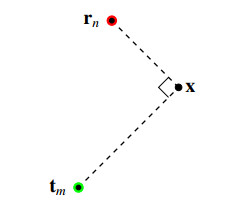
In this paper, we consider the application of the bifocusing method (BFM) for a fast identification of two-dimensional circle-like small inhomogeneities from measured scattered field data. Based on the asymptotic expansion formula for the scattered field in the presence of small inhomogeneities, we introduce the imaging functions of the BFM for both dielectric permittivity and magnetic permeability contrast cases. To examine the applicability and the various properties of the BFM, we show that the imaging functions can be expressed by the Bessel function of orders zero and one, as well as the characteristics (size, permittivity, and permeability) of the inhomogeneities. To support the theoretical results, various numerical results with synthetic and experimental data are presented.
Citation: Sangwoo Kang, Won-Kwang Park. A novel study on the bifocusing method for imaging unknown objects in two-dimensional inverse scattering problem[J]. AIMS Mathematics, 2023, 8(11): 27080-27112. doi: 10.3934/math.20231386
[1] | Won-Kwang Park . On the application of subspace migration from scattering matrix with constant-valued diagonal elements in microwave imaging. AIMS Mathematics, 2024, 9(8): 21356-21382. doi: 10.3934/math.20241037 |
[2] | Seong-Ho Son, Kwang-Jae Lee, Won-Kwang Park . Real-time tracking of moving objects from scattering matrix in real-world microwave imaging. AIMS Mathematics, 2024, 9(6): 13570-13588. doi: 10.3934/math.2024662 |
[3] | Bowen Tang, Xiaoying Yang, Lin Su . Shape reconstruction of acoustic obstacle with linear sampling method and neural network. AIMS Mathematics, 2024, 9(6): 13607-13623. doi: 10.3934/math.2024664 |
[4] | Shixian Ren, Yu Zhang, Ziqiang Wang . An efficient spectral-Galerkin method for a new Steklov eigenvalue problem in inverse scattering. AIMS Mathematics, 2022, 7(5): 7528-7551. doi: 10.3934/math.2022423 |
[5] | Zui-Cha Deng, Fan-Li Liu, Liu Yang . Numerical simulations for initial value inversion problem in a two-dimensional degenerate parabolic equation. AIMS Mathematics, 2021, 6(4): 3080-3104. doi: 10.3934/math.2021187 |
[6] | Benxue Gong, Zhenyu Zhao, Tiao Bian, Yingmei Wang . Numerical differentiation for two-dimensional scattered data on arbitrary domain base on Hermite extension with an implicit iteration process. AIMS Mathematics, 2022, 7(4): 5991-6015. doi: 10.3934/math.2022334 |
[7] | Yilihamujiang Yimamu, Zui-Cha Deng, Liu Yang . An inverse volatility problem in a degenerate parabolic equation in a bounded domain. AIMS Mathematics, 2022, 7(10): 19237-19266. doi: 10.3934/math.20221056 |
[8] | Yu Xu, Youjun Deng, Dong Wei . Numerical solution of forward and inverse problems of heat conduction in multi-layered media. AIMS Mathematics, 2025, 10(3): 6144-6167. doi: 10.3934/math.2025280 |
[9] | Cheng-Hung Huang, Ya-Rou Zhong . An inverse problem to estimate simultaneously the heat source strength for multiple integrated circuit chips on a printed circuit board. AIMS Mathematics, 2024, 9(4): 8848-8867. doi: 10.3934/math.2024431 |
[10] | Lei Hu . A weighted online regularization for a fully nonparametric model with heteroscedasticity. AIMS Mathematics, 2023, 8(11): 26991-27008. doi: 10.3934/math.20231381 |
In this paper, we consider the application of the bifocusing method (BFM) for a fast identification of two-dimensional circle-like small inhomogeneities from measured scattered field data. Based on the asymptotic expansion formula for the scattered field in the presence of small inhomogeneities, we introduce the imaging functions of the BFM for both dielectric permittivity and magnetic permeability contrast cases. To examine the applicability and the various properties of the BFM, we show that the imaging functions can be expressed by the Bessel function of orders zero and one, as well as the characteristics (size, permittivity, and permeability) of the inhomogeneities. To support the theoretical results, various numerical results with synthetic and experimental data are presented.
In this paper, we consider the localization of a set of small two-dimensional (2D) homogeneous inhomogeneities using various electromagnetic source transmitters and receivers. The problem is formulated as an inverse scattering problem for the 2D electromagnetic system. To solve this problem, various remarkable techniques have been investigated, most of which are classified as quantitative or qualitative methods.
In order to reconstruct the parameter (such as dielectric permittivity, electric conductivity, or magnetic permeability at a given frequency) distribution or identify an unknown object larger than the given wavelength, various quantitative methods (or iterative methods) have been developed, such as Newton's method [47,62], Gauss–Newton method [1,16], optimal control approach [3], Levenberg–Marquardt algorithm [13,27], Born iterative method [33,50], level-set technique [23,64], and subspace optimization method [18,69]. Although these algorithms have shown feasibility and applicability in inverse scattering problems, good initial guess must be generated before the iteration procedure because the success of iterative-based techniques significantly depend on the initial guess [48,61].
Alternatively, for obtaining a good initial guess or identifying the location or determining the outline shape of small objects, various qualitative methods (or non-iterative methods) have been investigated, such as the direct sampling method [34,37,39], factorization method [15,20,45], Kirchhoff and subspace migrations [5,54,59], MUltiple SIgnal Classification (MUSIC) algorithm [22,42,58], linear sampling method [9,19,46], topological derivative strategy [4,51,53], orthogonality sampling method [31,36,60], and variational algorithm based on the Fourier inversion [6,7,8].
The bifocusing method (BFM) is classified as a qualitative method using a multistatic measurement system. Recently, it has been successfully applied to various interesting problems, such as damage detection of inhomogeneous structures [26,44], radar imaging [35], and anomaly detection in microwave imaging [66]. {Let us emphasize that a reliable mathematical theory of BFM in the presence of small anomaly has been developed only for the transverse magnetic polarization (TM) case, refer to [43]. However, to the best of our knowledge, the application of BFM in inverse scattering problem for both transverse magnetic and electric (TE) polarizations is still heuristic; thus, developing an appropriate mathematical theory and performing numerical simulations with synthetic and experimental data are necessary to explain the applicability, fundamental limitations, and various intrinsic properties of the BFM in TM and TE polarizations.
In this paper, we design the imaging functions of the BFM for the dielectric permittivity (TM polarization) and magnetic permeability (TE polarization) contrast cases in full-aperture inverse scattering problem. Based on the asymptotic expansion formula in the presence of small inhomogeneities, we show that the designed imaging functions comprise the Bessel function of order zero and order one for the dielectric permittivity and magnetic permeability contrast cases, respectively, and the material properties (such as the total number, size, dielectric permittivity, and magnetic permeability) of the inhomogeneities. This analysis confirms the reason of the unique determination of inhomogeneities for the permittivity contrast case and the identification of two locations instead of true locations of inhomogeneities for the permeability contrast case. To demonstrate the theoretical results, various results of the numerical simulation with synthetic and experimental data are presented.
This study is organized as follows. In Section 2, we introduce the basic concept of the 2D direct scattering problem in the presence of a set of small, well-separated inhomogeneities and the asymptotic expansion formula for the scattered field. In Section 3, we design the imaging functions of the BFM for the dielectric permittivity and magnetic permeability contrast cases, explore the mathematical structures of the imaging functions, and discuss their applicability, limitations, and various properties. In Section 4, we present some numerical simulation results with synthetic and experimental data, and compared with those obtained by other qualitative methods such as direct sampling method, MUSIC, subspace migration, and topological derivative method. In Section 5, we present a short conclusion including an outline of future research.
Finally, let us emphasize that the imaging result via experimental data obtained through the BFM does not guarantee the exact shape of object. However, retrieved result can be adopted as a good initial guess and correspondingly, it will be possible to retrieve a better shape through the iterative-based algorithms. Let us mention some classical but very useful approaches of the retrieval of objects via Fresnel data; Bayesian approach [10], modified Born method [12], contrast source inversion method [14], conjugate gradient fast Fourier transform (CG-FFT) method [21], modified gradient method [24], image fusion approach [25], diffraction tomography, modified gradient, and real-coded genetic algorithms [52], level set method [63], linear spectral estimation technique [67], and distorted-wave Born approach [68].
