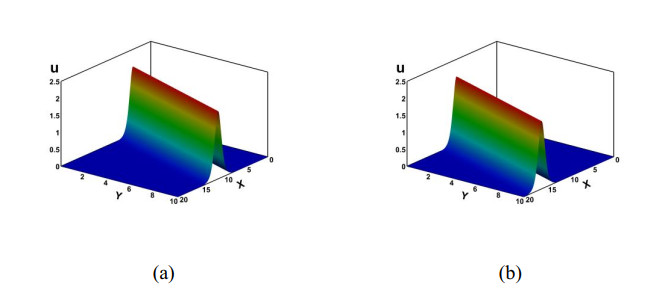
Recently, considerable attention has been given to (2+1)-dimensional Kadomtsev-Petviashvili equations due to their extensive applications in solitons that widely exist in nonlinear science. Therefore, developing a reliable numerical algorithm for the Kadomtsev-Petviashvili equations is crucial. The lattice Boltzmann method, which has been an efficient simulation method in the last three decades, is a promising technique for solving Kadomtsev-Petviashvili equations. However, the traditional higher-order moment lattice Boltzmann model for the Kadomtsev-Petviashvili equations suffers from low accuracy because of error accumulation. To overcome this shortcoming, a splitting lattice Boltzmann scheme for (2+1)-dimensional Kadomtsev-Petviashvili-Ⅰ type equations is proposed in this paper. The variable substitution method is applied to transform the Kadomtsev-Petviashvili-Ⅰ type equation into two macroscopic equations. Two sets of distribution functions are employed to construct these two macroscopic equations. Moreover, three types of soliton solutions are numerically simulated by this algorithm. The numerical results imply that the splitting lattice Boltzmann schemes have an advantage over the traditional high-order moment lattice Boltzmann model in simulating the Kadomtsev-Petviashvili-Ⅰ type equations.
Citation: Boyu Wang. A splitting lattice Boltzmann scheme for (2+1)-dimensional soliton solutions of the Kadomtsev-Petviashvili equation[J]. AIMS Mathematics, 2023, 8(11): 28071-28089. doi: 10.3934/math.20231436
[1] | Yu-Lan Ma, Bang-Qing Li . Mixed lump and soliton solutions for a generalized (3+1)-dimensional Kadomtsev-Petviashvili equation. AIMS Mathematics, 2020, 5(2): 1162-1176. doi: 10.3934/math.2020080 |
[2] | Abeer S. Khalifa, Hamdy M. Ahmed, Niveen M. Badra, Jalil Manafian, Khaled H. Mahmoud, Kottakkaran Sooppy Nisar, Wafaa B. Rabie . Derivation of some solitary wave solutions for the (3+1)- dimensional pKP-BKP equation via the IME tanh function method. AIMS Mathematics, 2024, 9(10): 27704-27720. doi: 10.3934/math.20241345 |
[3] | Wafaa B. Rabie, Hamdy M. Ahmed, Taher A. Nofal, Soliman Alkhatib . Wave solutions for the (3+1)-dimensional fractional Boussinesq-KP-type equation using the modified extended direct algebraic method. AIMS Mathematics, 2024, 9(11): 31882-31897. doi: 10.3934/math.20241532 |
[4] | Zhe Ji, Yifan Nie, Lingfei Li, Yingying Xie, Mancang Wang . Rational solutions of an extended (2+1)-dimensional Camassa-Holm- Kadomtsev-Petviashvili equation in liquid drop. AIMS Mathematics, 2023, 8(2): 3163-3184. doi: 10.3934/math.2023162 |
[5] | Gulnur Yel, Haci Mehmet Baskonus, Wei Gao . New dark-bright soliton in the shallow water wave model. AIMS Mathematics, 2020, 5(4): 4027-4044. doi: 10.3934/math.2020259 |
[6] | Junjie Li, Gurpreet Singh, Onur Alp İlhan, Jalil Manafian, Yusif S. Gasimov . Modulational instability, multiple Exp-function method, SIVP, solitary and cross-kink solutions for the generalized KP equation. AIMS Mathematics, 2021, 6(7): 7555-7584. doi: 10.3934/math.2021441 |
[7] | Weaam Alhejaili, Mohammed. K. Elboree, Abdelraheem M. Aly . A symbolic computation approach and its application to the Kadomtsev-Petviashvili equation in two (3+1)-dimensional extensions. AIMS Mathematics, 2022, 7(11): 20085-20104. doi: 10.3934/math.20221099 |
[8] | Cheng Chen . Hyperbolic function solutions of time-fractional Kadomtsev-Petviashvili equation with variable-coefficients. AIMS Mathematics, 2022, 7(6): 10378-10386. doi: 10.3934/math.2022578 |
[9] | Abdulah A. Alghamdi . Analytical discovery of dark soliton lattices in (2+1)-dimensional generalized fractional Kundu-Mukherjee-Naskar equation. AIMS Mathematics, 2024, 9(8): 23100-23127. doi: 10.3934/math.20241123 |
[10] | Khudhayr A. Rashedi, Saima Noor, Tariq S. Alshammari, Imran Khan . Lump and kink soliton phenomena of Vakhnenko equation. AIMS Mathematics, 2024, 9(8): 21079-21093. doi: 10.3934/math.20241024 |
Recently, considerable attention has been given to (2+1)-dimensional Kadomtsev-Petviashvili equations due to their extensive applications in solitons that widely exist in nonlinear science. Therefore, developing a reliable numerical algorithm for the Kadomtsev-Petviashvili equations is crucial. The lattice Boltzmann method, which has been an efficient simulation method in the last three decades, is a promising technique for solving Kadomtsev-Petviashvili equations. However, the traditional higher-order moment lattice Boltzmann model for the Kadomtsev-Petviashvili equations suffers from low accuracy because of error accumulation. To overcome this shortcoming, a splitting lattice Boltzmann scheme for (2+1)-dimensional Kadomtsev-Petviashvili-Ⅰ type equations is proposed in this paper. The variable substitution method is applied to transform the Kadomtsev-Petviashvili-Ⅰ type equation into two macroscopic equations. Two sets of distribution functions are employed to construct these two macroscopic equations. Moreover, three types of soliton solutions are numerically simulated by this algorithm. The numerical results imply that the splitting lattice Boltzmann schemes have an advantage over the traditional high-order moment lattice Boltzmann model in simulating the Kadomtsev-Petviashvili-Ⅰ type equations.
