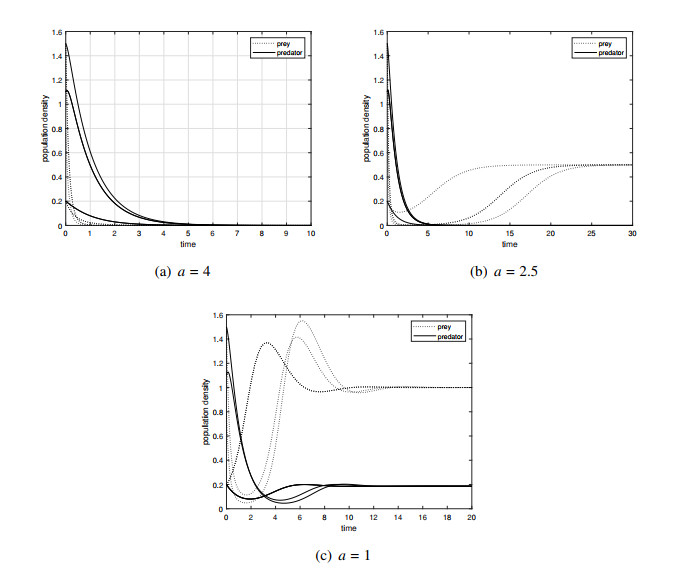
In [J. Combin. Theory Ser. B, 99 (2009), 447-454)], Li characterized the classification of vertex-transitive embeddings of complete graphs, and proposed how to enumerate such maps. In this paper, we study the counting problem of orientable vertex-transitive embeddings of Kp, where p≥5 is a prime. Moreover, we obtain the number of non-isomorphic orientable vertex-transitive complete maps with p vertices.
Citation: Xue Yu, Qingshan Zhang. Orientable vertex transitive embeddings of Kp[J]. AIMS Mathematics, 2023, 8(7): 15024-15034. doi: 10.3934/math.2023767
[1] | Afrah Ahmad Noman Abdou . Chatterjea type theorems for complex valued extended b-metric spaces with applications. AIMS Mathematics, 2023, 8(8): 19142-19160. doi: 10.3934/math.2023977 |
[2] | Mustafa Mudhesh, Hasanen A. Hammad, Eskandar Ameer, Muhammad Arshad, Fahd Jarad . Novel results on fixed-point methodologies for hybrid contraction mappings in Mb-metric spaces with an application. AIMS Mathematics, 2023, 8(1): 1530-1549. doi: 10.3934/math.2023077 |
[3] | Rashid Ali, Faisar Mehmood, Aqib Saghir, Hassen Aydi, Saber Mansour, Wajdi Kallel . Solution of integral equations for multivalued maps in fuzzy b-metric spaces using Geraghty type contractions. AIMS Mathematics, 2023, 8(7): 16633-16654. doi: 10.3934/math.2023851 |
[4] | Samina Batul, Faisar Mehmood, Azhar Hussain, Dur-e-Shehwar Sagheer, Hassen Aydi, Aiman Mukheimer . Multivalued contraction maps on fuzzy b-metric spaces and an application. AIMS Mathematics, 2022, 7(4): 5925-5942. doi: 10.3934/math.2022330 |
[5] | Zeynep Kalkan, Aynur Şahin, Ahmad Aloqaily, Nabil Mlaiki . Some fixed point and stability results in b-metric-like spaces with an application to integral equations on time scales. AIMS Mathematics, 2024, 9(5): 11335-11351. doi: 10.3934/math.2024556 |
[6] | Nehad Abduallah Alhajaji, Afrah Ahmad Noman Abdou, Jamshaid Ahmad . Application of fixed point theory to synaptic delay differential equations in neural networks. AIMS Mathematics, 2024, 9(11): 30989-31009. doi: 10.3934/math.20241495 |
[7] | Monairah Alansari, Mohammed Shehu Shagari, Akbar Azam, Nawab Hussain . Admissible multivalued hybrid Z-contractions with applications. AIMS Mathematics, 2021, 6(1): 420-441. doi: 10.3934/math.2021026 |
[8] | Amjad Ali, Muhammad Arshad, Awais Asif, Ekrem Savas, Choonkil Park, Dong Yun Shin . On multivalued maps for φ-contractions involving orbits with application. AIMS Mathematics, 2021, 6(7): 7532-7554. doi: 10.3934/math.2021440 |
[9] | Tahair Rasham, Muhammad Nazam, Hassen Aydi, Abdullah Shoaib, Choonkil Park, Jung Rye Lee . Hybrid pair of multivalued mappings in modular-like metric spaces and applications. AIMS Mathematics, 2022, 7(6): 10582-10595. doi: 10.3934/math.2022590 |
[10] | Abdullah Shoaib, Poom Kumam, Shaif Saleh Alshoraify, Muhammad Arshad . Fixed point results in double controlled quasi metric type spaces. AIMS Mathematics, 2021, 6(2): 1851-1864. doi: 10.3934/math.2021112 |
In [J. Combin. Theory Ser. B, 99 (2009), 447-454)], Li characterized the classification of vertex-transitive embeddings of complete graphs, and proposed how to enumerate such maps. In this paper, we study the counting problem of orientable vertex-transitive embeddings of Kp, where p≥5 is a prime. Moreover, we obtain the number of non-isomorphic orientable vertex-transitive complete maps with p vertices.
Population dynamics is one of the subjects that scholars discuss the most. Based on the Lotka-Volterra system, numerous theoretical studies have been developed and applied in various fields [1,2,3,4,5,6]. The behavior of both predators and prey significantly impacts the dynamics of their populations in a predator-prey system. The use of mathematical models to predict the behavior of predators and prey is of great significance, and scientists can use this as a basis to adopt new strategies to protect species. The predator-prey model is crucial for characterizing the long term evolution of both populations with certain behavior, and its importance can be seen through a large number of models. There are models established based on different theories such as ordinary differential equation [7], delay differential equation [8], partial differential equation [9], stochastic [10], cross-diffusion [11], reaction-diffusion [12], and difference equation, as well as models describing different functional responses such as Holling type [10], Holling-Tanner [12], ratio-dependence [13], Beddington-DeAngelis [7], Leslie-Gower [14], and Crowley-Martin. There are also models that depict certain behavior such as fear, cooperation, refuge [15], herd [8], solitary, and social [9,16].
All animals in nature need to pay attention to predators even those at the top of the food chain are no exception. Even plants can evade predation through direct or indirect reactions. Fear is a very powerful evolutionary force. Research in [17] has shown that using an electrical fence to prevent direct predation of prey can reduce their breeding rates by over 40% throughout an entire breeding season. Similar studies on other species of birds and vertebrates produced consistent results [18,19,20]. All of these real experiments indicate that the appearance of predators can lead to a decrease in the number of prey. Scholars have analyzed various models with the fear effect.
