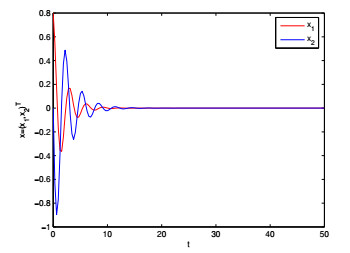
In this paper, we introduce a class of neutral-type neural networks with delay in the leakage terms. Using coincidence degree theory, Lyapunov functional method and the properties of neutral operator, we establish some new sufficient criteria for the existence and global attractiveness of periodic solutions. Finally, an example demonstrates our findings.
Citation: Shihong Zhu, Bo Du. Global attractive periodic solutions of neutral-type neural networks with delays in the leakage terms[J]. AIMS Mathematics, 2023, 8(11): 26731-26744. doi: 10.3934/math.20231368
[1] | Jin Gao, Lihua Dai . Weighted pseudo almost periodic solutions of octonion-valued neural networks with mixed time-varying delays and leakage delays. AIMS Mathematics, 2023, 8(6): 14867-14893. doi: 10.3934/math.2023760 |
[2] | Yijia Zhang, Tao Xie, Yunlong Ma . Robustness analysis of exponential stability of Cohen-Grossberg neural network with neutral terms. AIMS Mathematics, 2025, 10(3): 4938-4954. doi: 10.3934/math.2025226 |
[3] | Xiaofang Meng, Yongkun Li . Pseudo almost periodic solutions for quaternion-valued high-order Hopfield neural networks with time-varying delays and leakage delays on time scales. AIMS Mathematics, 2021, 6(9): 10070-10091. doi: 10.3934/math.2021585 |
[4] | Yunlong Ma, Tao Xie, Yijia Zhang . Robustness analysis of neutral fuzzy cellular neural networks with stochastic disturbances and time delays. AIMS Mathematics, 2024, 9(10): 29556-29572. doi: 10.3934/math.20241431 |
[5] | Călin-Adrian Popa . Neutral-type, leakage, and mixed delays in fractional-order neural networks: asymptotic synchronization analysis. AIMS Mathematics, 2023, 8(7): 15969-15992. doi: 10.3934/math.2023815 |
[6] | Abdulaziz M. Alanazi, R. Sriraman, R. Gurusamy, S. Athithan, P. Vignesh, Zaid Bassfar, Adel R. Alharbi, Amer Aljaedi . System decomposition method-based global stability criteria for T-S fuzzy Clifford-valued delayed neural networks with impulses and leakage term. AIMS Mathematics, 2023, 8(7): 15166-15188. doi: 10.3934/math.2023774 |
[7] | Huahai Qiu, Li Wan, Zhigang Zhou, Qunjiao Zhang, Qinghua Zhou . Global exponential periodicity of nonlinear neural networks with multiple time-varying delays. AIMS Mathematics, 2023, 8(5): 12472-12485. doi: 10.3934/math.2023626 |
[8] | Li Zhu, Er-yong Cong, Xian Zhang . Global exponential stability conditions for quaternion-valued neural networks with leakage, transmission and distribution delays. AIMS Mathematics, 2023, 8(8): 19018-19038. doi: 10.3934/math.2023970 |
[9] | M. Syed Ali, M. Hymavathi, Bandana Priya, Syeda Asma Kauser, Ganesh Kumar Thakur . Stability analysis of stochastic fractional-order competitive neural networks with leakage delay. AIMS Mathematics, 2021, 6(4): 3205-3241. doi: 10.3934/math.2021193 |
[10] | Shuting Chen, Ke Wang, Jiang Liu, Xiaojie Lin . Periodic solutions of Cohen-Grossberg-type Bi-directional associative memory neural networks with neutral delays and impulses. AIMS Mathematics, 2021, 6(3): 2539-2558. doi: 10.3934/math.2021154 |
In this paper, we introduce a class of neutral-type neural networks with delay in the leakage terms. Using coincidence degree theory, Lyapunov functional method and the properties of neutral operator, we establish some new sufficient criteria for the existence and global attractiveness of periodic solutions. Finally, an example demonstrates our findings.
In this paper, we are devoted to investigating a class of neutral-type neural networks with delay in the leakage terms as follows:
(xi(t)−ci(t)xi(t−σ))′=−aixi(t−τ)−bi∫∞0hi(s)xi(t−s)ds+n∑j=1dijfj(xj(t−γ(t)))+Ii(t), | (1.1) |
where i=1,2,⋯,n,ai,bi and dij are positive constants, σ,τ>0 are constant delays, ci(t),γ(t) and Ii(t) are ω-periodic continuous functions, hi∈C(R+,R+),fj(⋅)∈C(R,R),R+=[0,∞). For more detailed practical significance of system (1.1), refer to [1,2,3].
