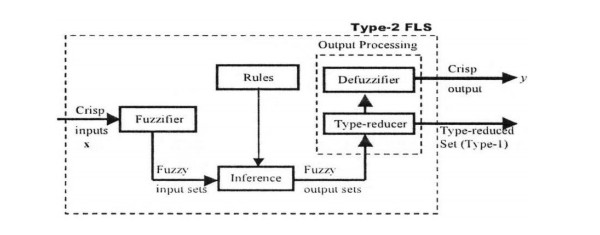
Citation: Xiangmei Li, Kamran, Absar Ul Haq, Xiujun Zhang. Numerical solution of the linear time fractional Klein-Gordon equation using transform based localized RBF method and quadrature[J]. AIMS Mathematics, 2020, 5(5): 5287-5308. doi: 10.3934/math.2020339
[1] | Yinyin Wu, Fanfan Chen, Qingchi Ma, Dingbian Qian . Subharmonic solutions for degenerate periodic systems of Lotka-Volterra type with impulsive effects. AIMS Mathematics, 2023, 8(9): 20080-20096. doi: 10.3934/math.20231023 |
[2] | Chunmei Song, Qihuai Liu, Guirong Jiang . Harmonic and subharmonic solutions of quadratic Liénard type systems with sublinearity. AIMS Mathematics, 2021, 6(11): 12913-12928. doi: 10.3934/math.2021747 |
[3] | Faizan A. Khan, Rohit K. Bhardwaj, Tirth Ram, Mohammed A. S. Tom . On approximate vector variational inequalities and vector optimization problem using convexificator. AIMS Mathematics, 2022, 7(10): 18870-18882. doi: 10.3934/math.20221039 |
[4] | Kirti Kaushik, Anoop Kumar, Aziz Khan, Thabet Abdeljawad . Existence of solutions by fixed point theorem of general delay fractional differential equation with p-Laplacian operator. AIMS Mathematics, 2023, 8(5): 10160-10176. doi: 10.3934/math.2023514 |
[5] | José L. Díaz . Non-Lipschitz heterogeneous reaction with a p-Laplacian operator. AIMS Mathematics, 2022, 7(3): 3395-3417. doi: 10.3934/math.2022189 |
[6] | Sabbavarapu Nageswara Rao, Abdullah Ali H. Ahmadini . Multiple positive solutions for system of mixed Hadamard fractional boundary value problems with (p1,p2)-Laplacian operator. AIMS Mathematics, 2023, 8(6): 14767-14791. doi: 10.3934/math.2023755 |
[7] | Hai-yun Deng, Jue-liang Zhou, Yu-bo He . Existence of periodic solutions for a class of (ϕ1,ϕ2)-Laplacian discrete Hamiltonian systems. AIMS Mathematics, 2023, 8(5): 10579-10595. doi: 10.3934/math.2023537 |
[8] | Qitong Ou, Huashui Zhan . The viscosity solutions of a nonlinear equation related to the p-Laplacian. AIMS Mathematics, 2017, 2(3): 400-421. doi: 10.3934/Math.2017.3.400 |
[9] | Shaomin Wang, Cunji Yang, Guozhi Cha . On the variational principle and applications for a class of damped vibration systems with a small forcing term. AIMS Mathematics, 2023, 8(9): 22162-22177. doi: 10.3934/math.20231129 |
[10] | Hany A. Hosham, Alaa A. Alzulaibani, Tarek Sellami, Khaled Sioud, Thoraya N. Alharthi . A class of discontinuous systems exhibit perturbed period doubling bifurcation. AIMS Mathematics, 2024, 9(9): 25098-25113. doi: 10.3934/math.20241223 |
As we all know, the membership grades of type-1 fuzzy sets (T1 FSs) are crisp numbers as 0 or 1, therefore, the membership functions (MFs) of T1 FSs are not inherently uncertain and they can only measure uncertainties in a limited scope. The membership grades of interval type-2 fuzzy sets (IT2 FSs) are intervals, so the IT2 FSs are capable of better model uncertainties [1,2]. In the past decades, great progresses have been made transiting from type-1 fuzzy logic systems (T1 FLSs) to interval type-2 fuzzy logic systems (IT2 FLSs). IT2 FLSs based on IT2 FSs can approximate real continuous functions defined on compact sets with arbitrary accuracy. Furthermore, IT2 FLSs have been successfully applied in many fields with uncertainty, nonlinearity and time-varying characteristics like autonomous mobile robots [3,4], intelligent controllers [5], financial systems [6], power systems [7,8], permanent magnetic drive [9,10,11], edge detection [12], medical systems [13] and hot strip mill [14,15] and so on.
Generally speaking, IT2 FLSs (see Figure 1) are composed of fuzzifier, rules, inference [16], type-reducer and defuzzifier. Among which, the block of type-reduction (TR) under the guidance of inference plays the central role, and its main function is to transform the IT2 FS to the T1 FS. Then the defuzzification changes the T1 FS to the crisp output. The operations in TR are the differences between T1 and IT2 FLSs, which makes the latter with more challenges.
