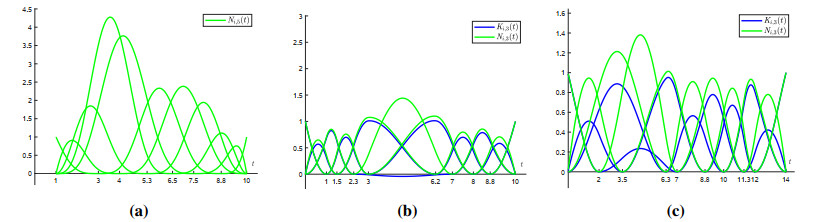
Citation: Lanlan Liu, Yuxue Zhu, Feng Wang, Yuanjie Geng. Infinity norm bounds for the inverse of SDD+1 matrices with applications[J]. AIMS Mathematics, 2024, 9(8): 21294-21320. doi: 10.3934/math.20241034
[1] | Narongrit Kaewbanjak, Watcharin Chartbupapan, Kamsing Nonlaopon, Kanit Mukdasai . The Lyapunov-Razumikhin theorem for the conformable fractional system with delay. AIMS Mathematics, 2022, 7(3): 4795-4802. doi: 10.3934/math.2022267 |
[2] | Hadjer Belbali, Maamar Benbachir, Sina Etemad, Choonkil Park, Shahram Rezapour . Existence theory and generalized Mittag-Leffler stability for a nonlinear Caputo-Hadamard FIVP via the Lyapunov method. AIMS Mathematics, 2022, 7(8): 14419-14433. doi: 10.3934/math.2022794 |
[3] | Michael Precious Ineh, Edet Peter Akpan, Hossam A. Nabwey . A novel approach to Lyapunov stability of Caputo fractional dynamic equations on time scale using a new generalized derivative. AIMS Mathematics, 2024, 9(12): 34406-34434. doi: 10.3934/math.20241639 |
[4] | Kamal Shah, Muhammad Sher, Muhammad Sarwar, Thabet Abdeljawad . Analysis of a nonlinear problem involving discrete and proportional delay with application to Houseflies model. AIMS Mathematics, 2024, 9(3): 7321-7339. doi: 10.3934/math.2024355 |
[5] | Dinghong Jiang, Chuanzhi Bai . On coupled Gronwall inequalities involving a ψ-fractional integral operator with its applications. AIMS Mathematics, 2022, 7(5): 7728-7741. doi: 10.3934/math.2022434 |
[6] | Pratap Anbalagan, Evren Hincal, Raja Ramachandran, Dumitru Baleanu, Jinde Cao, Chuangxia Huang, Michal Niezabitowski . Delay-coupled fractional order complex Cohen-Grossberg neural networks under parameter uncertainty: Synchronization stability criteria. AIMS Mathematics, 2021, 6(3): 2844-2873. doi: 10.3934/math.2021172 |
[7] | Mengmeng Jiang, Xiao Niu . A new design method to global asymptotic stabilization of strict-feedforward nonlinear systems with state and input delays. AIMS Mathematics, 2024, 9(4): 9494-9507. doi: 10.3934/math.2024463 |
[8] | Huizhen Qu, Jianwen Zhou . S-asymptotically ω-periodic dynamics in a fractional-order dual inertial neural networks with time-varying lags. AIMS Mathematics, 2022, 7(2): 2782-2809. doi: 10.3934/math.2022154 |
[9] | Jingfeng Wang, Chuanzhi Bai . Global Mittag-Leffler stability of Caputo fractional-order fuzzy inertial neural networks with delay. AIMS Mathematics, 2023, 8(10): 22538-22552. doi: 10.3934/math.20231148 |
[10] | Ravi P. Agarwal, Snezhana Hristova . Stability of delay Hopfield neural networks with generalized proportional Riemann-Liouville fractional derivative. AIMS Mathematics, 2023, 8(11): 26801-26820. doi: 10.3934/math.20231372 |
Schoenberg introduced the trigonometric spline functions defined by divided differences in [18]. And the trigonometric spline functions have been shown to possess many B-spline-like properties. In view of this, many scholars call the trigonometric splines the trigonometric B-splines, in [4,8]. It is well-known that the trigonometric B-splines are piecewise functions corresponding to the spaces
T2n+1:=span{1,cost,sint,cos2t,sin2t,⋯,cosnt,sinnt}, |
for odd-order, and
H2n:=span{cost2,sint2,cos3t2,sin3t2,⋯,cos(2n−1)t2,sin(2n−1)t2}, |
for even-order. Odd-order trigonometric B-splines in T2n+1 form a partition of unity, a desirable property for curve design. However, even-order B-splines in H2n lack this partition of unity, creating challenges in certain applications where this property is critical. As a result, extensive research has focused on the odd-order basis, particularly on its normalization. The author of [8] established the recurrence relation for the trigonometric B-splines of arbitrary order and derived trigonometric Marsden identity. The author of [20] utilized the trigonometric Marsden identity to derive the normalized odd-order trigonometric B-splines over uniform knots. Building on the trigonometric Marsden identity expansion introduced by [7], [11] explicitly derived the normalization coefficients required to ensure that the trigonometric B-spline basis functions are properly normalized. [15] provided the p-Bézier basis functions in the space T2n+1, which is defined over any interval of length <π. Those basis functions are a subcase of the normalized trigonometric B-splines. [2] presented the normalized Bernstein-like basis functions in the space T2n+1, which is defined over the interval [0,π/2]. [21] established the C-B spline basis. In this work, the C-B spline basis of order 3 is just the normalized trigonometric B-spline basis corresponding to the space T3. In a more general context, the normalized trigonometric B-splines are also considered in [16] as a special case.
Curve and surface design is an important area in Computer Aided Geometric Design (CAGD), where trigonometric B-splines are a foundational tool. Normalized trigonometric B-splines enable enhanced construction and control of curves and surfaces, suggesting their potential applications in aircraft design [9]. Additionally, trigonometric B-splines show promising applications in other fields, such as physical simulations (see [5,12,19]).
The purpose of this paper is to introduce the integral formula for odd-order trigonometric B-splines. Given the significant applications and theoretical importance of integral formulas in Chebyshev systems for fields such as numerical analysis, signal processing, and function approximation, it is notable that the integral properties of sin and cos in trigonometric B-spline bases render them incapable of being directly derived like other bases in Chebyshev systems. The aim of this study is to provide a similar integral formula for trigonometric B-spline bases. To achieve this, this paper first constructs a novel set of even-order trigonometric B-spline curve basis functions and, through integration of these functions, successfully derives the traditional odd-order trigonometric B-spline basis functions, thereby establishing the integral formula for odd-order trigonometric B-spline bases. During this derivation process, a determinant of even order with structural symmetry is obtained. Furthermore, this study refines the conditions for knot sequences to ensure that the corresponding normalized trigonometric B-spline bases possess nonnegativity.
Our main contributions are
● A set of trigonometric spline bases corresponding to the DC component-free space is provided, along with the integral representation of normalized trigonometric B-spline bases corresponding to space T2n+1.
● Adjusting the conditions imposed on the knot sequence to guarantee the nonnegativity of the normalized trigonometric B-spline basis functions.
● A structurally symmetric even-order determinant is presented.
The remainder of this article is organized as follows: Section 2 reviews the related concepts and properties. The improvements to knot sequences are discussed in Section 3. In Section 4, the trigonometric spline basis corresponding to the DC component-free space and the integral formula for the normalized trigonometric B-spline basis are presented. The final conclusions are drawn in the last section.
In this section, we will recall some established concepts and conclusions.
[1] has demonstrated that the knot sequences for B-spline basis functions can be finite, infinite, or bi-infinite. Analogous to the case of traditional B-spline basis functions, this paper focuses on the study of trigonometric B-spline basis functions corresponding to bi-infinite knot sequences.
The normalized trigonometric B-spline basis functions are defined in a manner analogous to the de Boor-Cox formula [4,7,8,11].