Leakage delay or forgetting delay widely exists in network systems, and its research has important practical value. In 2007, Gopalsamy [2] studied the following BAM neural networks with constant delays in the leakage terms:
{x′i(t)=−aixi(t−τ(1)i)+∑nj=1aijfj(yj(t−σ(2)j))+Ii,y′i(t)=−biyi(t−τ(2)i)+∑nj=1bijgj(xj(t−σ(1)j))+ji, | (1.2) |
where xi(t−τ(1)i) and yi(t−τ(2)i) are leakage terms, τ(1)i and τ(2)i are called leakage delays. Li and Cao [4] considered delay-dependent stability of neutral-type neural networks with delay in the leakage term as follows:
{x′i(t)=−Cx(t−σ)+Af(x(t−τ(t)))+B∫t−∞K(t−s)f(x(s))ds+Dx′(t−h(t))+J,t>0,x(s)=ϕ(s),s∈(−∞,0], | (1.3) |
where x(t−σ) is leakage term, σ is leakage delay. System (1.2) only contains constant delay, system (1.3) contains mixed delays including time-varying delays and continuously distributed delays. Peng [5] investigated the existence and global attractivity of periodic solutions for BAM neural networks with continuously distributed delays in the leakage terms:
{x′i(t)=−ai∫∞0h(1)i(s)xi(t−s)ds+∑pj=1aijfj(∫∞0hij(s)yj(t−s)ds)+Ii(t),y′j(t)=−bj∫∞0h(2)j(s)yj(t−s)ds+∑mi=1bjigi(∫∞0lji(s)xi(t−s)ds)+Jj(t), |
where xi(t−s) and yj(t−s) are leakage terms. In [6], the authors considered existence, uniqueness and the global asymptotic stability of fuzzy cellular neural networks with mixed delays by using the Lyapunov method and the linear matrix inequality approach. For more results about neural networks with delays in the leakage terms, see e.g [7,8,9,10,11].
The dynamic characteristics of the neutral-type neural networks with delay in the leakage terms have been widely studied and can be applied in various fields such as artificial neural networks, intelligent fuzzy recognition, automatic control, etc. In addition, due to the limited speed of information processing, the existence of time delays often leads to oscillation, divergence, or instability of neural networks. Therefore, It is important to consider the impact of delay on network stability. Many authors have extensively studied networks with various types of delays, see [12,13,14,15,16]. Neutral-type neural networks is a system which its delay term contains derivative. In fact, many dynamic systems can be modelled as neutral-type differential systems. Due to the fact that neutral-type systems are more extensive and complex than non neutral systems, they have always been a hot topic of research. Kong and Zhu [17] discussed the finite-time stabilization of a class of discontinuous fuzzy neutral-type neural networks with multiple time-varying delays. Zhang et al. [18] studied synchronization control of neutral-type neural networks with sampled-data via adaptive event-triggered communication scheme. Karthick et al. [19] investigated memory feedback finite-time control for memristive neutral-type neural networks with quantization by using proper Lyapunov Krasovskii functional and linear matrix inequalities. System (1.1) shows the neutral character by the operator (Aixi)(t)=xi(t)−ci(t)xi(t−σ),i=1,⋯,n, which is a D-operator form, see [20]. System (1.3) shows the neutral character by the term Dx′(t−h(t)) which is different from one in system (1.1). Since the D-operator (Aixi)(t) has many important properties, we can conveniently use these properties to study system (1.1). For more results about neutral-type neural networks, see [21,22,23,24].
We give the main contributions of this paper as follows:
(1) We first study a class neutral-type neural networks with time delay in the leakage term and D-operator form which is deferent from the existing neutral-type neural networks, see [18,19].
(2) System (1.1) includes leakage constant delays, time-varying delays and continuously distributed delays which generalizes the corresponding ones of [3,4,5]. The neural networks with leakage delays has extensive applications in real world. Therefore, our study has important practical value.
(3) Using properties of neutral-type operators, coincidence degree theory, Lyapunov functional method we obtain existence and global attractivity of periodic solutions to system (1.1). Our main results are also valid for the case of non neutral-type neural networks. The research methods in this paper can be used other type dynamic systems and neural networks.
The remainder of this paper are organized as follows: Section 2 gives some basic lemmas which can be used in this paper. In Section 3, we obtain some sufficient conditions for existence of periodic solution of system (1.1). Section 4 gives some sufficient conditions for guaranteeing the global attractivity of periodic solution of system (1.1). In Section 5, an example is given to show the effectiveness of the main results. Finally, some conclusions and discussions are given.
Let X and Y be two Banach spaces. Let L:D(L)⊂X→Y be a Fredholm operator with index zero which means that ImL is closed in Y and dimKerL=codimImL<+∞. Let projectors P:X→X,Q:Y→Y such that ImP=KerL,ImL=KerQ. Furthermore, LD(L)∩KerP:(I−P)X→ImL is invertible. Denote by Kp the inverse of LP.
Let Ω be an open bounded subset of X. Let the operator N:ˉΩ→Y be L-compact in ˉΩ which means that QN(ˉΩ) is bounded and the operator Kp(I−Q)N(ˉΩ) is relatively compact. We first give the famous Mawhin's continuation theorem.