The lattice Boltzmann (LB) method, originating from lattice gas automata (LGA), is a powerful computational fluid technique that has achieved much progress in the past three decades. In an LGA model, the flow of fluids is simulated by the movement and collision of a large number of indistinguishable particles on a regular spatial lattice. The Boolean variables are used to describe the particle distribution in the system. The density and velocity of the fluids are calculated through statistical processing of these Boolean variables. Although the LGA appears promising in simulating fluid problems, it has several disadvantages due to the iteration of Boolean variables. To improve on the LGA, in a general LB model, the ensemble-averaged particle distribution functions and the linearized Bhatnagar-Gross-Krook (BGK) approximation is applied to overcome the statistical noise and exponential complexity from which the LGA suffers [1,2,3]. Among the various numerical methods, the LB model has emerged as an impressive candidate because of its efficiency and simplicity in simulating multiphase flows [4,5,6,7,8], compressible flows [9,10], turbulent flows [11,12], combustion [13,14], non-Newtonian flows [15,16] and more.
In addition, by selecting specific forms of distribution functions, the LB method has been widely employed to solve partial differential equations (PDEs) numerically, such as wave equations [17,18,19], Korteweg-de Vries (KdV) equations [20,21,22], convection-diffusion equations [23,24,25], reaction-diffusion equations [26,27], and nonlinear Schrödinger equations [28,29].
In this research, the following (2+1)-dimensional Kadomtsev-Petviashvili (KP) equation will be investigated:
∂∂x(∂u∂t+6u∂u∂x+∂3u∂x3)+γ∂2u∂y2=0. | (1) |
If the parameter is γ=−3, Eq (1) is called the Kadomtsev-Petviashvili-Ⅰ type (KPI) equation. Conversely, in the case γ=3, Equation (1) is called the Kadomtsev-Petviashvili-Ⅱ type (KPII) equation. Both the KPI and KPII equations are fully integrable. The equation was first introduced by Kadomtsev and Petviashvili to analyze the stability of one-dimensional solitary pulses under transverse perturbations [30]. Regarded as an extension of the KdV equation in two-dimensional space, Eq (1) was developed for many application areas. For example, under the assumptions of long wavelengths and micro amplitudes, the water waves are governed by KP equations [30]. Bryant found that the propagation of weakly dispersive waves can be described with KP equations [31]. The two-dimensional shallow water waves with finite amplitude can be modeled from the KP equation [32]. Moreover, Xue found that several dust-acoustic solitons can be obtained by KP equations [33].
Given a wide range of applications in nonlinear science, KP equations have attracted much attention. To date, considerable theoretical studies and numerical simulations have been performed on KP equations. For example, Kricher et al. investigated the explicit solutions of KP equations [34,35]. Zhao proposed the extended mapping method to derive several exact solutions of KP equations and analyzed the interactions among different solitary waves [36]. Qin et al. introduced the extended homoclinic test method to study the breather wave and rogue wave solutions of the KP equations, which are important for describing several phenomena in plasma physics and fluid mechanics [37]. The Hirota bilinear method, which can be used to find the analytical solutions of PDEs [38,39], has been widely used to develop both rational and rogue wave solutions of KP equations [40,41,42,43,44]. The variable-coefficient symbolic computation method is carried out to obtain several multi-rogue wave solutions of the KP equation [45]. Using the generalized extended tanh method combined with the F-expansion method, several solitary wave solutions of the KP equations were exploited by Seadawy et al. [46]. The explicit finite difference model for the initial or boundary-value problems of KP equations were developed by Bratsos and Twizell [47]. To improve the stability of the algorithm, Feng and Mitsui utilized a linearized implicit finite difference method based on the Crank-Nicolson scheme to solve KP equations [48]. The pseudo-spectral method was carried out by Minzoni and Smyth to analyze the evolution of lump solitons of KP equations [49]. A computational approach based on the Adomian decomposition method was developed by Wazwaz to study the movement of solitons in KP equations [50]. A higher-order moment LB method was constructed by Wang to simulate single-line solitons and lump solitons in KP equations [51]. Cai et al. proposed a linearized local energy-preserving method to analyze the evolution of solitons in KP equations [52].
Notably, although the higher-order moment LB model presented by Wang successfully simulated the evolution of KP equations [51], the error of the model was unsatisfactory. The macroscopic quantity of the model was selected as Eq (2):
∂u∂x=∑αfeqα(x,y,t). | (2) |
Thus, the true macroscopic quantity u must be obtained by the central difference in the x direction. This could cause error accumulation, which reduces the accuracy of the model. In this research, a more reliable numerical scheme for the KPI equation based on the LB model will be developed.
Inspired by [49], to achieve the aim of solving KPI equations more effectively, a splitting LB scheme is proposed in this paper. First, Eq (1) is translated as follows:
∂u∂t+6u∂u∂x+∂3u∂x3=Kw, | (3) |
∂w∂x=δK∂2u∂y2, | (4) |
where δ=−γ. We can see that Eq (3) is a KdV equation with the source term Kw.
It is reasonable to rewrite the KPI equation into Eqs (3) and (4). On one hand, as an extension of the KdV equation in two-dimensional space, the (2+1)-dimensional KP equation is derived from one-dimensional solitary pulses under transverse perturbations [30]. Equation (4) describes the second-order derivative term in the y-direction as transverse perturbations, which becomes the source term for the movement of solitons governed by the KdV equation in the x-direction. On the other hand, Beji [53] noted that in the nonlinear water wave equation regarding surface displacement, the KP equation can be obtained by taking the x-direction as the main propagation direction of waves, discarding the nonlinear and dispersive terms in the y-direction and retaining only the non-dispersive term in the y-direction. This point of view also verifies the rationality of Eqs (3) and (4).
The KdV equations with the source term were first calculated by Fornberg and Whitham [54]. In their work, the pseudo-spectral method combined with the leap-frog scheme was used. Meanwhile, the form of Eq (4) is similar to a diffusion-type equation. Although the characteristic of Eq (4) is different from the diffusion equation because the quantities u and w are not linearly related, Eq (4) can be integrated by using the Crank-Nicolson scheme.
In this paper, we focus on the LB solutions for these two equations. Our strategy is that two sets of distribution functions will be employed to recover these equations. A one-dimensional LB model is used to simulate the KdV equation with a source term, and a spatial evolution LB model is applied to develop (4).
The remainder of this research is constructed as follows. In Section 2, a splitting LB scheme for KPI equations is proposed. In Section 3, some detailed numerical simulations are conducted to verify the scheme. Finally, conclusions are provided in the last section.
