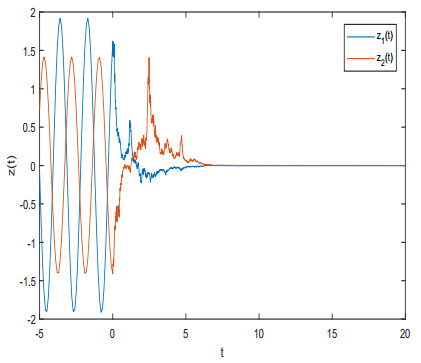
Citation: M. Syed Ali, M. Hymavathi, Bandana Priya, Syeda Asma Kauser, Ganesh Kumar Thakur. Stability analysis of stochastic fractional-order competitive neural networks with leakage delay[J]. AIMS Mathematics, 2021, 6(4): 3205-3241. doi: 10.3934/math.2021193
[1] | Jin Gao, Lihua Dai . Weighted pseudo almost periodic solutions of octonion-valued neural networks with mixed time-varying delays and leakage delays. AIMS Mathematics, 2023, 8(6): 14867-14893. doi: 10.3934/math.2023760 |
[2] | Abdulaziz M. Alanazi, R. Sriraman, R. Gurusamy, S. Athithan, P. Vignesh, Zaid Bassfar, Adel R. Alharbi, Amer Aljaedi . System decomposition method-based global stability criteria for T-S fuzzy Clifford-valued delayed neural networks with impulses and leakage term. AIMS Mathematics, 2023, 8(7): 15166-15188. doi: 10.3934/math.2023774 |
[3] | Xiaofang Meng, Yongkun Li . Pseudo almost periodic solutions for quaternion-valued high-order Hopfield neural networks with time-varying delays and leakage delays on time scales. AIMS Mathematics, 2021, 6(9): 10070-10091. doi: 10.3934/math.2021585 |
[4] | R. Sriraman, R. Samidurai, V. C. Amritha, G. Rachakit, Prasanalakshmi Balaji . System decomposition-based stability criteria for Takagi-Sugeno fuzzy uncertain stochastic delayed neural networks in quaternion field. AIMS Mathematics, 2023, 8(5): 11589-11616. doi: 10.3934/math.2023587 |
[5] | Ivanka Stamova, Gani Stamov . Impulsive control strategy for the Mittag-Leffler synchronization of fractional-order neural networks with mixed bounded and unbounded delays. AIMS Mathematics, 2021, 6(3): 2287-2303. doi: 10.3934/math.2021138 |
[6] | Călin-Adrian Popa . Neutral-type, leakage, and mixed delays in fractional-order neural networks: asymptotic synchronization analysis. AIMS Mathematics, 2023, 8(7): 15969-15992. doi: 10.3934/math.2023815 |
[7] | Călin-Adrian Popa . Synchronization of Clifford-valued neural networks with leakage, time-varying, and infinite distributed delays on time scales. AIMS Mathematics, 2024, 9(7): 18796-18823. doi: 10.3934/math.2024915 |
[8] | Chengqiang Wang, Xiangqing Zhao, Yang Wang . Finite-time stochastic synchronization of fuzzy bi-directional associative memory neural networks with Markovian switching and mixed time delays via intermittent quantized control. AIMS Mathematics, 2023, 8(2): 4098-4125. doi: 10.3934/math.2023204 |
[9] | Yuehong Zhang, Zhiying Li, Wangdong Jiang, Wei Liu . The stability of anti-periodic solutions for fractional-order inertial BAM neural networks with time-delays. AIMS Mathematics, 2023, 8(3): 6176-6190. doi: 10.3934/math.2023312 |
[10] | Tianwei Zhang, Zhouhong Li, Jianwen Zhou . 2p-th mean dynamic behaviors for semi-discrete stochastic competitive neural networks with time delays. AIMS Mathematics, 2020, 5(6): 6419-6435. doi: 10.3934/math.2020413 |
The fresh concept of fractional order calculus and differential equations has three hundred years old of branch. For long period, the theory of fractional calculus is developed only on pure mathematics. In 1695, the establishment of non-integer order math, which is a speculation integer order differential and integrals, was most importantly talked about through Guillaume de Leibnitz and Gottfried Wilhelm Leibnitz, furthermore, its advancement were continuous for extensive stretch [1]. Owing to lack of solution methods, the development of fractional order calculus has not much attracted more mathematicians in those periods. In recent years, fractional order dynamical system has aroused interest of many researchers in the field of nonlinear science and technology. Differential condition and dynamic framework displaying have gotten significant research themes in normal science and building innovation [2,3,4,5,6]. In recent years, fractional-order differential equations are thought of as a recent topic. A geometric interpretation of fractional integral and derivative is given in [7]. Although there are very many possible generalizations of dndtnf(t), the most commonly used definitions are Riemann-Liouville and Caputo fractional derivatives. A strong motivation for studying fractional differential equations comes from the fact that have been proved to be valuable tools in the modeling of many phenomena in various fields of engineering, physics, and economics. For more interesting theoretical results of fractional differential equations, see [8,9,10,11,12]. In most of these techniques, either the solutions of integer order differential equation versions of the given fractional differential equations or the series expansions in the neighborhood of the initial conditions are used.
Recently, because of the introduction of fractional calculus, fractional-order competitive network is attracting more and more attentions. At present, various kinds of neural network model exist, which include competitive type-neural networks [13], Cohen-Gross berg-type neural networks [14], cellular-type neural networks [15], recurrent type neural networks [16], BAM-type neural networks [17,18] and so on. In the genuine neural frameworks model, there are two kinds of state factors: the short-term memory (STM) variable depicting the quick neural movement, and the long-term memory(LTM) variable portraying the moderate unaided synaptic adjustments. STM describes the rapidly changing behavior of neuronal dynamics, whereas LTM describes the slow behavior of unsupervised neuronal synapses. Therefore, there are two time scales in the competitive neural networks model, one of which corresponds to the quick difference in the state, and the other to the moderate difference in the neural connection by outside improvements. However, considerable attention paid on the study of FCNN has just started with a few literatures reported [19,20]. As far as it goes, there are few literatures on incommensurate FCNN with different time scales, which is more general and less conservative than common one.
As is well known, the applications of fractional-order neural networks heavily depend on the stability of networks. In recent years, the fractional-order dynamic behaviors plays a crucial role in stability of neural networks and the research of the fractional-order dynamical system has been a hot research topic. As we know, there are various types of stability results have been demonstrated in the literature, for example, Robust stability [21], Exponential stability [22], Finite time stability [23] and so on. It is pointed that many of the researchers mainly targeted fractional-order dynamic behavior of other types of neural network model. In recent several years, there have been a lot of excellent works on the stability analysis of fractional-order BAM neural networks [24,25].
Actually, the synaptic transmission in real neural networks can be viewed as a noisy process introduced by random fluctuations from the release of neurotransmitters and other probabilistic causes. Hence, noise is unavoidable and should be taken into consideration in modeling. Moreover, it is important to check the stability issue of neural networks with stochastic disturbance. Practically, noises are omnipresent each in nature and in artificial systems. So, the stochastic influence exists doubtless. Therefore, the study of stochastic neural networks aren't solely vital however additionally necessary. It is acknowledge that in real system, there exists the abrupt phenomena such as abrupt environments changes, and conjugation transmission could be rip-roaring method brought on by random probabilistic causes, and it should degrade the stability of the neural systems. Hence, considerable attention has been paid on the study of stochastic neural networks theory and various interesting results have been reported in [26,27,28,29,30,31,32,33,34]. A large number of stochastic financial models appeared in the literature, see, for example, [35,36,37,38,39,40] and the references therein. Also, the important effect of noise disturbances should be taken into account in studying the dynamics of a financial system by means of the neural network approach.
Stochastic differential equations becomes a extraordinary interest due to its applications in characterizing numerous issues in physics, biology, mechanics, etc. Qualitative properties such as existence, uniqueness, controllability and stability for various stochastic differential systems have been extensively studied by many researchers, see for instance [41,42,43,44,45,46,47,48] and the references therein. Around 1960, for obvious mathematical reasons, systems of ordinary stochastic differential equations of Ito type [49,50,51], stochastic partial differential equations [52,53], stochastic fractional differential equations [54,55]. The effects of random environmental fluctuations are characterized by normalized Wiener process [56]. So it is characteristic and important to explore dynamical properties of the solutions for SDEs to discover the impacts of random perturbations in the relating realistic systems. The numerical models got have been extraordinarily produced for SDEs under an irregular disturbance of the Gaussian white noise, namely, the examinations concerning SDEs driven by Brownian movement have been extremely plentiful up to now.
To the best of our knowledge, stability analysis of stochastic fractional-order competitive neural networks with leakage delay has not been fully investigated, and there is still much room left for further investigation. Motivated by the above discussions, this paper devotes to presenting a sufficient criterion for stability analysis of stochastic fractional-order competitive neural networks with leakage delay model. Meanwhile, the existence, uniqueness, and uniform stability in mean square are proved.
The main aim and contribution of our paper are highlighted as follows:
(1) We get stochastic fractional-order competitive neural networks with leakage delay model by use fractional-order instead of integer-order.
(2) Our main theme of our paper is to design both the stability analysis of stochastic fractional-order competitive neural networks with leakage delay by using Cauchy-Schwartz inequality, Burkholder-Davis-Gundy inequality, some sufficient condition for guarantee the stability.
(3) We establish a new set of sufficient criterion ensuring the uniform stability in mean square of the system and existence and uniqueness of solutions also proved by using contraction mapping principle.
(4) Various lemma's and fractional-order theory are applied to derive the main results.
This paper is organized as follows. In Section 2, we introduce the definitions and lemmas and stochastic fractional-order competitive neural networks with leakage delay model.In Section 3, we shall establish a new set of sufficient criterion ensuring the uniform stability in mean square of the system and the existence, uniqueness, and uniform stability in mean square. In Section 4, we give a numerical examples which confirm the theoretical results. Finally, the paper is concluded in Section 5.
Notations: The Caputo fractional derivative operator Dp is chosen for fractional-order derivative with order p; Rn and Rn×n denote the n-dimensional Euclidean space and the set of all n×n real matrices, respectively; C the complex number set; R, R+ and Z+ are the set of all real numbers, the set of all nonnegative numbers and the set of al nonnegative integer numbers, respectively; E(⋅) stands for the mathematical expectation with some probability measure; Ω=(L2F0([0,T],Rn),||⋅||); for any z=(z1,...,zn)T∈Rn, we define the vector.
||z(t)||=√n∑i=1supt∈(0,T]{e−2Nt|z2i(t)|}. | (1.1) |
For any ϕ=(ϕ1(t),....,ϕn(t))T∈L2F0([−τ,0],Rn), we define the vector norm
||ϕ(t)||=√n∑i=1supt∈[−τ,0]{e−2Nt|ϕi(t)|2}. | (1.2) |
In this section we present some definitions, lemma and recall the well-known results about fractional differential equations.
Definition 2.1. [57] The Caputo fractional-order derivative with order p for a differential function z(t) is defined as
Dpz(t)=1Γ(m−p)∫t0zm(s)(t−s)p+1−mds, | (2.1) |
where t≥0 and m−1<p<m∈Z+. Peculiarly, when p∈(0,1),
Dpz(t)=1Γ(1−p)∫t0z′(s)(t−s)pds. |
Definition 2.2. [57] The e Riemann-Liouville fractional integral of order p∈(0,1) for a function z(t) is defined as
Ipz(t)=1Γ(p)∫t0z(s)(t−s)1−pds, | (2.2) |
where Γ(⋅) is the gamma function,
Γ(s)=Ns∫∞0ts−1e−Ntdt. | (2.3) |
Definition 2.3. The solution of system Eq (3.1) is said to be stable if for any ϵ>0 there exists δ(t0,ϵ)>0 such that t≥t0≥0,E||ψ(t)−ϕ(t)||2<δ imply E||u(t,t0,ψ)−v(t,t0,ϕ||2<ϵ for any two solutions u(t,t0,ψ) and v(t,t0,ϕ). It is uniformly stable in mean square if the above δ is independent of t0.