Lemma 2.1. [25] Assume that X and Y are two Banach spaces, and L:D(L)⊂X→Y, is a Fredholm operator with index zero. Furthermore, Ω⊂X is an open bounded set and N:ˉΩ→Y is L-compact on ˉΩ. If all the following conditions hold:
(1) Lx≠λNx,\forallx∈∂Ω∩D(L),∀λ∈(0,1),
(2) Nx∉ImL,\forallx∈∂Ω∩KerL,
(3) deg{QN,Ω∩KerL,0}≠0.
Then equation Lx=Nx has a solution on ˉΩ∩D(L).
Let CT={x:x∈C(R,R),x(t+T)≡x(t)} with the norm ||x||=maxt∈[0,T]|x(t)|. Obviously, CT is a Banach space.
Lemma 2.2. [26] Let
A:CT→CT,[Ax](t)=x(t)−c(t)x(t−τ),∀t∈R, |
where CT is a T−periodic continuous function space, c(t)∈CT, τ>0 is a constant. If |c(t)|≠1, then operator A has continuous inverse A−1 on CT, satisfying
(1)
[A−1f](t)={f(t)+∞∑j=1j∏i=1c(t−(i−1)τ)f(t−jτ),c0<1,∀f∈CT,−f(t+τ)c(t+τ)−∞∑j=1j+1∏i=11c(t+iτ)f(t+jτ+τ),σ>1,∀f∈CT, |
(2)
∫T0|[A−1f](t)|dt≤{11−c0∫T0|f(t)|dt,c0<1,∀f∈CT,1σ−1∫T0|f(t)|dt,σ>1,∀f∈CT, |
(3)
|A−1f|0≤{11−c0|f|0,c0<1,∀f∈CT,1σ−1|f|0,σ>1,∀f∈CT, |
where c0=maxt∈[0,T]|c(t)|,σ=mint∈[0,T]|c(t)|. Throughout this paper, let
X=Y={x∈C(R,Rn):x(t+T)=x(t)} |
with the norm ||x||=maxi=1,⋯,n|xi|0, x=(x1,⋯,xn)T∈Rn,|xi|0=maxt∈[0,T]|xi(t)|.
For the sake of convenience, we list the following conditions.
(H1) There exists pj>0 such that
|fj(x)|≤pjforx∈R,j=1,⋯,n. |
(H2) There exists a constant D>0 such that
xjfj(xj)<0for|xj|>D,j=1,⋯,n. |
Theorem 3.1. Suppose that ∫∞0hi(s)ds=1,∫T0Ii(s)ds=0, ∫T0ψ(s)ψT(s)ds≠0, |ci(t)|≠1,1−γ′(t)>0 for all t∈R, and assumptions (H1)–(H2) hold, where ψ(t) is defined by (3.6). Then system (1.1) has at least one T-periodic solution, if
T(ai+bi+˜c)1−c0<1forc0<12, | (3.1) |
or if
T(ai+bi+˜c)˘c−1<1for˘c>1, | (3.2) |
where c0=max|ci(t)|,˘c=min|ci(t)|,˜c=max|c′i(t)|,t∈R,i=1,⋯,n.
Proof. Let (Aixi)(t)=xi(t)−ci(t)xi(t−σ),i=1,⋯,n. System (1.1) can be rewritten by the following system:
(Aixi)′(t)=−aixi(t−τ)−bi∫∞0hi(s)xi(t−s)ds+n∑j=1dijfj(xj(t−γ(t)))+Ii(t). | (3.3) |
Let
L:D(L)⊂X→Y,(Lx)(t)=(Ax)′(t), | (3.4) |
where
(Lx)(t)=((L1x1)(t),⋯,(Lnxn)(t))T,(Ax)(t)=((A1x1)(t),⋯,(Anxn)(t))T,(Lixi)(t)=(Aixi)′(t). |
Define N:ˉΩ⊂X→Y by
(Nixi)(t)=−aixi(t−τ)−bi∫∞0hi(s)xi(t−s)ds+n∑j=1dijfj(xj(t−γ(t)))+Ii(t), | (3.5) |
where (Nx)(t)=((N1x1)(t),⋯,(Nnxn)(t))T. Thus, system (3.3) is equivalent to the following operator system:
(Lixi)(t)=(Nixi)(t),i=1,⋯,n, |
where Li and Ni are defined by (3.4) and (3.5), respectively. Obviously, ImL={y:y∈Y,∫T0y(t)dt=0}. For each x∈KerL, we get (x(t)−c(t)x(t−σ))′=0. Without loss of generality, let
x(t)−c(t)x(t−σ)=I, | (3.6) |
where I is a n×n identity matrix. Let ψ(t)∈Rn be the unique T−periodic solution of (3.6), then ψ(t)≠0 and KerL={aψ(t):a∈Rn}. It is to see that ImL is closed in Y and dimKerL=codimImL=n. So L is a Fredholm operator with index zero. Define continuous projectors P and Q by, respectively,
P:X→KerL,(Px)(t)=∫T0x(t)ψ(t)dt∫T0ψ(t)ψT(t)dtψ(t) |
and
Q:Y→Y/ImL,Qy=1T∫T0y(s)ds, |
where ψ is defined by (3.6). Let
LP=L|D(L)∩KerP:D(L)∩KerP→ImL, |
then
L−1P=Kp:ImL→D(L)∩KerP. |
Since Kp is an embedding operator, then Kp is a completely operator in ImL. By the definitions of Q and N, it knows that QN(ˉΩ) is bounded on ˉΩ. Hence nonlinear operator N is L-compact on ¯Ω.
