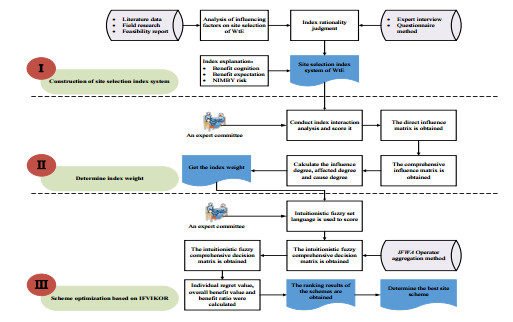
In this paper, we revisited the previously proposed key exchange protocol based on the matrix power function. We prove that the entries of the public key matrices of both parties of the protocol are uniform. Using this result we defined a security game for our protocol and show that the malicious attacker cannot gain any significant advantage in winning this game by applying faithful representation or the linearization approaches. Moreover, we showed that the shared key is computationally indistinguishable from the imitation key if the security parameters are properly chosen.
Citation: Aleksejus Mihalkovich, Jokubas Zitkevicius, Eligijus Sakalauskas. The security analysis of the key exchange protocol based on the matrix power function defined over a family of non-commuting groups[J]. AIMS Mathematics, 2024, 9(10): 26961-26982. doi: 10.3934/math.20241312
[1] | Dilshad Alghazzawi, Maryam Liaqat, Hanan Alolaiyan, Hamiden Abd El-Wahed Khalifa, Alhanouf Alburaikan, Qin Xin, Umer Shuaib . Innovative approaches to solar cell selection under complex intuitionistic fuzzy dynamic settings. AIMS Mathematics, 2024, 9(4): 8406-8438. doi: 10.3934/math.2024409 |
[2] | Sumaira Yasmin, Muhammad Qiyas, Lazim Abdullah, Muhammad Naeem . Linguistics complex intuitionistic fuzzy aggregation operators and their applications to plastic waste management approach selection. AIMS Mathematics, 2024, 9(11): 30122-30152. doi: 10.3934/math.20241455 |
[3] | Doaa Al-Sharoa . (α1, 2, β1, 2)-complex intuitionistic fuzzy subgroups and its algebraic structure. AIMS Mathematics, 2023, 8(4): 8082-8116. doi: 10.3934/math.2023409 |
[4] | Changlin Xu, Yaqing Wen . New measure of circular intuitionistic fuzzy sets and its application in decision making. AIMS Mathematics, 2023, 8(10): 24053-24074. doi: 10.3934/math.20231226 |
[5] | Rana Muhammad Zulqarnain, Xiao Long Xin, Muhammad Saeed . Extension of TOPSIS method under intuitionistic fuzzy hypersoft environment based on correlation coefficient and aggregation operators to solve decision making problem. AIMS Mathematics, 2021, 6(3): 2732-2755. doi: 10.3934/math.2021167 |
[6] | Muhammad Qiyas, Neelam Khan, Muhammad Naeem, Saleem Abdullah . Intuitionistic fuzzy credibility Dombi aggregation operators and their application of railway train selection in Pakistan. AIMS Mathematics, 2023, 8(3): 6520-6542. doi: 10.3934/math.2023329 |
[7] | Muhammad Jawad, Niat Nigar, Sarka Hoskova-Mayerova, Bijan Davvaz, Muhammad Haris Mateen . Fundamental theorems of group isomorphism under the framework of complex intuitionistic fuzzy set. AIMS Mathematics, 2025, 10(1): 1900-1920. doi: 10.3934/math.2025088 |
[8] | Admi Nazra, Jenizon, Yudiantri Asdi, Zulvera . Generalized hesitant intuitionistic fuzzy N-soft sets-first result. AIMS Mathematics, 2022, 7(7): 12650-12670. doi: 10.3934/math.2022700 |
[9] | Tareq Saeed . Intuitionistic fuzzy variational inequalities and their applications. AIMS Mathematics, 2024, 9(12): 34289-34310. doi: 10.3934/math.20241634 |
[10] | Shichao Li, Zeeshan Ali, Peide Liu . Prioritized Hamy mean operators based on Dombi t-norm and t-conorm for the complex interval-valued Atanassov-Intuitionistic fuzzy sets and their applications in strategic decision-making problems. AIMS Mathematics, 2025, 10(3): 6589-6635. doi: 10.3934/math.2025302 |
In this paper, we revisited the previously proposed key exchange protocol based on the matrix power function. We prove that the entries of the public key matrices of both parties of the protocol are uniform. Using this result we defined a security game for our protocol and show that the malicious attacker cannot gain any significant advantage in winning this game by applying faithful representation or the linearization approaches. Moreover, we showed that the shared key is computationally indistinguishable from the imitation key if the security parameters are properly chosen.
Waste-to-energy (WtE) is a method of generating electricity by exchanging the high-temperature flue gas from waste incineration for hot steam, which in turn drives a generating unit [1]. This method has a short treatment cycle, a small footprint, is not easily affected by weather factors, and waste incineration treatment can use the heat to generate electricity, generating certain economic benefits [2,3]. During construction and operation, WtE projects may produce emissions, fumes, wastewater, dust particles, and noise, causing secondary pollution that affects the local environment and the health of the surrounding population [4,5]. Due to the public's concern for sustainable development and environmental protection, public resistance and protests against the WtE project may occur during its implementation, triggering "Not In My Back Yard" (NIMBY) risks [6]. Some WtE projects in China have been canceled due to public opposition, such as the Luoding WtE project in Guangdong and the Xishui WtE project in Hubei. The issue of NIMBY risk in the site selection decision process has been a significant factor in the successful implementation of WtE projects. The NIMBY risk of WtE projects refers to the negative externalities generated during the construction and operation of the project, where all members of society share the benefits of the project and the external costs of the project are borne by the public in the area where the project is located. The public is concerned about the negative impact of the construction and operation of the project on physical and mental health, property values and the quality of the surrounding environment, thus stimulating negative emotions and generating opposition and resistance [7,8].
At present, the research on NIMBY risk is mainly conducted from two aspects of NIMBY risk formation mechanism and avoidance strategy. In the study of NIMBY risk formation mechanism, the mechanism of NIMBY risk formation is mainly analyzed from the aspects of decision-making behavior, public participation, information disclosure, and compensation scheme[9,10,11]. In terms of NIMBY risk avoidance, the existing research results mostly analyze the causes of the NIMBY risk, rely on case studies and questionnaire surveys, and formulate NIMBY risk response strategies by combining the stakeholders involved in the project and the NIMBY risk influencing factors [12,13,14]. For example, Jenkins-smith and Kunreuther [15] believe that the higher the environmental risk in the construction process of engineering projects, the greater the rejection of residents and the greater the NIMBY risk. Wu et al. [16] evaluated the acceptability of NIMBY risk among college students in China and Japan through a questionnaire survey and found that Chinese students paid more attention to the impact of real estate value, while Japanese students paid more attention to environmental pollution and health hazards. Devine-wright [17] believes that NIMBY risk management needs to consider the project location's population characteristics and risk perception level. Johnson and Sdcchitano [13] analyzed the factors that may affect the public interest in the landfill site selection process through the survey data of seven counties in Florida. The analysis results showed that the main factors include: Media coverage, the distance of the project to the community/public space, trust in the government, impact on public health, environmental quality, and impact of the project on property values. Schwenkenbecher [18] pointed out that environmental impact has gradually become the core factor of renewable energy project site selection and proposed that attention should be paid to the public interest demands and ensure the fairness and justice of the decision-making process so as to overcome the impact of NIMBY risks on projects. Mclaughlin and Cutts [14] analyzed the influencing factors that lead to public opposition to project location, including environmental hazards, economic factors, public health, climate change impacts, property factors, and trust in government. The Related studies can provide a reference for conducting WtE project site selection studies. However, how to scientifically and rationally determine the site selection of WtE projects still needs to be studied in depth.
However, the existing literature on the site selection decision of the WtE project did not fully consider NIMBY risk, and the site selection decision method needs to be further optimized. At present, relevant studies have been conducted on the site selection decision for WtE projects in terms of site selection methods and indicator systems. Zavadskas et al. [19] carried out a site selection decision study by the weighted aggregated sum product assessment method based on the economic, environmental, social, and engineering factors' impact on WtE projects. Tavares et al. [20] used the Analytic Hierarchy Process (AHP) and the Geographic Information System (GIS) to determine the best site for a WtE project, taking into account economic, social, technical, and environmental factors. In addition, the improved fuzzy Technique for Order Preference by Similarity to Ideal Solution (TOPSIS) and the Analytic Network Process (ANP) is also used to determine the location of WtE projects and to construct a system of indicators for site selection decisions in terms of economic, environmental, and social aspects in the site selection process [21,22]. Pan et al. [23] constructed an evaluation index system from the perspectives of sustainable development, such as economy, environment, and society. They proposed a large-scale group decision-making method to select the optimal site. Gao et al. [24] proposed a WtE project location decision model based on the extended Cloud TOmada de Decisao Interativa Multicriterio (C-TODIM) from the perspective of low carbon. In addition, Wu et al. [25] believe that public satisfaction should be fully considered in site selection decisions, and the public should be invited as the evaluation group. On this basis, a WtE project decision-making model based on the Interval 2-Tuple Linguistic Ordered Weighted Averaging (ITL-OWA) operator, and VIKOR method is proposed. In other renewable energy construction projects, Rezaei M. et al. [26,27] adopted the TOPSIS method to conduct decision analysis on the location of wind power plants and solar power plants, respectively. In addition, the successful application of the fuzzy VIKOR method in the field of renewable energy decision-making also provides ideas for the construction of the WtE project location decision framework [28,29].
The existing studies can provide a reference for this study to carry out the site selection decision of WtE projects. But in the decision-making process, decision-makers are still faced with the following limitations: (1) When constructing the index system, indicators were mainly selected from three aspects: economy, society, and environment, and the NIMBY attribute of WtE project was not fully considered, and related NIMBY risk factors were ignored. (2) AHP and ANP are considered to be common methods for determining weight. However, many of pair-to-pair comparisons cannot guarantee consistency and improve computing efficiency, and may increase computing costs. Moreover, OWA operator and entropy-weight method do not fully consider the correlation between the factors when calculating the weight. (3) In the previous literature, TOPSIS cannot solve the problem of decision makers' risk preference. Although some methods consider the risk preference of decision makers, such as TODIM and VIKOR, they ignore the fuzziness and uncertainty of the decision-making process. In addition, the above research also neglects verifying the decision result's stability and reliability. This may increase the risk of decision making and affect the evaluation results. Therefore, this study builds a new decision-making framework to address these problems, combined with the needs of WtE project site selection. The innovations of this paper are as follows: (1) This research fully considers the NIMBY effect in the selection process of the WtE project, identifies potential NIMBY risks, and incorporates them into the decision index system. (2) In the decision-making framework, Decision Making Trial and Evaluation Laboratory (DEMATEL) is adopted to replace the traditional index weighting method. By analyzing the interaction between factors, a more reasonable weight is obtained, and the computational efficiency is improved. (3) It must be pointed out that NIMBY risk consideration intensifies the fuzziness of the decision-making process, and intuitive fuzzy sets play an important role in multi-attribute decision-making. M. Akram and J. C. R. Alcantud [30,31] have carried out in-depth research on intuitionistic fuzzy sets and verified their superiority in processing fuzzy information. Therefore, this paper introduces intuitionistic fuzzy theory into decision making, and applies the VIKOR method of intuitionistic fuzzy extension to site selection. Meanwhile, sensitivity analysis was carried out to ensure the authenticity and stability of the results.
This study aims to construct a siting decision system for WtE projects and to avoid the NIMBY risk problem in the siting process to determine the project site scientifically and rationally. The specific structure of the manuscript is as follows: the second part focuses constructing of a site selection decision system for WtE projects; the third part is verifying the feasibility of the site selection decision system through case studies.