Lemma 2.4. [58] Let m be a positive integer such that m−1<p<m. If g(t)∈Cm−1([b,T]), Then
D−pb,tDpb,tg(t)=g(t)−m−1∑j=0gk(b)k!(t−b)k. | (2.4) |
Lemma 2.5. [59] Let g(s)∈L2([0,T]),h(s)∈L2([0,T]), then
(∫T0|g(s)h(s)ds|)2≤(∫T0|g(s)|2ds)(∫T0|h(s)|2ds) | (2.5) |
Assumption 1. [60] We assume that the non-linear functions gj(⋅) and σij(⋅,⋅) satisfy the following conditions: There exist positive constants Lj and ηij such that
|gj(x)−gj(y)|≤Fj|x−y|,|σij(x,ˉx)−σij(y,ˉy)|≤ηij(|x−y|2+|ˉx−ˉy|2), |
for any x,y,ˉx,ˉy∈R,i,j=1,...,n. For convenience, we introduce the following notation related to model Eq (3.1).
||F||=n∑k=1F2k,||A||=n∑i=1max∀k{a2ik},||B||=max∀i{b2i},||D||=n∑i=1max∀k{d2ik},||K||=8nmax∀k{ηik},||V||=max∀i{v2i},||W||=max∀i{w2i}. |
In this paper, the stochastic fractional-order competitive neural networks with leakage delays is defined by
Dpzi(t)=−bizi(t−μ)+n∑k=1aikgk(zk(t))+n∑k=1dikgk(zk(t−η))+cim∑l=1ril(t)ξlDpril(t)=−viril(t−δ)+plwigi(zi(t)),i=1,2,...,n,l=1,2,...,m, | (3.1) |
where Dp denotes Caputo fractional derivative of order p with 0<p<1, zi(t) is the corresponding state variable of the number of n neurons at time t, bi>0 and vi>0, represent the self feedback connection weight matrices, aik, dik are represents the synaptic connection weight matrix and delayed synaptic connection weight matrix, respectively to ith and kth neurons, pl denotes the constant external stimulus, ci denotes the external strengths of the stimulus, ril denotes the synaptic efficiency, μ>0, δ>0 denote the leakage delays, gk(zk(t)) and gk(zk(t−η)) are referred the bounded neuron output activation, where the time varying delay η is bounded and differentiable.
After setting si(t)=∑ml=1ril(t)ξl=rTi(t)ξ, where ri(t)=(ri1,.......,rim)T, ξ=(ξ1,.............,ξm)T. Without loss of generality, ξ is is assumed to normalized with unit magnitude |ξ|2=1, where ξ is the input stimulus. Then the model (3.1) can be simplified the following state-space form such as
Dpzi(t)=−bizi(t−μ)+n∑k=1aikgk(zk(t))+n∑k=1dikgk(zk(t−η))+cisi(t)Dpsi(t)=−viril(t−δ)+wigi(zi(t)),i=1,2,...,n, | (3.2) |
Initial conditions of the model(3.1) is described as:
zi=ϕi(γ),si=ψi(γ),γ∈[−τ,0],i=1,2...,n. | (3.3) |
Now we applying stochastic terms in the above equation we get,
Dpzi(t)=−bizi(t−μ)+n∑k=1aikgk(zk(t))+n∑k=1dikgk(zk(t−η))+cisi(t)+n∑k=1σik(zk(t),zk(t−η))dwk(t)dt,t∈[0,T],Dpsi(t)=−visi(t−δ)+wigi(zi(t));i=1,2,...,n, | (3.4) |
σ(⋅,⋅)=(σ(⋅,⋅))n×n is the diffusion coefficient matrix and ω(⋅)=(ω1(⋅),...,ωn(⋅))T is an n-dimensional Brownian motion defined on a complete probability space (Ω,F,P) with a natural filtration {Ft}t≥0. ϕi(t) is the initial function where ϕi(t)∈L2F0([−τ,0],R), here L2F0([−τ,0],R) denotes the family of all C-valued random processes γ(s) such that γ(s) is F0-measurable and ∫0−τE||γ(s)||2ds<∞.
Theorem 3.1. If assume Assumption 1 hold, then the system Eq (3.1) has a unique solution.
Proof. According to the properties of the fractional calculus, one can obtain that system Eq (3.4) is equivalent to the following Volterra fractional integral with memory
zi(t)=ϕi(0)+IpDpzi(t)=ϕi(0)+1Γ(p)∫t0(t−s)p−1[−bizi(s−μ)+n∑k=1aikgk(zk(s))+n∑k=1dikgk(zk(s−η))+cisi(s)+n∑k=1σik(zk(s),zk(s−η))dwk(s)ds]ds, | (3.5) |
where t∈[0,T]. We consider a mapping ϕ:Rn→Rn, defined by:
ϕizi(t)=ϕi(0)+1Γ(p)∫t0(t−s)p−1[−bizi(s−μ)+n∑k=1aikgk(zk(s))+n∑k=1dikgk(zk(s−η))+cisi(s)+n∑k=1σik(zk(s),zk(s−η))dwk(s)ds]ds, | (3.6) |
For any two different functions (z1(t),...,zn(t))T, (y1(t),...,yn(t))T, we have
ϕiyi(t)−ϕizi(t)=1Γ(p)∫t0(t−s)p−1[−bi[yi(s−μ)−zi(s−μ)]+n∑k=1[aikgk(yk(s))−aikgk(zk(s))]+n∑k=1[dikgk(yk(s−η))−dikgk(zk(s−η))]+n∑k=1[σik(yk(s),yk(s−η))−σik(zk(s),zk(s−η))]dwk(s)ds]ds, |
Then, applying elementary inequality, one sees that
|ϕiyi(t)−ϕizi(t)|2≤4Γ2(p)[|∫t0(t−s)p−1biyi(s−μ)−zi(s−μ)ds|2+n∑k=1|∫t0(t−s)P−1aikgk(yk(s))−aikgk(zk(s))ds|2+n∑k=1|∫t0(t−s)P−1dikgk(yk(s−η))−dikgk(zk(s−η))ds|2+n∑k=1|∫t0(t−s)p−1[σik(yk(s),yk(s−η))−σik(zk(s),zk(s−η))]ds|2],e−2Rt|ϕiyi(t)−ϕizi(t)|2≤4Γ2(p)[b2i|∫t0(t−s)p−1e−Rtyi(s−μ)−zi(s−μ)ds|2+n∑k=1|∫t0(t−s)P−1e−Rt[aikgk(yk(s))−aikgk(zk(s))]ds|2+n∑k=1|∫t0(t−s)p−1e−Rt[dikgk(yk(s−η))−dikgk(zk(s−η))]ds|2+n∑k=1|∫t0(t−s)p−1e−Rt[σik(yk(s),yk(s−η))−σik(zk(s),zk(s−η))]dwk(s)|2], | (3.7) |
First, we have a tendency to valuate the primary term of the right hand side of the above inequality by using Cauchy inequality to obtain
b2i|∫t0(t−s)p−1e−Rtyi(s−μ)−zi(s−μ)ds|2≤b2i(∫t0(t−s)p−1e−R(t−s)ds)(∫t0(t−s)P−1e−R(t−s)e−2Rs|yi(s−μ)−zi(s−μ)ds|2)≤Γ(p)Rpb2i(∫t0(t−s)p−1e−R(t−s)e−2Rs|yi(s−μ)−zi(s−μ)|2ds)≤Γ(p)Rpb2i(∫tμ(t−s)p−1e−R(t−s)e−2Rs|yi(s−μ)−zi(s−μ)|2ds)+Γ(p)Rpb2i(∫μ0(t−s)p−1e−R(t−s)e−2Rs|ϕi(s−μ)−ϕi(s−μ)|2ds)≤Γ(P)Rpb2i(∫tμ(t−s)p−1e−R(t−s)e−2Rs|yi(s−μ)−zi(s−μ)|2ds)≤Γ(p)Rpb2i(∫t−μ0(t−γ−μ)p−1e−R(t−γ−μ)e−2Rγe−2Rμ|yi(γ)−zi(γ)|2dγ)≤Γ(p)Rpb2isupt∈(0,T]{e−2Rt|yi(t)−zi(t)|2}e−2Rμ∫t−μ0ξp−1e−Rξdξ≤[Γ(p)Rp]2b2isupt∈(0,T]{e−2Rt|yi(t)−zi(t)|2} | (3.8) |
Next, we evaluate the second term by using Assumption 1, we have
n∑k=1|∫t0(t−s)p−1e−Rt[aikgk(yk(s))−aikgk(zk(s))]ds|2≤(∫t0(t−s)p−1e−R(t−s)e−Rsn∑k=1|aik||gk(yk(s))−gkzk(s)|ds)2≤(∫t0(t−s)p−1e−R(t−s)e−Rsn∑k=1|aik|Fk|yk(s)−zk(s)|ds)2≤(∫t0(t−s)p−1e−R(t−s)ds)(∫t0(t−s)p−1e−R(t−s)dse−2Rs(n∑k=1|aik|Fk|yk(s)−zk(s)|)2ds)≤(∫t0(t−s)P−1e−R(t−s)ds)(∫t0(t−s)p−1e−R(t−s)e−2Rs(n∑k=1a2ikF2k)(n∑k=1|yk(s)−zk(s)|)2ds)≤(n∑k=1a2ikF2k)(∫t0(t−s)p−1e−R(t−s)ds)(∫t0(t−s)p−1e−R(t−s)n∑k=1e−2Rs|yk(s)−zk(s)|)2ds)≤(n∑k=1a2ikF2k)n∑k=1supt∈(0,T]{e−2Rt|yk(t)−zk(t)|2ds}(∫t0(t−s)p−1e−R(t−s)ds)2≤[Γ(p)Rp]2(n∑k=1a2ikF2k)n∑k=1supt∈(0,T]{e−2Rt|yk(t)−zk(t)|2ds} | (3.9) |
|∫t0(t−s)p−1e−Rt[n∑k=1dikgk(yk(s−η))−n∑k=1dikgk(zk(s−η))]ds|2≤(∫t0(t−s)p−1e−R(t−s)e−Rsn∑k=1Fk|dik||yk(s−η)−zk(s−η)|ds)2≤(∫t0(t−s)p−1e−R(t−s)ds)(∫t0(t−s)p−1e−R(t−s)e−2Rs(n∑k=1Fk|dik||yk(s−η)−zk(s−η)|2)ds)≤[Γ(p)Rp](n∑k=1d2ikF2k)(∫t0(t−s)p−1e−R(t−s)e−2Rs(n∑k=1|yk(s−η)−zk(s−η)|2)ds)≤[Γ(p)Rp](n∑k=1d2ikF2k)[∫η0(t−s)p−1e−R(t−s)e−2Rsn∑k=1|ϕk(s−η)−ϕk(s−η)|2ds+∫tη(t−s)p−1e−R(t−s)e−2Rsn∑k=1|yk(s−η)−zk(s−η)|2ds]≤[Γ(p)Rp](n∑k=1d2ikF2k)∫t−η0(t−γ−η)p−1e−R(t−γ−η)e−2Rγe−2Rηn∑k=1|yk(γ)−zk(t)|2dγ≤[Γ(p)Rp](n∑k=1d2ikF2k)n∑k=1supt∈(0,T]{e−2Rt|yk(t)−zk(t)|2}∫t−η0ξp−1e−Rξdξ≤[Γ(p)Rp]2(n∑k=1d2ikF2k)n∑k=1supt∈(0,T]{e−2Rt|yk(t)−zk(t)|2} | (3.10) |
