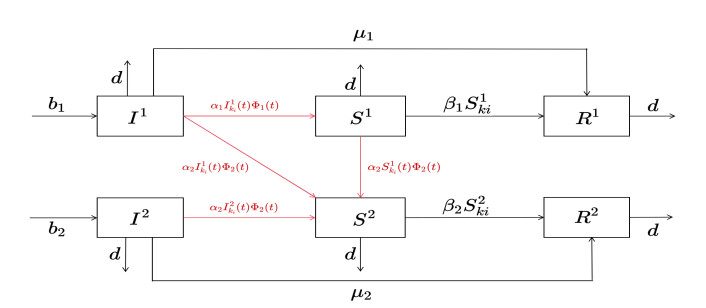
This paper investigates rumor propagation in a multilingual environment, taking into account language usage variations. Firstly, a 2I2S2R model is proposed within a heterogeneous network framework that incorporates both immunologic and cross-transmitted mechanisms. Secondly, the paper calculates the basic reproduction number R0 by the next-generation matrix method. Thirdly, the local asymptotic stability and the global asymptotic stability are further explored, which indicate that whether the rumor continuously spreads or becomes extinct is determined by the threshold. Finally, the numerical simulation and sensitivity analysis are given to illustrate the effectiveness of theoretical results and the influence of model parameters on rumor spreading.
Citation: Liuqin Huang, Jinling Wang, Jiarong Li, Tianlong Ma. Analysis of rumor spreading with different usage ranges in a multilingual environment[J]. AIMS Mathematics, 2024, 9(9): 24018-24038. doi: 10.3934/math.20241168
[1] | Hongshuang Wang, Sida Kang, Yuhan Hu . Dynamic analysis and optimal control of rumor propagation models considering different education levels and hesitation mechanisms. AIMS Mathematics, 2024, 9(8): 20089-20117. doi: 10.3934/math.2024979 |
[2] | Yanchao Liu, Pengzhou Zhang, Deyu Li, Junpeng Gong . Dynamic analysis and optimum control of a rumor spreading model with multivariate gatekeepers. AIMS Mathematics, 2024, 9(11): 31658-31678. doi: 10.3934/math.20241522 |
[3] | Xintong Wang, Sida Kang, Yuhan Hu . Dynamic analysis of a rumor propagation model considering individual identification ability. AIMS Mathematics, 2025, 10(2): 2295-2320. doi: 10.3934/math.2025107 |
[4] | Xuefeng Yue, Weiwei Zhu . The dynamics and control of an ISCRM fractional-order rumor propagation model containing media reports. AIMS Mathematics, 2024, 9(4): 9721-9745. doi: 10.3934/math.2024476 |
[5] | Hui Wang, Shuzhen Yu, Haijun Jiang . Rumor model on social networks contemplating self-awareness and saturated transmission rate. AIMS Mathematics, 2024, 9(9): 25513-25531. doi: 10.3934/math.20241246 |
[6] | Ying Yu, Jiaomin Liu, Jiadong Ren, Qian Wang, Cuiyi Xiao . Minimize the impact of rumors by optimizing the control of comments on the complex network. AIMS Mathematics, 2021, 6(6): 6140-6159. doi: 10.3934/math.2021360 |
[7] | Chaodong Chen, Dapeng Gao, Peng Guo . The stationary distribution of a stochastic rumor spreading model. AIMS Mathematics, 2021, 6(2): 1234-1248. doi: 10.3934/math.2021076 |
[8] | Rongrong Yin, Ahmadjan Muhammadhaji . Dynamics in a delayed rumor propagation model with logistic growth and saturation incidence. AIMS Mathematics, 2024, 9(2): 4962-4989. doi: 10.3934/math.2024241 |
[9] | Saumen Barua, Mahmoud A. Ibrahim, Attila Dénes . A compartmental model for the spread of Nipah virus in a periodic environment. AIMS Mathematics, 2023, 8(12): 29604-29627. doi: 10.3934/math.20231516 |
[10] | Ramsha Shafqat, Ateq Alsaadi . Artificial neural networks for stability analysis and simulation of delayed rabies spread models. AIMS Mathematics, 2024, 9(12): 33495-33531. doi: 10.3934/math.20241599 |
This paper investigates rumor propagation in a multilingual environment, taking into account language usage variations. Firstly, a 2I2S2R model is proposed within a heterogeneous network framework that incorporates both immunologic and cross-transmitted mechanisms. Secondly, the paper calculates the basic reproduction number R0 by the next-generation matrix method. Thirdly, the local asymptotic stability and the global asymptotic stability are further explored, which indicate that whether the rumor continuously spreads or becomes extinct is determined by the threshold. Finally, the numerical simulation and sensitivity analysis are given to illustrate the effectiveness of theoretical results and the influence of model parameters on rumor spreading.
Recently, the dissemination of rumors has invariably harmed society. Rumors often conceal the truth and cause people to be misled and deceived, which may affect people's judgment and decision-making ability. What is more serious is that rumors often spread unreliable information, creating panic and chaos, which poses a great threat to social stability and security. With the development of social networks, rumors spread more rapidly and widely, so the issue of rumor propagation needs to be given more attention. Hence, understanding the patterns and traits of rumor dissemination empowers governments and individuals to effectively implement appropriate measures, steer public opinion, and safeguard social stability.
Research on rumor propagation has been underway since the last century. In 1965, Daley and Kendall [1] established a connection between rumors and epidemics, introducing the classical DK rumor-spreading model. Subsequently, numerous scholars have identified increasing distinctions between rumor propagation and epidemic transmission, leading to the development of various rumor propagation models, such as, the SIR model [2], the SIHR model [3], the stochastic model [4], the diffusion model [5], and so on [6,7,8,9]. Concretely, in reference [2], an enhanced rumor propagation model based on the SIR model was utilized to investigate the rumor issue in complex social networks. Reference [3] introduced a SIHR model, which considered the interplay between forgetting and memory mechanisms. Additionally, an extended rumor spreading model incorporating knowledge education was presented in [6]. Notably, the above research is conducted on the basis of homogeneous networks.
In a homogeneous network, all nodes belong to the same type and share similar features or attributes. However, the applicability of the above studies is limited, as it is challenging to find a social network where all users have the same degree of reality. Especially with the increasing development of social networks, users with different characteristics or attributes are more easily accessible[10,11,12]. Hence, more research on the spread of rumors on heterogeneous networks has begun to emerge[14,15,16]. Some heterogeneous network models were introduced to analyze the dynamic behaviors of the rumor propagation [17,18,19,20]. For example, an IFCD model was addressed, which took full consideration of the heterogeneity of network users and stochastic disturbances in the network environment [11]. In references [16,19], some delay rumor propagation models were proposed to study its stability and bifurcation occurs in heterogeneous networks. Anti-rumor mechanism was introduced to control rumor spreading in heterogeneous networks [17]. The dynamical behaviors and control of rumor propagation model incorporating delay were investigated under a heterogeneous social networks [20].
Notably, most research on rumor dissemination focuses on a single-language environment. However, in recent years, particularly in multi-ethnic regions of China, the escalation of social issues due to rumors has underscored the growing significance of studying rumor propagation in multilingual settings. This is related to social stability and national unity. At present, some scholars have redirected their attention towards examining rumor dissemination in multilingual environments, such as, some extended multi-lingual SIR rumor spreading models that were proposed to delve into its dynamical behaviors and control strategies [21,22]. However, the above research landscape on multilingual rumor propagation within homogeneous networks cannot achieve a comprehensive and practical study of the multilingual rumor spreading mechanism.