The authors in [21] proposed a model that modifies the Lotka-Vloterra predator-prey model by incorporating a factor related to fear into the birth rate of the prey:
{˙x=rxf(s,y)−dx−ax2−g(x)y,˙y=y(−n+cg(x)). |
They showed that the fear effect did not impact system dynamics when predators exhibited a linear response. However, they demonstrated that considering predators with the Holling-Ⅱ response and the fear factor f(s,y)=11+sy under certain conditions can stabilize the entire system. The authors also performed numerical simulations on different forms of expression of fear factors and obtained similar results.
Afterward, scholars primarily utilized the first fear factor mentioned above to study various predator-prey models. Recently, Zhu et al. proposed a model [22] where the predator is omnivorous and exhibits a linear response:
{˙x=rx1+sy−dx−ax2−pxy,˙y=cpxy+ny−d1y2. |
The dynamics of this system are significantly different from the situation without omnivorous predators. One notable development is the emergence of a new boundary equilibrium point, which is globally asymptotically stable under certain conditions (allowing predators to survive without prey). The second point is that when a positive equilibrium point exists, it is globally asymptotically stable. The third point is that the fear effect can lead to species extinction. Finally, the authors also noted that as the level of fear increases, the population density of predators and prey decreases.
Scholars have studied predator-prey models with fear effects by incorporating other species' behaviors. The authors in [23] examined a modified Leslie-Gower predator-prey model in which predators present cooperative hunting behavior. They concluded that the inclusion of a fear factor makes the model more robust than cooperative hunting alone. Sasmal in [24] introduced a strong Allee effect in the predator-prey model by incorporating a fear factor. They believed that fear does not have an impact on the dynamics of the positive equilibrium point. They also pointed out that the density of predators decreases as the strength of fear increases. Using the idea presented in [25], Lai et al. [26] studied the additive Allee effect rather than the multiplicative Allee effect. According to their findings, the multiplicative Allee effect results in less complex dynamics than the additive Allee effect. In [27], the authors also researched a model that combines the fear effect and prey refuge. More relevant work can be found in [28,29,30].
The fundamental idea of all the studies on predator-prey models relevant to the fear effect discussed above is that the perceived danger of predators by the prey only lowers the birth and survival rate of their offspring, while ignoring the influence on the mature prey's mortality rate. Nevertheless, researchers in [17,31,32] believe that the diversity of the food web or long-term physiological consequences may cause the fear effect to raise the death rate of mature prey. In the dangerous natural world, animals that are not at the top of the food chain must remain constantly vigilant against the threat of being preyed upon by native predators; even the smallest mistake could cost them their lives. These bottom-of-the-food-chain species have developed various anti-predator strategies to increase their chances of survival due to their historical fear of predators. However, this anti-predatory behavior can often be detrimental to specialized predators. Predators typically cooperate in the process of predation to facilitate their development.
Experiments have confirmed that fear can reduce the birth and death rates of the prey. Current studies of predator-prey models of the fear effect have been conducted under the assumption that fear reduces the intrinsic growth rate of the prey. We believe that if fear simultaneously reduces the birth and death rates of the prey, the intrinsic growth rate of the prey may be a more complex function of the fear factor, or even a positive function of the fear factor. It is therefore reasonable to consider the effects of fear on the birth and death rates of the prey separately, something that no academic has yet done. In this article, we aim to discuss the dynamics of the model we have proposed. The model combines fear factor and cooperative hunting, particularly emphasizing the fear factor, which not only reduces the birth rate of the prey but also increases their mortality rate. As this is our first attempt at this work, the first issue we need to address is how to construct realistic functions to describe the impact of fear on birth and mortality rates. The solution to this problem is to use the commonly used fear factor by scholars to describe the impact of fear on mortality rate, and to add an additional term with saturation effect to describe the additional mortality caused by fear. Our model is expressed as
{dxdt=rx1+s1y−a(1+s2s1y1+s1y)x−bx2−(c+ey)xy≐F1(x,y),dydt=y(−n+ε(c+ey)x)≐F2(x,y), | (1.1) |
where x(t) and y(t) represent the densities of the prey and predators, respectively. Furthermore, r, a, and b represent the birth rate, death rate, and density constraint coefficient of the prey, respectively, while c and e indicate per capita searching efficiency and degree of cooperation among predators. Additionally, n and ε represent the death rate and conversion rate of predators. Like other researchers, we use the term 11+s1y as a measure of fear to describe its impact on the birth rate, where s1 represents the level of fear of the prey towards predators. To illustrate the impact of the fear effect on mortality rate, an additional Holling-Ⅱ type functional response s2s1y1+s1y has been incorporated to account for the saturation effect, where s2 represents the maximum level of impact of fear on mortality rate. The reason for using the Holling-Ⅱ type function to describe the effect of predators on the prey mortality is that we believe that the fear function should be an increasing function of predator density with an upper bound.
Our model is more general. It can include no fear effect (s1=0), fear affecting only the birth rate (s1≠0,s2=0), and fear affecting both birth and death rates (s1≠0,s2≠0). The first two cases can be regarded as special cases of the third case, so we will mainly consider the third case here. From uniqueness, it follows that the trajectories in the first quadrant cannot cross into other quadrants. Therefore, it is sufficient to discuss only the trajectories in the first quadrant. We focus on the existence and stability of equilibrium points, as well as the impact of the fear effect and cooperative hunting on the system in Section 2. In Section 3, we discuss the different types of bifurcations in the system. In Section 4, we combine an example to illustrate the possibility of bistability in the system. In Section 5, a brief discussion is provided. The final conclusion section elucidates the impact of our findings on ecology or biology.
We mainly investigated the impact of the fear on the dynamics of the system when there is no cooperative hunting between predators and when there is cooperative hunting.
It is clear that the system (1.1) allows a equilibrium E0(0,0). In this situation, the existence of the remaining equilibrium points depends on the intrinsic growth rate (r−a) of the prey. There is a boundary equilibrium point E1(r−ab,0) if r−a>0, and a unique positive equilibrium point E∗(x∗,y∗) if r−a>bnεc, where
x∗=nεc,y∗=−k2+√k22−4k1k32k1,k1=εc2s1,k2=εc(as1s2+as1+c)+bns1,k3=bn−εc(r−a). |
Proposition 2.1. The following statements are true.
(i) E0(0,0) is a globally asymptotically stable node if r−a<0 holds, a saddle if r−a>0 holds, and a saddle-node point if r=a holds;
(ii) E1(x1,0) is a globally asymptotically stable node if 0<r−a<nbεc holds, a saddle if r−a>nbεc holds, and a saddle-node point if r−a=nbεc holds;
(iii) E∗(x∗,y∗) is a globally asymptotically stable node as long as it exists.