For any λ∈(0,1),i=1,⋯,n, consider the following operator system:
(Lixi)(t)=λ(Nixi)(t), |
i.e.,
(Aixi)′(t)=−λaixi(t−τ)−λbi∫∞0hi(s)xi(t−s)ds+λn∑j=1dijfj(xj(t−γ(t)))+λIi(t). | (3.7) |
Integrating both sides of (3.7) on [0,T], one can see
∫T0[−aixi(t)−bi∫∞0hi(s)xi(t−s)ds+n∑j=1dij1−γ′(˜γ(t))fj(xj(t))]dt=0, | (3.8) |
where ˜γ(t) is a inverse function of t−γ(t). We claim that there exists a point t1∈[0,T] such that
|xi(t1)|≤D,i=1,⋯,n, | (3.9) |
where D is defined by assumption (H2). If |x(t)|>D for all t∈[0,T], then by assumption (H2) we get
∫T0[−aixi(t)−bi∫∞0hi(s)xi(t−s)ds+n∑j=1dij1−γ′(v(t))fj(xj(t))]dt≠0, |
which contradicts (3.8). Hence, (3.9) holds. From (3.9) and assumption (H1), one can see
|xi|0≤D+∫T0|x′i(s)|ds | (3.10) |
and
|(Aixi)′|0≤(ai+bi)|xi|0+n∑j=1dijpj+|Ii|0. | (3.11) |
From (Aixi)(t)=xi(t)−ci(t)xi(t−σ), we have
(Aix′i)(t)=(Aixi)′(t)+c′i(t)xi(t−σ). | (3.12) |
If c0<12, in view of Lemma 2.2, (3.11) and (3.12), we have
∫T0|x′i(t)|dt=∫T0|(A−1iAix′i)(t)|dt≤∫T0|(Aix′i)(t)|1−c0dt=∫T0|(Aixi)′(t)+c′i(t)xi(t−σ)|1−c0dt≤T1−c0(|(Aixi)′|0+˜c|xi|0)≤T(ai+bi+˜c)1−c0|xi|0+T1−c0(n∑j=1dijpj+|Ii|0). | (3.13) |
From (3.10) and (3.13), we have
|xi|0≤D+T(ai+bi+˜c)1−c0|xi|0+T1−c0(n∑j=1dijpj+|Ii|0). | (3.14) |
It follows by (3.1) and (3.14) that
|xi|0≤(1−c0)D1−T(ai+bi+˜c)+T1−T(ai+bi+˜c)(n∑j=1dijpj+|Ii|0)≤M1. | (3.15) |
On the other hand, if ˘c>1, similarly to the above proof, by (3.2), there exists a constant M2>0 such that
|xi|0≤M2. | (3.16) |
From (3.15) and (3.16), we have
|xi|0≤max{M1,M2}=M3. | (3.17) |
Let Ω1={x∈KerL:QNx=0}. We show that Ω1 is a bounded set. For each x∈Ω1, there exists xi=ηiψi(t) such that
∫T0[−aiηiψi(t)−bi∫∞0hi(s)ηiψi(t)(t−s)ds+n∑j=1dij1−γ′(v(t))fj(ηjψj(t)(t))]dt=0, | (3.18) |
where i=1,⋯,n,ηi∈R. When c0<12, we have
ψi(t)=A−1i(1)=1+∞∑j=1j∏i=1ci(t−(i−1)σ)≤1+∞∑j=1j∏i=1c0=1−c01−c0:=γ1>0. |
Then, we have ηi≤Dγ1. Otherwise, for all t∈[0,T], we have ηiψi(t)>D and
∫T0[−aiηiψi(t)−bi∫∞0hi(s)ηiψi(t)(t−s)ds+n∑j=1dij1−γ′(v(t))fj(ηjψj(t)(t))]dt≠0, |
which contradicts (3.18). On the other hand, when ˘c>1, we have
ψi(t)=A−1i(1)=−1ci(t+τ)−∞∑j=1j+1∏i=11ci(t+iτ)≤−1˘c−∞∑j=1j+1∏i=11˘c=−1˘c−1:=γ2<0. |
Then, we have ηi≤−Dγ2. Otherwise, for all t∈[0,T], we have ηiψi(t)<−D and
∫T0[−aiηiψi(t)−bi∫∞0hi(s)ηiψi(t)(t−s)ds+n∑j=1dij1−γ′(v(t))fj(ηjψj(t)(t))]dt≠0, |
which contradicts (3.18). Hence, Ω1 is a bounded set. Denote
|ηiψi(t)|≤M4andM=max{M3,M4}+1, |
where M3 is defined by (3.17). Let Ω={x∈X:||x||<M}. From the above proof, conditions (1) and (2) of Lemma 2.1 hold. Now, we show that condition (3) of Lemma 2.1 holds. Take the homotopy
H(x,κ)=−κx+(1−κ)QNx,x∈ˉΩ∩KerL,κ∈[0,1]. |
We claim that
H(x,κ)≠0forallx∈∂Ω∩KerL. | (3.19) |
If (3.19) does not hold, then
κxi=1−κT∫T0[−aixi(t)−bi∫∞0hi(s)xi(t−s)ds+n∑j=1dij1−γ′(˜γ(t))fj(xj(t))]dt. | (3.20) |
Since |xi|>D, then (3.20) does not holds. Hence, (3.19) holds. So we have
deg{QN,Ω∩KerL,0}=deg{H(⋅,0),Ω∩KerL,0}=deg{H(⋅,1),Ω∩KerL,0}≠0. |
So, condition (3) of Lemma 2.1 holds. Applying Lemma 2.1, we reach the conclusion.