Currently, the computationally intensive iterative Karnik-Mendel (KM) algorithms and enhanced Karnik-Mendel (EKM) algorithms [18,19,20] are the most popular approaches for performing the TR. These two types of algorithms have the advantages of preserving the uncertainties flow in the systems and converge in super-convergence speed. However, the initializations of KM and EKM algorithms are usually given by trial and error of extensive simulation experiments. This paper analyzes the initializations [21] of KM and EKM algorithms, and provides reasonable initialization EKM (RIEKM) algorithms for performing the centroid TR and defuzzification of IT2 FLSs. According the accurate benchmark CNT algorithms [22], the proposed RIEKM algorithms have smaller absolute errors and faster convergence speeds compared with the EKM algorithms.
The rest of this paper is organized as follows. Section 2 gives the background of IT2 FLSs. Section 3 provides the RIEKM algorithms, and how to adopt them to perform the centroid TR of IT2 FLSs. In Section 4, four computer simulation experiments are used to illustrate and analyze the performances of RIEKM algorithms. Finally the conclusions and expectations are given in Section 5.
The rules uncertainties of IT2 FLSs generate from numerical or language uncertainties in knowledge, while these uncertainties can be solved by T2 FSs. In fact, the concept of T2 FSs can be viewed as the extension of concept of T1 FSs.
Definition 1. A T2 FS ˜A can be characterized by its T2 MF μ˜A(x,u), i.e.,
˜A={(x,u),μ˜A(x,u)|∀x∈X,∀u∈[0,1]} | (1) |
in which the primary variable x∈X, and the secondary variable u∈[0,1], here equation (1) is usually referred to as the point-value expression, and whose compact form is as:
˜A=∫x∈X∫u∈Jx⊆[0,1]μ˜A(x,u)/(x,u). | (2) |
Definition 2. A vertical slice of μ˜A(x,u) is the secondary MF, i.e.,
μ˜A(x=x′,u)≡μ˜A(x′)=∫∀u∈Jx′fx′(u)/u. | (3) |
Definition 3. The two dimensional support of μ˜A(x,u) is called as the footprint of uncertainty (FOU), i.e.,
FOU(˜A)={(x,u)∈X×[0,1]|μ˜A(x,u)>0} | (4) |
here the upper and lower bounds of FOU(˜A) are referred to as the upper MF (UMF) and lower MF (LMF), respectively, i.e.,
UMF(˜A)=¯μ˜A(x)=¯FOU(˜A),LMF(˜A)=μ_˜A(x)=FOU(˜A)_. | (5) |
For any IT2 FSs, they can be completely characterized by their UMFs and LMFs. That’s because the secondary membership grades of IT2 FSs must be equal to 1, i.e., fx(u)≡1.
From the aspect of inference structure, IT2 FLSs can be divided into Mamdani type [7,11,14] and Takagi-Sugeno-Kang (TSK) type [10,15]. Without loss of generality, consider a Mamdani IT2 FLS with p inputs x1∈X1,x2∈X2,⋯,xp∈Xp and one output y∈Y, there are a total of M fuzzy rules, where the lth rule is of the form:
If x1 is ˜Fl1 and x2 is ˜Fl2 and … and xp is ˜Flp, then y is ˜Gl (l=1,Λ,M) | (6) |
In order to simplify the expressions, we model the input measurements as crisp sets, i.e., singleton fuzzifier is adopted. Then the process of fuzzy reasoning [7,16,23] is given as follows:
The fuzzy relation of each fuzzy rule is as:
˜Rl:˜Fl1טFl2⋯טFlp→˜Gl=˜Al→˜Gl | (7) |
whose MF is as:
μ˜Rl(x,y)=μ˜Al→˜Gl(x,y)=[∩pi=1μ˜Fli(x′i)]∩μ˜Gl(y) | (8) |
where the sign ∩ is the product or minimum t-norm [24].
The T2 output of each fuzzy rule is ˜Bl=Ax∘˜Rl, and whose MF is as:
μ˜Bl(y)=∪x∈X[μAx(x)∩μ˜Al→˜Gl(x,y)]=μ˜Gl(y)∩[∩pi=1μ˜Fli(x′i)]=μ˜Gl(y)∩Fl(x′) | (9) |
in which ∘ is the composition operation, and ∪ is the maximum t-norm. Fl(x′) is the defined firing interval, i.e.,
Fl:{Fl(x′)≡[f_l(x′),¯fl(x′)]f_l(x′)≡∩pi=1μ_˜Fli(x′i)¯fl(x′)≡∩pi=1¯μ˜Fli(x′i) | (10) |
Here the most popular centroid TR approach [21] is selected, i.e., the output IT2 FS ˜Bl of each rule (which generates by merging each fuzzy rule and its corresponding consequent IT2 FS) is described as:
{FOU(˜Bl)=[μ_˜Bl(y|x′),¯μ˜Bl(y|x′)]μ_˜Bl(y|x′)=f_l(x′)∗μ_˜Gl(y)¯μ˜Bl(y|x′)=¯fl(x′)∗¯μ˜Gl(y) | (11) |
where ∗ denotes the product or minimum t-norm.