Subsequently, more dynamic models and control strategies were addressed to analyze the problem of multilingual rumor propagation in the heterogeneous network [6,23,24,25]. For instance, a rumor propagation model with two language spreaders was proposed to analyze its stability, which considered the network topology [23]. However, it is worth noting that the above studies, whether based on homogeneous or heterogeneous networks [21,22,23], mostly treat multiple languages as equally prevalent, assuming consistent usage across all languages. While this approach may be suitable for some situations, it does not reflect the reality of many multilingual areas. For example, in China, Mandarin is widely spoken, but proficiency in other languages is limited, which results in networks with varying language hierarchies. Motivated by these analyses, the language usage variations are taken into account in this paper, and a novel rumor propagation model tailored to such asymmetric language networks is introduced to address the complexities of real-world multilingual settings.
Based on the mechanism described above, this paper focuses on studying the global stability of a multilingual SIR rumor propagation model with unidirectional propagation patterns between different groups. The main contributions of this paper include the following aspects:
1) Compared to existing results [21,22,23], this paper explores the impact of language usage scope, considers the unidirectional transmission relationship between two language groups, which can fill a gap in the dissemination of multilingual rumors to a certain extent.
2) Based on a one-way propagation relationship in a multilingual environment, a 2I2S2R model is proposed to analyze the dynamics of multilingual rumor propagation by applying the Lyapunov function and the
3) In the numerical simulations, the sensitivity analysis of the basic reproduction number is addressed to further illustrate the influence of model parameters on the process of rumor propagation and provide control strategies for rumor suppression.
Inspired by these analyses, the paper is organized as follows: The network model is introduced in Section 2. The existence and stability of equilibrium solutions are shown in Section 3. Some numerical examples are further addressed to demonstrate the validity of Section 4. The conclusions are given in Section 5.
In this section, we construct a SIR multilingual rumor propagation model. Six states are proposed to indicate the different statuses of the rumor-spreading process. Ignorants-1 (I1(t)) and Ignorants-2 (I2(t)) represent the people who do not know the rumor. Language-1 is the one with a smaller usage range, while Language-2 has a larger one. In our hypothetical environment, people who can speak Language-1 will definitely speak Language-2. Spreaders-1 (S1(t)) represent the people who know Rumor-1, and Spreaders-2 (S2(t)) represent those who know Rumor-2. Removers-1 (R1(t)) and Removers-2 (R2(t)) mean the people who know Rumor-1 and Rumor-2, respectively, but have not spread them. Rumor-1 and Rumor-2 are the same rumor, which are popular in the environments of Language-1 and Language-2, respectively. I1(t), S1(t), and R1(t) form Group-1, and Group-2 consists of I2(t), S2(t), and R2(t). People in Group-1 can speak both Language-1 and Language-2, so people from Group-1 will have a certain probability of transferring to Group-2. The people who are originally in Group-2 can only speak Language-2, for which people in Group-2 will not transfer to Group-1. Next, the state transition is depicted in Figure 1 with the following rules, and the meaning of main parameters is shown in Table 1.
Parameters | Meaning |
bv | Coming rate of the group v |
d | Leaving rate of the different compartment |
αv | Rumor conversion rate (i.e., cross transmission showed in Figure 1) |
βv | Probability of Svki cured and transformed into Rvki |
μv | Immunity rate of Ignorants against rumors |
⟨k⟩ | The average degree |
θ(ki) | Infectivity of a user with degree ki |
Φv(t) | The probabilities that an ignorant whether in Group-1 or Group-2 gets in touch with spreaders |
1) A user changes state I1 to state Sv by believing the rumor with different possible routes of transmission (v=1,2). An ignorant I1 becomes Sv(v=1,2) with rumor conversion rate αv(v=1,2), and I1 is connected to one or more Sv(v=1,2) with probability Φv(t)(v=1,2) at time t. Hence, the infected probability of I1 becoming S1 (or S2) is α1I1ki(t)Φ1(t) (or α2I1ki(t)Φ2(t)), respectively.
2) An ignorant I2 becomes S2 with rumor conversion rate α2, and is connected to one or more S2 with probability Φ2(t). Additionally, S1 can also become S2 with probability α2S1ki(t)Φ2(t).
3) In this process of rumor propagation, the population enters each group in a ratio of bv(v=1,2). The proportion of users leaving the system in each group is d. I1 and I2 can both be directly converted into R1 and R2 with probabilities of μ1 and μ2, respectively.
From the above analysis, the 2I2S2R rumor propagation is described as follows:
{dI1ki(t)dt= b1−α1I1ki(t)Φ1(t)−α2I1ki(t)Φ2(t)−(μ1+d)I1ki(t),dI2ki(t)dt= b2−α2I2ki(t)Φ2(t)−(μ2+d)I2ki(t),dS1ki(t)dt= α1I1ki(t)Φ1(t)−α2S1ki(t)Φ2(t)−(β1+d)S1ki(t),dS2ki(t)dt= α2[I1ki(t)+I2ki(t)+S1ki(t)]Φ2(t)−(β2+d)S2ki(t),dR1ki(t)dt= μ1I1ki(t)+β1S1ki(t)−dR1ki(t),dR2ki(t)dt= μ2I2ki(t)+β2S2ki(t)−dR2ki(t), | (2.1) |
where Φv(t) represents the probabilities that an ignorant person whether in Group-1 or Group-2 gets in touch with spreaders, and Φv(t) is defined as
Φv(t)=∑ni=1θ(ki)Z(ki)Svki(t)⟨k⟩, v=1,2. | (2.2) |
It represents the contact rate between group Sv and another group. Z(ki) is defined as the ratio of people with degree ki to all users in the network; therefore, ∑ni=1Z(ki)=1 and ⟨k⟩=∑ni=1kiZ(ki) measures the average degree. The active nodes in the network satisfy I1ki(t)+I2ki(t)+S1ki(t)+S2ki(t)+R1ki(t)+R2ki(t)=1 and d=b1+b2.
Remark 1. In contrast to references[2,3,21], both the multilingual environment and homogeneous networks are considered in constructing the rumor propagation model (2.1), which is more in line with the actual social networks. Besides, it is note worthy that θ(ki) has several cases, such as, θ(ki) is a constant [26], θ(ki)=ki [27], or θ(ki) being a nonlinear function [28]. Here, we select θ(ki)=kpi/(1+kqi) since a larger degree yields larger infectivity in practice.
In this section, we will calculate equilibrium solutions, including the zero-equilibrium solutions and positive-equilibrium solutions, and analyze their existence and properties. To begin with, the next-generation matrix method [29] is used to calculate the basic reproduction number of the model (2.1).
