed nonlinear systems with unmodeled dynamics and distributed time-varying delays, IET Control Theory Appl., 8 (2014), 1071–1082. https://doi.org/10.1049/iet-cta.2013.0803 doi: 10.1049/iet-cta.2013.0803
![]() |
[32] |
Q. Zhu, S. M. Fei, T. P. Zhang, T. Li, Adaptive RBF neural-networks control for a class of time-delay nonlinear systems, Neurocomput, 71 (2008), 3617–3624. https://doi.org/10.1016/j.neucom.2008.04.012 doi: 10.1016/j.neucom.2008.04.012
![]() |
[33] |
L. D. Fang, L. Ma, S. H. Ding, Finite-time fuzzy output-feedback control for p-norm stochastic nonlinear systems with output constraints, AIMS Math., 6 (2021), 2244–2267. https://doi.org/10.3934/math.2021136 doi: 10.3934/math.2021136
![]() |
[34] |
A. L. Li, X. L. Ye, Finite-time anti-synchronization for delayed inertial neural networks via the fractional and polynomial controllers of time variable, AIMS Math., 6 (2021), 8173–8190. https://doi.org/10.3934/math.2021473 doi: 10.3934/math.2021473
![]() |
[35] |
S. P. Bhat, D. S. Bernstein, Finite-time stability of continuous autonomous systems, SIAM J. Control Optim., 38 (2000), 751–766. https://doi.org/10.1137/S0363012997321358 doi: 10.1137/S0363012997321358
![]() |
[36] |
Y. M. Li, K. W. Li, S. C. Tong, Finite-time adaptive fuzzy output feedback dynamic surface control for MIMO nonstrict feedback systems, IEEE T. Fuzzy Syst., 27 (2019), 96–110. https://doi.org/10.1109/TFUZZ.2018.2868898 doi: 10.1109/TFUZZ.2018.2868898
![]() |
[37] |
C. X. Wang, J. L. Du, J. B. Yu, Adaptive finite-time tracking control for time-varying output constrained nonlinear systems with unmatched uncertainties, IET Control Theory Appl., 13 (2019), 2416–2424. https://doi.org/10.1049/iet-cta.2018.5458 doi: 10.1049/iet-cta.2018.5458
![]() |
[38] |
Y. Zhang, F. Wang, J. Zhang, Adaptive finite-time tracking control for output-constrained nonlinear systems with non-strict-feedback structure, Int. J. Adapt. Control Signal Process., 34 (2020), 560–574. https://doi.org/10.1002/acs.3099 doi: 10.1002/acs.3099
![]() |
[39] |
J. P. Li, Y. Yang, C. C. Hua, X. P. Guan, Fixed-time backstepping control design for high-order strict-feedback nonlinear systems via terminal sliding mode, IET Control Theory Appl., 11 (2017), 1184–1193. https://doi.org/10.1049/iet-cta.2016.1143 doi: 10.1049/iet-cta.2016.1143
![]() |
[40] |
Y. Hua, T. P. Zhang, Adaptive finite-time optimal fuzzy control for novel constrained uncertain nonstrict feedback mixed multiagent systems via modified dynamic surface control, Inform. Sci., 681 (2024), 121216. https://doi.org/10.1016/j.ins.2024.121216 doi: 10.1016/j.ins.2024.121216
![]() |
[41] |
Y. X. Li, Finite time command filtered adaptive fault tolerant control for a class of uncertain nonlinear systems, Automatica, 106 (2019), 117–123. https://doi.org/10.1016/j.automatica.2019.04.022 doi: 10.1016/j.automatica.2019.04.022
![]() |
[42] |
H. J. Brascamp, E. H. Lieb, Best constants in Young's inequality, its converse, and its generalization to more than three functions, Adv. Math., 20 (1976), 151–173. https://doi.org/10.1016/0001-8708(76)90184-5 doi: 10.1016/0001-8708(76)90184-5
![]() |
[43] |
S. Y. Gao, F. S. Li, H. Wang, Evaluation of the effects of oxygen enrichment on combustion stability of biodiesel through a PSO-EMD-RBF model: An experimental study, AIMS Math., 9 (2024), 4844–4862. https://doi.org/10.3934/math.2024235 doi: 10.3934/math.2024235
![]() |
[44] |
H. H. Qiu, L. Wan, Z. G. Zhou, Q. J. Zhang, Q. H. Zhou, Global exponential periodicity of nonlinear neural networks with multiple time-varying delays, AIMS Math., 8 (2023), 12472–12485. https://doi.org/10.3934/math.2023626 doi: 10.3934/math.2023626
![]() |
[45] |
Y. Linde, A. Buzo, R. Gray, An algorithm for vector quantizer design, IEEE T. Commun., 28 (1980), 84–95. https://doi.org/10.1109/TCOM.1980.1094577 doi: 10.1109/TCOM.1980.1094577
![]() |
[46] |
Y. F. Sun, L. L. Li, D. W. C. Ho, Quantized synchronization control of networked nonlinear systems: Dynamic quantizer design with event-triggered mechanism, IEEE T. Cybernetics, 53 (2023), 184–196. https://doi.org/10.1109/TCYB.2021.3090999 doi: 10.1109/TCYB.2021.3090999
![]() |
1. | Cheng Yang, Jing Liu, Research on teaching quality evaluation of higher vocational architecture majors based on enterprise platform with spherical fuzzy MAGDM, 2024, 33, 2191-026X, 10.1515/jisys-2023-0080 | |
2. | Masfa Nasrullah Ansari, Abdul Razaq, Hanan Alolaiyan, Umer Shuaib, Mohammed Abdullah Salman, Qin Xin, Empowering decentralized identity systems for Web 3.0 in complex spherical fuzzy knowledge, 2024, 14, 2045-2322, 10.1038/s41598-024-73488-4 | |
3. | Xiaofan Hao, Extended technique for interval-valued intuitionistic fuzzy MAGDM and applications to performance evaluation of intangible assets operation and management in sports events, 2024, 46, 10641246, 879, 10.3233/JIFS-233465 | |
4. | Binoy Krishna Giri, Sankar Kumar Roy, Muhammet Deveci, Projection based regret theory on three-way decision model in probabilistic interval-valued q-rung orthopair hesitant fuzzy set and its application to medicine company, 2023, 56, 0269-2821, 3617, 10.1007/s10462-023-10611-3 | |
5. | Weizhong Wang, Yi Wang, Yan Hu, Tinglong Zhang, Qun Wu, Assessment of metaverse integration options in the higher education institution using interval-valued T-spherical fuzzy-WHMA-CEM-GLDS framework, 2024, 156, 15684946, 111514, 10.1016/j.asoc.2024.111514 | |
6. | Ying Wang, Usman Khalid, Jawad Ali, Muhammad Ahsan Binyamin, Naeem Jan, A novel approach to decision making in rice quality management using interval-valued Pythagorean fuzzy Schweizer and Sklar power aggregation operators, 2024, 19, 1932-6203, e0311525, 10.1371/journal.pone.0311525 | |
7. | Rongfeng Li, Paul Augustine Ejegwa, Kun Li, Iorshase Agaji, Yuming Feng, Idoko Charles Onyeke, A new similarity function for Pythagorean fuzzy sets with application in football analysis, 2024, 9, 2473-6988, 4990, 10.3934/math.2024242 | |