Then agammaegating all the firing rule IT2 FS ˜Bl to obtain the final output ˜B, i.e.,
˜B:{FOU(˜B)=[μ_˜B(y|x′),¯μ˜B(y|x′)]μ_˜B(y|x′)=μ_˜B1(y|x′)∨⋯∨μ_˜BM(y|x′)¯μ˜B(y|x′)=¯μ˜B1(y|x′)∨⋯∨¯μ˜BM(y|x′) | (12) |
where ∨ represents the maximum operation. Then the type-reduced set YC can be obtained by computing the centroid C˜B of ˜B, i.e.,
YC=C˜B=1/[l˜B,r˜B] | (13) |
where l˜B and r˜B can be computed by KM types of algorithms as:
l˜B=mink=1,⋯,Nl˜B(k)=mink=1,⋯,Nk∑i=1yi¯μ˜B(yi)+N∑i=k+1yiμ_˜B(yi)k∑i=1¯μ˜B(yi)+N∑i=k+1μ_˜B(yi) | (14) |
r˜B=maxk=1,⋯,Nr˜B(k)=maxk=1,⋯,Nk∑i=1yiμ_˜B(yi)+N∑i=k+1yi¯μ˜B(yi)k∑i=1μ_˜B(yi)+N∑i=k+1¯μ˜B(yi) | (15) |
where N is the number of sampling of primary variable y, and k is the switch point.
Finally the crisp output is computed by taking the arithmetic average of l˜B and r˜B, i.e.,
y=(l˜B+r˜B)/2. | (16) |
First of all, we derive the theoretical interpretations of initialization of KM and EKM algorithms. Let a=y1<y2<⋯<yN=b, then the continuous KM and EKM algorithms compute as (see Eqs (14) and (15)):
l˜B=minξ∈[a,b]Fl(ξ)=minξ∈[a,b]∫ξay¯μ˜B(y)dy+∫bξyμ_˜B(y)dy∫ξa¯μ˜B(y)dy+∫bξμ_˜B(y)dy | (17) |
r˜B=minξ∈[a,b]Fr(ξ)=minξ∈[a,b]∫ξayμ_˜B(y)dy+∫bξy¯μ˜B(y)dy∫ξaμ_˜B(y)dy+∫bξ¯μ˜B(y)dy. | (18) |
In addition, the specific computation steps of CKM and CEKM algorithms are given in Tables 1 and 2. According to the notations Fl in (17) and Fr in (18), from Steps 2 and 4 in Table 1, we can find:
ξl=Fl(ξ′), and ξl=ξ′ | (19) |
ξr=Fr(ξ′), and ξr=ξ′. | (20) |
Step | CKM algorithm for l˜B, l˜B=min∀θ(y)∈[μ_˜B(y),¯μ˜B(y)]∫bayθ(y)dy∫baθ(y)dy |
1 | Let θ(y)=[μ_˜B(y)+¯μ˜B(y)]/2, and compute ξ′=∫bayθ(y)dy∫baθ(y)dy. |
2 | Set θ(y)=¯μ˜B(y) when y⩽ξ′, and θ(y)=μ_˜B(y) when y>ξ′, and compute ξl=∫bayθ(y)dy∫baθ(y)dy. |
3 | Check if |ξ′−ξl|⩽ε(ε is a given error bound), if yes, stop and set l˜B=ξl, if no, go to Step 4. |
4 | Set ξ′=ξl and go to Step 2. |
Step | CKM algorithm for r˜B, r˜B=max∀θ(y)∈[μ_˜B(y),¯μ˜B(y)]∫bayθ(y)dy∫baθ(y)dy |
1 | Let θ(y)=[μ_˜B(y)+¯μ˜B(y)]/2, and compute ξ′=∫bayθ(y)dy∫baθ(y)dy. |
2 | Set θ(y)=μ_˜B(y) when y⩽ξ′, and θ(y)=¯μ˜B(y) when y>ξ′, and compute ξr=∫bayθ(y)dy∫baθ(y)dy. |
3 | Check if |ξ′−ξr|⩽ε(ε is a given error bound), if yes, stop and set r˜B=ξr, if no, go to Step 4. |
4 | Set ξ′=ξl and go to Step 2. |
Step | CEKM algorithm for l˜B |
1 | Set c=a+(b−a)/2.4, and compute α=∫cay¯μ˜B(y)dy+∫bcyμ_˜B(y)dy, β=∫ca¯μ˜B(y)dy+∫bcμ_˜B(y)dy, c′=α/β. |
2 | Check if |c′−c|⩽ε, if yes, stop and set c′=l˜B, if no, go to Step 4. |
3 | Compute s=sign(c′−c), α′=α+s∫max(c,c′)min(c,c′)y[¯μ˜B(y)−μ_˜B(y)]dy, β′=β+s∫max(c,c′)min(c,c′)[¯μ˜B(y)−μ_˜B(y)]dy, c″. |
4 | Set c = c', c' = c'', \alpha = \alpha ', \beta = \beta ' and go to Step 2. |
Step | CEKM algorithm for {r_{\tilde B}} |
1 | Set c = a + (b - a)/1.7, and compute \alpha = \int_a^c {y{{\underline \mu }_{\tilde B}}} (y)dy + \int_c^b {y{{\overline \mu }_{\tilde B}}} (y)dy, \beta = \int_a^c {{{\underline \mu }_{\tilde B}}} (y)dy + \int_c^b {{{\overline \mu }_{\tilde B}}} (y)dy, c' = \alpha /\beta . |
2 | Check if |c' - c| \leqslant \varepsilon , if yes, stop and set c' = {r_{\tilde B}}, if no, go to Step 4. |
3 | Compute s = sign(c' - c), \alpha ' = \alpha - s\int_{\min (c, c')}^{\max (c, c')} {y[{{\overline \mu }_{\tilde B}}(y) - {{\underline \mu }_{\tilde B}}(y)]dy} , \beta ' = \beta - s\int_{\min (c, c')}^{\max (c, c')} {[{{\overline \mu }_{\tilde B}}(y) - {{\underline \mu }_{\tilde B}}(y)]dy} , c'' = \alpha '/\beta '. |
4 | Set c = c', c' = c'', \alpha = \alpha ', \beta = \beta ' and go to Step 2. |
When iterations terminate, {l_{\tilde B}} = {\xi _l} and {r_{\tilde B}} = {\xi _r}, therefore
{l_{\tilde B}} = {F_l}({l_{\tilde B}}), {r_{\tilde B}} = {F_r}({r_{\tilde B}}) | (21) |
here {l_{\tilde B}} and {r_{\tilde B}} are the fixed points of {F_l}(\xi) and {F_r}(\xi), respectively. In like manner, the relations of (21) still hold for the CEKM algorithms as in Table 2.