This study proposes a site selection decision-making framework for WtE projects that considers NIMBY risk, including three stages: the construction of an indicator system for site selection of WtE projects, the determination of indicator weights and the ranking of preferred siting options. The main methods used in the construction of the indicator system are literature analysis, questionnaire survey, and field interviews. In determining the indicator weights, the DEMATEL is used to analyze the interactions between the indicators and calculate the indicator weights. The main methods used in the preferential ranking of site selection options are intuitionistic fuzzy sets, the IFWA agglomerative operator, and the VIKOR method. The framework clarifies the specific tasks and operational steps to be carried out at each stage and improves the efficiency of decision-making (see Figure 1).
The construction of a scientific and reasonable indicator system is an important basis for the smooth implementation of WtE project site selection. In the past, the design of the indicator system was often considered from the perspective of cost and benefit, without fully considering the feelings and wishes of nearby residents. In contrast, as typical NIMBY facilities, waste-to-energy projects have negative externalities and the NIMBY risks arising during implementation need to be fully considered in the siting decision process [15]. Therefore, when making decisions on site selection for WtE projects, the possible NIMBY risks should be fully analyzed. In addition to cost and benefit factors, environmental and social risks likely to cause NIMBY conflicts need to considered. In this regard, this study constructed a comprehensive decision indicator system consisting of cost-benefit-risk from the perspective of NIMBY risk. The specific steps for constructing the indicator system are as follows: first, the indicator set was initially determined through existing literature, feasibility study reports, and field research; then, the reasonableness of the indicators was judged through expert interviews and questionnaires; finally, the results of the expert evaluation were summarized to form the site selection decision indicator system for WtE projects. The expert group mainly consisted of 12 members, including 4 government department personnel, 4 university scholars engaged in research on NIMBY issues, project management, and other related aspects (including 4 experts from consulting units, 4 experts from construction units, and 10 experts from universities), and 4 experts from other industries (2 experts from construction units, 1 expert from consulting units and 1 legal practitioner).
Therefore, based on a comprehensive consideration of economic, social, and environmental factors, this study constructs a site selection decision indicator system for WtE projects from three aspects: cost perception, benefit expectation, and NIMBY risk (see Table 1).
First-level indicators | Second-level indicators | Explanation of indicators |
Cost perception (V1) | Operation and maintenance costs (V11) | This indicator is an important indicator for achieving economic sustainability. It refers to the various types of costs incurred during the construction and operation, and maintenance of the project, which was determined mainly based of waste generation potential, interest rates, and inflation rates [32,33]. |
Local land prices (V12) | This indicator refers to the price of land where the project is being built, and the price of local land is an important factor affecting investment capital. When determining the site of the WtE project, the local land price should be fully considered [34,35]. | |
Fuel acquisition costs (V13) | This indicator refers to the expenditure during the project's operation, which includes fuel purchase costs and transportation costs [36,37]. | |
Distance to ancillary facilities (V14) | The construction and operation of a WtE project require appropriate supporting facilities, and this indicator measures the distance between the alternative site and facilities such as electricity, water, and gas [19,35]. | |
Wind conditions and hydrogeological conditions (V15) | Wind and hydrogeological conditions in the vicinity of the construction site can directly influence the project's economic viability and technical choice. If the WtE project is located downwind of the annual prevailing wind direction, pollutant gases can reach further into the area, leading to a higher possibility of gas leakage accidents. In addition, a survey of local hydrological and geological conditions is also necessary to avoid unnecessary damage caused by flooding and geological hazards [35,38]. | |
Benefit Expectations (V2) | Improving living standards (V21) | This indicator measures the extent to which a WtE project has improved the living standards of the people in the area where it is located, mainly in material and spiritual terms. The WtE project is an effective solution to the problem of "rubbish encircling the city", maintaining an attractive and clean living environment and increasing the employment and income levels of the surrounding population [39,40]. |
Service capacity (V22) | This indicator measures the daily waste throughput of the WtE project, as well as the project's service area [37]. | |
Benefit Expectations (V2) | Promoting urbanisation (V23) | The construction and operation of WtE projects require the involvement of a large number of people, which can provide more employment opportunities for the public in the areas surrounding the projects. In this way, the city will have a more substantial agglomeration and radiation drive, absorbing the remaining rural population and speeding up the urbanization process [37,39]. |
Promoting local economic development (V24) | After the project is completed and running, it can promote local economic development. It will create new employment opportunities for residents, transform urban waste into renewable energy, drive the development of the surrounding industries, realize the adjustment of the industrial structure and increase the region's tax reveune [37,39,41]. | |
Risk of environmental damage (V31) | This indicator measures the risk that WtE projects may hurt the daily lives of residents and the ecological environment. It is an exogenous natural risk, including air pollution, water pollution, and noise pollution. Although waste incineration reduces greenhouse gas emissions, it may bring about emissions of atmospheric particulate matter, such as dioxins, which can lead to air pollution [37,39,42]. | |
NIMBY risk (V3) | Health risks to the local population (V32) | This indicator measures the generation of various toxic gases and environmental pollutants such as dioxins during incineration, which inevitably lead to environmental pollution and human health risks [43,44]. |
Risk of public conflict (V33) | The construction of waste-to-energy plants may lead to public conflict risks. Such NIMBY facilities are closely related to the vital interests of the surrounding public, such as life and health, property gains, and environmental rights. When the rights and interests of the public are compromised as a result of the construction and operation of the facility, they may frequently initiate advocacy actions against decision-makers, project contractors, and facility operators, resisting and interfering with the implementation of the project, resulting in conflicts between the government, enterprises and the public [33,45,46]. | |
Risk of safety incidents (V34) | This indicator is an endogenous natural risk arising from design flaws in the processes used at NIMBY facilities or the hazardous properties of raw materials and product by-products (flammable, explosive, toxic), as well as inappropriate process layout and equipment installation flaws. It is mainly due to fires caused by raw materials and by-products, biochemical hazards caused by the leakage of toxic and hazardous substances, as well as health hazards to operators caused by high temperatures, pressures, noise, and dust [10,44]. | |
Risk of asset losses (V35) | This indicator measures the potential risk of loss of asset value during the construction and operation of the project, such as the depreciation of house prices in the area where the project is located [46,47]. |
DEMATEL (Decision Making Trial and Evaluation Laboratory), also known as the decision laboratory method, is a systems engineering model that is mainly used for factor analysis. The main idea is to use matrix tools and a graphical combination method to analyze the degree of mutual influence of indicators on the relevant indicators in the evaluation index system, simplify the problem, find out the influence of each factor on the other, and get the key indicators through analysis, stripping and further dissection [48]. The steps for assigning weights using the DEMATEL method are as follows.
(1) Initialisation directly affects the matrix P.
Experts were invited to rate the degree of interaction between the evaluation indicators, pijexpressed as the degree of direct influence of the indicators vi on the indicators vj, and the ratings of all experts were arithmetically averaged to form an initial direct influence matrix P.
P=[p11p12⋯p1np21p22⋯p2n⋮⋮...⋮pn1pn2⋯pnn]. | (1) |
(2) Normalisation directly affects the matrix.
Normalize the direct influence matrix to obtain the normalized direct influence matrix Q.
Q=(qij)n×n=P(max0⩽i⩽nn∑j=1pij)−1. | (2) |
(3) Calculation of the combined impact matrix between evaluation indicators T.
T=(tij)n×n=limh→∞(D1+D2+⋯+Dh). | (3) |
where when is h large enough to satisfy Dh=0.
(4) Calculate the degree of influence A, the degree of being influenced B, the degree of centrality M and the degree of cause U.
Determine the degree of influence of each factor Ai=n∑j=1tij, obtained by summing each element of the combined influence matrix T by row; determine the degree of being influenced of each factor Bi=n∑i=1tij, obtained by summing each element of the combined influence matrix T by column; determine the centrality of each element as Mi=Ai+Bi; the degree of cause as Ui=Ai−Bi, if U>0 denotes the cause factor, indicating that the factor has a greater influence on the other factors, and if U<0 denotes the effect factor, indicating that the other factors have a greater influence on the factor.
(5) Determination of indicator weights.
ω′k=√(Mi)2+(Ui)2/√(Mi)2+(Ui)2n∑i=1√(Mi)2+(Ui)2n∑i=1√(Mi)2+(Ui)2. | (4) |
Intuitionistic Fuzzy Set (IFS) was proposed by Atanassov to better fit the decision maker's state of mind when describing decisions [49]. In the siting decision process for the WtE project, the decision makers are uncertain when it comes to choosing and evaluating attributes, and it is not possible to accurately represent decision information in terms of real numbers. By using intuitionistic fuzzy sets to describe information, the decision maker's satisfaction, dissatisfaction, and hesitation with an attribute can be effectively reflected, making the decision more realistic.
Definition 1. [49]: The set A={μA(x),υA(x)|x∈X} is an intuitionistic fuzzy set over the domain X of the argument. μA(x):X→[0, 1] denotes the subordination function of the set A; υA(x):X→[0, 1] denotes the unaffiliated function of the set A; and for any x∈X, there is 0⩽μA(x)+υA(x)⩽1. In addition, πA(x)=1 - μA(x) - υA(x) denotes the hesitant subordination degree, and for anyx∈X, there is 0⩽πA(x)⩽1.
The subordinate and non-subordinate degrees of the intuitive fuzzy numbers express the decision maker's satisfaction and dissatisfaction with the decision objective, while the degree of hesitation expresses the degree of uncertainty between satisfaction and dissatisfaction with the objective. For example, a decision maker scored the "service capacity" indicator in one of the alternative sites for the WtE project as (0.7, 0.2, 0.1), indicating that the decision maker's satisfaction with the indicator is 0.7, dissatisfaction is 0.2, and hesitation is 0.1.
Definition 2. [50]: Let a=(μ,υ), a1=(μa1,υa1) and a2=(μa2,υa2) be three intuitionistic fuzzy numbers, which are calculated as follows.
(1)¯a=(μ,υ).
(2)a1⊕a2=(μa1+μa2−μa1μa2,υa1υa2).
(3)a1⊗a2=(μa1μa2,υa1+υa2−υa1μa2).
(4)λa=(1-(1−μa)λ,υλa).
(5)aλ=(μλa,1-(1−υa)λ).
Definition 3. [51]: Denote the intuitionistic fuzzy number a=(μ,υ), the score value asM(a)=μ-υ, and the exact value Δ(a)=μ-υ, then the ranking rules for the two intuitionistic fuzzy numbers a1 and a2 are as follows.
If M(a1) > M(a2), then a1 > a2;
If M(a1) < M(a2), then a1 < a2;
If M(a1) = M(a2), then:
a) If Δ(a1)=Δ(a2), then a1 = a2;
b) If Δ(a1)<Δ(a2), then a1 < a2;
c) If Δ(a1)>Δ(a2), then a1 > a2.
Definition 4. [51]: Let a set of intuitionistic fuzzy numbers be aj(j=1,2,⋯,n), then the integrated values obtained by the IFWA operator are also the intuitionistic fuzzy numbers.
IFWAλ(a1,a2,⋯an)=λ1a1⊕λ1a2⊕⋯⊕λnan=(1−n∏k=1(1−μlij)λl,n∏k=1(υlij)λl), | (5) |
Where λk is the weight of the kth decision maker and satisfies the condition 0≤λ1≤1, n∑kλk=1,(k=1,2,⋯,n).
Definition 5. [52]: Let a1 and a2 be any two Intuitionistic fuzzy sets, defining their Hemming distance as
d(a1,a2)=12n∑j=1(|μa1(xj)−μa2(xj)|+|υa1(xj)−υa2(xj)|+|πa1(xj)−πa2(xj)|). | (6) |
The evaluation language was evaluated on a 7-point scale and the linguistic variables were extended to intuitionistic fuzzy numbers [49] using the transformation relations in Table 2 to facilitate subsequent data processing. For both the cost perception and benefit expectation indicators, the linguistic evaluation criterion was "whether the program performs well for this indicator", which corresponded to the linguistic word set "Very Good, Good, Moderately Good, Moderate, Moderately Poor, Poor, Very Poor"; for the NIMBY risk indicators, the linguistic evaluation criterion was "whether the program performs well for this indicator". For indicators under NIMBY risk, the linguistic evaluation criterion is "how well the program performs for this risk", which corresponds to the set of words "Very Low, Low, Moderately Low, Moderate, Moderately High, High, Very High".