Let
ψ=(S1ki(t),S2ki(t),I1ki(t),I2ki(t),R1ki(t),R2ki(t)). |
Then model (2.1) can be written as
dψdt=F(ψ)−V(ψ), |
where
F(ψ)=(α1I1ki(t)Φ1(t)α2(I1ki(t)+I2ki(t)+S1ki(t))Φ2(t)0000), |
V(ψ)=(α2S1ki(t)Φ2(t)+(β1+d)S1ki(t)(β2+d)S2ki(t)−b1+α1I1ki(t)Φ1(t)+α2I1ki(t)Φ2(t)+(μ1+d)I1ki(t)−b2+α2I2ki(t)Φ2(t)+(μ2+d)I2ki(t)−μ1I1ki(t)−β1S1ki(t)+dR1ki(t)−μ2I2ki(t)−β2S2ki(t)+dR2ki(t)). |
Obviously, the zero-equilibrium solution is
E0={(b1d+μ1,b2d+μ2,0,0,μ1b1d(μ1+d),μ2b2d(μ2+d)),⋯,(b1d+μ1,b2d+μ2,0,0,μ1b1d(μ1+d),μ2b2d(μ2+d))}, |
and the Jacobian matrices of F(ψ) and V(ψ) at E0 are
DF(E0)=(F00000000),DV(E0)=(V00J1J20J3J4J5), |
where F=(F100F2), V=(V100V2), Vi=diag(βi+d)n×n,i=1,2 and
F1=(α1I1k1(t)θ(k1)Z(k1)⟨k⟩α1I1k1(t)θ(k2)Z(k2)⟨k⟩⋯α1I1k1(t)θ(kn)Z(kn)⟨k⟩α1I1k2(t)θ(k1)Z(k1)⟨k⟩α1I1k2(t)θ(k2)Z(k2)⟨k⟩⋯α1I1k2(t)θ(kn)Z(kn)⟨k⟩⋮⋮⋱⋮α1I1kn(t)θ(k1)Z(k1)⟨k⟩α1I1kn(t)θ(k2)Z(k2)⟨k⟩⋯α1I1kn(t)θ(kn)Z(kn)⟨k⟩). |
Next, according to the elementary transformation of a matrix, one has
F1→1⟨k⟩(α1∑ni=1θ(ki)Z(ki)I1ki(t)α1I1k1(t)θ(k2)Z(k2) ⋯ α1I1k1(t)θ(kn)Z(kn)00 ⋯ 0⋮⋮⋮⋮00 ⋯ 0). |
Similarly, it follows
F2→1⟨k⟩(α2∑ni=1θ(ki)Z(ki)(I1ki(t)+I2ki(t)+S1ki(t)) ⋯⋯ ⋯00⋯0⋮⋮⋮⋮00⋯0). |
Hence, the basic reproduction number R0 of the model is computed as
R0=ρ(FV−1)=max{R01,R02}, |
where
R01=α1b1n∑i=1θ(ki)Z(ki)⟨k⟩(β1+d)(μ1+d),R02=α2(b1d+μ1+b2d+μ2)n∑i=1θ(ki)Z(ki)⟨k⟩(β2+d). |
Theorem 3.1. For the basic reproduction number of the model (2.1), the equilibrium solution is unique in the following three different cases:
(ⅰ) If R0<1, model (2.1) only has a zero-equilibrium solution.
(ⅱ) If R01<1 and R02>1, model (2.1) has a unique positive-equilibrium solution.
(ⅲ) If R01>1 and R02>1, model (2.1) has a unique positive-equilibrium solution.
Proof. (ⅰ) Obviously, when R0<1, model (2.1) only has a zero-equilibrium solution, E0.
(ⅱ) When R01<1,R02>1, define the solution as E∗1, i.e.,
E∗1=(I1∗1ki,I2∗1ki,S1∗1ki,S2∗1ki,R1∗1ki,R2∗1ki), |
where
S1∗1ki=0,I1∗1ki=b1α2Φ∗2+μ1+d,I2∗1ki=b2α2Φ∗2+μ2+d, |
S2∗1ki=α2(I1∗1ki+I2∗1ki)Φ∗2β2+d,R1∗1ki=μ1I1∗1kid,R2∗1ki=μ2I2∗1ki+β2S2∗1kid. |
It is obviously that Φ∗1=0, and
Φ∗2=1⟨k⟩n∑i=1θ(ki)Z(ki)α2(b1α2Φ∗2+μ1+d+b2α2Φ∗2+μ2+d)β2+dΦ∗2. | (3.1) |
Further, we set
G1(Φ∗2)=1−1⟨k⟩n∑i=1θ(ki)Z(ki)α2(b1α2Φ∗2+μ1+d+b2α2Φ∗2+μ2+d)β2+d, |
and it yields that to any Φ∗2>0, G′1(Φ∗2)>0 and G′1(+∞)=1. Then, we have
limΦ∗2→0+G1(Φ∗2)=1−1⟨k⟩n∑i=1θ(ki)Z(ki)α2(b1μ1+d+b2μ2+d)β2+d=1−R02≤0. |
It proves that model (2.1) has a unique equilibrium solution when R01<1,R02>1.
(ⅲ) When R01>1,R02>1, define the solution as E∗2,
E∗2=(I1∗2ki,I2∗2ki,S1∗2ki,S2∗2ki,R1∗2ki,R2∗2ki), |
where
I1∗2ki=b1α1Φ∗1+α2Φ∗2+μ1+d,I2∗2ki=b2α2Φ∗2+μ2+d,S1∗2ki=α1I1∗2kiΦ∗1α2Φ∗2+β1+d,S2∗2ki=α2(I1∗2ki+I2∗2ki+S1∗2ki)Φ∗2β2+d,R1∗2ki=μ1I1∗2ki+β1∗1S1∗2kid,R2∗2ki=μ2I2∗2ki+β2S2∗2kid. |
Next, expanding S1∗2ki and S2∗2ki, we obtain
S1∗2ki = α1b1Φ∗1(α2Φ∗2+β1+d)(α1Φ∗1+α2Φ∗2+μ1+d) |
and
S2∗2ki=α2Φ∗2β2+d[b1α1Φ∗1+α2Φ∗2+μ1+d+b2α2Φ∗2+μ2+d+b1α1Φ∗1(α1Φ∗1+α2Φ∗2+μ1+d)(α2Φ∗2+μ2+d)]. |
Similarly to case (ⅱ), one has
Φ∗1=1⟨k⟩n∑i=1θ(ki)Z(ki)S1∗2ki=1⟨k⟩n∑i=1θ(ki)Z(ki)α1b1Φ∗1(α2Φ∗2+β1+d)(α1Φ∗1+α2Φ∗2+μ1+d),Φ∗2=1⟨k⟩n∑i=1θ(ki)Z(ki)α2Φ∗2β2+d[b1α1Φ∗1+α2Φ∗2+μ1+d+b2α2Φ∗2+μ2+d+b1α1Φ∗1(α1Φ∗1+α2Φ∗2+μ1+d)(α2Φ∗2+μ2+d)].G2(Φ∗1,Φ∗2)=1−1⟨k⟩n∑i=1θ(ki)Z(ki)α1b1(α2Φ∗2+β1+d)(α1Φ∗1+α2Φ∗2+μ1+d), |
and
G3(Φ∗1,Φ∗2)=1−1⟨k⟩n∑i=1θ(ki)Z(ki)α2β2+d[b1α1Φ∗1+α2Φ∗2+μ1+d+b2α2Φ∗2+μ2+d+b1α1Φ∗1(α1Φ∗1+α2Φ∗2+μ1+d)(α2Φ∗2+μ2+d)]. |
It is easy to obtain that for all Φ∗1,
∂G2(Φ∗1,Φ∗2)∂Φ∗1>0,limΦ∗1→+∞G2(Φ∗1,Φ∗2)=1,∂G3(Φ∗1,Φ∗2)∂Φ∗2>0,limΦ∗2→+∞G3(Φ∗1,Φ∗2)=1. |
Moreover, it shows that
limΦ∗1→0+G2(Φ∗1,0)=1−1⟨k⟩n∑i=1θ(ki)Z(ki)α1b1(β1+d)(μ1+d)=1−R01<0,limΦ∗2→0+G3(0,Φ∗2)=1−1⟨k⟩n∑i=1θ(ki)Z(ki)α2β2+d[b1μ1+d+b2μ2+d]=1−R02<0. |
Hence, model (2.1) has a unique positive-equilibrium solution if R01>1 and R02>1.