In order to set the initialization of the algorithms, let {\underline \mu _{\tilde B}}(y) = {\overline \mu _{\tilde B}}(y) = \theta (y) for all y \in [a, b], then \theta (y) = [{\underline \mu _{\tilde B}}(y) + {\overline \mu _{\tilde B}}(y)]/2. In this case, Eqs (17) and (18) become the same, so that,
{l_{\tilde B}} = {r_{\tilde B}} = \frac{{\int_a^b {y\theta (y)dy} }}{{\int_a^b {\theta (y)dy} }} = \frac{{\int_a^b {y[{{\underline \mu }_{\tilde B}}(y) + {{\overline \mu }_{\tilde B}}(y)]/2dy} }}{{\int_a^b {[{{\underline \mu }_{\tilde B}}(y) + {{\overline \mu }_{\tilde B}}(y)]/2dy} }} | (22) |
Equation (22) is the initialization approach of CKM algorithms given in Table 1, denoted here as {\xi ^{(1)}}, i.e.,
{\xi ^{(1)}} = \frac{{\int_a^b {y\theta (y)dy} }}{{\int_a^b {\theta (y)dy} }} | (23) |
in which
The specific computation steps of discrete KM and EKM algorithms are provided in Tables 3 and 4. And the discrete form of {\xi ^{(1)}} is established in Steps 1 and 2 in Table 3, i.e.,
{k^{(1)}} = \{ k|{y_k} \leqslant \frac{{\sum\limits_{i = 1}^N {{y_i}[{{\underline \mu }_{\tilde B}}({y_i}) + {{\overline \mu }_{\tilde B}}({y_i})]} }}{{\sum\limits_{i = 1}^N {[{{\underline \mu }_{\tilde B}}({y_i}) + {{\overline \mu }_{\tilde B}}({y_i})]} }} \lt {y_{k + 1}}, 1 \leqslant k \leqslant N - 1\} | (24) |
Step | KM algorithm for {l_{\tilde B}}, {l_{\tilde B}} = \mathop {\min }\limits_{\forall {\theta _i} \in [{{\underline \mu }_{\tilde B}}({y_i}), {{\overline \mu }_{\tilde B}}({y_i})]} (\sum\limits_{i = 1}^N {{y_i}{\theta _i}})/(\sum\limits_{i = 1}^N {{\theta _i}}) |
1 | Set \theta (i) = [{\underline \mu _{\tilde B}}({y_i}) + {\overline \mu _{\tilde B}}({y_i})]/2, i = 1, \cdots, N and compute c' = (\sum\limits_{i = 1}^N {{y_i}} {\theta _i})/(\sum\limits_{i = 1}^N {{\theta _i}}). |
2 | Find k'(1 \leqslant k' \leqslant N - 1) such that {y_{k'}} \leqslant c' < {y_{k' + 1}}. |
3 | Set {\theta _i} = {\overline \mu _{\tilde B}}({y_i}) when i \leqslant k', and {\theta _i} = {\underline \mu _{\tilde B}}({y_i}) when i > k', and compute {l_{\tilde B}}(k') = (\sum\limits_{i = 1}^N {{y_i}} {\theta _i})/(\sum\limits_{i = 1}^N {{\theta _i}}). |
4 | Check if {l_{\tilde B}}(k') = c', if yes, stop and set {l_{\tilde B}}(k') = {l_{\tilde B}} and k' = L, if no, go to Step 5. |
5 | Set c' = {l_{\tilde B}}(k') and go to Step 2. |
Step | KM algorithm for {r_{\tilde B}}, {r_{\tilde B}} = \mathop {\max }\limits_{\forall {\theta _i} \in [{{\underline \mu }_{\tilde B}}({y_i}), {{\overline \mu }_{\tilde B}}({y_i})]} (\sum\limits_{i = 1}^N {{y_i}{\theta _i}})/(\sum\limits_{i = 1}^N {{\theta _i}}) |
1 | Set \theta (i) = [{\underline \mu _{\tilde B}}({y_i}) + {\overline \mu _{\tilde B}}({y_i})]/2, i = 1, \cdots, N and compute c' = (\sum\limits_{i = 1}^N {{y_i}} {\theta _i})/(\sum\limits_{i = 1}^N {{\theta _i}}). |
2 | Find k'(1 \leqslant k' \leqslant N - 1) such that {y_{k'}} \leqslant c' < {y_{k' + 1}}. |
3 | Set {\theta _i} = {\underline \mu _{\tilde B}}({y_i}) when i \leqslant k', and {\theta _i} = {\overline \mu _{\tilde B}}({y_i}) when i > k', and compute {r_{\tilde B}}(k') = (\sum\limits_{i = 1}^N {{y_i}} {\theta _i})/(\sum\limits_{i = 1}^N {{\theta _i}}). |