Language word collection | Number of intuitive blurs | |
Very Good (VG) | Very Low (VL) | (0.72, 0.13, 0.15) |
Good (G) | Low (L) | (0.63, 0.24, 0.13) |
Moderately Good (MG) | Moderately Low (ML) | (0.51, 0.35, 0.14) |
Moderate (M) | Moderate (M) | (0.50, 0.50, 0.00) |
Moderately Poor (MP) | Moderately High (MH) | (0.35, 0.51, 0.14) |
Poor (P) | High (H) | (0.24, 0.63, 0.13) |
Very Poor (VP) | Very High (VH) | (0.13, 0.72, 0.15) |
The VIKOR method is an ideal method based compromise ranking method proposed by Opricovic et al. It achieves a preferential ranking of a limited number of decision options by maximizing group utility and minimizing individual regret. The basic idea is to determine the positive ideal solution (PIS) and the negative ideal solution (NIS), and then compare the evaluation values of the options to be selected and select the best one according to the size of its distance from the ideal indicator value, and obtain a compromise solution in which the attributes of the options yield to each other by maximizing group benefits and minimizing individual losses [53]. The positive ideal solution is the best value of each evaluation criterion, while the negative ideal solution is the worst value of each evaluation criterion. The advantage of this method over other multi-criteria decision-making methods is that it can solve conflicting criteria problems, integrates group and individual compromise relationships, and can determine the compromise solution of a solution from a set of ranked solutions to help decision-makers make a final decision.
The VIKOR method uses an aggregation function developed from the Lpj-metric:
Lp={n∑i=1[wi(f∗i−fij)(f∗i−fi−)]}1/P. | (7) |
The measure Lpj is the distance between the solution to be evaluated and the ideal solution, where, 1≤P≤∞,j−1,2,…,J, n is the number of attributes, f∗i is the ideal value, and wi is the attribute weight.
The VIKOR method compromise solution is illustrated as follows[53]:
In the figure, f∗1 and f∗2 are ideal values under two different criteria. F∗ are optimal solutions. Fc are compromise solutions. fc1 and fc2 are values taken under two different criteria. The compromise solution is the closest to the optimal solution among all feasible solutions and is a compromise solution in which the two attributes yield to each other. The specific steps of the VIKOR method are as follows.
Determine the positive f∗ and negative ideal solutions f - for each indicator based on the integrated intuitionistic fuzzy matrix.
f∗=[(μ∗1,υ∗1),(μ∗2,υ∗2),⋯,(μ∗n,υ∗n)]T=[(1,0),(1,0),⋯,(1,0)]T, | (8) |
f−=[(μ−1,υ−1),(μ−2,υ−2),⋯,(μ−n,υ−n)]T=[(0,1),(0,1),⋯,(0,1)]T. | (9) |
Calculation of group benefit values Si and individual regret values for the options Ri.
Si=n∑j=1wj(a∗j−aija∗j−a−j)=n∑j=1wj(d(a∗j,aij)d(a∗j,a−j)). | (10) |
Ri=maxj(a∗j−aija∗j−a−j)=maxjwj(d(a∗j,aij)d(a∗j,a−j)), | (11) |
where: Si and Ri denote the group benefit value and the individual regret value respectively, Si the smaller the group effect, the larger the group effect. The smaller the individual regret Ri, the smaller the individual regret Ri.wj is the weight of the attribute.
(3) Calculate the benefit ratio of the alternative Qi.
Qi=φSi−S∗iS−i−S∗i+(1−φ)Ri−R∗iR−i−R∗i. | (12) |
Where S∗i=miniSi denotes the minimum value of group benefit, S - i=maxiSi denotes the maximum value of group benefit, R∗i=miniRidenotes the minimum value of individual regret, R - i=maxiRidenotes the maximum value of individual regret, φ and denotes the decision maker's preference.
(4) Determining the ranking of options and compromises.
The options to be decided are ranked in descending order according to Si, Ri and Qi, and the object to be evaluated is ranked first. If the following two conditions are met, then the ranking is based on the magnitude of Qi, the smaller the value Qi, the better the solution to be decided. If condition ② is not satisfied, then Y1 and Y2 is a compromise solution; if the solution ranked 1st and the other solutions do not satisfy condition ①, but only condition ②, then the overall evaluation of the solutions that do not satisfy condition ① is determined to be optimal.
Q(Y2)−Q(Y1)≥DQ,DQ=1m - 1, | (12) |
where Y1 is Qi the best-evaluated object in the ranking and Y2 is Qi the second best-evaluated object in the ranking.
②Y1 is the object preceding the Si or Ri.
As urbanization continues, the urban population is increasing, and the amount of domestic waste generated is growing. Under this pressure, cities are placing increasingly high demands on the scale and quantity of waste disposal facilities. The construction of waste disposal facilities is an important means to alleviate the pressure of waste siege on the city. In order to establish a sound system of sanitation facilities, the scientific configuration of sanitation facilities, to better solve the problem of urban waste accumulation, and to effectively achieve harmless, resourceful, and reduced waste treatment. A city expects domestic waste production to increase by 100 tonnes per day every five years for the next 30 years, reaching 2,638 tonnes per day in 2048. Under this pressure, the city's Urban Management Bureau plans to build a WtE project in the city through the PPP model, which will mainly serve the five administrative districts in the north-central part of the city after completion.
Based on the service radius requirements and site selection principles for the WtE project, the relevant departments and experts conducted extensive field research. They identified three options for comparison among the many proposed sites. All three alternative sites align with the relevant requirements of the overall urban planning, land use planning, and environmental sanitation special planning. For ease of calculation, the three alternatives are denoted as A1, A2, and A3.
To enable a comprehensive and intuitive site selection decision for the WtE project, 12 experts have been invited to construct a decision indicator system from the perspective of considering NIMBY risk, and the 12 experts invited are now formed into three separate expert groups, including a group of practicing business managers, a group of government experts and a group of research scholars, to facilitate subsequent option evaluation and decision making.
In order to make a better decision on the site selection decision of the WtE project, the first step was to analyze and assign weights to the decision-making indicator system established in the previous section. No influence, 1-slight influence, 2-moderate influence, 3-large influence, 4-great impact". Firstly, the experts in the three groups discussed the mutual influence of the indicators. Then, the evaluation data that could form a unified opinion was directly used as the final data; for the evaluation data that could not form a unified opinion, it was discussed again, and if it could not reach a unified opinion, the median data was used as the final data. Finally, the final evaluation data given by the three expert groups were collated, according to Eq (1) to obtain the direct influence matrix between indicators (see Table 3).
Indicators | V11 | V12 | V13 | V14 | V15 | V21 | V22 | V23 | V24 | V31 | V32 | V33 | V34 | V35 |
V11 | 0 | 0 | 0 | 0 | 0 | 0 | 0 | 0 | 0 | 0 | 0 | 0 | 0 | 0 |
V12 | 3 | 0 | 1 | 2 | 1 | 0 | 0 | 0 | 0 | 0 | 0 | 0 | 0 | 0 |
V13 | 3 | 0 | 0 | 0 | 0 | 0 | 0 | 0 | 0 | 0 | 0 | 0 | 0 | 0 |
V14 | 2 | 0 | 0 | 0 | 0 | 0 | 0 | 0 | 0 | 0 | 0 | 0 | 0 | 0 |
V15 | 3 | 1 | 2 | 0 | 0 | 0 | 0 | 0 | 0 | 0 | 0 | 0 | 0 | 0 |
V21 | 0 | 0 | 0 | 0 | 0 | 0 | 2 | 4 | 4 | 0 | 0 | 0 | 0 | 0 |
V22 | 0 | 0 | 0 | 0 | 0 | 4 | 0 | 3 | 4 | 0 | 0 | 3 | 0 | 0 |
V23 | 0 | 0 | 0 | 0 | 0 | 3 | 2 | 0 | 4 | 0 | 0 | 2 | 0 | 0 |
V24 | 0 | 0 | 0 | 0 | 0 | 4 | 3 | 4 | 0 | 0 | 0 | 2 | 0 | 0 |
V31 | 0 | 0 | 0 | 0 | 0 | 3 | 3 | 3 | 3 | 0 | 4 | 4 | 3 | 3 |
V32 | 0 | 0 | 0 | 0 | 0 | 4 | 2 | 3 | 3 | 0 | 0 | 4 | 1 | 3 |
V33 | 0 | 0 | 0 | 0 | 0 | 0 | 0 | 0 | 0 | 0 | 0 | 0 | 0 | 0 |
V34 | 0 | 0 | 0 | 0 | 0 | 0 | 2 | 0 | 0 | 2 | 4 | 4 | 0 | 3 |
V35 | 0 | 0 | 0 | 0 | 0 | 0 | 0 | 0 | 0 | 0 | 0 | 0 | 0 | 0 |
The combined impact matrix can be calculated from Eq (2) to Eq (3) in the DEMATEL method (see Table 4). The influence degree A, the degree of being influenced B, the centrality degree M and the reason degree U of each indicator were further obtained according to the integrated influence matrix (see Table 5), where the influence degree stated the degree of influence of the indicator on other indicators, the degree of being influenced indicated the degree of influence of the indicator on other indicators, the centrality degree indicated the degree of influence of the indicator on WtE project site select decision, and the reason degree stated a positive value indicates that the indicator of this category has a strong influence on a positive value stated that the indicator has a strong influence on other indicators and is called a cause indicator; a negative value indicates that the indicator is influenced by other indicators and is called a result indicator. Based on the data obtained from Table 5, the weight of each indicator could be obtained from the calculation of formula (4) (see Table 6).
