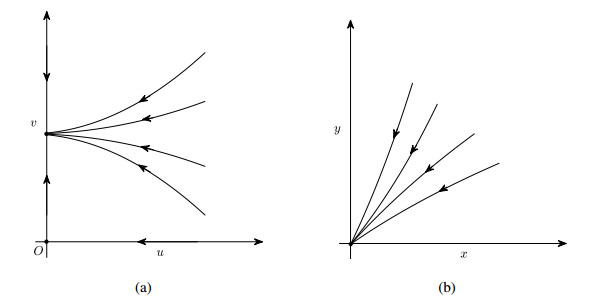
This research introduced cubic bipolar neutrosophic sets (CBNSs), a novel framework that significantly enhanced the capabilities of bipolar neutrosophic sets (BNSs) in handling uncertainty and vagueness within data analysis. By integrating bipolarity and cubic sets, CBNSs provide a more comprehensive and accurate representation of information. We have defined key operations for CBNSs and thoroughly investigated their structural properties. Additionally, we have introduced cubic bipolar neutrosophic soft sets (CBNSSs) as a flexible parameterization tool for CBNSs. To validate the practical utility of CBNSs, we conducted a case study in decision-making. Our algorithmic approach effectively addressed the challenges posed by uncertainty and vagueness in the decision-making process. The results of our research unequivocally demonstrated the superiority of CBNSs over existing methods in terms of accuracy, flexibility, and applicability. By offering a more nuanced representation of information, CBNSs provide a valuable tool for researchers and practitioners tackling complex decision problems.
Citation: Khulud Fahad Bin Muhaya, Kholood Mohammad Alsager. Extending neutrosophic set theory: Cubic bipolar neutrosophic soft sets for decision making[J]. AIMS Mathematics, 2024, 9(10): 27739-27769. doi: 10.3934/math.20241347
[1] | Weili Kong, Yuanfu Shao . Bifurcations of a Leslie-Gower predator-prey model with fear, strong Allee effect and hunting cooperation. AIMS Mathematics, 2024, 9(11): 31607-31635. doi: 10.3934/math.20241520 |
[2] | Fatao Wang, Ruizhi Yang, Yining Xie, Jing Zhao . Hopf bifurcation in a delayed reaction diffusion predator-prey model with weak Allee effect on prey and fear effect on predator. AIMS Mathematics, 2023, 8(8): 17719-17743. doi: 10.3934/math.2023905 |
[3] | Yudan Ma, Ming Zhao, Yunfei Du . Impact of the strong Allee effect in a predator-prey model. AIMS Mathematics, 2022, 7(9): 16296-16314. doi: 10.3934/math.2022890 |
[4] | Chengchong Lu, Xinxin Liu, Zhicheng Li . The dynamics and harvesting strategies of a predator-prey system with Allee effect on prey. AIMS Mathematics, 2023, 8(12): 28897-28925. doi: 10.3934/math.20231481 |
[5] | Teekam Singh, Ramu Dubey, Vishnu Narayan Mishra . Spatial dynamics of predator-prey system with hunting cooperation in predators and type I functional response. AIMS Mathematics, 2020, 5(1): 673-684. doi: 10.3934/math.2020045 |
[6] | Heping Jiang . Complex dynamics induced by harvesting rate and delay in a diffusive Leslie-Gower predator-prey model. AIMS Mathematics, 2023, 8(9): 20718-20730. doi: 10.3934/math.20231056 |
[7] | Khairul Saleh . Basins of attraction in a modified ratio-dependent predator-prey model with prey refugee. AIMS Mathematics, 2022, 7(8): 14875-14894. doi: 10.3934/math.2022816 |
[8] | Ali Yousef, Ashraf Adnan Thirthar, Abdesslem Larmani Alaoui, Prabir Panja, Thabet Abdeljawad . The hunting cooperation of a predator under two prey's competition and fear-effect in the prey-predator fractional-order model. AIMS Mathematics, 2022, 7(4): 5463-5479. doi: 10.3934/math.2022303 |
[9] | Kottakkaran Sooppy Nisar, G Ranjith Kumar, K Ramesh . The study on the complex nature of a predator-prey model with fractional-order derivatives incorporating refuge and nonlinear prey harvesting. AIMS Mathematics, 2024, 9(5): 13492-13507. doi: 10.3934/math.2024657 |
[10] | Nazmul Sk, Bapin Mondal, Abhijit Sarkar, Shyam Sundar Santra, Dumitru Baleanu, Mohamed Altanji . Chaos emergence and dissipation in a three-species food web model with intraguild predation and cooperative hunting. AIMS Mathematics, 2024, 9(1): 1023-1045. doi: 10.3934/math.2024051 |
This research introduced cubic bipolar neutrosophic sets (CBNSs), a novel framework that significantly enhanced the capabilities of bipolar neutrosophic sets (BNSs) in handling uncertainty and vagueness within data analysis. By integrating bipolarity and cubic sets, CBNSs provide a more comprehensive and accurate representation of information. We have defined key operations for CBNSs and thoroughly investigated their structural properties. Additionally, we have introduced cubic bipolar neutrosophic soft sets (CBNSSs) as a flexible parameterization tool for CBNSs. To validate the practical utility of CBNSs, we conducted a case study in decision-making. Our algorithmic approach effectively addressed the challenges posed by uncertainty and vagueness in the decision-making process. The results of our research unequivocally demonstrated the superiority of CBNSs over existing methods in terms of accuracy, flexibility, and applicability. By offering a more nuanced representation of information, CBNSs provide a valuable tool for researchers and practitioners tackling complex decision problems.
The establishment and analysis of differential equation models have proven to be an effective approach for understanding and predicting the dynamics of biological populations. The prey-predator relationship is one of basic relationships of species interactions in ecology, so a significant number of ordinary differential prey-predator models have been studied in recent years; refer to [1,2,3,4]. A key area of interest is the Leslie-Gower prey-predator model [5], which can be expressed in the following form:
{dxdt=x(1−x)−βxy,dydt=μy(1−yx), | (1.1) |
where x and y denote the densities of prey and predator, respectively, and the parameters β, μ are positive constants. Korobeinikov [6] has proved that the unique positive singularity of (1.1) is globally asymptotically stable by constructing Lyapunov function.
The prey in system (1.1) grows at a logistic pattern, which posits that population growth will accelerate when density is low. However, in the real natural environment, due to factors such as mate limitation, homologous reproduction, cooperative defense, and environmental conditioning, it was recognized that the prey species may have a growth rate of Allee effect in recent years; see references [7,8,9,10,11]. Mathematically, the Allee effect is often expressed as the following growth model:
dxdt=x(1−x)(xb−1), | (1.2) |
where x is the population size and b is the Allee threshold. The Allee effect is usually divided into two types: strong Allee effect when 0<b<1 and weak Allee effect when b≤0. The dynamic properties of strong and weak Allee effects are fundamentally distinct. In the case of strong Allee effect, if the population size falls below the Allee threshold b, the population will become extinct. The weak Allee effect shares similar dynamic properties with the logistic growth model, that is, the population tends to the maximum environmental carrying capacity as t→∞. Therefore, in order to avoid the extinction of populations, people are more interested in studying population models with strong Allee effects, and there have been many research achievements in recent years.
Considering the influence of strong Allee effect on prey in (1.1), Ni and Wang [7] established and discussed the following prey-predator system with strong Allee efect on prey
{dxdt=x(1−x)(x/b−1)−βxy,dydt=μy(1−yx), | (1.3) |
where 0<b<1 is the Allee threshold. They investigated the dynamical properties of solutions and the unstable and stable manifolds of the positive singularity when the system (1.3) has only one positive singularity. Min and Wang [8] discussed Hopf bifurcation of (1.3) in great detail and used the center manifold and normal form theory to determine the direction of the Hopf bifurcation and the stability of the branched periodic solutions.