We shall consider 2D direct scattering from a set of small electromagnetic inhomogeneities located in homogeneous space R2. We denote Ω be the region of interest (ROI) and assume that the inhomogeneity Σs, s=1,2,⋯,S, is a small ball with radius αs and location xs:
Σs=xs+αsS1,Σ=S⋃s=1Σs,⊂Ω, |
where S1 denotes the unit circle centered at the origin.
Let εb and μb denote the values of the dielectric permittivity and magnetic permeability of R2, respectively, at the given angular frequency ω=2πf, where f denotes the ordinary frequency measured in hertz. Similarly, we denote εs and μs as the values of the dielectric permittivity and magnetic permeability of Σs. With this, we denote ε(x) and μ(x) as the piecewise constants of dielectric permittivity and magnetic permeability, respectively, such that
ε(x)={εs,x∈Σsεb,x∈R2∖¯Σandμ(x)={μs,x∈Σsμb,x∈R2∖¯Σ. |
With this, we denote k as the background wavenumber that satisfies k2=ω2εbμb. Throughout this paper, we assume that all Σs are well-separated from each other and, correspondingly, that k satisfies the following:
|xs−xs′|≫34kfors≠s′. | (2.1) |
We assume that transmitters are located at tm, m=1,2,⋯,M, while receivers are located at rn, n=1,2,⋯,N. That is, tm and rn are given by the following:
tm=rtx(cosθm,sinθm),θm=2π(m−1)M | (2.2) |
and
rn=rrx(cosϑn,sinϑn),ϑn=2π(n−1)N, | (2.3) |
respectively. In this study, we consider the point-source illumination, letting
uinc(tm,x)=G(tm,x)=−i4H(1)0(k|tm−x|)=−i4(J0(k|tm−x|)+iY0(k|tm−x|)) |
be the incident field at source tm. Here, Jn and Yn denote the Bessel and Neumann functions of integer order n, respectively, and H(1)0 denotes the Hankel function or order zero of the first kind. Throughout this paper, we assume that the time dependence is of the form e−iωt. Then, the governing equation for the time-harmonic total field u(tm,x) emitted by a source at tm is
∇⋅(1μ(x)∇u(tm,x))+ω2ε(x)u(tm,x)=0, | (2.4) |
with transmission conditions at the boundaries of Σs. With this, we denote uscat(tm,rn) as the scattered field, which is obtained by subtracting the total and incident fields and satisfies the Sommerfeld radiation condition
lim|x|→∞√|x|(∂uscat(tm,rn)∂|x|−ikuscat(tm,rn))=0 |
uniformly in all directions x/|x|. Based on [7], uscat(tm,rn) can be represented as an asymptotic expansion formula, which plays a key role in designing the imaging function.
Lemma 2.1 (Asymptotic formula). For sufficiently large k, uscat(tm,rn) can be represented as follows:
uscat(tm,rn)≈S∑s=1α2sk2π(εs−εb√εbμb)G(tm,xs)G(rn,xs)+S∑s=1α2sπ∇G(tm,xs)M∇G(rn,xs)T, | (2.5) |
where M(xs) denotes 2×2 diagonal matrix with diagonal element 2πμb/(μs+μb).
In this section, we apply the asymptotic formula (2.5) to design an imaging function for identifying Σs with no prior information and to establish a mathematical theory. Consequently, we examine the structure of the MSR matrix K. The analysis is then led successively for two cases: dielectric permittivity (ε(x)≠εb and μ(x)=μb) and magnetic permeability contrast cases (ε(x)=εb and μ(x)≠μb). Throughout this section, we assume that there is no noise and the full MSR matrix K is available such that
K=[uscat(t1,r1)uscat(t1,r2)⋯uscat(t1,rN)uscat(t2,r1)uscat(t2,r2)⋯uscat(t2,rN)⋮⋮⋱⋮uscat(tM,r1)uscat(tM,r2)⋯uscat(tM,rN)]. |
First, we assume that ε(x)≠εb and μ(x)=μb. Based on the representation formula (2.5), u(ε)scat(tm,rn) can be written as follows:
u(ε)scat(tm,rn)≈S∑s=1k2α2sπ(εs−εb√εbμb)G(tm,xs)G(rn,xs). |
Correspondingly, the MSR matrix becomes
K≈S∑s=1k2α2sπ(εs−εb√εbμb)[G(t1,xs)G(r1,xs)G(t1,xs)G(r2,xs)⋯G(t1,xs)G(rN,xs)G(t2,xs)G(r1,xs)G(t2,xs)G(r2,xs)⋯G(t2,xs)G(rN,xs)⋮⋮⋱⋮G(tM,xs)G(r1,xs)G(tM,xs)G(r2,xs)⋯G(tM,xs)G(rN,xs)]. |
Based on the above representation, we can design the imaging function forms every image point through the synthesis inversion of two Green's functions, G(tm,x) and G(rn,x), for m=1,2,⋯,M and n=1,2,⋯,N. Correspondingly, the imaging function FTM(x) of BFM can be introduced as follows: for x∈Ω,
FTM(x)=|ΦTM(x)|maxx∈Ω|ΦTM(x)|,whereΦTM(x)=M∑m=1N∑n=1u(ε)scat(tm,rn)G(tm,x)G(rn,x). | (3.1) |
Here, Ω denotes the imaging area. The resulting plot of FTM(x) is expected to exhibit large peaks at xs∈Σs, s=1,2,⋯,S so that all inhomogeneities can be recognized through the map of FTM(x), refer to [35].
Although the location of the inhomogeneities can be retrieved from the map of FTM(x), further mathematical theories to explain the feasibilities, fundamental limitations, and some phenomena in the simulation results must be investigated. Here, we show that FTM(x) can be written by the Bessel function of order zero of the first kind. For a proper derivation, we introduce the following useful identity derived in [56].
Lemma 3.1. For sufficiently large M, θm,θ∈S1, and x∈R2, the following relation holds
1MM∑m=1eikθm⋅x≈12π∫S1eikθ⋅xdθ=J0(k|x−xs|). |
Then, we can obtain the following result.
Theorem 3.1. Let x∈Ω, θm=(cosθm,sinθm), ϑn=(cosϑn,sinϑn), and x−xs=|x−xs|(cosϕs,sinϕs), and assume that the values of M, N, k are sufficiently large such that |x−xs|≫0.25/k. Subsequently, for tm and rn respectively defined in (2.2) and (2.3), FTM(x) can be represented as follows:
FTM(x)=|ΨTM(x)|maxx∈Ω|ΨTM(x)|,whereΨTM(x)≈MNk2πS∑s=1α2s(εs−εb√εbμb)J0(k|x−xs|)2. | (3.2) |
Proof. Since |tm−x|,|rn−x|≫0.25/k and −2π<arg(k|tm−x|),arg(k|rn−x|)<π for all m and n, the following asymptotic forms hold
G(tm,x)≈(1−i)eik|tm−x|√kπ|tm−x| |
and
G(rn,x)≈(1−i)eik|rn−x|√kπ|rn−x|. |
Since
|tm−x|=√|t|2−2tm⋅x+|x|2=|tm|2−tm|tm|⋅x+O(1|tm|)≈|tm|−θm⋅x, |
we have
G(tm,x)≈(1−i)eik|tm|√kπ|tm|e−ikθm⋅xandG(rn,x)≈(1−i)eik|rn|√kπ|rn|e−ikϑn⋅x. | (3.3) |
Thus, ΦTM(x) can be written as follows:
ΦTM(x)=M∑m=1N∑n=1S∑s=1k2α2sπ(εs−εb√εbμb)G(tm,xs)G(rn,xs)G(tm,x)G(rn,x)≈M∑m=1N∑n=1S∑s=1k2α2sπ(εs−εb√εbμb)e−ikθm⋅xse−ikϑn⋅xse−ikθm⋅xe−ikϑn⋅x=S∑s=1k2α2sπ(εs−εb√εbμb)(M∑m=1eikθm⋅(x−xs))(N∑n=1eikϑn⋅(x−xs)). |
Since M and N are sufficiently large, applying Lemma 3.1 yields
M∑m=1eikθm⋅(x−xs)≈MJ0(k|x−xs|))andN∑n=1eikϑn⋅(x−xs)≈NJ0(k|x−xs|). |
Hence, we can obtain
ΦTM(x)≈MNk2πS∑s=1α2s(εs−εb√εbμb)J0(k|x−xs|)2 |
and correspondingly, (3.2) can be derived.