Definition 2.1. (Normalized trigonometric B-spline basis functions) Given a knot sequence T={ti}+∞i=−∞, such that
ti≤ti+1,0<ti+2n+1−ti<2π,i∈Z,n∈Z+, | (2.1) |
the normalized trigonometric B-spline basis functions of order 2n+1 are defined as follows:
Ki,2n+1(t)=ζi,2n+1Ni,2n+1(t), | (2.2) |
where
Ni,1(t)={1, if ti≤t<ti+1,0,otherwise. | (2.3) |
Ni,2n(t)=sin(t−ti2)sin(ti+2n−1−ti2)Ni,2n−1(t)+sin(ti+2n−t2)sin(ti+2n−ti+12)Ni+1,2n−1(t), | (2.4) |
Ni,2n+1(t)=sin(t−ti2)sin(ti+2n−ti2)Ni,2n(t)+sin(ti+2n+1−t2)sin(ti+2n+1−ti+12)Ni+1,2n(t), | (2.5) |
ζi,2n+1=1(2n)!∑μn∏j=1costi+μ(2j)−ti+μ(2j−1)2, | (2.6) |
and the sum is taken over all permutations μ:{1,2,⋯,2n}→{1,2,⋯,2n}.
Remark 2.1 In [4,8,11], the knot sequence satisfies the condition 0<ti+2n+1−ti<2π. However, the knot sequence described in [7] satisfies a slightly different condition, 0<ti+2n−ti<2π. In the subsequent section, the conditions of the knot sequence are reiterated.
If ti−1<ti=ti+1=⋯=ti+mi−1<ti+mi, where 1≤mi≤2n, the knots tj, where j=i,i+1,⋯,i+mi−1, are referred to as knots of multiplicity mi. Especially, we set 00=0. The space of trigonometric spline basis is defined by
Γ2n+1[T]:={Ni,2n+1(t)|Ni,2n+1(t)|t∈[ti,ti+1)∈T2n+1, and N(l)i,2n+1(ti−)=N(l)i,2n+1(ti+),0≤l≤2n−mi,i∈Z}. |
The trigonometric B-spline basis functions possess many B-spline-like properties [3,6,8,14].
Property 2.1. (Properties of the trigonometric B-spline basis functions) The trigonometric B-spline basis functions Ni,2n+1(t) defined in Eq (2.5) possess the following properties:
(1) (Local support) For any i∈Z and n∈Z+, there exists
Ni,2n+1(t)={>0,t∈(ti,ti+2n+1),=0,t∉[ti,ti+2n+1]. | (2.7) |
(2) (Continuity) The continuous order of Ni,2n+1(t) at tj (where i≤j≤i+2n+1), denoted as kji,2n+1, can be described as
kji,2n+1={2n−ξ,i≤j≤i+ξ−1,2n−mj, i+ξ≤j≤i+2n+1−η,2n−η, i+2n+2−η≤j≤i+2n+1, | (2.8) |
if ti=ti+1=⋯=ti+ξ−1<ti+ξ≤ti+ξ+1≤⋯≤ti+2n+1−η<ti+2n+2−η=⋯=ti+2n+1.
In this section, we will adjust the conditions of the knot sequence to ensure the nonnegativity of the normalized trigonometric B-spline basis functions Ki,2n+1(t) for i∈Z and n∈Z+.
First, the condition (2.1) can be relaxed.
The nonnegativity of the basis function Ni,2n+1(t) is ensured by the condition 0<ti+2n+1−ti<2π as stated in (2.1). Since the length of the support intervals for Ni,2n(t) and Ni+1,2n(t) in Eq (2.4) is 2n, replacing 0<ti+2n+1−ti<2π with the condition 0<ti+2n−ti<2π still guarantees the nonnegativity of Ni,2n+1(t). Therefore, the condition (2.1) can be relaxed to
ti≤ti+1,0<ti+2n−ti<2π,i∈Z,n∈Z+. | (3.1) |
Second, the condition to ensure the positivity of the normalized coefficients ζi,2n+1 in Eq (2.6) is presented. According to the representation in Eq (2.6), we obtain the condition to ensure the positivity of the normalized coefficient ζi,2n+1, that is
0≤ti+2n−1−ti<π, i∈Z,n∈Z+. | (3.2) |
The Bézier-like basis defined in the space T2n+1 is a subcase of the trigonometric B-spline basis. In [13], it was noted that the space T2n+1 does not have an NTP basis when the domain of T2n+1 is [0,π]. This implies the non-existence of normalized coefficients. Consequently, we conclude that ti+2n−1−ti≠π. In [15], it proved that there exist NTP bases provided that the domain of T2n+1 is any interval of length <π, specifically referred to as p-Bézier bases. This implies that the normalized coefficient satisfies the condition ti+2n−1−ti<π, in the special cases of Bézier.
Figure 1a presents an example of the basis functions Ni,2n+1(t) for i∈Z, whose corresponding knot sequence T satisfies condition (3.1) but fails to satisfy condition (2.1). Here n=2 and the knot sequence T={ti}16i=1={1,1,1,1,1,3,4,5.3,6.5,7.5,8.8,10,10,10,10,10}. Figure 1b shows the trigonometric B-spline basis functions Ni,3(t) and Ki,3(t) corresponding to the knot sequence that satisfies condition (3.1) but does not satisfy condition (3.2), while Figure 1c presents the functions Ni,3(t) and Ki,3(t) corresponding to the knot sequence that satisfies both conditions (3.1) and (3.2).
Although the coefficients of the normalized trigonometric B-spline basis have already been defined in Eq (2.6), for the convenience of deriving and proving the integral formula of the normalized trigonometric B-spline basis, this paper introduces a simplified normalized coefficient expression that is equivalent to Eq (2.6).
Lemma 3.1. Let
Ci,2n+1=1(2n−1)!!∑γ(1,2,…,2n)n∏r=1costi+m2r−ti+m2r−12,i∈Z,n∈Z+, | (3.3) |
where the sum is taken over all permutations γ(1,2,…,2n):{m1,m2,…,m2n}→{1,2,…,2n}, with m1,m2,…,m2n being a permutation of 1,2,…,2n that satisfies m1<m3<…<m2n−1 and m2r−1<m2r for each r=1,…,n. Then there exists
Ci,2n+1=ζi,2n+1, |
where ζi,2n+1 defined in Eq (2.6).
Third, a new definition for the normalized trigonometric B-spline basis that guarantees nonnegativity is presented.
Definition 3.1. (Nonnegative normalized trigonometric B-spline basis functions) Given a knot sequence T={ti}+∞i=−∞, such that
ti≤ti+1, 0<ti+2n−ti<2π and 0≤ti+2n−1−ti<π, i∈Z,n∈Z+, | (3.4) |
the nonnegative normalized trigonometric B-spline basis functions of order 2n+1 are defined as follows:
Ki,1(t)={1, if ti≤t<ti+1,0,otherwise. | (3.5) |
Ki,2n+1(t)=Ci,2n+1Ni,2n+1(t), | (3.6) |
where Ni,2n+1(t), Ci,2n+1 is defined in Eqs (2.5) and (3.3), respectively.
In this section, we generally set the knot sequence to be T={ti}+∞i=−∞ that satisfies condition (3.4), where the multiplicity of ti is mi with 1≤mi≤2n, and kji,2n+1 is defined in Eq (2.8) without further explanation. Let us define the DC component-free space as T2n:=span{cost,sint,⋯,cosnt,sinnt}. Its corresponding piecewise trigonometric polynomial space is
Γ2n[T]:={Fi,2n(t)|Fi,2n(t)|t∈[ti,ti+1)∈T2n, and F(l)i,2n(ti−)=F(l)i,2n(ti+),0≤l≤2n−mi−1, 1≤mi≤2n−1,i∈Z}. |
Clearly, Γ2n[T] is a linear space. We can derive the subspace of Γ2n[T] as follows:
Γ2n[ti,ti+2n]={Fi,2n(t)∈Γ2n[T]|Fi,2n(t)=0,t∉[ti,ti+2n] and Fi,2n(t)≠0,t∈(ti,ti+2n),F(l)i,2n(tj−)=F(l)i,2n(tj+),0≤l≤kji,2n, i≤j≤i+2n}. |
It can be shown that a function in the space \(\Gamma_{2n}[t_i, t_{i + 2n}]\) exhibits local support and a specific order of continuity.