However, by using the Burkholder-Davis-Gundy inequality and Assumption 1, we get that
E[supt∈(0,T]|∫t0(t−s)p−1e−Rtn∑k=1[σik(yk(s),yk(s−η))−σik(zk(s),zk(s−η))]dwk(s)|2]≤4E∫T0(T−s)(2p−1)−1e−2R(T−s)e−2Rsnn∑k=1|σik(yk(s),yk(s−η))−σik(zk(s),zk(s−η))|2ds≤4E∫T0(T−s)(2p−1)−1e−2R(T−s)−1e−2Rsnn∑k=1ηik[||yk(s)−zk(s)|2+|yk(s−η)−zk(s−η)|2]ds≤4nmaxk{ηik}{E∫T0(T−s)(2p−1)−1e−2R(T−s)e−2Rsn∑k=1|yk(s)−zk(s)|2ds+E∫A0(T−s)2(p−1)−1e−2R(T−s)e−2Rsn∑k=1|yk(s−η)−zk(s−η)|2ds}≤4nmaxk{ηik}{E∫T0(T−s)2(p−1)e−2R(T−s)e−2Rsn∑k=1|yk(s)−zk(s)|2ds+E∫η0(T−s)2(p−1)−1e−2R(T−s)e−2Rsn∑k=1|ϕk(s−η)−ϕk(s−η)|2ds+E∫Tη(T−s)2(p−1)−1e−2R(T−s)e−2Rsn∑k=1|yk(s−η)−zk(s−η)|2ds}≤4nn∑k=1maxk{ηik}En∑k=1supt∈(0,T]{e−2Rt|yk(t)−zk(t)|2}∫T0(T−s)2(p−1)−1e−2R(T−s)ds+En∑k=1supt∈(0,T]{e−2Rt|yk(t)−zk(t)|2}e−2Rη∫T−η0(T−γ−η)(2p−1)−1e−2R(T−γ−η)dγ≤8nn∑k=1maxk{ηik}E||y(t)−z(t)||2∫t0ξ(2p−1)e−2Rξdξ≤8nn∑k=1maxk{ηik}[Γ(2p−1)Rp]E||y(t)−z(t)||2 | (3.11) |
Thus, by combining the above inequalities together, one obtains
E||ϕy(t)−ϕz(t)||2≤ [Γ(p)Rp]2b2isupt∈(0,T]{e−2Rt|yi(t)−zi(t)|2}+[Γ(p)Rp]2(n∑k=1a2ikF2k)n∑k=1supt∈(0,T]{e−2Rt|yk(t)−zk(t)|2}+[Γ(p)Rp]2(n∑k=1d2ikF2k)n∑k=1supt∈(0,T]{e−2Rt|yk(t)−zk(t)|2}+8nmaxk{ηik}[Γ(2p−1)Rp]E||y(t)−z(t)||2≤[4Γ2(p)R2p][{||B||+||A||||F||+||D||||F||}E||y(t)−z(t)||2+4||K||Γ(2α−1)NαE||y(t)−z(t)||2]≤[4[||B||+||A||||F||+||D||||F||]R2p+4||K||Γ(2p−1)Γ2(p)Rp]E||y(t)−z(t)||2 | (3.12) |
Similarly, by the same procedures as the above inequality, we obtains
ui(t)−si(t)=1Γ(p)∫t0(t−s)p−1[−vi[ui(t−δ)−si(t−δ)]+wi[gi(yi(t))−gi(zi(t))]]ds|ϕi(ui(t))−ϕisi(t)|2≤2Γ2(p)[|∫t0(t−s)p−1vi[ui(s−δ)−si(s−δ)]ds|2+|∫t0(t−s)p−1wi[gi(yi(t))−gi(xi(t))]ds|2]e−2Rt|ϕiui(t)−ϕisi(t)|2≤2Γ2(p)[v2i|∫t0(t−s)p−1e−Rt[ui(s−δ)−si(s−δ)]ds|2+w2i|∫t0(t−s)p−1e−Rt[gi(yi(t))−gi(zi(t))]ds|2] | (3.13) |
By using Cauchy inequality
v2i|∫t0(t−s)p−1e−Rt[ui(s−δ)−si(s−δ)]ds|2≤v2i(∫t0(t−s)p−1e−R(t−s)ds)(∫t0(t−s)p−1e−R(t−s)e−2Rs|ui(s−δ)−si(s−δ)|2ds)≤Γ(p)Rpv2i[∫tδ(t−s)p−1e−R(t−s)e−2Rs|ui(s−δ)−si(s−δ)|2ds+∫δ0(t−s)p−1e−R(t−s)e−2Rs|ψi(s−δ)−ψi(s−δ)|2ds]≤Γ(p)Rpv2i[∫tδ(t−s)p−1e−R(t−s)e−2Rs|ui(s−δ)−si(s−δ)|2ds]≤Γ(p)RPv2i[∫t−δ0(t−γ−δ)p−1e−R(t−γ−δ)e−2Rγe−2Rδ|ui(γ)−si(γ)|2dγ]≤Γ(p)Rpv2isupt∈(0,T]{e−2Rt|ui(t)−si(t)|2}e−2Rτ∫t−δ0ξp−1e−Rξdξ}≤[Γ(p)RP]2v2isupt∈(0,T]{e−2Rt|ui(t)−si(t)|2} | (3.14) |
w2i|∫t0(t−s)p−1e−Rt[gi(yi(s))−gi(zi(s))]ds|2≤w2i(∫t0(t−s)p−1e−R(t−s)e−Rs|Fi|||yi(s)−zi(s)|ds)2≤w2i(∫t0(t−s)p−1e−R(t−s)ds)2(∫t0(t−s)p−1e−R(t−s)e−2Rs|Fi|yi(s)−zi(s)|2ds)≤w2i|Fi|supt∈(0,T]{e−2Rt|yi(t)−zi(t)|2}(∫t0(t−s)p−1e−R(t−s)ds)2≤[Γ(p)Rp]2w2i|Fi|supt∈(0,T]{e−2Rt|yi(t)−zi(t)|2} | (3.15) |
From (3.14) and (3.15) in (3.13)
E||ϕiui(t)−ϕisi(t)||2≤2Γ2(p)[[Γ(p)Rp]2v2isupt∈(0,T]{e−2Rt|ui(t)−si(t)|2}+[Γ(p)Rp]2w2i|Fi|supt∈(0,T]{e−2Rt|yi(t)−zi(t)|2}]E||ϕiui(t)−ϕisi(t)||2≤2(||V||)R2pE||u(t)−s(t)||2+2||W||||F||R2pE||y(t)−z(t)||2 | (3.16) |
From (3.12) and (3.16)
E||ϕiyi(t)−ϕizi(t)||2≤[4[||B||+||A||||F||+||D||||F||]R2p+4||K||Γ(2p−1)Γ2(p)Rp]E||y(t)−z(t)||2E||ϕiui(t)−ϕisi(t)||2≤2(||V||)R2pE||u(t)−s(t)||2+2||W||||F||R2pE||y(t)−z(t)||2 |
By combining the both the equations we get
E||ϕiyi(t)−ϕizi(t)||2+E||ϕiui(t)−ϕisi(t)||2≤[4[||B||+||A||||F||+||D||||F||]R2p+4||K||Γ(2p−1)Γ2(p)Rp]E||y(t)−z(t)||2+2(||V||)R2pE||u(t)−s(t)||2+2||W||||F||R2pE||y(t)−z(t)||2E||ϕiyi(t)−ϕizi(t)||2+E||ϕiui(t)−ϕisi(t)||2≤[4[||B||+||A||||F||+||D||||F||]R2p+4||K||Γ(2p−1)Γ2(p)Rp+2||W||||F||R2p]E||y(t)−z(t)||2+2(||V||)R2pE||u(t)−s(t)||2 |
where
K1=[4[||B||+||A||||F||+||D||||F||]R2p+4||K||Γ(2p−1)Γ2(P)Rp+2||W||||F||R2p], | (3.17) |
K2=2(||V||)R2p. | (3.18) |
E||ϕy(t)−ϕz(t)||2≤K1E||y(t)−z(t)||2, | (3.19) |
E||ϕ(u(t))−ϕ(s(t))||2≤K2E||u(t)−s(t)||2. | (3.20) |
E||ϕ(W)−ϕ(V)||2≤Max{E||y(t)−z(t)||2,E||u(t)−s(t)||2},E||ϕ(W)−ϕ(V)||2≤ME||W−V||2. |
Therefore the mapping ϕ is a contraction mapping. As a consequence of the Banach fixed point theorem, the problem Eq (3.5) has a unique fixed point, so that we conclude that system Eq (3.1) has a unique solution, which complete the proof of the theorem.
Theorem 3.2. If Assumption 1 hold, the solution of system given by Eq (3.1) satisfying initial condition is uniformly stable in mean square.
Proof. Assume that For any two different functions (z1(t),...,zn(t),s1(t),......,sn(t))T, (y1(t),...,yn(t),u1(t),.......,un(t))T, solutions of Eq (3.1) with the different initial conditions zi=ϕi(γ)∈L2F0([−τ,0],R),si=ψi(γ)∈L2F0([−τ,0],R),i∈n, one has
Dp[yi(t)−zi(t)]=−bi[yi(t−μ)−zi(t−μ)]+n∑k=1aik[gk(zk(t))−gk(zk(t))]+n∑k=1dik[gk(zk(t−η))−gk(zk(t−η))]+n∑k=1[σik(zk(t),zk(t−η))−σik(zk(t),zk(t−η))]dwk(t)dtDp[ui(t)−si(t)]=−vi[ui(t−δ)−si(t−δ)]+wi[gi(yi(t))−gi(zi(t))];i=1,2,...,n, | (3.21) |
Based on Lemma 2.4, the solution of the system Eq (3.21) can be expressed in the following form
yi(t)−zi(t)=ψi(0)−ϕi(0)+Iα[−bi[yi(t−μ)−zi(t−μ)]+n∑k=1aik[gk(zk(t))−gk(zk(t))]+n∑k=1dik[gk(zk(t−η))−gk(zk(t−η))]+n∑k=1[σik(zk(t),zk(t−η))−σik(zk(t),zk(t−η))]dwk(t)dt]=ψi(0)−ϕi(0)+1Γ(α)∫t0(t−s)p−1[−bi[yi(t−μ)−zi(t−μ)]+n∑k=1aik[gk(zk(t))−gk(zk(t))]+n∑k=1dik[gk(zk(t−η))−gk(zk(t−η))]+n∑k=1[σik(zk(t),zk(t−η))−σik(zk(t),zk(t−η))]dwk(t)ds]ds=ψi(0)−ϕi(0)+1Γ(α)∫t0(t−s)P−1−bi[yi(t−μ)−zi(t−μ)]ds+1Γ(α)∫t0(t−s)p−1n∑k=1aik[gk(zk(t))−gk(zk(t))]ds+1Γ(α)∫t0(t−s)p−1n∑i=1dik[gk(zk(t−η))−gk(zk(t−η))]ds+1Γ(α)∫t0(t−s)p−1n∑k=1[σik(zk(t),zk(t−η))−σik(zk(t),zk(t−η))]dwk(s) | (3.22) |
Then we have
e−2Rt|yi(t)−zi(t)|2≤5e−2Rt|ψi(0)−ϕi(0)|2+5Γ2(α)[b2i|∫t0(t−s)p−1e−Rt(yi(s−μ)−zi(s−μ)ds|2+|∫t0(t−s)p−1e−Rtn∑k=1aik[gk(yk(s))−gk(zk(s))]ds|2+|∫t0(t−s)p−1e−Rtn∑k=1dik[gk(zk(s−η))−gk(zk(s−η))]ds|2+|∫t0(t−s)p−1e−Rtn∑k=1[σik(yk,(yk(s−η))−σik(zk,(zk(s−η))]dwk(s)|2] | (3.23) |
Firstly, we observe that
b2i|∫t0(t−s)p−1e−Rt[yi(s−μ)−zi(s−μ)]ds|2≤Γ(p)Rpb2i(∫0−μ(t−γ−μ)p−1e−R(t−γ−μ)e−2Rγe−2Rμn∑k=1|ψk(γ)−ϕk(γ)|2dγ+∫t−μ0(t−γ−μ)p−1e−R(t−γ−μ)e−2Rγe−2Rμn∑k=1|yk(γ)−zk(γ)|2dγ)≤[Γ(p)Rp]2b2isupt∈[−μ,0]{e−2Rt|ψk(t)−ϕk(t)|2}+[Γ(p)Rp]2b2isupt∈(0,T]{e−2Rt|yk(t)−zk(t)|2} | (3.24) |