Remark 3.1. Lemma 2.1 is critical for estimating the prior bound of solution to system (1.1). In this section, we obtain the existence results of periodic solutions when the neutral operator is stable (|ci(t)|<1) or unstable (|ci(t)|>1). Therefore, the existence results of this article have broader theoretical and practical value.
For any t0≥0, we give the initial conditions associated with system (1.1) as follows:
xi(s)=ϕi(s),−∞<s≤t0,i=1,⋯,n, | (4.1) |
where ϕi∈C((−∞,t0),R) and ||ϕ||<∞.
Definition 4.1. Suppose that ˉx(t)=(ˉx1(t),⋯,ˉxn(t))T is a periodic solution of system (1.1), and x(t)=(x1(t),⋯,xn(t))T is a any solution of system (1.1). Let ui(t)=xi(t)−ˉxi(t),i=1,⋯,n. If
limt→+∞n∑i=1|ui(t)|=0, |
we call ˉx(t) is globally attractive.
Theorem 4.1. Assume that all conditions of Theorem 3.1 hold and ∫∞0shi(s)ds<∞. Suppose that
(H3) There exists Lj>0 such that
|fj(x)−fj(y)|≤Lj|x−y|forx,y∈R,j=1,⋯,n. |
Then system (1.1) has an T-periodic solution which is globally attractive provided that
qi=bi−3ai−αi−2b2iξi−2bi|ci|0−|ci|0αi−3|ci|0ai−2ξiaibi−2b2i−aibi−biαi−ρi>0, | (4.2) |
where i=1,⋯,n,ξi=∫∞0shi(s)ds,αi=∑nj=1dijLj,
ρi=maxt∈Rn∑j=1(dijLj+|ci|0+biξi)ω(t), |
ω(t)=11−γ′(˜γ(t)),˜γ(t) is an inverse function of t−γ(t).
Proof. From Theorem 3.1, system (1.1) has a T−periodic solution ˉx(t)=(ˉx1(t),⋯,ˉxn(t))T. Suppose that x(t)=(x1(t),⋯,xn(t))T is a any solution of system (1.1). Let
ui(t)=xi(t)−ˉxi(t),i=1,⋯,n. |
For t≥t0, we have
(Aiui)′(t)=−aiui(t−τ)−bi∫∞0hi(s)ui(t−s)ds+n∑j=1dij[fj(xj(t−γ(t)))−fj(ˉxj(t−γ(t)))]. | (4.3) |
Let
Vi(t)=[(Aiui)(t)−bi∫∞0hi(s)∫tt−sui(θ)dθds]2,i=1,⋯,n. | (4.4) |
Derivation of (4.4) along the solution of (4.3) gives
V′i(t)=2[(Aiui)(t)−bi∫∞0hi(s)∫tt−sui(θ)dθds][(Aiui)′(t)−biui+ai∫∞0hi(s)ui(t−s)ds]=−2aiui(t)ui(t−τ)+2aici(t)ui(t−σ)ui(t−τ)−2biui(t)∫∞0hi(s)ui(t−s)ds+2bici(t)ui(t−σ)∫∞0hi(s)ui(t−s)ds+2(ui(t)−ci(t)ui(t−σ))n∑j=1dij[fj(xj(t−γ(t)))−fj(ˉxj(t−γ(t)))]−2biu2i(t)+2bici(t)ui(t)ui(t−σ)+2aiui(t)∫∞0hi(s)ui(t−s)ds−2ci(t)aiui(t−σ)∫∞0hi(s)ui(t−s)ds+2aibiui(t−τ)∫∞0hi(s)∫tt−sui(θ)dθds+2b2i∫∞0hi(s)ui(t−s)ds∫∞0hi(s)∫tt−sui(θ)dθds−2bi∫∞0hi(s)∫tt−sui(θ)dθdsn∑j=1dij[fj(xj(t−γ(t)))−fj(ˉxj(t−γ(t)))]+2b2iui(t)∫∞0hi(s)∫tt−sui(θ)dθds−2aibi∫∞0hi(s)∫tt−sui(θ)dθds∫∞0hi(s)ui(t−s)ds. | (4.5) |
From (4.5) and a lengthy simplification (by using a2+b2≥2ab), we have
V′i(t)≤−(bi−ai−αi−b2iξi)u2i(t)+(bi|ci|0+|ci|0αi+|ci|0ai)u2i(t−σ)+(ai+|ci|0ai+ξiaibi)u2i(t−τ)+(b2i+bi|ci|0+ai+ai|ci|0+b2iξi+aibiξi)∫∞0hi(s)u2i(t−s)ds+n∑j=1(dijLj+|ci|0+biξi)|uj(t−γ(t))|2+(aibi+b2i+biαi)∫∞0hi(s)∫tt−su2i(θ)dθds. | (4.6) |
Let
Vσi(t)=∫tt−σ(bi|ci|0+|ci|0αi+|ci|0ai)u2i(s)ds, | (4.7) |
Vτi(t)=∫tt−τ(ai+|ci|0ai+ξiaibi)u2i(s)ds, | (4.8) |
Vγi(t)=n∑j=1(dijLj+|ci|0+biξi)∫tt−γ(t)ω(s)u2j(s)ds, | (4.9) |