The following determinant and its accompanying proof are presented to facilitate future derivations.
Lemma 4.1. For any n∈Z+, then the following identity holds.
D(t1,t2,⋯,t2n):=|cost1cost2…cost2nsint1sint2…sint2ncos2t1cos2t2…cos2t2nsin2t1sin2t2…sin2t2n⋮⋮⋮⋮cosnt1cosnt2…cosnt2nsinnt1sinnt2…sinnt2n|=22n2−nn!∏1≤l<j≤nsintj−tl2∑γ(1,2,…,2n)n∏r=1costm2r−tm2r−12, | (4.1) |
where γ(1,2,…,2n) defined in Lemma 3.1.
Proof. According to Euler's formula and the identity eitj−eits=2iei2(tj+ts)sintj−ts2, it follows that
D(t1,t2,⋯,t2n)=|eit1+e−it12eit2+e−it22…eit2n+e−it2n2eit1−e−it12ieit2−e−it22i…eit2n−e−it2n2i⋮⋮⋮⋮enit1+e−nit12enit2+e−nit22…enit2n+e−nit2n2enit1−e−nit12ienit2−e−nit22i…enit2n−e−nit2n2i|=(−1)n2+n(2i)ne−nit1…e−nit2n|11…1eit1eit2…eit2n⋮⋮⋮⋮e(n−1)it1e(n−1)it2…e(n−1)it2ne(n+1)it1e(n+1)it2…e(n+1)it2n⋮⋮⋮⋮e2nit1e2nit2…e2nit2n|=22n2−n2ne−nit1…e−nit2n∑1≤m1<m2<…<mn≤2neitm1…eitmn∏1≤s<j≤2nei2(tj+ts)sintj−ts2. |
For the sake of convenience, let
C(t1,t2,⋯,t2n)=∑γ(1,2,…,2n)n∏r=1costm2r−tm2r−12. | (4.2) |
Therefore, it suffices to prove that
C(t1,t2,⋯,t2n)=n!2ne−nit1…e−nit2n∑1≤m1<m2<…<mn≤2neitm1…eitmn∏1≤s<j≤2nei2(tj+ts)=n!2n∑1≤m1<m2<…<mn≤2nexp(i2mn∑q=m1tq−i2(2n∑h=1th−mn∑q=m1tq)). | (4.3) |
We establish the inductive hypothesis for n. For n=1, the result is straightforward. Assume that the conclusion holds for n≤p−1, where \(p\) is any positive integer. Specifically, we have
C(tn1,tn2,⋯,tn2p−2)=(p−1)!2(p−1)∑εexp(i2mp−1∑q=m1tq−i2(n2p−2∑h=n1th−mp−1∑q=m1tq)), |
where the sum is taken over all permutations ε:{m1,m2,⋯,mp−1}→{n1,n2,⋯,n2p−2}. Here, n1,n2,⋯,n2p−2 represent a permutation of 1,2,⋯,2n such that n1<n2<⋯<n2p−2, while m1,m2,⋯,mp−1 is a subset of n1,n2,⋯,n2p−2 satisfying m1<m2<⋯<mp−1.
Next, consider the case where n=p. Based on this assumption, we have
C(t1,t2,⋯,t2p)=cost2p−t2p−12∑γ(1,2,⋯,2p−2)p−1∏r=1costm2r−tm2r−12+cost2p−t2p−22∑γ(1,2,⋯,2p−3,2p−1)p−1∏r=1costm2r−tm2r−12+⋯+cost2p−t22∑γ(1,3,4,⋯,2p−1)p−1∏r=1costm2r−tm2r−12+cost2p−t12∑γ(2,3,⋯,2p−1)p−1∏r=1costm2r−tm2r−12=12(exp(i2(t2p−t2p−1))+exp(−i2(t2p−t2p−1)))C(t1,t2,⋯,t2p−2)+12(exp(i2(t2p−t2p−2))+exp(−i2(t2p−t2p−2)))C(t1,t2,⋯,t2p−3,t2p−1)+⋯+12(exp(i2(t2p−t2))+exp(−i2(t2p−t2)))C(t1,t3,t4,⋯,t2p−1)+12(exp(i2(t2p−t1))+exp(−i2(t2p−t1)))C(t2,t3,⋯,t2p−1)=p!2p∑1≤m1<m2<…<mp≤2pexp(i2mp∑q=m1tq−i2(2p∑h=1th−mp∑q=m1tq)), |
where the sum is taken over all permutations γ(n1,n2,⋯,n2p−2):{m1,m2,⋯,m2p−2}→{n1,n2,⋯,n2p−2}, with m1,m2,⋯,m2p−2 being a permutation of n1,n2,⋯,n2p−2 that satisfies m1<m3<⋯<m2p−3 and m2r−1<m2r for each r=1,⋯,p−1. Here, the sequence {n1,n2,⋯,n2p−2} represents, in order, the sequences {1,2,⋯,2p−2}, {1,2,⋯,2p−3,2p−1}, ⋯, {1,3,4,⋯,2p−1}, and \(\{2, 3, \cdots, 2p-1\}\). Eq (4.3) is valid for any positive integer n. Thus, the lemma is proved.
This subsection defines a set of truncated functions and demonstrates that any function in the space Γ2n[ti,ti+2n] can be expressed as a linear combination of these functions.
Let g2n(t):=sintsin2n−2(t2)(n∈Z+), the truncated functions Gi,2n(t), where i∈Z and n∈Z+, are defined as follows: If ti=ti+1=⋯=ti+ξ−1<ti+ξ, then
Gi,2n(t):={0,t<ti,g(ξ−1)2n(t−ti),t≥ti. | (4.4) |
Figure 2a–2c illustrate examples of the function g4(t) and the truncated functions Gi,4(t), where i∈Z, over single and multiple knots, respectively.
To prove that any function in the space Γ2n[ti,ti+2n] can be represented as a linear combination of {Gi,2n(t)}i∈Z, the following lemmas will be utilized.
Lemma 4.2. There exist n+1 real numbers d0,d1,⋯,dn such that
sin2n(t2)=d0+d1cost+d2cos2t+⋯+dncosnt, |
where d0=(2n−1)!!(2n)!!.
Proof. It is well known that
sin2n(t2)∈span{1,cost,cos2t,⋯,cosnt}, |
since
sin2n(t2)=(sin2(t2))n=(1−cost2)n. |
Thus, there are n+1 real numbers d0,d1,⋯,dn such that
sin2n(t2)=d0+d1cost+d2cos2t+⋯+dncosnt. |
We deduce that, based on Euler's formula,
(eit2−e−it22i)2n=d0+d1eit+e−it2+d2e2it+e−2it2+⋯+dnenit+e−nit2. | (4.5) |
Expanding the left side of Eq (4.5) using the binomial theorem results in d0=(2n−1)!!(2n)!!.
Lemma 4.3. The function Gi,2n(t) defined in Eq (4.4) lies in Γ2n[T] for any i∈Z,n∈Z+.
Proof. First, according to Lemma 4.2, we obtain
sin2n−2(t2)∈span{1,cost,cos2t,⋯,cos(n−1)t}, |
Thus, we have
sintsin2n−2(t2)∈span{sint,sin2t,⋯,sinnt}, |
and
g2n(t−ti)∈T2n for i∈Z. |
Second, the order of continuity of g2n(t−ti) at ti is 2n−2, because that
g(l)2n(t−ti)|t=ti=0,l=0,1,⋯,2n−2, and g(2n−1)2n(t−ti)|t=ti≠0. |
Third, it is easy to see that the order of continuity of Gi,2n(t) is 2n−ξ−1 at ti and is ∞ at the other knots.
