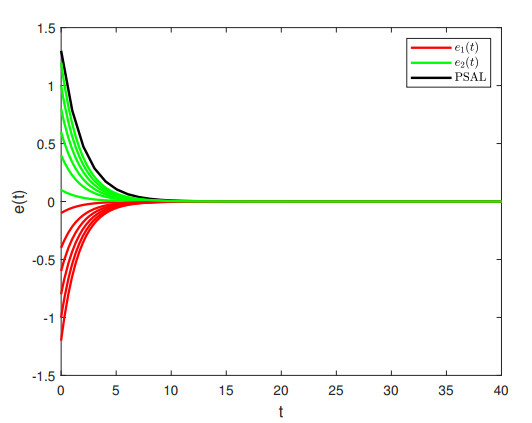
This paper discusses the robustness of neutral-type Cohen-Grossberg neural networks with time delays and stochastic disturbances. And the problem is whether the Cohen-Grossberg neural networks, which originally maintain exponential stability, still achieves exponential stability when subjected to three simultaneous disturbances, namely, time delays, stochastic perturbations, and neutral terms. First, the width of the time delays, the strength of the stochastic disturbances, and the neutral term preset parameter size are derived through the Bellman-Gronwall Lemma, the Itô formula, and the properties of integrals. Next, the values of the three perturbation factors of time delay, stochastic disturbance, and neutral term are obtained by solving a multivariate privacy transcendental equation, which allows the Cohen-Grossberg neural networks to remain exponentially stable after being disturbed. Finally, the numerical example is provided to validate the results of this brief.
Citation: Yijia Zhang, Tao Xie, Yunlong Ma. Robustness analysis of exponential stability of Cohen-Grossberg neural network with neutral terms[J]. AIMS Mathematics, 2025, 10(3): 4938-4954. doi: 10.3934/math.2025226
[1] | Tao Xie, Wenqing Zheng . Robustness analysis of Cohen-Grossberg neural network with piecewise constant argument and stochastic disturbances. AIMS Mathematics, 2024, 9(2): 3097-3125. doi: 10.3934/math.2024151 |
[2] | Ruoyu Wei, Jinde Cao, Wenhua Qian, Changfeng Xue, Xiaoshuai Ding . Finite-time and fixed-time stabilization of inertial memristive Cohen-Grossberg neural networks via non-reduced order method. AIMS Mathematics, 2021, 6(7): 6915-6932. doi: 10.3934/math.2021405 |
[3] | Pratap Anbalagan, Evren Hincal, Raja Ramachandran, Dumitru Baleanu, Jinde Cao, Chuangxia Huang, Michal Niezabitowski . Delay-coupled fractional order complex Cohen-Grossberg neural networks under parameter uncertainty: Synchronization stability criteria. AIMS Mathematics, 2021, 6(3): 2844-2873. doi: 10.3934/math.2021172 |
[4] | Mohammed D. Kassim . A fractional Halanay inequality for neutral systems and its application to Cohen-Grossberg neural networks. AIMS Mathematics, 2025, 10(2): 2466-2491. doi: 10.3934/math.2025115 |
[5] | Yunlong Ma, Tao Xie, Yijia Zhang . Robustness analysis of neutral fuzzy cellular neural networks with stochastic disturbances and time delays. AIMS Mathematics, 2024, 9(10): 29556-29572. doi: 10.3934/math.20241431 |
[6] | Qinghua Zhou, Li Wan, Hongshan Wang, Hongbo Fu, Qunjiao Zhang . Exponential stability of Cohen-Grossberg neural networks with multiple time-varying delays and distributed delays. AIMS Mathematics, 2023, 8(8): 19161-19171. doi: 10.3934/math.2023978 |
[7] | Biwen Li, Yibo Sun . Stability analysis of Cohen-Grossberg neural networks with time-varying delay by flexible terminal interpolation method. AIMS Mathematics, 2023, 8(8): 17744-17764. doi: 10.3934/math.2023906 |
[8] | Shuting Chen, Ke Wang, Jiang Liu, Xiaojie Lin . Periodic solutions of Cohen-Grossberg-type Bi-directional associative memory neural networks with neutral delays and impulses. AIMS Mathematics, 2021, 6(3): 2539-2558. doi: 10.3934/math.2021154 |
[9] | R. Sriraman, R. Samidurai, V. C. Amritha, G. Rachakit, Prasanalakshmi Balaji . System decomposition-based stability criteria for Takagi-Sugeno fuzzy uncertain stochastic delayed neural networks in quaternion field. AIMS Mathematics, 2023, 8(5): 11589-11616. doi: 10.3934/math.2023587 |
[10] | Hongmei Zhang, Xiangnian Yin, Hai Zhang, Weiwei Zhang . New criteria on global Mittag-Leffler synchronization for Caputo-type delayed Cohen-Grossberg Inertial Neural Networks. AIMS Mathematics, 2023, 8(12): 29239-29259. doi: 10.3934/math.20231497 |
This paper discusses the robustness of neutral-type Cohen-Grossberg neural networks with time delays and stochastic disturbances. And the problem is whether the Cohen-Grossberg neural networks, which originally maintain exponential stability, still achieves exponential stability when subjected to three simultaneous disturbances, namely, time delays, stochastic perturbations, and neutral terms. First, the width of the time delays, the strength of the stochastic disturbances, and the neutral term preset parameter size are derived through the Bellman-Gronwall Lemma, the Itô formula, and the properties of integrals. Next, the values of the three perturbation factors of time delay, stochastic disturbance, and neutral term are obtained by solving a multivariate privacy transcendental equation, which allows the Cohen-Grossberg neural networks to remain exponentially stable after being disturbed. Finally, the numerical example is provided to validate the results of this brief.
The Cohen-Grossberg network model was first proposed by Cohen and Grossberg [1], and it has become one of the most important network models. In particular, recurrent neural networks and Hopfield neural networks are special cases of Cohen-Grossberg neural networks (CGNNs) [1]. The CGNNs have received more and more attention from scholars due to their potential applications in many fields such as signal processing [2], image processing [3], associative memory [4] and combinatorial optimisation [5]. The evolution of many control systems is not only determined by the states at the current and past moments, but also by the changing rate of the states at the past moments. The system with the derivatives of states at the past moments plays a decisive role, as a very important class of systems, which is the neutral system [6]. Different from general systems, the neutral system can more accurately and deeply reflect the law of system changing. The majority of systems can be regarded as a special case of the neutral system. Consequently, neutral CGNNs have received considerable attention; for instance, here are some interesting findings on CGNNs with NTs [2,6,7,8,9,10,11].