Based on the identified structure (3.2), we can examine some properties of the imaging function FTM(x).
Remark 3.1 (Unique detectability and resolution). Since J0(0)=1, xs can be identified uniquely because the local maxima of the FTM(x) will appear at xs. Moreover, several artifacts that depend on k also appear in the map of FTM(x) due to the oscillating property of the J0. That is, if the value of k is sufficiently large, high-resolution results can be obtained, but several artifacts will be included in the map of FTM(x). Conversely, if the value of k is small, a blurred image will appear, but less artifacts will be included. Thus, xs cannot be identified using the map of FTM(x) if the value k is extremely small (see Figure 11).
Remark 3.2 (Material properties). Based on (3.2), we can examine the value of FTM(x) as proportional to α2s(εs−εb). This means that the value of FTM(x) depends on the size and permittivity of the inhomogeneities. That is, if there are two inhomogeneities Σ1 and Σ2 satisfying α21(ε1−εb)>α22(ε2−εb), then FTM(x1)>FTM(x2) so that the identification of x1 is guaranteed. However, the identification of x2 is not guaranteed in some cases because the value of FTM(x2) cannot be distinguished from the artifacts. Hence, if the size or permittivity of an inhomogeneity is larger than that of another inhomogeneity, its location can be identified. If α21(ε1−εb)≫α22(ε2−εb), then the location x1 can be identified, while x2 cannot.
Based on the Remark 3.1, we can examine the following result of unique determination.
Corollary 3.1 (Unique determination in permittivity contrast case). With the same condition of the Theorem 3.1, the location of small inhomogeneities can be identified uniquely through the map of FTM(x).
Next, we assume that ε(x)=εb and μ(x)≠μb. Based on the representation formula (2.5), u(μ)scat(tm,rn) can be written as follows:
u(μ)scat(tm,rn)≈S∑s=1α2sπ(2μbμs+μb)∇G(tm,xs)⋅∇G(rn,xs). |
Correspondingly, the MSR matrix becomes
K≈S∑s=1α2sπ(2μbμs+μb)[∇G(t1,xs)⋅∇G(r1,xs)∇G(t1,xs)⋅∇G(r2,xs)⋯∇G(t1,xs)⋅∇G(rN,xs)∇G(t2,xs)⋅∇G(r1,xs)∇G(t2,xs)⋅∇G(r2,xs)⋯∇G(t2,xs)⋅∇G(rN,xs)⋮⋮⋱⋮∇G(tM,xs)⋅∇G(r1,xs)∇G(tM,xs)⋅∇G(r2,xs)⋯∇G(tM,xs)⋅∇G(rN,xs)]. |
Based on the above representation, we can regard that the imaging function forms every image point through the synthesis inversion of ∇G(tm,x)⋅∇G(rn,x) for m=1,2,⋯,M and n=1,2,⋯,N. Correspondingly, the imaging function f(x) can be introduced as follows: for x∈Ω,
F(x)=|Φ(x)|maxx∈Ω|Φ(x)|,whereΦ(x)=M∑m=1N∑n=1u(μ)scat(tm,rn)∇G(tm,x)⋅∇G(rn,x). | (3.4) |
Remark 3.3 Notably, since
∇xG(y,x)=ik(y−x)4|y−x|H(1)1(k|y−x|),y≠x, |
sometimes m,n∈N and x∈Ω exist such that
∇G(tm,x)⋅∇G(rn,x)=−k2(tm−x)⋅(rn−x)16|tm−x||rn−x|H(1)1(k|tm−x|)H(1)1(k|rn−x|)=0. |
This means that the denominator of Φ(x) defined in (3.4) can be zero, we refer to Figure 1 for an illustration.
Based on the Remark 3.3, using the F(x) of (3.4) to identify xs is inappropriate. Thus, motivated by the permittivity contrast case, we consider the following imaging function of the BFM: for x∈Ω,
FTE(x)=|ΦTE(x)|maxx∈Ω|ΦTE(x)|,whereΦTE(x)=M∑m=1N∑n=1u(μ)scat(tm,rn)G(tm,x)G(rn,x). | (3.5) |
In order to explore some properties of FTE(x), we explore its structure. To this end, we introduce the following useful identity derived in [56].
Lemma 3.2. For sufficiently large M, θm,θ,ϑn,∈S1, and x∈R2, the following relation holds
1MM∑m=1(θm⋅ϑn)eikθm⋅x≈12π∫S1(θ⋅ϑn)eikθ⋅xdθ=i(ϑn⋅x|x|)J1(k|x|). |
Then, we can obtain the following result.
Theorem 3.2. Let x∈Ω, θm=(cosθm,sinθm), ϑn=(cosϑn,sinϑn), and x−xs=|x−xs|(cosϕs,sinϕs), and assume that the values of M, N, k are sufficiently large such that |x−xs|≫0.25/k. Then, for tm and rn respectively defined in (2.2) and (2.3), FTE(x) can be represented as follows:
FTE(x)=|ΨTE(x)|maxx∈Ω|ΨTE(x)|,whereΨTE(x)≈−2MNk2πS∑s=1α2s(μbμs+μb)J1(k|x−xs|)2. | (3.6) |
Proof. Since |tm−x|≫0.25/k, |rn−x|≫0.25/k, −2π<arg(k|tm−x|)<π, and −2π<arg(k|rn−x|)<π for all m and n, we have
∇xG(tm,x)≈k(1−i)eik|tm|√kπ|tm|(θme−ikθm⋅x)and∇xG(rn,x)≈k(1−i)eik|rn|√kπ|rn|(ϑne−ikϑn⋅x). | (3.7) |
Subsequently, using (3.3) and (3.7), ΦTE(x) can be written as follows:
ΦTE(x)=M∑m=1N∑n=1S∑s=1α2sπ(2μbμs+μb)∇G(tm,xs)⋅∇G(rn,xs)G(tm,x)G(rn,x)≈M∑m=1N∑n=1S∑s=1k2α2sπ(2μbμs+μb)(θm⋅ϑn)e−ikθm⋅xse−ikϑn⋅xse−ikθm⋅xe−ikϑn⋅x=S∑s=1k2α2sπ(2μbμs+μb)N∑n=1(M∑m=1(θm⋅ϑn)eikθm⋅(x−xs))eikϑn⋅(x−xs). | (3.8) |
Since M is sufficiently large, applying Lemma 3.2 yields
M∑m=1(θm⋅ϑn)eikθm⋅(x−xs)≈iM(ϑn⋅x−xs|x−xs|)J1(k|x−xs|). | (3.9) |
Since N is sufficiently large, applying Lemma 3.2 again, we can obtain
N∑n=1(ϑn⋅x−xs|x−xs|)eikϑn⋅(x−xs)≈iN(x−xs|x−xs|⋅x−xs|x−xs|)J1(k|x−xs|)=iNJ1(k|x−xs|). | (3.10) |
Therefore, by combining (3.9) and (3.10), ΦTE(x) of (3.8) becomes
ΦTE(x)≈−2MNk2πS∑s=1α2s(μbμs+μb)J1(k|x−xs|)2 |
and correspondingly, (3.6) can be derived.
Based on the identified structure (3.6), we can examine some properties of the imaging function FTE(x).
Remark 3.4 (Detectability and resolution). Since J1(0)=0, contrary to the permittivity contrast case, xs cannot be identified through the map of FTE(x). Instead, since J1(|x|) has its maximum value at x≈±1.8412, the map of FTE(x) has a large magnitude ring at each location xs satisfies k|x−xs|=1.8412. Therefore, it can be confirmed that the center of the ring is the location of xs. Similar to the permittivity contrast case, several artifacts that depend on the value of k are also included in the map of FTE(x) due to the oscillation property of J1.
If an extremely large value of k is applied, since |x−xs|=1.8412/k⟶0, the ring with large magnitude is concentrated to the center xs so that an almost accurate location xs can be identified through the map of FTE(x), refer to Figure 15 at f=10GHz and f=12GHz.
Remark 3.5 (Material properties). Based on (3.6), we can examine the value of FTE(x) as proportional to α2s/(μs+μb). This means that the value of FTE(x) depends on the size and permeability of the inhomogeneities. That is, if there are two inhomogeneities Σ1 and Σ2 satisfying α21/(μ1+μb)>α22/(μ2+μb), then FTE(x1)>FTE(x2) so that the identification of x1 is guaranteed. However, the identification of x2 is not guaranteed in some cases because the value of FTE(x2) cannot be distinguished from the artifacts. Hence, if the size of an inhomogeneity is larger than that of another inhomogeneity or the permeability of an inhomogeneity is smaller than that of another inhomogeneity, its location can be identified. If α21/(μ1+μb)≫α22/(μ2+μb), then the location x1 can be identified, while x2 cannot.