The functional response plays a crucial role in prey-predator models, as it describes the interaction between the prey and predator populations in nature. Functional responses are often divided into prey-dependent type, such as Holling type functional response and prey-predator-dependent, like ratio-dependent functional response and Bddington-DeAngelis functional response. The interference and cooperation between conspecific predators is a common phenomenon. For example, bats prevent other bats from feeding by emitting special interference sounds, while wild dogs cooperate to attack prey to increase their chances of success in hunting. Therefore, prey-predator-dependent functional responses are more consistent with the real situation in nature than prey-dependent functional responses. However, as far as we know, most of prey-predator-dependent functional responses assume that predators are mutually interfering with each other, such as the ratio-dependent functional response and Bddington-DeAngelis functional response. On the contrary, the cooperative hunting relationships between predators are rarely considered. In 2017, considering the cooperative hunting behavior observed among predators, Alves and Hilker [12] developed the following prey-predator-dependent type functional response
Q(x,y)=(λ+ay)x, |
where λ is the attack rate of the predator, and a describes the intensity of hunting cooperation among predators. In recent years, some scholars have begun to investigate the impact of cooperative hunting on predator-prey models; see references [13,14,15,16,17]. Ye and Wu [13] incorporated the factor of cooperative hunting in system (1.3) and studied its dynamic properties.
As people's demand for natural resources increases, the issue of how to meet this demand without disrupting the balance of ecosystems has become an increasingly important topic. Therefore, introducing a harvesting term into prey-predator models has significant practical significance. In fact, harvesting of biological populations has been widely practiced in industries such as forestry, fishing, and wildlife resource management. The introduction of harvesting will make the dynamics of ecosystems more complex and diverse, so the impact of harvesting on ecosystems and the role of harvesting in resource management have both been studied by more and more scholars in depth. A common harvesting mechanism is constant-yield harvesting, where the harvesting rate is independent of the number of species being harvested. This type of harvesting has recently attracted the attention of scholars; see references [18,19,20,21,22]. However, it is evident that there are some drawbacks to constant yield harvesting from both a biological and economic perspective. For example, if the population being harvested is very small and below the harvest constant, constant-yield harvesting can easily lead to the extinction of the biological population. On the other hand, May et al. proposed the concept of proportional harvesting, also known as constant-effort harvesting in their paper [23], in which the harvesting rate is proportional to both the number of the harvested species and the human effort invested. Clearly, from an ecological perspective, the constant-effort harvesting is more realistic than constant-yield harvesting.
According to our investigation, there is no research on prey-predator models currently that considers strong Allee effects and harvesting effects in prey and cooperative hunting effects in predators. We are very interested in the dynamics of such models, therefore, this article mainly discusses the following system:
{dxdt=x(1−x)(xb−1)−(1+ay)xy−hx,dydt=μy(1−yx), | (1.4) |
where x and y represent the densities of prey and predator, respectively; paremeters a, μ, h are positive constants, 0<b<1; b represents the threshold of strong Allee effect on the prey, and h represents the harvestable yield of the prey and human effort. Obviously, the system (1.4) is defined on the set Ω={(x,y)|x>0,y≥0} and not defined along the axis x=0, especially at the point (0, 0).
From a mathematical research perspective, the analysis of system (1.4) presents considerable challenges. On the one hand, unlike the classical prey-predator systems, (0,0) is not an equilibrium of (1.4), and the system is not even defined at the axis x=0. Specifically, when 0<x<b and y>0,
x(1−x)(x/b−1)−(1+ay)xy−hx<0, |
which means that x may tend to zero and the term y/x may be unbounded. Such a bad structure will bring a lot of difficulties in the analysis. On the other hand, the interaction of three ecological factors, namely, Allee effect, harvesting effect, and hunting cooperation, makes theoretical analysis more complex. This paper aims to explain how the three ecological factors mentioned above collectively influence the dynamic properties of species.
The layout of this paper is as follows. In Section 2, the positivity and boundedness of solutions are obtained. Specifically, we give the uniform persistence of the system under some conditions. In Section 3, we discuss the existence of nonnegative singularity of (1.4). In Section 4, we analyze the type of all singularities including primary singularities and higher-order singularities. In Section 5, the origin is proved to be an attractor and the basin of attraction is given. In Section 6, we discuss saddle node bifurcation and Hopf bifurcation that occur in system (1.4). In Section 7, we illustrate the conclusions of our theoretical analysis through some numerical simulations.
It is evident that the system (1.4) with nonnegative initial values (x0,y0) has a unique solution (x(t),y(t)) in [0,+∞) by the existence and uniqueness theorem and extension theorem of solutions for ordinary differential equations [24]. In this section, we will give some other basic properties about the system (1.4), such as the positivity and boundedness of solutions, the uniform persistence of the system (1.4), etc.
Proposition 2.1. If x0>0 and y0>0, then any solution (x(t),y(t)) of (1.4) is positive and bounded for t≥0.
Proof. It is obvious that x=0 is an invariant set from the first equation of (1.4). According to the theorem of existence and uniqueness of solutions [24], solutions starting from x0>0 will not intersect with the solusion x=0. Therefore, if x0>0, then x(t)>0 for all t≥0. Similarly, one can also know that if y0>0, then y(t)>0 for all t≥0. Accordingly, every solution of (1.4) with the initial values x0>0 and y0>0 is positive.
On the other hand, since x(t) satisfies
{dxdt≤x(1−x)(xb−1),x(0)=x0>0, | (2.1) |
it follows from the comparison principle that x(t)≤max{1,x0} for t≥0. Denote A=max{1,x0}. From the second equation of (1.4), we have
{dydt≤μy(1−yA),y(0)=y0>0. |
Once again, based on the principle of comparison, it follows that y(t)≤max{A,y0} for t≥0. Therefore, every solution of (1.4) with the initial values x0>0 and y0>0 is bounded.
Remark 2.1. The proof of proposition 2.1 suggests that the set Ω is an invariant set for the system (1.4).
Proposition 2.2. If 0<x0≤b and (x0,y0)≠(b,0), then limt→∞(u(t),v(t))=(0,0).
Proof. If 0<x0<b and y0≥0, then it follows (2.1) that x(t) converges to 0 as t→∞. Thus, for any ε>0, there exists T>0 such that x(t)<ε for t≥T. From the second equation of (1.4), we have
dydt<μy(1−yε), t>T. | (2.2) |
As a result, lim supt→∞y(t)≤ε. The arbitrariness of ε leads to limt→∞y(t)=0.
If x0=b and y0>0, from the first equation of (1.4),
dxdt|t=0=−(1+ay0)x0y0−hx0<0. |
According to the proof of proposition 2.1, we know that if y0>0, then y(t)>0 for all t≥0. To sum up, the solution (x(t),y(t)) of (1.4) satisfies x(t0)<b and y(t0)>0 at some small time t0>0. Then, utilizing results of 0<x0<b and y0≥0, we have
limt→∞x(t)=0, limt→∞y(t)=0. |
In conclusion, the proof is finished.
Remark 2.2. Proposition 2.2 indicates that when the prey density is too low, the prey will perish and the predator also becomes extinct. This phenomenon contradicts the traditional perspective of population dynamics. In fact, this is caused by the Allee effect. From a biological perspective, congregating is conducive to the reproduction and survival of the population. Each species has its own optimal density, and too dense or too sparse will have a restraining effect on the population.
Definition 2.1. [25] If there are two positive numbers ω1 and ω2, such that any positive solution of the system (1.4) with nonnegative initial values (x0,y0) satisfies the following inequalities
min{lim inft→∞x(t),lim inft→∞y(t)}≥ω1,max{lim supt→∞x(t),lim supt→∞y(t)}≤ω2, |
then the system (1.4) is said to be uniformly persistent.
Theorem 2.1. If (1−b)2/(4b)>1+a+h and x0≥x∗0, then the system (1.4) is uniformly persistent, where x∗0=1+b−√(1+b)2−4b(2+a+h)2a.