Theorem 3.2. The zero-equilibrium solution E0 of the model (2.1) is locally asymptotically stable if R0<1.
Proof. According to stability theory [30], we first derive the Jacobin matrix J(E0)
J(E0)=(A11A12⋯A1nA21A22⋯A2n⋮⋮⋱⋮An1An2⋯Ann), | (3.2) |
where
f1=α1b1θ(ki)Z(ki)⟨k⟩(d+μ1)−β1−d,f2=α2θ(ki)Z(ki)⟨k⟩(b1d+μ1+b2d+μ2)−β2−d, |
and
Aii=(f1000000f20000−α1b1θ(ki)Z(ki)⟨k⟩(d+μ1)−α2b1θ(ki)Z(ki)⟨k⟩(d+μ1)−μ1−d0000−α2b2θ(ki)Z(ki)⟨k⟩(d+μ2)0−μ2−d00β10μ10−d00β20μ20−d), |
Aij=(α1b1θ(ki)Z(ki)⟨k⟩(d+μ1)000000α2θ(ki)Z(ki)⟨k⟩(b1d+μ1+b2d+μ2)0000−α1b1θ(ki)Z(ki)⟨k⟩(d+μ1)−α2b1θ(ki)Z(ki)⟨k⟩(d+μ1)00000−α2b2θ(ki)Z(ki)⟨k⟩(d+μ2)0000000000000000). |
Further, we have
|λ−J(E0)|=(λ+β1+d)n−1(λ+β2+d)n−1(λ+μ1+d)2n(λ+μ2+d)2n×[λ+(β1+d)(1−1⟨k⟩n∑i=1θ(ki)Z(ki)α1b1(β1+d)(μ1+d))]×(λ+(β2+d)[1−1⟨k⟩n∑i=1θ(ki)Z(ki)α2β2+d(b1μ1+d+b2μ2+d)]). |
Then, the eigenvalues of (3.2) are λ1=λ2=⋯=λn−1=−(β1+d), λn=λn+1=⋯=λ2n−2=−(β2+d), λ2n−1=λ2n=⋯=λ4n−2=−(μ1+d), λ4n−1=λ4n=⋯=λ6n−2=−(μ2+d), λ6n−1=−(β1+d))(1−R01), λ6n=−(β2+d))(1−R02). Since R0<1, it is obvious that for any i from 1 to 6n, λi<0. Therefore, the zero-equilibrium solution E0 is locally asymptotically stable in model (2.1).
Theorem 3.3. The zero-equilibrium solution E∗0 of model (2.1) is globally asymptotically stable if R0<1.
Proof. According to model (2.1) and [31], one has
dI1ki(t)dt=b1−α1I1ki(t)Φ1(t)−α2I1ki(t)Φ2(t)−(μ1+d)I1ki(t)≤b1−(μ1+d)I1ki(t), | (3.3) |
which means that supI1ki(t)≤b1μ1+d=˜I1.
Next, amuse ϵ1>0 that is sufficiently small. So for t→+∞, we have
supI1ki(t)≤˜I1+ϵ1, |
and
dS1ki(t)dt≤α1Φ1(t)(˜I1+ϵ1)−(d+β1)S1ki(t). |
From the principle of comparison, we set a new function Q1ki(t), Q1ki(0)=S1ki(0)=0 and
dQ1ki(t)dt=α1˜Φ1(t)(˜I1+ϵ1)−(d+β1)Q1ki(t), |
where
˜Φ1(t)=1⟨k⟩n∑i=1θ(ki)Z(ki)Q1ki(t). |
Construct a Lyapunov function
V1(t)=1⟨k⟩n∑i=1θ(ki)Z(ki)Q1ki(t). | (3.4) |
Then, it derives that
dV1(t)dt=1⟨k⟩n∑i=1θ(ki)Z(ki)[α1~Φ1(t)(~I1+ϵ1)−(d+β1)Q1ki(t)]=˜Φ1(t)(β1+d)[R01+1⟨k⟩n∑i=1θ(ki)Z(ki)α1ϵ1β1+d−1]. |
Since R01<1 and the definition of ϵ1, we select a small enough ϵ1, so we can obtain that dV1(t)dt≤0. Similarly, one has
dI2ki(t)dt≤b2−(μ2+d)I2ki(t), | (3.5) |
and supI2ki(t)≤b2μ2+d=˜I2. Besides, construct a small enough ϵ2>0, I2ki(t)≤˜I2+ϵ2, 0<S1ki(t)<ϵ2. Hence, it follows that
dS2ki(t)dt≤α2Φ2(t)(~I1+ϵ1+~I2+ϵ2)−(d+β2)S2ki(t). | (3.6) |
Then we set a new function, Q2ki(t), which satisfies
Q2ki(0)=S2ki(0)=0, dQ2ki(t)dt=α2˜Φ2(t)(˜I1+ϵ1+˜I2+ϵ2)−(d+β2)Q2ki(t), |
where
˜Φ2(t)=∑ni=1θ(ki)Z(ki)Q2ki(t)⟨k⟩. |
Similarly, construct a Lyapunov function
V2(t)=1⟨k⟩n∑i=1θ(ki)Z(ki)Q2ki(t). |
Then, one has
dV2(t)dt=1⟨k⟩n∑i=1θ(ki)Z(ki)[α2˜Φ2(t)(~I1+ϵ1+~I2+ϵ2)−(d+β2)Q2ki(t)]=˜Φ2(t)(β2+d)[1⟨k⟩n∑i=1θ(ki)Z(ki)α2(~I1+ϵ1+~I2+ϵ2)β2+d−1]=˜Φ2(t)(β2+d)[α2(b1d+μ1+b2d+μ2)n∑i=1θ(ki)Z(ki)⟨k⟩(β2+d)+1⟨k⟩n∑i=1θ(ki)Z(ki)α2(ϵ1+ϵ2)β2+d−1]=˜Φ2(t)(β2+d)[R02+1⟨k⟩n∑i=1θ(ki)Z(ki)α2(ϵ1+ϵ2)β2+d−1]. |
Since R02<1 and the definition of ϵ1 and ϵ2, select small enough ϵ1 and ϵ2. So we can obtain that dV2(t)dt≤0. Similarly, we construct a small enough ϵ3>0, for t→+∞ , 0<S1ki(t)<ϵ3, 0<S2ki(t)<ϵ3.
Hence,
dI1ki(t)dt≥b1−[(α1+α2)∑ni=1θ(ki)Z(ki)ϵ3⟨k⟩+μ1+d]I1ki(t). |
Then,
infI1ki(t)≤b1(α1+α2)∑ni=1θ(ki)Z(ki)ϵ3⟨k⟩+μ1+d=˜I1. |
Set ϵ3→0, so it follows infI1ki(t)≤b1μ1+d=supT1ki(t) for t→+∞. Hence, E∗0 is globally asymptotically stable if R0<1.