Secondly, we can get from Assumption 1
n∑k=1|∫t0(t−s)p−1e−Rt[aikgkyk(s)−aikgk(zk(s))]ds|2≤(∫t0(t−s)p−1e−R(t−s)e−Rsn∑k=1|aik||gk(yk(s))−gk(zk(s))|ds)2≤(∫t0(t−s)p−1e−R(t−s)e−Rsn∑k=1|aik|Fk|yk(s)−zk(s)|ds)2≤(∫t0(t−s)p−1e−R(t−s)ds)(∫t0(t−s)p−1e−R(t−s)e−2Rsn∑k=1|aik|Fk|yk(s)−zk(s)|2ds)≤(∫t0(t−s)p−1e−R(t−s)ds)(∫t0(t−s)p−1e−R(t−s)e−2Rs(n∑k=1a2ikF2k)(n∑k=1|yk(s)−zk(s)|)2ds)≤(n∑k=1a2ikF2k)(∫t0(t−s)p−1e−R(t−s)ds)(∫t0(t−s)p−1e−R(t−s)e−2Rs|yk(s)−zk(s)|)2ds)≤(n∑k=1a2ikF2k)n∑k=1supt∈(0,T]{e−2Rt|yk(t)−zk(t)|2}(∫t0(t−s)p−1e−R(t−s)ds)2≤[Γ(p)Rp]2(n∑k=1a2ikF2k)n∑k=1supt∈(0,T]{e−2Rt|yk(t)−zk(t)|2} | (3.25) |
|∫t0(t−s)p−1e−Rt[n∑k=1dikgk(yk(s−η))−n∑k=1dikgk(zk(s−η))]ds|2≤(∫t0(t−s)p−1e−R(t−s)e−Rsn∑k=1Fk|dik||yk(s−η)−zk(s−η)|ds)2≤(∫t0(t−s)p−1e−R(t−s)ds)(∫t0(t−s)p−1e−2R(t−s)e−2Rsn∑k=1Fk|dik||yk(s−η)−zk(s−η)|2ds)≤[Γ(P)Rp](n∑k=1d2ikF2k)[∫0−η(t−γ−η)p−1e−R(t−γ−η)e−2Rγe−2Rηn∑k=1|ψk(γ)−ϕk(γ)|2dγ+∫t−η0(t−γ−η)p−1e−R(t−γ−η)e−2Rγe−2Rηn∑k=1|yk(γ)−zk(γ)|2dγ≤[Γ(p)Rp]2(n∑k=1F2kd2ik)n∑k=1supt∈[−η,0]{e−2Rt|ψk(γ)−ϕk(γ)|2}+[Γ(p)Rp]2(n∑k=1F2kd2ik)n∑k=1supt∈(0,T]{e−2Rt|yk(γ)−zk(γ)|2} | (3.26) |
However, by using the Burkholder-Davis-Gundy's inequality and Assumption 1, we get that
E[supt∈(0,T]5Γ2(p)|∫t0(t−s)(p−1)e−Rtn∑k=1[σik(yk(s),yk(s−η))−σik(zk(s),zk(s−η))]dwk(s)|2]≤5Γ2(p)En∑k=1supt∈(0,T]|∫t0(t−s)(p−1)e−Rtn∑k=1[σik(yk(s),yk(s−η))−σik(zk(s),zk(s−η))]dwk(s)|2 |
En∑k=1supt∈(0,T]|∫t0(t−s)(p−1)e−Rtn∑k=1[σik(yk(s),yk(s−η))−σik(zk(s),zk(s−η))]dwk(s)|2≤4E∫A0(T−s)(2p−1)e−2R(T−s)e−2Rsnn∑k=1ηik[||yk(s)−zk(s)|2+|yk(s−η)−zk(s−η)|2]dwk(s)≤4nmaxk{ηik}{E∫T0(T−s)(2p−1)−1e−2R(T−s)e−2Rsn∑k=1|yk(s)−zk(s)|2ds+E∫0−η(T−γ−η)(2p−1)−1e−2R(T−γ−η)n∑k=1|ψk(γ)−ϕk(γ)|2dγ}+E∫t−η0(A−γ−η)(2p−1)−1e−2R(T−γ−η)e−2RTe−2Rηn∑k=1|yk(γ)−zk(γ)|2dγ}≤4nmaxk{ηik}{En∑k=1supt∈(0,T]{e−2Nt|yk(s)−zk(s)|2}∫T0(T−s)(2p−1)−1e−2R(T−s)ds+En∑k=1supt∈(0,T]{e−2Nt|ψk(s)−ϕk(s)|2}e−2Nη∫0−η(T−γ−η)(2p−1)e−2R(T−γ−η)dγ+En∑k=1supt∈(0,T]{e−2Nt|yk(s)−zk(s)|2}e−2Nη∫t−η0(T−γ−η)(2p−1)−1e−2R(T−γ−η)dγ}≤Γ(2p−1)Rp4nmaxk{ηik}{2E||y(t)−z(t)||2+E||ψ(t)−ϕ(t)||2} | (3.27) |
Consequently, by combining the above inequalities together, we have
E||yi(t)−zi(t)||2≤5e−2Rt|ψi(0)−ϕi(0)|2+5Γ2(p)[Γ(p)Rp]2b2in∑k=1supt∈[μ,0]{e−2Rt|ψk(γ)−ϕk(γ)|2}+5Γ2(p)[Γ(p)Rp]2b2in∑k=1supt∈(0,T]{e−2Rt|yk(γ)−zk(γ)|2}+5Γ2(p)[Γ(p)Rp]2(n∑k=1a2ikF2k)n∑k=1supt∈(0,T]{e−2Rt|yk(t)−zk(t)|2}+5Γ2(p)[Γ(p)Rp]2(n∑k=1F2kd2ik)n∑k=1supt∈(−η,0){e−2Rt|ψk(γ)−ϕk(γ)|2}+5Γ2(p)[Γ(p)Rp]2(n∑k=1F2kd2ik)n∑k=1supt∈(−η,0){e−2Rt|yk(γ)−zk(γ)|2}+5Γ2(p)Γ(2p−1)Rp4nmaxk{ηik}{2E||y(t)−x(t)||2+E||ψ(t)−ϕ(t)||2}≤5||ψ(t)−ϕ(t)||+5R2P||B||||ψ(t)−ϕ(t)||2+5R2p||B||||y(t)−z(t)||2+5R2p||A||||F||||y(t)−z(t)||2+5R2p||D||||F||||ψ(t)−ϕ(t)||2+5R2p||D||||F||||y(t)−z(t)||2+5Γ(2p−1)RpΓ2(p)||K||||y(t)−z(t)||2+5Γ(2p−1)RpΓ2(p)||K||||ψ(t)−ϕ(t)||2E||y(t)−z(t)||2≤5[1+||B||+||F||||D||R2p+Γ(2p−1)||K||RpΓ2(p)]E||ψ(t)−ϕ(t)||2+5[||B||+||F||||A||+||F||||D||R2p+Γ(2p−1)||K||RpΓ2(p)]E||y(t)−z(t)||2E||y(t)−z(t)||2≤[5[1+||B||+||F||||D||R2p+Γ(2p−1)||K||Γ2(P)Rp]1−5[||B||+||A||||D||+||F||||D||R2p+Γ(2p−1)||K||Γ2(p)Rp]]E||ψ(t)−ϕ(t)||2 | (3.28) |
where,
L1=[5[1+||B||+||F||||D||R2p+Γ(2p−1)||K||Γ2(p)Rp]1−5[||B||+||A||||D||+||F||||D||Rp+Γ(2p−1)||K||Γ2(p)Rp]]
Similarly, by the same procedures as the above inequality, we obtains
ui(t)−si(t)=ψi(0)−ϕi(0)+1Γ(p)∫t0(t−s)p−1[−vi[ui(s−δ)−si(s−δ)]+wi[gi(yi(s))−gi(zi(s))]]dse−2Rt|ui(t)−si(t)|2≤5e−2Rt|ψi(0)−ϕi(0)|2+5Γ2(p)[v2i|∫t0(t−s)p−1e−Rt[ui(s−δ)−si(s−δ)|2]+w2i|∫t0(t−s)p−1e−Rt[gi(yi(s))−gi(zi(s))|2]]ds | (3.29) |
w2i|∫t0(t−s)p−1e−Rt[gi(yi(s))−gi(zi(s))|2]]ds≤[Γ(p)Rp]2w2i||F||supt∈(0,T]{e−2Rt|yk(t)−zk(t)|2} | (3.30) |
v2i|∫t0(t−s)p−1e−Rt[ui(s−δ)−si(s−δ)|2]]ds≤Γ(p)Rpv2i∫t0(t−s)p−1e−R(t−s)e−2Rsn∑k=1|uk(s−δ)−sk(s−δ)|2ds≤Γ(p)Rpv2i∫0−δ(t−γ−δ)p−1e−R(t−γ−δ)e−2Rγe−2Rδn∑k=1|ψk(γ)−ϕk(γ)|2dγ+∫t−δ0(t−γ−δ)p−1e−R(t−γ−δ)e−2Rγe−2Rδn∑k=1|uk(γ)−sk(γ)|2dγ≤[Γ(p)Rp]2v2isup[−δ,0]{e−2Rt|ψk(t)−ϕk(t)|2}+[Γ(p)Rp]2v2isup(0,T]{e−2Rt|uk(t)−sk(t)|2} | (3.31) |
Substituting the above (3.30) and (3.31) in the above equation (3.29) we get
e−2Rt|ui(t)−si(t)|2≤5e−2Rt|ψi(0)−ϕi(0)|2+5Γ2(p){[Γ(P)Rp]2w2i||F||supt∈(0,T]{e−2Rt|yk(t)−zk(t)|2}+[Γ(p)Rp]2v2isup[−δ,0]{e−2Rt|ψk(t)−ϕk(t)|2}+[Γ(p)Rp]2v2isup(0,T]{e−2Rt|uk(t)−vk(t)|2}}≤5||ψ(t)−ϕ(t)||2+5R2p||W||||F||E||y(t)−z(t)||2+5R2p||V|||E||ψ(t)−ϕ(t)||2+5R2p||V||E||u(t)−s(t)||2E||u(t)−s(t)||2≤5[1+||V||R2p]E||ψ(t)−ϕ(t)||2+5R2p[||W||||F||]E||y(t)−z(t)||2+5R2p||V||E||u(t)−s(t)||2 | (3.32) |
From Eqs (3.28) and (3.32)
E||y(t)−z(t)||2≤[5[1+||B||+||F||||D||R2p+Γ(2p−1)||K||Γ2(P)Rp]1−5[||B||+||A||||D||+||F||||D||R2p+Γ(2P−1)||K||Γ2(P)Rp]]E||ψ(t)−ϕ(t)||2E||u(t)−s(t)||2≤5[1+||V||R2p]E||ψ(t)−ϕ(t)||2+5R2p[||W||||F||]E||y(t)−z(t)||2+5||V||R2pE||u(t)−s(t)||2[1−5||V||R2p]E||u(t)−s(t)||2≤5[1+||V||R2p]E||ψ(t)−ϕ(t)||2+5R2p[||W||||F||]E||y(t)−z(t)||2 |
E||u(t)−s(t)||2≤5[1+||V||R2p]E||ψ(t)−ϕ(t)||2+5R2p[||W||||F||][[5[1+||B||+||F||||D||R2p+Γ(2p−1)||K||Γ2(p)Rp]1−5[||B||+||A||||D||+||F||||D||R2p+Γ(2p−1)||K||Γ2(p)Rp]]E||ψ(t)−ϕ(t)||2][1−5||V||R2p]≤{5[1+||V||R2p]+5R2p[||W||||F||][[5[1+||B||+||F||||D||R2p+Γ(2p−1)||K||Γ2(p)Rp]1−5[||B||+||A||||D||+||F||||D||R2p+Γ(2p−1)2||K||Γ2(p)Rp]]][1−5||V||R2p]}E||ψ(t)−ϕ(t)||2 | (3.33) |
L1=[5[1+||B||+||F||||D||R2p+Γ(2p−1)||K||Γ2(P)Rp]1−5[||B||+||A||||D||+||F||||D||R2p+Γ(2p−1)||K||Γ2(p)Rp]]L2={5[1+||V||R2p]+5R2p[||w||||F||][[5[1+||B||+||F||||D||R2p+Γ(2p−1)||K||Γ2(p)Rp]1−5[||B||+||A||||D||+||F||||D||R2p+Γ(2P−1)||K||Γ2(P)Rp]]][1−5||V||R2p]}. |
by the above two equations we get
E||y(t)−z(t)||2≤δ1E||ψ(t)−ϕ(t)||2E||u(t)−s(t)||2≤δ2E||ψ(t)−ϕ(t)||2E||y(t)−z(t)||2≤ε1 | (3.34) |
E||ψ(t)−ϕ(t)||2≤δ1 | (3.35) |
E||u(t)−s(t)||2≤ε2 | (3.36) |
E||ψ(t)−ϕ(t)||2≤δ2. | (3.37) |
which means that the solution of system Eq (3.1) is uniformly stable in mean square.
Remark 1. In the proof of Theorem 3.2, we investigated stochastic fractional-order competitive neural networks with leakage delay without constructing Lyapunov function and by using Cauchy inequality, analysis method and Burkholder DavisGundy inequality.