A one-dimensional LB equation with a BGK collision term is considered as follows:
fα(x+eαδt,t+δt)−fα(x,t)=−1τ[fα(x,t)−feqα(x,t)]+ωα(x,t), | (5) |
where the relaxation time is denoted as τ. fα(x,t) and feqα(x,t) represent the discrete distribution function and equilibrium distribution function in the α direction, respectively. They should satisfy the following conservation law:
∑αfeqα(x,t)=∑αfα(x,t). | (6) |
The additional term ωα(x,t) is used to simulate the source term of the macroscopic equation.
Now, applying the Chapman-Enskog expansion [55] to fα(x,t), it yields:
fα(x,t)=∞∑i=0εif(i)α(x,t). | (7) |
Here, in Eq (7), f(0)α(x,t)≡feqα(x,t). The parameter ε is called the Knudsen number. It is reasonable to assume that ε is a small number. Therefore, we can take it as the time step: ε=δt [56]. Thus, the LB equation becomes:
fα(x+εeα,t+ε)−fα(x,t)=−1τ[fα(x,t)−feqα(x,t)]+ωα(x,t). | (8) |
Since the Knudsen number ε is small, we can employ the Taylor expansion to the left-hand side of the LB equation:
fα(x+εeα,t+ε)−fα(x,t)=∞∑i=1εii!(∂∂t+∂∂xeα)ifα(x,t). | (9) |
Moreover, the multiscale expansion to the additional term ωα(x,t) is obtained as follows:
ωα(x,t)=∞∑i=1εiω(i)α(x,t). | (10) |
To quantify changes in the LB equation at different time scales, the following is introduced:
ti=εit,i=0,1,⋯ | (11) |
and
∂∂t=∞∑i=0εi∂∂ti. | (12) |
Next, Eqs (7), (9), (10) and (12) are substituted into Eq (8), and the terms are separated based on the orders of ε:
C1Δf(0)α=−1τf(1)α+ω(1)α, | (13) |
C2Δ2f(0)α+∂∂t1f(0)α+Δτω(1)α=−1τf(2)α+ω(2)α, | (14) |
C3Δ3f(0)α+2C2Δ∂∂t1f(0)α+∂∂t2f(0)α+τ∂∂t1ω(1)α+C2τΔ2ω(1)α+τΔω(2)α=−1τf(3)α+ω(3)α, | (15) |
C4Δ4f(0)α+3C3Δ2∂∂t1f(0)α+2C2Δ∂∂t2f(0)α+∂∂t3f(0)α+C2∂2∂t21f(0)α+τ∂∂t2ω(1)α+∂∂t1[2C2Δτω(1)α+τω(2)α]+τC3Δ3ω(1)α+τC2Δ2ω(2)α+τΔω(3)α=−1τf(4)α+ω(4)α. | (16) |
In the above equations, Δ=∂/∂t0+eα⋅∂/∂x is a kind of partial differential operator. Ci are several polynomials related to τ:
Ci=i−1∑n=1−τCn(i−n)!+1i!. i=1,2,3,4. | (17) |
To recover the KdV equation with the source term, u(x,t) is considered as:
u(x,t)=∑αfα(x,t). | (18) |
Due to Eq (6), the distribution functions take the following forms:
∑αf(0)α(x,t)=u(x,t) | (19) |
and
∑αf(i)α(x,t)=0.i=1,2,⋯ | (20) |
Now, the following functions of f(0)α(x,t) are selected:
m(x,t)=∑αf(0)α(x,t)eα=3u2, | (21) |
π(x,t)=∑αf(0)α(x,t)e2α=12u3, | (22) |
P(x,t)=∑αf(0)α(x,t)e3α=54u4+χu, | (23) |
Q(x,t)=∑αf(0)α(x,t)e4α=12965u5+12χu2. | (24) |
In Eqs (23) and (24), the parameter χ=1/ε2C3 can be determined. These functions are equivalent to those in [20].
By taking a summation (13)+(14)×ε+(15)×ε2+(16)×ε3 with respect to α, we can obtain the following macroscopic equation:
∂u∂t+6u∂u∂x+∂3u∂x3=Kw+O(ε4). | (25) |
This is the fourth-order accuracy KdV equation with a source term, in which the recovery of the source term Kw depends on the selection of additional distribution functions ω(i)α(x,t). More detailed information regarding this aspect can be found in Appendix A.
Equations (21)–(24) can be simply used to determine feqα(x,t) by solving linear algebraic equations. For a one-dimensional five-bit lattice (D1Q5) where the discrete velocities are selected as eα={e0,e1,e2,e3,e4}={0,c,−c,2c,−2c}, the equilibrium distribution functions are:
feq1(x,t)=16c4(4mc3+4πc2−Pc−Q), | (26) |
feq2(x,t)=16c4(−4mc3+4πc2+Pc−Q), | (27) |
feq3(x,t)=124c4(−2mc3−πc2+2Pc+Q), | (28) |
feq4(x,t)=124c4(2mc3−πc2−2Pc+Q), | (29) |
feq0(x,t)=u(x,t)−4∑α=1feqα(x,t). | (30) |
For the purpose of simulating Eq (4), we consider the following spatial evolution LB equation with the BGK collision term:
gβ(y + eβδx,x+δx)−gβ(y,x)=−1˜τ[gβ(y,x)−geqβ(y,x)]. | (31) |
In Eq (31), ˜τ is a new relaxation time. δx is the spatial step in the x-direction. gβ(y,x) and geqβ(y,x) are the distribution function and equilibrium distribution function at position (y,x) with velocity eβ, respectively. They satisfy the following conservation condition:
∑βgeqβ(y,x)=∑βgβ(y,x). | (32) |
Now, introducing a new dimensionless parameter ˜ε that numerically equals δx. Then, Eq (31) can be rewritten as:
gβ(y + eβ˜ε,x+˜ε)−gβ(y,x)=−1˜τ[gβ(y,x)−geqβ(y,x)]. | (33) |
Next, the Taylor expansion
gβ(y + eβ˜ε,x+˜ε)−gβ(y,x)=∞∑j=1˜εnj!(∂∂x+eβ∂∂y)jgβ(y,x) | (34) |
and the Chapman–Enskog expansion [55]:
gβ(y,x)=∞∑j=0˜εjg(j)β(y,x) | (35) |
can be adopted when ˜ε is a small parameter. In (35), g(0)β(y,x)≡geqβ(y,x). By denoting different spatial scales x0, x1, ..., they are
xj=˜εjx,j=0,1,⋯ | (36) |
∂∂x=∞∑j=0˜εj∂∂xj. | (37) |
Substitute Eqs (34), (35) and (37) into Eq (33) and separate terms based on different orders of ˜ε:
˜Δg(0)β=−1˜τg(1)β, | (38) |
∂∂x1g(0)β+˜C2˜Δ2g(0)β=−1˜τg(2)β, | (39) |
where ˜C2=0.5−˜τ and the partial differential operator is ˜Δ=∂/∂x0+eβ⋅∂/∂y.