Indicators | V11 | V12 | V13 | V14 | V15 | V21 | V22 | V23 | V24 | V31 | V32 | V33 | V34 | V35 |
V11 | 0.000 | 0.000 | 0.000 | 0.000 | 0.000 | 0.000 | 0.000 | 0.000 | 0.000 | 0.000 | 0.000 | 0.000 | 0.000 | 0.000 |
V12 | 0.131 | 0.001 | 0.041 | 0.077 | 0.039 | 0.000 | 0.000 | 0.000 | 0.000 | 0.000 | 0.000 | 0.000 | 0.000 | 0.000 |
V13 | 0.115 | 0.000 | 0.000 | 0.000 | 0.000 | 0.000 | 0.000 | 0.000 | 0.000 | 0.000 | 0.000 | 0.000 | 0.000 | 0.000 |
V14 | 0.077 | 0.000 | 0.000 | 0.000 | 0.000 | 0.000 | 0.000 | 0.000 | 0.000 | 0.000 | 0.000 | 0.000 | 0.000 | 0.000 |
V15 | 0.129 | 0.039 | 0.079 | 0.003 | 0.001 | 0.000 | 0.000 | 0.000 | 0.000 | 0.000 | 0.000 | 0.000 | 0.000 | 0.000 |
V21 | 0.000 | 0.000 | 0.000 | 0.000 | 0.000 | 0.077 | 0.124 | 0.214 | 0.218 | 0.000 | 0.000 | 0.048 | 0.000 | 0.000 |
V22 | 0.000 | 0.000 | 0.000 | 0.000 | 0.000 | 0.219 | 0.058 | 0.191 | 0.226 | 0.000 | 0.000 | 0.154 | 0.000 | 0.000 |
V23 | 0.000 | 0.000 | 0.000 | 0.000 | 0.000 | 0.175 | 0.120 | 0.073 | 0.210 | 0.000 | 0.000 | 0.113 | 0.000 | 0.000 |
V24 | 0.000 | 0.000 | 0.000 | 0.000 | 0.000 | 0.218 | 0.160 | 0.220 | 0.092 | 0.000 | 0.000 | 0.119 | 0.000 | 0.000 |
V31 | 0.000 | 0.000 | 0.000 | 0.000 | 0.000 | 0.239 | 0.203 | 0.234 | 0.241 | 0.009 | 0.174 | 0.261 | 0.123 | 0.151 |
V32 | 0.000 | 0.000 | 0.000 | 0.000 | 0.000 | 0.231 | 0.137 | 0.199 | 0.204 | 0.003 | 0.006 | 0.208 | 0.039 | 0.121 |
V33 | 0.000 | 0.000 | 0.000 | 0.000 | 0.000 | 0.000 | 0.000 | 0.000 | 0.000 | 0.000 | 0.000 | 0.000 | 0.000 | 0.000 |
V34 | 0.000 | 0.000 | 0.000 | 0.000 | 0.000 | 0.071 | 0.118 | 0.063 | 0.067 | 0.078 | 0.168 | 0.218 | 0.015 | 0.146 |
V35 | 0.000 | 0.000 | 0.000 | 0.000 | 0.000 | 0.000 | 0.000 | 0.000 | 0.000 | 0.000 | 0.000 | 0.000 | 0.000 | 0.000 |
Indicators | Impact Degree A | Influenced degree B | Centrality M | Cause Degree U |
V11 | 0.000 | 0.452 | 0.452 | -0.452 |
V12 | 0.289 | 0.040 | 0.329 | 0.249 |
V13 | 0.115 | 0.120 | 0.235 | -0.005 |
V14 | 0.077 | 0.080 | 0.157 | -0.003 |
V15 | 0.251 | 0.040 | 0.291 | 0.211 |
V21 | 0.681 | 1.229 | 1.910 | -0.549 |
V22 | 0.847 | 0.921 | 1.768 | -0.074 |
V23 | 0.691 | 1.193 | 1.885 | -0.502 |
V24 | 0.809 | 1.257 | 2.066 | -0.449 |
V31 | 1.635 | 0.091 | 1.726 | 1.544 |
V32 | 1.149 | 0.349 | 1.497 | 0.800 |
V33 | 0.000 | 1.121 | 1.121 | -1.121 |
V34 | 0.945 | 0.178 | 1.122 | 0.767 |
V35 | 0.000 | 0.417 | 0.417 | -0.417 |
Indicators | Weighting | Indicators | Weighting |
V11 | 0.037 | V23 | 0.114 |
V12 | 0.024 | V24 | 0.123 |
V13 | 0.014 | V31 | 0.135 |
V14 | 0.009 | V32 | 0.099 |
V15 | 0.021 | V33 | 0.092 |
V21 | 0.116 | V34 | 0.079 |
V22 | 0.103 | V35 | 0.034 |
Once the expert group had determined the decision indicator system and indicator weights based on years of expertise and work experience, the next step was to evaluate the identified proposed site options in order to obtain and process the decision data. The experts first scored the indicators of the three alternatives based on their own experience, using intuitionistic fuzzy language directly for qualitative indicators. For the quantitative indicators, as the decision was an antecedent event, this type of indicator was predictive data and also has a certain degree of fuzziness, so the experts also used intuitive fuzzy language for scoring, and the scoring results were shown in Table 6. For the indicators under cost perception and benefit expectation, the linguistic evaluation criterion was "whether the solution performs well under this indicator", and the corresponding linguistic word set is "Very Good, Good, Moderately Good, Moderate, Moderately Poor, Poor, Very Poor"; for the indicators under NIMBY risk, the linguistic evaluation criterion is "whether the solution performs well under this indicator". For the indicators under NIMBY risk, the criteria are "how well the program performs for this risk", which corresponds to the set of words "Very Low, Low, Moderately Low, Moderate, Moderately High, High, Very High".
Firstly, the four experts in each expert group discussed the indicators under each scheme separately; then, the evaluation data that could form a unified opinion were directly used as the final data, and those that could not form a unified opinion were discussed again, and if a unified opinion could not be reached, the plural of the data was used as the final data; finally, the final evaluation data given by the three expert groups were aggregated and collated. According to the scoring table formed by the three groups of experts (see Table 7), the evaluation language was converted into intuitionistic fuzzy numbers by the conversion rules corresponding to Table 1, and the three intuitionistic fuzzy sets were assembled by the IFWA operator to form a comprehensive intuitionistic fuzzy decision matrix (see Table 8).
Indicators | Expert Group 1 | Expert Group 2 | Expert Group 3 | ||||||
A1 | A2 | A3 | A1 | A2 | A3 | A1 | A2 | A3 | |
V11 | G | VG | VG | VG | VG | VG | MG | MG | MG |
V12 | MP | MG | M | M | G | MG | M | VG | G |
V13 | MG | MG | MG | G | G | G | M | M | M |
V14 | M | MP | MG | MP | P | M | M | M | MG |
V15 | M | G | M | G | G | MG | M | M | MP |
V21 | G | MG | VG | G | G | VG | M | MG | G |
V22 | VG | MG | VG | VG | G | VG | G | M | G |
V23 | G | MG | G | MG | M | MG | MG | MG | G |
V24 | G | MG | G | MG | M | G | G | M | G |
V31 | MH | MH | M | H | H | MP | MH | MH | M |
V32 | ML | ML | L | MH | ML | ML | MH | M | MH |
V33 | MH | ML | ML | H | H | MH | MH | MH | M |
V34 | L | ML | L | L | ML | L | L | ML | L |
V35 | L | L | L | M | M | M | ML | ML | ML |
Indicators | A1 | A2 | A3 |
V11 | (0.55, 0.30, 0.15) | (0.57, 0.26, 0.17) | (0.57, 0.26, 0.17) |
V12 | (0.39, 0.58, 0.03) | (0.56, 0.29, 0.15) | (0.48, 0.41, 0.11) |
V13 | (0.48, 0.43, 0.09) | (0.48, 0.43, 0.09) | (0.48, 0.43, 0.09) |
V14 | (0.38, 0.58, 0.04) | (0.31, 0.62, 0.07) | (0.43, 0.48, 0.09) |
V15 | (0.48, 0.46, 0.06) | (0.51, 0.40, 0.10) | (0.38, 0.52, 0.10) |
V21 | (0.51, 0.40, 0.10) | (0.48, 0.39, 0.13) | (0.61, 0.23, 0.16) |
V22 | (0.61, 0.23, 0.16) | (0.48, 0.43, 0.09) | (0.61, 0.23, 0.16) |
V23 | (0.47, 0.40, 0.13) | (0.43, 0.48, 0.09) | (0.51, 0.36, 0.13) |
V24 | (0.51, 0.36, 0.13) | (0.43, 0.53, 0.04) | (0.55, 0.32, 0.13) |
V31 | (0.26, 0.62, 0.12) | (0.26, 0.62, 0.12) | (0.38, 0.58, 0.04) |
V32 | (0.33, 0.54, 0.13) | (0.48, 0.43, 0.09) | (0.42, 0.45, 0.13) |
V33 | (0.26, 0.62, 0.12) | (0.30, 0.58, 0.13) | (0.38, 0.54, 0.08) |
V34 | (0.55, 0.32, 0.13) | (0.43, 0.43, 0.13) | (0.55, 0.32, 0.13) |
V35 | (0.46, 0.45, 0.09) | (0.46, 0.45, 0.09) | (0.46, 0.45, 0.09) |
Determine the positive ideal solution A+ and negative ideal solution A - for each scenario based on the combined intuitionistic fuzzy matrix.
A+=(1,0,0),(1,0,0),⋯,(1,0,0) |
A−=(0,1,0),(0,1,0),⋯,(0,1,0) |
The group utility value S, individual regret value R, and compromise evaluation value Q are calculated from the intuitionistic fuzzy set distance measure Eq (6) and Eqs (10)–(12), and the solution ranking is obtained from them (see Table 9). The decision preference coefficient in Eq (12) indicates the decision maker's preference φ, φ>0.5 indicates that the decision maker prefers to maximize the group benefit. φ<0.5 indicates that the decision maker prefers to minimize individual regret, and φ=0.5 indicates that the decision maker prefers to choose the solution with a compromising attitude.
Alternative sites | S | Rank | R | Rank | Q | Rank |
A1 | 0.5630 | 2 | 0.1001 | 2 | 0.8717 | 2 |
A2 | 0.5835 | 3 | 0.1001 | 2 | 1 | 3 |
A3 | 0.5036 | 1 | 0.0838 | 1 | 0 | 1 |
In view of the fact that the decision object is the three proposed site options, i.e. m = 3, we can obtain DQ = 1/(m-1) = 0.5. According to the calculation results in Table 7 and the two judgment conditions of the compromise option, we can finally obtain the comprehensive ranking of the three proposed site options as A3 > A1 > A2. As a result, Option 3 is the best choice of site. Although Option 1 is ranked second, it does not have a clear advantage and the trade-off value Q is closer to the worst option 2 and does not have a competitive advantage over Option 1.
From the previous model calculations it can be seen that with a decision preference factor of φ=0.5, the three alternative sites are ranked as A3 > A1 > A2. To further validate the stability of the model, this section presents a sensitivity analysis of the impact of φ changes in the decision preference coefficients and indicator weights on the results.
The value of the decision preference coefficient indicates the decision maker's attitude towards risk preference, and changes in it may result in changes in outcomes. The valueφof the decision preference coefficient indicates the decision maker's attitude towards risk preference, and changes in it may result in changes in outcomes. In this paper, φ values of 0, 0.1, 0.2, 0.3, 0.4, 0.5, 0.6, 0.7, 0.8, 0.9, and 1 were taken to represent different decision-making attitudes, and the ranking of the options under the different value of φis calculated and obtained as shown in Table 10. From the sensitivity analysis diagram (see Figure 3), no matter what attitude the decision maker holds, option A3 is always the best option and option A2 is always the worst option, while option A3 has the same value as option A2 at φ=0, and as the value of φ increases, its superiority improves compared to option 1, but its disadvantage is always obvious compared to option 3, thus verifying the robustness of the model.
φ | Q1 | Q2 | Q3 |
φ=0 | 1 | 1 | 0 |
φ=0.1 | 0.9743 | 1 | 0 |
φ=0.2 | 0.9487 | 1 | 0 |
φ=0.3 | 0.9230 | 1 | 0 |
φ=0.4 | 0.8974 | 1 | 0 |
φ=0.5 | 0.8717 | 1 | 0 |
φ=0.6 | 0.8461 | 1 | 0 |
φ=0.7 | 0.8204 | 1 | 0 |
φ=0.8 | 0.7947 | 1 | 0 |
φ=0.9 | 0.7691 | 1 | 0 |
φ=1 | 0.7434 | 1 | 0 |
Does a change in the weighting of the indicators determined by the DEMATEL method have an impact on the ranking of the schemes? What impact would it have? Sensitivity analysis allows for the identification of deviations from the decision outcome that would result from potential changes in the indicator weights of the decision, which is key to the effective use of the model and the implementation of quantitative decision-making [54]. Sensitivity analysis of indicator weights, i.e., the corresponding change in the priority order of each potential supplier when the indicator weights in the decision are perturbed, is carried out using the regression method.
Let the initial weight of the decision indicator Vij be ωj, and the perturbed indicator weights be denoted as ϖj=ζωj, where0⩽ϖj⩽1, 0⩽ξ⩽1/ωj. Since the sum of the weights should be 1, when one of the weights ωj changes, the other weights will also change, denoted as ϖk=εωk, k≠j, k=1,2,⋯,m and satisfying ϖj+m∑k≠i,k=1ϖk=1⇒ξωj+εm∑k≠i,k=1ωk=1, which leads to ε=(1−ξωj)/(1−ωj). For each decision indicator, when taking different parameters ξ, the VIKOR method is used to obtain the corresponding alternative ranking. The 14 decision indicator weights are perturbed separately, and the values of ξ are taken as 1/2 and 2 accordingly. 28 perturbation experiments are conducted, and the sensitivity analysis results are obtained as shown in Figure 4.