Remark 2. If there are no stochastic disturbance, then system (3.4) becomes the following fractional-order competitive neural networks with leakage delay:
DPzi(t)=−bizi(t−μ)+n∑k=1aikgk(zk(t))+n∑k=1dikgk(zk(t−η))+cisi(t)DPsi(t)=−visi(t−δ)+wigi(zi(t));i=1,2,...,n, | (3.38) |
Theorem 3.3. If assume Assumption 1 hold, then the system Eq (3.38) has a unique solution.
Proof. According to the properties of the fractional calculus, one can obtain that system Eq (3.38) is equivalent to the following Volterra fractional integral with memory
zi(t)=ϕi(0)+IpDpzi(t)=ϕi(0)+1Γ(p)∫t0(t−s)p−1[−bizi(s−μ)+n∑k=1aikgk(zk(s))+n∑k=1dikgk(zk(s−η))+cisi(s)]ds | (3.39) |
where t∈[0,T]. We consider a mapping ϕ:Rn→Rn, defined by:
ϕizi(t)=ϕi(0)+1Γ(p)∫t0(t−s)P−1[−bizi(s−μ)+n∑k=1aikgk(zk(s))+n∑k=1dikgk(zk(s−η))+cisi(s)]ds | (3.40) |
where ϕ(u)=(ϕ1(u),ϕ2(u),....,ϕn(u))T. For any two different functions z(t)=(z1(t),...,zn(t))T, y(t)=(y1(t),...,yn(t))T, we have
ϕiyi(t)−ϕizi(t)=1Γ(p)∫t0(t−s)p−1[−bi[yi(s−μ)−zi(s−μ)]+n∑k=1[aikgk(yk(s))−aikgk(zk(s))]+n∑k=1[dikgk(yk(s−η))−dikgk(zk(s−η))]ds, |
Then, applying elementary inequality, one sees that
|ϕiyi(t)−ϕizi(t)|2≤3Γ2(p)[|∫t0(t−s)p−1biyi(s−μ)−zi(s−μ)ds|2+n∑k=1|∫t0(t−s)p−1aikgk(yk(s))−aikgk(zk(s))ds|2+n∑k=1|∫t0(t−s)p−1dikgk(yk(s−η))−dikgk(zk(s−η))ds|2,e−2Rt|ϕiyi(t)−ϕizi(t)|2≤3Γ2(p)[b2i|∫t0(t−s)p−1e−Rtyi(s−μ)−zi(s−μ)ds|2+n∑k=1|∫t0(t−s)p−1e−Rt[aikgk(yk(s))−aikgk(zk(s))]ds|2+n∑k=1|∫t0(t−s)p−1e−Rt[dikgk(yk(s−η))−dikgk(zk(s−η))]ds|2], | (3.41) |
First, we evaluate the first term of the right hand side of the above inequality by using Cauchy's inequality to obtain
b2i|∫t0(t−s)p−1e−Rtyi(s−μ)−zi(s−μ)ds|2≤b2i(∫t0(t−s)p−1e−R(t−s)ds)(∫t0(t−s)p−1e−R(t−s)e−2Rs|yi(s−μ)−zi(s−μ)|2ds)≤Γ(p)Rpb2i(∫t0(t−s)p−1e−R(t−s)e−2Rs|yi(s−μ)−zi(s−μ)|2ds)≤Γ(p)Rpb2i(∫tμ(t−s)p−1e−R(t−s)e−2Rs|yi(s−μ)−zi(s−μ)|2ds)+Γ(p)Rpb2i(∫μ0(t−s)p−1e−R(t−s)e−2Rs|ϕi(s−μ)−ϕi(s−μ)|2ds)≤Γ(p)Rpb2i(∫tμ(t−s)p−1e−R(t−s)e−2Rs|yi(s−μ)−zi(s−μ)|2ds)≤Γ(p)Rpb2i(∫t−μ0(t−γ−μ)p−1e−R(t−γ−μ)e−2Rγe−2Rμ|yi(γ)−zi(γ)|2dγ)≤Γ(p)Rpb2isupt∈(0,T]{e−2Rt|yi(t)−zi(t)|2}e−2Rμ∫t−μ0ξP−1e−Rξdξ≤[Γ(p)Rp]2b2isupt∈(0,T]{e−2Rt|yi(t)−zi(t)|2} | (3.42) |
Next, we evaluate the second term of Eq (3.41) by using Assumption 1, we have
n∑k=1|∫t0(t−s)p−1e−Rt[aikgk(yk(s))−aikgk(zk(s))]ds|2≤(∫t0(t−s)p−1e−R(t−s)e−Rsn∑k=1|aik||gk(yk(s))−gk(zk(s))|ds)2≤(∫t0(t−s)p−1e−R(t−s)e−Rsn∑k=1|aik|Fk|yk(s)−zk(s)|ds)2≤(∫t0(t−s)p−1e−R(t−s)ds)(∫t0(t−s)p−1e−R(t−s)dse−2Rs(n∑k=1|aik|Fk|yk(s)−zk(s)|ds)2)≤(∫t0(t−s)p−1e−R(t−s)ds)(∫t0(t−s)p−1e−R(t−s)e−2Rs(n∑k=1a2ikF2k)(n∑k=1|yk(s)−zk(s)|)2ds)≤(n∑k=1a2ikF2k)(∫t0(t−s)p−1e−R(t−s)ds)(∫t0(t−s)p−1e−R(t−s)n∑k=1e−2Rs|yk(s)−zk(s)|)2ds)≤(n∑k=1a2ikF2k)n∑k=1supt∈(0,T]{e−2Rt|yk(t)−zk(t)|2}(∫t0(t−s)p−1e−R(t−s)ds)2≤[Γ(p)Rp]2(n∑k=1a2ikF2k)n∑k=1supt∈(0,T]{e−2Rt|yk(t)−zk(t)|2} | (3.43) |
|∫t0(t−s)p−1e−Rt[n∑k=1dikgk(yk(s−η))−n∑k=1dikgk(zk(s−η))]ds|2≤(∫t0(t−s)p−1e−R(t−s)e−Rsn∑k=1Fk|dik||yk(s−η)−zk(s−η)|ds)2≤(∫t0(t−s)p−1e−R(t−s)ds)(∫t0(t−s)p−1e−R(t−s)e−2Rs(n∑k=1Fk|dik||yk(s−η)−zk(s−η)|2)ds)≤[Γ(p)Rp](n∑k=1d2ikF2k)(∫t0(t−s)p−1e−R(t−s)e−2Rs(n∑k=1|yk(s−η)−zk(s−η)|2)ds)≤[Γ(p)Rp](n∑k=1d2ikF2k)[∫η0(t−s)p−1e−R(t−s)e−2Rsn∑k=1|ϕk(s−η)−ϕk(s−η)|2ds+∫tη(t−s)p−1e−R(t−s)e−2Rsn∑k=1|yk(s−η)−zk(s−η)|2ds]≤[Γ(p)Rp](n∑k=1d2ikF2k)∫t−η0(t−γ−η)p−1e−R(t−γ−η)e−2Rγe−2Rηn∑k=1|yk(γ)−zk(t)|2dγ≤[Γ(p)Rp](n∑k=1d2ikF2k)n∑k=1supt∈(0,T]{e−2Rt|yk(t)−zk(t)|2}∫t−η0ξp−1e−Rξdξ≤[Γ(p)Rp]2(n∑k=1d2ikF2k)n∑k=1supt∈(0,T]{e−2Rt|yk(t)−zk(t)|2} | (3.44) |
Thus, by combining the above inequalities together, one obtains
E||ϕy(t)−ϕz(t)||2≤ 3Γ2(p)[[Γ(P)Rp]2b2isupt∈(0,T]{e−2Rt|yi(t)−zi(t)|2}+[Γ(p)RP]2(n∑k=1a2ikF2k)n∑k=1supt∈(0,T]{e−2Rt|yk(t)−zk(t)|2}+[Γ(p)Rp]2(n∑k=1d2ikF2k)n∑k=1supt∈(0,T]{e−2Rt|yk(t)−zk(t)|2}]≤[3R2p][{||B||+||A||||F||+||D||||F||}E||y(t)−z(t)||2]≤[3[||B||+||A||||F||+||D||||F||]R2p]E||y(t)−z(t)||2 | (3.45) |
Similarly, by the same procedures as the above inequality, we obtains
ui(t)−si(t)=1Γ(p)∫t0(t−s)p−1[−vi[ui(t−δ)−si(t−δ)]+wi[gi(yi(t−δ))−gi(zi(t−δ))]]ds|ϕi(ui(t))−ϕisi(t)|2≤2Γ2(p)[|∫t0(t−s)p−1vi[ui(s−δ)−si(s−δ)]ds|2+|∫t0(t−s)p−1wi[gi(yi(t))−gi(xi(t))]ds|2]e−2Rt|ϕiui(t)−ϕisi(t)|2≤2Γ2(p)[v2i|∫t0(t−s)p−1e−Rt[ui(s−δ)−si(s−δ)]ds|2+w2i|∫t0(t−s)p−1e−Rt[gi(yi(t))−gi(zi(t))]ds|2] | (3.46) |
By using Cauchy inequality
v2i|∫t0(t−s)p−1e−Rt[ui(s−δ)−si(s−δ)]ds|2≤v2i(∫t0(t−s)p−1e−R(t−s)ds)(∫t0(t−s)p−1e−R(t−s)e−2Rs|ui(s−δ)−si(s−δ)|2ds)≤Γ(p)Rpv2i[∫tδ(t−s)p−1e−R(t−s)e−2Rs|ui(s−δ)−si(s−δ)|2ds+∫δ0(t−s)p−1e−R(t−s)e−2Rs|ψi(s−δ)−ψi(s−δ)|2ds]≤Γ(p)Rpv2i[∫tδ(t−s)p−1e−R(t−s)e−2Rs|ui(s−δ)−si(s−δ)|2ds]≤Γ(p)Rpv2i[∫t−δ0(t−γ−δ)p−1e−R(t−γ−δ)e−2Rγe−2Rδ|ui(γ)−si(γ)|2ds]≤Γ(p)Rpv2isupt∈(0,T]{e−2Rt|ui(t)−si(t)|2}e−2Rδ∫t−δ0ξp−1e−Rξdξ}≤[Γ(p)Rp]2v2isupt∈(0,T]{e−2Rt|ui(t)−si(t)|2} | (3.47) |
w2i|∫t0(t−s)p−1e−Rt[giyi(s)−gi(zi(s))]ds|2≤w2i(∫t0(t−s)p−1e−R(t−s)e−Rs|Fi|||yi(s)−zi(s)|ds)2≤w2i(∫t0(t−s)p−1e−R(t−s)ds)2(∫t0(t−s)p−1e−R(t−s)e−2Rs|Fi|yi(s)−zi(s)|2ds)≤w2i|Fi|supt∈(0,T]{e−2Rt|yi(t)−zi(t)|2}(∫t0(t−s)p−1e−R(t−s)ds)2≤[Γ(p)Rp]2w2i|Fi|supt∈(0,T]{e−2Rt|yi(t)−zi(t)|2} | (3.48) |
From (3.47) and (3.48) in (3.46)
E||ϕiui(t)−ϕisi(t)||2≤2Γ2(p)[[Γ(p)Rp]2v2isupt∈(0,T]{e−2Rt|ui(t)−si(t)|2}+[Γ(p)Rp]2w2i|Fi|supt∈(0,T]{e−2Rt|yi(t)−zi(t)|2}]E||ϕiui(t)−ϕisi(t)||2≤2(||V||)R2pE||u(t)−s(t)||2+2||W||||F||R2pE||y(t)−z(t)||2 | (3.49) |
From (3.45) and (3.49)
E||ϕiyi(t)−ϕizi(t)||2≤[3[||B||+||A||||F||+||D||||F||]R2p]E||y(t)−z(t)||2E||ϕiui(t)−ϕisi(t)||2≤2(||V||)R2pE||u(t)−s(t)||2+2||W||||F||R2pE||y(t)−z(t)||2 |
By combining the both the equations we get
E||ϕiyi(t)−ϕizi(t)||2+E||ϕiui(t)−ϕisi(t)||2≤[3[||B||+||A||||F||+||D||||F||]R2p]E||y(t)−z(t)||2+2(||V||)R2pE||u(t)−s(t)||2+2||W||||F||R2pE||y(t)−z(t)||2E||ϕiyi(t)−ϕizi(t)||2+E||ϕiui(t)−ϕisi(t)||2≤[3[||B||+||A||||F||+||D||||F||]R2p+2||W||||F||R2p]E||y(t)−z(t)||2+2(||V||)R2pE||u(t)−s(t)||2 |
where
K∗1=[3[||B||+||A||||F||+||D||||F||]R2p+2||W||||F||R2p], | (3.50) |
K∗2=2(||V||)R2p. | (3.51) |
E||ϕy(t)−ϕz(t)||2≤K∗1E||y(t)−z(t)||2, | (3.52) |
E||ϕ(u(t))−ϕ(s(t))||2≤K∗2E||u(t)−s(t)||2. | (3.53) |
Therefore the mapping ϕ is a contraction mapping. As a consequence of the Banach fixed point theorem, the problem Eq (3.40) has a unique fixed point, so that we conclude that system Eq (3.38) has a unique solution, which complete the proof of the theorem.