4 | Check if {r_{\tilde B}}(k') = c', if yes, stop and set {r_{\tilde B}}(k') = {r_{\tilde B}} and k' = R, if no, go to Step 5. |
5 | Set c' = {r_{\tilde B}}(k') and go to Step 2. |
Step | EKM algorithm for {l_{\tilde B}} |
1 | Set k = [N/2.4] (the nearest integer to N/2.4) and compute\alpha = \sum\limits_{i = 1}^k {{y_i}} {\overline \mu _{\tilde B}}({y_i}) + \sum\limits_{i = k + 1}^N {{y_i}} {\underline \mu _{\tilde B}}({y_i}), \beta = \sum\limits_{i = 1}^k {{{\overline \mu }_{\tilde B}}({y_i})} + \sum\limits_{i = k + 1}^N {{{\underline \mu }_{\tilde B}}({y_i})} , c' = \alpha /\beta . |
2 | Find k'(1 \leqslant k' < N - 1) such that {y_{k'}} \leqslant c' < {y_{k' + 1}}. |
3 | Check if k' = k, if yes, stop and set c' = {l_{\tilde B}} and k = L, if no, go to Step 4. |
4 | Compute s = sign(k' - k), \alpha ' = \alpha + s\sum\limits_{i = \min (k, k') + 1}^{\max (k, k')} {{y_i}} [{\overline \mu _{\tilde B}}({y_i}) - {\underline \mu _{\tilde B}}({y_i})], \beta ' = \beta + s\sum\limits_{i = \min (k, k') + 1}^{\max (k, k')} {[{{\overline \mu }_{\tilde B}}({y_i}) - {{\underline \mu }_{\tilde B}}({y_i})]} , c'' = \alpha '/\beta '. |
5 | Set c' = c'', \alpha = \alpha ', \beta = \beta ', k = k' and go to Step 2. |
Step | EKM algorithm for {r_{\tilde B}} |
1 | Set k = [N/1.7] and compute \alpha = \sum\limits_{i = 1}^k {{y_i}} {\underline \mu _{\tilde B}}({y_i}) + \sum\limits_{i = k + 1}^N {{y_i}} {\overline \mu _{\tilde B}}({y_i}), \beta = \sum\limits_{i = 1}^k {{{\underline \mu }_{\tilde B}}({y_i})} + \sum\limits_{i = k + 1}^N {{{\overline \mu }_{\tilde B}}({y_i})} , c' = \alpha /\beta . |
2 | Find k'(1 \leqslant k' < N - 1) such that {y_{k'}} \leqslant c' < {y_{k' + 1}}. |
3 | Check if k' = k, if yes, stop and set c' = {r_{\tilde B}} and k = R, if no, go to Step 4. |
4 | Compute s = sign(k' - k), \alpha ' = \alpha - s\sum\limits_{i = \min (k, k') + 1}^{\max (k, k')} {{y_i}} [{\overline \mu _{\tilde B}}({y_i}) - {\underline \mu _{\tilde B}}({y_i})], \beta ' = \beta - s\sum\limits_{i = \min (k, k') + 1}^{\max (k, k')} {[{{\overline \mu }_{\tilde B}}({y_i}) - {{\underline \mu }_{\tilde B}}({y_i})]} , c'' = \alpha '/\beta '. |
5 | Set c' = c'', \alpha = \alpha ', \beta = \beta ', k = k' and go to Step 2. |
this KM initialization approach can provide excellent results as {\underline \mu _{\tilde B}}(y) and {\overline \mu _{\tilde B}}(y) are very close to each other, that’s because it becomes the exact optimal solution of (14) or (15) when {\underline \mu _{\tilde B}}(y) = {\overline \mu _{\tilde B}}(y).
For the EKM algorithms, the initialization approach is based on the difference between {\underline \mu _{\tilde B}}(y) and {\overline \mu _{\tilde B}}(y). Let’s make an assumption that
\rho = \frac{{\int_a^b {{{\overline \mu }_{\tilde B}}(y)dy} }}{{\int_a^b {{{\underline \mu }_{\tilde B}}(y)dy} }}. | (25) |
Attention that \rho \geqslant 1, because {\overline \mu _{\tilde B}}(y) \geqslant {\underline \mu _{\tilde B}}(y) for all y \in [a, b].
