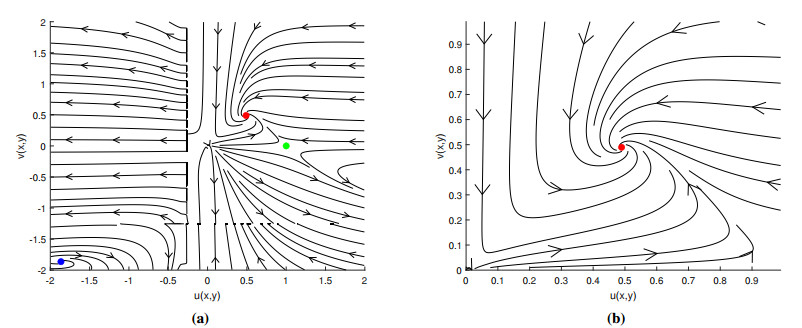
In this work, a Leslie-Gower model with a weak Allee effect on the prey and a fear effect on the predator is proposed. By using qualitative analyses, the local stability of the coexisting equilibrium and the existence of Turing instable are discussed. By analyzing the distribution of eigenvalues, the existence of a Hopf bifurcation is studied by using the gestation time delay as a bifurcation parameter. By utilizing the normal form method and the center manifold theorem, we calculate the direction of the Hopf bifurcation and the stability of bifurcating periodic solutions. We indicate that both the weak Allee effect on the prey and fear effect on the predator have an important impact on the dynamical behaviour of the new Leslie-Gower model. We also verify the obtained results by some numerical examples.
Citation: Fatao Wang, Ruizhi Yang, Yining Xie, Jing Zhao. Hopf bifurcation in a delayed reaction diffusion predator-prey model with weak Allee effect on prey and fear effect on predator[J]. AIMS Mathematics, 2023, 8(8): 17719-17743. doi: 10.3934/math.2023905
[1] | Chenxuan Nie, Dan Jin, Ruizhi Yang . Hopf bifurcation analysis in a delayed diffusive predator-prey system with nonlocal competition and generalist predator. AIMS Mathematics, 2022, 7(7): 13344-13360. doi: 10.3934/math.2022737 |
[2] | Weili Kong, Yuanfu Shao . Bifurcations of a Leslie-Gower predator-prey model with fear, strong Allee effect and hunting cooperation. AIMS Mathematics, 2024, 9(11): 31607-31635. doi: 10.3934/math.20241520 |
[3] | Weili Kong, Yuanfu Shao . The effects of fear and delay on a predator-prey model with Crowley-Martin functional response and stage structure for predator. AIMS Mathematics, 2023, 8(12): 29260-29289. doi: 10.3934/math.20231498 |
[4] | Xuyang Cao, Qinglong Wang, Jie Liu . Hopf bifurcation in a predator-prey model under fuzzy parameters involving prey refuge and fear effects. AIMS Mathematics, 2024, 9(9): 23945-23970. doi: 10.3934/math.20241164 |
[5] | Yudan Ma, Ming Zhao, Yunfei Du . Impact of the strong Allee effect in a predator-prey model. AIMS Mathematics, 2022, 7(9): 16296-16314. doi: 10.3934/math.2022890 |
[6] | Na Min, Hongyang Zhang, Xiaobin Gao, Pengyu Zeng . Impacts of hunting cooperation and prey harvesting in a Leslie-Gower prey-predator system with strong Allee effect. AIMS Mathematics, 2024, 9(12): 34618-34646. doi: 10.3934/math.20241649 |
[7] | San-Xing Wu, Xin-You Meng . Dynamics of a delayed predator-prey system with fear effect, herd behavior and disease in the susceptible prey. AIMS Mathematics, 2021, 6(4): 3654-3685. doi: 10.3934/math.2021218 |
[8] | Jing Zhang, Shengmao Fu . Hopf bifurcation and Turing pattern of a diffusive Rosenzweig-MacArthur model with fear factor. AIMS Mathematics, 2024, 9(11): 32514-32551. doi: 10.3934/math.20241558 |
[9] | Binfeng Xie, Na Zhang . Influence of fear effect on a Holling type III prey-predator system with the prey refuge. AIMS Mathematics, 2022, 7(2): 1811-1830. doi: 10.3934/math.2022104 |
[10] | Yaping Wang, Yuanfu Shao, Chuanfu Chai . Dynamics of a predator-prey model with fear effects and gestation delays. AIMS Mathematics, 2023, 8(3): 7535-7559. doi: 10.3934/math.2023378 |
In this work, a Leslie-Gower model with a weak Allee effect on the prey and a fear effect on the predator is proposed. By using qualitative analyses, the local stability of the coexisting equilibrium and the existence of Turing instable are discussed. By analyzing the distribution of eigenvalues, the existence of a Hopf bifurcation is studied by using the gestation time delay as a bifurcation parameter. By utilizing the normal form method and the center manifold theorem, we calculate the direction of the Hopf bifurcation and the stability of bifurcating periodic solutions. We indicate that both the weak Allee effect on the prey and fear effect on the predator have an important impact on the dynamical behaviour of the new Leslie-Gower model. We also verify the obtained results by some numerical examples.
One of the most important factors in ecology is the predator-prey interaction, which is usually complex and diverse. Scholars have long been committed to using mathematical methods to explain and predict it [1,2,3,4,5,6,7]. The population dynamics of predator and prey could easily be affected in nature [8,9,10]. In this work, we investigate a self-diffusive Leslie-Gower model with a time delay, fear effect on the predator, weak Allee effect, and nonlocal competition on the prey.
Many researchers only pay attention to the direct killing of prey by predators. However, with the development of society and the advancement of biomathematics, researchers [11,12,13,14,15,16] have a deeper understanding of the interactions between predator and prey, which are complex and multiple in nature. The existence of predators not only directly affects the density and growth rate of prey populations by eating prey, but also indirectly affects prey populations by influencing their dynamical behavior. Some experiments have shown that prey will make various anti-predation responses to protect itself when it faces a predator, such as physiological changes, vigilance, foraging behavior. The phenomenon that the prey is always alert to possible attacks, is called fear effect. It is a physiological change related to the behavior and stress of a prey population in the presence of a predator.
Until now, most literatures have studied the fear effect on prey but few literatures have studied it on predator. Some scientists [17,18,19] have conducted experiments to study the fear effect on predators. They have found that the fear effect caused by a large predator could lead to a similar predation effect on medium-sized predators, leading the medium-sized predators to produce the anti-predation responses. This anti-predation behavior produced by the medium-sized predator will directly affect its consumption of prey. We can maintain the balance of the ecosystem by controlling the number of large predators to avoid excessive consumption of prey at the bottom of the food chain by the medium-sized predator.
When the density of the species population is low, the change law of the species population will be greatly affected by the internal mating of the population. W. Allee proposed the famous Allee effect for the first time [20]. Additionally, many scholars were concerned about the Allee effect in the predator-prey model [21,22,23,24,25,26,27,28,29]. Since the Allee effect usually occurs when the population density is either small or sparse. Thus, the Allee effects are strongly related to the extinction vulnerability of populations. The Allee effect is generally divided into either the strong or weak Allee effect. Whether the Allee effect is weak or strong depends on the opposing strengths of positive and negative density dependence. A strong Allee effect involves an Allee threshold. The Allee threshold is either a critical population size or a density below which the per capita population growth rate becomes negative. As mentioned in [23], the term (u−m) is added to the logistic growth function ru(1−uK) to investigate the influence of Allee threshold on prey. In [24], Courchamp revealed that studies of the strong Allee effect help support the relationship between the species populations at low densities and the population growth rate. Authors in [29] studied the following model:
{dudt=ru(1−uK)(u−m)−cuvu+kv,dvdt=ev1+kv(1−vnu). | (1.1) |
Here u and v represent the prey and predator density, respectively. K, m, c, r, and e denote the environmental capacity, Allee threshold, maximal per capita consumption rate, and the intrinsic growth rates of the prey and predator, respectively. n is a measure of food quality that the prey provides for the conversion into predator birth. k measures the fear effect on the predator.