In Table 11, the results for perturbations 1 to 14 are for the value of ξ is 1/2 and for perturbations 15 to 28 are the value of ξ is 2. As can be seen from the results, the value of Q of option A3 is always 0, while the value of Q of option A2 is always 1, while the value of Q of option A1 is still far from the dominant effect of option A3, although there are obvious fluctuations in the process of 28 perturbations. This shows that the model proposed in this study has strong stability and accuracy.
Number of perturbations | Q1 | Q2 | Q3 | Number of perturbations | Q1 | Q2 | Q3 |
1 | 0.8689 | 1 | 0 | 15 | 0.8780 | 1 | 0 |
2 | 0.8609 | 1 | 0 | 16 | 0.8953 | 1 | 0 |
3 | 0.8718 | 1 | 0 | 17 | 0.8718 | 1 | 0 |
4 | 0.8728 | 1 | 0 | 18 | 0.8698 | 1 | 0 |
5 | 0.8889 | 1 | 0 | 19 | 0.8716 | 1 | 0 |
6 | 0.8688 | 1 | 0 | 20 | 0.8772 | 1 | 0 |
7 | 0.9078 | 1 | 0 | 21 | 0.8070 | 1 | 0 |
8 | 0.8776 | 1 | 0 | 22 | 0.8602 | 1 | 0 |
9 | 0.8946 | 1 | 0 | 23 | 0.8308 | 1 | 0 |
10 | 0.7841 | 1 | 0 | 24 | 0.8998 | 1 | 0 |
11 | 0.8403 | 1 | 0 | 25 | 0.9486 | 1 | 0 |
12 | 0.8520 | 1 | 0 | 26 | 0.9111 | 1 | 0 |
13 | 0.8950 | 1 | 0 | 27 | 0.8279 | 1 | 0 |
14 | 0.8718 | 1 | 0 | 28 | 0.8718 | 1 | 0 |
WtE projects are prone to NIMBY risk issues in the siting decision process, which can lead to public resistance and opposition to the project. One of the important ways to solve the location decision problem of the WtE project is to establish a comprehensive location decision framework. Therefore, this paper constructs the WtE project location decision framework from two aspects: the index system and the decision method. The main conclusions are as follows:
● First of all, this article will avoid risk in the location decisions, from the expected cost awareness, efficiency, and adjacent to avoid risk from three aspects of 14 indicators, constructs the WtE project site selection index system, and detailed analysis of the index meaning.
● DEMATEL method is used to determine the location of index weight, and intuitionistic fuzzy VIKOR extends into the environment, sort of WtE spare location. This method takes into account the interaction between decision indicators and the psychological preference of decision makers and solves the ambiguity and uncertainty of the decision environment.
● The decision-making framework was applied to a specific case projects. Among the three alternative sites in the case, A3 is considered to be the best site for the project, while A1 is the less favorable. In order to ensure the reliability of the results, sensitivity analysis is used to verify the stability of the site selection results and prove the reliability of the site selection decision-making framework of the WtE project.
The contribution of this study includes two aspects. For the theoretical contribution, this study analyzes the characteristics of the NIMBY problem, improves the site selection decision index system, and considers the sustainability of the WtE project and the impact of the project on residents' lives. In addition, the proposed decision-making framework can be extended to other renewable energy fields, which fully solves the shortcomings of previous decision-making methods, such as not fully considering the correlation and influence relationship between factors, the fuzziness of evaluation and decision-making environment, the stability and reliability of ranking, and is a theoretical supplement to similar decision-making problems. For the practical contribution, the site selection decision-making framework can solve complex decision-making problems, help decision makers determine site selection scientifically and reasonably, reduce the impact of NIMBY problems on the project, and has certain theoretical significance for the sustainable development of society.
However, there are still issues with this study that require further refinement in subsequent studies. This work identified indicators mainly by consulting experts and searching relevant literature, which may not include all relevant influencing factors. The indicator system can be slightly modified according to the actual situation of the project. And the way of public participation in the site selection decision-making process can be further explored so as to fully understand the opinions of the residents in the area of the project.
We thank the editors and reviewers for their efforts to improve the quality of this article.
The authors declare no conflicts of interest.
[1] |
W. Diffie, M. Hellman, New directions in cryptography, IEEE T. Inform. Theory, 22 (1976), 644–654. http://dx.doi.org/10.1109/TIT.1976.1055638 doi: 10.1109/TIT.1976.1055638
![]() |
[2] | D. Boneh, V. Shoup, A graduate course in applied cryptography, 0.6 Eds., 2023, unpublished work. Available from: https://toc.cryptobook.us/book.pdf. |
[3] | E. Bresson, Y. Lakhnech, L. Mazaré, B. Warinschi, A generalization of DDH with applications to protocol analysis and computational soundness, In: Advances in cryptology-CRYPTO 2007, Berlin: Springer, 2007,482–499. http://dx.doi.org/10.1007/978-3-540-74143-5_27 |
[4] |
R. Rivest, A. Shamir, L. Adleman, A method for obtaining digital signatures and public-key cryptosystems, Commun. ACM, 21 (1978), 120–126. http://dx.doi.org/10.1145/359340.359342 doi: 10.1145/359340.359342
![]() |
[5] |
P. Shor, Polynomial-time algorithms for prime factorization and discrete logarithms on a quantum computer, SIAM Rev., 41 (1999), 303–332. http://dx.doi.org/10.1137/S0036144598347011 doi: 10.1137/S0036144598347011
![]() |
[6] | Submission requirements and evaluation criteria for the post-quantum cryptography standardization process, NIST Computer Security Resource Center, 2016. Available from: https://csrc.nist.gov/CSRC/media/Projects/Post-Quantum-Cryptography/documents/call-for-proposals-final-dec-2016.pdf. |
[7] | Post-quantum cryptography, selected algorithms 2022, NIST Computer Security Resource Center, 2022. Available from: https://csrc.nist.gov/Projects/post-quantum-cryptography/selected-algorithms-2022. |
[8] | Post-quantum cryptography, round 4 submissions, NIST Computer Security Resource Center, 2022. Available from: https://csrc.nist.gov/Projects/post-quantum-cryptography/round-4-submissions. |
[9] | K. Ko, S. Lee, J. Cheon, J. Han, J. Kang, C. Park, New public-key cryptosystem using braid groups, In: Advances in cryptology-CRYPTO 2000, Berlin: Springer, 2000,166–183. http://dx.doi.org/10.1007/3-540-44598-6_10 |
[10] |
V. Shpilrain, A. Ushakov, The conjugacy search problem in public key cryptography: unnecessary and insufficient, AAECC, 17 (2006), 285–289. http://dx.doi.org/10.1007/s00200-006-0009-6 doi: 10.1007/s00200-006-0009-6
![]() |
[11] | E. Sakalauskas, N. Listopadskis, P. Tvarijonas, Key agreement protocol (KAP) based on matrix power function, Proceedings of Sixth International Conference on Information Research and Applications, 2008, 92–96. |
[12] |
A. Mihalkovich, E. Sakalauskas, Asymmetric cipher based on MPF and its security parameters evaluation, Lietuvos Matematikos Rinkinys, 53 (2012), 72–77. http://dx.doi.org/10.15388/LMR.A.2012.13 doi: 10.15388/LMR.A.2012.13
![]() |
[13] | J. Liu, H. Zhang, J. Jia, A linear algebra attack on the non-commuting cryptography class based on matrix power function, In: Information security and cryptology, Cham: Springer, 2017,343–354. http://dx.doi.org/10.1007/978-3-319-54705-3_21 |
[14] |
E. Sakalauskas, A. Mihalkovich, Improved asymmetric cipher based on matrix power function resistant to linear algebra attack, Informatica, 28 (2017), 517–524. http://dx.doi.org/10.15388/Informatica.2017.142 doi: 10.15388/Informatica.2017.142
![]() |
[15] | A. Mihalkovich, M. Levinskas, Investigation of matrix power asymmetric cipher resistant to linear algebra attack, In: Information and software technologies, Cham: Springer, 2019,197–208. http://dx.doi.org/10.1007/978-3-030-30275-7_16 |
[16] |
A. Mihalkovich, E. Sakalauskas, K. Luksys, Key exchange protocol defined over a non-commuting group based on an NP-complete decisional problem, Symmetry, 12 (2020), 1389. http://dx.doi.org/10.3390/sym12091389 doi: 10.3390/sym12091389
![]() |
[17] |
A. Mihalkovich, K. Luksys, E. Sakalauskas, Sigma identification protocol construction based on MPF defined over non-commuting platform group, Mathematics, 10 (2022), 2649. http://dx.doi.org/10.3390/math10152649 doi: 10.3390/math10152649
![]() |
[18] |
M. Durcheva, TrES: tropical encryption scheme based on double key exchange, European Journal of Information Technologies and Computer Science, 2 (2022), 11–17. http://dx.doi.org/10.24018/compute.2022.2.4.70 doi: 10.24018/compute.2022.2.4.70
![]() |
[19] |
X. Jiang, H. Huang, G. Pan, Cryptanalysis of tropical encryption scheme based on double key exchange, Journal of Cyber Security and Mobility, 12 (2023), 205–220. http://dx.doi.org/10.13052/jcsm2245-1439.1224 doi: 10.13052/jcsm2245-1439.1224
![]() |
[20] | Modular maximal-cyclic group, Groupprops, 2023, Available from: https://groupprops.subwiki.org/wiki/Modular_maximal-cyclic_group. |
[21] |
H. Grundman, T. Smith, Automatic realizability of Galois groups of order 16, Proc. Amer. Math. Soc., 124 (1996), 2631–2640. http://dx.doi.org/10.1090/S0002-9939-96-03345-X doi: 10.1090/S0002-9939-96-03345-X
![]() |
[22] |