Proof. The Jacobian matrix of the system is given as
J=(J11J12J21J22), | (2.1) |
where
J11=r1+s1y−a(1+s1s2y1+s1y)−2bx−cy,J12=−s1(r+as2)x(1+s1y)2−cx,J21=εcy,J22=−n+εcx. |
(i) The two eigenvalues of J(E0) are r−a and −n. Clearly, E0 is a saddle if r−a>0 holds, and it is a stable node if r−a<0 holds. If r=a, by applying the time transformation dτ=−ndx and expanding the system into a power series around E0, we have
{dxdτ=bnx2+(c+rs1+rs1s2)nxy+r(1+s2)n∞∑i=1(−s1y)i+1x,dydτ=y−εcnxy. | (2.2) |
Based on the implicit function theorem, we can conclude from the equation y−εcnxy=0 that there exists only one function y=φ(x) satisfying the conditions φ(0)=φ′(0)=0. By simple calculation, we have y=φ(x)≡0. Substituting this into the first equation of the system (2.2), we get dxdτ=bnx2. As a result, according to Theorem 7.1 in [33], E0(0,0) is identified as a saddle-node point.
Because we assume that predators are specialists, it is believed that there cannot be predators if there is no prey. This indicates that the equilibrium point cannot occur on the vertical axis. One can easily demonstrate that as t approaches infinity, x(t) and y(t) converge to zero by applying relevant theories from [34]. Therefore, E0 is a globally asymptotically stable node, leading to the extinction of both species.
(ii) Through an analysis similar to E0, we can easily determine that E1 is a saddle if r−a>nbεc holds, and a stable node if r−a<nbεc holds. When r−a=nbεc, we can transform E1 to the origin by setting (z,y)=(x−x1,y), and expanding the system into a power series as follows:
{dzdt=−m1z−m1m2by−bz2+m1s21(as2+r)by2−m2yz+P(|y,z|3),dydt=εcyz, |
where m1=r−a and m2=as1s2+rs1+c. After a non-degenerate linear transformation
(zy)=(1m1m2b0−m1)(uv) |
and a time transformation τ=−m1t, the system is reduced to
{dudτ=u+bm1u2−bm21s21(as2+r)−m1m22εcb2v2+m2(b+εc)buv−Q(|u,v|3)m1≐u+R(u,v),dvdτ=−εcm1vu−εcm2bv2. |
Based on the implicit function theorem, we know from the equation u+R(u,v)=0 that there exists only one function u=φ(v) such that φ(0)=φ′(0)=0. Suppose the lowest power of the variable v is m, and its coefficient is lm, then u=φ(v)=lmvm+lm+1vm+1+⋅⋅⋅,m≥2. Therefore,
dvdτ=−εcm2bv2−εclmm1vm+1+⋅⋅⋅,m≥2. |
As a result, according to Theorem 7.1 in [33], E1(x1,0) is a saddle-node point.
If r−a<bnεc, the system will not have a positive equilibrium point. At this point, the system degenerates to dxdt=x(r−a−bx), and its positive equilibrium point is globally asymptotically stable. So, the boundary equilibrium point E1 of the system is a globally asymptotically stable node, indicating the survival of the prey and the extinction of predators.
(iii) It is not difficult to confirm that the two eigenvalues λ1 and λ2 of J(E∗) satisfy
λ1+λ2=tr(J(E∗))=−bx∗<0,λ1λ2=det(J(E∗))=cεx∗y∗(c+s1(r+as1)(1+s1y∗)2)>0. |
This indicates that both eigenvalues have negative real parts, so E∗ is a locally stable node. Now let's consider the Dulac function B(x,y)=1xy. By simple calculation, we have
∂[B(x,y)⋅F1(x,y)]∂x+∂[B(x,y)⋅F2(x,y)]∂y=−by<0,(x,y)∈(0,+∞)×(0,+∞). |
The Dulac-Bendixson theorem states that the system does not have a closed orbit, and since E∗ is the unique internal equilibrium point, every positive solution will tend toward E∗. This, along with the local stability state mentioned above, implies that it is a globally asymptotically stable node, ensuring the survival of both species.
The proposition shows that as the parameter r increases, the system's equilibria follow two paths. When r∈(0,a), E0 is the unique equilibrium point, which is a globally asymptotically stable node. When r passes through a to enter (a,a+bnεc), E0 loses its stability to a globally asymptotically stable E1; and when r further passes through a+bnεc, E1 loses its stability to a globally asymptotically stable E∗. When a new equilibrium point emerges, it will inevitably lead to the loss of stability of the previous equilibrium point, and the new equilibrium point will be globally asymptotically stable. The proposition also demonstrates that the fear effect has no impact on the stability of the system.
Theorem 2.1. The dynamics of the system depend on the intrinsic growth rate of the prey. There are two critical values of 0 and nbεc. When it is less than the first critical value, both species will become extinct. When it is between the two critical values, the prey survives while the predator becomes extinct. When it is greater than the second critical value, both species survive.
Furthermore, we note that the expression x∗=nεc is independent of the fear factor, indicating that the fear effect does not change the density of the prey. We will now focus on the effect of the fear effect on predators. First, we will examine the effect of s1 on y∗. Let
p=aεcs2+aεc+bn,q=2εc2(p−2k3), |
then,
y∗(s1)=−(ps1+εc2)+√p2s21+qs1+ε2c42εc2s1. |
Because q−εc2p=εc2(p−2k3)>0, we have
(qs1+2ε2c4)2−(εc2√p2s21+qs1+ε2c4)2=(q+εc2p)(q−εc2p)s21+3ε2c4qs1+3ε4c8>0. |
Therefore,
dy∗(s1)ds1=−qs1−2ε2c4+εc2√p2s21+qs1+ε2c44εc2s1√p2s21+qs1+ε2c4<0,lims1→+∞y∗(s1)=0. |
We also investigated the impact of s2 on y∗ and obtained similar results. For this reason, let
p1=aεcs1,p2=aεcs1+εc2+bns1, |
then,
y∗(s2)=12k1[−(p1s2+p2)+√p21s22+2p1p2s2+p22−4k1k3]. |
Therefore,
dy∗(s2)ds2=p1[−√(p1s2+p2)2−4k1k3+(p1s2+p2)]2k1√p21s22+2p1p2s2+p22−4k1k3<0,lims2→+∞y∗(s2)=0. |
The above discussion indicates that the density of predators will monotonically decrease as parameters continue to increase, eventually leading to extinction. We can observe that the presence of the fear effect only results in a shift in the location of the positive equilibrium point.
Example 2.1. Letting r=3,b=1,n=1,c=2,ε=0.5,s1=1,s2=1. Fix the parameter r, and then let a take different values so that they fall into the interval (0,2), (2,3), and (3,+∞), separately. Figure 1 shows that as a decreases, the predator and prey populations go from initial total extinction to predator-only extinction, and then to coexistence. When the birth rate of the prey is lower than the mortality rate, it will inevitably lead to its ultimate extinction. At this point, specialist predators will inevitably become extinct due to a lack of food (Figure 1(a)). When the birth rate of the prey is greater than the mortality rate, the prey will continue to survive (Figure 1(b) and 1(c)). At this point, predators may exhibit two different states. If the energy gain obtained by predators from the prey cannot offset its natural death due to the low density of the prey, the predator will still eventually become extinct (Figure 1(b)). Otherwise, predators will continue to survive (Figure 1(c)). In each case, the newly emerging equilibrium point is always globally asymptotically stable.