Proof. Because (1−b)2/(4b)>1+a+h, there exists ε>0 such that (1−b)2/(4b)>1+a+h+ε(1+2a+aε). Let (x(t),y(t)) be the unique solution of (1.4) with nonnegative initial values (x0,y0). From (2.1), we easily get lim supt→∞x(t)≤1. Then, for the above ε, there is T>0 such that x(t)<1+ε for all t≥T. Replace ε with 1+ε in (2.2). Similarly to the proof of Proposition 2.2, since ε here can be arbitrary, we have lim supt→∞y(t)≤1. Consequently,
max{lim supt→∞x(t),lim supt→∞y(t)}≤1. |
In view of the second equation of (1.4), when t is sufficiently large, we have
dxdt≥x(1−x)(xb−1)−[1+a(1+ε)](1+ε)x−hx=−xb{x2−(1+b)x+b[2+a+h+ε(2a+1+aε)]}≜−xbΦ(x) |
It is clear that Φ(x)=0 has two positive roots when (1−b)2/(4b)>1+a+h, which can be denoted as ˆx and ˜x, where
ˆx=1+b−√(1+b)2−4b[2+a+h+ε(2a+1+aε)]2a, |
˜x=1+b+√(1+b)2−4b[2+a+h+ε(2a+1+aε)]2a. |
Furthermore, we have
dxdt≥−xb(x−ˆx)(x−˜x), |
which implies that lim inft→∞x(t)≥ˆx when x0>x∗0.
Similarly, according to the second equation of (1.4), when t is sufficiently large, we have
dydt≥μy(1−yˆx) |
Obviously, lim inft→∞y(t)≥ˆx. Hence, min{lim inft→∞x(t),lim inft→∞y(t)}≥ˆx. In summary, it can be concluded that system (1.4) is uniformly persistent.
Remark 2.3. Theorem 2.1 indicates that prey and predators will always coexist when the quantity of prey is sufficient and the human capture intensity of prey is moderate. From a biological perspective, when the quantity of prey is sufficient and the capture amount is not large, the prey can satisfy the capture of both predator and humans so that the prey and the predator can always coexist.
If (x∗,y∗) is the nonnegative singularities of (1.4), then (x∗,y∗) satisfies the following equations:
{(1−x)(xb−1)−(1+ay)y−h=0,μy(1−yx)=0. | (3.1) |
From the second equation of (3.1), we know that y∗=0 or y∗=x∗. Obviously, the singularities obtained when y∗=0 are the boundary singularities, and the singularities obtained when y∗=x∗ are the positive singularities. Next, we will discuss the two cases of y∗=0 and y∗=x, respectively.
For the case of y∗=0, x∗ is the positive root of equation
x2−(1+b)x+b(1+h)=0. | (3.2) |
Letting Δ1=(1+b)2−4b(1+h) and h1=(1−b)2/(4b), we have the following results:
● If h>h1, then Eq (3.2) has no roots.
● If h=h1, then Eq (3.2) has unique positive root x1, and x1=(1+b)/2.
● If h<h1, then Eq (3.2) has two positive roots x2 and x3, where
x2=12[1+b−√(1+b)2−4b(1+h)] and x3=12[1+b+√(1+b)2−4b(1+h)]. |
For the case of y∗=x∗, x∗ is the positive root of equation
(1+ab)x2−x+b(1+h)=0. | (3.3) |
Denote Δ2=1−4b(1+ab)(1+h), h2=[1−4b(1+ab)]/[4b(1+ab)], and a∗=(1−4b)/(4b2). It easy to obtain h2<h1 by direct calculation. Then, the situation about the roots of (3.3) is as follows:
● When 1/4≤b<1 or a≥a∗, Δ2<0, which means that the Eq (3.3) has no roots.
● When 0<b<1/4 and a<a∗, the value of Δ2 is also related to the parameter h.
(1) If h>h2, then Δ2<0, so the Eq (3.3) has no roots;
(2) If h=h2, then Δ2=0, so the Eq (3.3) has unique positive roots x4 with x4=1/[2(1+ab)];
(3) If h<h2, then Δ2>0, so the Eq (3.3) has two positive roots x5 and x6, where
x5=1−√1−4b(1+ab)(1+h)2(1+ab) and x6=1+√1−4b(1+ab)(1+h)2(1+ab). |
Base on the above discussion, we obtain the following theorem about the existence of nonnegative singularities.
Theorem 3.1. Assume a,h,μ>0, and 0<b<1. Denote h1=(1−b)2/(4b), h2=[1−4b(1+ab)]/[4b(1+ab)], and a∗=(1−4b)/(4b2). Then, the following conclusions about the existence of singularities for the system (1.4) are valid.
(1) If h>h1, then the system (1.4) has no singularity;
(2) If h=h1, then the system (1.4) has unique boundary singularities denoted by E1(x1,0);
(3) If h<h1, then the system (1.4) has two boundary singularities denoted by E2(x2,0) and E3(x3,0), respectively;
(4) When 1/4≤b<1 or a≥a∗, the system (1.4) has no positive singularity;
(5) When 0<b<1/4 and a<a∗. If h>h2, then the system (1.4) has no positive singularity; if h=h2, then the system (1.4) has a unique positive singularity denoted by E4(x4,x4); if h<h2, then the system (1.4) has two positive singularities denoted by E5(x5,x5) and E6(x6,x6), respectively;
where x1, x2, x3, x4, x5, x6 are defined as in above discussions.
From Theorem (3.1), harvesting behavior, hunting cooperation, and Allee effect collectively affect the number of singularities and the system (1.4) has at most four singularities, which imply the system (1.4) is likely to exhibit rich dynamic properties.
Denote x=(x,y) and
G(x)=(f(x,y)g(x,y))=(x(1−x)(xb−1)−(1+ay)xy−hxμy(1−yx)). |
For the simplicity of the notations, we denote
A(xi)=−2bx2i+(1+1b)xi, i=1,2,3,4,5,6. | (4.1) |
The direct analysis reveals what the sign of A(xi) is when i=1,2,3, i.e.,
A(x1)=0, A(x2)>0 and A(x3)<0. |
Because xi(i=1,2,3) satisfy Eq (3.2), the Jacobian matrix of (1.4) at the boundary singularities Ei(xi,0) is
JEi≜Gx((xi,0))=(A(xi) −xi0μ), i=1,2,3. | (4.2) |
Furthermore, the determinant and trace of the Jacobian matrix JEi are, respectively,
det(JEi)=μA(xi) and tr(JEi)=A(xi)+μ, i=1,2,3. | (4.3) |
Similarly, since xi(i=4,5,6) satisfy the Eq (3.3), the Jacobian matrix of (1.4) at the positive singularities Ei(xi,xi) is
JEi≜Gx((xi,xi))=(A(xi) −xi−2ax2iμ−μ), i=4,5,6. | (4.4) |
Accordingly, the determinant and trace of the Jacobian matrix JEi are, respectively,
det(JEi)=μbxi[2(1+ab)xi−1] and tr(JEi)=A(xi)−μ, i=4,5,6. | (4.5) |
Based on (4.3) and (4.5), it is easy to know that Ei(i=2,3,5,6) are primary singularities and Ei(i=1,4) are higher-order singularities. For the primary singularities, the traditional linearization method is used to determine their types. For higher-order singularities, we will apply the method of Theorem 1 and Theorem 3 of Chapter 2 in reference [26] to give their types.
Theorem 4.1. Let a,h,μ>0, and 0<b<1. If h<h1, the boundary singularities E2(x2,0) and E3(x3,0) are an unstable node and a saddle, respectively. Moreover, E2(x2,0) is a degenerate node when μ=A(x2), where x2, x3, h1, and A(x2) are defined as in Theorem 3.1 and (4.1).
Proof. (1) From (4.3) with i=2 and A(x2)>0, we get the determinant and trace of JE2, i.e.,
det(JE2)=μA(x2)>0; tr(JE2)=A(x2)+μ>0. |
Then, further calculation generates
[tr(JE2)]2−4det(JE2)=[μ−A(x2)]2≥0. | (4.6) |
It follows that the linearized matrix JE2 may have a double positive real eigenvalue or two different positive real eigenvalues, respectively, when μ=A(x2) and μ≠A(x2). Thus, E2 is an unstable node. Especially, when μ=A(x2), JE2 is an upper triangular matrix that cannot be diagonalized, so the eigen-space corresponding to its double eigenvalue is one-dimensional, which implies E2 is a degenerate node.