Unlike the strong Allee effect, there is no threshold for the weak Allee effect. Many literatures [30,31,32] have investigated the ecological model with the weak Allee effect. Authors in [21] have mentioned the following model:
{dudt=ru(1−uK)uβ+u−cuvu+kv,dvdt=ev1+kv(1−vnu), | (1.2) |
where β is the weak Allee constant. Let (u,v,t)=(Kˉu,Knˉv,ˉtr) and ignore the bar. Then, Eq (1.2) becomes
{dudt=u((1−u)ub+u−qv1+pv),dvdt=sv1+pv(1−vu), | (1.3) |
where b=βK, q=Kncr, p=Knk, s=er.
In nature, due to the particularity of species breeding conditions and the necessity of gestational length, the species population density and birth rate of the species population at this stage are affected by the past period. Additionally, the energy conversion between predator and prey is not instantaneous. The influence of past history on the per capita growth rate of predators cannot be ignored [33,34,35,36]; therefore, we consider a time delay parameter τ for the predator-prey model. In addition, we assume that the distribution of population is uniform in model (1.3), which is generally not the situation in nature. In nature, due to the widespread self-diffusion phenomenon, few species populations have homogeneous spatial distribution [37,38,39]. Since the existence of diffusion phenomenon, the population model often shows some more abundant dynamic phenomena.
Another very important point is that the limited resources in nature makes the unlimited growth of species impossible, which will inevitably lead to competition among the prey population. Since the spatial distributions for the predator and prey population are inhomogeneous and disperse, this competition is usually nonlocal. Many scholars have studied the influence of it on the dynamic behavior of species [40,41,42,43,44,45,46]. In [47,48], the authors modified the uK as 1K∫ΩG(x,y)u(y,t)dy with some kernel function G(x,y) to describe this competition. Due to the above factors, we added time delay and self-diffusion terms into Eq (1.3), and considered the nonlocal competition:
{∂u(x,t)∂t=d1Δu+u((1−∫ΩG(x,y)u(y,t)dy)ub+u−qv1+pv),∂v(x,t)∂t=d2Δv+sv1+pv(1−v(t−τ)u(t−τ)),u∈Ω,t>0,∂u(x,t)∂ˉν=∂v(x,t)∂ˉν=0,x∈∂Ω,t>0,u(x,θ)=u0(x,θ)≥0,v(x,θ)=v0(x,θ)≥0,x∈ˉΩ,θ∈[−τ,0]. | (1.4) |
Here ∂u(x,t)∂t and ∂v(x,t)∂t represent the density gradients of the prey populations and predator populations, respectively. d1,d2>0 denote the diffusion coefficients of prey and predator, respectively. The notation Δ denotes the Laplace operator, and the notation Ω denotes a bounded domain with a smooth boundary ∂Ω. τ describes either a gestation period or reaction time. The integral term ∫ΩG(x,y)u(y,t)dy in the first equation of (1.4) accounts for the nonlocal competition among the prey individuals. The kernel function is of the following form:
G(x,y)=1|Ω|=1lπ,x,y∈Ω, |
which can be regarded as a measurement of the competition pressure at location x from the individuals at another location y. In this case, the competition strength among all prey individuals is the same across the habitat.
This paper mainly studies the significant effects of the weak Allee effect on prey and the fear effect on predators in the predator-prey system. We shall separately study the influence of the weak Allee effect and the fear effect on the spatial bifurcating periodic solutions.
This paper is organized as follows. In Section 2, we investigate the existence and stability of a coexisting equilibrium. In Section 3, we analyze the existence of a Hopf bifurcation. In Section 4, we consider the property of the Hopf bifurcation. In Section 5, we conduct a series of numerical simulations to illustrate the theoretical results. In Section 6, we elaborate a short conclusion.
A discussion of the equilibria has been given in [21], but for the completeness of the paper, we still give the following lemma.
Lemma 2.1. The equilibria of system (1.4) admit the following statements:
(ⅰ) The system (1.4) always has a distinct boundary equilibria given by E0(1,0) for all positive parameters.
(ⅱ) When bq>1 and
(ⅱa) if p−q−1>0 and 0<b<b∗, then system (1.4) has two positive equilibria E1(u−,v−) and E2(u+,v+),
(ⅱb) if p−q−1>0 and b=b∗, then system (1.4) has a unique positive equilibrium E3(u3,v3),
(ⅱc) if p−q−1>0, b>b∗ or p−q−1≤0, then system (1.4) has no positive equilibrium.
(ⅲ) When bq=1 and
(ⅲa) if p−q−1>0, then system (1.4) has a unique positive equilibrium E2(u+,v+),
(ⅲc) if p−q−1≤0, then system (1.4) has no positive equilibrium.
(ⅳ) When 0<bq<1, then system (1.4) has a unique positive equilibrium E2(u+,v+).
In the above, b∗=(1+p+q)2−4pq4pq, u±=v±=(p−1−q)±√(1−p+q)2−4p(bq−1)2p, and u3=v3=(p−1−q)2p. Considering the biological significance of the system equilibrium, the rest of our discussion is focused on positive equilibrium.
Furthermore, we focus on the dynamics of system (1.3) in a neighbordhood of each equilibrium. Figure 1 shows the phase portraits of system (1.3) b=0.3, q=0.9, p=0.8, and s=0.29. The "green dot" represents the boundary point E0, the "blue dot" represents the equilibrium E1, and the "red dot" represents the unique positive equilibrium E2. By calculating, we obtain the real parts of eigenvalues of equilibrium E2 of −0.195771 and −0.195771. Therefore, the equilibrium E2 is a stable node. We selected the equilibrium point E2 for numerical simulations under the confirmed biological significance.
Assume that Ω=(0,lπ) and G(x,y)=1lπ. Let N denote the positive integer set, and N0 denote the nonnegative integer set. Without loss of generality, let us say that the positive equilibrium point is E∗(u∗,v∗). Then, linearize system (1.4) at E∗(u∗,v∗).
∂∂t(u(x,t)u(x,t))=D(Δu(x,t)Δv(x,t))+J1(u(x,t)v(x,t))+J2(u(x,t−τ)v(x,t−τ))+J3(ˆu(x,t)ˆv(x,t)), | (2.1) |
where
D=(d100d2),J1=(a11a120a22),J2=(00b21b22),J3=(ˆa000), |
a11=−(1+2b)u∗2(b+u∗)2−u∗3(b+u∗)2+2u∗b+u∗−qv∗1+pv∗,a12=−qu∗(1+pv∗)2<0,a22=s(u∗−v∗)u∗(1+pv∗)2,b21=sv∗2u∗2(1+pv∗)>0,b22=−sv∗u∗(1+pv∗)<0,ˆa=−u∗2b+u∗<0, |
and ˆu=1lπ∫lπ0u(y,t)dy.
Naturally, the characteristic equation is as follows:
λ2+Anλ+Bn+(Cn−b22λ)e−λτ=0,n∈N0, | (2.2) |
where
A0=−(a11+a22+ˆa),B0=a22(ˆa+a11),C0=a11b22+ˆab22−a12b21,An=(d1+d2)n2l2−(a11+a22),Bn=d1d2n4l4−(a22d1+a11d2)n2l2+a11a22,Cn=−b22d1n2l2+a11b22−a12b21,n∈N. | (2.3) |
Then, we make the following hypothesis:
(H1)(a11+ˆa)(a22+b22)>a12b21,a11+a22+ˆa+b22<0,An−b22>0,Bn+Cn>0,for alln∈N,(H2)(a11+ˆa)(a22+b22)>a12b21,a11+a22+ˆa+b22<0,Ak−b22<0(orBk+Ck<0),for somek∈N. |
Furthermore, we come to the following situations.
Theorem 2.2. Assume τ=0. Then, the following statements are true for system (2.1).
(ⅰ) If (H1) holds, then E∗(u∗,v∗) is locally asymptotically stable.