Letting r=3,a=1,b=1,n=1,c=2,ε=0.5. Figure 2 illustrates the influence of parameters s1 and s2 on the system. It can be seen from the figure that as the corresponding parameters increase, the density of predators will decreases, while there is no effect on the density of the prey. Figure 2(a) shows the dynamics of the system when s2=1 is fixed. This indicates that different levels of fear effects will not alter the fact that two populations coexist, nor will they alter the density of the prey. When the level of fear is fixed (s1=1), the different levels of impact of fear on prey mortality also exhibit similar results (Figure 2(b)). Furthermore, we note that when one of these two parameters is fixed, the density of predators decreases to different levels as the other parameter increases. This indicates that fear can lead to an increase in the evasion behavior of the prey, thereby reducing its probability of being preyed upon, lowering the success rate of predators, and ultimately leading to a decrease in predator density. Also, both populations take longer time to reach the final states.
We summarize the theoretical analysis above into the following Theorem 2.2.
Theorem 2.2. (i) Fear factors do not change the dynamics of the system and the density of the prey; (ii) Fear factors can lower the density of predators.
The author in [31] believed that the outcome of fear will increase the density of the prey over a long period of time. However, our research results are very different: fear does not affect the density of the prey. This may be because, although the prey avoids predation by predators, due to other factors such as environmental or resource limitations, it does not change its environmental capacity. In addition, this study has shown that fear reduces the density of predators, which can be explained by the fact that when the prey perceives fear, they choose to stay away from the predator, making the predatory process more difficult, thereby achieving the effect of reducing predator density. The partial results of Theorem 2.2 are similar to those of [21,24,26,27]. From the perspective of biological conservation, there are certain situations in which such actions should be permitted. For instance, if predators consists of an invasive species, we can manipulate specific parameters to ultimately eradicate it. However, most of the time, we want two populations to coexist to maintain the diversity of the ecosystem. We are examining the net growth rate of predator populations by increasing their cooperative hunting efforts in the hope of controlling predator numbers to some extent.
In this case, the system always has two equilibrium points E0 and E1, which are identical to those in the system without cooperative hunting. By analyzing the equilibrium points E0 and E1 in the same manner, we can establish the stability of both equilibrium points.
Next, we consider the existence of positive equilibrium points. The positive equilibrium points ˉE(ˉx,ˉy) are the solutions of the following system of equations:
{r1+s1y−a(1+s1s2y1+s1y)−bx−(c+ey)y=0,−n+ε(c+ey)x=0. | (2.3) |
From the second equation of (2.3), we know that x=nε(c+ey). By substituting it into the first equation, we know that ˉy are the positive roots of the equation
σ0y4+σ1y3+σ2y2+σ3y+σ4=0, | (2.4) |
where
σ0=εe2s1>0,σ1=εe(2cs1+e)>0,σ2=ε(c2s1+e(as1+as1s2+2c))>0,σ3=εc(as1+as1s2+c)+bns1−εe(r−a),σ4=bn−εc(r−a). |
The equation can have at most two positive roots, depending on the signs of σ3 and σ4. For this, we define the function
h(y)=σ0y4+σ1y3+σ2y2+σ3y+σ4. |
Now, we will find the positive roots of Eq (2.4) by examining the positive zero points of the function h(y). The derivative of h(y) is
h′(y)=4σ0y3+3σ1y2+2σ2y+σ3. |
If σ3≥0, then for any y>0, there is h′(y)>0. The function h(y) is monotonically increasing on the interval (0,+∞), and h(y)>h(0)=σ4. Note that as limy→+∞h(y)=+∞, the function h(y) has no positive zero root if σ4≥0, and has a single positive zero root if σ4<0.
The subsequent analysis indicates that the function h′(y) must be monotonically increasing on the interval (0,+∞). The discriminant for the quadratic equation h″(y)=12σ0y2+6σ1y+2σ2=0 is Δ=12(3σ21−8σ0σ2). If Δ>0, then the equation h″(y)=0 has two distinct real roots, denoted as y1 and y2. Both of these real roots are negative. Without loss of generality, we assume that y1<y2<0. Thus, the function h′(y) monotonically increases in the interval (y2,+∞). If Δ≤0, for any y∈R, there is h″(y)≥0. The function h′(y) monotonically increases over the interval (−∞,+∞).
If σ3<0, it is obvious that h′(0)=σ3<0. Note that as limy→+∞h′(y)=+∞, the equation h′(y)=0 always has a unique positive root, denoted as y3. In fact, y3 is the real root that always exists in the cubic equation h′(y)=0, and it has the following expression:
y3=−σ14σ0+3√−8σ20σ3−4σ0σ1σ2+σ3164σ30+√(8σ20σ3−4σ0σ1σ2+σ3164σ30)2+(8σ0σ2−3σ2148σ20)3+3√−8σ20σ3−4σ0σ1σ2+σ3164σ30−√(8σ20σ3−4σ0σ1σ2+σ3164σ30)2+(8σ0σ2−3σ2148σ20)3. |
So, the function h(y) monotonically decreases in the interval (0,y3) and monotonically increases in the interval (y3,+∞). If h(0)=σ4≤0, then the function h(y) has a single positive zero root. If h(0)>0, then the function h(y) has no positive zero root if h(y3)>0, a single positive zero root if h(y3)=0, and two distinct positive zero roots if h(y3)<0.
Proposition 2.2. We have the following statement regarding the existence of positive equilibrium points:
(i) The system does not have a positive equilibrium point if σ3≥0, σ4≥0 or if σ3<0, σ4>0, and h(y3)>0 holds.
(ii) The system has a unique positive equilibrium point if σ3≥0, σ4<0 or if σ3<0, σ4≤0, or if σ3<0, σ4>0 and h(y3)=0 holds.
(iii) The system will have two positive equilibrium points if σ3<0, σ4>0, and h(y3)<0 holds.
The E∗ must be globally asymptotically stable as long as it exists. However, the stability of the ˉE will become more complex. The Jacobian matrix of the system at ˉE is given by
J(ˉE)=(−bˉx−(s1(r+as2)(1+s1ˉy)2+c+2eˉy)ˉxnˉyˉxeεˉxˉy). |
The local stability of ˉE depends on the trace and determinant values of matrix J(ˉE), where
Tr(J(ˉE))=(−b+eεˉy)ˉx,Det(J(ˉE))=[−beεˉx2+n(s1(r+as2)(1+s1ˉy)2+c+2eˉy)]ˉy. |
We substitute ˉx=nε(c+eˉy) into Det(J(ˉE)) for convenience and use functions f(y) and g(y) to determine the signs of the trace and determinant. The expressions for f(y) and g(y) are determined by
f(y)=ε[(2ey+c)(c+ey)2(1+s1y)2+s1(r+as2)(c+ey)2]−ben(1+s1y)2,g(y)=eεy−b. |
Proposition 2.3. Suppose ˉy is the solution of Eq (2.4). Then ˉE(ˉx,ˉy) is a saddle point if f(ˉy)<0 holds, a stable node if f(ˉy)>0 and g(ˉy)<0 hold, and an unstable node if f(ˉy)>0 and g(ˉy)>0 hold.