Remark 3. If there are no stochastic disturbance, then system (3.4) becomes the following fractional-order competitive neural networks with leakage delay:
Dpzi(t)=−bizi(t−μ)+n∑k=1aikgk(zk(t))+n∑k=1dikgk(zk(t−η))+cisi(t)Dpsi(t)=−visi(t−δ)+wigi(zi(t));i=1,2,...,n, | (3.54) |
Theorem 3.4. If Assumption 1 hold, the solution of system given by Eq (3.54) satisfying initial condition is uniformly stable in mean square.
Proof. Assume that z(t)=(z1(t),...,zn(t),s1(t),.........,sn(t))T and y(t)=(y1(t),...,yn(t),u1(t),.....,un(t))T are solutions of Eq (3.54) with the different initial conditions zi=ϕi(γ)∈L2F0([−τ,R],R),si=ψi(γ)∈L2F0([−τ,R],R),i∈n, one has
Dp[yi(t)−zi(t)]=−bi[yi(t−μ)−zi(t−μ)]+n∑k=1aik[gk(zk(t))−gk(zk(t))]+n∑i=1dik[gk(zk(t−η))−gk(zk(t−η))]Dp[ui(t)−si(t)]=−vi[ui(t−δ)−si(t−δ)]+wi[gi(yi(t))−gi(zi(t))];i=1,2,...,n, | (3.55) |
Based on Lemma 2.4, the solution of the system Eq (3.55) can be expressed in the following form
yi(t)−zi(t)=ψi(0)−ϕi(0)+Ip[−bi[yi(t−μ)−zi(t−μ)]+n∑k=1aik[gk(zk(t))−gk(zk(t))]+n∑k=1dik[gk(zk(t−η))−gk(zk(t−η))]]=ψi(0)−ϕi(0)+1Γ(α)∫t0(t−s)p−1[−bi[yi(t−μ)−zi(t−μ)]+n∑k=1aik[gk(zk(t))−gk(zk(t))]+n∑k=1dik[gk(zk(t−η))−gk(zk(t−η))]]ds=ψi(0)−ϕi(0)+1Γ(α)∫t0(t−s)p−1[−biyi(t−μ)−zi(t−μ)]ds+1Γ(α)∫t0(t−s)p−1n∑k=1aik[gk(zk(t))−gk(zk(t))]ds+1Γ(α)∫t0(t−s)p−1n∑i=1dik[gk(zk(t−η))−gk(zk(t−η))]]ds | (3.56) |
Then we have
e−2Rt|yi(t)−zi(t)|2≤5e−2Rt|ψi(0)−ϕi(0)|2+5Γ2(α)[b2i|∫t0(t−s)p−1e−Rt(yi(s−μ)−zi(s−μ)ds|2+|∫t0(t−s)p−1e−Rtn∑k=1aik[gk(yk(s))−gk(zk(s))]ds|2+|∫t0(t−s)p−1e−Rtn∑k=1dik[gk(zk(s−η))−gk(zk(s−η))]ds2] | (3.57) |
Firstly, we observe that
b2i|∫t0(t−s)p−1e−Rt[yi(s−μ)−zi(s−μ)]ds|2≤Γ(p)Rpb2i∫t0(t−s)p−1e−R(t−s)e−2Rsn∑k=1|yk(s−μ)−zk(s−μ)|2ds≤Γ(p)Rpb2i(∫0−μ(t−γ−μ)p−1e−R(t−γ−μ)e−2Rγe−2Rμn∑k=1|ψk(γ)−ϕk(γ)|2dγ+∫t−μ0(t−γ−μ)p−1e−R(t−γ−μ)e−2Rγe−2Rμn∑k=1|yk(γ)−zk(γ)|2dγ)≤[Γ(p)Rp]2b2isupt∈[−μ,0]{e−2Rt|ψk(t)−ϕk(t)|2}+[Γ(p)Rp]2b2isupt∈(0,T]{e−2Rt|yk(t)−zk(t)|2} | (3.58) |
Secondly, we can get form Assumption 1
n∑k=1|∫t0(t−s)p−1e−Rt[aikgkyk(s)−aikgk(zk(s))]ds|2≤(∫t0(t−s)p−1e−R(t−s)e−Rsn∑k=1|aik||gkyk(s)−gkzk(s)|ds)2≤(∫t0(t−s)p−1e−R(t−s)e−Rsn∑k=1|aik|Fk|yk(s)−zk(s)|ds)2≤(∫t0(t−s)p−1e−R(t−s))(∫t0(t−s)p−1e−R(t−s)dse−2Rsn∑k=1|aik|Fk|yk(s)−zk(s)|2ds)≤(∫t0(t−s)p−1e−R(t−s)ds)(∫t0(t−s)p−1e−R(t−s)e−2Rs(n∑k=1a2ikF2k)(n∑k=1|yk(s)−zk(s)|)2ds)≤(n∑k=1a2ikF2k)(∫t0(t−s)p−1e−R(t−s)ds)(∫t0(t−s)p−1e−R(t−s)e−2Rs|yk(s)−zk(s)|)2ds)≤(n∑k=1a2ikF2k)n∑k=1supt∈(0,T]{e−2Rt|yk(t)−zk(t)|2}(∫t0(t−s)p−1e−R(t−s)ds)2≤[Γ(p)Rp]2(a2ikF2k)n∑k=1supt∈(0,T]{e−2Rt|yk(t)−zk(t)|2} | (3.59) |
|∫t0(t−s)p−1e−Rt[n∑k=1dikgk(yk(s−η))−n∑k=1dikgk(zk(s−η))]ds|2≤(∫t0(t−s)p−1e−R(t−s)e−Rsn∑k=1Fk|dik||yk(s−η)−zk(s−η)|ds)2≤(∫t0(t−s)p−1e−R(t−s)ds)(∫t0(t−s)p−1e−2R(t−s)e−2Rsn∑k=1Fk|dik||yk(s−η)−zk(s−η)|2ds)≤[Γ(p)Rp](n∑k=1d2ikF2k)[∫0−η(t−γ−η)p−1e−R(t−γ−η)e−2Rγe−2Rηn∑k=1|ψk(γ)−ϕk(γ)|2dγ+∫t−η0(t−γ−η)p−1e−R(t−γ−η)e−2Rγe−2Rηn∑k=1|yk(γ)−zk(γ)|2dγ≤[Γ(p)Rp]2(n∑k=1F2kd2ik)n∑k=1supt∈[−η,0]{e−2Rt|ψk(γ)−ϕk(γ)|2}+[Γ(p)Rp]2(n∑k=1F2kd2ik)n∑k=1supt∈(0,T]{e−2Rt|yk(γ)−zk(γ)|2} | (3.60) |
Consequently, by combining the above inequalities together, we have
E||yi(t)−zi(t)||2≤5e−2Rt|ψi(0)−ϕi(0)|2+5Γ2(p)[Γ(p)Rp]2b2in∑k=1supt∈[μ,0]{e−2Rt|ψk(γ)−ϕk(γ)|2}+5Γ2(p)[Γ(p)Rp]2b2in∑k=1supt∈(0,T]{e−2Rt|yk(γ)−zk(γ)|2}+5Γ2(p)[Γ(p)Rp]2(n∑k=1a2ikF2k)n∑k=1supt∈(0,T]{e−2Rt|yk(t)−zk(t)|2}+5Γ2(p)[Γ(p)Rp]2(n∑k=1F2kd2ik)n∑k=1supt∈(−η,0){e−2Rt|ψk(γ)−ϕk(γ)|2}+5Γ2(p)[Γ(p)Rp]2(n∑k=1F2kd2ik)n∑k=1supt∈(−η,0){e−2Rt|yk(γ)−zk(γ)|2}≤5||ψ(t)−ϕ(t)||+5R2p||B||||ψ(t)−ϕ(t)||2+5R2p||B||||y(t)−z(t)||2+5R2p||A||||F||||y(t)−z(t)||2+5R2p||D||||F||||ψ(t)−ϕ(t)||2+5R2p||D||||F||||y(t)−z(t)||2E||y(t)−z(t)||2≤5[1+||B||+||F||||D||R2p]E||ψ(t)−ϕ(t)||2+5[||B||+||F||||A||+||F||||D||R2p]E||y(t)−z(t)||2E||y(t)−z(t)||2≤[5[1+||B||+||F||||D||R2p]1−5[||B||+||A||||F||+||F||||D||R2p]]E||ψ(t)−ϕ(t)||2 | (3.61) |
where,
L1=[5[1+||B||+||F||||D||R2p]1−5[||B||+||A||||F||+||F||||D||R2p]]
Similarly, by the same procedures as the above inequality, we obtains
ui(t)−si(t)=ψi(0)−ϕi(0)+1Γ(p)∫t0(t−s)p−1[−vi[ui(s−δ)−si(s−δ)]+wi[gi(yi(s))−gi(zi(s))]]dse−2Rt|ui(t)−si(t)|2≤5e−2Rt|ψi(0)−ϕi(0)|2+5Γ2(p)[v2i|∫t0(t−s)p−1e−Rt[ui(s−δ)−si(s−δ)|2]+w2i|∫t0(t−s)p−1e−Rt[gi(yi(s))−gi(zi(s))|2]]ds | (3.62) |
w2i|∫t0(t−s)p−1e−Rt[gi(yi(s))−gi(zi(s))|2]]ds≤[Γ(p)Rp]2w2i||F||supt∈(0,A]{e−2Rt|yk(t)−zk(t)|2} | (3.63) |
v2i|∫t0(t−s)p−1e−Rt[ui(s−δ)−si(s−δ)|2]]ds≤Γ(p)Rpv2i∫t0(t−s)p−1e−R(t−s)e−2Rsn∑k=1|uk(s−δ)−sk(s−δ)|2ds≤Γ(p)Rpv2i∫0−δ(t−γ−δ)p−1e−R(t−γ−δ)e−2Rγe−2Rδn∑k=1|ψk(γ)−ϕk(γ)|2dγ+∫t−δ0(t−γ−δ)p−1e−R(t−γ−δ)e−2Rγe−2Rδn∑k=1|uk(γ)−sk(γ)|2dγ≤[Γ(p)Rp]2v2isup[−δ,0]{e−2Rt|ψk(t)−ϕk(t)|2}+[Γ(p)Rp]2v2isup(0,T]{e−2Rt|uk(t)−sk(t)|2} | (3.64) |
Substituting the above (3.63) and (3.64) in the above equation (3.62) we get
e−2Rt|ui(t)−si(t)|2≤5e−2Rt|ψi(0)−ϕi(0)|2+5Γ2(p){[Γ(p)Rp]2w2i||F||supt∈(0,T]{e−2Rt|yk(t)−zk(t)|2}+[Γ(p)Rp]2v2isup[−δ,0]{e−2Rt|ψk(t)−ϕk(t)|2}+[Γ(p)Rp]2v2isup(0,T]{e−2Rt|uk(t)−sk(t)|2}}≤5||ψ(t)−ϕ(t)||2+5R2p||W||||F||E||y(t)−z(t)||2+5R2p||V|||E||ψ(t)−ϕ(t)||2+5R2p||V||E||u(t)−s(t)||2E||u(t)−s(t)||2≤5[1+||V||R2p]E||ψ(t)−ϕ(t)||2+5R2p[||W||||F||]E||y(t)−z(t)||2+5R2p||V||E||u(t)−s(t)||2 | (3.65) |
From Eqs (3.61) and (3.65)
E||y(t)−z(t)||2≤[5[1+||B||+||F||||D||R2p]1−5[||B||+||A||||D||+||F||||D||R2p]]E||ψ(t)−ϕ(t)||2E||u(t)−s(t)||2≤5[1+||V||R2p]E||ψ(t)−ϕ(t)||2+5R2p[||W||||F||]E||y(t)−x(t)||2+5||V||R2pE||u(t)−s(t)||2[1−5||V||R2p]E||u(t)−s(t)||2≤5[1+||V||R2p]E||ψ(t)−ϕ(t)||2+5R2p[||W||||F||]E||y(t)−z(t)||2 |
E||u(t)−s(t)||2≤5[1+||V||R2p]E||ψ(t)−ϕ(t)||2+5R2p[||W||||F||][[5[1+||B||+||F||||D||R2p]1−5[||B||+||A||||D||+||F||||D||R2p]]E||ψ(t)−ϕ(t)||2][1−5||V||R2p]≤{5[1+||V||R2p]+5R2p[||W||||F||][[5[1+||B||+||F||||D||R2p]1−5[||B||+||A||||D||+||F||||D||R2p]]][1−5||V||R2p]}E||ψ(t)−ϕ(t)||2 |
L∗1=[5[1+||B||+||F||||D||R2p1−5[||B||+||A||||D||+||F||||D||R2P]]L∗2={5[1+||V||R2p]+5R2p[||W||||F||][[5[1+||B||+||F||||D||R2p1−5[||B||+||A||||D||+||F||||D||R2p]][1−5||V||R2p]}. |
By the above two equations we get
E||y(t)−z(t)||2≤δ1E||ψ(t)−ϕ(t)||2E||u(t)−s(t)||2≤δ2E||ψ(t)−ϕ(t)||2, |
E||y(t)−z(t)||2≤ε1 | (3.66) |
E||ψ(t)−ϕ(t)||2≤δ1 | (3.67) |
E||u(t)−s(t)||2≤ε2 | (3.68) |
E||ψ(t)−ϕ(t)||2≤δ2. | (3.69) |
which means that the solution of system Eq (3.54) is uniformly stable in mean square.