(ⅱ) If (H2) holds, then E∗(u∗,v∗) is Turing instable.
Proof. Assume τ=0, and then (2.2) becomes to
λ2+(A0−b22)λ+(B0+C0)=0 | (2.4) |
and
λ2+(An−b22)λ+(Bn+Cn)=0,n∈N. | (2.5) |
When (H1) holds, the roots of Eqs (2.4) and (2.5) are all with negative real parts. Therefore, the equilibrium E∗(u∗,v∗) is locally asymptotically stable. When (H2) holds, the roots of Eq (2.4) are all with negative real parts, but Eq (2.5) has at least one root with positive real part. Therefore, E∗(u∗,v∗) is Turing unstable.
Lemma 2.3. If (H1) holds, then Eq (2.5) has a pair of purely imaginary roots ±iωn at τjn,j∈N0,n∈F, where
ωn=√12[−(A2n−2Bn−b222)±√(A2n−2Bn−b222)2−4(B2n−C2n)], | (2.6) |
and
τjn={1ωnarccos(V(n)cos)+2jπ,V(n)sin≥0,1ωn[2π−arccos(V(n)cos)]+2jπ,V(n)sin<0.V(n)cos=ω2(b22An+Cn)−BnCnC2n+b222ω2,V(n)sin=ω(AnCn+Bnb22−b22ω2)C2n+b222ω2,F={n|n∈M1orμn=h±}∪{n|n∈M2∖M1,μn≠h+,μn≠h−}. | (2.7) |
Proof. We suppose iω (ω>0) is a solution of Eq (2.2), which leads to
−ω2+iωAn+Bn+(Cn−b22iω)e−iωτ=0,n∈N0. |
Then, separating the real and imaginary parts, we have
{Anω=b22ωcosωτ+Cnsinωτ,ω2−Bn=Cncosωτ−b22ωsinωτ. |
Thus, we can obtain
cosωτ=ω2(b22An+Cn)−BnCnC2n+b222ω2 |
and
sinωτ=ω(AnCn+Bnb22−b22ω2)C2n+b222ω2. |
Due to cos2ωτ+sin2ωτ=1, we have
ω4+ω2(A2n−2Bn−b222)+B2n−C2n=0,n∈N0. | (2.8) |
Let m=ω2, then Eq (2.8) becomes
m2+m(A2n−2Bn−b222)+B2n−C2n=0,n∈N0. | (2.9) |
Let Pn=A2n−2Bn−b222 and Qn=B2n−C2n. The roots of Eq (2.9) are m±=12[−Pn±√P2n−4Qn]. If (H1) holds, then Bn+Cn>0(n∈N0).
Define
{h±=a22d1−b22d1+a11d2±√−4(a11a22+a12b21−a11b22)d1d2+(−a22d1+b22d1−a11d2)22d1d2,a∗=a222d21−2a22b22d21+b222d21−2a11a22d1d2+2a11b22d1d2+a211d224b21d1d2,M1={n|h−<μn<h+,n∈N},μn=n2l2,M2={n|Pn<0,P2n−4Qn≥0,n∈N}. |
Then, we have
{Bn−Cn<0,fora12<a∗,n∈M1,Bn−Cn≥0,fora12<a∗,n∉M1,Bn−Cn≥0,fora12≥a∗,n∈N. |
Based on the above analysis, we will discuss the existence of purely imaginary roots of Eq (2.5) in the following three cases.
Case 1: a12>a∗. For n∈M2, we can obtain that m±>0 if P2n−4Qn>0 and m+=m−>0 if P2n−4Qn=0. Then, Eq (2.5) has either one or two pairs of purely imaginary roots ±iωn at τjn,j∈N0, where iω±n=√m±.
Case 2: a12=a∗. For n∈M2∖M1, we can obtain that m±>0 if P2n−4Qn>0 and m+=m−>0 if P2n−4Qn=0 under the condition μn≠h+ and μn≠h−. Then, Eq (2.5) has either one or two pairs of purely imaginary roots ±iωn at τjn,j∈N0. For μn=h+ or μn=h−, the Eq (2.5) has a pair of purely imaginary roots ±iωn at τjn,j∈N0 when Pn<0 and P2n−4Qn≥0.
Case 3: a12<a∗. For n∈M2∖M1, we can obtain that m±>0 if P2n−4Qn>0 and m+=m−>0 if P2n−4Qn=0 under the condition μn≠h+ and μn≠h−. Then, Eq (2.5) has either one or two pairs of purely imaginary roots ±iωn at τjn,j∈N0. For μn=h+ or μn=h−, Eq (2.5) has a pair of purely imaginary roots ±iωn at τjn,j∈N0 when Pn<0 and P2n−4Qn≥0. For n∈M1, Eq (2.5) has a pair of purely imaginary roots ±iωn at τjn,j∈N0 when P2n−4Qn≥0.
Define
F={n|n∈M1orμn=h±}∪{n|n∈M2∖M1,μn≠h+,μn≠h−}. |
F is a finite set obviously, since
limn→∞(A2n−2Bn−b222)→+∞, |
limn→∞(Bn−Cn)→+∞. |
Lemma 2.4. If (H1) is satisfied, then Re[dλdτ|τ=τjn]>0 for n∈F, j∈N0 are true.
Proof. By Eq (2.2), we have
(dλdτ)−1=2λ+An−b22e−λτ(Cn−b22λ)λe−λτ−τλ. |
Then,
(Re(dλdτ)−1)|τ=τjn=Re(2λ+An−b22e−λτ(Cn−b22λ)λe−λτ−τλ)|τ=τjn=(1C2n+b222ω2(2ω2+A2n−2Bn−b222))|τ=τjn=(1C2n+b222ω2√(A2n−2Bn−b222)2−4(B2n−C2n))|τ=τjn>0. |
Then, we have the following theorem.
Theorem 2.5. Assume (H1) are satisfied, the following statements are true for system (1.4).
(ⅰ) E∗(u∗,v∗) is locally asymptotically stable for τ∈[0,τ∗), where τ∗=min{τ0n|n∈F}.
(ⅱ) E∗(u∗,v∗) is unstable for τ∈[τ∗,+∞).
(ⅲ) The Hopf bifurcation values of system (2.1) are τ=τjorτjn (n∈F,j∈N0).
In this section, we will give some conditions regarding the property of the Hopf bifurcation through the methods "the normal form theory" and "the center manifold theorem" in [40,41].