8. | Muhammad Azeem, Jawad Ali, Jawad Ali, Muhammad I. Syam, Interval-valued picture fuzzy decision-making framework with partitioned maclaurin symmetric mean aggregation operators, 2024, 14, 2045-2322, 10.1038/s41598-024-72726-z | |
9. | Jialin He, Sine function similarity-based multi-attribute decision making technique of type-2 neutrosophic number sets and application to computer software quality evaluation, 2024, 46, 10641246, 563, 10.3233/JIFS-233407 | |
10. | Ke Xu, Kyunghwan Choi, Fengshuo Rao, Enhanced LogTODIM-TOPSIS framework for interval-valued intuitionistic fuzzy MAGDM and applications to intangible assets operational management performance evaluation of commercial sporting events, 2024, 10, 24058440, e26311, 10.1016/j.heliyon.2024.e26311 | |
11. | Alanoud Fetais, Hasan Dincer, Serhat Yüksel, Ahmet Aysan, Analysis of sustainable investment policies for housing demand in Qatar via hybrid quantum fuzzy decision-making model, 2024, 0368-492X, 10.1108/K-01-2024-0092 | |
12. | Ruoyu Zhang, Jun Ye, Multiple attribute decision making technique using single-valued neutrosophic trigonometric Dombi aggregation operators, 2024, 28, 1432-7643, 4217, 10.1007/s00500-023-09440-x | |
13. | Amirhossein Nafei, S. Pourmohammad Azizi, Seyed Ahmad Edalatpanah, Chien-Yi Huang, Smart TOPSIS: A Neural Network-Driven TOPSIS with Neutrosophic Triplets for Green Supplier Selection in Sustainable Manufacturing, 2024, 255, 09574174, 124744, 10.1016/j.eswa.2024.124744 | |
14. | Murugan Palanikumar, Nasreen Kausar, Ponnaiah Tharaniya, Željko Stević, Fikadu Tesgera Tolasa, Complex Diophantine interval-valued Pythagorean normal set for decision-making processes, 2025, 15, 2045-2322, 10.1038/s41598-024-82532-2 |
{Z_1} | |
{X_1} | \left\{ {\left\{ {{s_4}\left| {\left[ {.3, .5} \right]} \right.} \right\}, \left\{ {{s_5}\left| {\left[ {.1, .2} \right]} \right.} \right\}, \left\{ {{s_2}\left| {\left[ {.2, .4} \right]} \right.} \right\}} \right\} |
{X_2} | \left\{ {\left\{ {{s_3}\left| {\left[ {.1, .5} \right]} \right., {s_6}\left| {\left[ {.4, .5} \right]} \right.} \right\}, \left\{ {{s_1}\left| {\left[ {.1, .3} \right]} \right.} \right\}, \left\{ {{s_4}\left| {\left[ {.2, .6} \right]} \right.} \right\}} \right\} |
{X_3} | \left\{ {\left\{ {{s_4}\left| {\left[ {.1, .8} \right]} \right.} \right\}, \left\{ {{s_3}\left| {\left[ {.3, .4} \right]} \right.} \right\}, \left\{ {{s_5}\left| {\left[ {.2, .8} \right]} \right.} \right\}} \right\} |
{Z_2} | |
{X_1} | \left\{ {\left\{ {{s_2}\left| {\left[ {.2, .6} \right]} \right.} \right\}, \left\{ {{s_3}\left| {\left[ {.5, .7} \right]} \right.} \right\}, \left\{ {{s_1}\left| {\left[ {.1, .5} \right]} \right.} \right\}} \right\} |
{X_2} | \left\{ {\left\{ {{s_4}\left| {\left[ {.5, .7} \right]} \right.} \right\}, \left\{ {{s_1}\left| {\left[ {.2, .4} \right]} \right.} \right\}, \left\{ {{s_3}\left| {\left[ {.5, .7} \right]} \right.} \right\}} \right\} |
{X_3} | \left\{ {\left\{ {{s_2}\left| {\left[ {.4, .7} \right]} \right.} \right\}, \left\{ {{s_1}\left| {\left[ {.2, .4} \right]} \right., {s_6}\left| {\left[ {.3, .6} \right]} \right.} \right\}, \left\{ {{s_4}\left| {\left[ {.1, .3} \right]} \right.} \right\}} \right\} |
{Z_3} | |
{X_1} | \left\{ {\left\{ {{s_6}\left| {\left[ {.3, .4} \right]} \right.} \right\}, \left\{ {{s_4}\left| {\left[ {.2, .5} \right]} \right.} \right\}, \left\{ {{s_1}\left| {\left[ {.1, .9} \right]} \right.} \right\}} \right\} |
{X_2} | \left\{ {\left\{ {{s_3}\left| {\left[ {.2, .5} \right]} \right.} \right\}, \left\{ {{s_4}\left| {\left[ {.4, .6} \right]} \right.} \right\}, \left\{ {{s_4}\left| {\left[ {.3, .4} \right]} \right., {s_5}\left| {\left[ {.3, .6} \right]} \right.} \right\}} \right\} |
{X_3} | \left\{ {\left\{ {{s_2}\left| {\left[ {.6, .7} \right]} \right.} \right\}, \left\{ {{s_1}\left| {\left[ {.3, .6} \right]} \right.} \right\}, \left\{ {{s_6}\left| {\left[ {.2, .4} \right]} \right.} \right\}} \right\} |
{Z_4} | |
{X_1} | \left\{ {\left\{ {{s_3}\left| {\left[ {.2, .5} \right]} \right.} \right\}, \left\{ {{s_4}\left| {\left[ {.1, .6} \right], {s_6}\left| {\left[ {.2, .4} \right]} \right.} \right.} \right\}, \left\{ {{s_1}\left| {\left[ {.1, .3} \right]} \right., {s_2}\left| {\left[ {.1, .4} \right]} \right.} \right\}} \right\} |
{X_2} | \left\{ {\left\{ {{s_4}\left| {\left[ {.4, .6} \right]} \right.} \right\}, \left\{ {{s_2}\left| {\left[ {.4, .7} \right], } \right.} \right\}, \left\{ {{s_3}\left| {\left[ {.3, .8} \right]} \right.} \right\}} \right\} |
{X_3} | \left\{ {\left\{ {{s_4}\left| {\left[ {.4, .7} \right]} \right.} \right\}, \left\{ {{s_3}\left| {\left[ {.1, .4} \right]} \right.} \right\}, \left\{ {{s_5}\left| {\left[ {.2, .6} \right]} \right.} \right\}} \right\} |
{Z_1} | |
{X_1} | \left\{ {\left\{ {{s_5}\left| {\left[ {.3, .5} \right]} \right.} \right\}, \left\{ {{s_4}\left| {\left[ {.2, .3} \right]} \right.} \right\}, \left\{ {{s_2}\left| {\left[ {.4, .5} \right]} \right.} \right\}} \right\} |
{X_2} | \left\{ {\left\{ {{s_4}\left| {\left[ {.4, .5} \right]} \right.} \right\}, \left\{ {{s_2}\left| {\left[ {.1, .3} \right]} \right.} \right\}, \left\{ {{s_5}\left| {\left[ {.5, .6} \right]} \right.} \right\}} \right\} |
{X_3} | \left\{ {\left\{ {{s_4}\left| {\left[ {.2, .7} \right]} \right.} \right\}, \left\{ {{s_3}\left| {\left[ {.5, .8} \right]} \right.} \right\}, \left\{ {{s_2}\left| {\left[ {.4, .5} \right]} \right.} \right\}} \right\} |
{Z_2} | |
{X_1} | \left\{ {\left\{ {{s_3}\left| {\left[ {.2, .6} \right]} \right., {s_4}\left| {\left[ {.2, .6} \right]} \right.} \right\}, \left\{ {{s_3}\left| {\left[ {.2, .4} \right]} \right.} \right\}, \left\{ {{s_4}\left| {\left[ {.3, .5} \right]} \right.} \right\}} \right\} |