In conclusion, Gi,2n(t)∈Γ2n[T], for i∈Z.
Lemma 4.4. The functions Gi,2n(t) for i∈Z in Eq (4.4) are linearly independent.
Proof. If ti−1<ti=ti+1=⋯=ti+mi−1<ti+mi, the mi functions Gj,2n(t) for i≤j≤i+mi−1 are linearly independent since they have different continuous orders at ti.
For the sake of simplicity, let r=i+mi and tr−1<tr=tr+1=⋯=tr+mr−1<tr+mr. We can then similarly conclude that the functions Gj,2n(t) for r≤j≤r+mr−1 are linearly independent. In addition, it is straightforward to derive that
span{Gi,2n(t),Gi+1,2n(t),⋯,Gi+mi−1,2n(t)}∩span{Gr,2n(t),Gr+1,2n(t),⋯,Gr+mr−1,2n(t)}={0}. |
Thus, Gi,2n(t),Gi+1,2n(t),⋯,Gi+mi−1,2n(t),Gr,2n(t),Gr+1,2n(t),⋯,Gr+mr−1,2n(t) are linearly independent. Consequently, the functions Gi,2n(t) for i∈Z are linearly independent.
From the above lemmas, we conclude that any function in the space Γ2n[ti,ti+2n] can be expressed as a linear combination of Gi,2n(t) for i∈Z.
Theorem 4.1. For any function Fi,2n(t)∈Γ2n[ti,ti+2n], there exist 2n−η+1 real numbers νi,νi+1,⋯,νi+2n−η such that
Fi,2n(t)=i+2n−η∑j=iνjGj,2n(t), t∈[ti,ti+2n), |
where η is the multiplicity of ti+2n in the interval [ti,ti+2n] and the functions Gj,2n(t), where i≤j≤i+2n−η, are defined in Eq (4.4).
Proof. Since Fi,2n(t) in space Γ2n[ti,ti+2n] is a piecewise function, it can first be linearly represented by the functions Gj,2n(t) for i≤j≤i+2n−η in Eq (4.4) over a non-zero interval within its support interval.
Suppose ti=ti+1=⋯=ti+ξ−1<ti+ξ≤⋯≤ti+2n−η<ti+2n−η+1=⋯=ti+2n. We consider that the function Fi,2n(t) can be linearly represented in the interval [ti,ti+ξ). Thus, we prove that there exist ξ real numbers κi,κi+1,⋯,κi+ξ−1 such that
Fi,2n(t)|[ti,ti+ξ)=i+ξ−1∑j=iκjGj,2n(t). |
For simplicity, assume that
f1(t)=Fi,2n(t)|[ti,ti+ξ), t∈[ti,ti+ξ). |
Based on the definition of the space Γ2n[ti,ti+2n], we know that f1(t)∈T2n, which means that there exist 2n real numbers x1,x2,⋯,x2n such that
f1(t)=x1cost+x2sint+⋯+x2n−1cosnt+x2nsinnt, |
and
f(s)1(ti−)=f(s)1(ti)=f(s)1(ti+), 0≤s≤2n−ξ−1, |
which implies that
f(s)1(ti)=0, 0≤s≤2n−ξ−1. |
Let ρ=2n−ξ−1, Ωρ:={f1(t)|f1(t)∈T2n,f(s)1(ti)=0,0≤s≤ρ}, and Ψρ:=span{g2n(t−ti),g′2n(t−ti+1),⋯,g(2n−ρ−2)2n(t−ti+ξ−1)}=span{g2n(t−ti),g′2n(t−ti),⋯,g(2n−ρ−2)2n(t−ti)}.
Since g2n(t−ti),g′2n(t−ti),⋯,g(2n−ρ−2)2n(t−ti) are linearly independent, we conclude that the dimension of Ψρ is 2n−ρ−1. According to the definition of the function g2n(t), we obtain that g(l)2n(t−ti)∈Ωρ, l=0,1,⋯,2n−ρ−2. Hence, it follows naturally that Ψρ is the subspace of Ωρ.
The dimension of the space Ωρ is equal to the dimension of the solution space corresponding to the following linear equations.
{f1(ti)=0,f′1(ti)=0,⋮f(ρ)1(ti)=0. | (4.6) |
Thus, the following linear equations holds:
{x1costi+x2sinti+x3cos2ti+x4sin2ti+⋯+x2n−1cosnti+x2nsinnti=0,−x1sinti+x2costi−x32sin2ti+x42cos2ti+⋯−x2n−1nsinnti+x2nncosnti=0,−x1costi−x2sinti−x322cos2ti−x422sin2ti+⋯−x2n−1n2cosnti−x2nn2sinnti=0,x1sinti−x2costi+x323sin2ti−x423cos2ti+⋯+x2n−1n3sinnti−x2nn3cosnti=0,⋮x1cos(π2ρ+ti)+x2sin(π2ρ+ti)+⋯+x2n−1nρcos(π2ρ+nti)+x2nnρsin(π2ρ+nti)=0, | (4.7) |
where the corresponding coefficient matrix is given as
(costisinticos2tisin2ti…cosntisinnti−sinticosti−2sin2ti2cos2ti…−nsinntincosnti−costi−sinti−22cos2ti−22sin2ti…−n2cosnti−n2sinntisinti−costi23sin2ti−23cos2ti…n3sinnti−n3cosnti⋮⋮⋮⋮⋮⋮cos(π2ρ+ti)sin(π2ρ+ti)2ρcos(π2ρ+2ti)2ρsin(π2ρ+2ti)…nρcos(π2ρ+nti)nρsin(π2ρ+nti)). | (4.8) |
By performing elementary row operations, it is demonstrated that the matrix (4.8) maintains full row rank, independent of the parity of ρ. This result establishes that the dimension of the solution space for the linear system (4.7) is
2n−(ρ+1)=2n−ρ−1. |
The dimension of Ωρ is determined to be 2n−ρ−1. Consequently, it follows that Ωρ=Ψρ. In other words, we know that there exist 2n−ρ−1=2n−(2n−ξ−1)−1=ξ real numbers κi,κi+1,⋯,κi+ξ−1 such that
f1(t)=i+ξ−1∑j=iκjg(j−i)2n(t−tj). |
According to the definition of Gi,2n(t), the function \(f_1(t)\) can be expressed as
f1(t)=i+ξ−1∑j=iκjGj,2n(t). |
Consider the non-zero interval [ti+ξ,ti+ξ+mi+ξ). We define
f2(t)=Fi,2n(t)|[ti+ξ,ti+ξ+mi+ξ),t∈[ti+ξ,ti+ξ+mi+ξ). |
According to the continuous order of Fi,2n(t) at ti+ξ, we deduce that
f(s)1(ti+ξ)=f(s)1(ti+ξ−)=f(s)2(ti+ξ+)=f(s)2(ti+ξ),0≤s≤2n−mi+ξ−1. |
This implies that
f(s)2(ti+ξ)−f(s)1(ti+ξ)=0,0≤s≤2n−mi+ξ−1. |
Thus, from the above analysis, it follows that there are mi+ξ real numbers κi+ξ,κi+ξ+1,⋯,κi+ξ+mi+ξ−1 such that
f2(t)−f1(t)=i+ξ+mi+ξ−1∑j=i+ξκjGj,2n(t). |
Consequently, we have
f2(t)=i+ξ+mi+ξ−1∑j=iκjGj,2n(t). |
For every non-zero subinterval of the support interval of Fi,2n(t), we consider it this way. It can be concluded that there exist 2n−η+1 real numbers νi,νi+1,⋯,νi+2n−η such that
Fi,2n(t)=i+2n−η∑j=iνjGj,2n(t), t∈[ti,ti+2n−η+1)=[ti,ti+2n), |
where νj for i≤j≤i+2n−η is expressed as a linear combination of κi,κi+1,⋯,κi+2n−η.