Remark 4. The author derived the existence and uniqueness results using Banach contraction fixed point theorem, sufficient conditions for uniform stability of equilibrium point for the networks. But, it is more complicated to study the stochastic fractional-order competitive neural networks with leakage delays. This interesting problem competitive neural networks model.
Remark 5. In this paper we investigated the mean square stability of stochastic fractional-order competitive neural networks with leakage delays with 12≤α<1 by using Cauchy Schwarz inequality. Many authors have focused on studying the stability analysis of fractional-order neural networks which depends on the orders a of fractional derivatives. Unlike the previous works, we analyzed the stability of stochastic fractional-order neural networks with delays and leakage terms which are dependent on the orders α and β different fractional derivatives and reflect the close relation between neuron activation functions, time-delay of network parameters, and coefficient terms. This was motivated by the long range delay dependent dynamic process that is part of our current project. However, we propose to investigate the proposed problem for not only 0<α<1, but also more general set of linearly independent multi time-scales.
Example 4.1. Consider the stochastic fractional-order competitive neural networks with leakage delay terms as follows:
DPzi(t)=−bizi(t−μ)+n∑k=1aikgk(zk(t))+n∑i=1dikgk(zk(t−η))+cisi(t)+n∑k=1σik(zk(t),zk(t−η))dwk(t)dtDPsi(t)=−visi(t−δ)+wigi(zi(t));i=1,2,...,n, | (4.1) |
where p=0.9, g1(z1(t))=12(|z1(t)+1|+|z1(t)−1|), g2(x2(t))=z2(t).σik(zk(t),zk(t−η))=√640zj(t),i,j=1,2. Obviously, η=12. Choose b1=0.8, b2=0.5, a11=a12=0.89,a21=0.67,a22=0.76, d11=0.98,d12=0.99,d21=0.67,d22=−0.96. Clearly, the nonlinear functions gj(⋅) and σ(⋅) satisfy condition Assumption 1. We can get the following inequality easily ||B||=4.297,||A||=6.429,||D||=9.267,||F||=0.428,||K||=8.876,||V||=5.245,||W||=6.356,p=3.467,R=4.274.
Substituting in Theorem 3.1, we get
K1=[4[||B||+||A||||F||+||D||||F||]R2p+4||K||Γ(2p−1)Γ2(p)Rp]+2||F||||W||R2p=2.5576,K2=2||V||R2p=0.0005 |
which satisfy K1>K2, By Theorem 3.1, the system (4.1) is uniformly stable in mean square. State trajectories of the system are given in Figure 1.
Example 4.2. Consider the stochastic fractional-order competitive neural networks with leakage delay terms as follows:
Dpzi(t)=−bizi(t−μ)+n∑k=1aikgk(zk(t))+n∑i=1dikgk(zk(t−η))+cisi(t)+n∑k=1σik(zk(t),zk(t−η))dwk(t)dtDpsi(t)=−visi(t−δ)+wigi(zi(t));i=1,2,...,n, | (4.2) |
where p=0.2, g1(z1(t))=12(|z1(t)+1|+|z1(t)−1|), g2(x2(t))=z2(t).σik(zk(t),zk(t−η))=√320zj(t),i,j=1,2. Obviously, η=12. Choose b1=0.89, b2=0.15, a11=a12=0.19,a21=0.27,a22=0.16, d11=0.18,d12=0.1,d21=0.27,d22=−2.96. We can get the following inequality easily ||B||=2.256,||A||=4.257,||D||=7.749,||F||=9.467,||K||=6.474,||V||=6.667,||W||=5.678,P=2.227,R=5.729.
Substituting in Theorem 3.2, we get
L1=[5[1+||B||+||F||||D||R2p+Γ(2p−1)||K||Γ2(p)Rp]1−5[||B||+||A||||D||+||F||||D||R2p+Γ(2p−1)2||K||Γ2(p)Rp]]=65.7507.L2={5[1+||v||R2p]+5R2p[||w||||F||][[5[1+||B||+||F||||D||R2p+Γ(2p−1)||K||Γ2(p)Rp]1−5[||B||+||A||||D||+||F||||D||R2p+Γ(2p−1)||K||Γ2(p)Rp]]][1−5||v||R2P]}L2=5.15940.9833=5.2470. |
which satisfy L1>L2, By Theorem 3.2, the system (4.2) is uniformly stable in mean square.State trajectories of the system are given in Figure 2.
Example 4.3. Consider the fractional-order competitive neural networks with leakage delay terms as follows:
Dpzi(t)=−bizi(t−μ)+n∑k=1aikgk(zk(t))+n∑i=1dikgk(zk(t−η))+cisi(t)Dpsi(t)=−visi(t−δ)+wigi(zi(t));i=1,2,...,n, | (4.3) |
where p=0.9, g1(z1(t))=12(|z1(t)+1|+|z1(t)−1|), g2(x2(t))=z2(t).σik(zk(t),zk(t−η))=√740zj(t),i,j=1,2. Obviously, η=12. Choose b1=0.6, b2=0.45, a11=a12=0.39,a21=0.17,a22=0.64, d11=0.38,d12=0.99,d21=0.27,d22=−2.96. Clearly, the nonlinear functions gj(⋅) and η(⋅) satisfy condition Assumption 1. We can get the following inequality easily, ||B||=0.264,||A||=0.467,||D||=0.124,||F||=1.246,||V||=0.276,||W||=0.227,R=0.224. Substituting above values in Theorem 3.3, we get
K∗1=3[||B||+||A||||F||+||D||||F||]R2p+2||W||||F||R2p=8.1616,K∗2=2(||V||)R2p=0.9864. |
which satisfy K∗1>K∗2, By Theorem 3.3, the system (4.3) is uniformly stable in mean square.State trajectories of the system are given in Figure 3.
Example 4.4. Consider the fractional-order competitive neural networks with leakage delay terms as follows:
Dpzi(t)=−bizi(t−μ)+n∑k=1aikgk(zk(t))+n∑i=1dikgk(zk(t−η))+cisi(t)Dpsi(t)=−visi(t−δ)+wigi(zi(t));i=1,2,...,n, | (4.4) |
where p=0.9, g1(z1(t))=12(|z1(t)+1|+|z1(t)−1|), g2(x2(t))=z2(t).σik(zk(t),zk(t−η))=√940zj(t),i,j=1,2. Obviously, η=12. Choose b1=0.2, b2=0.1, a11=a12=0.19,a21=0.27,a22=0.16, d11=0.28,d12=0.19,d21=0.47,d22=−0.46. We can get the following inequality easily ||B||=2.227,||A||=1.246,||D||=4.222,||F||=3.328,||V||=4.242,||W||=6.267,R=9.474. Substituting above values in Theorem 3.4, we get
L∗1=[5[1+||B||+||F||||D||R2p]1−5[||B||+||A||||F||+||F||||D||R2p]]=3.1883,L∗2={5[1+||v||R2p]+5R2p[||w||||F||][[5[1+||B||+||F||||D||R2p]1−5[||B||+||A||||D||+||F||||D||R2P]]][1−5||v||R2p]}=6.6008−2.8153=−2.3446 |
which satisfy L∗1>L∗2, By Theorem 3.4, the system (4.4) is uniformly stable in mean square. State trajectories of the system are given in Figure 4.
In this paper, we investigate the stability analysis of stochastic fractional-order competitive neural networks with leakage delay. As is well known, there are many stability results about integer-order neural networks in the past few decades, most of which are obtained by constructing Lyapunov function, but these results and methods could not be extended and applied to fractional-order case. According to the Cauchy-Schwartz inequality, Burkholder-Davis-Gundy inequality, analysis techniques, some sufficient conditions were derived to guarantee the existence and uniqueness and the uniform stability in mean square. Furthermore, the main tools used in this paper are stochastic analysis techniques, fractional calculations and Banach contraction principle. Finally, four numerical examples are given to illustrate the effectiveness of the proposed theories.
The author Syeda Asma Kauser express her thanks to the deanship of scientific research (DSR) Prince Sattam bin Abdul Aziz Univeristy, Saudi Arabia for providing facilities and support.
All authors declare there is no conflict of interest in this paper.