{X_2} | \left\{ {\left\{ {{s_4}\left| {\left[ {.2, .45} \right]} \right.} \right\}, \left\{ {{s_2}\left| {\left[ {.2, .25} \right]} \right.} \right\}, \left\{ {{s_5}\left| {\left[ {.1, .2} \right]} \right.} \right\}} \right\} |
{X_3} | \left\{ {\left\{ {{s_6}\left| {\left[ {.3, .6} \right]} \right.} \right\}, \left\{ {{s_2}\left| {\left[ {.2, .4} \right]} \right.} \right\}, \left\{ {{s_4}\left| {\left[ {.3, .6} \right]} \right.} \right\}} \right\} |
{Z_3} | |
{X_1} | \left\{ {\left\{ {{s_6}\left| {\left[ {.3, .4} \right]} \right.} \right\}, \left\{ {{s_4}\left| {\left[ {.2, .5} \right]} \right.} \right\}, \left\{ {{s_1}\left| {\left[ {.1, .9} \right]} \right.} \right\}} \right\} |
{X_2} | \left\{ {\left\{ {{s_5}\left| {\left[ {.3, .4} \right]} \right.} \right\}, \left\{ {{s_1}\left| {\left[ {.1, .3} \right]} \right.} \right\}, \left\{ {{s_4}\left| {\left[ {.3, .4} \right]} \right.} \right\}} \right\} |
{X_3} | \left\{ {\left\{ {{s_5}\left| {\left[ {.3, .5} \right]} \right.} \right\}, \left\{ {{s_3}\left| {\left[ {.4, .5} \right]} \right.} \right\}, \left\{ {{s_3}\left| {\left[ {.1, .6} \right]} \right.} \right\}} \right\} |
{Z_4} | |
{X_1} | \left\{ {\left\{ {{s_4}\left| {\left[ {.4, .7} \right]} \right.} \right\}, \left\{ {{s_3}\left| {\left[ {.1, .4} \right]} \right.} \right\}, \left\{ {{s_5}\left| {\left[ {.2, .6} \right]} \right.} \right\}} \right\} |
{X_2} | \left\{ {\left\{ {{s_1}\left| {\left[ {0.4, 0.5} \right], {s_4}\left| {\left[ {0.2, 0.5} \right]} \right.} \right.} \right\}, \left\{ {{s_2}\left| {\left[ {0.1, 0.3} \right]} \right.} \right\}, \left\{ {{s_5}\left| {\left[ {0.5, 0.6} \right]} \right.} \right\}} \right\} |
{X_3} | \left\{ {\left\{ {{s_2}\left| {\left[ {.1, .5} \right]} \right.} \right\}, \left\{ {{s_3}\left| {\left[ {.2, .4} \right]} \right.} \right\}, \left\{ {{s_2}\left| {\left[ {.3, .8} \right]} \right.} \right\}} \right\} |
{Z_1} | |
{X_1} | \left\{ {\left\{ {{s_5}\left| {\left[ {.4, .5} \right]} \right., {s_6}\left| {\left[ {.3, .5} \right]} \right.} \right\}, \left\{ {{s_2}\left| {\left[ {.3, .9} \right]} \right.} \right\}, \left\{ {{s_6}\left| {\left[ {.3, .6} \right]} \right.} \right\}} \right\} |
{X_2} | \left\{ {\left\{ {{s_4}\left| {\left[ {.2, .45} \right]} \right.} \right\}, \left\{ {{s_4}\left| {\left[ {.2, .25} \right]} \right.} \right\}, \left\{ {{s_2}\left| {\left[ {.1, 0.7} \right], {s_5}\left| {\left[ {.2, 0.3} \right]} \right.} \right.} \right\}} \right\} |
{X_3} | \left\{ {\left\{ {{s_5}\left| {\left[ {.1, .2} \right]} \right.} \right\}, \left\{ {{s_3}\left| {\left[ {.4, .5} \right]} \right.} \right\}, \left\{ {{s_6}\left| {\left[ {.6, .9} \right]} \right.} \right\}} \right\} |
{Z_2} | |
{X_1} | \left\{ {\left\{ {{s_3}\left| {\left[ {.2, .45} \right], {s_5}\left| {\left[ {.4, .55} \right]} \right.} \right.} \right\}, \left\{ {{s_3}\left| {\left[ {.2, .6} \right]} \right.} \right\}, \left\{ {{s_6}\left| {\left[ {.7, .9} \right]} \right.} \right\}} \right\} |
{X_2} | \left\{ {\left\{ {{s_2}\left| {\left[ {.3, .6} \right]} \right.} \right\}, \left\{ {{s_6}\left| {\left[ {.4, .5} \right]} \right.} \right\}, \left\{ {{s_3}\left| {\left[ {.3, .9} \right]} \right.} \right\}} \right\} |
{X_3} | \left\{ {\left\{ {{s_5}\left| {\left[ {.2, .4} \right]} \right.} \right\}, \left\{ {{s_3}\left| {\left[ {.3, .2} \right], {s_4}\left| {\left[ {.1, .8} \right]} \right.} \right.} \right\}, \left\{ {{s_6}\left| {\left[ {.3, .6} \right]} \right.} \right\}} \right\} |
{Z_3} | |
{X_1} | \left\{ {\left\{ {{s_3}\left| {\left[ {.3, .6} \right]} \right., {s_5}\left| {\left[ {.2, .4} \right]} \right.} \right\}, \left\{ {{s_2}\left| {\left[ {.2, .5} \right]} \right.} \right\}, \left\{ {{s_1}\left| {\left[ {.4, .7} \right]} \right.} \right\}} \right\} |
{X_2} | \left\{ {\left\{ {{s_3}\left| {\left[ {.3, .5} \right]} \right.} \right\}, \left\{ {{s_3}\left| {\left[ {.1, .4} \right]} \right.} \right\}, \left\{ {{s_4}\left| {\left[ {.4, .6} \right]} \right.} \right\}} \right\} |
{X_3} | \left\{ {\left\{ {{s_6}\left| {\left[ {.3, .6} \right]} \right.} \right\}, \left\{ {{s_2}\left| {\left[ {.2, .4} \right]} \right.} \right\}, \left\{ {{s_4}\left| {\left[ {.3, .6} \right]} \right.} \right\}} \right\} |
{Z_4} | |
{X_1} | \left\{ {\left\{ {{s_3}\left| {\left[ {.5, .6} \right]} \right.} \right\}, \left\{ {{s_1}\left| {\left[ {.3, .4} \right]} \right.} \right\}, \left\{ {{s_4}\left| {\left[ {.4, .6} \right]} \right.} \right\}} \right\} |
{X_2} | \left\{ {\left\{ {{s_5}\left| {\left[ {.3, .5} \right], {s_6}\left| {\left[ {.3, .4} \right]} \right.} \right.} \right\}, \left\{ {{s_3}\left| {\left[ {.1, .4} \right]} \right.} \right\}, \left\{ {{s_4}\left| {\left[ {.3, .5} \right]} \right.} \right\}} \right\} |
{X_3} | \left\{ {\left\{ {{s_3}\left| {\left[ {.4, .5} \right]} \right.} \right\}, \left\{ {{s_5}\left| {\left[ {.2, .5} \right]} \right.} \right\}, \left\{ {{s_4}\left| {\left[ {.5, .6} \right]} \right.} \right\}} \right\} |
{Z_1} | |
{X_1} | \left\{ {\left\{ {{s_{4.714}}\left| {\left[ {0.036, 0.125} \right], {s_{5.3258}}\left| {\left[ {0.027, 0.125} \right]} \right.} \right.} \right\}, \left\{ {{s_{3.285}}\left| {\left[ {0.006, 0.054} \right]} \right.} \right\}, \left\{ {{s_{3.1037}}\left| {\left[ {0.024, 0.12} \right]} \right.} \right\}} \right\} |
{X_2} | \left\{ \begin{array}{l} \left\{ {{s_{3.7135}}\left| {\left[ {0.008, 0.1125} \right], {s_{5.1024}}\left| {\left[ {0.032, 0.1125} \right]} \right.} \right.} \right\}, \left\{ {{s_{3.9193}}\left| {\left[ {0.001, 0.063} \right]} \right.} \right\}, \\ \left\{ {{s_{3.1982}}\left| {\left[ {0.01, 0.252} \right], {s_{4.6141}}\left| {\left[ {0.02, 0.108} \right]} \right.} \right.} \right\} \\ \end{array} \right\} |
{X_3} | \left\{ {\left\{ {{s_{4.485}}\left| {\left[ {0.002, 0.112} \right]} \right.} \right\}, \left\{ {{s_3}\left| {\left[ {0.06, 0.16} \right]} \right.} \right\}, \left\{ {{s_{4.3166}}\left| {\left[ {0.048, 0.36} \right]} \right.} \right\}} \right\} |
{Z_2} | |