The subsection demonstrates that the dimension of Γ2n[ti,ti+2n] is 1. To support this, we first require the following lemma.
Lemma 4.5. Given an integer i and positive integers n,η such that η≤2n−1, then the determinant
|Gi,2n(ti+2n)Gi+1,2n(ti+2n)…Gi+2n−η−1,2n(ti+2n)G′i,2n(ti+2n)G′i+1,2n(ti+2n)…G′i+2n−η−1,2n(ti+2n)⋮⋮⋮⋮G(2n−η−1)i,2n(ti+2n)G(2n−η−1)i+1,2n(ti+2n)…G(2n−η−1)i+2n−η−1,2n(ti+2n)|≠0, |
where the functions Gj,2n(t) for i≤j≤i+2n−η−1 are defined in Eq (4.4).
Proof. Use reduction to absurdity. Assume that the determinant is equal to zero. Then, there are 2n−η real numbers zi,zi+1,⋯,zi+2n−η−1, which are not all equal to zero, satisfying
i+2n−η−1∑l=izl(Gl,2n(ti+2n)G′l,2n(ti+2n)⋮G(2n−η−1)l,2n(ti+2n))=0. |
Let Y(t)=i+2n−η−1∑l=izlGl,2n(t). Then, we find that the first 2n−η−1 derivatives of Y(t) at ti+2n are all zero. According to Theorem 4.1, there exist mi+2n real numbers, such that
Y(t)=i+2n+mi+2n−η−1∑u=i+2n−ηzuGu,2n(t). |
Therefore,
Y(t)=i+2n−η−1∑l=izlGl,2n(t)=i+2n+mi+2n−η−1∑u=i+2n−ηzuGu,2n(t), |
and
i+2n−η−1∑l=izlGl,2n(t)−i+2n+mi+2n−η−1∑u=i+2n−ηzuGu,2n(t)=0. |
According to Lemma 4.4, we obtain that zi=⋯=zi+2n−η−1=0. This conflicts with the assumption. So, the lemma is proved.
From Theorem 4.1 and Lemma 4.5, we obtain the dimension of Γ2n[ti,ti+2n].
Theorem 4.2. The dimension of the linear space Γ2n[ti,ti+2n] is 1.
Proof. Suppose that u(t) is an arbitrary function in Γ2n[ti,ti+2n]. Thus, according to Theorem 4.1, there are 2n−η+1 real numbers νi,νi+1,⋯,νi+2n−η such that
u(t)=i+2n−η∑j=iνjGj,2n(t),t∈[ti,ti+2n)=[ti,ti+2n−η+1), |
where η denotes the multiplicity of ti+2n in the interval [ti,ti+2n]. Consider the continuous order of function u(t) at ti+2n. We have
u(l)(ti+2n−)=u(l)(ti+2n)=u(l)(ti+2n+)=0,l=0,1,2,⋯,2n−η−1, |
which implies that
u(l)(ti+2n)=0,l=0,1,2,⋯,2n−η−1. |
Thus, the equation representing the continuous order of u(t)s at ti+2n is given by
{u(ti+2n)=0,u′(ti+2n)=0,⋮u(2n−η−1)(ti+2n)=0. | (4.9) |
which can be expressed in matrix form as follows:
(Gi,2n(ti+2n)Gi+1,2n(ti+2n)…Gi+2n−η,2n(ti+2n)G′i,2n(ti+2n)G′i+1,2n(ti+2n)…G′i+2n−η,2n(ti+2n)⋮⋮⋮⋮G(2n−η−1)i,2n(ti+2n)G(2n−η−1)i+1,2n(ti+2n)…G(2n−η−1)i+2n−η,2n(ti+2n))(νiνi+1⋮νi+2n−η)=0. | (4.10) |
This system consists of linear equations with νi,νi+1,⋯,νi+2n−η as variables. Based on Lemma 4.5, the coefficient matrix of these linear equations is full row rank, with a rank of 2n−η+1−(2n−η)=1. This indicates that the dimension of Γ2n[ti,ti+2n] is 1.
In this subsection, we consider single knots and assume that ti<ti+1 for any i∈Z. Then the even-order trigonometric spline basis functions corresponding to the DC component-free space T2n, and the integral expression for the trigonometric B-spline basis Ki,2n+1(t) are presented.
According to Theorem 4.2 and the definition of Γ2n[ti,ti+2n], it is established that Fi,2n(t)∈Γ2n[ti,ti+2n] and the dimension of Γ2n[ti,ti+2n] is 1. If we find a function H(t)∈Γ2n[ti,ti+2n], then it follows that Fi,2n(t)=αH(t),t∈[ti,ti+2n) for some real number α. Thus, the following theorem is provided.
Theorem 4.3. (The function expression in the space Γ2n[ti,ti+2n] over single knots) For any function Fi,2n(t)∈Γ2n[ti,ti+2n], there exists a real number α such that
Fi,2n(t)=αH(t),t∈[ti,ti+2n), |
where
H(t)=|Gi,2n(t)Gi+1,2n(t)…Gi+2n−1,2n(t)Gi+2n,2n(t)costicosti+1…costi+2n−1costi+2nsintisinti+1…sinti+2n−1sinti+2ncos2ticos2ti+1…cos2ti+2n−1cos2ti+2nsin2tisin2ti+1…sin2ti+2n−1sin2ti+2n⋮⋮⋮⋮⋮cosnticosnti+1…cosnti+2n−1cosnti+2nsinntisinnti+1…sinnti+2n−1sinnti+2n|, |
and α=−1D(ti,ti+1,⋯,ti+2n−1). Here D(ti,ti+1,⋯,ti+2n−1) and the functions Gj,2n(t) for i≤j≤i+2n are defined in Eqs (4.1) and (4.4), respectively.