As we all know, stability is often a prerequisite before the practical applications, so there have been some previous efforts, to name a few, as follows, [12,13,14,15,16,17,18,19,20] report the stable conditions of various types of neural networks under different exogenous perturbations, including neutral terms (NTs) [10,14,20], time delays (TDs) [15,17,18,21], stochastic disturbances (SDs) [16,17,20], or other external disturbances such as uncertainty parameters [22,23], reaction-diffusion terms [24,25], etc. Noting that the aforementioned literature has focused on the issue of stability or synchronization, with only a few scholars addressing the robustness of stability (RoS). Robustness is the capacity of a system to retain its properties across a spectrum of parameters or structural alterations. Although results on system robustness are relatively few compared to various stability studies, there are still some interesting findings [20,26,27,28,29]. However, the problem of robustness for CGNNs with neutral terms is more complex due to the existence of the amplification functions and the derivative time delay terms, which is the focus of this brief.
In the decades, Zhang et al. [30] conducted the global exponential adaptive synchronization of neutral neural networks with random perturbations. Faydasicok et al. [31] investigated the global asymptotic stability of a class of CGNNs with TDs and NTs. Wan and Zhou [32,33] discussed the stability or exponential stability of the neutral CGNNs with TDs, respectively. Fang et al. [26] addressed the robustness of fuzzy systems with segmented variables and random disturbances. Zhu and Cao [35] investigated the robust exponential stability of CGNNs with impulsive stochastic Markov switching and TDs. Wang et al. [20] studied the global robust exponential synchronization problem for CGNNs with neutral-type intervals and mixed time delays. Despite the numerous references, the combined effect of the aforementioned perturbations on the neutral CGNNs with stochastic disturbances and time delays (STNCGNNs) with RoS has not yet been obtained. Moreover, in the previous explorations on the RoS analysis of the system [10,26,36], the calculation of the upper limit the system can withstand is complicated in the presence of multiple disruptions. A binary linkage criterion for the robustness of a nonlinear BAM with two disturbing factors was provided in [29], which reduced the computational complexity. This also prompts us to consider the influence of three or even more factors. How to formulate the interactions between the perturbations mentioned above more succinctly that warrants further study.
Following the aforementioned discussions, this brief discusses the sufficient condition to guarantee the robustness of the exponential stability of STNCGNNs. The main contributions to the objectives of concern are as follows:
(1) By employing some inequality techniques, including the Bellman-Gronwall Lemma, the Itô formula, and the Cauchy inequality, the multivariate implicit transcendental equation incorporating random perturbations, time delays, and contraction coefficients of the neutral term is obtained. Consequently, the upper bounds on the impact of these perturbations on the stability of CGNNs are estimated, which guarantees the initially stabilized CGNNs are able to maintain GES when subjected to perturbations. The idea of RoS can be utilized to control scenarios where disturbances are present.
(2) This paper investigates the robust exponential stability of Cohen-Grossberg neural networks with NTs, TDs, and SDs. This paper considers the effects of three factors, rather than just one or two as previously discussed in the literature ([13,14,16,32,35,36,37]). It not only enriches the theoretical study of CGNNs but also provides theoretical support for the analysis and design of the stability of the Cohen-Grossberg system.
(3) Instead of solving multiple transcendence equations as [26,36], when there are multiple disturbances, the upper bounds on the disturbances that the system can withstand are calculated by solving the same implicit transcendence equation that characterizes the coupling between the NTs, TDs, or SDs in the form of a boundary constraint, which simplifies the computational process. The method for establishing the upper bound is further simplified.
(4) CGNNs are a generalized recurrent neural network that contains numerous neural networks due to its amplification function. How to deal with the effect of the amplification function on CGNNs is an issue. In this brief, we consider a class of bounded amplification functions, which ensures that neurons respond efficiently to input variations throughout the range of activity and accelerate system convergence to a steady state.
The following is an overview of the structure of this brief. Preliminaries and modeling are presented in Section Ⅱ. The theoretical result of the RoS of STNCGNNs is shown in Section Ⅲ. Simulations are given in Section Ⅳ. Finally, conclusions are drawn in Section Ⅴ.
For the remainder of this article, the set of real numbers is denoted by R, and N∗ denotes the set of integers from 1 to N. Denote R+=[0,+∞), and let the n-dimensional Euclidean space be denoted by Rn. The vector norm ||ζ||=∑ni=1|ζi| for any vector ζ∈RN, and for a matrix M, ||M||=max1≤j≤n∑ni=1|kij|. Let (Ω,F,{Ft}t≥0,P) be a complete probability space. {Ft}t≥0, a filtration, is right-continuous and increasing, and contains all P-null sets. E(⋅) stands for the mathematical expectation operator about the probability measure P.
Consider the following model of CGNNs:
˙ui(t)=di(ui(t))[−hi(ui(t))+N∑j=1cijfj(uj(t))+Ii],t≥t0,u(t0)=u0,i∈N∗, | (2.1) |
where N represents the number of cells, t0 is the initial moment, and u0 is the initial state of CGNNs (2.1). The vector u(t)=(u1(t),...,uN(t)) describes the state of the ith unit at time t, and di(t) refers to an amplification function. The function hi is a well-behaved function that ensures the solutions of CGNNs (2.1) are bounded. The coefficient cij is the configuration strength between cell i and cell j. fi represents the activation function of the ith cell, and Ii is a constant parameter that represents the external input.
Assuming u∗ is an equilibrium point of CGNNs (2.1), and we translate the equilibrium point to the origin. Let e(t)=u(t)−u∗; consequently, system (2.1) can be converted to the following form:
˙ei(t)=−ai(ei(t))[bi(ei(t))−N∑j=1cijgj(ej(t))],t≥t0,e(t0)=e0,i∈N∗, | (2.2) |
where e0=u0−u∗, ai(ei(t))=di(ei(t)+u∗i), bi(ei(t))=hi(ei(t)+u∗i)−hi(u∗i), gj(ei(t))=fj(ei(t)+u∗i)−fj(u∗i). It is evident that the origin is a point of equilibrium of CGNNs (2.2). Therefore, the stability of u∗ is equivalent to the stability of the origin of CGNNs (2.2).