{X_1} | \left\{ \begin{array}{l} \left\{ {{s_{2.7274}}\left| {\left[ {0.008, 0.162} \right], {s_{3.1}}\left| {\left[ {0.008, 0.162} \right]} \right., } \right.{s_{4.017}}\left| {\left[ {0.016, 0.198} \right], {s_{4.1796}}\left| {\left[ {0.016, 0.198} \right]} \right.} \right.} \right\} \\ \left\{ {{s_3}\left| {\left[ {0.02, 0.168} \right]} \right.} \right\}, \left\{ {{s_{2.856}}\left| {\left[ {0.021, 0.225} \right]} \right.} \right\} \\ \end{array} \right\} |
{X_2} | \left\{ {\left\{ {{s_{3.4985}}\left| {\left[ {0.03, 0.189} \right]} \right.} \right\}, \left\{ {{s_{2.4183}}\left| {\left[ {0.016, 0.05} \right]} \right.} \right\}, \left\{ {{s_{3.3913}}\left| {\left[ {0.015, 0.126} \right]} \right.} \right\}} \right\} |
{X_3} | \left\{ {\left\{ {{s_{4.9253}}\left| {\left[ {0.024, 0.168} \right]} \right.} \right\}, \left\{ \begin{array}{l} {s_{1.8327}}\left| {\left[ {0.012, 0.032} \right], {s_{3.4933}}\left| {\left[ {0.018, 0.048} \right]} \right., } \right. \\ {s_{2.0562}}\left| {\left[ {0.004, 0.128} \right], {s_{3.9193}}\left| {\left[ {0.006, 0.192} \right]} \right.} \right. \\ \end{array} \right\}, \left\{ {{s_{4.7043}}\left| {\left[ {0.009, 0.108} \right]} \right.} \right\}} \right\} |
{Z_3} | |
{X_1} | \left\{ {\left\{ {{s_{5.4302}}\left| {\left[ {0.027, 0.096} \right], {s_{5.7017}}\left| {\left[ {0.018, 0.064} \right]} \right.} \right.} \right\}, \left\{ {{s_{3.0314}}\left| {\left[ {0.008, 0.125} \right]} \right.} \right\}, \left\{ {{s_1}\left| {\left[ {0.004, 0.567} \right]} \right.} \right\}} \right\} |
{X_2} | \left\{ {\left\{ {{s_{3.7783}}\left| {\left[ {0.018, 0.1} \right]} \right.} \right\}, \left\{ {{s_{2.5562}}\left| {\left[ {0.004, 0.072} \right]} \right.} \right\}, \left\{ {{s_4}\left| {\left[ {0.036, 0.096} \right], {s_{4.3346}}\left| {\left[ {0.036, 0.144} \right]} \right.} \right.} \right\}} \right\} |
{X_3} | \left\{ {\left\{ {{s_{5.1676}}\left| {\left[ {0.054, 0.21} \right]} \right.} \right\}, \left\{ {{s_{1.7176}}\left| {\left[ {0.024, 0.12} \right]} \right.} \right\}, \left\{ {{s_{4.3198}}\left| {\left[ {0.006, 0.144} \right]} \right.} \right\}} \right\} |
{Z_4} | |
{X_1} | \left\{ \begin{array}{l} \left\{ {{s_{3.3112}}\left| {\left[ {0.04, 0.21} \right]} \right.} \right\}, \left\{ {{s_{2.1441}}\left| {\left[ {0.003, 0.096} \right], {s_{2.4811}}\left| {\left[ {0.006, 0.064} \right]} \right.} \right.} \right\}, \\ \left\{ {{s_{2.562}}\left| {\left[ {0.008, 0.108} \right], {s_{3.2881}}\left| {\left[ {0.008, 0.144} \right]} \right.} \right.} \right\} \\ \end{array} \right\} |
{X_2} | \left\{ \begin{array}{l} \left\{ {{s_{4.2646}}\left| {\left[ {0.048, 0.15} \right], {s_{4.485}}\left| {\left[ {0.024, 0.15} \right]} \right.} \right., {s_{5.0548}}\left| {\left[ {0.048, 0.12} \right]} \right., {s_{5.1844}}\left| {\left[ {0.024, 0.12} \right]} \right.} \right\}, \\ \left\{ {{s_{2.3522}}\left| {\left[ {0.004, 0.084} \right]} \right.} \right\}, \left\{ {{s_{3.8049}}\left| {\left[ {0.045, 0.24} \right]} \right.} \right\} \\ \end{array} \right\} |
{X_3} | \left\{ {\left\{ {{s_{3.3199}}\left| {\left[ {0.016, 0.175} \right]} \right.} \right\}, \left\{ {{s_{3.6801}}\left| {\left[ {0.004, 0.08} \right]} \right.} \right\}, \left\{ {{s_{3.6703}}\left| {\left[ {0.03, 0.288} \right]} \right.} \right\}} \right\} |
{Z_1} | |
{X_1} | \left\{ {\left\{ {{s_{2.3068}}\left| {\left[ {0.036, 0.125} \right], {s_{2.6845}}\left| {\left[ {0.027, 0.125} \right]} \right.} \right.} \right\}, \left\{ {{s_{6.49}}\left| {\left[ {0.006, 0.054} \right]} \right.} \right\}, \left\{ {{s_{6.4532}}\left| {\left[ {0.024, 0.12} \right]} \right.} \right\}} \right\} |
{X_2} | \left\{ \begin{array}{l} \left\{ {{s_{1.7653}}\left| {\left[ {0.008, 0.1125} \right], {s_{2.5405}}\left| {\left[ {0.032, 0.1125} \right]} \right.} \right.} \right\}, \left\{ {{s_{6.6055}}\left| {\left[ {0.001, 0.063} \right]} \right.} \right\}, \\ \left\{ {{s_{6.4726}}\left| {\left[ {0.01, 0.252} \right], {s_{6.7142}}\left| {\left[ {0.02, 0.108} \right]} \right.} \right.} \right\} \\ \end{array} \right\} |
{X_3} | \left\{ {\left\{ {{s_{2.1765}}\left| {\left[ {0.002, 0.112} \right]} \right.} \right\}, \left\{ {{s_{6.4313}}\left| {\left[ {0.06, 0.16} \right]} \right.} \right\}, \left\{ {{s_{6.6696}}\left| {\left[ {0.048, 0.36} \right]} \right.} \right\}} \right\} |
{Z_2} | |
{X_1} | \left\{ \begin{array}{l} \left\{ {{s_{1.8387}}\left| {\left[ {0.008, 0.162} \right], {s_{2.0971}}\left| {\left[ {0.008, 0.162} \right]} \right., } \right.{s_{2.7541}}\left| {\left[ {0.016, 0.198} \right], {s_{2.8751}}\left| {\left[ {0.016, 0.198} \right]} \right.} \right.} \right\} \\ \left\{ {{s_{5.4288}}\left| {\left[ {0.02, 0.168} \right]} \right.} \right\}, \left\{ {{s_{5.3493}}\left| {\left[ {0.021, 0.225} \right]} \right.} \right\} \\ \end{array} \right\} |
{X_2} | \left\{ {\left\{ {{s_{2.3782}}\left| {\left[ {0.03, 0.189} \right]} \right.} \right\}, \left\{ {{s_{5.0889}}\left| {\left[ {0.016, 0.05} \right]} \right.} \right\}, \left\{ {{s_{5.6322}}\left| {\left[ {0.015, 0.126} \right]} \right.} \right\}} \right\} |
{X_3} | \left\{ {\left\{ {{s_{3.4581}}\left| {\left[ {0.024, 0.168} \right]} \right.} \right\}, \left\{ \begin{array}{l} {s_{4.6827}}\left| {\left[ {0.012, 0.032} \right], {s_{5.6825}}\left| {\left[ {0.018, 0.048} \right]} \right., } \right. \\ {s_{4.8472}}\left| {\left[ {0.004, 0.128} \right], {s_{5.8821}}\left| {\left[ {0.006, 0.192} \right]} \right.} \right. \\ \end{array} \right\}, \left\{ {{s_{6.2132}}\left| {\left[ {0.009, 0.108} \right]} \right.} \right\}} \right\} |
{Z_3} | |
{X_1} | \left\{ {\left\{ {{s_{3.1373}}\left| {\left[ {0.027, 0.096} \right], {s_{3.3547}}\left| {\left[ {0.018, 0.064} \right]} \right.} \right.} \right\}, \left\{ {{s_{6.1742}}\left| {\left[ {0.008, 0.125} \right]} \right.} \right\}, \left\{ {{s_{5.228}}\left| {\left[ {0.004, 0.567} \right]} \right.} \right\}} \right\} |