Lemma 3.4. If R01<1 and R02>1, the positive-equilibrium solution of model (2.1) satisfies S1ki(t)=S1∗1ki=Φ1(t)=Φ∗1=0 when t→+∞,
S2∗1ki=α2(I1∗1ki+I2∗1ki)Φ∗2β2+d→β2+d=α2(I1∗1ki+I2∗1ki)Φ∗2S2∗1ki=1⟨k⟩n∑i=1θ(ki)Z(ki)α2(I1∗1ki+I2∗1ki). | (3.7) |
Proof. From the definition of Φ1(t), there is
˙Φ1(t)≤1⟨k⟩n∑i=1θ(ki)Z(ki)[α1I1ki(t)Φ1(t)−(β1+d)S1ki(t)]≤1⟨k⟩n∑i=1θ(ki)Z(ki)[α1b1Φ1(t)μ1+d−(β1+d)S1ki(t)]=Φ1(t)(β1+d)(R01−1)<0. |
which means that (3.7) holds based on reference[32].
Theorem 3.5. The positive-equilibrium solution E∗1 of model (2.1) is globally asymptotically stable if R01<1 and R02>1.
Proof. Construct the Lyapunov V3(t) as
V3(t)=12⟨k⟩n∑i=11I1∗1kiθ(ki)Z(ki)(I1ki(t)−I1∗1ki)2+(Φ2(t)−Φ∗2−Φ∗2ln(Φ2(t)Φ∗2))+12⟨k⟩n∑i=11I2∗1kiθ(ki)Z(ki)(I2ki(t)−I2∗1ki)2. |
According to Lemma 3.4, it follows that
dV3(t)dt=1⟨k⟩n∑i=11I1∗1kiθ(ki)Z(ki)(I1ki(t)−I1∗1ki)˙I1ki(t)+Φ2(t)−Φ∗2Φ2(t)˙Φ2(t)+1⟨k⟩n∑i=11I2∗1kiθ(ki)Z(ki)(I2ki(t)−I2∗1ki)˙I2ki(t)=f1+f2+f3, |
where
f1=1⟨k⟩n∑i=11I1∗1kiθ(ki)Z(ki)(I1ki(t)−I1∗1ki)[α2I1∗1kiΦ∗2+(μ1+d)I1∗1ki−α2I1ki(t)Φ2(t)−(μ1+d)I1ki(t)]=1⟨k⟩n∑i=1−1I1∗1kiθ(ki)Z(ki)(I1ki(t)−I1∗1ki)[(μ1+d)(I1ki(t)−I1∗1ki)+α2Ik1i(t)Φ2(t)−α2I2∗1kiΦ2(t)+α2I2∗1kiΦ2(t)−α2I2∗1kiΦ∗2]=1⟨k⟩n∑i=1[−1I1∗1kiθ(ki)Z(ki)(α2Φ2(t)+μ1+d)(I1ki(t)−I1∗1ki)2−θ(ki)Z(ki)α2(Φ2(t)−Φ∗2)(I1ki(t)−I1∗1ki)],f2=1⟨k⟩n∑i=11I2∗1kiθ(ki)Z(ki)(I2ki(t)−I2∗1ki)(b2−α2I2ki(t)Φ2−(μ2+d)Ik2i(t))=1⟨k⟩n∑i=1[−1I2∗1kiθ(ki)Z(ki)(α2Φ2(t)+μ2+d)(I2ki(t)−I2∗1ki)2−θ(ki)Z(ki)α2(Φ2(t)−Φ∗2)(I2ki(t)−I2∗1ki)],f3=(Φ2(t)−Φ∗2)[1⟨k⟩n∑i=1θ(ki)Z(ki)α2(I1ki(t)+I2ki(t))−(β2+d)]=(Φ2(t)−Φ∗2)[1⟨k⟩n∑i=1θ(ki)Z(ki)α2(I1ki(t)+I2ki(t))−1⟨k⟩n∑i=1θ(ki)Z(ki)α2(I1∗1ki+I2∗1ki)]. |
Hence, one further has
dV3(t)dt=1⟨k⟩n∑i=1[−1I1∗1kiθ(ki)Z(ki)(α2Φ2(t)+μ1+d)(I1ki(t)−I1∗1ki)2−1I2∗1kiθ(ki)Z(ki)(α2Φ2(t)+μ2+d)(I2ki(t)−I2∗1ki)2]≤0. |
Therefore, we can conclude that the positive-equilibrium solution E∗1 of model (2.1) is globally asymptotically stable if R01<1 and R02>1.
Lemma 3.6. If R01>1 and R02>1, when t→+∞, the positive-equilibrium solution of model (2.1) satisfies
β1+d=α1I1∗2kiΦ∗1S1∗2ki−α2Φ∗2=1⟨k⟩n∑i=1α1θ(ki)Z(ki)I1∗2ki−α2Φ∗2, |
and
β2+d=α2(I1∗2ki+I2∗2ki+S1∗2ki)Φ∗2S2∗2ki=1⟨k⟩n∑i=1α2θ(ki)Z(ki)(I1∗2ki+I2∗2ki+S1∗2ki). |
Theorem 3.7. The positive-equilibrium solution E∗1 of model (2.1) is globally asymptotically stable if R01>1 and R02>1.
Proof. Construct the Lyapunov V4(t) as
V4(t)=12⟨k⟩n∑i=11I1∗2kiθ(ki)Z(ki)(I1ki(t)−I1∗2ki)2+12⟨k⟩n∑i=11I2∗2kiθ(ki)Z(ki)(I2ki(t)−I2∗2ki)2+(Φ1(t)−Φ∗1−Φ∗1ln(Φ1(t)Φ∗1))+(Φ2(t)−Φ∗2−Φ∗2ln(Φ2(t)Φ∗2)). |
Then, based on Lemma 3.6, it yields that
dV4(t)dt=1⟨k⟩n∑i=11I1∗2kiθ(ki)Z(ki)(I1ki(t)−I1∗2ki)˙I1ki(t)+Φ1(t)−Φ∗1Φ1(t)˙Φ1(t)+1⟨k⟩n∑i=11I2∗2kiθ(ki)Z(ki)(I2ki(t)−I2∗2ki)˙I2ki(t)+Φ2(t)−Φ∗2Φ2(t)˙Φ2(t)=g1+g2+g3+g4, |
where
g1=1⟨k⟩n∑i=11I1∗2kiθ(ki)Z(ki)(I1ki(t)−I1∗2ki)[α2I1∗2kiΦ∗2+α1I1∗2kiΦ∗1+(μ1+d)I1∗2ki−α2I1ki(t)Φ2(t)−α1I1ki(t)Φ1(t)−(μ1+d)I1ki(t)]=−1⟨k⟩n∑i=11I1∗2kiθ(ki)Z(ki)(α1Φ1(t)+α2Φ2(t)+μ1+d)(I1ki(t)−I1∗2ki)2−1⟨k⟩n∑i=1θ(ki)Z(ki)[α2(Φ2(t)−Φ∗2)+α1(Φ1(t)−Φ∗1)](I1ki(t)−I1∗2ki),g2=1⟨k⟩n∑i=1−1I2∗2kiθ(ki)Z(ki)(α2Φ2(t)+μ2+d)(I2ki(t)−I2∗2ki)2−1⟨k⟩n∑i=1θ(ki)Z(ki)α2(Φ2(t)−Φ∗2)(I2ki(t)−I2∗2ki),g3=(Φ1(t)−Φ∗1)1Φ1(t)1⟨k⟩n∑i=1θ(ki)Z(ki)[α1I1ki(t)Φ1(t)−α2S1ki(t)Φ2(t)−(β1+d)S1ki(t)]=(Φ1(t)−Φ∗1)[1⟨k⟩n∑i=1θ(ki)Z(ki)α1I1ki(t)−1⟨k⟩n∑i=1θ(ki)Z(ki)α1I1∗2ki+α2Φ∗2−α2Φ2(t)]=1⟨k⟩n∑i=1θ(ki)Z(ki)α1(I1ki(t)−I1∗1ki)(Φ1(t)−Φ∗1)−α2(Φ2(t)−Φ∗2)(Φ1(t)−Φ∗1),g4=(Φ2(t)−Φ∗2)1Φ2(t)1⟨k⟩n∑i=1θ(ki)Z(ki)[α2(I1ki(t)+I2ki(t)+S1ki(t))Φ2(t)−(β2+d)S2ki(t)]=(Φ2(t)−Φ∗2)1⟨k⟩n∑i=1α2θ(ki)Z(ki)[(I1ki(t)−I1∗2ki)+(I2ki(t)−I2∗2ki)]+(Φ2(t)−Φ∗2)α2(Φ1(t)−Φ∗1). |
Further, one has
dV4(t)dt=−1⟨k⟩n∑i=11I1∗2kiθ(ki)Z(ki)(α1Φ1(t)+α2Φ2(t)+μ1+d)(I1ki(t)−I1∗2ki)2−1⟨k⟩n∑i=11I2∗2kiθ(ki)Z(ki)(α2Φ2(t)+μ2+d)(I2ki(t)−I2∗2ki)2≤0. |
Therefore, we can conclude that the positive-equilibrium solution E∗2 of model (2.1) is globally asymptotically stable if R01>1 and R02>1.