For the purpose of this paper, we give some necessary assumptions.
Assumption 1. [3] There are ¯a>0 and a_>0 satisfying
a_≤ai(e)≤¯a,∀e∈R,i∈N∗, |
for functions ai(⋅), and ai(⋅) are continuous and bounded.
Assumption 2. [11] For functions bi(⋅), there are constants Bi>0, i∈N∗, satisfying
bi(y)−bi(x)y−x≤Bi,∀y,x∈R,y≠x. |
We investigate the model of neutral Cohen-Grossberg neural networks with TDs, and SDs in this paper, which is given as follows:
d[qi(t)−N∑j=1Gij(qj(t−δ))]=−ai(qi(t))[bi(qi(t))−N∑j=1cijgj(qj(t))−N∑j=1lijwj(qj(t−δ))]dt+σqi(t)dB(t),t≥t0,qi(t)=φi(t−t0),i∈N∗, | (2.3) |
where ai(⋅), bi(⋅), gj(⋅), and cij are the same as in (2.2). Gij(⋅) is the neutral term, and δ is the time delay. lij and wj(⋅) are the connection strength and activation function with time delay, respectively. σ represents the noise strength, and the process B(t) is a scalar Brownian motion, defined on the probability space (Ω,F,{Ft}t≥0,P).
Assumption 3. [10] These functions gj(⋅), wj(⋅) and Gij(⋅) satisfy the Lipschitz condition
|gj(y)−gj(x)|≤Kj|y−x|,∀x,y∈R,|wj(y)−wj(x)|≤Wj|y−x|,∀x,y∈R,|Gij(y)−Gij(x)|≤Pij|y−x|,∀x,y∈R, |
where Kj and Wj are known constants, g(0)=0, and w(0)=0,j=1,2,...,N, and the value of Pij is constrained to the interval (0, 1) to guarantee the non-increasing monotonicity of the system.
Remark 1. The Lipschitz conditions are common in the existing literature [2,10,34,38], and Assumptions 3–5 are included here. The value of the neutral term preset parameter P∈(0,1) is in order to ensure that the functions Gij(⋅) of (2.3) are decaying rather than growing and finally reach the GES. The same constraint is found in the literature [10,38].
From Assumption 3, for any initial value t0 and e0, CGNNs (2.2) have a unique state e(t;t0,e0) for t≥t0. And for any initial value t0∈R,φ(⋅)∈Rn, STNCGNNs (2.3) exhibit a unique solution, denoted by q(t;t0,φ(⋅)) for t≥t0, and q=0 is the equilibrium point of STNCGNNs (2.3).
If system (2.3) does not have neutral terms, time delays, and random disturbances, it degenerates into the following form:
˙ei(t)=−ai(ei(t))[bi(ei(t))−N∑j=1cijgj(ej(t))−N∑j=1lijwj(ej(t))],t≥t0,e(t0)=e0=φ(0),i∈N∗. | (2.4) |
Now we give definitions for the global exponential stability of CGNNs (2.4) and the mean square global exponential stability of STNCGNNs (2.3).
Definition 1. [10] The state of CGNNs (2.4) is global exponential stability (GES) if there are positive scalars α and β such that ||e(t;t0,e0)||≤||e0||αexp(−β(t−t0)) holds, where t≥t0, t0∈R+, e0∈Rn.
Definition 2. [30] STNCGNNs (2.3) is almost surely global exponential stability (ASGES), if for any t0∈R+, q0∈Rn, the Lyapunov exponent
limsupt⟶∞(ln|q(t;t0,q0)|t)<0 |
almost surely, where q(t;t0,q0) is the state of STNCGNNs (2.3).
Definition 3. [30] STNCGNNs (2.3) is mean square global exponential stability (MSGES), if for any t0∈R+, q0∈Rn, the Lyapunov exponent
limsupt⟶∞(ln|q(t;t0,q0)|2t)<0, |
where q(t;t0,q0) is the state of STNCGNNs (2.3).
Remark 2. It can be seen from Definitions 2 and 3 that ASGES implies the MSGES, but the converse is not true. It is worth noting that when Assumption 3 holds, and STNCGNNs (2.3) is MSGES, then STNCGNNs (2.3) is ASGES.
Definition 4. [26] The state of STNCGNNs (2.3) is MSGES if there are positive scalars ¯α and ¯β satisfying E||q(t;t0,φ(t0))||2≤||φ(t0)2||¯αexp(−¯β(t−t0)) for t≥t0, t0∈R+, φ(t0)∈Rn.
Assumption 4.
P2(1008Θ2(¯a−a_)2||L||2W2+6)<exp(−2Θ˜h)/2, | (2.5) |
where ˜h=z1+2z5, z1=42Θ[ξΘ+2(¯a−a_)2||C||2K2]+6σ2, z5=126κδ(6ξ+σ2).
Assumption 5.
42ζα2/βexp(84Θ[ξ+2Θ(¯a−a_)2||C||2K2])+2α2exp(−2βΘ)<1. | (2.6) |
where ξ=Θ¯a2(||B||2+||C||2K2+||L||2W2), ζ=Θ(¯a−a_)2(||B||2+2||C||2K2+||L||2W2).
In this section, we are going to give the sufficiency criterion that the perturbed neutral CGNNs can still remain stable.
Theorem 1. Let Assumption 1 through Assumption 5 be true, and system (2.4) achieves GES. The MSGES and ASGES of STNCGNNs (2.3) are guaranteed if 0<P<˜P, 0<δ<˜δ, and 0<σ<˜σ, ˜P, ˜δ, and ˜σ can be obtained by solving the transcendental equation:
2h2exp(2Θ˜h)1−h1exp(2Θ˜h)+2α2exp(−2β(Θ−δ))=1, | (3.1) |
where z1=42Θ[ξΘ+2(¯a−a_)2||C||2K2]+6σ2, z2=42ζ+6σ2, z3=42κδ(ϖ+4), z4=1008Θκ||P||2, z5=126κδ(6ξ+σ2), h1=2z4+12||P||2, h2=z3+z4+18||P||2+(z2/2+z5)α2/β+h1α2exp(−2β(Θ−δ)), ˜h=z1+2z5, ˆm=supt0−δ+Θ≤t≤t0−δ+2ΘE||e(t)−q(t)||2, m=supt0−δ≤t≤t0−δ+ΘE||q(t)||2, Θ≥ln2α2/β.