Although, it can be recognized the existence of small inhomogeneities through the appearance of rings in the map of FTE(x), it is still difficult to identity their true locations. Here, we design a new imaging function of the BFM for accurate localization of small inhomogeneities. The main idea is to convert the factor J1(k|x−xs|)2 of (3.6) to the form J0(k|x−xs|). To this end, we consider the following result derived in [57].
Lemma 3.3. For sufficiently large M, θm,θ∈S1, and x,y∈R2, the following relation holds
1MM∑m=1(θm⋅y)2eikθm⋅x≈12π∫S1(θ⋅y)2eikθ⋅xdθ=12(J0(k|x|)+J2(k|x|))−(x|x|⋅y)2J2(k|x|). |
On the basis of Lemma 3.3, we introduce the following imaging function
FITE(x)=|ΦITE(x)|maxx∈Ω|ΦITE(x)|,whereΦITE(x)=M∑m=1N∑n=1(tm⋅rn)u(μ)scat(θm,ϑn)G(tm,x)G(rn,x). | (3.11) |
Then, the location of small inhomogeneities can be retrieved through the map of FITE(x). To support this assertion, we derive the following result.
Theorem 3.3. Let x∈Ω, θm=(cosθm,sinθm), ϑn=(cosϑn,sinϑn), and x−xs=|x−xs|(cosϕs,sinϕs), and assume that the values of M, N, k are sufficiently large such that |x−xs|≫0.25/k. Then, for tm and rn respectively defined in (2.2) and (2.3), FITE(x) can be represented as follows:
FITE(x)=|ΨITE(x)|maxx∈Ω|ΨITE(x)|, | (3.12) |
where
ΨITE(x)≈MNk2πrtxrrxS∑s=1α2s(μbμs+μb)(J0(k|x−xs|)2+J2(k|x−xs|)2). |
Proof. Based on the (3.3) and (3.7), ΦITE(x) can be written as follows:
ΦITE(x)=M∑m=1N∑n=1S∑s=1α2sπ(2μbμs+μb)(tm⋅rn)∇G(tm,xs)⋅∇G(rn,xs)G(tm,x)G(rn,x)≈M∑m=1N∑n=1S∑s=1k2α2sπ(2μbμs+μb)rtxrrx(θm⋅ϑn)2e−ikθm⋅xse−ikϑn⋅xse−ikθm⋅xe−ikϑn⋅x=S∑s=1k2α2srtxrrxπ(2μbμs+μb)N∑n=1(M∑m=1(θm⋅ϑn)2eikθm⋅(x−xs))eikϑn⋅(x−xs). | (3.13) |
Since M is sufficiently large, θm⋅(x−xs)=|x−xs|cos(θm−ϕs), and θm⋅ϑn=cos(θm−ϑn), applying Lemma 3.3 yields the following:
M∑m=1(θm⋅ϑn)2eikθm⋅(x−xs)≈M2(J0(k|x−xs|)+J2(k|x−xs|))−M(x−xs|x−xs|⋅θn)2J2(k|x−xs|). |
Hence,
ΦITE(x)≈S∑s=1Mk2α2sπrtxrrx(μbμs+μb)(J0(k|x−xs|)+J2(k|x−xs|))N∑n=1eikϑn⋅(x−xs)−S∑s=1Mk2α2sπrtxrrx(2μbμs+μb)J2(k|x−xs|)N∑n=1(x−xs|x−xs|⋅θn)2eikϑn⋅(x−xs). | (3.14) |
Since N is sufficiently large, applying Lemma 3.3 again, we can derive
N∑n=1(x−xs|x−xs|⋅ϑn)2eikϑn⋅(x−xs)≈N2(J0(k|x−xs|)+J2(k|x−xs|))−N(x−xs|x−xs|⋅x−xs|x−xs|)2J2(k|x−xs|)=N2(J0(k|x−xs|)−J2(k|x−xs|)). | (3.15) |
Therefore, by combining (3.14) and (3.15), ΦITE(x) of (3.13) becomes
ΦITE(x)≈MNk2πrtxrrxS∑s=1α2s(μbμs+μb)(J0(k|x−xs|)2+J2(k|x−xs|)2) |
and correspondingly, (3.12) can be derived.
Based on the identified structure (3.6), we can examine some properties of the imaging function FTE(x).
Remark 3.6 (Detectability). Since FITE(x) contains the factor J0(k|x−xs|)2, similar to the permittivity contrast case, xs can be identified through the map of FITE(x). Notice that the factor J2(k|x−xs|)2 is also included in the FITE(x) but since (see [49] for instance)
J2(x)≤{b3√2,c3√|x|:b=0.674885…,c=0.7857468704…}, |
it generates some artifacts with small magnitudes. Numerically, J2(x)2 has maximum value 0.2367 at |x|=0.1940. Therefore, by regarding local maxima of J0(k|x−xs|)2+J2(k|x−xs|)2, the factor J2(k|x−xs|)2 does not disturb the identification significantly so almost accurate location xs can be identified through the map of FITE(x). We refer to the Figure 2 for related illustration.
Correspondingly, we can obtain the following result of unique determination in the magnetic permeability contrast case.
Corollary 3.2 (Unique determination in permeability contrast case). With the same condition of the Theorem 3.3, the location of small inhomogeneities can be identified uniquely through the map of FITE(x).
In this section, a set of simulation results are shown to validate the investigated results of Theorems 3.1–3.3. To this end, we applied the background wavenumber of the form k=2π/λ with λ=0.6m, 0.4m, and 0.2m, and selected S=3 small circles with locations x1=(0.7m,0.5m), x2=(−0.7m,0.0m), and x3=(0.4m,−0.6m). The background permittivity and permeability are set to εb=μb=1, and the size, permittivity, and permeability of the inhomogeneities Σs are given in Tables 1 and 2. We selected M=64 and N=32, different transmitters and receivers with |tm|=3m and |rn|=2m (see Figure 3), and Ω as a square region [−1m,1m]×[−1m,1m]. With this, the scattered field data uscat(tm,rn) of K are generated by solving the Foldy-Lax formulation introduced in [32]. After generating the MSR matrix, 20dB white Gaussian random noise is added to show the robustness.
Permittivity contrast case | ε1 | ε2 | ε3 | α1 | α2 | α3 |
Case 1 | 5 | 5 | 5 | 0.1m | 0.1m | 0.1m |
Case 2 | 3 | 5 | 7 | 0.1m | 0.1m | 0.1m |
Case 3 | 5 | 5 | 5 | 0.15m | 0.1m | 0.05m |
Case 4 | 3 | 5 | 7 | 0.15m | 0.1m | 0.05m |
Permeability contrast case | μ1 | μ2 | μ3 | α1 | α2 | α3 |
Case 1 | 5 | 5 | 5 | 0.1m | 0.1m | 0.1m |
Case 2 | 3 | 5 | 7 | 0.1m | 0.1m | 0.1m |
Case 3 | 5 | 5 | 5 | 0.15m | 0.1m | 0.05m |
Case 4 | 3 | 5 | 7 | 0.15m | 0.1m | 0.05m |
Example 4.1 (Permittivity contrast case) Figure 4 shows the maps of FTM(x) for Cases 1–4. Based on the results, we determined that every location xs can be identified for Case 1. For Case 2, since ε3>ε2>ε1, the location x3 can be recognized clearly, but due to the appearance of several artifacts, identifying x1 is very difficult. Similarly, since α1>α2>α3 for Case 3, the location x1 can be identified through the map of FTM(x), but x3 cannot be recognized. Notice that the value of FTM(x2) is greater than the values in the neighborhood of x2, but it is difficult to distinguish from the artifacts. For Case 4, since
α21(ε1−εb)=0.045≈α22(ε2−εb)=0.040>α23(ε3−εb)=0.015, |
the locations x1 and x2 can be recognized. Similar to Case 3, although the value of FTM(x3) is greater than the values in the neighborhood of x3, it is difficult to distinguish from the artifacts.