H. Grundman, T. Smith, Realizability and automatic realizability of Galois groups of order 32, Open Math., 8 (2010), 244–260. https://doi.org/10.2478/s11533-009-0072-x doi: 10.2478/s11533-009-0072-x
![]() |
[23] |
H. Grundman, T. Smith, Galois realizability of groups of order 64, Centr. Eur. J. Math., 8 (2010), 846–854. http://dx.doi.org/10.2478/s11533-010-0052-1 doi: 10.2478/s11533-010-0052-1
![]() |
[24] | A. Mihalkovich, E. Sakalauskas, M. Levinskas, Key exchange protocol based on the matrix power function defined over M16, In: Intelligent computing, Cham: Springer, 2022,511–531. http://dx.doi.org/10.1007/978-3-031-10467-1_32 |
[25] | Faithful irreducible representation of M16, Groupprops, 2023, Available from: https://groupprops.subwiki.org/wiki/Faithful_irreducible_representation_of_M16. |
[26] | J. Faugère, A. Joux, Algebraic cryptanalysis of hidden field equation (HFE) cryptosystems using Gröbner bases, In: Advances in cryptology-CRYPTO 2003, Berlin: Springer, 2003, 44–60. http://dx.doi.org/10.1007/978-3-540-45146-4_3 |
[27] | A. Kipnis, J. Patarin, L. Goubin, Unbalanced oil and vinegar signature schemes, Advances in Cryptology-EUROCRYPT'99, Berlin: Springer, 1999,206–222. http://dx.doi.org/10.1007/3-540-48910-X_15 |
[28] | R. Benadjila, T. Feneuil, M. Rivain, MQ on my mind: post-quantum signatures from the non-structured multivariate quadratic problem, Proceedings of IEEE 9th European Symposium on Security and Privacy, 2024,468–485. http://dx.doi.org/10.1109/EuroSP60621.2024.00032 |
[29] |
A. Mihalkovich, J. Zitkevicius, On the decisional problem based on matrix power function defined over non-commutative group, Mathematical Models in Engineering, 10 (2024), 1–9. http://dx.doi.org/10.21595/mme.2024.24071 doi: 10.21595/mme.2024.24071
![]() |
1. | Tao Jiang, Zhenchao Xu, Busheng Zhou, Qingyun Zhang, Yong Liu, Underlying Causes of NIMBY Conflicts in Power Grid Construction Projects: An ISM–BN Model Perspective, 2024, 14, 2075-5309, 2140, 10.3390/buildings14072140 | |
2. | Jih-Chang Wang, Ting-Yu Chen, A compromise decision-support technique with an augmented scoring function within circular intuitionistic fuzzy settings, 2024, 128, 09521976, 107359, 10.1016/j.engappai.2023.107359 | |
3. | Ting-Yu Chen, Multiple criteria decision analytic methods in management with T-spherical fuzzy information, 2023, 56, 0269-2821, 14087, 10.1007/s10462-023-10461-z | |
4. | Pratibha Rani, Arunodaya Raj Mishra, Fausto Cavallaro, Adel Fahad Alrasheedi, Location selection for offshore wind power station using interval-valued intuitionistic fuzzy distance measure-RANCOM-WISP method, 2024, 14, 2045-2322, 10.1038/s41598-024-54929-6 | |
5. | Md. Ruhul Ferdoush, Ridwan Al Aziz, Chitra Lekha Karmaker, Binoy Debnath, Mohammad Hossain Limon, A.B.M. Mainul Bari, Unraveling the challenges of waste-to-energy transition in emerging economies: Implications for sustainability, 2024, 3, 29497531, 100121, 10.1016/j.igd.2023.100121 | |
6. | Shengbin Ma, Zhongfu Li, Jingqi Zhang, A multi-attribute group decision-making framework for site selection of waste-to-energy project considering public participation, 2024, 0969-9988, 10.1108/ECAM-05-2024-0664 |
First-level indicators | Second-level indicators | Explanation of indicators |
Cost perception (V1) | Operation and maintenance costs (V11) | This indicator is an important indicator for achieving economic sustainability. It refers to the various types of costs incurred during the construction and operation, and maintenance of the project, which was determined mainly based of waste generation potential, interest rates, and inflation rates [32,33]. |
Local land prices (V12) | This indicator refers to the price of land where the project is being built, and the price of local land is an important factor affecting investment capital. When determining the site of the WtE project, the local land price should be fully considered [34,35]. | |
Fuel acquisition costs (V13) | This indicator refers to the expenditure during the project's operation, which includes fuel purchase costs and transportation costs [36,37]. | |
Distance to ancillary facilities (V14) | The construction and operation of a WtE project require appropriate supporting facilities, and this indicator measures the distance between the alternative site and facilities such as electricity, water, and gas [19,35]. | |
Wind conditions and hydrogeological conditions (V15) | Wind and hydrogeological conditions in the vicinity of the construction site can directly influence the project's economic viability and technical choice. If the WtE project is located downwind of the annual prevailing wind direction, pollutant gases can reach further into the area, leading to a higher possibility of gas leakage accidents. In addition, a survey of local hydrological and geological conditions is also necessary to avoid unnecessary damage caused by flooding and geological hazards [35,38]. | |
Benefit Expectations (V2) | Improving living standards (V21) | This indicator measures the extent to which a WtE project has improved the living standards of the people in the area where it is located, mainly in material and spiritual terms. The WtE project is an effective solution to the problem of "rubbish encircling the city", maintaining an attractive and clean living environment and increasing the employment and income levels of the surrounding population [39,40]. |
Service capacity (V22) | This indicator measures the daily waste throughput of the WtE project, as well as the project's service area [37]. | |
Benefit Expectations (V2) | Promoting urbanisation (V23) | The construction and operation of WtE projects require the involvement of a large number of people, which can provide more employment opportunities for the public in the areas surrounding the projects. In this way, the city will have a more substantial agglomeration and radiation drive, absorbing the remaining rural population and speeding up the urbanization process [37,39]. |
Promoting local economic development (V24) | After the project is completed and running, it can promote local economic development. It will create new employment opportunities for residents, transform urban waste into renewable energy, drive the development of the surrounding industries, realize the adjustment of the industrial structure and increase the region's tax reveune [37,39,41]. | |
Risk of environmental damage (V31) | This indicator measures the risk that WtE projects may hurt the daily lives of residents and the ecological environment. It is an exogenous natural risk, including air pollution, water pollution, and noise pollution. Although waste incineration reduces greenhouse gas emissions, it may bring about emissions of atmospheric particulate matter, such as dioxins, which can lead to air pollution [37,39,42]. | |
NIMBY risk (V3) | Health risks to the local population (V32) | This indicator measures the generation of various toxic gases and environmental pollutants such as dioxins during incineration, which inevitably lead to environmental pollution and human health risks [43,44]. |
Risk of public conflict (V33) | The construction of waste-to-energy plants may lead to public conflict risks. Such NIMBY facilities are closely related to the vital interests of the surrounding public, such as life and health, property gains, and environmental rights. When the rights and interests of the public are compromised as a result of the construction and operation of the facility, they may frequently initiate advocacy actions against decision-makers, project contractors, and facility operators, resisting and interfering with the implementation of the project, resulting in conflicts between the government, enterprises and the public [33,45,46]. | |
Risk of safety incidents (V34) | This indicator is an endogenous natural risk arising from design flaws in the processes used at NIMBY facilities or the hazardous properties of raw materials and product by-products (flammable, explosive, toxic), as well as inappropriate process layout and equipment installation flaws. It is mainly due to fires caused by raw materials and by-products, biochemical hazards caused by the leakage of toxic and hazardous substances, as well as health hazards to operators caused by high temperatures, pressures, noise, and dust [10,44]. | |
Risk of asset losses (V35) | This indicator measures the potential risk of loss of asset value during the construction and operation of the project, such as the depreciation of house prices in the area where the project is located [46,47]. |
Language word collection | Number of intuitive blurs | |
Very Good (VG) | Very Low (VL) | (0.72, 0.13, 0.15) |
Good (G) | Low (L) | (0.63, 0.24, 0.13) |
Moderately Good (MG) | Moderately Low (ML) | (0.51, 0.35, 0.14) |
Moderate (M) | Moderate (M) | (0.50, 0.50, 0.00) |
Moderately Poor (MP) | Moderately High (MH) | (0.35, 0.51, 0.14) |
Poor (P) | High (H) | (0.24, 0.63, 0.13) |
Very Poor (VP) | Very High (VH) | (0.13, 0.72, 0.15) |
Indicators | V11 | V12 | V13 | V14 | V15 | V21 | V22 | V23 | V24 | V31 | V32 | V33 | V34 | V35 |
V11 | 0 | 0 | 0 | 0 | 0 | 0 | 0 | 0 | 0 | 0 | 0 | 0 | 0 | 0 |
V12 | 3 | 0 | 1 | 2 | 1 | 0 | 0 | 0 | 0 | 0 | 0 | 0 | 0 | 0 |
V13 | 3 | 0 | 0 | 0 | 0 | 0 | 0 | 0 | 0 | 0 | 0 | 0 | 0 | 0 |