The expression ˉx=nε(c+eˉy) gives the relationship between ˉx and ˉy. A decrease in one population's position must be accompanied by an increase in the other's position. This is not what happens when predators fail to cooperate.
To investigate the impact of parameters on the equilibrium position, we analyze ˉy=ˉy(s1,s2,e) and derive the following equation:
σ0(s1,e)ˉy4+σ1(s1,e)ˉy3+σ2(s1,s2,e)ˉy2+σ3(s1,s2,e)ˉy+σ4(e)=0. |
Taking the partial derivatives of s1 on both sides, we get
∂ˉy∂s1=−εe2ˉy4+2εceˉy3+ε(aes2+ae+c)ˉy2+(εacs2+εac+bn)ˉy4σ0ˉy3+3σ1ˉy2+2σ2ˉy+σ3≐−G1(s1)G2(s1). |
Notice that σ3 can be positive or negative. Therefore, the density of predators decreases while the density of the prey increases if G2(s1)>0. The conclusion is reversed if G2(s1)<0. Specifically, fear does not alter the density of two species if G2(s1)=0. This indicates that fear may have no effect on population density, or have a positive or negative impact.
If we consider s2 as an argument, we have
∂ˉy∂s2=−εas1(eˉy(s2+c))4σ0ˉy3+3σ1ˉy2+2σ2ˉy<0. |
As a result, the density of predators will continue to decline. Combined with the previous discussion, we know that even though an increase in the parameter s2 leads to a continuous increase in the density of predators, it will never exceed r−ab.
To look at the impact of cooperative hunting on the system, we can take partial derivatives of e on both sides,
∂ˉy∂e−2εes1ˉy4+2ε(cs1+e)ˉy3+ε(as1s2+as1+4e)ˉy2+ε(as1s2+as1−(r−a))ˉy−ε(r−a)4σ0ˉy3+3σ1ˉy2+2σ2ˉy+σ3≐−H1(e)H2(e). |
The influence of cooperative hunting on the system depends on the sign of H1(e) and H2(e). The density of predators decreases as the density of the prey increases when H1(e)H2(e)>0. The conclusion is reversed if H1(e)H2(e)<0. Specifically, if H1(e)=0, then the cooperative hunting does not affect the density of the two populations. This demonstrates that when the strength of cooperative hunting falls within a certain range, increasing the density of predators will be advantageous.
Example 2.2. Let r=3,a=1,b=1,n=1,c=2,ε=0.5,s1=1,s2=1. When the strength of hunting cooperation is small (e=2), the unique internal equilibrium point ˉE(0.8304,0.2042) is stable (Figure 3). As the strength of cooperative hunting increases, it makes the internal equilibrium point unstable and the periodic oscillation of different amplitudes appears (Figure 4(a)). When the strength of cooperative hunting is further strengthened, the periodic oscillation of the system shows a steady state, and a stable limit cycle appears (Figure 4(b)). This example indicates that cooperative hunting may be an unstable force (the internal equilibrium point unstable) or a stable force (a stable limit cycle) in the system. In addition, Figure 4(b) also shows that with the increase of parameter e, the density of predators no longer presents monotonicity, but increases first and then decreases. At this point, the blue curve in Figure 4(a) will change from right to left, indicating that the density of the prey is monotonically decreasing.
Example 2.3. Let r=3,a=1,b=1,n=1,c=2,ε=0.5,s2=1. We mainly investigate the effects of fear effects and cooperative hunting on the system when the internal equilibrium point is globally asymptotically stable. In Figure 5(a), e=0 and e=2 correspond to the red and black solution curves, and in Figure 5(b), s1=0 and s1=2 correspond to the red and black solution curves, respectively. From Figure 5(a), it can be seen that the number of prey decreases while the number of the predator slightly increases. This indicates that cooperative hunting, as a widely existing form of hunting among individuals within a species, has an extremely important significance in protecting rare or even endangered predators. As in [22], the authors obtained the result that the fear effect would simultaneously reduce the population density of both predator and prey. However, we draw an interesting and different conclusion based on the following analysis: the fear effect makes the system more stable. Figure 5(b) shows that the presence of the fear effect increases the final density of the prey and reduces the final density of predators, which makes it less likely to become extinct due to the increase in available resources possessed by each predator, despite a decrease in their final density. In addition, we believe that the increase in prey density is attributed to the fact that, although the fear effect can reduce the birth rate and increase the mortality rate of the prey, the amount of decrease in birth rate is smaller than the amount of increase in mortality rate, resulting in a higher net growth rate than the system without the fear effect. Overall, increase of fear effect and cooperative hunting makes the density of two species show the opposite trend.
Theorem 2.3. (i) Cooperative hunting can be a stable or unstable force in the system.
(ii) Both the fear and cooperative hunting may have no effect on population density, or have a positive or negative impact.
The authors of [19,23], as well as most other scholars, believe that fear reduces the densities of two species, which is significantly different from our result in Theorem 2.3. First, fear increases or decreases the density of the prey, depending on the value of its parameters, which contradicts the majority of research and common sense on fear. The reason is that, although fear simultaneously reduces the birth rate and mortality rate of the prey population, the degree of reduction varies, which leads to an increase in the net growth rate of the prey and an increase in its density. Second, the impact of fear on the density of predators and the prey will not be synchronous.
Theorem 3.1. Regardless of whether there is cooperative hunting among predators, the system experiences a transcritical bifurcation at the trivial equilibrium E0 as the parameter r passes through the bifurcation value r=rSN=a.
Proof. The proof that there is no cooperative hunting among predators is similar to having cooperative hunting. The Jacobian matrix with r=rSN at E0 is
A=DF(E0,rSN)=(000−n). |
Obviously, the matrix A has a single eigenvalue λ=0 with eigenvector V, and AT has an eigenvector W corresponding to the eigenvalue λ. After a simple calculation, we get V=W=(1,0)T, and
Fr(E0,rSN)=(0,0)T,DFr(E0,rSN)V=(1,0)T,D2F(E0,rSN)(V,V)=(−2b,0)T. |
Therefore,
WTFr(E0,rSN)=0,WT[DFr(E0,rSN)V]=1≠0,WT[D2F(E0,rSN)(V,V)]=−2b≠0. |
According to Sotomayor's theorem in [35], the system experiences a transcritical bifurcation at E0 as the parameter r crosses the bifurcation value rSN.