(2) Based on (4.3) and A(x3)<0, it is obvious that
det(JE3)=μA(x3)<0, |
so E3 is a saddle point.
To analyze the stability of E6, we will make the following preparations. According to Theorem 3.1, the following analysis is based on the conditions 0<b<1/4, 0<a<a∗, and 0<h<h2. We care about the sign of A(x6). Let
˜μ=1+b−2x6=1+b−1+√1−4b(1+ab)(1+h)1+ab. |
It is evident that ˜μ and A(x6) have the same sign. Direct observation shows that ˜μ is strictly increasing on h. Moreover,
limh→0˜μ=1+b−1+√1−4b(1+ab)1+ab |
and
limh→h2˜μ=b(1+a+ab)1+ab>0. |
To determine the sign of the limit of ˜μ as h→0, let
˜a=14b2[1−(1−3b)2(1+b)2−4b]. |
Direct calculation shows that ˜a<a∗ and the sign of ˜a is related to the key value b=√5−2. Specifically, if √5−2≤b<1/4, then ˜a≤0. Now, a>˜a, which implies ˜μ>0, i.e., A(x6)>0. If 0<b<√5−2, then ˜a>0. Furthermore, if a≥˜a, then ˜μ≥0, i.e., A(x6)≥0; if 0<a<˜a, then limh→0˜μ<0, and then there exists ˜h such that ˜μ=0. Therefore, when 0<b<√5−2, 0<a<˜a, if 0<h<˜h(h=˜h, ˜h<h<h2, respectively), then ˜μ<0(=0, >0, respectively), i.e., A(x6)<0(=0, >0, respectively).
For the convenience of description, based on the above analysis, the following conditions are summarized:
(a1) 0<b<√5−2, a≥˜a, and 0<h<h2;
(a2) 0<b<√5−2, 0<a<˜a, and 0<h<˜h;
(a3) 0<b<√5−2, 0<a<˜a, and h=˜h;
(a4) 0<b<√5−2, 0<a<˜a, and ˜h<h<h2;
(a5) √5−2≤b<1/4, 0<a<a∗, and 0<h<h2.
According to the above analysis, the following lemma can be obtained.
Lemma 4.1. Let 0<b<1/4, 0<a<a∗, 0<h<h2, ˜a, and ˜h be defined in the above analysis, where x6, a∗, and h2 are defined in Theorem 3.1. Then,
(1) If one of the three conditions (a1), (a4), and (a5) holds, then A(x6)>0;
(2) If the condition (a2) holds, then A(x6)<0;
(3) If the condition (a3) holds, then A(x6)=0.
Theorem 4.2. Suppose 0<b<1/4, 0<a<a∗, 0<h<h2, and μ>0. Let a∗, h2, A(x6), ˜a, and ˜h be defined as in Lemma 4.1. Then, the following conclusions are true.
(1) The positive singularity E5(x5,x5) always is a saddle point.
(2) When one of the three conditions (a1), (a4), and (a5) holds.
(2.1) If μ<A(x6), the positive singularity E6(x6,x6) is an unstable node or focus;
(2.2) If μ>A(x6), E6(x6,x6) is a stable node or focus;
(2.3) If μ=A(x6), E6(x6,x6) is a center or fine focus.
(3) Either the condition (a2) or the condition (a3) holds, and E6(x6,x6) is a stable node or focus.
Proof. Acoording to (4.5) with i=5, it is clear that det(JE5)<0, which implies that E5 a saddle point. On the other hand, we can also know that det(JE6)>0 and tr(JE6)=A(x6)−μ. Therefore, when μ<A(x6), i.e., tr(JE6)>0, E6 is an unstable node or focus; when μ>A(x6), i.e., tr(JE6)<0, E6 is a stable node or focus; when μ=A(x6), i.e., tr(JE6)=0, E6 is a center or fine focus. Combined with the conclusion of Lemma 4.1, this theorem is proved.
Theorem 4.3. Let a,h,μ>0, 0<b<1, and h1 be defined as in Theorem 3.1. When h=h1, the system (1.4) has a unique singularity E1(x1,0), which is a repelling saddle-node.
Proof. From (4.2) and (4.3) with i=1, the eigenvalues of JE1 are 0 and μ>0, which means that E1 is a higher-order singularity of (1.4). To study the type of E1, we first transform E1 to (0,0) by letting (X,Y)=(x−(1+b)/2,y). Then, the system (1.4) becomes
{dXdt=−1+b2Y−1+b2bX2−XY−a(1+b)2Y2+P1(X,Y),dYdt=μY−2μ1+bY2+Q1(X,Y), | (4.7) |
where P1(X,Y) and Q1(X,Y) are C∞ functions of at least third order in terms of (X,Y). Continuing with the transformation (u,v)=(X+(1+b)/(2μ)Y,Y), the system (4.7) becomes
{dudt=−1+b2bu2+[(1+b)22bμ−1]uv−[(1+b)38bμ2+a(1+b)2+1−1+b2μ]v2+P2(u,v),dvdt=μv−2μ1+bv2+Q2(u,v), |
where P2(u,v) and Q2(u,v) are C∞ functions of at least third order in terms of (u,v). Introduce a new time variable τ by τ=μt, and we get
{dudτ=−1+b2bμu2+[(1+b)22bμ2−1μ]uv−[(1+b)38bμ3+α(1+b)2μ+1μ−(1+b)2μ2]v2+P3(u,v),dvdτ=v−21+bv2+Q3(u,v), |
where P3(u,v) and Q3(u,v) are C∞ functions of at least third order in terms of (u,v).
Since the coefficient of u2 is −(1+b)/(2bμ)<0, by Theorem 1 of Chapter 2 in reference [26], the boundary singularity E1 is a repelling saddle-node.
Lemma 4.2. [26] The system
{dxdt=y+a20x2+a11xy+a03y2+o(|x,y|2),dydt=b20x2+b11xy+b02y2+o(|x,y|2), | (4.8) |
is equivalent to the system
{dxdt=y,dydt=b20x2+(b11+2a20)xy+o(|x,y|2), | (4.9) |
after some non-singular transformations in the neighborhood of (0,0).
Theorem 4.4. Let a,h,μ>0, and 0<b<1. If h=h2, the system (1.4) has a unique positive singularity E4(x4,x4). Moreover,
(1) if μ<A(x4), E4 is a repelling saddle-node;
(2) if μ>A(x4), E4 is an attracting saddle-node;
(3) if μ=A(x4) and a2b3+(3a2+2a)b2+(4a2+2a+1)b−a−1≠0, then E4 is a cusp of co-dimension 2;
where h2 and x4 are defined as in Theorem 3.1 and A(x4) are defined in (4.1).
Proof. According to (4.4) and (4.5) with i=4, both eigenvalues of JE4 are λ1=0 and λ2=A(x4)−μ. First, transform E4(x4,x4) to (0,0) by making (X,Y)=(x−x4,y−x4). Then, the system (1.4) becomes
{dXdt=a10X+a01Y+a20X2+a11XY+a02Y2+~P1(X,Y),dYdt=b10X+b01Y+b20X2+b11XY+b02Y2+~Q1(X,Y), | (4.10) |
where
a10=(−3b+a)x24+(1+2b)x4−h2−1, a01=−x4−2ax24, a20=−3bx4+1+1b, |
a11=−1−2ax4, a02=−ax4, b10=μ, b01=−μ, b11=2μx4, b20=−μx4, b02=−μx4, |
and ~P1(X,Y), ~Q1(X,Y) are C∞ functions of at least third order in terms of (X,Y).