Proof. From systems (2.3) and (2.4), we have
ei(t)−qi(t)+N∑j=1Gij(qj(t−δ))−N∑j=1Gij(qj(t0−δ))=∫tt0{−ai(ei(s))[bi(ei(s))−N∑j=1cijgj(ej(s))−N∑j=1lijwj(ej(s))]+ai(qi(s))[bi(qi(s))−N∑j=1cijgj(qj(s))−N∑j=1lijwj(qj(s−δ))]}ds−∫tt0σqi(s)dB(s). | (3.2) |
When t0≤t≤t0+2Θ, we further obtain
E||e(t)−q(t)||2≤3EN∑i=1|N∑j=1Gij(qj(t0−δ))−N∑j=1Gij(qj(t−δ))|2+3EN∑i=1|∫tt0{ai(qi(s))bi(qi(s))−ai(ei(s)bi(ei(s)))+N∑j=1cij[ai(ei(s))gj(ej(s))−ai(qi(s))gj(qj(s))]+N∑j=1lij[ai(ei(s))wj(ej(s))−ai(qi(s))wj(qj(s−δ))]}ds|2+3EN∑i=1|∫tt0σqi(s)dB(s)|2≤3EN∑i=1[N∑j=1Pij|qj(t0−δ)−qj(t−δ)|]2+3EN∑i=1{∫tt0{¯aBi|qi(s)−ei(s)|+(¯a−a_)Bi|ei(s)|+N∑j=1|cij|[¯aKj|ej(s)−qj(s)|+(¯a−a_)Kj|qj(s)|]+N∑j=1|lij|[(¯a−a_)Wj|ej(s)|+¯aWj|ej(s)−qj(s)|+¯aWj|qj(s)−qj(s−δ)|]}ds}2+3EN∑i=1∫tt0|σqi(s)|2ds≤6||P||2E||q(t0−δ)||2+6||P||2E||q(t−δ)||2+21(t−t0)[¯a2||B||2∫tt0E||q(s)−e(s)||2ds+(¯a−a_)2||B||2∫tt0E||e(s)||2ds+¯a2||C||2K2∫tt0E||e(s)−q(s)||2ds+(¯a−a_)2||C||2K2∫tt0E||q(s)||2ds+(¯a−a_)2||L||2W2∫tt0E||e(s)||2ds+¯a2||L||2W2∫tt0E||e(s)−q(s)||2ds+¯a2||L||2W2∫tt0E||q(s)−q(s−δ)||2ds]+6σ2∫tt0E||e(s)||2ds+6σ2∫tt0E||q(s)−e(s)||2ds. | (3.3) |
When t0≤t≤t0+2Θ, we have
E||e(t)−q(t)||2≤6||P||2supt0−δ≤t≤t0E||q(t)||2+6||P||2E||q(t−δ)||2+(42ζ+6σ2)∫tt0E||e(s)||2ds+z1∫tt0E||e(s)−q(s)||2ds+42κ∫tt0E||q(s)−q(s−δ)||2ds. | (3.4) |
where κ=Θ¯a2||L||2W2, ξ=Θ¯a2(||B||2+||C||2K2+||L||2W2), and ζ=Θ(¯a−a_)2(||B||2+2||C||2K2+||L||2W2), z1=42Θ[ξΘ+2(¯a−a_)2||C||2K2]+6σ2.
Next, we deal with the time delay term.
∫tt0E||q(s)−q(s−δ)||2ds=∫t0+δt0E||q(s)−q(s−δ)||2ds+∫tt0+δE||q(s)−q(s−δ)||2ds. | (3.5) |
When t0≤t≤t0+δ, we have
∫t0+δt0E||q(s)−q(s−δ)||2ds≤2∫t0+δt0E||q(s)||2ds+2∫t0+δt0E||q(s−δ)||2ds≤4δsupt0−δ≤s≤t0+δE||q(s)||2. | (3.6) |
When t0+δ≤t≤t0+2Θ, by (2.3), the Cauchy inequality and the expectation inequality, we obtain
∫tt0+δE||q(s)−q(s−δ)||2≤∫tt0+δ{3EN∑i=1|N∑j=1Gij(qj(s−δ))−N∑j=1Gij(qj(s−2δ))|2+3EN∑i=1|∫ss−δσqi(r)dB(r)|2+3EN∑i=1|∫ss−δ−ai(qi(r))[bi(qi(r))−N∑j=1cijgj(qj(r))−N∑j=1lijwj(qj(r−δ))]dr|2}ds≤6||P||2∫tt0+δ(E||q(s−δ)||2+E||q(s−2δ)||2)ds+[18(ξ−κ)+3σ2]∫tt0+δds∫ss−δE||q(r)||2dr+18κ∫tt0+δds∫ss−δE||q(r−δ)||2dr. | (3.7) |
For the first item in the inequality (3.7), we have
∫tt0+δ(E||q(s−δ)||2+E||q(s−2δ)||2≤∫t−δt0E||q(s)||2ds+∫t−2δt0−δE||q(s)||2ds≤δsupt0−δ≤s≤t0E||q(s)||2+2∫t−δt0E||q(s)||2ds≤3δsupt0−δ≤s≤t0+δE||q(s)||2+4Θsupt0+δ≤s≤t0−δ+2ΘE||q(s)||2. | (3.8) |
By changing the order of integrations, we have
∫tt0+δds∫ss−δE||q(r)||2dr=∫tt0dr∫min(r+δ,t)max(t0+δ,r)E||q(r)||2ds≤δ∫tt0E||q(r)||2dr, | (3.9) |
and
∫tt0+δds∫ss−δE||q(r−δ)||2dr=∫tt0dr∫min(r+δ,t)max(t0+δ,r)E||q(r−δ)||2ds≤δ∫tt0E||q(r−δ)||2dr≤δ2supt0−δ≤s≤t0E||q(s)||2+δ∫tt0E||q(r)||2dr. | (3.10) |
Substituting (3.8)–(3.10) into (3.7) for t0+δ≤t≤t0+2Θ, we obtain
∫tt0+δE||q(s)−q(s−δ)||2ds≤6||P||2(3δsupt0−δ≤s≤t0+δE||q(s)||2+4Θsupt0+δ≤s≤t0−δ+2ΘE||q(s)||2)+δ[18(ξ−κ)+3σ2]∫tt0E||q(s)||2ds+18κδ2supt0−δ≤s≤t0E||q(s)||2+18κδ∫tt0E||q(s)||2ds)≤δϖsupt0−δ≤s≤t0+δE||q(s)||2+24Θ||P||2supt0+δ≤s≤t0−δ+2ΘE||q(s)||2+3δ(6ξ+σ2)∫tt0E||q(s)||2ds, | (3.11) |
where ϖ=18(||P||2+Θ¯a2||L||2W2δ).