Example 4.2 (Comparison with different algorithms). Figure 5 shows the imaging results obtained by MUSIC algorithm [55], subspace migration technique [56], and direct sampling method [39] to compare the imaging performances. Based on the simulation results, it is possible to recognize the existence of small inhomogeneities by using MUSIC (map of FMUSIC(r)) and subspace migration (map of FSUB(r)) very clearly. However, it is impossible to distinguish the characteristics (relative proportions of the size and permittivity) of inhomogeneities. It is interesting to observe that the imaging results by using the direct sampling method with multiple sources (map of FDSM(r)) are almost similar to the results via the maps of FTM(x) but map of the FDSM(r)) contains more artifacts than the one of the FTM(x).
Example 4.3 (Permeability contrast case). Figure 6 shows the maps of FTE(x) for Cases 1–4. Similar to the permittivity contrast case, we determined that every location xs can be identified for Case 1. For Case 2, since μ3>μ2>μ1, the rings in the neighborhood of all xs can be recognized, but the magnitudes of each ring are different. In contrast, since α1>α2>α3 for Case 3, the rings with large magnitudes in the neighborhood of x1 and x2 can be identified through the map of FTE(x). However, it is difficult to find a ring in the neighborhood of x3 so that the existence of Σ3 cannot be recognized. For Case 4, since
α21/(μ1+μb)=0.0056>α22/(μ2+μb)=0.0017≫α23/(μ3+μb)=3.1250e−04, |
the ring in the neighborhood of x1 can be recognized, but it is difficult to distinguish the ring in the neighborhood of x2 and the artifacts in the map. Furthermore, the ring in the neighborhood of x3 cannot be recognized because the value of α23/(μ3+μb) is close to zero.
Example 4.4 (Comparison with different algorithms). Figure 7 shows the imaging results obtained by MUSIC algorithm, subspace migration technique, and direct sampling method. Similar to the results in Example 4.2, it is possible to recognize the existence of small inhomogeneities through the maps of FMUSIC(r) and FSUB(r) by regarding the rings with large magnitudes but it is still impossible to distinguish size and permeability of inhomogeneities. Map of FTE(x) contains less artifacts than the map of FDSM(r) however, opposite to the results in Example 4.2, imaging results via the maps of FDSM(r) are quite different.
Example 4.5 (Accurate localization in permeability contrast case). Figure 8 shows the maps of FITE(x) for Cases 1–4. As we already discussed in the Remark 3.6, locations of all inhomogeneities are successfully identified for Cases 1 and 2. Moreover, similar to the Example 4.3, it is very difficult to recognize the location x3 for Cases 3 and 4. Notice that opposite to the results in Example 4.1, rings of large magnitudes are included in the neighborhood of all xs due to the effect of the factor J2(k|x−xs|)2 but they do not disturb the recognition of inhomogeneities.
Example 4.6 (Application of multiple frequencies). On the basis of several studies [2,5,28,29,30,38,56,61,65], we consider the multi-frequency imaging to improve the quality of the reconstruction. For this purpose, we introduce the following multi-frequency imaging functions
FMTM(x)=1PP∑p=1FTM(x,kp), |
FMTE(x)=1PP∑p=1FTE(x,kp), |
and
FMITE(x)=1PP∑p=1FITE(x,kp), |
where FTM(x,kp), FTE(x,kp), and FITE(x,kp) are the imaging functions at kp defined in (3.1), (3.5), and (3.11), respectively. Here, applied wavenumbers kp are equidistributed in the interval [k1,kP] with k1=2π/0.6 and kP=k10=2π/0.2.
Figure 9 exhibits multi-frequency imaging results. On the basis of the results, we can examine that several artifacts in the single-frequency imaging were successfully eliminated. Nevertheless, it is very difficult to recognize the inhomogeneity Σ3 for the Case 3 via the maps of FMTM(x), FMTE(x), and FMITE(x), and for the Case 4 via the maps of FMTE(x) and FMITE(x). Hence, development of imaging technique for a further improvement is required.
Subsequently, we show the simulation results using the Fresnel data introduced in [11] at various frequencies. The transmitters and receivers are placed on the circles with radii 0.72m and 0.76m, respectively, and the ROI Ω was chosen as the square [−0.15m,0.15m]×[−0.15m,0.15m]. Notably, due to the limitation of the experimental setting, the range of receivers is restricted from 60° to 300°, with a step size of 5° based on each direction of the transmitters. The transmitters are evenly distributed with step sizes of 10° from 0° to 350° (Figure 10). Thus, the simulation results are obtained with limited-aperture data, even though the theoretical results are derived under the full-aperture measurement hypothesis.
Example 4.7 (Imaging of dielectric objects: transverse magnetic case). Figure 11 shows maps of FTM(x) at various frequencies in the presence of single and multiple dielectric circular objects with the same radii in transverse magnetic (TM) polarization. As we already discussed in Remark 3.1, a blurred imaging result was obtained when f=1GHz. Moreover, the presence of the two objects at f=1GHz cannot be recognized because a peak of large magnitude appeared at their center. Fortunately, every location of objects can be identified by increasing the value of frequency e.g., f=4GHz and 8GHz.
Example 4.8 (Imaging of metallic objects: transverse magnetic case). Here, we consider the imaging of a small rectangular-shaped and U-shaped metallic objects in TM polarization. Figure 12 includes maps of FTM(x) with various frequencies. Based on the results, the center of the small rectangular-shaped object can be recognized for any frequency. Interestingly, unlike the other results, a considerable number of artifacts with small magnitudes are included in the map when f=8GHz. Notice that it is very hard to retrieve the shape of U-shaped object at any frequency. Hence, further improvement is required still.
Example 4.9 (Comparison with different methods: transverse magnetic case). Figures 13 and 14 exhibit the imaging results obtained by MUSIC algorithm and direct sampling method, respectively. Same as the results in Example 4.7 and Figure 11, it is impossible to recognize the existence of multiple objects when applied frequency is low (f=1GHz). Moreover, the shape of U-shaped object cannot be recognized through the MUSIC and direct sampling method for any frequency. It is interesting to examine the opposite to the synthetic data experiments (see Example 4.2 and Figure 5), it is very difficult to recognize the outline shape of multiple objects through the maps of MUSIC when a high frequency f=8GHz was applied.
Additionally, let us consider the imaging results of U-shaped object obtained by the topological derivative (TD) method introduced in [17,Figure 10]. Similar to the results in Figure 12, it is possible to recognize the existence of object at low frequency f=2GHz. Opposite to the results via the BFM, although some artifacts are also included, one can recognize some features of the shape via the TD at high frequencies f=10 and 12GHz.
Example 4.10 (Imaging of metallic object: transverse electric case). Now, we consider the imaging of a rectangular-shaped metallic object in transverse electric (TE) polarization. Figure 15 shows maps of FTE(x) at various frequencies. Similar to the results in Figure 6, we can observe that two peaks of large magnitudes are included in the map when f=2,4,6,8GHz instead of the true location of the object. As discussed in Remark 3.3, an almost exact location of the object is identified through the map when the value of the applied frequency is sufficiently large enough such as f=10GHz and 12GHz.
Example 4.11 (Comparison with different methods: transverse electric case). Figures 16 and 17 show the imaging results for a rectangular-shaped metallic object in transverse electric case through the MUSIC algorithm and direct sampling method, respectively. Similar to the results in Example 4.10, two peaks of large magnitudes were included in the map of FMUSIC(x) and FDSM(x) when f=2 and 4GHz. Moreover, an almost exact shape of the object is retrieved via the direct sampling method when a high frequency f=8GHz was applied. Opposite to the TM case in Example 4.9 and Figure 13, it is very difficult to recognize the shape of the object via MUSIC algorithm when high frequencies f=8, 10, and 12GHz were applied.
Example 4.12 (Accurate imaging of metallic object: transverse electric case). Here, we apply FITE(x) for identifying a rectangular-shaped metallic object in transverse electric (TE) polarization. Similar to the results in Example 4.5, the location of the object was successfully identified for various frequencies, refer to Figure 18. However, at the f=2GHz, it seems to be difficult to recognize the object due to the blurring effect in the neighborhood of the object. Similarly with the results in Example 4.10, some artifacts are also included in the map but there is no difficulty to recognize the location of object.
Example 4.13 (Multi-frequency imaging: both transverse magnetic and electric cases). For the final example, we consider the multi-frequency imaging to improve the quality of the reconstruction. For this purpose, we adopted multi-frequency imaging functions FMTM(x), FMTE(x), and FMITE(x) defined in Example 4.6. We applied frequency ranges from 1 to 8GHz in steps of 1GHz for imaging single and multiple circular objects in TM polarization, from 2 to 16GHz in steps of 2GHz for imaging rectangular-shaped object in both TM and TE polarizations and U-shaped metallic objects in TM polarization.