Vhi(t)=(b2i+bi|ci|0+ai+ai|ci|0+b2iξi+aibiξi)∫∞0hi(s)∫tt−su2i(θ)dθds, | (4.10) |
V˜hi(t)=(aibi+b2i+biαi)∫∞0hi(s)∫tt−s∫tνu2i(θ)dθdνds. | (4.11) |
From (4.7)–(4.11), we have
V′σi(t)=(bi|ci|0+|ci|0αi+|ci|0ai)(u2i(t)−u2i(t−σ)), | (4.12) |
V′τi(t)=(ai+|ci|0ai+ξiaibi)(u2i(t)−(u2i(t−τ)), | (4.13) |
V′γi(t)=n∑j=1(dijLj+|ci|0+biξi)(ω(t)u2j(t)−u2j(t−γ(t))), | (4.14) |
V′hi(t)=(b2i+bi|ci|0+ai+ai|ci|0+b2iξi+aibiξi)(u2i(t)−∫∞0hi(s)u2i(t−s)ds), | (4.15) |
V′˜hi(t)=(aibi+b2i+biαi)(ξiu2i(t)−∫∞0hi(s)∫tt−su2i(θ)dθds). | (4.16) |
Construct the following Lyapunov functional:
V(t)=n∑i=1(Vi(t)+Vσi(t)+Vτi(t)+Vγi(t)+Vhi(t)+V˜hi(t)). | (4.17) |
From (4.6) and (4.12)–(4.17), we have
V′(t)≤n∑i=1−qiu2i(t)fort≥t0, | (4.18) |
where qi is defined by (4.2). In view of (4.1), we can see V(t0)<∞. Thus,
V(t)≤V(t0) |
and
|(Aiui)(t)−bi∫∞0hi(s)∫tt−sui(θ)dθds|≤√V(t0). |
Therefore,
|(Aiui)(t)|≤√V(t0)1−biξiΞ |
and
|ui(t)|=|(A−1iAiui)(t)|≤Ξ√V(t0)1−biξiΞ, | (4.19) |
where Ξ=max{11−c0,1˘c−1}. Since V(t0) can be made arbitrarily small for sufficient small initial values, it follows by (4.19) that the solution of system (1.1) is uniformly bounded on [t0,∞). By (4.19) we have
V(t)+∫tt0n∑i=1qiu2i(s)ds≤V(t0)<∞. |
In view of Barbalat's lemma (see Gopalsamy [27]), we get
ui(t)→0ast→+∞,i=1,⋯,n. |
This completes the proof of the global attractivity of T-periodic solution of system (1.1).
Remark 4.1. Since there is no fixed method for constructing Lyapunov functional, constructing proper Lyapunov functional is very difficult. In this section, utilizing the properties of neutral operators, we construct a new Lyapunov functional which has important application for the proof of Theorem 4.1.
Consider the following two-neuron neural networks with delay in the leakage terms:
{(x1(t)−0.01sintx1(t−π))′=−0.02x1(t−π)−0.5∫∞0h1(s)x1(t−s)ds+2∑j=1d1jfj(xj(t−γ(t))),(x2(t)−0.01costx2(t−π))′=−0.02x2(t−π)−0.5∫∞0h2(s)x2(t−s)ds+n∑j=1d2jfj(xj(t−γ(t))), | (5.1) |
where
c1(t)=0.01sin10t,c2(t)=0.01cos10t,σ=τ=π,a1=a2=0.02,b1=b2=0.5, |
d11=0.0025,d12=0.0015,d21=0.002,d22=0.001, |
h1(s)=h2(s)=2√πe−s22,f1(x)=f2(x)=−0.01xx2+1,γ(t)=0.005cos10t. |
Obviously, we have
T=π5,c0=|c1|0=|c2|0=0.01,˜c=0.1,L1=L2=0.01,ξ1=ξ2≈0.55, |
α1=4×10−5,α2=3×10−5,η1≈0.273,η2≈0.264. |
When c0=0.01<12, we have
T(ai+bi+˜c)1−c0≈0.39<1,i=1,2. |
Thus, condition (3.1) in Theorem 3.1 holds and system (5.1) exists a periodic solution x=(0,0)T. Furthermore, we have q1≈0.122>0 and q2≈0.113>0 and condition (4.2) in Theorem 4.1 holds and the periodic solution x=(0,0)T of system (5.1) is globally attractive. Figure 1 shows that the state trajectory of the system (5.1). From Figure 1, we find that the periodic solution x=(0,0)T of system (5.1) is globally attractive which verifies the correctness of the conclusions of Theorems 3.1 and 4.1.