V14 | 2 | 0 | 0 | 0 | 0 | 0 | 0 | 0 | 0 | 0 | 0 | 0 | 0 | 0 |
V15 | 3 | 1 | 2 | 0 | 0 | 0 | 0 | 0 | 0 | 0 | 0 | 0 | 0 | 0 |
V21 | 0 | 0 | 0 | 0 | 0 | 0 | 2 | 4 | 4 | 0 | 0 | 0 | 0 | 0 |
V22 | 0 | 0 | 0 | 0 | 0 | 4 | 0 | 3 | 4 | 0 | 0 | 3 | 0 | 0 |
V23 | 0 | 0 | 0 | 0 | 0 | 3 | 2 | 0 | 4 | 0 | 0 | 2 | 0 | 0 |
V24 | 0 | 0 | 0 | 0 | 0 | 4 | 3 | 4 | 0 | 0 | 0 | 2 | 0 | 0 |
V31 | 0 | 0 | 0 | 0 | 0 | 3 | 3 | 3 | 3 | 0 | 4 | 4 | 3 | 3 |
V32 | 0 | 0 | 0 | 0 | 0 | 4 | 2 | 3 | 3 | 0 | 0 | 4 | 1 | 3 |
V33 | 0 | 0 | 0 | 0 | 0 | 0 | 0 | 0 | 0 | 0 | 0 | 0 | 0 | 0 |
V34 | 0 | 0 | 0 | 0 | 0 | 0 | 2 | 0 | 0 | 2 | 4 | 4 | 0 | 3 |
V35 | 0 | 0 | 0 | 0 | 0 | 0 | 0 | 0 | 0 | 0 | 0 | 0 | 0 | 0 |
Indicators | V11 | V12 | V13 | V14 | V15 | V21 | V22 | V23 | V24 | V31 | V32 | V33 | V34 | V35 |
V11 | 0.000 | 0.000 | 0.000 | 0.000 | 0.000 | 0.000 | 0.000 | 0.000 | 0.000 | 0.000 | 0.000 | 0.000 | 0.000 | 0.000 |
V12 | 0.131 | 0.001 | 0.041 | 0.077 | 0.039 | 0.000 | 0.000 | 0.000 | 0.000 | 0.000 | 0.000 | 0.000 | 0.000 | 0.000 |
V13 | 0.115 | 0.000 | 0.000 | 0.000 | 0.000 | 0.000 | 0.000 | 0.000 | 0.000 | 0.000 | 0.000 | 0.000 | 0.000 | 0.000 |
V14 | 0.077 | 0.000 | 0.000 | 0.000 | 0.000 | 0.000 | 0.000 | 0.000 | 0.000 | 0.000 | 0.000 | 0.000 | 0.000 | 0.000 |
V15 | 0.129 | 0.039 | 0.079 | 0.003 | 0.001 | 0.000 | 0.000 | 0.000 | 0.000 | 0.000 | 0.000 | 0.000 | 0.000 | 0.000 |
V21 | 0.000 | 0.000 | 0.000 | 0.000 | 0.000 | 0.077 | 0.124 | 0.214 | 0.218 | 0.000 | 0.000 | 0.048 | 0.000 | 0.000 |
V22 | 0.000 | 0.000 | 0.000 | 0.000 | 0.000 | 0.219 | 0.058 | 0.191 | 0.226 | 0.000 | 0.000 | 0.154 | 0.000 | 0.000 |
V23 | 0.000 | 0.000 | 0.000 | 0.000 | 0.000 | 0.175 | 0.120 | 0.073 | 0.210 | 0.000 | 0.000 | 0.113 | 0.000 | 0.000 |
V24 | 0.000 | 0.000 | 0.000 | 0.000 | 0.000 | 0.218 | 0.160 | 0.220 | 0.092 | 0.000 | 0.000 | 0.119 | 0.000 | 0.000 |
V31 | 0.000 | 0.000 | 0.000 | 0.000 | 0.000 | 0.239 | 0.203 | 0.234 | 0.241 | 0.009 | 0.174 | 0.261 | 0.123 | 0.151 |
V32 | 0.000 | 0.000 | 0.000 | 0.000 | 0.000 | 0.231 | 0.137 | 0.199 | 0.204 | 0.003 | 0.006 | 0.208 | 0.039 | 0.121 |
V33 | 0.000 | 0.000 | 0.000 | 0.000 | 0.000 | 0.000 | 0.000 | 0.000 | 0.000 | 0.000 | 0.000 | 0.000 | 0.000 | 0.000 |
V34 | 0.000 | 0.000 | 0.000 | 0.000 | 0.000 | 0.071 | 0.118 | 0.063 | 0.067 | 0.078 | 0.168 | 0.218 | 0.015 | 0.146 |
V35 | 0.000 | 0.000 | 0.000 | 0.000 | 0.000 | 0.000 | 0.000 | 0.000 | 0.000 | 0.000 | 0.000 | 0.000 | 0.000 | 0.000 |
Indicators | Impact Degree A | Influenced degree B | Centrality M | Cause Degree U |
V11 | 0.000 | 0.452 | 0.452 | -0.452 |
V12 | 0.289 | 0.040 | 0.329 | 0.249 |
V13 | 0.115 | 0.120 | 0.235 | -0.005 |
V14 | 0.077 | 0.080 | 0.157 | -0.003 |
V15 | 0.251 | 0.040 | 0.291 | 0.211 |
V21 | 0.681 | 1.229 | 1.910 | -0.549 |
V22 | 0.847 | 0.921 | 1.768 | -0.074 |
V23 | 0.691 | 1.193 | 1.885 | -0.502 |
V24 | 0.809 | 1.257 | 2.066 | -0.449 |
V31 | 1.635 | 0.091 | 1.726 | 1.544 |
V32 | 1.149 | 0.349 | 1.497 | 0.800 |
V33 | 0.000 | 1.121 | 1.121 | -1.121 |
V34 | 0.945 | 0.178 | 1.122 | 0.767 |
V35 | 0.000 | 0.417 | 0.417 | -0.417 |
Indicators | Weighting | Indicators | Weighting |
V11 | 0.037 | V23 | 0.114 |
V12 | 0.024 | V24 | 0.123 |
V13 | 0.014 | V31 | 0.135 |
V14 | 0.009 | V32 | 0.099 |
V15 | 0.021 | V33 | 0.092 |
V21 | 0.116 | V34 | 0.079 |
V22 | 0.103 | V35 | 0.034 |
Indicators | Expert Group 1 | Expert Group 2 | Expert Group 3 | ||||||
A1 | A2 | A3 | A1 | A2 | A3 | A1 | A2 | A3 | |
V11 | G | VG | VG | VG | VG | VG | MG | MG | MG |
V12 | MP | MG | M | M | G | MG | M | VG | G |
V13 | MG | MG | MG | G | G | G | M | M | M |
V14 | M | MP | MG | MP | P | M | M | M | MG |
V15 | M | G | M | G | G | MG | M | M | MP |
V21 | G | MG | VG | G | G | VG | M | MG | G |
V22 | VG | MG | VG | VG | G | VG | G | M | G |
V23 | G | MG | G | MG | M | MG | MG | MG | G |
V24 | G | MG | G | MG | M | G | G | M | G |
V31 | MH | MH | M | H | H | MP | MH | MH | M |
V32 | ML | ML | L | MH | ML | ML | MH | M | MH |
V33 | MH | ML | ML | H | H | MH | MH | MH | M |
V34 | L | ML | L | L | ML | L | L | ML | L |
V35 | L | L | L | M | M | M | ML | ML | ML |
Indicators | A1 | A2 | A3 |
V11 | (0.55, 0.30, 0.15) | (0.57, 0.26, 0.17) | (0.57, 0.26, 0.17) |
V12 | (0.39, 0.58, 0.03) | (0.56, 0.29, 0.15) | (0.48, 0.41, 0.11) |
V13 | (0.48, 0.43, 0.09) | (0.48, 0.43, 0.09) | (0.48, 0.43, 0.09) |
V14 | (0.38, 0.58, 0.04) | (0.31, 0.62, 0.07) | (0.43, 0.48, 0.09) |
V15 | (0.48, 0.46, 0.06) | (0.51, 0.40, 0.10) | (0.38, 0.52, 0.10) |
V21 | (0.51, 0.40, 0.10) | (0.48, 0.39, 0.13) | (0.61, 0.23, 0.16) |
V22 | (0.61, 0.23, 0.16) | (0.48, 0.43, 0.09) | (0.61, 0.23, 0.16) |
V23 | (0.47, 0.40, 0.13) | (0.43, 0.48, 0.09) | (0.51, 0.36, 0.13) |
V24 | (0.51, 0.36, 0.13) | (0.43, 0.53, 0.04) | (0.55, 0.32, 0.13) |
V31 | (0.26, 0.62, 0.12) | (0.26, 0.62, 0.12) | (0.38, 0.58, 0.04) |
V32 | (0.33, 0.54, 0.13) | (0.48, 0.43, 0.09) | (0.42, 0.45, 0.13) |
V33 | (0.26, 0.62, 0.12) | (0.30, 0.58, 0.13) | (0.38, 0.54, 0.08) |
V34 | (0.55, 0.32, 0.13) | (0.43, 0.43, 0.13) | (0.55, 0.32, 0.13) |
V35 | (0.46, 0.45, 0.09) | (0.46, 0.45, 0.09) | (0.46, 0.45, 0.09) |
Alternative sites | S | Rank | R | Rank | Q | Rank |
A1 | 0.5630 | 2 | 0.1001 | 2 | 0.8717 | 2 |
A2 | 0.5835 | 3 | 0.1001 | 2 | 1 | 3 |
A3 | 0.5036 | 1 | 0.0838 | 1 | 0 | 1 |
φ | Q1 | Q2 | Q3 |
φ=0 | 1 | 1 | 0 |
φ=0.1 | 0.9743 | 1 | 0 |
φ=0.2 | 0.9487 | 1 | 0 |
φ=0.3 | 0.9230 | 1 | 0 |
φ=0.4 | 0.8974 | 1 | 0 |
φ=0.5 | 0.8717 | 1 | 0 |
φ=0.6 | 0.8461 | 1 | 0 |
φ=0.7 | 0.8204 | 1 | 0 |
φ=0.8 | 0.7947 | 1 | 0 |
φ=0.9 | 0.7691 | 1 | 0 |
φ=1 | 0.7434 | 1 | 0 |
Number of perturbations | Q1 | Q2 | Q3 | Number of perturbations | Q1 | Q2 | Q3 |
1 | 0.8689 | 1 | 0 | 15 | 0.8780 | 1 | 0 |
2 | 0.8609 | 1 | 0 | 16 | 0.8953 | 1 | 0 |
3 | 0.8718 | 1 | 0 | 17 | 0.8718 | 1 | 0 |
4 | 0.8728 | 1 | 0 | 18 | 0.8698 | 1 | 0 |
5 | 0.8889 | 1 | 0 | 19 | 0.8716 | 1 | 0 |
6 | 0.8688 | 1 | 0 | 20 | 0.8772 | 1 | 0 |
7 | 0.9078 | 1 | 0 | 21 | 0.8070 | 1 | 0 |
8 | 0.8776 | 1 | 0 | 22 | 0.8602 | 1 | 0 |
9 | 0.8946 | 1 | 0 | 23 | 0.8308 | 1 | 0 |
10 | 0.7841 | 1 | 0 | 24 | 0.8998 | 1 | 0 |
11 | 0.8403 | 1 | 0 | 25 | 0.9486 | 1 | 0 |
12 | 0.8520 | 1 | 0 | 26 | 0.9111 | 1 | 0 |
13 | 0.8950 | 1 | 0 | 27 | 0.8279 | 1 | 0 |
14 | 0.8718 | 1 | 0 | 28 | 0.8718 | 1 | 0 |
First-level indicators | Second-level indicators | Explanation of indicators |
Cost perception (V1) | Operation and maintenance costs (V11) | This indicator is an important indicator for achieving economic sustainability. It refers to the various types of costs incurred during the construction and operation, and maintenance of the project, which was determined mainly based of waste generation potential, interest rates, and inflation rates [32,33]. |
Local land prices (V12) | This indicator refers to the price of land where the project is being built, and the price of local land is an important factor affecting investment capital. When determining the site of the WtE project, the local land price should be fully considered [34,35]. | |
Fuel acquisition costs (V13) | This indicator refers to the expenditure during the project's operation, which includes fuel purchase costs and transportation costs [36,37]. | |
Distance to ancillary facilities (V14) | The construction and operation of a WtE project require appropriate supporting facilities, and this indicator measures the distance between the alternative site and facilities such as electricity, water, and gas [19,35]. | |
Wind conditions and hydrogeological conditions (V15) | Wind and hydrogeological conditions in the vicinity of the construction site can directly influence the project's economic viability and technical choice. If the WtE project is located downwind of the annual prevailing wind direction, pollutant gases can reach further into the area, leading to a higher possibility of gas leakage accidents. In addition, a survey of local hydrological and geological conditions is also necessary to avoid unnecessary damage caused by flooding and geological hazards [35,38]. | |
Benefit Expectations (V2) | Improving living standards (V21) | This indicator measures the extent to which a WtE project has improved the living standards of the people in the area where it is located, mainly in material and spiritual terms. The WtE project is an effective solution to the problem of "rubbish encircling the city", maintaining an attractive and clean living environment and increasing the employment and income levels of the surrounding population [39,40]. |
Service capacity (V22) | This indicator measures the daily waste throughput of the WtE project, as well as the project's service area [37]. | |
Benefit Expectations (V2) | Promoting urbanisation (V23) | The construction and operation of WtE projects require the involvement of a large number of people, which can provide more employment opportunities for the public in the areas surrounding the projects. In this way, the city will have a more substantial agglomeration and radiation drive, absorbing the remaining rural population and speeding up the urbanization process [37,39]. |
Promoting local economic development (V24) | After the project is completed and running, it can promote local economic development. It will create new employment opportunities for residents, transform urban waste into renewable energy, drive the development of the surrounding industries, realize the adjustment of the industrial structure and increase the region's tax reveune [37,39,41]. | |