Figure 19 shows multi-frequency imaging results. Throughout the results, we can observe that most of objects were successfully retrieved in both TM and TE polarizations. Moreover, by regarding the large magnitudes in the map, an outline shape of U-shaped metallic object can be recognized. Hence, same as the previous studies, application of multiple frequencies on the BFM guarantees better imaging results.
We compare the imaging results via multi-frequency topological derivative (MFTD) method in TM polarization case, refer to Figures 11–14 in [17]. By comparing the results, we can observe that obtained imaging results are very close to the ones via MFTD hence, multi-frequency BFM will guarantee successful reconstruction of shape, size and number of objects.
We considered the BFM for identifying small 2D inhomogeneities when their permittivities or permeabilities differ from the homogeneous background. Through careful analysis, we show that the imaging function can be expressed by the characteristics (size, permittivity, and permeability) of the inhomogeneities and the Bessel function of orders zero and one for the permittivity and permeability contrast cases, respectively. Based on this, we observed and verified the applicability and several properties of the BFM. Numerical simulations with synthetic and experimental data were provided to support the theoretical result.
To achieve the best imaging of inhomogeneities in the permeability contrast case, an improved version of the BFM is needed. Improving the BFM to identify the exact locations of inhomogeneities for permeability contrast case will be the focus of future studies. Based on the experimental data experiments, we determined that the BFM is highly effective in the limited-aperture inverse scattering problem. The theoretical study of BFM in limited-aperture inverse scattering problem will be a significant research topic. Moreover, imaging results from experimental data indicate that the BFM is still effective in situations not reduced to the case of penetrable objects. Hence, extension to the imaging of metallic objects will be also an interesting research. Furthermore, application to the BFM with monostatic [40] and bistatic [41] measurement configuration will be the forthcoming work.
The authors declare they have not used Artificial Intelligence (AI) tools in the creation of this article.
The authors would like to acknowledge the anonymous reviewers for their comments that help to increase the quality of the paper. This work was supported by the research program of the Kookmin University.
The authors declare no conflicts of interest regarding the publication of this paper.
[1] |
S. Ahmad, T. Strauss, S. Kupis, T. Khan, Comparison of statistical inversion with iteratively regularized Gauss Newton method for image reconstruction in electrical impedance tomography, Appl. Math. Comput., 358 (2019), 436–448. https://doi.org/10.1016/j.amc.2019.03.063 doi: 10.1016/j.amc.2019.03.063
![]() |
[2] |
H. F. Alqadah, N. Valdivia, A frequency based constraint for a multi-frequency linear sampling method, Inverse Probl., 29 (2013), 095019. https://doi.org/10.1088/0266-5611/29/9/095019 doi: 10.1088/0266-5611/29/9/095019
![]() |
[3] |
H. Ammari, P. Garapon, F. Jouve, H. Kang, M. Lim, S. Yu, A new optimal control approach for the reconstruction of extended inclusions, SIAM J. Control Optim., 51 (2013), 1372–1394. https://doi.org/10.1137/100808952 doi: 10.1137/100808952
![]() |
[4] |
H. Ammari, J. Garnier, V. Jugnon, H. Kang, Stability and resolution analysis for a topological derivative based imaging functional, SIAM J. Control Optim., 50 (2012), 48–76. https://doi.org/10.1137/100812501 doi: 10.1137/100812501
![]() |
[5] |
H. Ammari, J. Garnier, H. Kang, W. K. Park, K. Sølna, Imaging schemes for perfectly conducting cracks, SIAM J. Appl. Math., 71 (2011), 68–91. https://doi.org/10.1137/100800130 doi: 10.1137/100800130
![]() |
[6] |
H. Ammari, E. Iakovleva, S. Moskow, Recovery of small inhomogeneities from the scattering amplitude at a fixed frequency, SIAM J. Math. Anal., 34 (2003), 882–900. https://doi.org/10.1137/S0036141001392785 doi: 10.1137/S0036141001392785
![]() |
[7] | H. Ammari, H. Kang, Reconstruction of small inhomogeneities from boundary measurements, Vol. 1846, Lecture Notes in Mathematics, Berlin: Springer-Verlag, 2004. https://doi.org/10.1007/b98245 |
[8] |
H. Ammari, S. Moskow, M. Vogelius, Boundary integral formulae for the reconstruction of electric and electromagnetic inhomogeneities of small volume, ESAIM: Control Optim. Calc. Var., 9 (2003), 49–66. https://doi.org/10.1051/cocv:2002071 doi: 10.1051/cocv:2002071
![]() |
[9] |
L. Audibert, H. Haddar, The generalized linear sampling method for limited aperture measurements, SIAM J. Imag. Sci., 10 (2017), 845–870. https://doi.org/10.1137/16M110112X doi: 10.1137/16M110112X
![]() |
[10] |
A. Baussard, D. Prémel, O. Venard, A Bayesian approach for solving inverse scattering from microwave laboratory-controlled data, Inverse Probl., 17 (2001), 1659. https://doi.org/10.1088/0266-5611/17/6/309 doi: 10.1088/0266-5611/17/6/309
![]() |
[11] |
K. Belkebir, M. Saillard, Special section: testing inversion algorithms against experimental data, Inverse Probl., 17 (2001), 1565. https://doi.org/10.1088/0266-5611/17/6/301 doi: 10.1088/0266-5611/17/6/301
![]() |
[12] |
K. Belkebir, A. G. Tijhuis, Modified2 gradient method and modified Born method for solving a two-dimensional inverse scattering problem, Inverse Probl., 17 (2001), 1671. https://doi.org/10.1088/0266-5611/17/6/310 doi: 10.1088/0266-5611/17/6/310
![]() |
[13] |
E. Bergou, Y. Diouane, V. Kungurtsev, Convergence and complexity analysis of a Levenberg–Marquardt algorithm for inverse problems, J. Optim. Theory Appl., 185 (2020), 927–944. https://doi.org/10.1007/s10957-020-01666-1 doi: 10.1007/s10957-020-01666-1
![]() |
[14] |
R. F. Bloemenkamp, A. Abubakar, P. M. van den Berg, Inversion of experimental multi-frequency data using the contrast source inversion method, Inverse Probl., 17 (2001), 1611. https://doi.org/10.1088/0266-5611/17/6/305 doi: 10.1088/0266-5611/17/6/305
![]() |
[15] |
O. Bondarenko, A. Kirsch, X. Liu, The factorization method for inverse acoustic scattering in a layered medium, Inverse Probl., 29 (2013), 045010. https://doi.org/10.1088/0266-5611/29/4/045010 doi: 10.1088/0266-5611/29/4/045010
![]() |
[16] |
A. Carpio, T. G. Dimiduk, F. L. Louër, M. L. Rapún, When topological derivatives met regularized Gauss–Newton iterations in holographic 3D imagingg, J. Comput. Phys., 388 (2019), 224–251. https://doi.org/10.1016/j.jcp.2019.03.027 doi: 10.1016/j.jcp.2019.03.027
![]() |
[17] |
A. Carpio, M. Pena, M. L. Rapún, Processing the 2D and 3D Fresnel experimental databases via topological derivative methodss, Inverse Probl., 37 (2021), 105012. https://doi.org/10.1088/1361-6420/ac21c8 doi: 10.1088/1361-6420/ac21c8