In this paper, we have dealt with the existence and global attractivity of periodic solution to a class of neutral-type neural networks with delay in the leakage terms. System (1.1) contains mixed delays including leakage constant delays, time-varying delays and continuously distributed delays. As is well known, delay has a fundamental impact on the dynamic behavior of network systems. The leakage delay is a special type of delay, and its research has important theoretical and practical value. From main results of this paper, we find that the leakage term has great impact on the dynamical properties of neural networks. our results greatly generalize the corresponding ones of [3,4,5,6]. Based on coincidence degree theory and the Lyapunov functional, some sufficient conditions ensuring the existence, and global attractivity of periodic solution have been presented. It should be pointed out that by utilizing the properties of neutral operators, we have constructed a new Lyapunov functional that can conveniently obtain the dynamic behavior of periodic solutions. A numerical example has been illustrated to demonstrate the usefulness of the proposed method. The research method in this article can be used to study various types of systems, neutral-type neural networks with delay in the leakage terms, for example, neutral-type neural networks with impulses and delay in the leakage terms, neutral-type neural networks with stochastic disturbances and delay in the leakage terms, and so on.
The authors declare they have not used Artificial Intelligence (AI) tools in the creation of this article.
This paper is supported by Anhui University Natural Sciences fund: Kj2019A0703.
The authors declare no conflict of interest.
[1] |
L. O. Chua, L. Yang, Cellular neural networks: Theory, IEEE Trans. Circuits Syst., 35 (1988), 1257–1272. https://doi.org/10.1109/31.7600 doi: 10.1109/31.7600
![]() |
[2] | K. Gopalsamy, Leakage delays in BAM, J. Math. Anal. Appl., 325 (2007), 1117–1132. https://doi.org/10.1016/j.jmaa.2006.02.039 |
[3] |
C. Li, T. Huang, On the stability of nonlinear systems with leakage delay, J. Franklin Inst., 346 (2009), 366–377. https://doi.org/10.1016/j.jfranklin.2008.12.001 doi: 10.1016/j.jfranklin.2008.12.001
![]() |
[4] |
X. Li, J. Cao, Delay-dependent stability of neural networks of neutral type with time delay in the leakage term, Nonlinearity, 23 (2010), 1709–1726. https://doi.org/10.1088/0951-7715/23/7/010 doi: 10.1088/0951-7715/23/7/010
![]() |
[5] |
S. Peng, Global attractive periodic solutions of BAM neural networks with continuously distributed delays in the leakage terms, Nonlinear Anal. Real World Appl., 11 (2010), 2141–2151. https://doi.org/10.1016/j.nonrwa.2009.06.004 doi: 10.1016/j.nonrwa.2009.06.004
![]() |
[6] |
X. Li, R. Rakkiyappan, P. Balasubramaniam, Existence and global stability analysis of equilibrium of fuzzy cellular neural networks with time delay in the leakage term under impulsive perturbations, J. Franklin Inst., 348 (2011), 135–155. https://doi.org/10.1016/j.jfranklin.2010.10.009 doi: 10.1016/j.jfranklin.2010.10.009
![]() |
[7] | M. Ali, M. Hymavathi, B. Priya, S. Kauser, G. Thakur, The periodic Ambrosetti-Prodi problem for nonlinear perturbations of the p-Laplacian, AIMS Mathematics, 6 (2021), 3205–3241. |
[8] |
L. Zhang, Q. Song, Z. Zhao, Stability analysis of fractional-order complex-valued neural networks with both leakage and discrete delays, Appl. Math. Comput., 298 (2017), 296–309. https://doi.org/10.1016/j.amc.2016.11.027 doi: 10.1016/j.amc.2016.11.027
![]() |
[9] |
A. Singh, J. N. Rai, Stability analysis of fractional order fuzzy cellular neural networks with leakage delay and time varying delays, Chinese J. Phys., 73 (2021), 589–599. https://doi.org/10.1016/j.cjph.2021.07.029 doi: 10.1016/j.cjph.2021.07.029
![]() |
[10] |
C. D. Zheng, Y. Wang, Z. Wang, Stability analysis of stochastic fuzzy Markovian jumping neural networks with leakage delay under impulsive perturbations, J. Franklin Inst., 351 (2014), 1728–1755. https://doi.org/10.1016/j.jfranklin.2013.12.013 doi: 10.1016/j.jfranklin.2013.12.013
![]() |
[11] |
J. Alzabut, S. Tyagi, S. Abbas, Discrete fractional-order BAM neural networks with leakage delay: Existence and stability results, Asian J. Control, 22 (2020), 143–155. https://doi.org/10.1002/asjc.1918 doi: 10.1002/asjc.1918