Denote ˜τ=τjn for j∈N0 and n∈F. Let ˉu(x,t)=u(x,τt)−u∗ and ˉv(x,t)=v(x,τt)−v∗. Then, system (1.4) (ignore the bar) becomes
{∂u∂t=τ(d1Δu+(u+u∗)((1−1lπ∫lπ0(u(y,t)+u∗)dy)(u+u∗)b+u+u∗−q(v+v∗)1+p(v+v∗))),∂v∂t=τ(d2Δv+s(v+v∗)1+p(v+v∗)(1−v(t−1)+v∗u(t−1)+u∗)). | (3.1) |
System (3.1) can be rewritten in the following form:
{∂u∂t=τ[d1Δu+a11u+a12v+ˆaˆu+α1u2+ˆα1uˆu+α2uv+α3v2+α4u3+ˆα2u2ˆu+α5uv2+α6v3]+h.o.t.,∂v∂t=τ[d2Δv+a22v+b21u(t−1)+b22v(t−1)+β1u(t−1)v+β2v2+β3u2(t−1)+β4u(t−1)v(t−1)+β5vv(t−1)+β6v3+β7v2u(t−1)+β8v2v(t−1)+β9vu2(t−1)+β10u3(t−1)+β11u2(t−1)v(t−1)]+h.o.t., | (3.2) |
where
a11=−(1+2b)u∗2(b+u∗)2−u∗3(b+u∗)2+2u∗b+u∗−qv∗1+pv∗,a12=−qu∗(1+pv∗)2,ˆa=−u∗2b+u∗,α1=−2b2(u∗−1)(b+u∗)3,ˆα1=−u∗(2b+u∗)(b+u∗)2,α2=−q(1+pv∗)2,α3=2pqu∗(1+pv∗)3,α4=6b2(u∗−1)(b+u∗)4,ˆα2=−2b2(b+u∗)3,α5=2pq(1+pv∗)3,α6=−6p2qu∗(1+pv∗)4,a22=s(u∗−v∗)u∗(1+pv∗)2,b21=sv∗2u∗2(1+pv∗),b22=−sv∗u∗+pu∗v∗,β1=sv∗(u∗+pu∗v∗)2,β2=−2ps(u∗−v∗)u∗(1+pv∗)3,β3=−2sv∗2u∗3(1+pv∗),β4=sv∗u∗2(1+pv∗),β5=−su∗(1+pv∗)2,β6=6p2s(u∗−v∗)u∗(1+pv∗)4,β7=−2psv∗u∗2(1+pv∗)3,β8=2psu∗(1+pv∗)3,β9=−2sv∗u∗3(1+pv∗)2,β10=6sv∗2u∗4(1+pv∗),β11=−2sv∗u∗3(1+pv∗). |
Define a Hilbert space
X:={(a,b)T:(a,b)∈H2(0,lπ)×H2(0,lπ),(∂a∂x,∂b∂x)|x=0,lπ=0}. |
The corresponding complexification XC has the form XC:=X⊕iX={a+ib|a,b∈X}. The complex-valued L2 inner product is provided by ⟨a,b⟩:=∫lπ0(¯a1b1+¯a2b2)dx, for a=(a1,a2)T, b=(b1,b2)T∈XC. Define a notation C:=C([−1,0],XC), which means the phase space with the sup norm, and we could write ϕt∈C, ϕt(ρ)=ϕ(t+ρ) for ρ∈[−1,0]. Let χ(1)n(a)=(γn(a),0)T, χ(2)n(a)=(0,γn(a))T and χn={χ(1)n(a),χ(2)n(a)}, where {χ(i)n(a)}(i=1,2) is an orthonormal basis of X. Define the subspace of C, which is, Bn:=span{⟨ϕ(⋅),χ(j)n⟩χ(j)n|ϕ∈C,j=1,2}, n∈N0. According to the Riesz representation theorem, there exists a 2×2 matrix function ηn(θ,˜τ) of the bounded variation for −1≤θ≤0, such that −˜τDn2l2ϕ(0)+˜τL(ϕ)=∫0−1dηn(θ,˜τ)ϕ(θ) for ϕ∈C. Define the bilinear form on C∗×C, that is,
(ψ,ϕ)=ψ(0)ϕ(0)−∫0−1∫θζ=0ψ(ζ−θ)dηn(θ,˜τ)ϕ(ζ)dζ,forϕ∈C,ψ∈C∗. | (3.3) |
Define τ=˜τ+μ. When μ=0, system Eq (3.2) undergoes a Hopf bifurcation at equilibrium (0,0), and the eigenfunctions has a pair of purely imaginary roots ±iωn0. A represents the infinitesimal generators of the semigroup with μ=0 and n=n0. The formal adjoint of A is denoted by A∗, which is under the bilinear pairing Eq (3.3). Then, define the following Boolean function:
δ(n0)={1n0=0,0n0∈N. | (3.4) |
Choose ηn0(0,˜τ)=˜τ(−n20/l2)D+˜τJ1+˜τJ3δ(nn0), ηn0(−1,˜τ)=−˜τJ2, ηn0(θ,˜τ)=0 for θ∈(−1,0). Let p(σ)=p(0)eiωn0˜τσ(σ∈[−1,0]) and q(θ)=q(0)e−iωn0˜τθ(θ∈[0,1]) be the eigenfunctions of A and A∗ corresponding to the eigenvalue iωn0˜τ, respectively. By calculation, we choose p(0)=(1,p1)T and q(0)=W(1,q2), where p1=1a12(−a11+d1n20l2−ˆaδ(n0)+iωn0), q2=−a12a22+b22eiτωn0−d2n20l2−iωn0, and W=(1+p1q2+˜τ(b21q2+b22p1q2)e−iωn0˜τ)−1. Thus, system (3.1) becomes
dU(t)dt=(˜τ+μ)DΔU(t)+(˜τ+μ)[J1(U(t))+J2U(t−1)+J3ˆU(t)]+G(μ,Ut,ˆUt), | (3.5) |
where
G(ϕ,μ)=(˜τ+μ)(α1ϕ21(0)+ˆα1ϕ1(0)ˆϕ1(0)+α2ϕ1(0)ϕ2(0)+α3ϕ22(0)+α4ϕ31(0)+ˆα2ϕ21(0)ˆϕ1(0)+α5ϕ1(0)ϕ22(0)+α6ϕ32(0)β1ϕ1(−1)ϕ2(0)+β2ϕ22(0)+β3ϕ21(−1)+β4ϕ1(−1)ϕ2(−1)+β5ϕ2(0)ϕ2(−1)+β6ϕ32(0)+β7ϕ1(−1)ϕ22(0)+β8ϕ22ϕ2(−1)+β9ϕ21(−1)ϕ2(0)+β10ϕ31(−1)+β11ϕ21(−1)ϕ2(−1)) | (3.6) |
for ϕ=(ϕ1,ϕ2)T∈C and ˆϕ1=1lπ∫lπ0ϕ1dx. Then, we decompose the space C as C=P⊕Q, where P={apγn0(x)+ˉaˉpγn0(x)|a∈C}, Q={ψ∈C|(qγn0(x),ψ)=0and(ˉqγn0(x),ψ)=0}. Thus, system (3.6) becomes Ut=f(t)p(⋅)γn0(x)+ˉf(t)ˉp(⋅)γn0(x)+ω(t,⋅) and ^Ut=1lπ∫lπ0Utdx, where
f(t)=(qγn0(x),Ut,ω(t,σ)=Ut(σ)−2Re{f(t)p(σ)γn0(x)}. | (3.7) |
Then, we get ˙f(t)=iωn0˜τf(t)+ˉq(0)<G(0,Ut),χn0>. There exists a center manifold C0 and we could write ω near (0,0) as follows:
ω(t,σ)=ω(f(t),ˉf(t),σ)=ω20(σ)f22+ω11(σ)fˉf+ω02(σ)ˉf22+⋯. | (3.8) |
Then, ˙f(t)=iωn0˜τf(t)+ϖ(f,ˉf) is the system restricted to the center manifold C0. Denote ϖ(f,ˉf)=ϖ20f22+ϖ11fˉf+ϖ02ˉf22+ϖ21f2ˉf2+⋯.