Proof. According to Theorem 4.1, there exist 2n real numbers αi,αi+1,⋯,αi+2n−1 such that
Fi,2n(t)=i+2n−1∑j=iαjGj,2n(t),t∈[ti,ti+2n), | (4.11) |
By the continuous order of Fi,2n(t) at ti+2n, it follows that
F(d)i,2n(ti+2n)=i+2n−1∑j=iαjG(d)j,2n(ti+2n)=0,t∈[ti,ti+2n),d=0,1,2,⋯,2n−2. | (4.12) |
This can be expressed equivalently as a system of equations
{i+2n−1∑j=iαjGj,2n(ti+2n)=0,i+2n−1∑j=iαjG′j,2n(ti+2n)=0,⋮i+2n−1∑j=iαjG(2n−2)j,2n(ti+2n)=0. | (4.13) |
In addition, we have
sin(t−ti+2n)sin2n−2(t−ti+2n2)=i+2n−1∑j=iβjsin(t−tj)sin2n−2(t−tj2), | (4.14) |
where βi,βi+1,⋯,βi+2n−1 are real numbers. Since Eq (4.14) when t=ti+2n is equivalent to Eq (4.13). We obtain that αj=βj,j=i,⋯,i+2n−1. Thus, we only focus on βj,i≤j≤i+2n−1. By proving Lemma 4.3, it can be concluded that there exist n numbers η1,η2,⋯,ηn such that
sin(t−tj)sin2n−2(t−tj2)=n∑l=1ηlsinl(t−tj),j=i,i+1,⋯,i+2n. | (4.15) |
So we can rewrite (4.14) as follows:
(costsint…cosntsinnt)(−η1sinti+2nη1costi+2n−η2sin2ti+2nη2cos2ti+2n⋮−ηnsinnti+2nηncosnti+2n)=(costsint…cosntsinnt)(−η1sinti…−η1sinti+2n−1η1costi…η1costi+2n−1−η2sin2ti…−η2sin2ti+2n−1η2cos2ti…η2cos2ti+2n−1⋮⋮⋮−ηnsinnti…−ηnsinnti+2n−1ηncosnti…ηncosnti+2n−1)(βiβi+1⋮βi+2n−2βi+2n−1). | (4.16) |
Based on the properties of matrix operations, we deduce that
(−η1sinti…−η1sinti+2n−1η1costi…η1costi+2n−1−η2sin2ti…−η2sin2ti+2n−1η2cos2ti…η2cos2ti+2n−1⋮⋮⋮−ηnsinnti…−ηnsinnti+2n−1ηncosnti…ηncosnti+2n−1)(βiβi+1⋮βi+2n−2βi+2n−1)=(−η1sinti+2nη1costi+2n−η2sin2ti+2nη2cos2ti+2n⋮−ηnsinnti+2nηncosnti+2n). | (4.17) |
The coefficient matrix of (4.17) is non-zero due to the linear independence of the functions cost,sint,⋯, cosnt,sinnt. According to Cramer's Rule, we have
βi+j−1=D(ti,⋯,ti+j−2,ti+2n,ti+j,⋯,ti+2n−1)D(ti,ti+1,⋯,ti+2n−1), for j=1,2,⋯,2n. |
We apply this result to Eq (4.11) to yield
Fi,2n(t)=2n∑j=1αi+j−1Gi+j−1,2n(t)=2n∑j=1D(ti,⋯,ti+j−2,ti+2n,ti+j,⋯,ti+2n−1)D(ti,ti+1,⋯,ti+2n−1)Gi+j−1,2n(t)=D(ti+2n,ti+1,⋯,ti+2n−1)D(ti,ti+1,⋯,ti+2n−1)Gi,2n(t)+D(ti,ti+2n,ti+2,⋯,ti+2n−1)D(ti,ti+1,⋯,ti+2n−1)Gi+1,2n(t)+⋯+D(ti,⋯,ti+2n−2,ti+2n)D(ti,ti+1,⋯,ti+2n−1)Gi+2n−1,2n(t)=(−1)2n−1D(ti+1,⋯,ti+2n)D(ti,ti+1,⋯,ti+2n−1)Gi,2n(t)+(−1)2n−2D(ti,ti+2,⋯,ti+2n)D(ti,ti+1,⋯,ti+2n−1)Gi+1,2n(t)+⋯+D(ti,⋯,ti+2n−2,ti+2n)D(ti,ti+1,⋯,ti+2n−1)Gi+2n−1,2n(t)+D(ti,⋯,ti+2n−2,ti+2n−1)D(ti,ti+1,⋯,ti+2n−1)Gi+2n,2n(t)=−1D(ti,ti+1,⋯,ti+2n−1)H(t), t∈[ti,ti+2n), |
where Gi+2n,2n(t)=0,t∈[ti,ti+2n).
The linear space Γ2n[ti,ti+2n] corresponds to space T2n. Similarly, the space Γ2n+1[ti,ti+2n+1], which corresponds to space T2n+1, can be defined as follows:
Γ2n+1[ti,ti+2n+1]:={Mi,2n+1(t)∈Γ2n+1[T]|Mi,2n+1(t)=0,t∉[ti,ti+2n+1] and Mi,2n+1(t)≠0,t∈(ti,ti+2n+1), M(l)i,2n+1(tj−)=M(l)i,2n+1(tj+),l=0,1,⋯,kji,2n+1, i≤j≤i+2n+1.} |
We can derive the following theorem analogously.
Theorem 4.4. The dimension of the linear space Γ2n+1[ti,ti+2n+1] is 1.
The proof is similar to the proof of Theorem 4.2.
Inspired by the method for constructing normalized B-basis in extended Chebyshev space presented in [10], we use the functions Fi,2n(t), where i∈Z, to construct the normalized function Mi,2n+1(t). Therefore, the following theorem holds.
Theorem 4.5. (The normalized function) Suppose that
Mi,2n+1(t)=∫t−∞Fi,2n(s)ds∫+∞−∞Fi,2n(t)dt−∫t−∞Fi+1,2n(s)ds∫+∞−∞Fi+1,2n(t)dt, | (4.18) |
where Fi,2n(t)∈Γ2n[ti,ti+2n] and Fi+1,2n(t)∈Γ2n[ti+1,ti+2n+1] are defined in Theorem 4.3, with i∈Z and n∈Z+. Then we have
+∞∑i=−∞Mi,2n+1(t)≡1, t∈[tj,tj+1). |
Proof. For t∈[tj,tj+1), there exists
+∞∑i=−∞Mi,2n+1(t)=+∞∑i=−∞(∫t−∞Fi,2n(s)ds∫+∞−∞Fi,2n(t)dt−∫t−∞Fi+1,2n(s)ds∫+∞−∞Fi+1,2n(t)dt)=j∑i=j−2n(∫t−∞Fi,2n(s)ds∫+∞−∞Fi,2n(t)dt−∫t−∞Fi+1,2n(s)ds∫+∞−∞Fi+1,2n(t)dt)=j∑i=j−2n(∫ttiFi,2n(s)ds∫ti+2ntiFi,2n(t)dt−∫tti+1Fi+1,2n(s)ds∫ti+2n+1ti+1Fi+1,2n(t)dt)=∫ttj−2nFj−2n,2n(s)ds∫tjtj−2nFj−2n,2n(t)dt−∫ttj+1Fj+1,2n(s)ds∫tj+2n+1tj+1Fj+1,2n(t)dt=∫tjtj−2nFj−2n,2n(s)ds+∫ttjFj−2n,2n(s)ds∫tjtj−2nFj−2n,2n(t)dt−0=1+∫ttjFj−2n⋅2n(s)ds∫tjtj−2nFj−2n⋅2n(t)dt−0=1. |
Lemma 4.6. The function Mi,2n+1(t) in Eq (4.18) lies in the space Γ2n+1[ti,ti+2n+1].
Proof. First, Eq (4.18) indicates that the support interval of the function Mi,2n+1(t) is the union of the interval of Fi,2n(t) and Fi+1,2n(t), denoted as [ti,ti+2n+1).
Second, since the integral operator increases the continuous order by 1, the continuous order of Mi,2n+1(t) at tj is greater than or equal to
kji,2n+1=kji,2n+1,i≤j≤i+2n+1. |
So, it is natural that
Mi,2n+1(t)∈Γ2n+1[ti,ti+2n+1]. |
According to Theorem 4.4 and Lemma 4.6, the normalized function Mi,2n+1(t) in Eq (4.18) must be equal to the trigonometric B-spline function Ni,2n+1(t) in Eq (2.5) multiplied by a constant. Therefore, the following theorem is established.
Theorem 4.6. (Integral representation of the normalized trigonometric B-spline basis) Given a knot sequence T={ti}+∞i=−∞ satisfying
ti<ti+1, 0<ti+2n−ti<2π and 0≤ti+2n−1−ti<π, i∈Z,n∈Z+, |
then there holds
Ki,2n+1(t)=Mi,2n+1(t), t∈[ti,ti+2n+1), |
where Ki,2n+1(t) and Mi,2n+1(t) are separately defined in Definition 3.1 and Eq (4.18).