{X_2} | \left\{ {\left\{ {{s_{2.056}}\left| {\left[ {0.018, 0.1} \right]} \right.} \right\}, \left\{ {{s_{6.0183}}\left| {\left[ {0.004, 0.072} \right]} \right.} \right\}, \left\{ {{s_{6.4364}}\left| {\left[ {0.036, 0.096} \right], {s_{6.5144}}\left| {\left[ {0.036, 0.144} \right]} \right.} \right.} \right\}} \right\} |
{X_3} | \left\{ {\left\{ {{s_{2.9428}}\left| {\left[ {0.054, 0.21} \right]} \right.} \right\}, \left\{ {{s_{5.6698}}\left| {\left[ {0.024, 0.12} \right]} \right.} \right\}, \left\{ {{s_{6.5111}}\left| {\left[ {0.006, 0.144} \right]} \right.} \right\}} \right\} |
{Z_4} | |
{X_1} | \left\{ \begin{array}{l} \left\{ {{s_{2.5632}}\left| {\left[ {0.04, 0.21} \right]} \right.} \right\}, \left\{ {{s_{4.1102}}\left| {\left[ {0.003, 0.096} \right], {s_{4.3893}}\left| {\left[ {0.006, 0.064} \right]} \right.} \right.} \right\}, \\ \left\{ {{s_{4.4531}}\left| {\left[ {0.008, 0.108} \right], {s_{4.9823}}\left| {\left[ {0.008, 0.144} \right]} \right.} \right.} \right\} \\ \end{array} \right\} |
{X_2} | \left\{ \begin{array}{l} \left\{ {{s_{3.3433}}\left| {\left[ {0.048, 0.15} \right], {s_{3.5308}}\left| {\left[ {0.024, 0.15} \right]} \right.} \right., {s_{4.035}}\left| {\left[ {0.048, 0.12} \right]} \right., {s_{4.1549}}\left| {\left[ {0.024, 0.12} \right]} \right.} \right\}, \\ \left\{ {{s_{4.2851}}\left| {\left[ {0.004, 0.084} \right]} \right.} \right\}, \left\{ {{s_{5.3206}}\left| {\left[ {0.045, 0.24} \right]} \right.} \right\} \\ \end{array} \right\} |
{X_3} | \left\{ {\left\{ {{s_{2.5701}}\left| {\left[ {0.016, 0.175} \right]} \right.} \right\}, \left\{ {{s_{5.2413}}\left| {\left[ {0.004, 0.08} \right]} \right.} \right\}, \left\{ {{s_{5.235}}\left| {\left[ {0.03, 0.288} \right]} \right.} \right\}} \right\} |
Parameter | Closeness ratio | Ranking order | Best alternative |
q = 3 | {\Xi _1} = 0.5679 , {\Xi _2} = 0.6124 , {\Xi _3} = 0.3880 | {X_2} > {X_1} > {X_3} | {X_2} |
q = 4 | {\Xi _1} = 0.5526 , {\Xi _2} = 0.6080 , {\Xi _3} = 0.3804 | {X_2} > {X_1} > {X_3} | {X_2} |
q = 5 | {\Xi _1} = 0.5314 , {\Xi _2} = 0.6027 , {\Xi _3} = 0.3740 | {X_2} > {X_1} > {X_3} | {X_2} |
q = 6 | {\Xi _1} = 0.5213 , {\Xi _2} = 0.5880 , {\Xi _3} = 0.3710 | {X_2} > {X_1} > {X_3} | {X_2} |
q = 7 | {\Xi _1} = 0.5160 , {\Xi _2} = 0.5769 , {\Xi _3} = 0.3660 | {X_2} > {X_1} > {X_3} | {X_2} |
q = 8 | {\Xi _1} = 0.5100 , {\Xi _2} = 0.5700 , {\Xi _3} = 0.3590 | {X_2} > {X_1} > {X_3} | {X_2} |
q = 9 | {\Xi _1} = 0.5055 , {\Xi _2} = 0.5650 , {\Xi _3} = 0.3509 | {X_2} > {X_1} > {X_3} | {X_2} |
q = 10 | {\Xi _1} = 0.4970 , {\Xi _2} = 0.5560 , {\Xi _3} = 0.3459 | {X_2} > {X_1} > {X_3} | {X_2} |
Methods | Closeness ratio/Score values | Ranking of alternatives | Best option |
PLq‐ROFWA [42] |
SC\left( {{X_1}} \right) = 0.4671 , SC\left( {{X_2}} \right) = 0.5890 , SC\left( {{X_3}} \right) = 0.3910 |
{X_2} > {X_1} > {X_3} | {X_2} |
IVPLq-ROFS [43] |
SC\left( {{X_1}} \right) = 0.4279 , SC\left( {{X_2}} \right) = 0.6511 , SC\left( {{X_3}} \right) = 0.6345 |
{X_2} > {X_3} > {X_1} | {X_2} |
Proposed method | {\Xi _1} = 0.5679 , {\Xi _2} = 0.6124 , {\Xi _3} = 0.3880 | {X_2} > {X_1} > {X_3} | {X_2} |
Set | Parameter | Involvement of abstinence degree | Involvement of linguistic term | Involvement of probability | Involvement of interval-valued probability | Computational complexity |
Lt-SFS [38] | Yes | Yes | Yes | No | No | Relatively high |
PLTS [60] | No | No | Yes | Yes | No | Relatively high |
IVPLTS [61] | No | No | Yes | Yes | Yes | Relatively high |
PLT [62] | No | No | Yes | Yes | No | Relatively high |
TOPSIS-based PLTS [63] | No | No | Yes | Yes | No | Relatively high |
Pt-SHFS [40] | Yes | Yes | No | Yes | No | Relatively high |
PLq‐ROFWA) [42] | Yes | No | Yes | Yes | No | Relatively high |
IVPLq-ROFS [43] | Yes | No | Yes | Yes | Yes | Relatively high |
Proposed method | Yes | Yes | Yes | Yes | Yes | Low |
{Z_1} | |
{X_1} | \left\{ {\left\{ {{s_4}\left| {\left[ {.3, .5} \right]} \right.} \right\}, \left\{ {{s_5}\left| {\left[ {.1, .2} \right]} \right.} \right\}, \left\{ {{s_2}\left| {\left[ {.2, .4} \right]} \right.} \right\}} \right\} |
{X_2} | \left\{ {\left\{ {{s_3}\left| {\left[ {.1, .5} \right]} \right., {s_6}\left| {\left[ {.4, .5} \right]} \right.} \right\}, \left\{ {{s_1}\left| {\left[ {.1, .3} \right]} \right.} \right\}, \left\{ {{s_4}\left| {\left[ {.2, .6} \right]} \right.} \right\}} \right\} |
{X_3} | \left\{ {\left\{ {{s_4}\left| {\left[ {.1, .8} \right]} \right.} \right\}, \left\{ {{s_3}\left| {\left[ {.3, .4} \right]} \right.} \right\}, \left\{ {{s_5}\left| {\left[ {.2, .8} \right]} \right.} \right\}} \right\} |
{Z_2} | |
{X_1} | \left\{ {\left\{ {{s_2}\left| {\left[ {.2, .6} \right]} \right.} \right\}, \left\{ {{s_3}\left| {\left[ {.5, .7} \right]} \right.} \right\}, \left\{ {{s_1}\left| {\left[ {.1, .5} \right]} \right.} \right\}} \right\} |
{X_2} | \left\{ {\left\{ {{s_4}\left| {\left[ {.5, .7} \right]} \right.} \right\}, \left\{ {{s_1}\left| {\left[ {.2, .4} \right]} \right.} \right\}, \left\{ {{s_3}\left| {\left[ {.5, .7} \right]} \right.} \right\}} \right\} |
{X_3} | \left\{ {\left\{ {{s_2}\left| {\left[ {.4, .7} \right]} \right.} \right\}, \left\{ {{s_1}\left| {\left[ {.2, .4} \right]} \right., {s_6}\left| {\left[ {.3, .6} \right]} \right.} \right\}, \left\{ {{s_4}\left| {\left[ {.1, .3} \right]} \right.} \right\}} \right\} |
{Z_3} | |
{X_1} | \left\{ {\left\{ {{s_6}\left| {\left[ {.3, .4} \right]} \right.} \right\}, \left\{ {{s_4}\left| {\left[ {.2, .5} \right]} \right.} \right\}, \left\{ {{s_1}\left| {\left[ {.1, .9} \right]} \right.} \right\}} \right\} |
{X_2} | \left\{ {\left\{ {{s_3}\left| {\left[ {.2, .5} \right]} \right.} \right\}, \left\{ {{s_4}\left| {\left[ {.4, .6} \right]} \right.} \right\}, \left\{ {{s_4}\left| {\left[ {.3, .4} \right]} \right., {s_5}\left| {\left[ {.3, .6} \right]} \right.} \right\}} \right\} |