By direct computation, we have
ϖ20=2˜τW(ϑ1+q2ϑ2)I3,ϖ11=˜τW(ϱ1+q2ϱ2)I3,ϖ02=ˉϖ20, |
ϖ21=2˜τW[(κ11+q2κ21)I2+(κ12+q2κ22)I4], |
where
I2=∫lπ0γ2n0(x)dx,I3=∫lπ0γ3n0(x)dx,I4=∫lπ0γ4n0(x)dx,ϑ1=α1+ˆα1δ(n0)+α2p1+α3p21,ϑ2=β2p21+e−iτωn0(β1p1+β5p21)+e−2iτωn0(β3+β4p1),ϱ1=12α1+12ˆα1δ(n0)+14α2(¯p1+p1)+12α3¯p1p1,ϱ2=12β2¯p1p1+12β3+14β4(p1+¯p1)+14e−iτωn0(β1¯p1+β5¯p1p1)+14eiτωn0(β1p1+β5¯p1p1),κ11=2ω(1)11(0)(2α1+ˆα1δ(n0)+ˆα1+α2p1)+2ω(2)11(0)(α2+2α3p1)+ω(1)20(0)(2α1+ˆα1δ(n0)+ˆα1+α2¯p1)+ω(2)20(0)(α2+2α3¯p1)+32ˆα2δ(n0),κ12=32α4+α5¯p1p1+12α5p21+32α6¯p1p21,κ21=2ω(1)11(−1)[β1p1+(β4p1+2β3)e−iτωn0]+2ω(2)11(−1)(β4e−i˜τωn0+β5p1)+ω(1)20(−1)[(2β3+β4¯p1)ei˜τωn0+β1¯p1]+ω(2)20(−1)(β4ei˜τωn0+β5¯p1)+2ω(2)11(0)[(β1+β5p1)e−i˜τωn0+2β2p1]+ω(2)20(0)[(β1+β5¯p1)ei˜τωn0+2β2¯p1],κ22=β9p1+32β6¯p1p21+e−i˜τωn0(32β10+12β11¯p1+β11p1+β7p1¯p1+β8p21¯p1)+ei˜τωn0(12β7p21+12β8¯p1p21)+12β9¯p1e−2i˜τωn0. |
Then, we should compute ω20 and ω11. Due to Eq (3.7), we have
˙ω=˙Ut−˙fpγn0(x)−˙ˉfˉpγn0(x)=Aω+H(f,ˉf,σ), | (3.9) |
where
H(f,¯f,σ)=H20(σ)f22+H11(σ)f¯f+H02(σ)¯f22+⋯. | (3.10) |
Comparing the coefficients of Eq (3.8) with Eq (3.9), we will get
(A−2iωn0˜τI)ω20(σ)=−H20(σ),Aω11(σ)=−H11(σ). | (3.11) |
Then, we have
ω20(σ)=−ϖ20iωn0˜τp(0)Q01−ˉϖ023iωn0˜τˉp(0)Q02+Q1e2iωn0˜τσ,ω11(σ)=ϖ11iωn0˜τp(0)Q01−ˉϖ11iωn0˜τˉp(0)Q02+Q2. | (3.12) |
Denote Q01=eiωn0˜τσγn0(x), Q02=e−iωn0˜τσγn0(x), Q1=∑∞n=0Q(n)1γn0(x) and Q2=∑∞n=0Q(n)2γn0(x), and Q1 and Q2 are described as follows:
Q(n)1=(2iωn0˜τI−∫0−1e2iωn0˜τσdηn0(σ,ˉτ))−1<˜G20,χn>,Q(n)2=−(∫0−1dηn0(σ,ˉτ))−1<˜G11,χn>,n∈N0, |
<˜G20,χn>={1lπˆG20,n0≠0,n=0,12lπˆG20,n0≠0,n=2n0,1lπˆG20,n0=0,n=0,0,other, |
<˜G11,χn>={1lπˆG11,n0≠0,n=0,12lπˆG11,n0≠0,n=2n0,1lπˆG11,n0=0,n=0,0,other, |
where
ˆG20=2(ϑ1,ϑ2)T,ˆG11=2(ϱ1,ϱ2)T. |
Therefore, we have
c1(0)=i2ωn˜τ(ϖ20ϖ11−2|ϖ11|2−|ϖ02|23)+12ϖ21,μ2=−Re(c1(0))Re(λ′(˜τ)),T2=−Im(c1(0))ωn0˜τ−μ2Im(λ′(τjn))ωn0˜τ,β2=2Re(c1(0)). | (3.13) |
Theorem 3.1. For any critical value τj or τjn (n∈F,j∈N0), the following statements are true for system (1.4).
(ⅰ) If μ2>0(resp.<0), the Hopf bifurcation is forward (resp. backward).
(ⅱ) If β2<0 (resp. >0), the bifurcation periodic solutions on the center manifold C0 are orbitally asymptotically stable (resp. unstable).
(ⅲ) If T2>0 (resp. T2<0), the Hopf bifurcation period increases (resp. decreases).
We analyze the effect of the parameter b, which is related to the weak Allee effect.
Fix parameters q=0.9, p=0.8, s=0.29, d1=0.18, and d2=0.13. The bifurcation diagram of system (1.4) is given in Figure 2. From this diagram, we can obtain the relationship between the curves τ0 and τ1, and we can also obtain the intersecting point b∗ (b∗≈0.1727).
Referring to the results of Figure 2, we can also observe that the spatial homogeneous periodic solution appears when b>b∗ and τ>τ0, which may be asymptotically stable. The spatial inhomogeneous periodic solution appears when b∈(0,b∗) and τ>τ1, which may also be asymptotically stable. The stable steady state (u∗,v∗) will be reached at the rest.
Then, we select different parameter values to calculate and obtain some detailed values for properties of the Hopf bifurication (see Table 1). We found a phenomenon that a system with different parameters has different behaviors. Thus, we take six different sets of numbers and compare their different behaviors, which are summarized in Table 2. There will be three dynamic behaviors, namely asymptotically stable coexistence equilibrium (ASCE), stable spatially homogeneous periodic solutions (SSHPS), and stably spatially inhomogeneous periodic solutions (SSIPS).
b | (u∗,v∗) | τ∗ | μ2 | β2 | T2 |
0.1 | (0.581419,0.581419) | 4.62517 | 109.505 | −6.37427 | −1.64733 |
0.3 | (0.489427,0.489427) | 4.51241 | 237.19 | −15.7077 | −0.427416 |
b | τ | Model (1.4) | b | τ | Model (1.4) |
0.1 | 3 | ASCE (Figure 3) | 0.3 | 3 | ASCE (Figure 6) |
0.1 | 4.8 | SSIPS (Figure 4) | 0.3 | 4.2 | SSHPS (Figure 7) |
0.1 | 5.2 | SSIPS (Figure 5) | 0.3 | 5.2 | SSHPS (Figure 8) |
Choose b=0.1 and τ=3 (τ<τ1<τ0). The coexistence equilibrium (u∗,v∗) in system (1.4) is asymptotically stable (see the Figure 3).
Choose b=0.1 and τ=4.8 (τ1<τ<τ0). As we can see from Figure 4, the coexistence equilibrium (u∗,v∗) is unstable, the spatial homogeneous periodic solution does not exist, and the stable spatial inhomogeneous periodic solution appears first. Therefore, we classify this equilibrium as a stable spatial inhomogeneous periodic solution.
Choose b=0.1 and τ=5.2 (τ1<τ0<τ). As we can see from Figure 5, the coexistence equilibrium (u∗,v∗) is unstable, and the spatial homogeneous periodic solution appears first, though is not stable. Thus, system (1.4) has a stable spatial inhomogeneous periodic solution.
Choose b=0.3 and τ=3 (τ<τ0<τ1). As we can see from Figure 6, the coexistence equilibrium (u∗,v∗) is asymptotically stable. Comparing detail diagrams between Figures 3(b) and 6(b), we can find that the coexistence equilibrium (u∗,v∗) with a lager weak Allee effect becomes stable faster and has a smaller amplitude. Thus, we can conclude that the existence of the weak Allee effect term is beneficial to the stability of the coexistence equilibrium.
Choose b=0.3 and τ=4.2 (τ0<τ<τ1). As we can see from Figure 7, the coexistence equilibrium (u∗,v∗) is unstable, the spatial inhomogeneous periodic solution does not exist, and the stable spatial homogeneous periodic solution appears first. Therefore, we classify this equilibrium as a stable spatial homogeneous periodic solution.
Choose b=0.3 and τ=5.2 (τ0<τ1<τ). As we can see from Figure 8, the coexistence equilibrium (u∗,v∗) is unstable, and the spatial inhomogeneous periodic solution appears first, though is not stable. The system (1.4) has stable spatial homogeneous periodic solution. Comparing detail diagrams between Figures 5(b) and 8(b), we can find that the weak Allee effect term has a slight effect on the solution but does not affect its stability. The period of the coexistence equilibrium (u∗,v∗) with a lager weak Allee effect has increased and has a smaller amplitude. It can be seen that the weak Allee effect can affect the homogeneity of the periodic solution.
We analyze the effect of parameter p, which is related to the fear effect.
Fix parameters q=0.45, b=0.1, s=0.25, d1=0.13, and d2=0.2. The bifurcation diagram of system (1.4) is given in Figure 9. In the diagram, the curves of τ0 and τ1 intersect at the points p∗, where p∗≈0.3059.