![]() |
[12] |
T. Zhang, L. Xiong, Periodic motion for impulsive fractional functional differential equations with piecewise Caputo derivative, Appl. Math. Lett., 101 (2020), 106072. doilinkhttps://doi.org/10.1016/j.aml.2019.106072 doi: 10.1016/j.aml.2019.106072}
![]() |
[13] |
T. Zhang, Y. Li, Global exponential stability of discrete-time almost automorphic Caputo CFabrizio BAM fuzzy neural networks via exponential Euler technique, Knowledge-Based Systems, 246 (2022), 108675. https://doi.org/10.1016/j.knosys.2022.108675 doi: 10.1016/j.knosys.2022.108675
![]() |
[14] |
T. Zhang, H. Qu, J. Zhou, Asymptotically almost periodic synchronization in fuzzy competitive neural networks with Caputo-Fabrizio operator, Fuzzy Sets Syst., 471 (2023), 108676. https://doi.org/10.1016/j.fss.2023.108676 doi: 10.1016/j.fss.2023.108676
![]() |
[15] |
X. Yang, F. Li, Y. Long, X. Cui, Existence of periodic solution for discrete-time cellular neural networks with complex deviating arguments and impulses, J. Franklin Inst., 347 (2010), 559-566. https://doi.org/10.1016/j.jfranklin.2009.12.004 doi: 10.1016/j.jfranklin.2009.12.004
![]() |
[16] |
J. H. Park, Further result on asymptotic stability criterion of cellular neural networks with time-varying discrete and distributed delays, Appl. Math. Comput., 182 (2006), 1661–1666. https://doi.org/10.1016/j.amc.2006.06.005 doi: 10.1016/j.amc.2006.06.005
![]() |
[17] |
F. Kong, Q. Zhu, Finite-time stabilization of discontinuous fuzzy neutral-type neural networks with D operator and multiple time-varying delays, Fuzzy Sets Syst., 449 (2022), 32–55. https://doi.org/10.1016/j.fss.2022.02.006 doi: 10.1016/j.fss.2022.02.006
![]() |
[18] |
H. Zhang, Q. Ma, J. Lu, Y. Chu, Y. Li, Synchronization control of neutral-type neural networks with sampled-data via adaptive event-triggered communication scheme, J. Franklin Inst., 358 (2021), 1999–2014. https://doi.org/10.1016/j.jfranklin.2021.01.005 doi: 10.1016/j.jfranklin.2021.01.005
![]() |
[19] |
S. A. Karthick, R. Sakthivel, C. Aouiti, A. Leelamani, Memory feedback finite-time control for memristive neutral-type neural networks with quantization, Chinese J. Phys., 70 (2021), 271–287. https://doi.org/10.1016/j.cjph.2019.09.016 doi: 10.1016/j.cjph.2019.09.016
![]() |
[20] | J. Hale, Theory of functional differential equations, New York: Springer-Verlag, 1993. |
[21] |
S. Saravanan, M. S. Ali, A. Alsaedi, B. Ahmad, Finite-time passivity for neutral-type neural networks with time-varying delays via auxiliary function-based integral inequalities, Nonlinear Anal. Model. Control, 25 (2020), 206–224. https://doi.org/10.15388/namc.2020.25.16513 doi: 10.15388/namc.2020.25.16513
![]() |
[22] |
X. Zhang, X. F. Fan, Y. Xue, Y. T. Wang, W. Cai, Robust exponential passive filtering for uncertain neutral-type neural networks with time-varying mixed delays via Wirtinger-based integral inequality, Int. J. Control Autom. Syst., 15 (2017), 585–594. https://doi.org/10.1007/s12555-015-0441-0 doi: 10.1007/s12555-015-0441-0
![]() |
[23] |
R. Rakkiyappan, P. Balasubramaniam, New global exponential stability results for neutral type neural networks with distributed time delays, Neurocomputing, 71 (2008), 1039–1045. https://doi.org/10.1016/j.neucom.2007.11.002 doi: 10.1016/j.neucom.2007.11.002
![]() |
[24] |
J. Park, O. M. Kwon, Global stability for neural networks of neutral-type with interval time-varying delays, Chaos Solitons Fractals, 41 (2009), 1174–1181. https://doi.org/10.1016/j.chaos.2008.04.049 doi: 10.1016/j.chaos.2008.04.049
![]() |
[25] | R. E. Gaines, J. Mawhin, Coincidence degree and nonlinear differential equations, In: Lecture notes in mathematics, Berlin: Springer, 568 (1977). |
[26] |
B. Du, L. Guo, W. Ge, S. Lu, Periodic solutions for generalized Liˊenard neutral equation with variable parameter, Nonlinear Anal., 70 (2009), 2387–2394. https://doi.org/10.1016/j.na.2008.03.021 doi: 10.1016/j.na.2008.03.021
![]() |
[27] |
K. Gopalsamy, X. Z. He, Delay-independent stability in bidirectional associative memory networks, IEEE Trans. Neural Networ., 5 (1994), 998–1002. https://doi.org/10.1109/72.329700 doi: 10.1109/72.329700
![]() |