Remark 2. In fact, the basic reproduction number R0 of model (2.1) is determined by the values of R01 and R02, which means that there exist more situations of rumor existence (see Theorem 3.1). Notedly, rumor spreaders S1(t) and S2(t) will be extinct with R0<1 (see Theorem 3.2), but the spreading of rumors is more complex when R0>1. Hence, to further explore its dynamics, Lyapunov function indirect and direct methods are used to analyze local asymptotically stability (see Theorem 3.2) and global asymptotically stability (see Theorems 3.3, 3.5, and 3.7), respectively.
In this section, we use numerical simulation to analyze the dynamic characteristics of the proposed rumor propagation model.
Combined with practical problems and existing results [8,33,34], the initial state of a rumor-spreading network typically comprises a predominant number of ignorant individuals, a small group of spreaders, and an even smaller contingent of removers in general. Furthermore, depending on the specific assumptions made regarding the model's context, it is often observed that the population of spreaders (Group-2) is more substantial than initially anticipated. Drawing on the insights from reference [23], suppose that the network obeys power law distribution, and choose ki=i, i=1,2,⋯,200, and Z(ki)=k−2i1.6399, so it satisfies that ∑ni=1Z(ki)=1. Hence, it is easy to obtain that the average degree ⟨k⟩=3.5844. As is mentioned above (see Remark 1), θ(ki)=kpi/(1+kqi), and select p=0.5,q=0.5. Moreover, to further demonstrate the effect of the parameters (see Figures 2–5), choose the following series of initial values for the model (2.1): I1ki(0)=0.3+ki3200, I2ki(0)=0.4+ki3200, S1ki(0)=0.1−ki3200, S2ki(0)=0.15−ki6400, R1ki(0)=0.03−ki6400, R2ki(0)=0.02−ki6400, ki=i, i=1,2,⋯,200.
Combined with Theorem 3.3 and the actual problem, choose α1=0.6,α2=0.5,b1=0.005,b2=0.005,d=0.01,μ1=0.005,μ2=0.005,β1=0.05 and β2=0.05. By simple calculation, it can deduce that R0≈0.873<1. From Theorem 3.3, we can know that the zero-equilibrium solution E∗0 of model (2.1) is globally asymptotically stable if R0<1. It is shown in Figure 2, which takes k=50 as an example.
Moreover, Figure 3 shows the dynamic state at all degrees from 1 to 200. From Figure 3, we can easily detect that I1ki(t) tends to b1μ1+d=13 and I2ki(t) tends to b2μ2+d=13, which is consistent with the conditions of Theorem 3.3. It also shows that the zero-equilibrium solution E0 is globally asymptotically stable for any k∈[1,200].
To further explore the effect of language usage variations on the multi-lingual rumor spreading, the following different parameters are selected with actual and empirical results [23,34], and two cases are explored based on Theorems 3.5 and 3.7 as follows:
Case 1. Stability of positive-equilibrium solution for R01<1 and R02>1.
Choose α1=0.4,α2=0.72,b1=0.01,b2=0.01,d=0.02,μ1=0.01,μ2=0.01,β1=0.03,β2=0.02, and it yields R01≈0.3250<1, R02≈1.4624>1. Figure 4 depicts that positive equilibrium E∗1 is globally asymptotically stable for k∈[1,200]. Notedly, S1ki(t) tends to 0 rapidly, and S2ki(t) remains prevalent thereafter.
Case 2. Stability of positive-equilibrium solution for R01>1 and R02>1.
Choose α1=0.55,α2=0.25,b1=0.003,b2=0.003,d=0.006,μ1=0.002,μ2=0.002,β1=0.0028,β2=0.005. Then, it derives that R01≈2.856>1 and R02≈2.007>1. Figure 5 shows that positive-equilibrium solution E∗2 is globally asymptotically stable for any k∈[1,200], and two cases of rumors are rare but still prevalent as time goes by.
Remark 3. Note that the stability of the zero-equilibrium solution and positive-equilibrium solutions of the model (2.1) is discussed, respectively. When R0<1, the system has a zero-equilibrium solution, and the rumor gradually disappears (see Figure 3). However, when the system has a positive-equilibrium solution, its stability is more complex. When R01<1 and R02>1, the rumor propagated in language 1 disappears, while the rumor propagated in Language-2 still prevalent (see Figure 4). Conversely, when R01>1 and R02>1, rumors propagated in both languages continue to exist over time (see Figure 5). Therefore, the study on the propagation of rumors in a multilingual environment holds significant research value.
To further analyze the different factors contribution to the rumor spreading, a sensitivity analysis is further shown here [35]. The normalized forward sensitivity index of a variable u depends differentiably on a parameter p. It is defined as:
γup:=∂u∂p×up. |
Next, in terms of the uncertainty of the basic reproductive number, we choose the parameter values from Section 4.1. Since the value R0 is related to R01,R02, we need to perform their sensitivity analyses, respectively. Here, we only show its results for R02 due to their similarity (see Table 2).
Parameter | Sensitivity index |
⟨k⟩ | −1.000 |
α1 | 0 |
α2 | +1.000 |
bi | +0.500 |
β1 | 0 |
β2 | −0.833 |
μi | −0.167 |
d | −1.333 |
From Table 2, it is evident that a 1% reduction in the leaving rate ⟨k⟩ leads to a 1% increase in R02, while a 1% reduction in β2 results in a 0.833% increase in R02. Conversely, a 1% reduction in the incoming rate bi(i=1,2) decreases R02 by 0.5%. Additionally, increasing μi(i=1,2) impacts rumor spreading, further reducing R02.