(1) When μ≠A(x4), JE4 has only one zero eigenvalue, and we still apply Theorem 7.1 of Chapter 2 in reference [26] to study the type of E4. Make the following transformation:
(X,Y)=(a01u+a10v,−a10u+b10v) and τ=(A(x4)−μ)t. |
Then, system (4.10) becomes (still denoting τ as t)
{dudt=c20u2+c11uv+c02y2+~P2(u,v),dvdt=v+d20u2+d11uv+d02v2+~Q2(u,v), |
where
c20=q1q0, c11=q2q0, c02=q3q0, d20=q4q0, d11=q5q0, d02=q6q0,q0=2a10a01b10+a01b10b01+a310,q1=a210a02b10+a210a01b11+a201a20b10−a10a201b20−a10a01a11b10−a310b02,q2=2a210b10b02−a210a11b10−2a210a01b20+a01a11b210−2a10a02b210+2a10a01a20b10−a10a01b10b11+a310b11,q3=a210a20b10−a210b10b11+a10a11b210−a10b210b02+b310a02−a310b20,q4=a10a201a20+a310a02−a210a01a11+a301b20−a10a201b11+a210a01b02,q5=2a210a01a20+a10a01a11b10−a310a11−2a210a02b10+2a10a201b20+a201b10b11−a210a01b11−2a10a01b10b02,q6=a310a20+a210a11b10+a10b210a02+a210a01b20+a10a01b10b11+b210a01b02, |
and ~P2(u,v), ~Q2(u,v) are C∞ functions of at least third order in terms of (u,v).
Next, we will determine the sign of c20. Rewrite q0 as follows:
q0(μ)=−a01μ2+2a10a01μ+a310 |
and denote its root discriminant as Δq=4a210a201+4a01a310. Direct calculations indicate that Δq<0; thus, it can be determined that q0<0. By calculation, we can know that the sum of the fourth and fifth terms of polynomial q1 is 0. Further calculations yield
q1=aμx242(1+ab)3[(ab+1)2−a(2ab+a+2)−(2ab+a+2)2]<0. |
Therefore, it shows that c20<0. Combining the time variable τ, it follows Theorem 1 of Chapter 2 in reference [26] that E4 is an attracting saddle-node if μ>A(x4), and a repelling saddle-node if μ<A(x4).
(2) When μ=A(x4), both eigenvalues are zero. Let (X,Y)=(a01u,−a10u+v), then system (4.10) becomes
{dudt=v+e20u2+e11uv+e02v2+¯P2(u,v),dvdt=f20u2+f11uv+f02v2+¯Q2(u,v), | (4.11) |
where
e20=l1a01, e11=l2a01, e02=a02a01, f20=l3a01, f11=l4a01, f02=l5a01,l1=a201a20−a10a01a11+a210a02, l2=a01a11−2a10a02,l3=a301b20−a10a201b11+a210a01b02+a10a201a20−a01a210a11+a310a02,l4=a210b11−2a10a01b02+a10a01a11−2a210a02, l5=a01b02+a10a02, |
and ˉP2(u,v), ˉQ2(u,v) are C∞ functions of at least third order in terms of (u,v).
By using Lemma 4.2, it can be obtained that system (4.11) is equivalent to
{dudt=v,dvdt=f20u2+(f11+2e20)uv+¯Q3(u,v), |
in the small neighborhood of (0,0), where ¯Q3(u,v) is a C∞ function of at least third order in terms of (u,v). After complex calculations, we have
f20=x24(1+ab)3[(ab+2a+1)f(a,b)−(ab+a+1)a2]≥x24(ab+a+1)(1+ab)3[f(a,b)−a2]>0, |
where
f(a,b)=(ab+a+1)(1+2ab)2b+a(ab+2a+1)22(ab+a+1), |
and
f11+2e20=−x4(ab+a+1)[a2b3+(3a2+2a)b2+(4a2+2a+1)b−a−1]b(ab+1)3(1+2ax4). |
Hence, if μ=A(x4) and a2b3+(3a2+2a)b2+(4a2+2a+1)b−a−1≠0, then E4 is a cusp of co-dimension 2 by the Theorem 3 of Chapter 2 in reference [26]. In addition, if μ=A(x4) and a2b3+(3a2+2a)b2+(4a2+2a+1)b−a−1=0, then E4 is a cusp of co-dimension at least 3.
Although the system (1.4) is not defined at (0,0), proposition 2.2 implies that the system may exhibit interesting dynamic behavior at (0,0). In order to facilitate the study of the dynamic properties of (1.4) at (0,0), we will use the idea of topological equivalence proposed in [27] to obtain a system that is topologically equivalent to system (1.4) and is defined at (0,0).
Lemma 5.1. The system (1.4) is topologically equivalent to system
{dxdτ=[(1−x)(xb−1)−(1+ay)y−h]x2,dydτ=μy(x−y), | (5.1) |
which is a continuous extension of the system (1.4) and is defined at ˜Ω={(x,y)∈R2|x≥0,y≥0}.
Proof. By means of the time rescaling given by t=xτ and using the chain rule we have
dxdt=dxdτdτdt=1xdxdτ, dydt=dydτdτdt=1xdydτ. |
In view of (1.4), the system (5.1) can be obtained. In fact, we have constructed the function ψ:˜Ω×R⟶Ω×R satifying
ψ(x,y,τ)=(x,y,xτ)=(x,y,t). |
Simple calculations can yield
det(Dψ(x,y,τ))=det(100010τ0x)=x>0. |
Hence, ψ is a diffeomorphism and preserves the orientation of time. It follows that system (5.1) is topologically equivalent to system (1.4). Furthermore, we note that system (5.1) is a fourth order polynomial differential equations system, which guarantees that it can be extended continuously to x=0.
Notice that the Jacobian matrix of system (5.1) at (0,0) is a null matrix. Thus, we will discuss the stability of (5.1) near orgin by the blow-up method in the following.
Theorem 5.1. The origin (0,0) is an attractor in system (1.4).
Proof. According to the Lemma 5.1, we can obtain the dynamical properties of the system (1.4) at (0,0) by studying the trajectory structure of (5.1) near the origin (0, 0). It was clear that the system (5.1) has an invariant line x=0 and dy/dτ|x=0=−μy2<0. Taking the transformation
ϕ:(x,y)↦(u,uv), |
the system (5.1) becomes
{dudτ=u2[(1−u)(ub−1)−(1+αuv)uv−h],dvdτ=v[μ(1−v)−(1−u)(ub−1)+(1+αuv)uv+h]. | (5.2) |
When x≠0, making a time transformation udt=dτ, the system (5.2) becomes
{dudt=u[(1−u)(ub−1)−(1+αuv)uv−h],dvdt=v[μ(1−v)−(1−u)(ub−1)+(1+αuv)uv+h]. | (5.3) |
Systems (5.1) and (5.3) are topologically equivalent, which means their trajectories are the same. The system (5.3) has two boundary singularities (0,0) and (0,1+(1+h)/μ). By direct calculation, the Jacobian matrices of (5.1) at (0,0) and (0,1+(1+h)/μ) are, respectively,
(−(h+1) 00h+1+μ) and (−(h+1) 0v2−(1+1b)v −(h+1+μ)). |
Hence, (0,0) is a saddle point and (0,1+(1+h)/μ) a stable node (see Figure 1(a)). After a blow-down, the orgin (0,0) of (5.1) is an attractor (see Figure 1(b)). In view of Lemma (5.1), the orgin (0,0) is an attractor in the system (1.4).
Theorem 5.2. Assume 0<b<1/4, 0<a<a∗, 0<h<h2, and μ>0. If x0<min{y0,x5}, then limt→∞(x(t),y(t))=(0,0), where a∗, h2, and x5 are defined as in Theorem 3.1.