[1] | K. Diethelm, The analysis of fractional differential equations, Springer, 2010. |
[2] | C. Huang, B. Liu, New studies on dynamic analysis of inertial neural networks involving non-reduced order method, Neurocomputing, 325 (2019), 283–287. |
[3] | J. Wang, X. Chen, L. Huang, The number and stability of limit cycles for planar piecewise linear systems of node-saddle type, J. Math. Anal. Appl., 469 (2019), 405–427. |
[4] | Y. Zuo, Y. Wang, X. Liu, Adaptive robust control strategy for rhombus-type lunar exploration wheeled mobile robot using wavelet transform and probabilistic neural network, Comput. Appl. Math., 37 (2018), 314–337. |
[5] | C. Song, S. Fei, J. Cao, C. Huang, Robust synchronization of fractional-order uncertain chaotic systems based on output feedback sliding mode control, Mathematics, 7 (2019), 599. |
[6] | C. Huang, J. Cao, F. Wen, X. Yang, Stability analysis of SIR model with distributed delay on complex networks, PLOS One, 11 (2016). |
[7] | I. Podlubny, Geometric and physical interpretation of fractional integration and fractional differentiation, Fract. Calc. Appl. Anal., 5 (2002), 367–386. |
[8] | Z. Rashidnejad, P. Karimaghaee, Synchronization of a class of uncertain chaotic systems utilizing a new finite-time fractional adaptive sliding mode control, Chaos, Solitons and Fractals: X, 5 (2020), 100042. |
[9] | Y. Zhou, F. Jiao, J. Pecaric, On the Cauchy problem for fractional functional differential equations in Banach spaces, Topol. Method. Nonl. An., 42 (2013), 119–136. |
[10] | A. Chauhan, J. Dabas, Local and global existence of mild solution to an impulsive fractional functional integro-differential equation with nonlocal condition, Commun. Nonlinear Sci., 19 (2014), 821–829. |
[11] | L. Xu, X. Chu, H. Hu, Exponential ultimate bounded ness of non-autonomous fractional differential systems with time delay and impulses, Appl. Math. Lett., 99 (2020), 106000. |
[12] | J. T. Edwards, N. J. Ford, A. C. Simpson, The numerical solution of linear multi-term fractional differential equations: systems of equations, J. Comput. Appl. Math., 148 (2002), 401–418. |
[13] | A. Arbi, J. Cao, Pseudo-almost periodic solution on time-space scales for a novel class of competitive neutral-type neural networks with mixed time-varying delays and leakage delays, Neural Process. Lett., 46 (2017), 719–745. |
[14] | Y. Liu, W. Liu, M. A. Obaid, I. A. Abbas, Exponential stability of Markovian jumping Cohen-Grossberg neural networks with mixed mode-dependent time-delays, Neurocomputing, 177 (2016), 409–415. |
[15] | I. Stamova, T. Stamov, X. Li, Global exponential stability of a class of impulsive cellular neural networks with Supremums, Int. J. Adapt. Control, 28 (2014), 1227–1239. |
[16] | X. Li, S. Song, Impulsive control for existence, uniqueness and global stability of periodic solutions of recurrent neural networks with discrete and continuously distributed delays, IEEE T. Neur. Net. Lear., 24(2013), 868–877. |
[17] | Q. Zhu, J. Cao, Stability analysis of Markovian jump stochastic BAM neural networks with impulse control and mixed time delays, IEEE T. Neur. Net. Lear., 23 (2012), 467–479. |
[18] | M. Syed Ali, P. Balasubramaniam, F. A. Rihan, S. Lakshmanan, Stability criteria for stochastic Takagi-Sugeno fuzzy Cohen-Grossberg BAM neural networks with mixed time-varying delays, Complexity, 21 (2016), 143–154. |
[19] | H. Zhang, M. Ye, J. Cao, A. Alsaedi, Synchronization control of Riemann-Liouville fractional competitive network systems with time-varying delay and different time scales, Int. J. Control Autom., 16 (2018), 1–11. |
[20] | P. Liu, X. Nie, J. Liang, J. Cao, Multiple Mittag-Leffler stability of fractional-order competitive neural networks with gaussian activation functions, Neural Networks, 108 (2018), 452–465. |
[21] | L. J. Banu, P. Balasubramaniam, Robust stability analysis for discrete-time neural networks with time-varying leakage delays and random parameter uncertainties, Neurocomputing, 179 (2016), 126–134. |
[22] | D. J. Lu, C. J. Li, Exponential stability of stochastic high-order BAM neural networks with time delays and impulsive effects, Neural Comput. Appl., 23 (2013), 1–8. |
[23] | X. Lv, X. Li, Finite time stability and controller design for nonlinear impulsive sampled-data systems with applications, ISA T., 70 (2017), 30–36. |
[24] | A. L. Wu, Z. G. Zeng, X. G. Song, Global Mittag-Leffler stabilization of fractional-order bidirectional associative memory neural networks, Neurocomputing, 177 (2016), 489–496. |
[25] | F. Wang, Y. Q. Yang, X. Y. Xu, L. Li, Global asymptotic stability of impulsive fractional-order BAM neural networks with time delay, Neural Comput. Appl., 28 (2017), 345–352. |
[26] | W. Chen, W. Zheng, Robust stability analysis for stochastic neural networks with time-varying delay, IEEE T. Neural Networks, 21 (2010), 508–514. |
[27] | H. Gu, Adaptive synchronization for competitive neural networks with different time scales and stochastic perturbation, Neurocomputing, 73 (2009), 350–356. |
[28] | O. M. Kwon, S. M. Lee, J. H. Park, Improved delay-dependent exponential stability for uncertain stochastic neural networks with time-varying delays, Phys. Lett. A, 374 (2010), 1232–1241. |
[29] | J. H. Park, O. M. Kwon, Analysis on global stability of stochastic neural networks of neutral type, Mod. Phys. Lett. B, 22 (2008), 3159–3170. |
[30] | J. H. Park, O. M. Kwon, Synchronization of neural networks of neutral type with stochastic perturbation, Mod. Phys. Lett. B, 23 (2009), 1743–1751. |
[31] | J. H. Park, S. M. Lee, H. Y. Jung, LMI optimization approach to synchronization of stochastic delayed discrete-time complex networks, J. Optimiz. Theory Appl., 143 (2009), 357–367. |
[32] | W. Su, Y. Chen, Global robust stability criteria of stochastic Cohen-Grossberg neural networks with discrete and distributed time-varying delays, Commun. Nonlinear Sci., 14 (2009), 520–528. |
[33] | X. Yang, J. Cao, Y. Long, R. Wei, Adaptive lag synchronization for competitive neural networks with mixed delays and uncertain hybrid perturbations, IEEE T. Neural Networks, 21 (2010), 1656–1667. |
[34] | X. Yang, C. Huang, J. Cao, An LMI approach for exponential synchronization of switched stochastic competitive neural networks with mixed delays, Neural Comput. Appl., 21 (2012), 2033–2047. |
[35] | D. G. Hobson, L. C. G. Rogers, Complete models with stochastic volatility, Mathematical Finance, 8 (1998), 27–48. |
[36] | J. Hull, A. White, The pricing of options on assets with stochastic volatilities, Journal of Finance, 42 (1987), 281–300. |
[37] | I. Karatzas, Steven E. Shreve, Brownian motion and stochastic calculus, Springer-Verlag, 1991. |
[38] | N. Laskin, Fractional market dynamics, Physica A, 287 (2000), 482–492. |
[39] | C. Andoh, Stochastic variance models in discrete time with feed forward neural networks, Neural Computation, 21 (2009), 1990–2008. |
[40] | S. Giebel, M. Rainer, Neural network calibrated stochastic processes: forecasting financial assets, Cent. Eur. J. Oper. Res., 21 (2013), 277–293. |
[41] | J. Cao, Q. Yang, Z. Huang, Q. Liu, Asymptotically almost periodic solutions of stochastic functional differential equations, Appl. Math. Comput., 218 (2011), 1499–1511. |
[42] | V. B. Kolmanovskii, A. Myshkis, Applied Theory of Functional Differential Equations, Kluwer Academic Publishers, Norwell, 1992. |
[43] | Y. Ren, L. Chen, A note on the neutral stochastic functional differential equations with infinite delay and Possion jumps in an abstract space, J. Math. Phys., 50 (2009), 082704. |
[44] | Y. Ren, N. Xia, Existence uniqueness and stability of the solutions to neutral stochastic functional differential equations with infinite delay, Appl. Math. Comput., 210 (2009), 72–79. |
[45] | R. Sakthivel, J. Luo, Asymptotic stability of impulsive stochastic partial differential equations with infinite delays, J. Math. Anal. Appl., 356 (2009), 1–6. |
[46] | R. Sakthivel, J. Luo, Asymptotic stability of nonlinear impulsive stochastic differential equations, Stat. Probabil. Lett., 79 (2009), 1219–1223. |
[47] | R. Sakthivel, J. J. Nieto, N. I. Mahmudov, Approximate controllability of nonlinear deterministic and stochastic systems with unbounded delay, Taiwan. J. Math., 14 (2010), 1777–1797. |
[48] | B. Xie, Stochastic differential equations with non-lipschitz coefficients in Hilbert spaces, Stoch. Anal. Appl., 26 (2008), 408–433. |
[49] | L. Arnold, Stochastic differential equations: theory and applications, Wiley, 1974. |
[50] | I. I. Gihman, A. V. Skorohod, Stochastic differential equations, Springer, 1972. |
[51] | G. S. Ladde, V. Lakshmikantham, Random differential inequalities, New York: Academic Press, 1980. |
[52] | A. Friedman, Stochastic differential equations and Applications, Academic Press, 1975. |
[53] | H. Holden, B. Oksendal, J. Uboe, T. Zhang, Stochastic partial differential equations: A modeling, white noise functional approach, Boston: BirkhMauser, 1996. |
[54] | F. Biagini, Y. Hu, B. Oksendal, T. Zhang, Stochastic calculus for fractional Brownian motion and applications, Springer, 2008. |
[55] | D. He, L. Xu, Boundedness analysis of stochastic integro-differential systems with Levy noise, J. Taibah Univ. Sci., 14 (2020), 87–93. |
[56] | A. G. Ladde, G. S. Ladde, Dynamic processes under random Environment, Bulletin of the Marathwada Mathematical Society, 8 (2007), 96–123. |
[57] | J. Chen, Z. Zeng, P. Jiang, Global mittag-leffler stability and synchronization of memristor-based fractional-order neural networks, Neural Networks, 51 (2014), 1–8. |
[58] | P. L. Butzer, U. Westphal, An Introduction to Fractional Calculus, World Scientific: Singapore, 2001. |
[59] | X. Yang, Q. Song, Y. Liu, Z. Zhao, Finite-time stability analysis of fractional-order neural networks with delay, Neurocomputing, 152 (2015), 19–26. |
[60] | L. Chen, R. Wu, D. Pan, Mean square exponential stability of impulsive stochastic fuzzy cellular neural networks with distributed delays, Expert Syst. Appl., 38 (2011), 6294–6299. |
1. | M. Hymavathi, G. Muhiuddin, M. Syed Ali, Jehad F. Al-Amri, Nallappan Gunasekaran, R. Vadivel, Global Exponential Stability of Fractional Order Complex-Valued Neural Networks with Leakage Delay and Mixed Time Varying Delays, 2022, 6, 2504-3110, 140, 10.3390/fractalfract6030140 | |
2. | Xiuping Han, M. Hymavathi, Sumaya Sanober, Bhawna Dhupia, M. Syed Ali, Robust Stability of Fractional Order Memristive BAM Neural Networks with Mixed and Additive Time Varying Delays, 2022, 6, 2504-3110, 62, 10.3390/fractalfract6020062 | |
3. | M. Syed Ali, M. Hymavathi, Hamed Alsulami, Tareq Saeed, Bashir Ahmad, Zhiwei Gao, Passivity Analysis of Fractional-Order Neutral-Type Fuzzy Cellular BAM Neural Networks with Time-Varying Delays, 2022, 2022, 1563-5147, 1, 10.1155/2022/9035736 | |
4. | Hamed Alsulami, M. Syed Ali, M. Hymavathi, Tareq Saeed, Bashir Ahmad, Ahmed Alsaedi, Asier Ibeas, Mixed ℋ ∞ and Passivity Analysis of Delayed Fractional-Order Complex Dynamical Networks with Hybrid Coupling, 2022, 2022, 1563-5147, 1, 10.1155/2022/6327922 | |
5. | M. Syed Ali, M. Hymavathi, Syeda Asma Kauser, Grienggrai Rajchakit, Porpattama Hammachukiattikul, Nattakan Boonsatit, Synchronization of Fractional Order Uncertain BAM Competitive Neural Networks, 2021, 6, 2504-3110, 14, 10.3390/fractalfract6010014 | |
6. | Yongkun Li, Xiaohui Wang, Almost periodic solutions in distribution of Clifford-valued stochastic recurrent neural networks with time-varying delays, 2021, 153, 09600779, 111536, 10.1016/j.chaos.2021.111536 | |
7. | A. Al Themairi, Manar A. Alqudah, M Syed Ali, Existence and Uniqueness of Caputo Fractional Predator-Prey Model of Holling-Type II with Numerical Simulations, 2021, 2021, 1563-5147, 1, 10.1155/2021/2990958 | |
8. | Chenguang Xu, Minghui Jiang, Junhao Hu, Mean-square finite-time synchronization of stochastic competitive neural networks with infinite time-varying delays and reaction–diffusion terms, 2023, 127, 10075704, 107535, 10.1016/j.cnsns.2023.107535 | |
9. | Dandan Tang, Baoxian Wang, Caiqing Hao, Dissipativity of Stochastic Competitive Neural Networks with Multiple Time Delays, 2024, 56, 1573-773X, 10.1007/s11063-024-11569-1 | |
10. | Jinde Cao, K. Udhayakumar, R. Rakkiyappan, Xiaodi Li, Jianquan Lu, A Comprehensive Review of Continuous-/Discontinuous-Time Fractional-Order Multidimensional Neural Networks, 2023, 34, 2162-237X, 5476, 10.1109/TNNLS.2021.3129829 | |
11. | Yang Cao, A.R. Subhashri, A. Chandrasekar, T. Radhika, Krzysztof Przybyszewski, Exponential State Estimation for Delayed Competitive Neural Network Via Stochastic Sampled-Data Control with Markov Jump Parameters Under Actuator Failure, 2024, 14, 2449-6499, 373, 10.2478/jaiscr-2024-0020 | |
12. | Ting Yuan, Huizhen Qu, Dong Pan, Random periodic oscillations and global mean-square exponential stability of discrete-space and discrete-time stochastic competitive neural networks with Dirichlet boundary condition, 2023, 45, 10641246, 3729, 10.3233/JIFS-230821 | |
13. | Soo-Oh Yang, Jea-Hyun Park, Analysis for the hierarchical architecture of the heterogeneous FitzHugh-Nagumo network inducing synchronization, 2023, 8, 2473-6988, 22385, 10.3934/math.20231142 |