Remark 4. In summary, various effective strategies can be employed to decrease R02, such as enhancing the leaving rate d, regulating the transmission rate among individuals, etc. These measures can be implemented through network consensus monitoring, educational campaigns, and other interventions. However, some specific control strategies are not proposed in the model, and an optimal solution to suppress rumours is also not provided here. Therefore, we will carry out an in-depth study on this aspect in the future.
The dynamical behaviors of the multilingual rumor propagation 2I2S2R model have been analyzed under heterogeneous networks. The basic reproduction number by the next-generation matrix method has been calculated, and the stability has been explored in different cases. Moreover, numerical simulations have been provided to further show the dynamic characteristics of the model. However, the research background presented in this paper is somewhat idealized and does not adequately address several critical real-world factors, including time delays, stochastic phenomena, and the influence of government surveillance. To enhance the robustness of our findings, in future research, we aim to develop a more comprehensive multilingual rumor propagation model that reflects the complexities of real-world scenarios. This will enable us to engage in a more in-depth discussion regarding the control mechanisms associated with the suppression and elimination of rumors.
Methodology, L.H. and J.W.; formal analysis, L.H. and J.W; resources, J.W.; writing original draft preparation, L.H. and J.W.; writing-review and editing, J.L. and T.M.; supervision, T.M.; software, T.M. All authors have read and agreed to the published version of the manuscript.
The authors declare they have not used Artificial Intelligence (AI) tools in the creation of this article.
This work was funded in part by the National Natural Science Foundation of Peoples Republic of China under Grant 62366049, Grant 62006196, Grant 62003380, in part by Natural Science Foundation of Xinjiang Uygur Autonomous Region under Grant 2021D01C113.
The authors declare there are no conflicts of interest.
[1] |
D. J. Daley, D. G. Kendall, Stochastic rumours, IMA J. Appl. Math., 1 (1965), 42–55. https://doi.org/10.1093/imamat/1.1.42 doi: 10.1093/imamat/1.1.42
![]() |
[2] |
L. Zhu, Y. Wang, Rumor spreading model with noise interference in complex social networks, Phys. A: Stat. Mech. Appl., 469 (2017), 750–760. https://doi.org/10.1016/j.physa.2016.11.119 doi: 10.1016/j.physa.2016.11.119
![]() |
[3] |
L. Zhao, J. Wang, Y. Chen, Q. Wang, J. Cheng, H. Cui, SIHR rumor spreading model in social networks, Phys. A: Stat. Mech. Appl., 391 (2012), 2444–2453. https://doi.org/10.1016/j.physa.2011.12.008 doi: 10.1016/j.physa.2011.12.008
![]() |
[4] |
A. Jain, J. Dhar, V. Gupta, Stochastic model of rumor propagation dynamics on homogeneous social network with expert interaction and fluctuations in contact transmissions, Phys. A: Stat. Mech. Appl., 519 (2019), 227–236. https://doi.org/10.1016/j.physa.2018.11.051 doi: 10.1016/j.physa.2018.11.051
![]() |
[5] |
Y. Xiao, Q. Yang, C. Sang, Y. Liu, Rumor diffusion model based on representation learning and anti-rumor, IEEE Trans. Netw. Serv. Manage., 17 (2020), 1910–1923. https://doi.org/10.1109/TNSM.2020.2994141 doi: 10.1109/TNSM.2020.2994141
![]() |
[6] |
D. Li, Y. Zhao, Y. Deng, Rumor spreading model with a focus on educational impact and optimal control, Nonlinear Dyn., 112 (2024), 1575–1597. https://doi.org/10.1007/s11071-023-09102-5 doi: 10.1007/s11071-023-09102-5
![]() |
[7] |
Z. Yu, S. Lu, D. Wang, Z. Li, Modeling and analysis of rumor propagation in social networks, Infor. Sci., 580 (2021), 857–873. https://doi.org/10.1016/j.ins.2021.09.012 doi: 10.1016/j.ins.2021.09.012
![]() |
[8] |
W. Pan, W. Yan, Y. Hu, R. He, L. Wu, Dynamic analysis of a SIDRW rumor propagation model considering the effect of media reports and rumor refuters, Nonlinear Dyn., 111 (2023), 3925–3936. https://doi.org/10.1007/s11071-022-07947-w doi: 10.1007/s11071-022-07947-w
![]() |
[9] |
Z. Zhang, X. Mei, H. Jiang, X. Luo, Y. Xia, Dynamical analysis of Hyper-SIR rumor spreading model, Appl. Math. Comput., 446 (2023), 127887. https://doi.org/10.1016/j.amc.2023.127887 doi: 10.1016/j.amc.2023.127887
![]() |
[10] |
Q. Liu, T. Li, M. Sun, The analysis of an SEIR rumor propagation model on heterogeneous network, Phys. A: Stat. Mech. Appl., 469 (2017), 372–380. https://doi.org/10.1016/j.physa.2016.11.067 doi: 10.1016/j.physa.2016.11.067
![]() |
[11] |
X. Tong, H. Jiang, J. Qiu, X. Luo, S. Chen, Dynamic analysis of the IFCD rumor propagation model under stochastic disturbance on heterogeneous networks, Chaos Soliton. Fract., 173 (2023), 113637. https://doi.org/10.1016/j.chaos.2023.113637 doi: 10.1016/j.chaos.2023.113637
![]() |
[12] |
J. Li, H. Jiang, X. Mei, C. Hu, G. Zhang, Dynamical analysis of rumor spreading model in multi-lingual environment and heterogeneous complex networks, Inform. Sci., 536 (2020), 391–408. https://doi.org/10.1016/j.ins.2020.05.037 doi: 10.1016/j.ins.2020.05.037
![]() |
[13] |
L. Zhu, X. Wang, Z. Zhang, C. Lei, Spatial dynamics and optimization method for a rumor propagation model in both homogeneous and heterogeneous environment, Nonlinear Dyn., 105 (2021), 3791–3817. https://doi.org/10.1007/s11071-021-06782-9 doi: 10.1007/s11071-021-06782-9
![]() |
[14] |
X. Luo, H. Jiang, S. Chen, J. Li, Stability and optimal control for delayed rumor-spreading model with nonlinear incidence over heterogeneous networks, Chinese Phys. B, 32 (2023), 058702. https://doi.org/10.1088/1674-1056/acb490 doi: 10.1088/1674-1056/acb490
![]() |
[15] |
D. Li, W. Qian, X. Sun, D. Han, M. Sun, Rumor spreading in a dual-relationship network with diverse propagation abilities, Appl. Math. Comput., 458 (2023), 128233. https://doi.org/10.1016/j.amc.2023.128233 doi: 10.1016/j.amc.2023.128233
![]() |
[16] |
X. Lv, D. Fan, Q. Li, J. Wang, L. Zhou, Simplicial SIR rumor propagation models with delay in both homogeneous and heterogeneous networks, Phys. A: Stat. Mech. Appl., 627 (2023), 129131. https://doi.org/10.1016/j.physa.2023.129131 doi: 10.1016/j.physa.2023.129131