Substituting (3.11) and (3.6) into (3.4), we have
E||e(t)−q(t)||2≤6||P||2(supt0−δ≤t≤t0E||q(t)||2+supt0−δ≤t≤t0−δ+2ΘE||q(t)||2)+z1∫tt0E||e(s)−q(s)||2ds+(42ζ+6σ2)∫tt0E||e(s)||2ds+42κδ(ϖ+4)supt0−δ≤s≤t0+δE||q(s)||2+1008Θκ||P||2supt0+δ≤s≤t0−δ+2ΘE||q(s)||2+126κδ(6ξ+σ2)∫tt0E||q(s)||2ds=12||P||2supt0−δ≤t≤t0+δE||q(t)||2+6||P||2supt0+δ≤s≤t0−δ+2ΘE||q(t)||2+z1∫tt0E||e(s)−q(s)||2ds+z2∫tt0E||e(s)||2ds+z3supt0−δ≤s≤t0+δE||q(s)||2+z5∫tt0E||q(s)||2ds+z4supt0+δ≤s≤t0−δ+2ΘE||q(s)||2≤(12||P||2+z3)supt0−δ≤t≤t0+δE||q(t)||2+(z4+6||P||2)supt0+δ≤t≤t0−δ+2ΘE||q(t)||2+(z1+2z5)∫tt0E||e(s)−q(s)||2ds+(z2+2z5)∫tt0E||e(s)||2ds≤(z1+2z5)∫tt0E||e(s)−q(s)||2ds+[z3+18||P||2+(z2/2+z5)α2/β+z4]supt0−δ≤t≤t0−δ+ΘE||q(t)||2+(z4+6||P||2)supt0−δ+Θ≤t≤t0−δ+2ΘE||q(t)||2≤(z1+2z5)∫tt0E||e(s)−q(s)||2ds+[z3+18||P||2+(z22+z5)α2/β+z4]supt0−δ≤t≤t0−δ+ΘE||q(t)||2+2(z4+6||P||2)supt0−δ+Θ≤t≤t0−δ+2ΘE||e(t)−q(t)||2+2(z4+6||P||2)supt0−δ+Θ≤t≤t0−δ+2ΘE||e(s)||2≤˜h∫tt0E||e(s)−q(s)||2ds+h1ˆm+h2m. | (3.12) |
Thus, by using the Bellman-Gronwall lemma, we obtain
E||e(t)−q(t)||2≤(h1ˆm+h2m)exp(2Θ˜h). |
Consequently, for t0−δ+Θ≤t≤t0−δ+2Θ, we have
ˆm=supt0−δ+Θ≤t≤t0−δ+2ΘE||e(s)−q(s)||2≤supt0≤t≤t0+2ΘE||e(t)−q(t)||2≤h2exp(2Θ˜h)1−h1exp(2Θ˜h)m, | (3.13) |
where z2=42ζ+6σ2, z3=42κδ(ϖ+4), z4=1008Θκ||P||2, z5=126κδ(6ξ+σ2), h1=2z4+12||P||2, h2=z3+z4+18||P||2+(z2/2+z5)α2/β+h1α2exp(−2β(Θ−δ)), ˜h=z1+2z5, ˆm=supt0−δ+Θ≤t≤t0−δ+2ΘE||e(t)−q(t)||2, m=supt0−δ≤t≤t0−δ+ΘE||q(t)||2.
Thus, by system (2.4) is GES, for t0−δ+Θ≤t≤t0−δ+2Θ, we have
E||q(t)||2≤2h2exp(2Θ˜h)1−h1exp(2Θ˜h)m+2E||e(t)||2≤[2h2exp(2Θ˜h)1−h1exp(2Θ˜h)+2α2exp(−2β(Θ−δ))]m. | (3.14) |
It is obviously that for t0≤t≤t0+2Θ, (3.14) is also true. Let
H(P,σ,δ)=2h2exp(2Θ˜h)1−h1exp(2Θ˜h)+2α2exp(−2β(Θ−δ)). | (3.15) |
If P=σ=δ=0, then
H(0,0,0)=42ζα2/β×exp(84Θ[ξ+2Θ(¯a−a_)2||C||2K2])+2α2exp(−2βΘ). |
From Assumption 7, we know that H(0,0,0)<1, and H(+∞,+∞,+∞)=+∞. This implies the existence of ˜P, ˜σ, and ˜δ, and this yields the transcendental equation H(˜P,˜σ,˜δ)=1, which provides the upper bounds for NTs, SDs, and TDs.
Define Υ=−lnH/Θ, one can see that Υ>0 when P<˜P, σ<˜σ, and δ<˜δ. From the existence and uniqueness of the solution of STNCGNNs (2.3), when t≥t0+(υ−1)Θ, for any υ=1,2,...,N, we have
q(t;t0,φ)=q(t;t0+(υ−1)Θ,q(t0+(υ−1)Θ;t0,φ)). | (3.16) |
By (3.14) and (3.16), it yields that
supt0−δ+υΘ≤t≤t0−δ+(υ+1)ΘE||q(t;t0,φ)||2≤exp(−ΥΘ)×supt0−δ+(υ−1)Θ≤t≤t0−δ+(υ−1)Θ+ΘE||q(t;t0,e0)||2≤...≤exp(−υΥΘ)supt0−δ≤t≤t0−δ+ΘE||q(t;t0,φ)||2=mexp(−υΥΘ). | (3.17) |
Therefore, for any t≥t0−δ+Θ, there exists a positive integer υ such that t0−δ+υΘ≤t≤t0−δ+(υ+1)Θ,
E||q(t;t0,φ)||2≤mexp(−Υ(t−t0+δ−Θ))=mexp(Υ(Θ−δ))exp(−Υ(t−t0)). | (3.18) |
Obviously, (3.18) also holds for t−δ≤t≤t0−δ+Θ. Therefore, system (2.3) is MSGES and ASGES.