Proof. Let A={(x,y)∣0<x<y,x<x5} and (x(t),y(t)) be the unique solution of (1.4) with (x0,y0)∈A. Set w(t)=y(t)−x(t), and
W(w,t)=μ(w+x)(1−w+xx)−x(1−x)(xb−1)+x[1+a(w+x)](w+x)+hx. |
Then, w satisfies
dwdt=W(w,t), t>0; w(0)=y0−x0. |
Due to x<x5, we have
W(0,t)=xb[(1+ab)x2−x+b(1+h)]>0. |
It follows from the first equation of (1.4) and x<y that
dxdt<x[(1−x)(xb−1)−(1+ax)x−h]=−xb[(1+ab)x2−x+b(1+h)]. |
Therefore,
d(x−x5)dt∣x=x5=dxdt∣x=x5<−x5b[(1+ab)x25−x5+b(1+h)]=0. |
In summary, it can be seen that A is an invariant region.
If (x0,y0)∈A, then
dxdt=x[(1−x)(xb−1)−(1+ay)xy−h]≤x[(1−x)(xb−1)−(1+ax)x−h]≤Kx, |
where K=(1−x0)(x0/b−1)−(1+ax0)x0−h<0. Thus, limt→∞x(t)=0. Similarly to the proof of Proposition 2.2, limt→∞y(t)=0.
Similarly to the proof of Theorem 5.2, we can also get the following result.
Theorem 5.3. Assume 0<b<1/4, 0<a<a∗, h=h2, and μ>0. If x0<min{y0,x4}, then limt→∞(x(t),y(t))=(0,0), where a∗, h2, and x4 are defined as in Theorem 3.1.
Section 4 shows that both E1 and E4 are higher-order singularities and E6 may be non-hyperbolic, which indicates that system (1.4) may undergo saddle-node bifurcations at E1 and E4 and Hopf bifurcation at E6. In this section, we will discuss saddle-node bifurcation and Hopf bifurcation that occur in system (1.4).
In view of Theorem 3.1, the number of singularities of (1.4) will vary as parameter h changes around at h=h1 and h=h2. In this subsection, based on Sotomayor's theorem in [28], we will prove that system (1.4) exhibits saddle-node bifurcation at E1 and E4.
Theorem 6.1. Assume a,h,μ>0, 0<b<1, and h1, h2 are defined as in Theorem 3.1.
(1)] As the parameter h passes through the threshold value h1, the system (1.4) will experience a saddle-node bifurcation at E1.
(2)]If 0<b<14, 0<a<a∗, and μ≠A(x4), then the system (1.4) experiences a saddle-node bifurcation at E4 as the parameter h passes through the threshold value h2.
Proof. (1) Reviewing (4.2) with i=1, we get
JE1=(−2bx21+(1+1b)x1 −x10μ)=(0 −1+b20μ). |
Clearly, the two eigenvalues of JE1 are 0 and μ. Let ξ and η be two eigenvectors respectively corresponding to eigenvalue 0 for the matrices JE1 and JTE1. After simple calculations, we get
ξ=(ξ1ξ2)=(10) |
and
η=(η1η2)=(11+b2μ). |
Let
G(x;h)=(f(x,y)g(x,y))=(x(1−x)(xb−1)−(1+ay)xy−hxμy(1−yx)). |
It is easy to obtain that
ηTGh(E1;h1)=(1 1+b2μ)(−x10)=−1+b2≠0. |
Furthermore,
D2G(E1,h1)(ξ,ξ)=(∂2f∂x2ξ21+2∂2f∂x∂yξ1ξ2+∂2f∂y2ξ22∂2g∂x2ξ21+2∂2g∂x∂yξ1ξ2+∂2g∂y2ξ22)(E1,k1)=(−1b−10). |
Clearly,
ηTD2G(E1,h1)(ξ,ξ)=(1 1+b2μ)(−1b−10)=−1b−1≠0. |
Therefore, it follows from Sotomayor's theorem that the system (1.4) undergoes a saddle-node bifurcation at E1 as the parameter h passes through the threshold value h1.
(2) Similar to the proof of (1), it follows from (4.4) and (4.5) that
JE4=(−2bx24+(1+1b)x4 −x4−2αx24μ−μ)=(ab+a+12(ab+1)2 −ab+a+12(ab+1)2μ−μ), |
det(JE4)=0, and tr(JE4)=A(x4)−μ. When μ≠A(x4), JE4 has a simple eigenvalue 0. Let V and W be two eigenvectors respectively corresponding to eigenvalue 0 for the matrices JE4 and JTE4. By simple calculation, we get
V=(v1v2)=(11) |
and
W=(w1w2)=(1−ab+a+12μ(ab+1)2). |
Moreover,
D2G(E4,h2)(V,V)=(∂2f∂x2v21+2∂2f∂x∂yv1v2+∂2f∂y2v22∂2g∂x2v21+2∂2g∂x∂yv1v2+∂2g∂y2v22)(E4,h2)=(5−abb(ab+1)0), |
Gh(E4,h2)=(−x40)=−12(ab+1). |
Therefore, it is easy to see that V and W satisfy the transversality conditions
WTGh(E4,h2)=(1 −ab+a+12μ(ab+1)2)(−x40)=−12(ab+1)≠0, |
WT[D2G(E4,h2)(V,V)]=(1 −ab+a+12μ(ab+1)2)(5−abb(ab+1)0)=−1−bb(ab+1))≠0. |
Hence, in view of Sotomayor's theorem, the system (1.4) experiences a saddle-node bifurcation at E4 as the parameter h passes through the threshold value h2.
As stated in Theorem 4.2, the stability of E6(x6,x6) will change as the parameter μ changes. Specially, if μ=A(x6), then E6(x6,x6) is a center or fine focus under some conditions. In this subsection, we will analyze the Hopf bifurcation occurring at E6 in system (1.4) by choosing μ as the bifurcation parameter.
Based on Theorem 4.2 and Lemma 4.1, when 0<b<1/4, 0<a<a∗, and 0<h<h2, if any one of the three conditions (a1), (a4), and (a5) in Subsection 4.1 holds, then A(x6)>0. Recalling
det(JE6)=μbx6[2(1+ab)x6−1] and tr(JE6)=A(x6)−μ |
in (4.5), we let λ=α(μ)±iω(μ) be the roots of λ2−λtr(JE6)+det(JE6)=0, where α(μ)=tr(JE6)/2 and ω(μ)=√det(JE6)−α2(μ). Moreover, the Jacobian matrix JE6 has a pair of imaginary eigenvalues λ when μ=A(x6) and α′(μ)|μ=A(x6)=−1/2≠0. By the poincarˊe-Andronov-Hopf Bifurcation theorem in [29], the system (1.4) undergoes a Hopf Bifurcation at E6 when μ=A(x6).
Next, we will employ the methods in the literature [30,31] to discuss the more detailed nature of hopf bifurcation generated in system (1.4), and we will directly adopt the notation of symbols utilized in [30,31].