![]() |
[18] |
X. Chen, Subspace-based optimization method for inverse scattering problems with an inhomogeneous background medium, Inverse Probl., 26 (2010), 074007. https://doi.org/10.1088/0266-5611/26/7/074007 doi: 10.1088/0266-5611/26/7/074007
![]() |
[19] |
M. Cheney, The linear sampling method and the MUSIC algorithm, Inverse Probl., 17 (2001), 591. https://doi.org/10.1088/0266-5611/17/4/301 doi: 10.1088/0266-5611/17/4/301
![]() |
[20] |
S. Coşğun, E. Bilgin, M. Çayören, Microwave imaging of breast cancer with factorization method: SPIONs as contrast agent, Med. Phys., 47 (2020), 3113–3122. https://doi.org/10.1002/mp.14156 doi: 10.1002/mp.14156
![]() |
[21] |
L. Crocco, T. Isernia, Inverse scattering with real data: detecting and imaging homogeneous dielectric objects, Inverse Probl., 17 (2001), 1573. https://doi.org/10.1088/0266-5611/17/6/302 doi: 10.1088/0266-5611/17/6/302
![]() |
[22] | A. J. Deveney, Super-resolution processing of multi-static data using time-reversal and MUSIC, unpublished work, 2002. |
[23] |
O. Dorn, D. Lesselier, Level set methods for inverse scattering, Inverse Probl., 22 (2006), R67. https://doi.org/10.1088/0266-5611/22/4/R01 doi: 10.1088/0266-5611/22/4/R01
![]() |
[24] |
B. Duchêne, Inversion of experimental data using linearized and binary specialized nonlinear inversion schemes, Inverse Probl., 17 (2001), 1623. https://doi.org/10.1088/0266-5611/17/6/306 doi: 10.1088/0266-5611/17/6/306
![]() |
[25] |
L. Fatone, P. Maponi, F. Zirilli, An image fusion approach to the numerical inversion of multifrequency electromagnetic scattering data, Inverse Probl., 17 (2001), 1689. https://doi.org/10.1088/0266-5611/17/6/311 doi: 10.1088/0266-5611/17/6/311
![]() |
[26] |
M. Q. Feng, F. D. Flaviis, Y. J. Kim, Use of microwaves for damage detection of fiber reinforced polymer-wrapped concrete structures, J. Eng. Mech., 128 (2002), 172–183. https://doi.org/10.1061/(ASCE)0733-9399(2002)128:2(172) doi: 10.1061/(ASCE)0733-9399(2002)128:2(172)
![]() |
[27] |
A. Franchois, C. Pichot, Microwave imaging-complex permittivity reconstruction with a Levenberg-Marquardt method, IEEE Trans. Antenn. Propag., 45 (1997), 203–215. https://doi.org/10.1109/8.560338 doi: 10.1109/8.560338
![]() |
[28] |
J. F. Funes, J. M. Perales, M. L. Rapún, J. M. Vega, Defect detection from multi-frequency limited data via topological sensitivity, J. Math. Imaging Vis., 55 (2016), 19–35. https://doi.org/10.1007/s10851-015-0611-y doi: 10.1007/s10851-015-0611-y
![]() |
[29] |
R. Griesmaier, Multi-frequency orthogonality sampling for inverse obstacle scattering problems, Inverse Probl., 27 (2011), 085005. https://doi.org/10.1088/0266-5611/27/8/085005 doi: 10.1088/0266-5611/27/8/085005
![]() |
[30] |
B. B. Guzina, F. Cakoni, C. Bellis, On the multi-frequency obstacle reconstruction via the linear sampling method, Inverse Probl., 26 (2010), 125005. https://doi.org/10.1088/0266-5611/26/12/125005 doi: 10.1088/0266-5611/26/12/125005
![]() |
[31] |
I. Harris, D. L. Nguyen, Orthogonality sampling method for the electromagnetic inverse scattering problem, SIAM J. Sci. Comput., 42 (2020), B722–B737. https://doi.org/10.1137/19M129783X doi: 10.1137/19M129783X
![]() |
[32] |
K. Huang, K. Sølna, H. Zhao, Generalized Foldy-Lax formulation, J. Comput. Phys., 229 (2010), 4544–4553. https://doi.org/10.1016/j.jcp.2010.02.021 doi: 10.1016/j.jcp.2010.02.021
![]() |
[33] | D. Ireland, K. Bialkowski, A. Abbosh, Microwave imaging for brain stroke detection using Born iterative method IET Microw. Antenn. Propag., 7 (2013), 909–915. https://doi.org/10.1049/iet-map.2013.0054 |
[34] |
K. Ito, B. Jin, J. Zou, A direct sampling method to an inverse medium scattering problem, Inverse Probl., 28 (2012), 025003. https://doi.org/10.1088/0266-5611/28/2/025003 doi: 10.1088/0266-5611/28/2/025003
![]() |
[35] |
L. Jofre, A. Broquetas, J. Romeu, S. Blanch, A. P. Toda, X. Fabregas et al., UWB tomographic radar imaging of penetrable and impenetrable objects, Proc. IEEE, 97 (2009), 451–464. https://doi.org/10.1109/JPROC.2008.2008854 doi: 10.1109/JPROC.2008.2008854
![]() |
[36] |
S. Kang, S. Chae, W. K. Park, A study on the orthogonality sampling method corresponding to the observation directions configuration, Results Phys., 33 (2022), 105108. https://doi.org/10.1016/j.rinp.2021.105108 doi: 10.1016/j.rinp.2021.105108
![]() |
[37] |
S. Kang, M. Lambert, Structure analysis of direct sampling method in 3D electromagnetic inverse problem: near- and far-field configuration, Inverse Probl., 37 (2021), 075002. https://doi.org/10.1088/1361-6420/abfe4e doi: 10.1088/1361-6420/abfe4e
![]() |
[38] | S. Kang, M. Lambert, C. Y. Ahn, T. Ha, W. K. Park, Single- and multi-frequency direct sampling methods in limited-aperture inverse scattering problem, IEEE Access, 8 (2020), 121637–121649. https://doi.org/10.1109/ACCESS.2020.3006341 |
[39] |
S. Kang, M. Lambert, W. K. Park, Direct sampling method for imaging small dielectric inhomogeneities: analysis and improvement, Inverse Probl., 34 (2018), 095005. https://doi.org/10.1088/1361-6420/aacf1d doi: 10.1088/1361-6420/aacf1d
![]() |
[40] | S. Kang, M. Lambert, W. K. Park, Analysis and improvement of direct sampling method in the mono-static configuration, IEEE Geosci. Remote Sens. Lett., 16 (2019), 1721–1725. https://doi.org/10.1109/LGRS.2019.2906366 |
[41] |
S. Kang, M. Lim, W. K. Park, Fast identification of short, linear perfectly conducting cracks in the bistatic measurement configuration, J. Comput. Phys., 468 (2022), 111479. https://doi.org/10.1016/j.jcp.2022.111479 doi: 10.1016/j.jcp.2022.111479
![]() |
[42] |
S. Kang, W. K. Park, Application of MUSIC algorithm for a fast identification of small perfectly conducting cracks in limited-aperture inverse scattering problem, Comput. Math. Appl., 117 (2022), 97–112. https://doi.org/10.1016/j.camwa.2022.04.015 doi: 10.1016/j.camwa.2022.04.015
![]() |
[43] |
S. Kang, W. K. Park, S. H. Son, A qualitative analysis of bifocusing method for a real-time anomaly detection in microwave imaging, Comput. Math. Appl., 137 (2023), 93–101. https://doi.org/10.1016/j.camwa.2023.02.017 doi: 10.1016/j.camwa.2023.02.017
![]() |
[44] |
Y. J. Kim, L. Jofre, F. D. Flaviis, M. Q. Feng, Microwave reflection tomographic array for damage detection of civil structures, IEEE Trans. Antenn. Propag., 51 (2003), 3022–3032. https://doi.org/10.1109/TAP.2003.818786 doi: 10.1109/TAP.2003.818786
![]() |
[45] |
A. Kirsch, The MUSIC-algorithm and the factorization method in inverse scattering theory for inhomogeneous media, Inverse Probl., 18 (2002), 1025. https://doi.org/10.1088/0266-5611/18/4/306 doi: 10.1088/0266-5611/18/4/306
![]() |
[46] |
A. Kirsch, S. Ritter, A linear sampling method for inverse scattering from an open arc, Inverse Probl., 16 (2000), 89. https://doi.org/10.1088/0266-5611/16/1/308 doi: 10.1088/0266-5611/16/1/308
![]() |
[47] |