The macroscopic quantity w(y,x) in Eq (4) is defined as
w(y,x)=∑βgβ(y,x). | (40) |
By reverting back to Eqs (32) and (35), we can obtain
w(y,x)=∑βg(0)β(y,x) | (41) |
and
∑βg(j)β=0.j=1,2,⋯ | (42) |
Furthermore, several functions related to g(0)β(y,x) are selected as:
˜m(y,x)=∑βg(0)β(y,x)eβ=0, | (43) |
˜π(y,x)=∑βg(0)β(y,x)e2β=−δuK˜ε˜C2. | (44) |
By taking (38)+(39)טε and summing about β, we can obtain the following:
∂w∂x+∂˜m∂y+˜C2˜ε[∂∂x0(∂w∂x0+∂˜m∂y)+∂∂y(∂˜m∂x0+∂˜π∂y)]=O(˜ε2). | (45) |
Due to Functions (43), (44) and Eq (38), Eq (4) with second-order accuracy of truncation error can be recovered:
∂w∂x=δK∂2u∂y2 + O(˜ε2). | (46) |
For the D1Q5 lattice where the discrete velocities are selected as eβ={˜e0,˜e1,˜e2,˜e3,˜e4}={0,˜c,−˜c,2˜c,−2˜c}, the equilibrium distribution functions can be derived by combining Eqs (41), (43) and (44). They are represented as follows:
geqβ(y,x)=λu6˜c2,β=1,2 | (47) |
geqβ(y,x)=λu12˜c2,β=3,4 | (48) |
geq0(y,x)=w−λu2˜c2. | (49) |
Here, the parameter is λ=−δ/(K˜ε˜C2).
In this section, three numerical experiments are proposed to verify the model.
First, we consider the single-line soliton solution of the KPI equation:
u(x,y,t)=2ξ2sech2{ξ[x+ηy−(4ξ2−3η2)t−x0]}. | (50) |
The following initial condition is employed:
u(x,y,0)=2ξ2sech2[ξ(x+ηy−x0)]. | (51) |
Equation (50) describes the propagation process of a single-line soliton wave. The propagation speed is 4ξ2−3η2, and arctan(−η−1) is the angle between the propagation direction and the positive x-axis [48].
The initial condition and the splitting LB result when t=2 are shown in Figure 1. The parameters are as follows: δt=0.0005, lattice size 200×100, τ=1.3, ˜τ=1.0, c=200, ˜c=1, γ=−3, ξ=1.0, η=−1.0/√2, x0=4.0, K=200.0, δx=0.1 and δy=0.1. This numerical simulation is carried out on the domain 20×10, and the exact Dirichlet boundary conditions are applied.
Additionally, with the parameters given in Figure 1, the comparison between the theoretical solutions and splitting LB results at y=5.0 when t=1.0 is presented in Figure 2(a), from which it can be seen that the splitting LB results are in good agreement with the exact solutions.
The relative errors at line y=5.0 when t=1.0 are also plotted in Figure 2(b). Here, the relative errors are defined as e=|uN−uE|/|uE|, in which uN is the splitting LB solution and uE is the exact solution. Figure 2(b) illustrates that the relative errors are lower than 1.5×10−5. The result demonstrates that the splitting LB solutions are acceptable.
The comparison of the general relative errors G between the splitting LB model and the traditional higher-order moment LB model is presented in Table 1. Here, the general relative errors G are defined as:
G=∑|uN−uE|∑|uE|. | (52) |
Splitting LB | Higher-order moment LB [51] | Linearized local energy-preserving method [52] | |
t = 1 | 7.432065×10−6 | 6.932046×10−2 | 2.607653×10−2 |
t = 2 | 6.942704×10−6 | 8.490834×10−2 | 2.119188×10−2 |
t = 3 | 7.547621×10−6 | 9.748673×10−2 | 3.286301×10−2 |
t = 4 | 6.783254×10−6 | 1.079479×10−1 | 2.955938×10−2 |
From Table 1, it can be clearly seen that the general relative errors of the splitting LB scheme slightly fluctuate but are consistently lower than 1.0×10−5. However, the general relative errors of the traditional higher-order moment LB model are approximately 10−1, which is significantly higher than the G in the splitting LB scheme. Furthermore, in the higher-order moment LB model, the general relative errors increase over time. This is due to the error accumulation of the model.
With time step δt=0.01, other parameters are the same as those in LB models, the general relative errors of the linearized local energy-preserving method [52] are also presented in Table 1, from which we can observe that the errors of the splitting LB scheme are also lower than those of the linearized local energy-preserving method.
In addition, the CPU times of these three numerical methods are considered. Here, the experiment is conducted on a computer with an Intel i5 (2.50 GHz) processor, and the computing program is written in Fortran and compiled in Gfortran. The CPU times of the splitting LB scheme, traditional higher-order moment LB model and linearized local energy-preserving method are 33.73s, 36.97s and 22.19s, respectively. Here, we can see that due to the large time step, the linearized local energy-preserving method has the lowest CPU time. The CPU time of the splitting LB scheme is lower than that of the higher-order moment LB model.
From the discussion above we can see that the splitting LB scheme has an advantage over the traditional high-order moment LB model in simulating KPI equations.
For the purpose of testing the convergence order of the splitting LB scheme, the relation between the general relative errors G and spatial step δx is shown in Figure 3(a). To compute the numerical convergence order, the linear fit is also adopted. The linear fit line is log10G=2.5547×log10δx +0.03765, which means that the model's convergence order is 2.5547 in space.
To quantify the order of the truncation error, we introduce another parameter ρ=e/(δx)2. Here, δx is the spatial step in the x-direction, and e is the absolute error. Parameter ρ represents the scale factor of the truncation error e and the square of the spatial step (δx)2. In Figure 3(b), the curve of ρ at times from t=0.0 to t=1.0 is plotted. From the graph, we can observe that ρ is a finite small number in the range of [0.12,0.16] in this example, which indicates that the splitting LB scheme in this paper has a second-order accuracy of the truncation error.