First, we translate E6(x6,x6) to the origin by using the transformation ˜x=x−x6 and ˜y=y−x6. For convenience, we still denote ˜x and ˜y with x and y, respectively. Then, the system (1.4) becomes
{dxdt=˜f(x,y,μ),dydt=˜g(x,y,μ), | (6.1) |
which is equivalent to the system
(dxdtdydt)=JE6(xy)+(˜f(x,y,μ)+2bx26x−(1+1b)x6x+(x6+2ax26)y˜g(x,y,μ)−μx+μy,), | (6.2) |
where
{˜f(x,y,μ)=(x+x6)(1−x−x6)(x+x6b−1)−[1+a(y+x6)](x+x6)(y+x6)−h(x+x6),˜g(x,y,μ)=μ(y+x6)(1−y+x6x+x6). |
Set matrix
B=(1 0N M), |
where
M=ω(μ)x6(1+2ax6) |
and
N=−2bx26+(1+1b)x6−α(μ)x6(1+2ax6). |
Apparently,
B−1=(1 0−NM 1M). |
By the transformation
(xy)=B(uv), |
then system (6.2) becomes
(dudtdvdt)=(α(μ) −ω(μ)ω(μ) α(μ))(uv)+(F1(u,v,μ)F2(u,v,μ)), |
where
(F1(u,v,μ)F2(u,v,μ))=B−1(˜f(u,Nu+Mv,μ)˜g(u,Nu+Mv,μ))−(α(μ) −ω(μ)ω(μ) α(μ))(uv). |
In order to determine the stability of the periodic solution, we need to calculate the sign of the coefficient a(A(x6)), which is given by
a(A(x6))=116[F1uuu+F1uvv+F2uuv+F2vvv]+116ω(μ0)[F1uv(F1uu+F1vv)−F2uv(F2uu+F2vv)−F1uuF2uu+F1vvF2vv] |
where
F1uuu(0,0,A(x6))=−2N2(2a+1)−6b, F1vvv(0,0,A(x6))=2aN2x6,F2uuv(0,0,A(x6))=4aN2−2A(x6)Nx6(2−1x6), F2vvv(0,0,A(x6))=0,F1uv(0,0,A(x6))=M(aNx6+ax6+1), F1vv(0,0,A(x6))=−2aM2x6,F1uu(0,0,A(x6))=−(a+1)N2x6−4Nx6−2N−2b(3x6−b−1)F2uu(0,0,A(x6))=NM((a+1)N2x6+4Nx6+2N+1b(6x6−2b−2))−2A(x6)N−1x6,F2uv(0,0,A(x6))=−(aN2x6+aNx6+N)−2A(x6)(N−1)x6,F2vv(0,0,A(x6))=2aMNx6−2A(x6)Mx6. |
Summarizing the above analysis, we obtain the main result of this section:
Theorem 6.2. Let 0<b<1/4, 0<a<a∗, 0<h<h2, and μ>0. Suppose that one of the three conditions (a1), (a4), and (a5) in Subsection 4.1 is true. Then, the system (1.4) will experience a Hopf bifurcation at E6 as μ passes through A(x6). Moreover,
(1)if a(A(x6))<0, then the system (1.4) undergoes a supercritical Hopf bifurcation and a stable limit cycle appears around E6;
(2)if a(A(x6))>0, then the system (1.4) undergoes a subcritical Hopf bifurcation and an unstable limit cycle appears around E6.
In this section, we will utilize the Matlab software (version 2021b) for conducting numerical simulations to support our theoretical analysis results. In the system (1.4), we take b=0.2, a=0.3, h=0.1, and μ=1 to vary Proposition 2.2. From Figure 2(a), we find that both species will go extinct when the initial value of prey is less than b. In fact, this phenomenon is known as the Allee effect. With b=0.1, a=0.9, h=0.1, and μ=1, we can obtain x∗0=5/9 in Theorem 2.1 by direct calculation. In Figure 2(b), we can see that the prey and predator will coexist when the density of the prey is greater than x∗0.
The boundary singularities E2(x2,0) is always an unstable node and E3(x3,0) is always a saddle in Figure 3(a). Especially, when μ=A(x2), E2(x2,0) is a degenerate node in Figure 3(b). In Theorem 4.2, the singularity E5 is always a saddle (see Figure 4(a)). The situation of the singularity E6(x6,x6) is complicated. Let b=0.2, a=1, h=0.03, then the parameters satisfy condition (a4) in Theorem 4.2 and A(x6)=0.6416. When μ=0.2<A(x6), E6(x6,x6) is an unstable focus (see Figure 4(b)); when μ=0.6<A(x6), E6(x6,x6) is an unstable node (see Figure 5(a)); when μ=0.7>A(x6), E6(x6,x6) is a focus (see Figure 5(b)); when μ=10>A(x6), E6(x6,x6) is a stable node (see Figure 6(a)); when μ=A(x6), E6(x6,x6) is a focus (see Figure 6(b)).
When h=h1, the system (1.4) has a unique higher-order singularity E1(x1,0). It always a repelling saddle-node (see Figure 7(a)). When h=h2, the system (1.4) has a unique higher-order singularity E4(x4,x4). If μ=0.25<A(x4), E4 is a repelling saddle-node (see Figure 7(b)); if μ=0.5>A(x4), E4 is an attracting saddle-node (see Figure 8(a)); if μ=A(x4)=17/64, E4 is a cusp of co-dimension 2 (see Figure 8(b)).
The origin (0,0) is an attractor in the system (1.4). When two distinct positive singularities coexist simultaneously, if the initial population of the prey is lower than that of the predator and x5, both species will ultimately perish (see Figure 9(a)). Similarly, when there is only one positive singularity, if the initial value of the prey is less than that of the predator and x4, then both species will perish (Figure 9(b)). The simulation results above are consistent with the conclusions of Theorems 5.1–5.3.
When the parameters b=0.2, a=1, and μ=1 are set, the system (1.4) experiences two saddle point bifurcations at h=h1 and h=h2, respectively; see Figure 10(a). With parameters b=0.1, a=5, h=0.5, and μ=0.97, the system (1.4) undergos a Hopf bifurcation, where a stale limit cycle emerges surrounding E6(x6,x6).
In this paper, we consider several ecological factors, including the Allee effect, the impact of harvesting on prey populations, and cooperative hunting behaviors among predators, within the framework of a Leslie-Gower prey-predator model. The consideration of various ecological factors makes the model (1.4) closer to the real world, while also making the theoretical results more instructive. These biological phenomena are well presented in the new system and bring rich dynamic properties. We have conducted a fairly comprehensive qualitative analysis and bifurcation study for the system (1.4). It is worth noting that we have studied the types of higher-order singularities and found that there may be two saddle-nodes and a cusp of co-dimension 2. The system (1.4) is not defined at (0,0). By using topological equivalent and the blow-up method, we prove that the origin is attractor, and a defined basin of attraction was given. We investigated the stability of limit cycles where Hopf bifurcation occurs by calculating the first Lyapunov coefficient.
There are four parameters in system (1.4), and as these parameters vary, the system demonstrates distinct dynamic properties. Next, we will discuss the significance of the theoretical results obtained in this article from the perspective of these four parameters and their ecological meanings.
The Allee effect parameter b is presented in the new model. When the prey density is too low, the prey will perish and the predator also becomes extinct (see Figure 2(a)). This phenomenon contradicts the traditional perspective of population dynamics. From a biological perspective, congregating is conducive to the reproduction and survival of the population. For example, a box of bees can generate and maintain considerable heat, which enables all members to survive in low-temperature conditions. Conversely, if they are isolated, they risk freezing to death. Therefore, each species has its own optimal density, and too dense or too sparse will have a restraining effect on the population. This is also evident from the mathematical model (1.2) of the Allee effect, where the population density will only increase when the prey density is between b and 1. In fact, for some low-density populations, such as tigers, b cannot be too large. If b is significantly high, it becomes improbable for the population density to surpass this threshold, which could ultimately result in the extinction of the population. Theorem 3.1 demonstrates that if the value of b exceeds 1/4, the population is destined to decline.
The harvesting parameter h affects the number of singularities (Theorem 3.1). When h>h1, the system has no any singularity; when h>h2, it has no positive singularity. It reveals that excessive human production activities can lead to ecological imbalances and species extinction. In addition, when h=h1 or h2, the system has undergone saddle-node bifurcation (Theorem 6.1). This means that the ecosystem is likely to experience collapse when the capture rate of the prey population reaches h1 and h2.
Cooperative hunting a also has a significant impact on singularities of the system (Theorem 3.1). When a>a∗, it has no positive singularity. This explains why certain invasive species can proliferate rapidly. Since native species are unfamiliar with invasive species, it enhances the efficiency of cooperative hunting by invasive species, leading to the extinction of local species.
The intrinsic growth rate μ of predator determines the stability of the singularity E6 (Theorem 4.2). The positive singularity E6 is stable(unstable) when μ>A(x6)(μ<A(x6)), and the system (1.4) will undergo a Hopf bifurcation when μ=A(x6) (see Figure 10(b)). Based on the above theoretical results, we can conclude that the intrinsic growth rate of predators should not be too low. If the population of predators decreases, it may have an indirect impact on the prey population, thereby disrupting the balance of the entire ecosystem.