Theorem 3.2. Let eSN be the solution of the equation g(ˉy(e))=0. The system experiences a Hopf bifurcation around ˉE at e=eSN if Det(ˉx(e),ˉy(e))>0 and dde(Tr(ˉx(e),ˉy(e)))≠0 at e=eSN.
Proof. The secular equation of the Jacobian matrix about the equilibrium ˉE is λ2−Tr(J(ˉE))λ+Det(J(ˉE))=0. For Hopf bifurcation, the matrix J(ˉE) must have a pair of purely imaginary eigenvalues. So, the characteristic equation becomes λ2+Det(ˉx(e),ˉy(e))∣e=eSN=0. If Det(ˉx(e),ˉy(e))∣e=eSN>0, the above characteristic equation has a pair of purely imaginary eigenvalues λ1,2=±iθ0, where θ0=√Det(ˉx(e),ˉy(e))∣e=eSN. To verify the transversality condition, we consider the any neighbouring point e of eSN, the eigenvalues are λ1,2=ρ(e)±iθ(e), where
ρ(e)=Tr(ˉx(e),ˉy(e))2,θ(e)=√Det(ˉx(e),ˉy(e))−Tr2(ˉx(e),ˉy(e))4. |
Now, η=dde(ρ(e))∣e=eSN=12⋅dde(Tr(ˉx(e),ˉy(e)))∣e=eSN. Hence, the system experiences a Hopf bifurcation around ˉE at e=eSN if dde(Tr(ˉx(e),ˉy(e)))∣e=eSN≠0.
The 1st Lyapunov coefficient needs to be calculated to determine the direction of Hopf bifurcation. First, we transform ˉE into the original by letting w1=x−ˉx and w2=y−ˉy.
{dw1dt=r(w1+ˉx)1+s1(w2+ˉy)−a(1+s1s2(w2+ˉy)1+s1(w2+ˉy))(w1+ˉx)−b(w1+ˉx)2−(c+e(w2+ˉy))(w1+ˉx)(w2+ˉy),dw2dt=(w2+ˉy)(−n+ε(c+e(w2+ˉy))(w1+ˉx)). |
Expanding Taylor's series of the above system at (w1,w2)=(0,0) up to terms of order 3 produces the following system:
{dw1dt=p10w1+p01w2+p20w21+p11w1w2+p02w22+p30w31+p21w21w2+p12w1w22+p03w32+O(|w|4),dw2dt=q10w1+q01w2+q20w21+q11w1w2+q02w22+q30w31+q21w21w2+q12w1w22+q03w32+O(|w|4), | (3.1) |
where
p10=J11(ˉE),p01=J12(ˉE),q10=J21(ˉE),q01=J22(ˉE),p20=−b,p11=−s1(r+as2)(1+s1ˉy)2−(c+2eˉy),p02=s21(r+as2)ˉx(1+s1ˉy)3−eˉx,p30=0,p21=0,p12=s21(r+as2)(1+s1ˉy)3−e,p03=−s31(r+as2)ˉx(1+s1ˉy)4,q20=0,q11=ε(c+2eˉy),q02=eεˉx,q30=0,q21=0,q12=eε,q03=0. |
If 4th order and above terms are omitted, the system (3.1) can be rewritten as
dUdt=J(ˉE)U+F(U), | (3.2) |
where
U=(u1u2),F=(F1F2)=(p20w21+p11w1w2+p02w22+p30w31+p21w21w2+p12w1w22+p03w32q20w21+q11w1w2+q02w22+q30w31+q21w21w2+q12w1w22+q03w32). |
For Hopf bifurcation, the characteristic equation becomes
λ2+Det(J(ˉE))|e=eSN=0. |
λ1,2=±iθ0 are the two roots of the equation, where θ0=√Det(J(ˉE))|e=eSN if Det(J(ˉE))>0 at e=eSN. The eigenvector of matrix J(ˉE) belonging to eigenvalue iθ0 at e=eSN is ˉv=(p01,iθ0−p10). Define
A=((Re(ˉv),−Im(ˉv)))=(p010−p10−θ0). |
Let Z=A−1U, where Z=(z1,z2)T. Then, the system (3.2) becomes
dZdt=(A−1J(ˉE)A)Z+A−1F(AZ). |
This can be written as
ddt(z1z2)=(0−θ0θ00)(z1z2)+(P1(z1,z2;e=eSN)P2(z1,z2;e=eSN)). | (3.3) |
The remaining parts of P1 and P2, apart from the linear parts of z1 and z2, are given by the following expression:
P1(z1,z2;e=eSN)=1p01F1,S2(z1,z2;e=eSN)=−1θ0p01(p10F1+p01F2), |
with
F1=(p20p201−p11p01p10)z21+θ0(2p02p10−p11p01)z1z2+θ20p02z22+(p12p01p210−p03p310+p30p301−p21p201p10)z31+θ0(2p12p10p01−p21p201−3p03p210)z21z2+θ20(p12p01−3p03p10)z1z22−θ30p03z32,F2=(q20p201−q11p01p10+q02p210)z21+θ0(2q02p10−q11p01)z1z2+θ20q02z22+(q30p301+q12p01p210−q21p201p10−q03p310)z31+θ0(2q12p01p10−q21p201−3q03p210)z21z2+θ20(q12p01−3q03p10)z1z22−θ30q03z32. |
Substituting p30=p21=q20=q21=q30=q03=0 into F1 and F2, we get
F1=(p20p201−p11p01p10)z21+θ0(2p02p10−p11p01)z1z2+θ20p02z22+(p12p01p210−p03p310)z31+θ0(2p12p10p01−3p03p210)z21z2+θ20(p12p01−3p03p10)z1z22−θ30p03z32,F2=(−q11p01p10+q02p210)z21+θ0(2q02p10−q11p01)z1z2+θ20q02z22+q12p01p210z31+2θ0q12p01p10z21z2+θ20q12p01z1z22. |
The 1st Lyapunov coefficient based on the normal form (3.3) is
R=116(P1z1z1z1+P1z1z2z2+P2z1z1z2+P2z2z2z2)+116θ0[P1z1z2(P1z1z1+P1z2z2)−P2z1z2(P2z1z1+P2z2z2)−P1z1z1P2z1z1+P1z2z2P2z2z2]. |
By applying the result given in [36], Hopf bifurcation is supercritical if R<0 and it is subcritical if R>0.
Cooperative hunting may increase the number of equilibrium points in the system. Just as the Proposition 2.2 indicates, system (1.1) can have at most two positive equilibrium points. When there are two distinct positive equilibrium points, the system may exhibit bistability. The bistable state here refers to a stable limit cycle and a stable node. We found through numerical simulation that one of the positive equilibrium points is an unstable node, and there is a stable limit cycle around it. In addition, the semi-trivial equilibrium point is a locally stable node.