Remark 3. Compared with the existing articles ([13,14,17,26]) that studied the robustness of the system, the system discussed in this article contains three factors, neutral terms, time delays, and random disturbances—which have more complexity and are more strongly connected to real-world applications.
Remark 4. Instead of solving multiple transcendental equations to compute the upper bounds on the disturbances that the system can withstand, a ternary implicit equation in the form of a boundary restriction is solved to obtain the upper bounds on the neutral terms, TDs, and SDs, which reduces the computational complexity.
Consider the system (2.3) with the following parameters:
C=[0.0060.0020.0030.008],L=[0.0120.0070.0020.005], |
a1(⋅)=1.5+0.5sin(⋅), a2(⋅)=1.5−0.5cos(⋅), bi(⋅)=0.015(⋅), f(⋅)=tanh(⋅), and g(⋅)=(⋅)/(1+exp(−(⋅))). Then, it can be obtained: ¯a=2, a_=1 and
B=[0.015000.015]. |
Based on the parameters above, the stable state trajectories of system (2.4) are shown in Figure 1, where PSAL is the function of the selected α=1.3 and β=0.5. By (3.1), when only a single disturbance occurs, the maximum values are ˜P=0.0318, ˜δ=0.1011, and ˜σ=0.0505, respectively. The trajectories of the system (2.3) with P=0.02, δ=0.0357, and σ=0.025 are depicted in Figure 2, where the initial values of states q1(t) and q2(t) are {0.5,0.1,0.3,0.8,−0.6,−0.4,−0.9,−0.2} and {−0.1,−0.5,−0.3,−0.8,0.6,0.4,0.9,0.2}.
From Eq (3.1), it can be seen that P, δ, and σ are interconnected, with a change in one value affecting the others. As an illustration, if we select P=0.0195 and δ=0.042, through the application of (3.1), we obtain ˜σ=0.0227. When P, δ, and σ no longer satisfy (3.1), the trajectories of system (2.3) are shown in Figure 3 for P=0.02, δ=0.2727, and σ=0.23, where the initial values of states q1(t) and q2(t) are {0.5,0.1,0.3,0.8,0.6,0.4,0.9,0.2} and {−0.1,−0.5,−0.3,−0.8,−0.6,−0.4,−0.9,−0.2}.
Remark 5. The RoS of Theorem 1 in Section III implies that if the actual values of perturbations of CGNNs (2.3) are limited to tolerable limits, then system (2.3) will again be stable. In Section IV, Figure 1 describes the original stable trajectories of (2.4). Figure 2 illustrates the state trajectories of STNCGNNs (2.3) when the actual values of the external disturbances satisfy the implicit Eq (3.1), which is stable. And Figure 3 presents the state trajectories of STNCGNNs (2.3) for the actual values of the external disturbances no longer satisfying the implicit equation, which is unstable.
Next, we give an application of the RoS of CGNNs to recover noisy images (see Figure 4). The main steps are as follows:
(1) Constructing CGNNs-type Auto-Encoder for training to learn noise-free images.
(2) Input noisy image.
(3) Auto-Encoder for processing.
(4) Output recovered image.
Where the auto-encoder is trained based on reconstruction error and mean square error is used as the loss function.
Remark 6. For comparison purposes, Table 1 shows how this article differs from previous pivotal references, with × meaning it does not exist and ✓ indicating its existence. The results demonstrate that the findings of this paper further extend and reinforce the existing work on RoS.
Models/Refs. | NTs | TDs | SDs | CGNNs | RoS |
[6] | ✓ | ✓ | × | × | × |
[7,8,9,11] | ✓ | ✓ | × | ✓ | × |
[10] | ✓ | ✓ | × | × | ✓ |
[13,35] | × | ✓ | × | ✓ | ✓ |
[15,16,34] | × | ✓ | ✓ | ✓ | × |
[20,37] | ✓ | ✓ | × | ✓ | ✓ |
[26] | × | × | ✓ | × | ✓ |
[36] | × | ✓ | ✓ | × | ✓ |
this article | ✓ | ✓ | ✓ | ✓ | ✓ |
This paper presents sufficient criteria for ensuring the robustness of global exponential stability of the neutral CGNNs with time delays and stochastic disturbances. According to the Itô integral, Gronwall lemma, and mathematical expectation inequality properties, a multivariable implicit transcendental equation can be used to derive upper bounds for the NTs, TDs and SDs when multiple disturbances are present, which greatly simplifies the computational complexity. A bounded restriction is imposed on the amplification function in order to accelerate system convergence and reduce time complexity.
In the future, we will further discuss the effect of other factors such as uncertain parameters, the derivative of deviation, and reaction-diffusion terms, etc., on the CGNNs with neutral terms and the case of expanding the amplification function to non-negative. Also consider combining famous methods like linear matrix inequalities and Liapunov function theory to reduce the conservatism.
Yijia Zhang: Theoretical derivation, simulation, and writing; Tao Xie: Supervision, writing-review, and editing; Yunlong Ma: Background survey and writing. All authors have read and approved the final version of the manuscript for publication.
The authors declare they have not used Artificial Intelligence (AI) tools in the creation of this article.
The authors declare that there are no conflicts of interest regarding the publication of this paper.