Referring to the results of Figure 9, we can also observe that the spatial homogeneous periodic solution appears when p>p∗ and τ>τ0, which may be asymptotically stable. The spatial inhomogeneous periodic solution appears when p∈(0,p∗) and τ>τ1, which may also be asymptotically stable. The stable steady state (u∗,v∗) will be reached at the rest.
Then, we select different parameter values to calculate and obtain some detailed values for the Hopf bifurcation properties (see Table 3). We took six different sets of numbers and compared their behavior, which are summarized into Table 4.
p | (u∗,v∗) | τ∗ | μ2 | β2 | T2 |
0.05 | (0.666288,0.666288) | 4.02552 | 44.1971 | −3.56918 | 1.19861 |
0.56 | (0.734021,0.734021) | 7.24064 | 8.91317 | −0.222507 | −1.38483 |
p | τ | Model (1.4) | p | τ | Model (1.4) |
0.05 | 3.9 | ASCE (Figure 10) | 0.56 | 6.5 | ASCE (Figure 13) |
0.05 | 4.15 | SSIPS (Figure 11) | 0.56 | 7.01 | SSHPS (Figure 14) |
0.05 | 4.2 | SSIPS (Figure 12) | 0.56 | 7.5 | SSHPS (Figure 15) |
Choose p=0.05 and τ=3.9 (τ<τ1<τ0). As we can see from Figure 10, the coexistence equilibrium (u∗,v∗) is asymptotically stable.
Choose p=0.05 and τ=4.15 (τ1<τ<τ0). As we can see from Figure 11, the coexistence equilibrium (u∗,v∗) is unstable, the spatial homogeneous periodic solution does not exist, and the stable spatial inhomogeneous periodic solution appears first. Therefore, we classify this equilibrium as a stable spatial inhomogeneous periodic solution.
Choose p=0.05 and τ=4.2 (τ1<τ0<τ). As we can see from Figure 12, the coexistence equilibrium (u∗,v∗) is unstable, and the spatial homogeneous periodic solution appears first, though is not stable. Thus, system (1.4) has stable spatial inhomogeneous periodic solution.
Choose p=0.56 and τ=6.5 (τ<τ0<τ1). As we can see from Figure 13, the coexistence equilibrium (u∗,v∗) is asymptotically stable. Comparing detail diagrams between Figures 10(b) and 13(b), we can find that the coexistence equilibrium (u∗,v∗) with a lager fear effect becomes stable slower and has a larger amplitude. Thus, we can conclude that the existence of the fear effect term is not beneficial to the stability of the coexistence equilibrium.
Choose p=0.56 and τ=4.15 (τ0<τ<τ1). As can be seen in Figure 14, the coexistence equilibrium (u∗,v∗) is unstable, the spatial inhomogeneous periodic solution does not exist, and the stable spatial homogeneous periodic solution appears first. Therefore, we classify this equilibrium as a stable spatial homogeneous periodic solution.
Choose p=0.56 and τ=4.2 (τ0<τ1<τ). As can be seen in Figure 15, the coexistence equilibrium (u∗,v∗) is unstable, and the spatial inhomogeneous periodic solution appears first, though is not stable. The system (1.4) has a stable spatial homogeneous periodic solution. We can find that the fear effect term has a slight effect on the solution and does not affect its stability. Additionally, it can affect the homogeneity of the periodic solution.
In this work, we studied the Hopf bifurcation of a delayed diffusive predator-prey model with a weak Allee effect on prey and a fear effect on predator. By the qualitative analytical theory, we have obtained the conditions of local stability of a coexisting equilibrium and the existence of a Hopf bifurcation and Turing instable. By using the methods of the normal form theory and center manifold theorem, we have studied the property of the bifurcating periodic solutions. We found that the weak Allee effect and fear effect greatly affected the dynamical behaviour of the new Leslie-Gower model.
First, we discuss the influence of the weak Allee effect. On the whole, the weak Allee effect at a larger value has a great influence on the ecological extinction, controlling stable coexisting equilibrium, and periodic oscillation. Specifically, under the premise that the fear effect remains unchanged, when the weak Allee effect is small, a small increase is not beneficial to the stability of the coexisting equilibrium and will be easy to produce an inhomogeneous periodic solution, which is not conducive to the survival of the population. Thus, when the weak Allee effect increases, either improved defending or hiding of the prey species form the predator may be difficult, and the prey population is generally at a low density. After increasing to a certain extent, the effect will be the completely opposite, and the homogeneity of periodic solutions usually changes from inhomogeneous to homogeneous. The homogeneity is either an invariance or regularity under a particular transformation. Ecologically, the periodic solutions are homogeneous means that the members or parts of prey specie have the same dynamic behavior at all times. Thus, increasing the weak Allee effect to a certain extent undermines this invariance or regularity of the prey species, which is beneficial to the survival of the population. When the weak Allee effect is large, increasing the weak Allee effect will be beneficial to the stability of the coexisting equilibrium and will easily produce a homogeneous periodic solution, which will make the prey population increase their likelihood of extinction.
Finally, we will discuss the influence of the fear effect on population dynamics. Specifically speaking, under the premise that the weak Allee effect remains unchanged, an increase of the fear effect is not beneficial to the stability of the coexisting equilibrium. The homogeneity of periodic solutions usually changes from inhomogeneous to homogeneous, which is conducive to the survival of the population, since the fear effect on predators can protect prey and predators from being eliminated.
The authors declare they have not used Artificial Intelligence (AI) tools in the creation of this article.
This research is supported by the Fundamental Research Funds for the Central Universities (Grant No. 2572022DJ05), Harbin Science and Technology Bureau Manufacturing Innovation Talent Project (CXRC20221110393), Heilongjiang Science and Technology Department Provincial Key R & D Program Applied Research Project (SC2022ZX06C0025), Heilongjiang Science and Technology Department Provincial Key R & D Program Guidance Project (GZ20220088) and Postdoctoral program of Heilongjiang Province (No. LBHQ21060).
The authors have no conflicts of interest to declare. All co-authors have seen and agree with the contents of the manuscript and there is no financial interest to report.