{X_3} | \left\{ {\left\{ {{s_2}\left| {\left[ {.6, .7} \right]} \right.} \right\}, \left\{ {{s_1}\left| {\left[ {.3, .6} \right]} \right.} \right\}, \left\{ {{s_6}\left| {\left[ {.2, .4} \right]} \right.} \right\}} \right\} |
{Z_4} | |
{X_1} | \left\{ {\left\{ {{s_3}\left| {\left[ {.2, .5} \right]} \right.} \right\}, \left\{ {{s_4}\left| {\left[ {.1, .6} \right], {s_6}\left| {\left[ {.2, .4} \right]} \right.} \right.} \right\}, \left\{ {{s_1}\left| {\left[ {.1, .3} \right]} \right., {s_2}\left| {\left[ {.1, .4} \right]} \right.} \right\}} \right\} |
{X_2} | \left\{ {\left\{ {{s_4}\left| {\left[ {.4, .6} \right]} \right.} \right\}, \left\{ {{s_2}\left| {\left[ {.4, .7} \right], } \right.} \right\}, \left\{ {{s_3}\left| {\left[ {.3, .8} \right]} \right.} \right\}} \right\} |
{X_3} | \left\{ {\left\{ {{s_4}\left| {\left[ {.4, .7} \right]} \right.} \right\}, \left\{ {{s_3}\left| {\left[ {.1, .4} \right]} \right.} \right\}, \left\{ {{s_5}\left| {\left[ {.2, .6} \right]} \right.} \right\}} \right\} |
{Z_1} | |
{X_1} | \left\{ {\left\{ {{s_5}\left| {\left[ {.3, .5} \right]} \right.} \right\}, \left\{ {{s_4}\left| {\left[ {.2, .3} \right]} \right.} \right\}, \left\{ {{s_2}\left| {\left[ {.4, .5} \right]} \right.} \right\}} \right\} |
{X_2} | \left\{ {\left\{ {{s_4}\left| {\left[ {.4, .5} \right]} \right.} \right\}, \left\{ {{s_2}\left| {\left[ {.1, .3} \right]} \right.} \right\}, \left\{ {{s_5}\left| {\left[ {.5, .6} \right]} \right.} \right\}} \right\} |
{X_3} | \left\{ {\left\{ {{s_4}\left| {\left[ {.2, .7} \right]} \right.} \right\}, \left\{ {{s_3}\left| {\left[ {.5, .8} \right]} \right.} \right\}, \left\{ {{s_2}\left| {\left[ {.4, .5} \right]} \right.} \right\}} \right\} |
{Z_2} | |
{X_1} | \left\{ {\left\{ {{s_3}\left| {\left[ {.2, .6} \right]} \right., {s_4}\left| {\left[ {.2, .6} \right]} \right.} \right\}, \left\{ {{s_3}\left| {\left[ {.2, .4} \right]} \right.} \right\}, \left\{ {{s_4}\left| {\left[ {.3, .5} \right]} \right.} \right\}} \right\} |
{X_2} | \left\{ {\left\{ {{s_4}\left| {\left[ {.2, .45} \right]} \right.} \right\}, \left\{ {{s_2}\left| {\left[ {.2, .25} \right]} \right.} \right\}, \left\{ {{s_5}\left| {\left[ {.1, .2} \right]} \right.} \right\}} \right\} |
{X_3} | \left\{ {\left\{ {{s_6}\left| {\left[ {.3, .6} \right]} \right.} \right\}, \left\{ {{s_2}\left| {\left[ {.2, .4} \right]} \right.} \right\}, \left\{ {{s_4}\left| {\left[ {.3, .6} \right]} \right.} \right\}} \right\} |
{Z_3} | |
{X_1} | \left\{ {\left\{ {{s_6}\left| {\left[ {.3, .4} \right]} \right.} \right\}, \left\{ {{s_4}\left| {\left[ {.2, .5} \right]} \right.} \right\}, \left\{ {{s_1}\left| {\left[ {.1, .9} \right]} \right.} \right\}} \right\} |
{X_2} | \left\{ {\left\{ {{s_5}\left| {\left[ {.3, .4} \right]} \right.} \right\}, \left\{ {{s_1}\left| {\left[ {.1, .3} \right]} \right.} \right\}, \left\{ {{s_4}\left| {\left[ {.3, .4} \right]} \right.} \right\}} \right\} |
{X_3} | \left\{ {\left\{ {{s_5}\left| {\left[ {.3, .5} \right]} \right.} \right\}, \left\{ {{s_3}\left| {\left[ {.4, .5} \right]} \right.} \right\}, \left\{ {{s_3}\left| {\left[ {.1, .6} \right]} \right.} \right\}} \right\} |
{Z_4} | |
{X_1} | \left\{ {\left\{ {{s_4}\left| {\left[ {.4, .7} \right]} \right.} \right\}, \left\{ {{s_3}\left| {\left[ {.1, .4} \right]} \right.} \right\}, \left\{ {{s_5}\left| {\left[ {.2, .6} \right]} \right.} \right\}} \right\} |
{X_2} | \left\{ {\left\{ {{s_1}\left| {\left[ {0.4, 0.5} \right], {s_4}\left| {\left[ {0.2, 0.5} \right]} \right.} \right.} \right\}, \left\{ {{s_2}\left| {\left[ {0.1, 0.3} \right]} \right.} \right\}, \left\{ {{s_5}\left| {\left[ {0.5, 0.6} \right]} \right.} \right\}} \right\} |
{X_3} | \left\{ {\left\{ {{s_2}\left| {\left[ {.1, .5} \right]} \right.} \right\}, \left\{ {{s_3}\left| {\left[ {.2, .4} \right]} \right.} \right\}, \left\{ {{s_2}\left| {\left[ {.3, .8} \right]} \right.} \right\}} \right\} |
{Z_1} | |
{X_1} | \left\{ {\left\{ {{s_5}\left| {\left[ {.4, .5} \right]} \right., {s_6}\left| {\left[ {.3, .5} \right]} \right.} \right\}, \left\{ {{s_2}\left| {\left[ {.3, .9} \right]} \right.} \right\}, \left\{ {{s_6}\left| {\left[ {.3, .6} \right]} \right.} \right\}} \right\} |
{X_2} | \left\{ {\left\{ {{s_4}\left| {\left[ {.2, .45} \right]} \right.} \right\}, \left\{ {{s_4}\left| {\left[ {.2, .25} \right]} \right.} \right\}, \left\{ {{s_2}\left| {\left[ {.1, 0.7} \right], {s_5}\left| {\left[ {.2, 0.3} \right]} \right.} \right.} \right\}} \right\} |
{X_3} | \left\{ {\left\{ {{s_5}\left| {\left[ {.1, .2} \right]} \right.} \right\}, \left\{ {{s_3}\left| {\left[ {.4, .5} \right]} \right.} \right\}, \left\{ {{s_6}\left| {\left[ {.6, .9} \right]} \right.} \right\}} \right\} |
{Z_2} | |
{X_1} | \left\{ {\left\{ {{s_3}\left| {\left[ {.2, .45} \right], {s_5}\left| {\left[ {.4, .55} \right]} \right.} \right.} \right\}, \left\{ {{s_3}\left| {\left[ {.2, .6} \right]} \right.} \right\}, \left\{ {{s_6}\left| {\left[ {.7, .9} \right]} \right.} \right\}} \right\} |
{X_2} | \left\{ {\left\{ {{s_2}\left| {\left[ {.3, .6} \right]} \right.} \right\}, \left\{ {{s_6}\left| {\left[ {.4, .5} \right]} \right.} \right\}, \left\{ {{s_3}\left| {\left[ {.3, .9} \right]} \right.} \right\}} \right\} |
{X_3} | \left\{ {\left\{ {{s_5}\left| {\left[ {.2, .4} \right]} \right.} \right\}, \left\{ {{s_3}\left| {\left[ {.3, .2} \right], {s_4}\left| {\left[ {.1, .8} \right]} \right.} \right.} \right\}, \left\{ {{s_6}\left| {\left[ {.3, .6} \right]} \right.} \right\}} \right\} |
{Z_3} | |
{X_1} | \left\{ {\left\{ {{s_3}\left| {\left[ {.3, .6} \right]} \right., {s_5}\left| {\left[ {.2, .4} \right]} \right.} \right\}, \left\{ {{s_2}\left| {\left[ {.2, .5} \right]} \right.} \right\}, \left\{ {{s_1}\left| {\left[ {.4, .7} \right]} \right.} \right\}} \right\} |
{X_2} | \left\{ {\left\{ {{s_3}\left| {\left[ {.3, .5} \right]} \right.} \right\}, \left\{ {{s_3}\left| {\left[ {.1, .4} \right]} \right.} \right\}, \left\{ {{s_4}\left| {\left[ {.4, .6} \right]} \right.} \right\}} \right\} |