We consider the KPI equation with the following initial condition:
u(x,y,0)=22∑i=1ξ2isech2{ξi[x+ηiy−x0,i]}. | (53) |
This problem has the following theoretical solution:
u(x,y,t)=22∑i=1ξ2isech2{ξi[x+ηiy−(4ξ2i−3η2i)t−x0,i]}. | (54) |
The computational domain is [0,40]×[0,40], and the exact Dirichlet boundary conditions are used in this experiment.
In Figure 4, the splitting LB solutions at different times t=0, t=3, t=5 and t=8 are carried out. The parameters are as follows: δt=0.0005, lattice size 200×100, τ=1.3, ˜τ=1.0, c=400, ˜c=2, γ=−3, ξ1=1.0, ξ2=1.0/√2, η1=−1.0/√3, η2=−1.0, x0,1=4.0, x0,2=14.0, K=200.0, δx=0.2 and δy=0.4. Figure 4(a) shows the initial condition Eq (53) in which two line solitons are allowed a collision to take place. Figure 4(b) demonstrates that two solitons collide when t=3. In Figure 4(c), when t=5, we find that the solitons collide at y>10. Finally, in Figure 4(d), the two solitons have separated completely when t=8. The simulation results in Figure 4 are consistent with the classical results [48].
To simulate lump soliton, the KPI equation with the following initial condition is given:
u(x,y,0)=4[−(x−x0)2+μ2(y−y0)2+1/μ2][(x−x0)2+μ2(y−y0)2+1/μ2]2. | (55) |
The theoretical solution to this problem is as follows:
u(x,y,0)=4[−(x−x0)2+μ2(y−y0)2+1/μ2][(x−x0)2+μ2(y−y0)2+1/μ2]2. | (56) |
which shows that the lump soliton will propagate along the x-direction at a speed of 3μ2. In this case, the exact Dirichlet boundary conditions are considered with the computation domain: [0,20]×[0,20].
In Figure 5(a), the initial condition is shown. The parameters are as follows: δt=0.0005, lattice size 200×100, τ=1.3, ˜τ=1.0, c=200, ˜c=2, γ=−3, μ=1.0, x0=10.0, y0=10.0, K=200.0, δx=0.1 and δy=0.2. Figures 5(b) and 5(c) show the splitting LB results at t=0.5 and t=1.0, respectively. These numerical results are consistent with those in [48].
In this paper, a splitting LB scheme for solving KPI equations was presented. In this algorithm, the variable substitution method was used. As a result, the KPI equation has been written into two macroscopic equations. Two sets of distribution functions are employed for the construction of these two macroscopic equations. This splitting scheme contains both time evolution and spatial evolution in which the second equation is based on a space variable rather than time. Three numerical examples were conducted to show that this splitting LB scheme was more suitable for simulating KPI equations than the traditional higher-order moment LB model.
It was laborious to apply the traditional LB model to higher order PDEs due to the complexity of the higher-order Chapman-Enskog expansion. However, the splitting LB method appeared promising in simulating higher order PDEs because the splitting technique can reduce the order of the macroscopic equation. How the splitting LB method can be applied to these equations still requires further research.
The author declares he has not used Artificial Intelligence (AI) tools in the creation of this article.
The research was funded by the Doctoral Research Project of Northeast Electric Power University (BSJXM-2021235).
The author declares no conflicts of interest.
In this section, the multiscale expansion technique is applied to recover the KdV equation with a source term. First, we selected the following summations of the additional distribution functions:
ξ1=∑αω(1)α=0, | (A.1) |
ξ2=∑αω(2)α=Kωε, | (A.2) |
ξ3=∑αω(3)α=−τδε∂2(3u2)∂y2, | (A.3) |
ξ4=∑αω(4)α=Kδ2ε2∫∂2w∂y2dx+2C2τKc2ε∂2w∂x2+τδ2ε∂2∂y2∂(12u3)∂x. | (A.4) |
By taking Eq (13) and summing over α, according to Eqs (19) and (21), the following relation can be derived:
∂u∂t0=−∂(3u2)∂x. | (A.5) |
The macroscopic Eq (4) and the summation Eq (A.1) can be modified to obtain the following:
∂w∂t0=−δK∂2(3u2)∂y2. | (A.6) |
Equations (A.5) and (A.6) are called conservation laws in the t0 scale.
Similarly, we take Eq (14) and sum over α. Based on Eqs (19), (21), (22) and (A.2), the following can be derived:
∂u∂t1=Kwε. | (A.7) |
Because of the macroscopic Eq (4), we can obtain the following:
∂w∂t1=δε∫∂2w∂y2dx. | (A.8) |
Equations (A.7) and (A.8) are called conservation laws in the t1 scale.
To recover the macroscopic equation, we take (13)+ε×(14)+ε2×(15) and sum over α. Thus, we obtain the following:
∂u∂t+∂m∂x+εC2[∂∂t0(∂u∂t0+∂m∂x)+∂∂x(∂m∂t0+∂π∂x)]+ε2C3[∂2∂t20(∂u∂t0+∂m∂x)+2∂2∂t0∂x(∂m∂t0+∂π∂x)+∂2∂x2(∂π∂t0+∂P∂x)]+τε2∂ξ2∂t0=εξ2+ε2ξ3+O(ε3). | (A.9) |
Due to Functions (21)–(22), the third term on the left-hand side of Eq (9) is equal to zero. To recover the term ∂3u/∂x3 in the macroscopic equation, the function P(x,t) should be selected in Eq (23). Substituting Eqs (A.3) and (A.6) into Eq (A.9) yields the following:
∂u∂t+6u∂u∂x+ε2C3χ∂3u∂x3=Kw+O(ε3). | (A.10) |
This is the KdV equation with a source term if χ=1/ε2C3.