Example 4.1. If r=10,a=0.1,b=0.9,n=1,c=0.01,ε=0.4,s1=0.1, and s2=0.2, then the system has four equilibrium points (intersection point of solid line and dotted line in Figure 6(a)), one of which is the origin, one is the boundary equilibrium point, and two are positive equilibrium points. When the initial density of the prey is fixed (x0=15), let the initial density of predators change from 0.1 to 3.7 in steps of 0.05, and (x0,y0)=(1,2.6) and (6.6,1.6), the phase diagram of the system is obtained (Figure 6(b)). The green trajectory gradually moves away from the positive equilibrium point, indicating that the equilibrium point it surrounds is an unstable node. In addition, when the time is large enough, the green, yellow, and magenta trajectories all exhibit periodic oscillations (Figure 6(c)), indicating the existence of at least one stable limit cycle and one unstable limit cycle within the area surrounded by the magenta trajectories. We can also know from Figure 6(b) and 6(d) that the other positive equilibrium point is saddle point and the boundary equilibrium point is a locally stable node point. In brief, under this set of parameter values, predators exhibit two states: extinction or survival. The reason for the extinction of predators may be that they are unable to offset their mortality rate through predation (red trajectories) or, although predators can offset their mortality rate through predation in the short term, an increase in their numbers can lead to a sharp decline in the number of prey, making it unable to meet their own growth needs (black trajectories). In this situation, in order for two populations to coexist, the density of the prey must exceed a certain critical value (such as the first component of the right positive equilibrium point), and the initial density of the predator cannot be too small or too large (between 0.25 and 2.25). In order to understand the impact of the strength of cooperative hunting on system dynamics when there are two positive equilibrium points, while keeping other parameters remain unchanged, the strength of cooperative hunting of 5 is taken to obtain Figure 7. Just like when e=1, there are also four equilibrium points. By comparing Figures 6 and 7, we found the following differences. First, both positive equilibrium points of are unstable nodes, and the boundary equilibrium point is saddle point (Figure 7(b)). Second, all trajectories ultimately exhibit periodic solutions with the same amplitude (Figure 7(c) and 7(d)). This indicates that only one stable limit cycle exists.
As the strength of cooperative hunting increases, trajectories will shift from periodic oscillations of different amplitudes to periodic oscillations of the same amplitude, and from the existence of stable and unstable limit cycles to the existence of a unique stable limit cycle. In this sense, we can say that cooperative hunting can enhance the stability of the system. Also, a higher strength of cooperative hunting can ultimately lead to the coexistence of two species. This is because as the strength of cooperative hunting increases, the boundary equilibrium point loses its stability (saddle point), and stable periodic oscillations occur in the vicinity of it.
The system we study in this paper proposes that the perceived risk of predation in prey populations not only reduces their birth rate, but may also lead to an increase in mortality.
If only the fear is considered, the system has a relatively simple dynamic, that is, the newly emerged equilibrium point must be globally asymptotically stable. The conclusion was drawn that fear only reduces predator density, and does not affect system dynamics and prey density, which is similar to the research results of other scholars, such as [21,24,27], on the fear effect models. In this case, both species will only exist in three states, namely extinction of both, or survival of the prey and extinction of predators, or coexistence.
When predators engage in cooperative hunting, the system exhibits completely different dynamic behaviors. According to Proposition 2.2 and Example 4.1, the number of equilibrium points may increase, some of which are stable and some are not. If the positive equilibrium point is unstable, there may be a bifurcation near it, which may lead to stable or unstable limit cycles. The research results also indicate that both the fear and cooperative hunting can be a stable or unstable force in the system. When there is a fixed level of fear, increasing the strength of cooperative hunting will make the system unstable around the positive equilibrium point and experience subcritical Hopf bifurcation, resulting in limit cycles oscillations dependent on the initial values. These limit cycle oscillations are ultimately replaced by a stable limit cycle. This indicates that cooperative hunting may be an unstable force (disrupting the stability of the positive equilibrium point) or a stable force (from limit cycle oscillations to a stable limit cycle). In addition, cooperative hunting has a positive impact on predators and a negative impact on the prey, as it increases the density of predators and decreases the density of the prey. Compared to the impact of cooperative hunting on the system, the impact of the fear on the system may be exactly the opposite. For example, the fear of predators by the prey can increase its density, which is different from the results of all previous scholars, and is a new discovery in our study of the fear effect. Another new finding is that fear has an opposite effect on the densities of two species, which is different from the result in [19] in which fear synchronously reduces the densities of both species. We provide a biological explanation for the reason why fear leads to an increase in the prey density in Example 2.3.
Finally, through numerical simulations, it was found that fear prolongs the time for a species to reach a survival state and accelerates the process of extinction. Cooperative hunting can also have an impact on the progress of species.
All animals, regardless of their phyla, should pay attention to predators. Even tigers have to watch out for humans. Fear is a very powerful evolutionary force. The challenge of studying the fear effect is that even if behaviors related to fear effects can be observed, we cannot observe how fear reduces the birth and mortality rates of the prey. We can only infer its impact from a theoretical perspective. In this article, we addressed a predator-prey model of cooperative hunting in which fear simultaneously reduces the birth and mortality rates of the prey, to reveal the effects of fear and cooperative hunting on population evolution. Through discussion and analysis of a specific model, some new conclusions have been obtained. We can emphasize that both fear and cooperative hunting have the potential to have a positive effect. From the ecological point of view of our study, predators can adjust the intensity of fear through cooperative hunting or through sound, smell, etc., so as to achieve the purpose of adjusting the prey density. This dynamic adjustment may have significant implications for regional ecosystems and may even cause a "trophic cascade" effect. The focus of our work is to discuss theoretically how fear works, not whether it is real or not. Such research will provide a basis for protecting population size or reintroducing new populations.
The authors declare they have not used Artificial Intelligence (AI) tools in the creation of this article.
This work was supported by the Natural Science Foundation of China (No. 11801291), the Natural Science Foundation of Fujian Province (No. 2021J011155) and the Education and Research Project for Middle and Young Teachers of Fujian Province (No. JAT210461).
The authors declare that they have no conflicts of interest.