Risk of environmental damage (V31) | This indicator measures the risk that WtE projects may hurt the daily lives of residents and the ecological environment. It is an exogenous natural risk, including air pollution, water pollution, and noise pollution. Although waste incineration reduces greenhouse gas emissions, it may bring about emissions of atmospheric particulate matter, such as dioxins, which can lead to air pollution [37,39,42]. | |
NIMBY risk (V3) | Health risks to the local population (V32) | This indicator measures the generation of various toxic gases and environmental pollutants such as dioxins during incineration, which inevitably lead to environmental pollution and human health risks [43,44]. |
Risk of public conflict (V33) | The construction of waste-to-energy plants may lead to public conflict risks. Such NIMBY facilities are closely related to the vital interests of the surrounding public, such as life and health, property gains, and environmental rights. When the rights and interests of the public are compromised as a result of the construction and operation of the facility, they may frequently initiate advocacy actions against decision-makers, project contractors, and facility operators, resisting and interfering with the implementation of the project, resulting in conflicts between the government, enterprises and the public [33,45,46]. | |
Risk of safety incidents (V34) | This indicator is an endogenous natural risk arising from design flaws in the processes used at NIMBY facilities or the hazardous properties of raw materials and product by-products (flammable, explosive, toxic), as well as inappropriate process layout and equipment installation flaws. It is mainly due to fires caused by raw materials and by-products, biochemical hazards caused by the leakage of toxic and hazardous substances, as well as health hazards to operators caused by high temperatures, pressures, noise, and dust [10,44]. | |
Risk of asset losses (V35) | This indicator measures the potential risk of loss of asset value during the construction and operation of the project, such as the depreciation of house prices in the area where the project is located [46,47]. |
Language word collection | Number of intuitive blurs | |
Very Good (VG) | Very Low (VL) | (0.72, 0.13, 0.15) |
Good (G) | Low (L) | (0.63, 0.24, 0.13) |
Moderately Good (MG) | Moderately Low (ML) | (0.51, 0.35, 0.14) |
Moderate (M) | Moderate (M) | (0.50, 0.50, 0.00) |
Moderately Poor (MP) | Moderately High (MH) | (0.35, 0.51, 0.14) |
Poor (P) | High (H) | (0.24, 0.63, 0.13) |
Very Poor (VP) | Very High (VH) | (0.13, 0.72, 0.15) |
Indicators | V11 | V12 | V13 | V14 | V15 | V21 | V22 | V23 | V24 | V31 | V32 | V33 | V34 | V35 |
V11 | 0 | 0 | 0 | 0 | 0 | 0 | 0 | 0 | 0 | 0 | 0 | 0 | 0 | 0 |
V12 | 3 | 0 | 1 | 2 | 1 | 0 | 0 | 0 | 0 | 0 | 0 | 0 | 0 | 0 |
V13 | 3 | 0 | 0 | 0 | 0 | 0 | 0 | 0 | 0 | 0 | 0 | 0 | 0 | 0 |
V14 | 2 | 0 | 0 | 0 | 0 | 0 | 0 | 0 | 0 | 0 | 0 | 0 | 0 | 0 |
V15 | 3 | 1 | 2 | 0 | 0 | 0 | 0 | 0 | 0 | 0 | 0 | 0 | 0 | 0 |
V21 | 0 | 0 | 0 | 0 | 0 | 0 | 2 | 4 | 4 | 0 | 0 | 0 | 0 | 0 |
V22 | 0 | 0 | 0 | 0 | 0 | 4 | 0 | 3 | 4 | 0 | 0 | 3 | 0 | 0 |
V23 | 0 | 0 | 0 | 0 | 0 | 3 | 2 | 0 | 4 | 0 | 0 | 2 | 0 | 0 |
V24 | 0 | 0 | 0 | 0 | 0 | 4 | 3 | 4 | 0 | 0 | 0 | 2 | 0 | 0 |
V31 | 0 | 0 | 0 | 0 | 0 | 3 | 3 | 3 | 3 | 0 | 4 | 4 | 3 | 3 |
V32 | 0 | 0 | 0 | 0 | 0 | 4 | 2 | 3 | 3 | 0 | 0 | 4 | 1 | 3 |
V33 | 0 | 0 | 0 | 0 | 0 | 0 | 0 | 0 | 0 | 0 | 0 | 0 | 0 | 0 |
V34 | 0 | 0 | 0 | 0 | 0 | 0 | 2 | 0 | 0 | 2 | 4 | 4 | 0 | 3 |
V35 | 0 | 0 | 0 | 0 | 0 | 0 | 0 | 0 | 0 | 0 | 0 | 0 | 0 | 0 |
Indicators | V11 | V12 | V13 | V14 | V15 | V21 | V22 | V23 | V24 | V31 | V32 | V33 | V34 | V35 |
V11 | 0.000 | 0.000 | 0.000 | 0.000 | 0.000 | 0.000 | 0.000 | 0.000 | 0.000 | 0.000 | 0.000 | 0.000 | 0.000 | 0.000 |
V12 | 0.131 | 0.001 | 0.041 | 0.077 | 0.039 | 0.000 | 0.000 | 0.000 | 0.000 | 0.000 | 0.000 | 0.000 | 0.000 | 0.000 |
V13 | 0.115 | 0.000 | 0.000 | 0.000 | 0.000 | 0.000 | 0.000 | 0.000 | 0.000 | 0.000 | 0.000 | 0.000 | 0.000 | 0.000 |
V14 | 0.077 | 0.000 | 0.000 | 0.000 | 0.000 | 0.000 | 0.000 | 0.000 | 0.000 | 0.000 | 0.000 | 0.000 | 0.000 | 0.000 |
V15 | 0.129 | 0.039 | 0.079 | 0.003 | 0.001 | 0.000 | 0.000 | 0.000 | 0.000 | 0.000 | 0.000 | 0.000 | 0.000 | 0.000 |
V21 | 0.000 | 0.000 | 0.000 | 0.000 | 0.000 | 0.077 | 0.124 | 0.214 | 0.218 | 0.000 | 0.000 | 0.048 | 0.000 | 0.000 |
V22 | 0.000 | 0.000 | 0.000 | 0.000 | 0.000 | 0.219 | 0.058 | 0.191 | 0.226 | 0.000 | 0.000 | 0.154 | 0.000 | 0.000 |
V23 | 0.000 | 0.000 | 0.000 | 0.000 | 0.000 | 0.175 | 0.120 | 0.073 | 0.210 | 0.000 | 0.000 | 0.113 | 0.000 | 0.000 |
V24 | 0.000 | 0.000 | 0.000 | 0.000 | 0.000 | 0.218 | 0.160 | 0.220 | 0.092 | 0.000 | 0.000 | 0.119 | 0.000 | 0.000 |
V31 | 0.000 | 0.000 | 0.000 | 0.000 | 0.000 | 0.239 | 0.203 | 0.234 | 0.241 | 0.009 | 0.174 | 0.261 | 0.123 | 0.151 |
V32 | 0.000 | 0.000 | 0.000 | 0.000 | 0.000 | 0.231 | 0.137 | 0.199 | 0.204 | 0.003 | 0.006 | 0.208 | 0.039 | 0.121 |
V33 | 0.000 | 0.000 | 0.000 | 0.000 | 0.000 | 0.000 | 0.000 | 0.000 | 0.000 | 0.000 | 0.000 | 0.000 | 0.000 | 0.000 |
V34 | 0.000 | 0.000 | 0.000 | 0.000 | 0.000 | 0.071 | 0.118 | 0.063 | 0.067 | 0.078 | 0.168 | 0.218 | 0.015 | 0.146 |
V35 | 0.000 | 0.000 | 0.000 | 0.000 | 0.000 | 0.000 | 0.000 | 0.000 | 0.000 | 0.000 | 0.000 | 0.000 | 0.000 | 0.000 |
Indicators | Impact Degree A | Influenced degree B | Centrality M | Cause Degree U |
V11 | 0.000 | 0.452 | 0.452 | -0.452 |
V12 | 0.289 | 0.040 | 0.329 | 0.249 |
V13 | 0.115 | 0.120 | 0.235 | -0.005 |
V14 | 0.077 | 0.080 | 0.157 | -0.003 |
V15 | 0.251 | 0.040 | 0.291 | 0.211 |
V21 | 0.681 | 1.229 | 1.910 | -0.549 |
V22 | 0.847 | 0.921 | 1.768 | -0.074 |
V23 | 0.691 | 1.193 | 1.885 | -0.502 |
V24 | 0.809 | 1.257 | 2.066 | -0.449 |
V31 | 1.635 | 0.091 | 1.726 | 1.544 |
V32 | 1.149 | 0.349 | 1.497 | 0.800 |
V33 | 0.000 | 1.121 | 1.121 | -1.121 |
V34 | 0.945 | 0.178 | 1.122 | 0.767 |
V35 | 0.000 | 0.417 | 0.417 | -0.417 |
Indicators | Weighting | Indicators | Weighting |
V11 | 0.037 | V23 | 0.114 |
V12 | 0.024 | V24 | 0.123 |
V13 | 0.014 | V31 | 0.135 |
V14 | 0.009 | V32 | 0.099 |
V15 | 0.021 | V33 | 0.092 |
V21 | 0.116 | V34 | 0.079 |
V22 | 0.103 | V35 | 0.034 |
Indicators | Expert Group 1 | Expert Group 2 | Expert Group 3 | ||||||
A1 | A2 | A3 | A1 | A2 | A3 | A1 | A2 | A3 | |
V11 | G | VG | VG | VG | VG | VG | MG | MG | MG |
V12 | MP | MG | M | M | G | MG | M | VG | G |
V13 | MG | MG | MG | G | G | G | M | M | M |
V14 | M | MP | MG | MP | P | M | M | M | MG |
V15 | M | G | M | G | G | MG | M | M | MP |
V21 | G | MG | VG | G | G | VG | M | MG | G |
V22 | VG | MG | VG | VG | G | VG | G | M | G |
V23 | G | MG | G | MG | M | MG | MG | MG | G |
V24 | G | MG | G | MG | M | G | G | M | G |
V31 | MH | MH | M | H | H | MP | MH | MH | M |
V32 | ML | ML | L | MH | ML | ML | MH | M | MH |
V33 | MH | ML | ML | H | H | MH | MH | MH | M |
V34 | L | ML | L | L | ML | L | L | ML | L |
V35 | L | L | L | M | M | M | ML | ML | ML |
Indicators | A1 | A2 | A3 |
V11 | (0.55, 0.30, 0.15) | (0.57, 0.26, 0.17) | (0.57, 0.26, 0.17) |
V12 | (0.39, 0.58, 0.03) | (0.56, 0.29, 0.15) | (0.48, 0.41, 0.11) |
V13 | (0.48, 0.43, 0.09) | (0.48, 0.43, 0.09) | (0.48, 0.43, 0.09) |
V14 | (0.38, 0.58, 0.04) | (0.31, 0.62, 0.07) | (0.43, 0.48, 0.09) |
V15 | (0.48, 0.46, 0.06) | (0.51, 0.40, 0.10) | (0.38, 0.52, 0.10) |
V21 | (0.51, 0.40, 0.10) | (0.48, 0.39, 0.13) | (0.61, 0.23, 0.16) |
V22 | (0.61, 0.23, 0.16) | (0.48, 0.43, 0.09) | (0.61, 0.23, 0.16) |
V23 | (0.47, 0.40, 0.13) | (0.43, 0.48, 0.09) | (0.51, 0.36, 0.13) |
V24 | (0.51, 0.36, 0.13) | (0.43, 0.53, 0.04) | (0.55, 0.32, 0.13) |
V31 | (0.26, 0.62, 0.12) | (0.26, 0.62, 0.12) | (0.38, 0.58, 0.04) |
V32 | (0.33, 0.54, 0.13) | (0.48, 0.43, 0.09) | (0.42, 0.45, 0.13) |
V33 | (0.26, 0.62, 0.12) | (0.30, 0.58, 0.13) | (0.38, 0.54, 0.08) |
V34 | (0.55, 0.32, 0.13) | (0.43, 0.43, 0.13) | (0.55, 0.32, 0.13) |
V35 | (0.46, 0.45, 0.09) | (0.46, 0.45, 0.09) | (0.46, 0.45, 0.09) |
Alternative sites | S | Rank | R | Rank | Q | Rank |
A1 | 0.5630 | 2 | 0.1001 | 2 | 0.8717 | 2 |
A2 | 0.5835 | 3 | 0.1001 | 2 | 1 | 3 |
A3 | 0.5036 | 1 | 0.0838 | 1 | 0 | 1 |
φ | Q1 | Q2 | Q3 |
φ=0 | 1 | 1 | 0 |
φ=0.1 | 0.9743 | 1 | 0 |
φ=0.2 | 0.9487 | 1 | 0 |
φ=0.3 | 0.9230 | 1 | 0 |
φ=0.4 | 0.8974 | 1 | 0 |
φ=0.5 | 0.8717 | 1 | 0 |
φ=0.6 | 0.8461 | 1 | 0 |
φ=0.7 | 0.8204 | 1 | 0 |
φ=0.8 | 0.7947 | 1 | 0 |
φ=0.9 | 0.7691 | 1 | 0 |
φ=1 | 0.7434 | 1 | 0 |
Number of perturbations | Q1 | Q2 | Q3 | Number of perturbations | Q1 | Q2 | Q3 |
1 | 0.8689 | 1 | 0 | 15 | 0.8780 | 1 | 0 |
2 | 0.8609 | 1 | 0 | 16 | 0.8953 | 1 | 0 |
3 | 0.8718 | 1 | 0 | 17 | 0.8718 | 1 | 0 |
4 | 0.8728 | 1 | 0 | 18 | 0.8698 | 1 | 0 |
5 | 0.8889 | 1 | 0 | 19 | 0.8716 | 1 | 0 |
6 | 0.8688 | 1 | 0 | 20 | 0.8772 | 1 | 0 |
7 | 0.9078 | 1 | 0 | 21 | 0.8070 | 1 | 0 |
8 | 0.8776 | 1 | 0 | 22 | 0.8602 | 1 | 0 |
9 | 0.8946 | 1 | 0 | 23 | 0.8308 | 1 | 0 |
10 | 0.7841 | 1 | 0 | 24 | 0.8998 | 1 | 0 |
11 | 0.8403 | 1 | 0 | 25 | 0.9486 | 1 | 0 |
12 | 0.8520 | 1 | 0 | 26 | 0.9111 | 1 | 0 |
13 | 0.8950 | 1 | 0 | 27 | 0.8279 | 1 | 0 |
14 | 0.8718 | 1 | 0 | 28 | 0.8718 | 1 | 0 |