[1] |
M. A. Cohen, S. Grossberg, Absolute stability of global pattern formation and parallel memory storage by competitive neural networks, IEEE T. Syst. Man Cy., 1983,815–826. http://dx.doi.org/10.1109/tsmc.1983.6313075 doi: 10.1109/tsmc.1983.6313075
![]() |
[2] |
C. J. Cheng, T. L. Liao, J. J. Yan, C. C. Hwang, Globally asymptotic stability of a class of neutral-type neural networks with delays, IEEE T. Syst. Man Cy. B, 36 (2006), 1191–1195. http://dx.doi.org/10.1109/TSMCB.2006.874677 doi: 10.1109/TSMCB.2006.874677
![]() |
[3] |
P. Kowsalya, S. S. Mohanrasu, A. Kashkynbayev, P. Gokul, R. Rakkiyappan, Fixed-time synchronization of inertial Cohen-Grossberg neural networks with state dependent delayed impulse control and its application to multi-image encryption, Chaos Soliton. Fract., 181 (2024), 114693. http://dx.doi.org/10.1016/j.chaos.2024.114693 doi: 10.1016/j.chaos.2024.114693
![]() |
[4] |
J. Li, Z. Peng, Multi-source image fusion algorithm based on cellular neural networks with genetic algorithm, Optik, 126 (2015), 5230–5236. http://dx.doi.org/10.1016/j.ijleo.2015.09.187 doi: 10.1016/j.ijleo.2015.09.187
![]() |
[5] |
X. Hu, G. Feng, S. Duan, L. Liu, A memristive multilayer cellular neural network with applications to image processing, IEEE T. Neur. Net. Lear., 28 (2016), 1889–1901. http://dx.doi.org/10.1109/TNNLS.2016.2552640 doi: 10.1109/TNNLS.2016.2552640
![]() |
[6] |
X. Yang, Z. Cheng, X. Li, T. Ma, Exponential synchronization of coupled neutral-type neural networks with mixed delays via quantized output control, J. Franklin I., 356 (2019), 8138–8153. http://dx.doi.org/10.1016/j.jfranklin.2019.07.006 doi: 10.1016/j.jfranklin.2019.07.006
![]() |
[7] |
R. Samli, S. Senan, E. Yucel, Z. Orman, Some generalized global stability criteria for delayed Cohen–Grossberg neural networks of neutral-type, Chaos Soliton. Fract., 116 (2019), 198–207. http://dx.doi.org/10.1016/j.neunet.2019.04.023 doi: 10.1016/j.neunet.2019.04.023
![]() |
[8] |
Z. Zhang, X. Zhang, T. Yu, Global exponential stability of neutral-type Cohen–Grossberg neural networks with multiple time-varying neutral and discrete delays, Neurocomputing, 490 (2022), 124–131. http://dx.doi.org/10.1016/j.neucom.2022.03.068 doi: 10.1016/j.neucom.2022.03.068
![]() |
[9] |
F. Kong, Q. Zhu, Antiperiodic dynamical behaviors of discontinuous neutral-type Cohen-Grossberg neural networks with mixed time delays, Comput. Intel., 36 (2020), 698–719. http://dx.doi.org/10.1111/coin.12262 doi: 10.1111/coin.12262
![]() |
[10] |
Y. Shen, J. Wang, Robustness analysis of global exponential stability of non-linear systems with time delays and neutral terms, IET Control Theory A., 7 (2013), 1227–1232. http://dx.doi.org/10.1049/iet-cta.2012.0781 doi: 10.1049/iet-cta.2012.0781
![]() |
[11] |
O. Faydasicok, S. Arik, The combined Lyapunov functionals method for stability analysis of neutral Cohen-Grossberg neural networks with multiple delays, Neural Networks, 180 (2024), 106641. http://dx.doi.org/10.1016/j.neunet.2024.106641 doi: 10.1016/j.neunet.2024.106641
![]() |
[12] |
R. Li, J. Cao, A. Alsaedi, F. Alsaadi, Exponential and fixed-time synchronization of Cohen–Grossberg neural networks with time-varying delays and reaction-diffusion terms, Appl. Math. Comput., 313 (2017), 37–51. http://dx.doi.org/10.1016/j.amc.2017.05.073 doi: 10.1016/j.amc.2017.05.073
![]() |
[13] |
Y. Wan, J. Cao, G. Wen, W. Yu, Robust fixed-time synchronization of delayed Cohen–Grossberg neural networks, Neural Networks, 73 (2016), 86–94. http://dx.doi.org/10.1016/j.neunet.2015.10.009 doi: 10.1016/j.neunet.2015.10.009
![]() |
[14] |
N. Ozcan, New conditions for global stability of neutral-type delayed Cohen–Grossberg neural networks, Neural Networks, 106 (2018), 1–7. http://dx.doi.org/10.1016/j.neunet.2018.06.009 doi: 10.1016/j.neunet.2018.06.009
![]() |
[15] |
H. Zhang, Inequalities and stability of stochastic fuzzy delayed Cohen–Grossberg neural networks, IEEE Access, 2023. http://dx.doi.org/10.1109/ACCESS.2023.3300581 doi: 10.1109/ACCESS.2023.3300581
![]() |
[16] |
X. Z. Liu, K. N. Wu, X. Ding, W. Zhang, Boundary stabilization of stochastic delayed Cohen–Grossberg neural networks with diffusion terms, IEEE T. Neur. Net. Lear., 33 (2021), 3227–3237. http://dx.doi.org/10.1109/TNNLS.2021.3051363 doi: 10.1109/TNNLS.2021.3051363
![]() |
[17] | M. A. Jamal, S. Das, S. Mukhopadhyay, Fixed-time synchronization of delayed inertial Cohen–Grossberg neural networks with desynchronizing impulses, Commun. Nonlinear Sci., 130 (2024), 107772. http://dx.doi.org/0.1016/j.cnsns.2023.107772 |
[18] |
J. Cheng, W. Liu, Stability analysis of anti-periodic solutions for Cohen–Grossberg neural networks with inertial term and time delays, Mathematics, 12 (2024), 198. http://dx.doi.org/10.3390/math12020198 doi: 10.3390/math12020198
![]() |
[19] |
M. A. Jamal, R. Kumar, S. Mukhopadhyay, O. M. Kwon, Fixed-time stability of Cohen-Grossberg BAM neural networks with impulsive perturbations, Neurocomputing, 550 (2023), 126501. http://dx.doi.org/10.1016/j.neucom.2023.126501 doi: 10.1016/j.neucom.2023.126501