{X_3} | \left\{ {\left\{ {{s_6}\left| {\left[ {.3, .6} \right]} \right.} \right\}, \left\{ {{s_2}\left| {\left[ {.2, .4} \right]} \right.} \right\}, \left\{ {{s_4}\left| {\left[ {.3, .6} \right]} \right.} \right\}} \right\} |
{Z_4} | |
{X_1} | \left\{ {\left\{ {{s_3}\left| {\left[ {.5, .6} \right]} \right.} \right\}, \left\{ {{s_1}\left| {\left[ {.3, .4} \right]} \right.} \right\}, \left\{ {{s_4}\left| {\left[ {.4, .6} \right]} \right.} \right\}} \right\} |
{X_2} | \left\{ {\left\{ {{s_5}\left| {\left[ {.3, .5} \right], {s_6}\left| {\left[ {.3, .4} \right]} \right.} \right.} \right\}, \left\{ {{s_3}\left| {\left[ {.1, .4} \right]} \right.} \right\}, \left\{ {{s_4}\left| {\left[ {.3, .5} \right]} \right.} \right\}} \right\} |
{X_3} | \left\{ {\left\{ {{s_3}\left| {\left[ {.4, .5} \right]} \right.} \right\}, \left\{ {{s_5}\left| {\left[ {.2, .5} \right]} \right.} \right\}, \left\{ {{s_4}\left| {\left[ {.5, .6} \right]} \right.} \right\}} \right\} |
{Z_1} | |
{X_1} | \left\{ {\left\{ {{s_{4.714}}\left| {\left[ {0.036, 0.125} \right], {s_{5.3258}}\left| {\left[ {0.027, 0.125} \right]} \right.} \right.} \right\}, \left\{ {{s_{3.285}}\left| {\left[ {0.006, 0.054} \right]} \right.} \right\}, \left\{ {{s_{3.1037}}\left| {\left[ {0.024, 0.12} \right]} \right.} \right\}} \right\} |
{X_2} | \left\{ \begin{array}{l} \left\{ {{s_{3.7135}}\left| {\left[ {0.008, 0.1125} \right], {s_{5.1024}}\left| {\left[ {0.032, 0.1125} \right]} \right.} \right.} \right\}, \left\{ {{s_{3.9193}}\left| {\left[ {0.001, 0.063} \right]} \right.} \right\}, \\ \left\{ {{s_{3.1982}}\left| {\left[ {0.01, 0.252} \right], {s_{4.6141}}\left| {\left[ {0.02, 0.108} \right]} \right.} \right.} \right\} \\ \end{array} \right\} |
{X_3} | \left\{ {\left\{ {{s_{4.485}}\left| {\left[ {0.002, 0.112} \right]} \right.} \right\}, \left\{ {{s_3}\left| {\left[ {0.06, 0.16} \right]} \right.} \right\}, \left\{ {{s_{4.3166}}\left| {\left[ {0.048, 0.36} \right]} \right.} \right\}} \right\} |
{Z_2} | |
{X_1} | \left\{ \begin{array}{l} \left\{ {{s_{2.7274}}\left| {\left[ {0.008, 0.162} \right], {s_{3.1}}\left| {\left[ {0.008, 0.162} \right]} \right., } \right.{s_{4.017}}\left| {\left[ {0.016, 0.198} \right], {s_{4.1796}}\left| {\left[ {0.016, 0.198} \right]} \right.} \right.} \right\} \\ \left\{ {{s_3}\left| {\left[ {0.02, 0.168} \right]} \right.} \right\}, \left\{ {{s_{2.856}}\left| {\left[ {0.021, 0.225} \right]} \right.} \right\} \\ \end{array} \right\} |
{X_2} | \left\{ {\left\{ {{s_{3.4985}}\left| {\left[ {0.03, 0.189} \right]} \right.} \right\}, \left\{ {{s_{2.4183}}\left| {\left[ {0.016, 0.05} \right]} \right.} \right\}, \left\{ {{s_{3.3913}}\left| {\left[ {0.015, 0.126} \right]} \right.} \right\}} \right\} |
{X_3} | \left\{ {\left\{ {{s_{4.9253}}\left| {\left[ {0.024, 0.168} \right]} \right.} \right\}, \left\{ \begin{array}{l} {s_{1.8327}}\left| {\left[ {0.012, 0.032} \right], {s_{3.4933}}\left| {\left[ {0.018, 0.048} \right]} \right., } \right. \\ {s_{2.0562}}\left| {\left[ {0.004, 0.128} \right], {s_{3.9193}}\left| {\left[ {0.006, 0.192} \right]} \right.} \right. \\ \end{array} \right\}, \left\{ {{s_{4.7043}}\left| {\left[ {0.009, 0.108} \right]} \right.} \right\}} \right\} |
{Z_3} | |
{X_1} | \left\{ {\left\{ {{s_{5.4302}}\left| {\left[ {0.027, 0.096} \right], {s_{5.7017}}\left| {\left[ {0.018, 0.064} \right]} \right.} \right.} \right\}, \left\{ {{s_{3.0314}}\left| {\left[ {0.008, 0.125} \right]} \right.} \right\}, \left\{ {{s_1}\left| {\left[ {0.004, 0.567} \right]} \right.} \right\}} \right\} |
{X_2} | \left\{ {\left\{ {{s_{3.7783}}\left| {\left[ {0.018, 0.1} \right]} \right.} \right\}, \left\{ {{s_{2.5562}}\left| {\left[ {0.004, 0.072} \right]} \right.} \right\}, \left\{ {{s_4}\left| {\left[ {0.036, 0.096} \right], {s_{4.3346}}\left| {\left[ {0.036, 0.144} \right]} \right.} \right.} \right\}} \right\} |
{X_3} | \left\{ {\left\{ {{s_{5.1676}}\left| {\left[ {0.054, 0.21} \right]} \right.} \right\}, \left\{ {{s_{1.7176}}\left| {\left[ {0.024, 0.12} \right]} \right.} \right\}, \left\{ {{s_{4.3198}}\left| {\left[ {0.006, 0.144} \right]} \right.} \right\}} \right\} |
{Z_4} | |
{X_1} | \left\{ \begin{array}{l} \left\{ {{s_{3.3112}}\left| {\left[ {0.04, 0.21} \right]} \right.} \right\}, \left\{ {{s_{2.1441}}\left| {\left[ {0.003, 0.096} \right], {s_{2.4811}}\left| {\left[ {0.006, 0.064} \right]} \right.} \right.} \right\}, \\ \left\{ {{s_{2.562}}\left| {\left[ {0.008, 0.108} \right], {s_{3.2881}}\left| {\left[ {0.008, 0.144} \right]} \right.} \right.} \right\} \\ \end{array} \right\} |
{X_2} | \left\{ \begin{array}{l} \left\{ {{s_{4.2646}}\left| {\left[ {0.048, 0.15} \right], {s_{4.485}}\left| {\left[ {0.024, 0.15} \right]} \right.} \right., {s_{5.0548}}\left| {\left[ {0.048, 0.12} \right]} \right., {s_{5.1844}}\left| {\left[ {0.024, 0.12} \right]} \right.} \right\}, \\ \left\{ {{s_{2.3522}}\left| {\left[ {0.004, 0.084} \right]} \right.} \right\}, \left\{ {{s_{3.8049}}\left| {\left[ {0.045, 0.24} \right]} \right.} \right\} \\ \end{array} \right\} |
{X_3} | \left\{ {\left\{ {{s_{3.3199}}\left| {\left[ {0.016, 0.175} \right]} \right.} \right\}, \left\{ {{s_{3.6801}}\left| {\left[ {0.004, 0.08} \right]} \right.} \right\}, \left\{ {{s_{3.6703}}\left| {\left[ {0.03, 0.288} \right]} \right.} \right\}} \right\} |
{Z_1} | |
{X_1} | \left\{ {\left\{ {{s_{2.3068}}\left| {\left[ {0.036, 0.125} \right], {s_{2.6845}}\left| {\left[ {0.027, 0.125} \right]} \right.} \right.} \right\}, \left\{ {{s_{6.49}}\left| {\left[ {0.006, 0.054} \right]} \right.} \right\}, \left\{ {{s_{6.4532}}\left| {\left[ {0.024, 0.12} \right]} \right.} \right\}} \right\} |
{X_2} | \left\{ \begin{array}{l} \left\{ {{s_{1.7653}}\left| {\left[ {0.008, 0.1125} \right], {s_{2.5405}}\left| {\left[ {0.032, 0.1125} \right]} \right.} \right.} \right\}, \left\{ {{s_{6.6055}}\left| {\left[ {0.001, 0.063} \right]} \right.} \right\}, \\ \left\{ {{s_{6.4726}}\left| {\left[ {0.01, 0.252} \right], {s_{6.7142}}\left| {\left[ {0.02, 0.108} \right]} \right.} \right.} \right\} \\ \end{array} \right\} |