Furthermore, to improve the accuracy of the equation, we take Eq (16) and sum it over α on account of the conservation law Eq (A.5), thus getting:
∑αC4(∂∂t0+∂∂xeα)4f(0)α=C4∂4∂x4(−12χu2−12965u5+Q). | (A.11) |
If we select:
Q(x,t)=12965u5+12χu, | (24) |
then
∑αC4(∂∂t0+∂∂xeα)4f(0)α=0. | (A.12) |
Moreover, several terms that are not equal to zero are:
∑αC2∂2∂t21f(0)α=C2Kδε2∫∂2w∂y2dx, | (A.13) |
∑ατ∂∂t1ω(2)α=τKδε2∫∂2w∂y2dx, | (A.14) |
∑ατ(∂∂t0+∂∂xeα)ω(3)α=τ2δε∂2∂y2∂(12u3)∂x. | (A.15) |
We assume that the additional distribution functions ω(i)α(i=1,2,3,4) are independent of α. Hence, for the D1Q5 lattice, the following can be obtained:
∑αC2τ(∂∂t0+∂∂xeα)2ω(2)α=C2τδε∂2∂y2∂(12u3)∂x+2C2τKc2ε∂2w∂x2. | (A.16) |
Because of the summation Eq (A.4), we derive:
∂u∂t3=0. | (A.17) |
Taking (13)+ε×(14)+ε2×(15)+ε3×(16) and summing over α, the KdV equation with a source term and fourth-order accuracy of truncation error can finally be achieved:
∂u∂t+6u∂u∂x+∂3u∂x3=Kw+O(ε4). | (25) |
From the summations Eqs (A.1)–(A.4) that we select, the additional distribution functions can finally be obtained:
ω(i)α=15ξi. α=0,1,⋯,4 | (A.18) |
[1] |
S. Chen, H. Chen, D. Martínez, W. Matthaeus, Lattice Boltzmann model for simulation of magneto-hydrodynamics, Phys. Rev. Lett., 67 (1991), 3776–3779. https://doi.org/10.1103/PhysRevLett.67.3776 doi: 10.1103/PhysRevLett.67.3776
![]() |
[2] |
R. Benzi, S. Succi, M. Vergassola, The lattice Boltzmann equation: theory and applications, Phys. Rep., 222 (1992), 145–197. https://doi.org/10.1016/0370-1573(92)90090-M doi: 10.1016/0370-1573(92)90090-M
![]() |
[3] |
Y. H. Qian, D. d'Humières, P. Lallemand, Lattice BGK models for Navier-Stokes equation, Europhys. Lett., 17 (1992), 479–484. https://doi.org/10.1209/0295-5075/17/6/001 doi: 10.1209/0295-5075/17/6/001
![]() |
[4] |
A. Fakhari, M. Geier, T. Lee, A mass-conserving lattice Boltzmann method with dynamic grid refinement for immiscible two-phase flows, J. Comput. Phys., 315 (2016), 434–457. https://doi.org/10.1016/j.jcp.2016.03.058 doi: 10.1016/j.jcp.2016.03.058
![]() |
[5] |
T. Reis, A lattice Boltzmann formulation of the one-fluid model for multiphase flow, J. Comput. Phys., 453 (2022), 110962. https://doi.org/10.1016/j.jcp.2022.110962 doi: 10.1016/j.jcp.2022.110962
![]() |
[6] |
Q. Z. Li, Z. L. Lu, Z. Chen, C. Shu, Y. Y. Liu, T. Q. Guo, et al., An efficient simplified phase-field lattice Boltzmann method for super-large-density-ratio multiphase flow, Int. J. Multiph. Flow, 160 (2023), 104368. https://doi.org/10.1016/j.ijmultiphaseflow.2022.104368 doi: 10.1016/j.ijmultiphaseflow.2022.104368
![]() |
[7] | S. Simonis, J. Nguyen, S. J. Avis, W. Dörfler, M. J. Krause, Binary fluid flow simulations with free energy lattice Boltzmann methods, Discrete Cont. Dyn. S, in press, 2023. http://doi.org/10.3934/dcdss.2023069 |
[8] |
F. Bukreev, S. Simonis, A. Kummerlӓnder, J. Jeßberger, M. J. Krause, Consistent lattice Boltzmann methods for the volume averaged Navier-Stokes equations, J. Comput. Phys., 490 (2023), 112301. https://doi.org/10.1016/j.jcp.2023.112301 doi: 10.1016/j.jcp.2023.112301
![]() |
[9] |
M. H. Saadat, F. Bösch, I. V. Karlin, Lattice Boltzmann model for compressible flows on standard lattices: Variable Prandtl number and adiabatic exponent, Phys. Rev. E, 99 (2019), 013306. https://doi.org/10.1103/PhysRevE.99.013306 doi: 10.1103/PhysRevE.99.013306
![]() |
[10] |
X. Zhao, L. Yang, C. Shu, An implicit lattice Boltzmann flux solver for simulation of compressible flows, Comput. Math. Appl., 107 (2022), 82–94. https://doi.org/10.1016/j.camwa.2021.12.014 doi: 10.1016/j.camwa.2021.12.014
![]() |
[11] |
K. Suga, Y. Kuwata, K. Takashima, R. Chikasue, A D3Q27 multiple-relaxation-time lattice Boltzmann method for turbulent flows, Comput. Math. Appl., 69 (2015), 518–529. https://doi.org/10.1016/j.camwa.2015.01.010 doi: 10.1016/j.camwa.2015.01.010
![]() |
[12] |
M. Taha, S. Zhao, A. Lamorlette, J. L. Consalvi, P. Boivin, Lattice-Boltzmann modeling of buoyancy-driven turbulent flows, Phys. Fluids, 34 (2022), 055131. https://doi.org/10.1063/5.0088409 doi: 10.1063/5.0088409
![]() |
[13] |
S. Chen, Z. Liu, C. Zhang, Z. He, Z. W. Tian, B. C. Shi, et al., A novel coupled lattice Boltzmann model for low Mach number combustion simulation, Appl. Math. Comput., 193 (2007), 266–284. https://doi.org/10.1016/j.amc.2007.03.087 doi: 10.1016/j.amc.2007.03.087
![]() |
[14] |
K. Bhairapurada, B. Denet, P. Boivin, A Lattice-Boltzmann study of premixed flames thermo-acoustic instabilities, Combust. Flame, 240 (2022), 112049. https://doi.org/10.1016/j.combustflame.2022.112049 doi: 10.1016/j.combustflame.2022.112049
![]() |
[15] |
Z. Chen, C. Shu, Simplified lattice Boltzmann method for non-Newtonian power-law fluid flows, Int. J. Numer. Methods Fluids, 92 (2019), 38–54. https://doi.org/10.1002/fld.4771 doi: 10.1002/fld.4771
![]() |
[16] |