R. Kress, Newton's method for inverse obstacle scattering meets the method of least squares, Inverse Probl., 19 (2003), S91. https://doi.org/10.1088/0266-5611/19/6/056 doi: 10.1088/0266-5611/19/6/056
![]() |
[48] |
O. Kwon, J. K. Seo, J. R. Yoon, A real-time algorithm for the location search of discontinuous conductivities with one measurement, Commun. Pure Appl. Math., 55 (2002), 1–29. https://doi.org/10.1002/cpa.3009 doi: 10.1002/cpa.3009
![]() |
[49] |
L. J. Landau, Bessel functions: monotonicity and bounds, J. London Math. Soc., 61 (2000), 197–215. https://doi.org/10.1112/S0024610799008352 doi: 10.1112/S0024610799008352
![]() |
[50] | Z. Liu, A new scheme based on Born iterative method for solving inverse scattering problems with noise disturbance, IEEE Geosci. Remote Sens. Lett., 16 (2019), 1021–1025. https://doi.org/10.1109/LGRS.2019.2891660 |
[51] |
F. L. Louër, M. L. Rapún, Topological sensitivity for solving inverse multiple scattering problems in 3D electromagnetism. Part I: one step method, SIAM J. Imaging Sci., 10 (2017), 1291–1321. https://doi.org/10.1137/17M1113850 doi: 10.1137/17M1113850
![]() |
[52] |
R. Marklein, K. Balasubramanian, A. Qing, K. J. Langenberg, Linear and nonlinear iterative scalar inversion of multi-frequency multi-bistatic experimental electromagnetic scattering data, Inverse Probl., 17 (2001), 1597. https://doi.org/10.1088/0266-5611/17/6/304 doi: 10.1088/0266-5611/17/6/304
![]() |
[53] |
W. K. Park, Topological derivative strategy for one-step iteration imaging of arbitrary shaped thin, curve-like electromagnetic inclusions, J. Comput. Phys., 231 (2012), 1426–1439. https://doi.org/10.1016/j.jcp.2011.10.014 doi: 10.1016/j.jcp.2011.10.014
![]() |
[54] |
W. K. Park, Analysis of a multi-frequency electromagnetic imaging functional for thin, crack-like electromagnetic inclusions, Appl. Numer. Math., 77 (2014), 31–42. https://doi.org/10.1016/j.apnum.2013.11.001 doi: 10.1016/j.apnum.2013.11.001
![]() |
[55] |
W. K. Park, Asymptotic properties of MUSIC-type imaging in two-dimensional inverse scattering from thin electromagnetic inclusions, SIAM J. Appl. Math., 75 (2015), 209–228. https://doi.org/10.1137/140975176 doi: 10.1137/140975176
![]() |
[56] |
W. K. Park, Multi-frequency subspace migration for imaging of perfectly conducting, arc-like cracks in full- and limited-view inverse scattering problems, J. Comput. Phys., 283 (2015), 52–80. https://doi.org/10.1016/j.jcp.2014.11.036 doi: 10.1016/j.jcp.2014.11.036
![]() |
[57] |
W. K. Park, A novel study on subspace migration for imaging of a sound-hard arc, Comput. Math. Appl., 74 (2017), 3000–3007. https://doi.org/10.1016/j.camwa.2017.07.045 doi: 10.1016/j.camwa.2017.07.045
![]() |
[58] |
W. K. Park, Application of MUSIC algorithm in real-world microwave imaging of unknown anomalies from scattering matrix, Mech. Syst. Signal Proc., 153 (2021), 107501. https://doi.org/10.1016/j.ymssp.2020.107501 doi: 10.1016/j.ymssp.2020.107501
![]() |
[59] |
W. K. Park, Real-time detection of small anomaly from limited-aperture measurements in real-world microwave imaging, Mech. Syst. Signal Proc., 171 (2022), 108937. https://doi.org/10.1016/j.ymssp.2022.108937 doi: 10.1016/j.ymssp.2022.108937
![]() |
[60] |
W. K. Park, On the application of orthogonality sampling method for object detection in microwave imaging, IEEE Trans. Antenn. Propag., 71 (2023), 934–946. https://doi.org/10.1109/TAP.2022.3220033 doi: 10.1109/TAP.2022.3220033
![]() |
[61] |
W. K. Park, D. Lesselier, Reconstruction of thin electromagnetic inclusions by a level set method, Inverse Probl., 25 (2009), 085010. https://doi.org/10.1088/0266-5611/25/8/085010 doi: 10.1088/0266-5611/25/8/085010
![]() |
[62] |
R. Potthast, On the convergence of a new {N}ewton-type method in inverse scattering, Inverse Probl., 17 (2001), 1419. https://doi.org/10.1088/0266-5611/17/5/312 doi: 10.1088/0266-5611/17/5/312
![]() |
[63] |
C. Ramananjaona, M. Lambert, D. Lesselier, Shape inversion from TM and TE real data by controlled evolution of level sets, Inverse Probl., 17 (2001), 1585–1595. https://doi.org/10.1088/0266-5611/17/6/303 doi: 10.1088/0266-5611/17/6/303
![]() |
[64] |
F. Santosa, A level-set approach for inverse problems involving obstacles, ESAIM: Control Optim. Calc. Var., 1 (1996), 17–33. https://doi.org/10.1051/cocv:1996101 doi: 10.1051/cocv:1996101
![]() |
[65] |
J. D. Shea, P. Kosmas, S. C. Hagness, B. D. V. Veen, Three-dimensional microwave imaging of realistic numerical breast phantoms via a multiple-frequency inverse scattering technique, Med. Phys., 37 (2010), 4210–4226. https://doi.org/10.1118/1.3443569 doi: 10.1118/1.3443569
![]() |
[66] |
S. H. Son, W. K. Park, Application of the bifocusing method in microwave imaging without background information, J. Korean Soc. Ind. Appl. Math., 27 (2023), 109–122. https://doi.org/10.12941/jksiam.2023.27.109 doi: 10.12941/jksiam.2023.27.109
![]() |
[67] |
M. Testorf, M. Fiddy, Imaging from real scattered field data using a linear spectral estimation technique, Inverse Probl., 17 (2001), 1645. https://doi.org/10.1088/0266-5611/17/6/308 doi: 10.1088/0266-5611/17/6/308
![]() |
[68] |
A. G. Tijhuis, K. Belkebir, A. Litman, B. P. de Hon, Multiple-frequency distorted-wave Born approach to 2D inverse profiling, Inverse Probl., 17 (2001), 1635. https://doi.org/10.1088/0266-5611/17/6/307 doi: 10.1088/0266-5611/17/6/307
![]() |
[69] |
Y. Zhong, X. Chen, Twofold subspace-based optimization method for solving inverse scattering problems, Inverse Probl., 25 (2009), 085003. https://doi.org/10.1088/0266-5611/25/8/085003 doi: 10.1088/0266-5611/25/8/085003
![]() |
1. | Seong-Ho Son, Kwang-Jae Lee, Won-Kwang Park, Real-time tracking of moving objects from scattering matrix in real-world microwave imaging, 2024, 9, 2473-6988, 13570, 10.3934/math.2024662 | |
2. | Junyong Eom, Won-Kwang Park, Real-time detection of small objects in transverse electric polarization: Evaluations on synthetic and experimental datasets, 2024, 9, 2473-6988, 22665, 10.3934/math.20241104 |
Permittivity contrast case | ε1 | ε2 | ε3 | α1 | α2 | α3 |
Case 1 | 5 | 5 | 5 | 0.1m | 0.1m | 0.1m |
Case 2 | 3 | 5 | 7 | 0.1m | 0.1m | 0.1m |
Case 3 | 5 | 5 | 5 | 0.15m | 0.1m | 0.05m |
Case 4 | 3 | 5 | 7 | 0.15m | 0.1m | 0.05m |
Permeability contrast case | μ1 | μ2 | μ3 | α1 | α2 | α3 |
Case 1 | 5 | 5 | 5 | 0.1m | 0.1m | 0.1m |
Case 2 | 3 | 5 | 7 | 0.1m | 0.1m | 0.1m |
Case 3 | 5 | 5 | 5 | 0.15m | 0.1m | 0.05m |
Case 4 | 3 | 5 | 7 | 0.15m | 0.1m | 0.05m |
Permittivity contrast case | ε1 | ε2 | ε3 | α1 | α2 | α3 |
Case 1 | 5 | 5 | 5 | 0.1m | 0.1m | 0.1m |
Case 2 | 3 | 5 | 7 | 0.1m | 0.1m | 0.1m |
Case 3 | 5 | 5 | 5 | 0.15m | 0.1m | 0.05m |
Case 4 | 3 | 5 | 7 | 0.15m | 0.1m | 0.05m |
Permeability contrast case | μ1 | μ2 | μ3 | α1 | α2 | α3 |
Case 1 | 5 | 5 | 5 | 0.1m | 0.1m | 0.1m |
Case 2 | 3 | 5 | 7 | 0.1m | 0.1m | 0.1m |
Case 3 | 5 | 5 | 5 | 0.15m | 0.1m | 0.05m |
Case 4 | 3 | 5 | 7 | 0.15m | 0.1m | 0.05m |