In order to initialize the EKM algorithms, suppose that both {\underline \mu _{\tilde B}}(y) and {\overline \mu _{\tilde B}}(y) be constants for y \in [a, b], i.e., {\underline \mu _{\tilde B}}(y) = n > 0, and {\overline \mu _{\tilde B}}(y) = \rho n > 0, according to Eq (14), so that
\begin{gathered} {F_l}(\xi ) = \frac{{\int_a^\xi {y{{\overline \mu }_{\tilde B}}(y)dy + \int_\xi ^b {y{{\underline \mu }_{\tilde B}}(y)dy} } }}{{\int_a^\xi {{{\overline \mu }_{\tilde B}}(y)dy + \int_\xi ^b {{{\underline \mu }_{\tilde B}}(y)dy} } }} = \frac{{\int_a^\xi {\rho nydy + \int_\xi ^b {nydy} } }}{{\int_a^\xi {\rho ndy + \int_\xi ^b {ndy} } }} \\ {\rm{ }} = \frac{{\int_a^\xi {\rho ydy + \int_\xi ^b {ydy} } }}{{\int_a^\xi {\rho dy + \int_\xi ^b {dy} } }} = \frac{{\rho ({\xi ^2} - {a^2}) + ({b^2} - {\xi ^2})}}{{2[\rho (\xi - a) + (b - \xi )]}} \\ \end{gathered} . | (26) |
Then find the derivative of {F_l}(\xi), so that
{F'_l}(\xi ) = \frac{{(\rho - 1)[\rho {{(\xi - a)}^2} - {{(b - \xi )}^2}]}}{{2{{[\rho (\xi - a) + (b - \xi )]}^2}}}. | (27) |
Setting {F'_l}(\xi) = 0, therefore
\frac{{{{(b - \xi )}^2}}}{{{{(\xi - a)}^2}}} = \rho, \frac{{b - \xi }}{{\xi - a}} = \sqrt \rho . | (28) |
Solving Eq (28) for \xi , we can obtain
{\xi _l} = \frac{{b + a\sqrt \rho }}{{1 + \sqrt \rho }} = a + \frac{{b - a}}{{1 + \sqrt \rho }}. | (29) |
For the Eq (27), {\xi _l} is the minimum value of {F_l}(\xi). Because {F'_l}(\xi) < 0 for \xi \in [a, {\xi _l}), and {F'_l}(\xi) > 0 for \xi \in ({\xi _l}, b]. Therefore, {l_{\tilde B}} = {F_l}({\xi _l}) = {\xi _l}.
In like manners, it can be obtained:
{F_r}(\xi ) = \frac{{({\xi ^2} - {a^2}) + \rho ({b^2} - {\xi ^2})}}{{2[(\xi - a) + \rho (b - \xi )]}} | (30) |
Therefore,
{F'_r}(\xi ) = - \frac{{(\rho - 1)[{{(\xi - a)}^2} - \rho {{(b - \xi )}^2}]}}{{2{{[(\xi - a) + \rho (b - \xi )]}^2}}}. | (31) |
Setting {F'_r}(\xi) = 0, it follows that:
\frac{{{{(b - \xi )}^2}}}{{{{(\xi - a)}^2}}} = \frac{1}{\rho }, \frac{{b - \xi }}{{\xi - a}} = \sqrt {1/\rho } . | (32) |
Solving Eq (30) for \xi , we can obtain
{\xi _r} = \frac{{b + a\sqrt {1/\rho } }}{{1 + \sqrt {1/\rho } }} = a + \frac{{b - a}}{{1 + \sqrt {1/\rho } }}. | (33) |
For the Eq (30), {\xi _r} is the maximum value of {F_r}(\xi). Because {F'_r}(\xi) > 0 for \xi \in [a, {\xi _r}), and {F'_r}(\xi) < 0 for \xi \in ({\xi _r}, b]. Therefore, {r_{\tilde B}} = {F_r}({\xi _r}) = {\xi _r}. Considering the Eqs (29) and (33) together, the new initialization approach for \xi can be denoted as {\xi ^{(2)}}, i.e.,
{\xi ^{(2)}} = \left\{ \begin{gathered} a + \frac{{b - a}}{{1 + \sqrt \rho }}{\rm{ for }}{l_{\tilde B}}, \\ a + \frac{{b - a}}{{1 + \sqrt {1/\rho } }}{\rm{ for }}{r_{\tilde B}}. \\ \end{gathered} \right. | (34) |
Here \rho \geqslant 1, therefore, {\xi ^{(2)}} \leqslant a + \frac{1}{2}(b - a) for {l_{\tilde B}}, and {\xi ^{(2)}} \geqslant a + \frac{1}{2}(b - a) for {r_{\tilde B}}.