S. Adam, F. Hajabdollahi, K. N. Premnath, Cascaded lattice Boltzmann modeling and simulations of three-dimensional non-Newtonian fluid flows, Comput. Phys. Commun., 262 (2021), 107858. https://doi.org/10.1016/j.cpc.2021.107858 doi: 10.1016/j.cpc.2021.107858
![]() |
[17] |
G. Yan, A lattice Boltzmann equation for waves, J. Comput. Phys., 161 (2000), 61–69. https://doi.org/10.1006/jcph.2000.6486 doi: 10.1006/jcph.2000.6486
![]() |
[18] |
A. M. Velasco, J. D. Muñoz, M. Mendoza, Lattice Boltzmann model for the simulation of the wave equation in curvilinear coordinates, J. Comput. Phys., 376 (2019), 76–97. https://doi.org/10.1016/j.jcp.2018.09.031 doi: 10.1016/j.jcp.2018.09.031
![]() |
[19] |
D. Li, H. Lai, B. Shi, Mesoscopic simulation of the (2+1)-dimensional wave equation with nonlinear damping and source terms using the lattice Boltzmann BGK model, Entropy, 21 (2019), 390. https://doi.org/10.3390/e21040390 doi: 10.3390/e21040390
![]() |
[20] |
G. Yan, J. Zhang, A higher-order moment method of the lattice Boltzmann model for the Korteweg-de Vries equation, Math. Comput. Simul., 79 (2009), 1554–1565. https://doi.org/10.1016/j.matcom.2008.07.006 doi: 10.1016/j.matcom.2008.07.006
![]() |
[21] |
H. Wang, Solitary wave of the Korteweg-de Vries equation based on lattice Boltzmann model with three conservation laws, Adv. Space Res., 59 (2017), 283–292. https://doi.org/10.1016/j.asr.2016.08.023 doi: 10.1016/j.asr.2016.08.023
![]() |
[22] |
W. Q. Hu, S. L. Jia, General propagation lattice Boltzmann model for variable-coefficient non-isospectral KdV equation, Appl. Math. Lett., 91 (2019), 61–67. https://doi.org/10.1016/j.aml.2018.12.002 doi: 10.1016/j.aml.2018.12.002
![]() |
[23] |
H. Yoshida, M. Nagaoka, Lattice Boltzmann method for the convection-diffusion equation in curvilinear coordinate systems, J. Comput. Phys., 257 (2014), 884–900. https://doi.org/10.1016/j.jcp.2013.09.035 doi: 10.1016/j.jcp.2013.09.035
![]() |
[24] |
L. Wang, B. Shi, Z. Chai, Regularized lattice Boltzmann model for a class of convection-diffusion equations, Phys. Rev. E, 92 (2015), 043311. https://doi.org/10.1103/PhysRevE.92.043311 doi: 10.1103/PhysRevE.92.043311
![]() |
[25] |
Z. Chai, B. Shi, Z. Guo, A multiple-relaxation-time lattice Boltzmann model for general nonlinear anisotropic convection-diffusion equations, J. Sci. Comput., 69 (2016), 355–390. https://doi.org/10.1007/s10915-016-0198-5 doi: 10.1007/s10915-016-0198-5
![]() |
[26] |
J. Zhang, G. Yan, A lattice Boltzmann model for reaction-diffusion equations with higher-order accuracy, J. Sci. Comput., 52 (2012), 1–16. https://doi.org/10.1007/s10915-011-9530-2 doi: 10.1007/s10915-011-9530-2
![]() |
[27] |
G. Silva, Discrete effects on the source term for the lattice Boltzmann modelling of one-dimensional reaction-diffusion equations, Comput. Fluids, 251 (2023), 105735. https://doi.org/10.1016/j.compfluid.2022.105735 doi: 10.1016/j.compfluid.2022.105735
![]() |
[28] |
L. Zhong, S. Feng, P. Dong, S. T. Gao, Lattice Boltzmann schemes for the nonlinear Schrödinger equation, Phys. Rev. E, 74 (2006), 036704. https://doi.org/10.1103/PhysRevE.74.036704 doi: 10.1103/PhysRevE.74.036704
![]() |
[29] |
B. Wang, J. Zhang, G. Yan, Curvilinear coordinate lattice Boltzmann simulation for necklace-ring beams in the nonlinear Schrödinger equation, Int. J. Mod. Phys. C, 31 (2020), 2050136. https://doi.org/10.1142/S0129183120501363 doi: 10.1142/S0129183120501363
![]() |
[30] | B. B. Kadomtsev, V. I. Petviashvili, On the stability of solitary waves in weakly dispersive media, Sov. Phys. Dokl., 15 (1970), 175–187. |
[31] |
P. J. Bryant, Two-dimensional periodic permanent waves in shallow water, J. Fluid Mech., 115 (1982), 525–532. https://doi.org/10.1017/S0022112082000895 doi: 10.1017/S0022112082000895
![]() |
[32] |
J. Hammack, N. Scheffner, H. Segur, Two-dimensional periodic waves in shallow water, J. Fluid Mech., 209 (1989), 567–589. https://doi.org/10.1017/S0022112089003228 doi: 10.1017/S0022112089003228
![]() |
[33] |
J. K. Xue, A spherical KP equation for dust acoustic waves, Phys. Lett. A, 314 (2003), 479–483. https://doi.org/10.1016/S0375-9601(03)00951-4 doi: 10.1016/S0375-9601(03)00951-4
![]() |
[34] |
I. M. Krichever, S. P. Novikov, Holomorphic bundles over algebraic curves and nonlinear equations, Russ. Math. Surv., 35 (1980), 53–64. https://doi.org/10.1070/RM1980v035n06ABEH001974 doi: 10.1070/RM1980v035n06ABEH001974
![]() |
[35] |
G. A. Latham, Solutions of the KP equation associated to rank-three commuting differential operators over a singular elliptic curve, Physica D, 41 (1990), 55–66. https://doi.org/10.1016/0167-2789(90)90027-M doi: 10.1016/0167-2789(90)90027-M
![]() |
[36] |
H. Zhao, Interactions of solitary waves under the conditions of the (3+1)-dimensional Kadomtsev-Petviashvili equation, Appl. Math. Comput., 215 (2010), 3383–3389. https://doi.org/10.1016/j.amc.2009.10.031 doi: 10.1016/j.amc.2009.10.031
![]() |
[37] |
C. Y. Qin, S. F. Tian, X. B. Wang, T. T. Zhang, J. Li, Rogue waves, bright–dark solitons and traveling wave solutions of the (3+1)-dimensional generalized Kadomtsev-Petviashvili equation, Comput. Math. Appl., 75 (2018), 4221–4231. https://doi.org/10.1016/j.camwa.2018.03.024 doi: 10.1016/j.camwa.2018.03.024