In order to present the key values of these parameters and their possible ecological significance in the real world, we have organized them in Table 1.
Parameters | Critical values | Biological implications |
b | 14 | In a real natural environment, for some low-density populations, such as tigers, lions, and whales, b cannot be too large. If b is high, it becomes improbable for the population density to surpass this threshold, which could ultimately result in the extinction of the population. |
h | h1, h2 | The excessive human production activities can lead to ecological imbalances and species extinction. In addition, when the capture rate of the prey population reaches h1 and h2, the ecosystem is likely to collapse. |
a | a∗ | Excessive cooperation among predators can have negative impacts on prey populations and predator populations, ultimately leading to the extinction of both populations. |
μ | A(x6) | The intrinsic growth rate of predators should not be too low. If the population of predators decreases, it may have an indirect impact on the prey population, thereby disrupting the balance of the entire ecosystem. |
Na Min: Conceptualization, Methodology, Software, Visualization, Formal analysis, Validation, Investigation, Writing-original draft; Hongyang Zhang: Validation, Investigation, Writing-original draft; Xiaobin Gao: Supervision, Funding acquisition; Pengyu Zeng: Software, Project administration. All the authors have read and approved the final version of the manuscript for publication.
The authors would like to thank the anonymous referees for their valuable comments and sugges- tions, which led to considerable improvement of the article. This work is supported by GABRP 2023A04J1317, NSFC 11901116, NSFC 62403152, and NSFC 62403133.
The authors declare that they have no competing interests.
[1] | L. A. Zadeh, Fuzzy sets, Inf. Control, 8 (1965), 338–353. https://doi.org/10.1016/S0019-9958(65)90241-X |
[2] |
L. A. Zadeh, Similarity relations and fuzzy orderings, Inform. Sciences, 3 (1971), 177–200. https://doi.org/10.1016/S0020-0255(71)80005-1 doi: 10.1016/S0020-0255(71)80005-1
![]() |
[3] | K. M. Lee, Bipolar-valued fuzzy sets and their operations, In: Proc. Int. Conf. Intell. Technol., Bangkok, Thailandgaiwan, 2000,307–312. |
[4] | W. R. Zhang, Bipolar fuzzy sets and relations: A computational framework for cognitive modeling and multiagent decision analysis, In NAFIPS/IFIS/NASA'94. Proceedings of the First International Joint Conference of The North American Fuzzy Information Processing Society Biannual Conference, The Industrial Fuzzy Control and Intellige, 1994,305–309. |
[5] | K. M. Lee, K. M. Lee, K. J. Cios, Comparison of interval-valued fuzzy sets, intuitionistic fuzzy sets, and bipolar-valued fuzzy sets, Comput. Inform. Technol. 2001,433–439. https://doi.org/10.1142/9789812810885-0055 |
[6] |
B. Q. Hu, K. F. C. Yiu, A bipolar-valued fuzzy set is an intersected interval-valued fuzzy set, Inform. Sciences, 657 (2024), 119980. https://doi.org/10.1016/j.ins.2023.119980 doi: 10.1016/j.ins.2023.119980
![]() |
[7] | J. G. Lee, K. Hur, Bipolar fuzzy relations, Mathematics, 7 (2019), 1044. https://doi.org/10.3390/math7111044 |
[8] |
G. Wei, C. Wei, H Gao, Multiple attribute decision making with interval-valued bipolar fuzzy information and their application to emerging technology commercialization evaluation, IEEE Access, 6 (2018), 60930–60955. https://doi.org/10.1109/ACCESS.2018.2875261 doi: 10.1109/ACCESS.2018.2875261
![]() |
[9] | Y. B. Jun, C. S. Kim, K. O. Yang, Cubic sets, Ann. Fuzzy Math. Inform., 4 (2011), 83–98. |
[10] |
M. Riaz, S. T. Tehrim, Cubic bipolar fuzzy ordered weighted geometric aggregation operators and their application using internal and external cubic bipolar fuzzy data, Comput. Appl. Math., 38 (2019), 87. https://doi.org/10.1007/s40314-019-0843-3 doi: 10.1007/s40314-019-0843-3
![]() |
[11] | F. Smarandache, Neutrosophic set—a generalisation of the intuitionistic fuzzy sets, In: 2006 IEEE International Conference on Granular Computing, 2006, 38–42. |
[12] | H. Wang, F. Smarandache, Y. Zhang, R. Sunderraman, Single valued neutrosophic sets, Infinite Study, 2010. |
[13] | I. Deli, M. Ali, F. marandache, Bipolar neutrosophic sets and their application based on multi-criteria decision making problems, In: 2015 International conference on advanced mechatronic systems (ICAMechS), 2015,249–254. https://doi.org/10.1109/ICAMechS.2015.7287068 |
[14] | I. Deli, S. Yusuf, F. Smarandache, M. Ali, Interval valued bipolar neutrosophic sets and their application in pattern recognition, In: 2016 IEEE International Conference on Fuzzy Systems (FUZZ-IEEE), 2016. https://doi.org/10.1109/FUZZ-IEEE.2016.7738002 |
[15] |
D. Molodtsov, Soft set theory-first results, Comput. Math. Appl., 37 (1999), 19–31. https://doi.org/10.1016/S0898-1221(99)00056-5 doi: 10.1016/S0898-1221(99)00056-5
![]() |
[16] | P. K. Maji, Neutrosophic soft set, Ann. Fuzzy Math. Inform., 5 (2013), 157–168. |
[17] |
M. Ali, L. H. Son, I. Deli, N. D. Tien, Bipolar neutrosophic soft sets and applications in decision making, J. Intell. Fuzzy Syst., 33 (2017), 4077–4087. https://doi.org/10.3233/JIFS-17999 doi: 10.3233/JIFS-17999
![]() |
[18] | P. Arulpandy, M. T. Pricilla, Bipolar neutrosophic graded soft sets and their topological spaces, Infinite Study, 48 (2022). |
[19] |
M. Riaz, S. T. Tehrim, Cubic bipolar fuzzy set with application to multi-criteria group decision making using geometric aggregation operators, Soft Comput., 24 (2020), 16111–16133. https://doi.org/10.1007/s00500-020-04927-3 doi: 10.1007/s00500-020-04927-3
![]() |
[20] |
Z. Liu, K. Qin, Z. Pei, Similarity measure and entropy of fuzzy soft sets, The Scientific World J., 1 (2014), 161607. https://doi.org/10.1155/2014/161607 doi: 10.1155/2014/161607
![]() |
Parameters | Critical values | Biological implications |
b | 14 | In a real natural environment, for some low-density populations, such as tigers, lions, and whales, b cannot be too large. If b is high, it becomes improbable for the population density to surpass this threshold, which could ultimately result in the extinction of the population. |
h | h1, h2 | The excessive human production activities can lead to ecological imbalances and species extinction. In addition, when the capture rate of the prey population reaches h1 and h2, the ecosystem is likely to collapse. |
a | a∗ | Excessive cooperation among predators can have negative impacts on prey populations and predator populations, ultimately leading to the extinction of both populations. |
μ | A(x6) | The intrinsic growth rate of predators should not be too low. If the population of predators decreases, it may have an indirect impact on the prey population, thereby disrupting the balance of the entire ecosystem. |
Parameters | Critical values | Biological implications |
b | 14 | In a real natural environment, for some low-density populations, such as tigers, lions, and whales, b cannot be too large. If b is high, it becomes improbable for the population density to surpass this threshold, which could ultimately result in the extinction of the population. |
h | h1, h2 | The excessive human production activities can lead to ecological imbalances and species extinction. In addition, when the capture rate of the prey population reaches h1 and h2, the ecosystem is likely to collapse. |
a | a∗ | Excessive cooperation among predators can have negative impacts on prey populations and predator populations, ultimately leading to the extinction of both populations. |
μ | A(x6) | The intrinsic growth rate of predators should not be too low. If the population of predators decreases, it may have an indirect impact on the prey population, thereby disrupting the balance of the entire ecosystem. |