![]() |
[20] |
X. Wang, J. Lan, X. Yang, X. Zhang, Global robust exponential synchronization of neutral-type interval Cohen-Grossberg neural networks with mixed time delays, Inform. Sciences, 676 (2024), 120806. http://dx.doi.org/10.1016/j.ins.2024.120806 doi: 10.1016/j.ins.2024.120806
![]() |
[21] |
M. Shen, Z. Wang, S. Zhu, X. Zhao, G. Zong, Q. G. Wang, Neural network adaptive iterative learning control for strict-feedback unknown delay systems against input saturation, IEEE T. Neur. Net. Lear., 2024, 1–10. http://dx.doi.org/10.1109/TNNLS.2024.3452721 doi: 10.1109/TNNLS.2024.3452721
![]() |
[22] |
Q. Meng, Q. Ma, Y. Shi, Adaptive fixed-time stabilization for a class of uncertain nonlinear systems, IEEE T. Automatic Contr., 68 (2023), 6929–6936. http://dx.doi.org/10.1109/TAC.2023.3244151 doi: 10.1109/TAC.2023.3244151
![]() |
[23] |
L. Xing, C. Wen, Dynamic event-triggered adaptive control for a class of uncertain nonlinear systems, Automatica, 158 (2023), 111286. http://dx.doi.org/10.1016/j.automatica.2023.111286 doi: 10.1016/j.automatica.2023.111286
![]() |
[24] |
M. Shen, C. Wang, Q. G. Wang, Y. Sun, G. Zong, Synchronization of fractional reaction-diffusion complex networks with unknown couplings, IEEE T. Netw. Sci. Eng., 11 (2024), 4503–4512. http://dx.doi.org/10.1109/TNSE.2024.3432997 doi: 10.1109/TNSE.2024.3432997
![]() |
[25] |
H. Liu, J. Cheng, J. Cao, I. Katib, Preassigned-time synchronization for complex-valued memristive neural networks with reaction–diffusion terms and Markov parameters, Neural Networks, 169 (2024), 520–531. http://dx.doi.org/10.1016/j.neunet.2023.11.011 doi: 10.1016/j.neunet.2023.11.011
![]() |
[26] |
W. Fang, T. Xie, B. Li, Robustness analysis of fuzzy cellular neural network with deviating argument and stochastic disturbances, IEEE Access, 11 (2023), 3717–3728. http://dx.doi.org/10.1109/ACCESS.2023.3233946 doi: 10.1109/ACCESS.2023.3233946
![]() |
[27] |
S. Majhi, B. Rakshit, A. Sharma, J. Kurths, D. Ghosh, Dynamical robustness of network of oscillators, Phys. Rep., 1082 (2024), 1–46. http://dx.doi.org/10.1016/j.physrep.2024.06.003 doi: 10.1016/j.physrep.2024.06.003
![]() |
[28] |
O. Artime, M. Grassia, M. Domenico, J. P. Gleeson, H. A. Makse, G. Mangioni, Robustness and resilience of complex networks, Nat. Rev. Phys., 6 (2024), 114–131. http://dx.doi.org/10.1038/s42254-023-00676-y doi: 10.1038/s42254-023-00676-y
![]() |
[29] |
W. Si, S. Gao, F. Yan, N. Zhao, H. Zhang, H. Dong, Linkage-constraint criteria for robust exponential stability of nonlinear BAM system with derivative contraction coefficients and piecewise constant arguments, Inform. Sciences, 612 (2022), 926–941. http://dx.doi.org/10.1016/j.ins.2022.08.078 doi: 10.1016/j.ins.2022.08.078
![]() |
[30] |
Y. Zhang, D. W. Gu, S. Xu, Global exponential adaptive synchronization of complex dynamical networks with neutral-type neural network nodes and stochastic disturbances, IEEE T. Circuits I, 60 (2013), 2709–2718. http://dx.doi.org/10.1109/TCSI.2013.2249151 doi: 10.1109/TCSI.2013.2249151
![]() |
[31] |
O. Faydasicok, S. Arik, An analysis of stability of multiple delayed Cohen-Grossberg neural networks of neutral type, 2022 7th International Conference on Mathematics and Computers in Sciences and Industry (MCSI), 2022, 55–60. http://dx.doi.org/10.1109/MCSI55933.2022.00016 doi: 10.1109/MCSI55933.2022.00016
![]() |
[32] |
L. Wan, Q. Zhou, Stability analysis of neutral-type Cohen-Grossberg neural networks with multiple time-varying delays, IEEE Access, 8 (2020), 27618–27623. http://dx.doi.org/10.1109/ACCESS.2020.2971839 doi: 10.1109/ACCESS.2020.2971839
![]() |
[33] |
L. Wan, Q. Zhou, Exponential stability of neutral-type Cohen-Grossberg neural networks with multiple time-varying delays, IEEE Access, 9 (2021), 48914–48922. http://dx.doi.org/10.1109/ACCESS.2021.3068191 doi: 10.1109/ACCESS.2021.3068191
![]() |
[34] |
W. Yao, C. Wang, Y. Sun, C. Zhou, H. Lin, Exponential multistability of memristive Cohen-Grossberg neural networks with stochastic parameter perturbations, Appl. Math. Comput., 386 (2020), 125483. http://dx.doi.org/10.1016/j.amc.2020.125483 doi: 10.1016/j.amc.2020.125483
![]() |
[35] |
Q. Zhu, J. Cao, Robust exponential stability of Markovian jump impulsive stochastic Cohen-Grossberg neural networks with mixed time delays, IEEE T. Neural Networks, 21 (2010), 1314–1325. http://dx.doi.org/10.1109/TNN.2010.2054108 doi: 10.1109/TNN.2010.2054108
![]() |
[36] |
Y. Shen, J. Wang, Robustness of global exponential stability of nonlinear systems with random disturbances and time delays, IEEE T. Syst. Man Cy.-S., 46 (2015), 1157–1166. http://dx.doi.org/10.1109/TSMC.2015.2497208 doi: 10.1109/TSMC.2015.2497208
![]() |
[37] |
N. Ozcan, Stability analysis of Cohen–Grossberg neural networks of neutral-type: Multiple delays case, Neural Networks, 113 (2019), 20–27. http://dx.doi.org/10.1016/j.neunet.2019.01.017 doi: 10.1016/j.neunet.2019.01.017
![]() |
[38] | X. Mao, Stochastic differential equations and applications, 2 Eds., UK: Woodhead Publishing, 2008. |