[1] |
N. L. Biggs, Cayley maps and symmetrical maps, Proc. Cambridge Philos. Soc., 72 (1972), 381–386. https://doi.org/10.1017/s0305004100047216 doi: 10.1017/s0305004100047216
![]() |
[2] | N. L. Biggs, Classification of complete maps on orientable surfaces, Rend. Mat.(6), 4 (1971), 645–655. |
[3] | N. L. Biggs, A. T. White, Permutation groups and combinatorial structures, vol. 33 of London Mathematical Society Lecture Note Series, Cambridge-New York: Cambridge University Press, 1979. |
[4] |
A. Devillers, W. Jin, C. H. Li, C. E. Praeger, On normal 2-geodesic transitive Cayley graphs, J. Algebraic Combin., 39 (2014), 903–918. https://doi.org/10.1007/s10801-013-0472-7 doi: 10.1007/s10801-013-0472-7
![]() |
[5] | J. D. Dixon, B. Mortimer, Permutation groups, vol. 163 of Graduate Texts in Mathematics, New York: Springer-Verlag, 1996. http://dx.doi.org/10.1007/978-1-4612-0731-3 |
[6] |
S. F. Du, J. H. Kwak, R. Nedela, Regular embeddings of complete multipartite graphs, Eur. J. Combin., 26 (2005), 505–519. https://doi.org/10.1016/j.ejc.2004.02.010 doi: 10.1016/j.ejc.2004.02.010
![]() |
[7] |
W. W. Fan, C. H. Li, The complete bipartite graphs with a unique edge-transitive embedding, J. Graph Theory, 87 (2018), 581–586. https://doi.org/10.1002/jgt.22176 doi: 10.1002/jgt.22176
![]() |
[8] |
W. W. Fan, C. H. Li, H. P. Qu, A classification of orientably edge-transitive circular embeddings of Kpe,pf, Ann. Comb., 22 (2018), 135–146. https://doi.org/10.1007/s00026-018-0373-5 doi: 10.1007/s00026-018-0373-5
![]() |
[9] |
W. W. Fan, C. H. Li, N. E. Wang, Edge-transitive uniface embeddings of bipartite multi-graphs, J. Algebr. Comb., 2 (2019), 125–134. https://doi.org/10.1007/s10801-018-0821-7 doi: 10.1007/s10801-018-0821-7
![]() |
[10] |
Y. Q. Feng, J. H. Kwak, J. X. Zhou, Enumerating reflexible 2-cell embeddings of connected graphs, Sci. China Math., 56 (2013), 933–950. https://doi.org/10.1007/s11425-012-4544-2 doi: 10.1007/s11425-012-4544-2
![]() |
[11] |
R. Jajcay, R. Nedela, Half-regular Cayley maps, Graphs Combin., 31 (2015), 1003–1018. https://doi.org/10.1007/s00373-014-1428-y doi: 10.1007/s00373-014-1428-y
![]() |
[12] | L. D. James, Imbeddings of the complete graph, Ars Combin., 16 (1983), 57–72. |
[13] |
L. D. James, Edge-symmetric orientable imbeddings of complete graphs, European J. Combin., 11 (1990), 133–144. https://doi.org/10.1016/S0195-6698(13)80067-4 doi: 10.1016/S0195-6698(13)80067-4
![]() |
[14] |
L. D. James, G. A. Jones, Regular orientable imbeddings of complete graphs, J. Combin. Theory Ser. B, 39 (1985), 353–367. https://doi.org/10.1016/0095-8956(85)90060-7 doi: 10.1016/0095-8956(85)90060-7
![]() |
[15] |
V. P. Korzhik, H. J. Voss, On the number of nonisomorphic orientable regular embeddings of complete graphs, J. Combin. Theory Ser. B, 81 (2001), 58–76. https://doi.org/10.1006/jctb.2000.1993 doi: 10.1006/jctb.2000.1993
![]() |
[16] |
V. P. Korzhik, H. J. Voss, Exponential families of non-isomorphic non-triangular orientable genus embeddings of complete graphs, J. Combin. Theory Ser. B, 86 (2002), 186–211. https://doi.org/10.1006/jctb.2002.2122 doi: 10.1006/jctb.2002.2122
![]() |
[17] |
C. H. Li, Vertex transitive embeddings of complete graphs, J. Combin. Theory Ser. B, 99 (2009), 447–454. https://doi.org/10.1016/j.jctb.2008.09.002 doi: 10.1016/j.jctb.2008.09.002
![]() |
[18] |
B. P. Mull, R. G. Rieper, A. T. White, Enumerating 2-cell imbeddings of connected graphs, Proc. Amer. Math. Soc., 103 (1988), 321–330. https://doi.org/10.2307/2047573 doi: 10.2307/2047573
![]() |
[19] |
R. B. Richter, J. Širáň, R. Jajcay, T. W. Tucker, M. E. Watkins, Cayley maps, J. Combin. Theory Ser. B, 95 (2005), 189–245. https://doi.org/10.1016/j.jctb.2005.04.007 doi: 10.1016/j.jctb.2005.04.007
![]() |
[20] |
J. Širáň, T. W. Tucker, Characterization of graphs which admit vertex-transitive embeddings, J. Graph Theory, 55 (2007), 233–248. https://doi.org/10.1002/jgt.20239 doi: 10.1002/jgt.20239
![]() |
[21] |
M. Škoviera, J. Širáň, Regular maps from Cayley graphs. Ⅰ. Balanced Cayley maps, Proc. Discrete Math., 109 (1992), 265–276. https://doi.org/ 10.1016/0012-365X(92)90296-R doi: 10.1016/0012-365X(92)90296-R
![]() |
[22] |
X. Yu, B. G. Lou, The edge-regular complete maps, Open Math., 18 (2020), 1719–1726. https://doi.org/10.1515/math-2020-0115 doi: 10.1515/math-2020-0115
![]() |
[23] | X. Yu, Enumeration of orientable vertex-transitive embeddings of Kpd. Submitted. |
[24] | X. Yu, C. H. Li, B. G. Lou, Orientable vertex primitive complete maps. Submitted. |
[25] |
X. Yu, B. G. Lou, W. W. Fan, The complete bipartite graphs which have exactly two orientably edge-transitive embeddings, Ars Math. Contemp., 18 (2020), 371–379. https://doi.org/10.26493/1855-3974.1900.cc1 doi: 10.26493/1855-3974.1900.cc1
![]() |
1. | Yalong Xue, Impact of both-density-dependent fear effect in a Leslie–Gower predator–prey model with Beddington–DeAngelis functional response, 2024, 185, 09600779, 115055, 10.1016/j.chaos.2024.115055 | |
2. | Zhanhao Zhang, Yuan Tian, Dynamics of a nonlinear state-dependent feedback control ecological model with fear effect, 2024, 9, 2473-6988, 24271, 10.3934/math.20241181 | |
3. | Na Min, Hongyang Zhang, Xiaobin Gao, Pengyu Zeng, Impacts of hunting cooperation and prey harvesting in a Leslie-Gower prey-predator system with strong Allee effect, 2024, 9, 2473-6988, 34618, 10.3934/math.20241649 | |
4. | Xinyu Meng, Lijuan Chen, Fengde Chen, Dynamics of a Predator-Prey System with Asymmetric Dispersal and Fear Effect, 2025, 17, 2073-8994, 329, 10.3390/sym17030329 | |
5. | Ivan Svynous, Vitalii Radko, Yurii Fedoruk, Tetiana Lozinska, ORGANIZATIONAL APPROACHES TO THE FORMATION OF THE MILK SALES SYSTEM AS A FACTOR IN INCREASING THE EFFICIENCY OF MILK PRODUCTION, 2024, 23091533, 98, 10.37332/2309-1533.2024.2.12 |