Proof. We will demonstrate that the expression of Mi,2n+1(t), as defined in Eq (4.18), is identical to that of Ki,2n+1(t) defined in Definition 3.1. The notations C(ti+1,ti+2,⋯,ti+2n) in Lemma 4.1 and U(ti,ti+1,⋯,ti+2n) (see [17]) are used in the following proof, where
U(ti,ti+1,⋯,ti+2n)=|11…11costicosti+1…costi+2n−1costi+2nsintisinti+1…sinti+2n−1sinti+2ncos2ticos2ti+1…cos2ti+2n−1cos2ti+2nsin2tisin2ti+1…sin2ti+2n−1sin2ti+2n⋮⋮⋮⋮⋮cosnticosnti+1…cosnti+2n−1cosnti+2nsinntisinnti+1…sinnti+2n−1sinnti+2n|=22n2∏i≤l<j≤i+2nsintj−tl2. | (4.19) |
Based on Definition 2.1, it suffices to prove the explicit expression of the function Mi,2n+1(t) over a non-zero subinterval within its support interval. Therefore, according to Lemmas 3.1, 4.1, and 4.2, and Theorems 4.3 and 4.5, we deduce that
Mi,2n+1(t)=∫ttiFi,2n(s)ds∫ti+2ntiFi,2n(t)dt=D(ti+1,ti+2,⋯,ti+2n)(2n−1)!!(2n)!!U(ti,ti+1,⋯,ti+2n)sin2n(t−ti2)=22n2−nn!∏i+1≤l<j≤i+2nsintj−tl2C(ti+1,ti+2,⋯,ti+2n)(2n−1)!!(2n)!!22n2∏i≤l<j≤i+2nsintj−tl2sin2n(t−ti2)=C(ti+1,ti+2,⋯,ti+2n)(2n−1)!!∏j=1,2,⋯,2nsinti+j−ti2sin2n(t−ti2)=Ci,2n+1∏j=1,2,⋯,2nsinti+j−ti2sin2n(t−ti2), t∈[ti,ti+1). |
Since
Ni,2n+1(t)=sin2n(t−ti2)∏j=1,2,⋯,2nsinti+j−ti2, t∈[ti,ti+1), |
we have
Mi,2n+1(t)=Ci,2n+1Ni,2n+1(t), t∈[ti,ti+1), |
consequently
Mi,2n+1(t)=Ci,2n+1Ni,2n+1(t)=Ki,2n+1(t), t∈[ti,ti+2n+1). |
In this subsection, we consider multiple knots and assume that the multiplicity of the knot ti in the interval [ti,ti+2n) is ξ, while the multiplicity of the knot ti+2n in the same interval is η. Similarly to the single knot case, there exist the following theorems.
Theorem 4.7. (The function expression in the space Γ2n[ti,ti+2n] over multiple knots) Let
Au,v=((cos(t+π2u))|t=tv, (sin(t+π2u))|t=tv, ⋯,nu(cos(nt+π2u))|t=tv, nu(sin(nt+π2u))|t=tv )T, |
Bu,v=((−1)u−1Au−1,v, (−1)u−2Au−2,v, ⋯,(−1)1A1,v, (−1)0A0,v ), |
Eu,v=(G(u−1)v,2n(t), G(u−2)v,2n(t), ⋯,G′v,2n(t), Gv,2n(t) ). |
For any function Fi,2n(t)∈Γ2n[ti,ti+2n], there exists a real number α such that
Fi,2n(t)=αH(t),t∈[ti,ti+2n), |
where
α=(−1)η|Bξ,iBmi+ξ,i+ξBmi+ξ+mi+ξ,i+ξ+mi+ξ⋯Bmi+2n−η,i+2n−ηBη−1,i+2n|, |
and
H(t)=|Eξ,iEmi+ξ,i+ξEmi+ξ+mi+ξ,i+ξ+mi+ξ⋯Emi+2n−η,i+2n−ηEη,i+2nBξ,iBmi+ξ,i+ξBmi+ξ+mi+ξ,i+ξ+mi+ξ⋯Bmi+2n−η,i+2n−ηBη,i+2n|. |
Here the functions Gj,2n(t) for i≤j≤i+2n are defined in Eq (4.4).
Proof. To simplify the notation, we define
φu,v=(sin(t−tv)sin2n−2(t−tv2))(u). |
Thus, we conclude that
φη−1,i+2n=βiφξ−1,i+βi+1φξ−2,i+⋯+βi+ξ−1φ0,i+βi+ξφmi+ξ−1,i+ξ+⋯+βi+ξ+mi+ξ−1φ0,i+ξ+⋯+βi+2n+1−η−mi+2n−ηφmi+2n−η−1,i+2n−η+⋯+βi+2n−ηφ0,i+2n−η+βi+2n−η+1φ0,i+2n+⋯+βi+2n−1φη−2,i+2n. | (4.20) |
Similar to Theorem 4.3, there exist
Fi,2n(t)=i+2n−η∑j=iαjGj,2n(t),t∈[ti,ti+2n)=[ti,ti+2n+1−η), | (4.21) |
F(d)i,2n(ti+2n)=i+2n−η∑j=iαjG(d)j,2n(ti+2n)=0,t∈[ti,ti+2n+1−η),d=0,1,2,⋯,2n−1−η. | (4.22) |
Additionally, it follows that Eq (4.20) is equivalent to Eq (4.22) when t=ti+2n. Thus, by applying the proof strategy from Theorem 4.3, we derive that
Fi,2n(t)=(−1)η|Eξ,iEmi+ξ,i+ξEmi+ξ+mi+ξ,i+ξ+mi+ξ⋯Emi+2n−η,i+2n−ηEη,i+2nBξ,iBmi+ξ,i+ξBmi+ξ+mi+ξ,i+ξ+mi+ξ⋯Bmi+2n−η,i+2n−ηBη,i+2n||Bξ,iBmi+ξ,i+ξBmi+ξ+mi+ξ,i+ξ+mi+ξ⋯Bmi+2n−η,i+2n−ηBη−1,i+2n|. |
Theorem 4.8. (The normalized function over generalized knots) Suppose that Eq (4.18) still holds, where Fi,2n(t)∈Γ2n[ti,ti+2n] and Fi+1,2n(t)∈Γ2n[ti+1,ti+2n+1] are defined in Theorem 4.7, with i∈Z and n∈Z+, then
+∞∑i=−∞Mi,2n+1(t)≡1, t∈[tj,tj+1). |
In addition, when Fi,2n(t)=0, we set
∫t−∞(∫+∞−∞Fi,2n(s)ds)−1Fi,2n(s)ds={0t<ti,1t≥ti. |
Theorem 4.9. (Integral representation of the normalized trigonometric B-spline basis over generalized knots) Given a knot sequence T={ti}+∞i=−∞ satisfying condition (3.4), then there holds
Ki,2n+1(t)=Mi,2n+1(t), t∈[ti,ti+2n+1), |
where Ki,2n+1(t) and Mi,2n+1(t) are separately defined in Definition 3.1 and Theorem 4.8.
This subsection presents examples of curve modeling to demonstrate that a curve possesses the convex hull property when the knot sequence satisfies condition (3.4).
Figures 3 and 4 illustrate examples of open and closed curves over different knot sequences, respectively. The red curves correspond to the knot sequences that satisfy condition (3.4) as defined in Definition 3.1, while the orange curves correspond to the knot sequences that satisfy condition (2.1) as defined in Definition 2.1. Additionally, the knot sequences in Figure 3 are T={0,2,3,3,3.5,5.3,6.1,6.6,8.4,9.1,9.5,11.2,12.2,12.6,14.2,15}, and T={0,0.8,3,3,3.5,5.3,6.6,8.6,9.1,9.1,10,12.2,12.2,13,14.2,15}, while those in Figure 4 are T={−8,−8,−8,−8,−8,−7,−6.3,−5.6,−4.9,−4.5,−1.2,−1.2,−1.2,0,1.2,1.2, 1.2,4.5,4.9,5.6,6.3,7,8,8,8,8,8}, and T={−8,−8,−8,−8,−8,−7,−6.3,−5.3,−4,−3.3,−1.2,−1.2, −1.2,0,1.2,1.2,1.2,3.3,4,5.3,6.3,7,8,8,8,8,8}. Clearly, if the knot sequence only satisfies condition (2.1), the convex hull property of the curve cannot be guaranteed.
In the Chebyshev system, due to the integral properties of sine and cosine in the trigonometric B-spline basis, the trigonometric B-spline basis cannot be directly derived from lower-order bases through integration, which leads to an unnormal Chebyshev system. This paper successfully derives the integral formula for the normalized odd-order trigonometric B-spline basis by constructing a new set of even-order trigonometric B-spline bases. This integral formula allows for the transition from even-order to odd-order bases but cannot be obtained through stepwise integration, indicating that it is only similar to a segment of the integral formula in the Chebyshev system. Although we are currently unable to provide a direct recursive formula for integrating from lower-order trigonometric spline bases to higher-order ones, we hope to use this as a foundation for further exploration of this issue in future work.