![]() |
[17] |
X. Zhong, Y. Yang, F. Deng, G. Liu, Rumor propagation control with anti-rumor mechanism and intermittent control strategies, IEEE Trans. Comput. Soc. Syst., 11 (2024), 2397–2409. https://doi.org/10.1109/TCSS.2023.3277465 doi: 10.1109/TCSS.2023.3277465
![]() |
[18] |
N. Ding, G. Guan, S. Shen, L. Zhu, Dynamical behaviors and optimal control of delayed S2IS rumor propagation model with saturated conversion function over complex networks, Commun. Nonlinear Sci. Numer. Simul., 128 (2024), 107603. https://doi.org/10.1016/j.cnsns.2023.107603 doi: 10.1016/j.cnsns.2023.107603
![]() |
[19] |
X. Lv, D. Fan, J. Yang, Q. Li, L. Zhou, Delay differential equation modeling of social contagion with higher-order interactions, Appl. Math. Comput., 466 (2024), 128464. https://doi.org/10.1016/j.amc.2023.128464 doi: 10.1016/j.amc.2023.128464
![]() |
[20] |
S. Yu, Z. Yu, H. Jiang, J. Li, Dynamical study and event-triggered impulsive control of rumor propagation model on heterogeneous social network incorporating delay, Chaos Soliton. Fract., 145 (2021), 110806. https://doi.org/10.1016/j.chaos.2021.110806 doi: 10.1016/j.chaos.2021.110806
![]() |
[21] |
J. Wang, H. Jiang, T. Ma, C. Hu, Global dynamics of the multi-lingual SIR rumor spreading model with cross-transmitted mechanism, Chaos Soliton. Fract., 126 (2019), 148–157. https://doi.org/10.1016/j.chaos.2019.05.027 doi: 10.1016/j.chaos.2019.05.027
![]() |
[22] |
J. Liao, J. Wang, J. Li, X. Jiang, The dynamics and control of a multi-lingual rumor propagation model with non-smooth inhibition mechanism, Math. Biosci. Eng., 21 (2024), 5068–5091. https://doi.org/10.3934/mbe.2024224 doi: 10.3934/mbe.2024224
![]() |
[23] |
S. Yu, Z. Yu, H. Jiang, X. Mei, J. Li, The spread and control of rumors in a multilingual environment, Nonlinear Dyn., 100 (2020), 2933–2951. https://doi.org/10.1007/s11071-020-05621-7 doi: 10.1007/s11071-020-05621-7
![]() |
[24] |
M. Ye, J. Li, H. Jiang, Dynamic analysis and optimal control of a novel fractional-order 2I2SR rumor spreading model, Nonlinear Anal.: Model. Control, 28 (2023), 1–24. https://doi.org/10.15388/namc.2023.28.32599 doi: 10.15388/namc.2023.28.32599
![]() |
[25] |
Y. Ding, L. Zhu, Turing instability analysis of a rumor propagation model with time delay on non-network and complex networks, Inform. Sci., 667 (2024), 120402. https://doi.org/10.1016/j.ins.2024.120402 doi: 10.1016/j.ins.2024.120402
![]() |
[26] |
R. Yang, B. H. Wang, J. Ren, W. J. Bai, Z. W. Shi, W. X. Wang, et al., Epidemic spreading on heterogeneous networks with identical infectivity, Phys. Lett. A, 364 (2007), 189–193. https://doi.org/10.1016/j.physleta.2006.12.021 doi: 10.1016/j.physleta.2006.12.021
![]() |
[27] |
R. Pastor-Satorras, A. Vespignani, Epidemic dynamics in finite size scale-free networks, Phys. Rev. E, 65 (2002), 035108. https://doi.org/10.1103/PhysRevE.65.035108 doi: 10.1103/PhysRevE.65.035108
![]() |
[28] |
H. Zhang, X. Fu, Spreading of epidemics on scale-ree networks with nonlinear infectivity, Nonlinear Anal., 70 (2009), 3273–3278. https://doi.org/10.1016/j.na.2008.04.031 doi: 10.1016/j.na.2008.04.031
![]() |
[29] |
P. Van den Driessche, J. Watmough, Reproduction numbers and sub-threshold endemic equilibria for compartmental models of disease transmission, Math. Biosci., 180 (2002), 29–48. https://doi.org/10.1016/S0025-5564(02)00108-6 doi: 10.1016/S0025-5564(02)00108-6
![]() |
[30] | J. P. LaSalle, Stability theory for ordinary differential equations, J. Differ. Equations, 4 (1968), 57–65. |
[31] |
F. Chen, On a nonlinear nonautonomous predator-prey model with diffusion and distributed delay, J. Comput. Appl. Math., 180 (2005), 33–49. https://doi.org/10.1016/j.cam.2004.10.001 doi: 10.1016/j.cam.2004.10.001
![]() |
[32] |
Z. He, Z. Cai, J. Yu, X. Wang, Y. Sun, Y. Li, Cost-efficient strategies for restraining rumor spreading in mobile social networks, IEEE Trans. Veh. Technol., 66 (2016), 2789–2800. https://doi.org/10.1109/TVT.2016.2585591 doi: 10.1109/TVT.2016.2585591
![]() |
[33] |
Y. Xia, H. Jiang, Z. Yu, S. Yu, X. Luo, Dynamic analysis and optimal control of a reaction-diffusion rumor propagation model in multi-lingual environments, J. Math. Anal. Appl., 521 (2023), 126967. https://doi.org/10.1016/j.jmaa.2022.126967 doi: 10.1016/j.jmaa.2022.126967
![]() |
[34] |
J. Wang, H. Jiang, C. Hu, Z. Yu, J. Li, Stability and Hopf bifurcation analysis of multi-lingual rumor spreading model with nonlinear inhibition mechanism, Chaos Soliton. Fract., 153 (2021), 111464. https://doi.org/10.1016/j.chaos.2021.111464 doi: 10.1016/j.chaos.2021.111464
![]() |
[35] |
N. Chitnis, J. M. Hyman, J. M. Cushing, Determining important parameters in the spread of malaria through the sensitivity analysis of a mathematical model, Bull. Math. Biol., 70 (2008), 1272–1296. https://doi.org/10.1007/s11538-008-9299-0 doi: 10.1007/s11538-008-9299-0
![]() |
Parameters | Meaning |
bv | Coming rate of the group v |
d | Leaving rate of the different compartment |
αv | Rumor conversion rate (i.e., cross transmission showed in Figure 1) |
βv | Probability of Svki cured and transformed into Rvki |
μv | Immunity rate of Ignorants against rumors |
⟨k⟩ | The average degree |
θ(ki) | Infectivity of a user with degree ki |
Φv(t) | The probabilities that an ignorant whether in Group-1 or Group-2 gets in touch with spreaders |
Parameter | Sensitivity index |
⟨k⟩ | −1.000 |
α1 | 0 |
α2 | +1.000 |
bi | +0.500 |
β1 | 0 |
β2 | −0.833 |
μi | −0.167 |
d | −1.333 |
Parameters | Meaning |
bv | Coming rate of the group v |
d | Leaving rate of the different compartment |
αv | Rumor conversion rate (i.e., cross transmission showed in Figure 1) |
βv | Probability of Svki cured and transformed into Rvki |
μv | Immunity rate of Ignorants against rumors |
⟨k⟩ | The average degree |
θ(ki) | Infectivity of a user with degree ki |
Φv(t) | The probabilities that an ignorant whether in Group-1 or Group-2 gets in touch with spreaders |
Parameter | Sensitivity index |
⟨k⟩ | −1.000 |
α1 | 0 |
α2 | +1.000 |
bi | +0.500 |
β1 | 0 |
β2 | −0.833 |
μi | −0.167 |
d | −1.333 |