{X_3} | \left\{ {\left\{ {{s_{2.1765}}\left| {\left[ {0.002, 0.112} \right]} \right.} \right\}, \left\{ {{s_{6.4313}}\left| {\left[ {0.06, 0.16} \right]} \right.} \right\}, \left\{ {{s_{6.6696}}\left| {\left[ {0.048, 0.36} \right]} \right.} \right\}} \right\} |
{Z_2} | |
{X_1} | \left\{ \begin{array}{l} \left\{ {{s_{1.8387}}\left| {\left[ {0.008, 0.162} \right], {s_{2.0971}}\left| {\left[ {0.008, 0.162} \right]} \right., } \right.{s_{2.7541}}\left| {\left[ {0.016, 0.198} \right], {s_{2.8751}}\left| {\left[ {0.016, 0.198} \right]} \right.} \right.} \right\} \\ \left\{ {{s_{5.4288}}\left| {\left[ {0.02, 0.168} \right]} \right.} \right\}, \left\{ {{s_{5.3493}}\left| {\left[ {0.021, 0.225} \right]} \right.} \right\} \\ \end{array} \right\} |
{X_2} | \left\{ {\left\{ {{s_{2.3782}}\left| {\left[ {0.03, 0.189} \right]} \right.} \right\}, \left\{ {{s_{5.0889}}\left| {\left[ {0.016, 0.05} \right]} \right.} \right\}, \left\{ {{s_{5.6322}}\left| {\left[ {0.015, 0.126} \right]} \right.} \right\}} \right\} |
{X_3} | \left\{ {\left\{ {{s_{3.4581}}\left| {\left[ {0.024, 0.168} \right]} \right.} \right\}, \left\{ \begin{array}{l} {s_{4.6827}}\left| {\left[ {0.012, 0.032} \right], {s_{5.6825}}\left| {\left[ {0.018, 0.048} \right]} \right., } \right. \\ {s_{4.8472}}\left| {\left[ {0.004, 0.128} \right], {s_{5.8821}}\left| {\left[ {0.006, 0.192} \right]} \right.} \right. \\ \end{array} \right\}, \left\{ {{s_{6.2132}}\left| {\left[ {0.009, 0.108} \right]} \right.} \right\}} \right\} |
{Z_3} | |
{X_1} | \left\{ {\left\{ {{s_{3.1373}}\left| {\left[ {0.027, 0.096} \right], {s_{3.3547}}\left| {\left[ {0.018, 0.064} \right]} \right.} \right.} \right\}, \left\{ {{s_{6.1742}}\left| {\left[ {0.008, 0.125} \right]} \right.} \right\}, \left\{ {{s_{5.228}}\left| {\left[ {0.004, 0.567} \right]} \right.} \right\}} \right\} |
{X_2} | \left\{ {\left\{ {{s_{2.056}}\left| {\left[ {0.018, 0.1} \right]} \right.} \right\}, \left\{ {{s_{6.0183}}\left| {\left[ {0.004, 0.072} \right]} \right.} \right\}, \left\{ {{s_{6.4364}}\left| {\left[ {0.036, 0.096} \right], {s_{6.5144}}\left| {\left[ {0.036, 0.144} \right]} \right.} \right.} \right\}} \right\} |
{X_3} | \left\{ {\left\{ {{s_{2.9428}}\left| {\left[ {0.054, 0.21} \right]} \right.} \right\}, \left\{ {{s_{5.6698}}\left| {\left[ {0.024, 0.12} \right]} \right.} \right\}, \left\{ {{s_{6.5111}}\left| {\left[ {0.006, 0.144} \right]} \right.} \right\}} \right\} |
{Z_4} | |
{X_1} | \left\{ \begin{array}{l} \left\{ {{s_{2.5632}}\left| {\left[ {0.04, 0.21} \right]} \right.} \right\}, \left\{ {{s_{4.1102}}\left| {\left[ {0.003, 0.096} \right], {s_{4.3893}}\left| {\left[ {0.006, 0.064} \right]} \right.} \right.} \right\}, \\ \left\{ {{s_{4.4531}}\left| {\left[ {0.008, 0.108} \right], {s_{4.9823}}\left| {\left[ {0.008, 0.144} \right]} \right.} \right.} \right\} \\ \end{array} \right\} |
{X_2} | \left\{ \begin{array}{l} \left\{ {{s_{3.3433}}\left| {\left[ {0.048, 0.15} \right], {s_{3.5308}}\left| {\left[ {0.024, 0.15} \right]} \right.} \right., {s_{4.035}}\left| {\left[ {0.048, 0.12} \right]} \right., {s_{4.1549}}\left| {\left[ {0.024, 0.12} \right]} \right.} \right\}, \\ \left\{ {{s_{4.2851}}\left| {\left[ {0.004, 0.084} \right]} \right.} \right\}, \left\{ {{s_{5.3206}}\left| {\left[ {0.045, 0.24} \right]} \right.} \right\} \\ \end{array} \right\} |
{X_3} | \left\{ {\left\{ {{s_{2.5701}}\left| {\left[ {0.016, 0.175} \right]} \right.} \right\}, \left\{ {{s_{5.2413}}\left| {\left[ {0.004, 0.08} \right]} \right.} \right\}, \left\{ {{s_{5.235}}\left| {\left[ {0.03, 0.288} \right]} \right.} \right\}} \right\} |
Parameter | Closeness ratio | Ranking order | Best alternative |
q = 3 | {\Xi _1} = 0.5679 , {\Xi _2} = 0.6124 , {\Xi _3} = 0.3880 | {X_2} > {X_1} > {X_3} | {X_2} |
q = 4 | {\Xi _1} = 0.5526 , {\Xi _2} = 0.6080 , {\Xi _3} = 0.3804 | {X_2} > {X_1} > {X_3} | {X_2} |
q = 5 | {\Xi _1} = 0.5314 , {\Xi _2} = 0.6027 , {\Xi _3} = 0.3740 | {X_2} > {X_1} > {X_3} | {X_2} |
q = 6 | {\Xi _1} = 0.5213 , {\Xi _2} = 0.5880 , {\Xi _3} = 0.3710 | {X_2} > {X_1} > {X_3} | {X_2} |
q = 7 | {\Xi _1} = 0.5160 , {\Xi _2} = 0.5769 , {\Xi _3} = 0.3660 | {X_2} > {X_1} > {X_3} | {X_2} |
q = 8 | {\Xi _1} = 0.5100 , {\Xi _2} = 0.5700 , {\Xi _3} = 0.3590 | {X_2} > {X_1} > {X_3} | {X_2} |
q = 9 | {\Xi _1} = 0.5055 , {\Xi _2} = 0.5650 , {\Xi _3} = 0.3509 | {X_2} > {X_1} > {X_3} | {X_2} |
q = 10 | {\Xi _1} = 0.4970 , {\Xi _2} = 0.5560 , {\Xi _3} = 0.3459 | {X_2} > {X_1} > {X_3} | {X_2} |
Methods | Closeness ratio/Score values | Ranking of alternatives | Best option |
PLq‐ROFWA [42] |
SC\left( {{X_1}} \right) = 0.4671 , SC\left( {{X_2}} \right) = 0.5890 , SC\left( {{X_3}} \right) = 0.3910 |
{X_2} > {X_1} > {X_3} | {X_2} |
IVPLq-ROFS [43] |
SC\left( {{X_1}} \right) = 0.4279 , SC\left( {{X_2}} \right) = 0.6511 , SC\left( {{X_3}} \right) = 0.6345 |
{X_2} > {X_3} > {X_1} | {X_2} |
Proposed method | {\Xi _1} = 0.5679 , {\Xi _2} = 0.6124 , {\Xi _3} = 0.3880 | {X_2} > {X_1} > {X_3} | {X_2} |
Set | Parameter | Involvement of abstinence degree | Involvement of linguistic term | Involvement of probability | Involvement of interval-valued probability | Computational complexity |
Lt-SFS [38] | Yes | Yes | Yes | No | No | Relatively high |
PLTS [60] | No | No | Yes | Yes | No | Relatively high |
IVPLTS [61] | No | No | Yes | Yes | Yes | Relatively high |
PLT [62] | No | No | Yes | Yes | No | Relatively high |
TOPSIS-based PLTS [63] | No | No | Yes | Yes | No | Relatively high |
Pt-SHFS [40] | Yes | Yes | No | Yes | No | Relatively high |
PLq‐ROFWA) [42] | Yes | No | Yes | Yes | No | Relatively high |
IVPLq-ROFS [43] | Yes | No | Yes | Yes | Yes | Relatively high |
Proposed method | Yes | Yes | Yes | Yes | Yes | Low |