Mei Li: Investigation, methodology, software, validation, writing—original draft preparation, writing—review and editing; Wanqiang Shen: Conceptualization, methodology, software, writing—original draft preparation, writing—review and editing. All authors have read and approved the final version of the manuscript for publication.
The authors declare they have not used Artificial Intelligence (AI) tools in the creation of this article.
This research is supported in part by the National Natural Science Foundation of China (Grant No. 61772013).
The authors declare that there are no conflicts of interest regarding the publication of this paper.
[1] | A. Berman, R. Plemmons, Nonnegative Matrices in the Mathematical Sciences, New York: SIAM, 1994. |
[2] | C. Zhang, New Advances in Research on H-matrices, Beijing: Science Press, 2017. |
[3] |
L. Cvetković, H-matrix theory vs. eigenvalue localization, Numer. Algor., 42 (2006), 229–245. http://doi.org/10.1007/s11075-006-9029-3 doi: 10.1007/s11075-006-9029-3
![]() |
[4] |
X. Gu, S. Wu, A parallel-in-time iterative algorithm for Volterra partial integro-differential problems with weakly singular kernel, J. Comput. Phys., 417 (2020), 109576. http://doi.org/10.1016/j.jcp.2020.109576 doi: 10.1016/j.jcp.2020.109576
![]() |
[5] | A. Berman, R. Plemmons, Nonnegative Matrix in the Mathematical Sciences, New York: Academic Press, 1979. |
[6] | R. Cottle, J. Pang, R. Stone, The Linear Complementarity Problem, New York: SIAM, 2009. |
[7] |
X. Chen, S. Xiang, Computation of error bounds for P-matrix linear complementarity problems, Math. Program., 106 (2006), 513–525. http://doi.org/10.1007/s10107-005-0645-9 doi: 10.1007/s10107-005-0645-9
![]() |
[8] |
P. Dai, J. Li, S. Zhao, Infinity norm bounds for the inverse for GSDD1 matrices using scaling matrices, Comput. Appl. Math., 42 (2023), 121–141. http://doi.org/10.1007/s40314-022-02165-x doi: 10.1007/s40314-022-02165-x
![]() |
[9] |
D. Cvetković, L. Cvetković, C. Li, CKV-type matrices with applications, Linear Algebra Appl., 608 (2020), 158–184. http://doi.org/10.1016/j.laa.2020.08.028 doi: 10.1016/j.laa.2020.08.028
![]() |
[10] |
L. Kolotilina, Some bounds for inverses involving matrix sparsity pattern, J. Math. Sci., 249 (2020), 242–255. http://doi.org/10.1007/s10958-020-04938-3 doi: 10.1007/s10958-020-04938-3
![]() |
[11] |
L. Cvetković, Kostić, S. Rauški, A new subclass of H-matrices, Appl. Math. Comput., 208 (2009), 206–210. http://dx.doi.org/10.1016/j.amc.2008.11.037 doi: 10.1016/j.amc.2008.11.037
![]() |
[12] | L. Cvetković, V. Kostic, R. Varga, A new Geršgorin-type eigenvalue inclusion set, Electron. Trans. Numer. Anal., 18 (2004), 73–80. |
[13] |
H. Orera, J. Peña, Infinity norm bounds for the inverse of Nekrasov matrices using scaling matrices, Appl. Math. Comput., 358 (2019), 119–127. http://doi.org/10.1016/j.amc.2019.04.027 doi: 10.1016/j.amc.2019.04.027
![]() |
[14] |
P. Dai, C. Lu, Y. Li, New error bounds for the linear complementarity problem with an SB-matrix, Numer. Algor., 64 (2013), 741–757. http://doi.org/10.1007/s11075-012-9691-6 doi: 10.1007/s11075-012-9691-6
![]() |
[15] |
P. Dai, Y. Li, C. Lu, Error bounds for linear complementarity problems for SB-matrices, Numer. Algor., 61 (2012), 121–139. http://doi.org/10.1007/s11075-012-9580-z doi: 10.1007/s11075-012-9580-z
![]() |
[16] |
C. Li, Y. Li, Note on error bounds for linear complementarity problems for B-matrices, Appl. Math. Lett., 57 (2016), 108–113. http://doi.org/10.1016/j.aml.2016.01.013 doi: 10.1016/j.aml.2016.01.013
![]() |
[17] | R. Cottle, J. Pang, R. E. Stone, The Linear Complementarity Problem, San Diego: Academic Press, 1992. |
[18] |
X. Song, L. Gao, CKV-type B-matrices and error bounds for linear complementarity problems, AIMS Math., 6 (2021), 10846–10860. http://doi.org/10.3934/math.2021630 doi: 10.3934/math.2021630
![]() |
[19] |
J. Peña, Diagonal dominance, Schur complements and some classes of H-matrices and P-matrices, Adv. Comput. Math., 35 (2011), 357–373. http://doi.org/10.1007/s10444-010-9160-5 doi: 10.1007/s10444-010-9160-5
![]() |
[20] |
J. Varah, A lower bound for the smallest singular value of a matrix, Linear Algebra Appl., 11 (1975), 3–5. http://doi.org/10.1016/0024-3795(75)90112-3 doi: 10.1016/0024-3795(75)90112-3
![]() |
[21] | R. S. Varga, Geršgorin and His Circles, Berlin: Springer-Verlag, 2004. |
[22] |
V. Kostić, L. Cvetković, D. Cvetković, Pseudospectra localizations and their applications, Numer. Linear Algebra Appl., 23 (2016), 356–372. http://doi.org/10.1002/nla.2028 doi: 10.1002/nla.2028
![]() |
[23] |
J. Peña, A class of P-matrices with applications to the localization of the eigenvalues of a real matrix, SIAM J. Matrix Anal. Appl., 22 (2001), 1027–1037. http://doi.org/10.1137/s0895479800370342 doi: 10.1137/s0895479800370342
![]() |
[24] |
M. Neumann, J. Peña, O. Pryporova, Some classes of nonsingular matrices and applications, Linear Algebra Appl., 438 (2013), 1936–1945. http://doi.org/10.1016/j.laa.2011.10.041 doi: 10.1016/j.laa.2011.10.041
![]() |
[25] |
J. Peña, On an alternative to Gerschgorin circles and ovals of Cassini, Numer. Math., 95 (2003), 337–345. http://doi.org/10.1007/s00211-002-0427-8 doi: 10.1007/s00211-002-0427-8
![]() |
[26] |
P. Dai, J. Li, Y. Li, C. Zhang, Error bounds for linear complementarity problems of QN-matrices, Calcolo, 53 (2016), 647–657. http://doi.org/10.1007/s10092-015-0167-7 doi: 10.1007/s10092-015-0167-7
![]() |
[27] |
J. Li, G. Li, Error bounds for linear complementarity problems of S-QN matrices, Numer. Algor., 83 (2020), 935–955. http://doi.org/10.1007/s11075-019-00710-0 doi: 10.1007/s11075-019-00710-0
![]() |
[28] |
C. Li, P. Dai, Y. Li, New error bounds for linear complementarity problems of Nekrasov matrices and B-Nekrasov matrices, Numer. Algor., 74 (2016), 997–1009. http://doi.org/10.1007/s11075-016-0181-0 doi: 10.1007/s11075-016-0181-0
![]() |
[29] |
M. García-Esnaola, J. Peña, A comparison of error bounds for linear complementarity problems of H-matrices, Linear Algebra Appl., 433 (2010), 956–964. http://doi.org/10.1016/j.laa.2010.04.024 doi: 10.1016/j.laa.2010.04.024
![]() |