![]() |
[38] |
L. Li, Y. Xie, M. Wang, Characteristics of the interaction behavior between solitons in (2+1)-dimensional caudrey-dodd-gibbon-kotera-sawada equation, Results Phys., 19 (2020), 103697. https://doi.org/10.1016/j.rinp.2020.103697 doi: 10.1016/j.rinp.2020.103697
![]() |
[39] |
J. G. Liu, W. H. Zhu, Y. K. Wu, G. H. Jin, Application of multivariate bilinear neural network method to fractional partial differential equations, Results Phys., 47 (2023), 106341. https://doi.org/10.1016/j.rinp.2023.106341 doi: 10.1016/j.rinp.2023.106341
![]() |
[40] |
J. G. Liu, M. Eslami, H. Rezazadeh, M. Mirzazadeh, Rational solutions and lump solutions to a non-isospectral and generalized variable-coefficient Kadomtsev-Petviashvili equation, Nonlinear Dyn., 95 (2019), 1027–1033. https://doi.org/10.1007/s11071-018-4612-4 doi: 10.1007/s11071-018-4612-4
![]() |
[41] |
J. G. Liu, Q. Ye, Stripe solitons and lump solutions for a generalized Kadomtsev–Petviashvili equation with variable coefficients in fluid mechanics, Nonlinear Dyn., 96 (2019), 23–29. https://doi.org/10.1007/s11071-019-04770-8 doi: 10.1007/s11071-019-04770-8
![]() |
[42] |
L. Li, Y. Xie, L. Mei, Multiple-order rogue waves for the generalized (2+1)-dimensional Kadomtsev-Petviashvili equation, Appl. Math. Lett., 117 (2021), 107079. https://doi.org/10.1016/j.aml.2021.107079 doi: 10.1016/j.aml.2021.107079
![]() |
[43] |
L. Li, Y. Xie, Rogue wave solutions of the generalized (3+1)-dimensional Kadomtsev-Petviashvili equation, Chaos Soliton Fract., 147 (2021), 110935. https://doi.org/10.1016/j.chaos.2021.110935 doi: 10.1016/j.chaos.2021.110935
![]() |
[44] |
Y. Xie, Y. Yan, L. Li, Rational solutions and rogue waves of the generalized (2+1)-dimensional Kadomtsev-Petviashvili equation, Chinese J. Phys., 77 (2022), 2047–2059. https://doi.org/10.1016/j.cjph.2021.11.010 doi: 10.1016/j.cjph.2021.11.010
![]() |
[45] |
J. G. Liu, W. H. Zhu, Y. He, Variable-coefficient symbolic computation approach for finding multiple rogue wave solutions of nonlinear system with variable coefficients, Z. Angew. Math. Phys., 72 (2021), 154. https://doi.org/10.1007/s00033-021-01584-w doi: 10.1007/s00033-021-01584-w
![]() |
[46] |
A. R. Seadawy, K. El-Rashidy, Dispersive solitary wave solutions of Kadomtsev-Petviashvili and modified Kadomtsev-Petviashvili dynamical equations in unmagnetized dust plasma, Results Phys., 8 (2018), 1216–1222. https://doi.org/10.1016/j.rinp.2018.01.053 doi: 10.1016/j.rinp.2018.01.053
![]() |
[47] |
A. G. Bratsos, E. H. Twizell, An explicit finite difference scheme for the solution of Kadomtsev-Petviashvili equation, Int. J. Comput. Math., 68 (1998), 175–187. https://doi.org/10.1080/00207169808804685 doi: 10.1080/00207169808804685
![]() |
[48] |
B. F. Feng, T. Mitsui, A finite difference method for the Korteweg-de Vries and the Kadomtsev-Petviashvili equations, J. Comput. Appl. Math., 90 (1998), 95–116. https://doi.org/10.1016/S0377-0427(98)00006-5 doi: 10.1016/S0377-0427(98)00006-5
![]() |
[49] |
A. A. Minzoni, N. F. Smyth, Evolution of lump solutions for the KP equation, Wave Motion, 24 (1996), 291–305. https://doi.org/10.1016/S0165-2125(96)00023-6 doi: 10.1016/S0165-2125(96)00023-6
![]() |
[50] |
A. M. Wazwaz, A computational approach to soliton solutions of the Kadomtsev-Petviashvili equation, Appl. Math. Comput., 123 (2001), 205–217. https://doi.org/10.1016/S0096-3003(00)00065-5 doi: 10.1016/S0096-3003(00)00065-5
![]() |
[51] |
H. M. Wang, Solitons of the Kadomtsev-Petviashvili equation based on lattice Boltzmann model, Adv. Space Res., 59 (2017), 293–301. https://doi.org/10.1016/j.asr.2016.08.029 doi: 10.1016/j.asr.2016.08.029
![]() |
[52] | J. Cai, J. Chen, M. Chen, Efficient linearized local energy-preserving method for the Kadomtsev-Petviashvili equation, Discrete Cont. Dyn. B, 27 (2022), 2441–2453. |
[53] | S. Beji, Kadomtsev-Petviashvili type equation for uneven water depths, Ocean Eng., 154 (2018), 226–233. |
[54] |
B. Fornberg, G. B. Whitham, A numerical and theoretical study of certain nonlinear wave phenomena, Philos. Trans. Roy. Soc. A, 289 (1978), 373–403. https://doi.org/10.1098/rsta.1978.0064 doi: 10.1098/rsta.1978.0064
![]() |
[55] | S. Chapman, T. G. Cowling, The Mathematical Theory of Non-Uniform Gases, Cambridge: Cambridge University Press, 1970. |
[56] |
S. Hou, Q. Zhou, S. Chen, G. Doolen, A. C. Cogley, Simulation of cavity flow by the lattice Boltzmann method, J. Comput. Phys., 118 (1995), 329–347. https://doi.org/10.1006/jcph.1995.1103 doi: 10.1006/jcph.1995.1103
![]() |
Splitting LB | Higher-order moment LB [51] | Linearized local energy-preserving method [52] | |
t = 1 | 7.432065×10−6 | 6.932046×10−2 | 2.607653×10−2 |
t = 2 | 6.942704×10−6 | 8.490834×10−2 | 2.119188×10−2 |
t = 3 | 7.547621×10−6 | 9.748673×10−2 | 3.286301×10−2 |
t = 4 | 6.783254×10−6 | 1.079479×10−1 | 2.955938×10−2 |