Comparing the initializations of EKM algorithms in Table 4 and CEKM algorithms in Table 2, the discrete form of Eq (34) can be as:
{{k}^{(2)}}=\left\{ \begin{align} & [N/(1+\sqrt{\rho })]\ \ \text{for}\ \ {{l}_{{\tilde{B}}}}, \\ & [N/(1+\sqrt{1/\rho })]\ \ \text{for}\ \ {{r}_{{\tilde{B}}}}. \\ \end{align} \right. | (35) |
where N denotes the number of sampling of primary variable, and this \rho = [\sum\limits_{i = 1}^N {{{\overline \mu }_{\tilde B}}(y)}]/[\sum\limits_{i = 1}^N {{{\underline \mu }_{\tilde B}}(y)}].
Interestingly, when \rho = 2, it can be obtained that1 + \sqrt \rho = 1 + \sqrt 2 \approx 2.4, and 1 + \sqrt {1/\rho } = 1 + \sqrt {1/2} \approx 1.7, therefore, the equation (35) becomes:
{{k}^{(2)}}=\left\{ \begin{align} & [N/2.4]\ \ \text{for}\ \ {{l}_{{\tilde{B}}}}, \\ & [N/1.7]\ \ \text{for}\ \ {{r}_{{\tilde{B}}}}. \\ \end{align} \right. | (36) |
Equation (36) is just the initialization approach of EKM algorithms, which generates from empirical extensive simulation experiments. For the proposed reasonable initialization EKM (RIEKM) algorithms, we should determine each specific \rho for the corresponding simulations. Finally the specific computation steps of discrete RIEKM algorithms are provided in Table 5.
Step | RIEKM algorithm for {l_{\tilde B}} |
1 | Set k = [N/(1 + \sqrt \rho)] (\rho = [\sum\limits_{i = 1}^N {{{\overline \mu }_{\tilde B}}(y)]/[\sum\limits_{i = 1}^N {{{\underline \mu }_{\tilde B}}(y)]} } , which is depend on the specific examples) and compute\alpha = \sum\limits_{i = 1}^k {{y_i}} {\overline \mu _{\tilde B}}({y_i}) + \sum\limits_{i = k + 1}^N {{y_i}} {\underline \mu _{\tilde B}}({y_i}), \beta = \sum\limits_{i = 1}^k {{{\overline \mu }_{\tilde B}}({y_i})} + \sum\limits_{i = k + 1}^N {{{\underline \mu }_{\tilde B}}({y_i})} , c' = \alpha /\beta . |
2 | Find k'(1 \leqslant k' < N - 1) such that {y_{k'}} \leqslant c' < {y_{k' + 1}}. |
3 | Check if k' = k, if yes, stop and set c' = {l_{\tilde B}} and k = L, if no, go to Step 4. |
4 | Compute s = sign(k' - k), \alpha ' = \alpha + s\sum\limits_{i = \min (k, k') + 1}^{\max (k, k')} {{y_i}} [{\overline \mu _{\tilde B}}({y_i}) - {\underline \mu _{\tilde B}}({y_i})], \beta ' = \beta + s\sum\limits_{i = \min (k, k') + 1}^{\max (k, k')} {[{{\overline \mu }_{\tilde B}}({y_i}) - {{\underline \mu }_{\tilde B}}({y_i})]} , c'' = \alpha '/\beta '. |
5 | Set c' = c'', \alpha = \alpha ', \beta = \beta ', k = k' and return to Step 2. |
Step | RIEKM algorithm for {r_{\tilde B}} |
1 | Set k = [N/(1 + \sqrt {1/\rho })] and compute \alpha = \sum\limits_{i = 1}^k {{y_i}} {\underline \mu _{\tilde B}}({y_i}) + \sum\limits_{i = k + 1}^N {{y_i}} {\overline \mu _{\tilde B}}({y_i}), \beta = \sum\limits_{i = 1}^k {{{\underline \mu }_{\tilde B}}({y_i})} + \sum\limits_{i = k + 1}^N {{{\overline \mu }_{\tilde B}}({y_i})} , c' = \alpha /\beta |
2 | Find k'(1 \leqslant k' < N - 1) such that {y_{k'}} \leqslant c' < {y_{k' + 1}}. |
3 | Check if k' = k, if yes, stop and set c' = {r_{\tilde B}} and k = R, if no, go to Step 4. |
4 | Compute s = sign(k' - k), \alpha ' = \alpha - s\sum\limits_{i = \min (k, k') + 1}^{\max (k, k')} {{y_i}} [{\overline \mu _{\tilde B}}({y_i}) - {\underline \mu _{\tilde B}}({y_i})], \beta ' = \beta - s\sum\limits_{i = \min (k, k') + 1}^{\max (k, k')} {[{{\overline \mu }_{\tilde B}}({y_i}) - {{\underline \mu }_{\tilde B}}({y_i})]} , c'' = \alpha '/\beta '. |
5 | Set c' = c'', \alpha = \alpha ', \beta = \beta ', k = k' and return to Step 2. |
Four computer simulation examples are provided in this section to illustrate the performances RIEKM algorithms. Before performing the centroid TR, suppose that the FOU of centroid output IT2 FS be known by weighting and agammaegating all the fuzzy rules under the guidance of inference. Here the primary variable of centroid output IT2 FS is denoted by the letter x, x is uniformly sampled, and the number of sampling is chosen as N = 50:50:9000.