[1] |
T. Faria, L. T. Magalhaes, Normal forms for retarded functional differential equations with parameters and applications to Hopf bifurcation, J. Differ. Equations, 122 (1995), 181–200. https://doi.org/10.1006/JDEQ.1995.1144 doi: 10.1006/JDEQ.1995.1144
![]() |
[2] |
T. Faria, Normal forms and Hopf bifurcation for partial differential equations with delays, Trans. Amer. Math. Soc., 352 (2000), 2217–2238. https://doi.org/10.1090/S0002-9947-00-02280-7 doi: 10.1090/S0002-9947-00-02280-7
![]() |
[3] |
F. Yi, J. Wei, J. Shi, Bifurcation and spatiotemporal patterns in a homogeneous diffusive predator-prey system, J. Differ. Equations, 246 (2009), 1944–1977. https://doi.org/10.1016/J.JDE.2008.10.024 doi: 10.1016/J.JDE.2008.10.024
![]() |
[4] |
B. Messaoud, M. B. Almatrafi, Bifurcation and stability of two-dimensional activator-inhibitor model with fractional-order derivative, Fractal Fract., 7 (2023), 344. https://doi.org/10.3390/fractalfract7050344 doi: 10.3390/fractalfract7050344
![]() |
[5] |
A. Q. Khan, S. A. H. Bukhari, M. B. Almatrafi, Global dynamics, Neimark-Sacker bifurcation and hybrid control in a Leslie's prey-predator model, Alexandria Eng. J., 61 (2022), 11391–11404. https://doi.org/10.1016/j.aej.2022.04.042 doi: 10.1016/j.aej.2022.04.042
![]() |
[6] |
A. Q. Khan, F. Nazir, M. B. Almatrafi, Bifurcation analysis of a discrete Phytoplankton-Zooplankton model with linear predational response function and toxic substance distribution, Int. J. Biomath., 16 (2022), 2250095. https://doi.org/10.1142/s1793524522500954 doi: 10.1142/s1793524522500954
![]() |
[7] |
A. Q. Khan, M. Tasneem, M. B. Almatrafi, Discrete-time COVID-19 epidemic model with bifurcation and control, Math. Biosci. Eng., 19 (2022), 1944–1969. https://doi.org/10.3934/mbe.2022092 doi: 10.3934/mbe.2022092
![]() |
[8] |
J. Li, Y. Song, Spatially inhomogeneous periodic patterns induced by distributed memory in the memory-based single population model, Appl. Math. Lett., 137 (2023), 108490. https://doi.org/10.1016/j.aml.2022.108490 doi: 10.1016/j.aml.2022.108490
![]() |
[9] |
H. Shen, Y. Song, H. Wang, Bifurcations in a diffusive resource-consumer model with distributed memory, J. Differ. Equations, 347 (2023), 170–211. https://doi.org/10.1016/j.jde.2022.11.044 doi: 10.1016/j.jde.2022.11.044
![]() |
[10] |
S. Pal, S. Majhi, S. Mandal, N. Pal, Role of fear in a predator-prey model with Beddington-DeAngelis functional response, Z. Nat. A, 74 (2019), 581–595. https://doi.org/10.1515/ZNA-2018-0449 doi: 10.1515/ZNA-2018-0449
![]() |
[11] |
E. L. Preisser, D. I. Bolnick, The many faces of fear: comparing the pathways and impacts of nonconsumptive predator effects on prey populations, PLoS ONE, 3 (2008), e2465. https://doi.org/10.1371/journal.pone.0002465 doi: 10.1371/journal.pone.0002465
![]() |
[12] |
S. Creel, D. Christianson, Relationships between direct predation and risk effects, Trends Ecol. Evol., 23 (2008), 194–201. https://doi.org/10.1016/j.tree.2007.12.004 doi: 10.1016/j.tree.2007.12.004
![]() |
[13] |
R. Yang, Q. Song, Y. An, Spatiotemporal dynamics in a predator-prey model with functional response increasing in both predator and prey densities, Mathematics, 10 (2022), 17. https://doi.org/10.3390/math10010017 doi: 10.3390/math10010017
![]() |
[14] |
M. Clinchy, M. J. Sheriff, L. Y. Zanette, Predator-induced stress and the ecology of fear, Funct. Ecol., 27 (2013), 56–65. https://doi.org/10.1111/1365-2435.12007 doi: 10.1111/1365-2435.12007
![]() |
[15] |
Y. Song, Y. Peng, T. Zhang, The spatially inhomogeneous Hopf bifurcation induced by memory delay in a memory-based diffusion system, J. Differ. Equations, 300 (2021), 597–624. https://doi.org/10.1016/J.JDE.2021.08.010 doi: 10.1016/J.JDE.2021.08.010
![]() |
[16] |
X. Wang, L. Y. Zanette, X. Zou, Modelling the fear effect in predator-prey interactions, J. Math. Biol., 73 (2016), 1179–1204. https://doi.org/10.1007/S00285-016-0989-1 doi: 10.1007/S00285-016-0989-1
![]() |
[17] |
R. Pringle, T. Kartzinel, T. Palmer, T. J. Thurman, K. Fox-Dobbs, C. C. Y. Xu, et al., Predator-induced collapse of niche structure and species coexistence, Nature, 570 (2019), 58–64. https://doi.org/10.1038/s41586-019-1264-6 doi: 10.1038/s41586-019-1264-6
![]() |
[18] |
P. Pandy, N. Pal, S. Samanta, J. Chattopadhyay, A three species food chain model with fear induced trophic cascade, Int. J. Appl. Comput. Math., 5 (2019), 100. https://doi.org/10.1007/s40819-019-0688-x doi: 10.1007/s40819-019-0688-x
![]() |
[19] |
J. P. Suraci, M. Clinchy, L. M. Dill, D. Roberts, L. Y. Zanette, Fear of large carnivores causes a trophic cascade, Nat. Commun., 7 (2016), 10698. https://doi.org/10.1038/ncomms10698 doi: 10.1038/ncomms10698
![]() |
[20] | W. C. Allee, A. Aggregations, A study in general sociology, University of Chicago Press, 1931. https://doi.org/10.2307/2961735 |
[21] |
T. Liu, L. Chen, F. Chen, Z. Li, Dynamics of a Leslie-Gower model with weak Allee effect on prey and fear effect on predator, Int. J. Bifurcation Chaos, 33 (2023), 2350008. https://doi.org/10.1142/s0218127423500086 doi: 10.1142/s0218127423500086
![]() |
[22] |
J. Jiao, C. Chen, Bogdanov-Takens bifurcation analysis of a delayed predator-prey system with double Allee effect, Nonlinear Dyn., 104 (2021), 1697–1707. https://doi.org/10.1007/s11071-021-06338-x doi: 10.1007/s11071-021-06338-x
![]() |
[23] |
P. Aguirre, A general class of predation models with multiplicative Allee effect, Nonlinear Dyn., 78 (2014), 629–648. https://doi.org/10.1007/S11071-014-1465-3 doi: 10.1007/S11071-014-1465-3
![]() |
[24] |
F. Courchamp, T. Clutton-Brock, B. Grenfell, F. Courchamp T. Clutton-Brock, B. Grenfell, et al., Inverse density dependence and the Allee effect, Trends Ecol. Evol., 14 (1999), 405–410. https://doi.org/10.1016/S0169-5347(99)01683-3 doi: 10.1016/S0169-5347(99)01683-3
![]() |
[25] |
P. Feng, Y. Kang, Dynamics of a modified Leslie-Gower model with double Allee effects, Nonlinear Dyn., 80 (2015), 1051–1062. https://doi.org/10.1007/S11071-015-1927-2 doi: 10.1007/S11071-015-1927-2
![]() |
[26] |
N. Iqbal, R. Wu, Turing patterns induced by cross-diffusion in a 2D domain with strong Allee effect, C. R. Math., 357 (2019), 863–877. https://doi.org/10.1016/j.crma.2019.10.011 doi: 10.1016/j.crma.2019.10.011
![]() |
[27] |
D. S. Boukal, L. Berec, Modelling mate-finding Allee effects and populations dynamics, with applications in pest control, Popul. Ecol., 51 (2009), 445–458. https://doi.org/10.1007/s10144-009-0154-4 doi: 10.1007/s10144-009-0154-4
![]() |
[28] |
M. H. Wang, M. Kot, Speeds of invasion in a model with strong or weak Allee effects, Math. Biosci., 171 (2001), 83–97. https://doi.org/10.1016/S0025-5564(01)00048-7 doi: 10.1016/S0025-5564(01)00048-7
![]() |
[29] |
T. Liu, L. Chen, F. Chen, Z. Li, Stability analysis of a Leslie-Gower model with strong Allee effect on prey and fear effect on predator, Int. J. Bifurcation Chaos, 32 (2022), 2250082. https://doi.org/10.1142/S0218127422500821 doi: 10.1142/S0218127422500821
![]() |
[30] |
K. Fang, Z. L. Zhu, F. D. Chen, Z. Li, Qualitative and bifurcation analysis in a Leslie-Gower model with Allee effect, Qual. Theory Dyn. Syst., 21 (2022), 86. https://doi.org/10.1007/s12346-022-00591-0 doi: 10.1007/s12346-022-00591-0
![]() |
[31] |
L. M. Zhang, Y. K. Xu, G. Y. Liao, Codimension-two bifurcations and bifurcation controls in a discrete biological system with weak Allee effect, Int. J. Bifurcation Chaos, 32 (2022), 2250036. https://doi.org/10.1142/s0218127422500365 doi: 10.1142/s0218127422500365
![]() |
[32] |
L. Zhao, J. H. Shen, Relaxation oscillations in a slow-fast predator-prey model with weak Allee effect and Holling-Ⅳ functional response, Commun. Nonlin. Sci. Numer. Simul., 112 (2022), 106517. https://doi.org/10.1016/j.cnsns.2022.106517 doi: 10.1016/j.cnsns.2022.106517
![]() |
[33] |
R. Yang, X. Zhao, Y. An, Dynamical analysis of a delayed diffusive predator-prey model with additional food provided and anti-predator behavior, Mathematics, 10 (2022), 469. https://doi.org/10.3390/math10030469 doi: 10.3390/math10030469
![]() |
[34] |
W. Zuo, J. Wei, Stability and Hopf bifurcation in a diffusive predator-prey system with delay effect, Nonlinear Anal.: Real World Appl., 12 (2011), 1998–2011. https://doi.org/10.1016/J.NONRWA.2010.12.016 doi: 10.1016/J.NONRWA.2010.12.016
![]() |
[35] |
R. Yang, D. Jin, W. Wang, A diffusive predator-prey model with generalist predator and time delay, AIMS Math., 7 (2022), 4574–4591. https://doi.org/10.3934/math.2022255 doi: 10.3934/math.2022255
![]() |
[36] |
J. F. Zhang, X. P. Yan, Effects of delay and diffusion on the dynamics of a Leslie-Gower type predator-prey model, Int. J. Bifurcation Chaos, 24 (2014), 1450043. https://doi.org/10.1142/S0218127414500436 doi: 10.1142/S0218127414500436
![]() |
[37] |
Y. Song, Y. Peng, T. Zhang, Double Hopf bifurcation analysis in the memory-based diffusion system, J. Dyn. Differ. Equ., 2022. https://doi.org/10.1007/s10884-022-10180-z doi: 10.1007/s10884-022-10180-z
![]() |
[38] |
M. U. Akhmet, M. Beklioglu, T. Ergenc, V. I. Tkachenko, An impulsive ratio-dependent predator-prey system with diffusion, Nonlinear Anal.: Real World Appl., 7 (2006), 1255–1267. https://doi.org/10.1016/j.nonrwa.2005.11.007 doi: 10.1016/j.nonrwa.2005.11.007
![]() |
[39] |
Y. Liu, J. Wei, Double Hopf bifurcation of a diffusive predator-prey system with strong Allee effect and two delays, Nonlinear Anal.: Model. Control, 26 (2021), 72–92. https://doi.org/10.15388/namc.2021.26.20561 doi: 10.15388/namc.2021.26.20561
![]() |
[40] |
Y. Liu, D. Duan, B. Niu, Spatiotemporal dynamics in a diffusive predator-prey model with group defense and nonlocal competition, Appl. Math. Lett., 103 (2019), 106175. https://doi.org/10.1016/j.aml.2019.106175 doi: 10.1016/j.aml.2019.106175
![]() |
[41] |
R. Yang, F. Wang, D. Jin, Spatially inhomogeneous bifurcating periodic solutions induced by nonlocal competition in a predator-prey system with additional food, Math. Methods Appl. Sci., 45 (2022), 9967–9978. https://doi.org/10.1002/mma.8349 doi: 10.1002/mma.8349
![]() |
[42] |
S. Chen, J. Yu, Stability and bifurcation on predator-prey systems with nonlocal prey competition, Discrete Contin. Dyn. Syst., 38 (2018), 43–62. https://doi.org/10.3934/DCDS.2018002 doi: 10.3934/DCDS.2018002
![]() |
[43] |
R. Yang, C. Nie, D. Jin, Spatiotemporal dynamics induced by nonlocal competition in a diffusive predator-prey system with habitat complexity, Nonlinear Dyn., 110 (2022), 879–900. https://doi.org/10.1007/s11071-022-07625-x doi: 10.1007/s11071-022-07625-x
![]() |
[44] |
D. Geng, W. Jiang, Y. Lou, H. Wang, Spatiotemporal patterns in a diffusive predator-prey system with nonlocal intraspecific prey competition, Stud. Appl. Math., 148 (2021), 396–432. https://doi.org/10.1111/sapm.12444 doi: 10.1111/sapm.12444
![]() |
[45] |
M. G. Clerc, D. Escaff, V. M. Kenkre, Analytical studies of fronts, colonies, and patterns: combination of the Allee effect and nonlocal competition interactions, Phys. Rev. E, 82 (2010), 036210. https://doi.org/10.1103/PHYSREVE.82.036210 doi: 10.1103/PHYSREVE.82.036210
![]() |
[46] |
Y. E. Maruvka, T. Kalisky, N. M. Shnerb, Nonlocal competition and the speciation transition on random networks, Phys. Rev. E, 78 (2008), 031920. https://doi.org/10.1103/PHYSREVE.78.031920 doi: 10.1103/PHYSREVE.78.031920
![]() |
[47] |
N. F. Britton, Aggregation and the competitive exclusion principle, J. Theor. Biol., 136 (1989), 57–66. https://doi.org/10.1016/S0022-5193(89)80189-4 doi: 10.1016/S0022-5193(89)80189-4
![]() |
[48] |
J. Furter, M. Grinfeld, Local vs. non-local interactions in population dynamics, J. Math. Biol., 27 (1989), 65–80. https://doi.org/10.1007/BF00276081 doi: 10.1007/BF00276081
![]() |
1. | Ahmad Suleman, Rizwan Ahmed, Fehaid Salem Alshammari, Nehad Ali Shah, Dynamic complexity of a slow-fast predator-prey model with herd behavior, 2023, 8, 2473-6988, 24446, 10.3934/math.20231247 | |
2. | Pallav Jyoti Pal, Gourav Mandal, Lakshmi Narayan Guin, Tapan Saha, Allee effect and hunting-induced bifurcation inquisition and pattern formation in a modified Leslie–Gower interacting species system, 2024, 182, 09600779, 114784, 10.1016/j.chaos.2024.114784 | |
3. | Muhammad Aqib Abbasi, Periodic behavior and dynamical analysis of a prey–predator model incorporating the Allee effect and fear effect, 2024, 139, 2190-5444, 10.1140/epjp/s13360-024-04909-6 |
b | (u∗,v∗) | τ∗ | μ2 | β2 | T2 |
0.1 | (0.581419,0.581419) | 4.62517 | 109.505 | −6.37427 | −1.64733 |
0.3 | (0.489427,0.489427) | 4.51241 | 237.19 | −15.7077 | −0.427416 |
p | (u∗,v∗) | τ∗ | μ2 | β2 | T2 |
0.05 | (0.666288,0.666288) | 4.02552 | 44.1971 | −3.56918 | 1.19861 |
0.56 | (0.734021,0.734021) | 7.24064 | 8.91317 | −0.222507 | −1.38483 |
b | (u∗,v∗) | τ∗ | μ2 | β2 | T2 |
0.1 | (0.581419,0.581419) | 4.62517 | 109.505 | −6.37427 | −1.64733 |
0.3 | (0.489427,0.489427) | 4.51241 | 237.19 | −15.7077 | −0.427416 |
b | τ | Model (1.4) | b | τ | Model (1.4) |
0.1 | 3 | ASCE (Figure 3) | 0.3 | 3 | ASCE (Figure 6) |
0.1 | 4.8 | SSIPS (Figure 4) | 0.3 | 4.2 | SSHPS (Figure 7) |
0.1 | 5.2 | SSIPS (Figure 5) | 0.3 | 5.2 | SSHPS (Figure 8) |
p | (u∗,v∗) | τ∗ | μ2 | β2 | T2 |
0.05 | (0.666288,0.666288) | 4.02552 | 44.1971 | −3.56918 | 1.19861 |
0.56 | (0.734021,0.734021) | 7.24064 | 8.91317 | −0.222507 | −1.38483 |
p | τ | Model (1.4) | p | τ | Model (1.4) |
0.05 | 3.9 | ASCE (Figure 10) | 0.56 | 6.5 | ASCE (Figure 13) |
0.05 | 4.15 | SSIPS (Figure 11) | 0.56 | 7.01 | SSHPS (Figure 14) |
0.05 | 4.2 | SSIPS (Figure 12) | 0.56 | 7.5 | SSHPS (Figure 15) |