In the first example, the FOU is bounded by the piecewise linear functions [21,22,23,25,26]. In the second example, the FOU is bounded by both the Gaussian functions and piecewise linear functions [20,21,22,27,28,29,30,31]. In the third example, the FOU is bounded by the Gaussian functions [21,22,23,25,26]. In the last example, the FOU is a Gaussian IT2 MF with uncertainty standard deviation [20,21,22,27,28,29,30,31]. Then the Figure 2 and Table 6 provide the defined FOUs for four examples.
Num | Expressions |
1 | {\underline \mu _{{{\tilde A}_1}}}(x) = \max \{ \left[\begin{gathered} \frac{{x - 1}}{6}, {\rm{ 1}} \leqslant x \leqslant 4 \\ \frac{{7 - x}}{6}, {\rm{ }}4 < x \leqslant 7 \\ 0, {\rm{ otherwise}} \\ \end{gathered} \right], \left[\begin{gathered} \frac{{x - 3}}{6}, {\rm{ 3}} \leqslant x \leqslant 5 \\ \frac{{8 - x}}{9}, {\rm{ }}5 < x \leqslant 8 \\ 0, {\rm{ otherwise}} \\ \end{gathered} \right]\} {\overline \mu _{{{\tilde A}_1}}}(x) = \max \{ \left[\begin{gathered} \frac{{x - 1}}{2}, {\rm{ }}1 \leqslant x \leqslant 3 \\ \frac{{7 - x}}{4}, {\rm{ }}3 < x \leqslant 7 \\ 0, {\rm{ otherwise}} \\ \end{gathered} \right], \left[\begin{gathered} \frac{{x - 2}}{5}, {\rm{ 2}} \leqslant x \leqslant 6 \\ \frac{{16 - 2x}}{5}, {\rm{ }}6 < x \leqslant 8 \\ 0, {\rm{ otherwise}} \\ \end{gathered} \right]\} |
2 | {\underline \mu _{{{\tilde A}_2}}}(x) = \left\{ \begin{gathered} \frac{{0.6(x + 5)}}{{19}}, - 5 \leqslant x \leqslant 2.6 \\ \frac{{0.4(14 - x)}}{{19}}, 2.6 < x \leqslant 14 \\ \end{gathered} \right.{\overline \mu _{{{\tilde A}_2}}}(x) = \left\{ \begin{gathered} \exp [- \frac{1}{2}{(\frac{{x -2}}{5})^2}], - 5 \leqslant x \leqslant 7.185 \\ \exp [- \frac{1}{2}{(\frac{{x -9}}{{1.75}})^2}], 7.185 < x \leqslant 14 \\ \end{gathered} \right. |
3 | {\underline \mu _{{{\tilde A}_3}}}(x) = \max \{ 0.5\exp [- \frac{{{{(x -3)}^2}}}{2}], 0.4\exp [- \frac{{{{(x -6)}^2}}}{2}]\} {\overline \mu _{{{\tilde A}_3}}}(x) = \max \{ \exp [- 0.5\frac{{{{(x -3)}^2}}}{4}], 0.8\exp [- 0.5\frac{{{{(x -6)}^2}}}{4}]\} |
4 | {\underline \mu _{{{\tilde A}_4}}}(x) = \exp [- \frac{1}{2}{(\frac{{x -3}}{{0.25}})^2}], {\overline \mu _{{{\tilde A}_4}}}(x) = \exp [- \frac{1}{2}{(\frac{{x -3}}{{1.75}})^2}] |
In examples 1, 2 and 4, let the primary variable x \in [0, {\rm{ }}10]. In addition, let the primary variable x \in [- 5, {\rm{ }}14]in example 2. The accurate continuous Nie-Tan (CNT) algorithms [22,25] compute the centroid output of IT2 FS \tilde A as:
{y_{CNT}} = \frac{{\int_a^b {x[{{\underline \mu }_{\tilde A}}(x) + {{\overline \mu }_{\tilde A}}(x)]dx} }}{{\int_a^b {[{{\underline \mu }_{\tilde A}}(x) + {{\overline \mu }_{\tilde A}}(x)]dx} }}. | (37) |
Therefore, the CNT algorithms are firstly considered as the benchmark to compute the defuzzified values for four examples as: y_1^ * = 4.320794, y_2^ * = 3.714087, y_3^ * = 4.395260, and y_4^ * = 4.999999. Then graphs of defuzzified values computed by two types of discrete EKM algorithms are shown in Figure 3.
Furthermore, the absolute errors between the benchmark CNT algorithms and two types of discrete EKM algorithms are provided in Figure 4.
In order to further measure the performances of two types of algorithms, here we define the absolute errors sum \sum\limits_{i = 1}^{180} {|y_{CN{T_i}}^ * - {y_{EK{M_i}(RIEK{M_i})}}|} of defuzzified values for four examples, and they are shown in Table 7, in which the last column denotes the total average of absolute errors sum for the EKM algorithms and RIEKM algorithms.
Algorithms | EKM | RIEKM |
Example 1 | 0.932160 | 0.889015 |
Example 2 | 15.595056 | 14.933244 |
Example 3 | 3.577131 | 3.546304 |
Example 4 | 0.027159 | 0.000251 |
Total average | 5.032877 | 4.842204 |
Observing from the Figures 3, 4 and the Table 7, the following conclusions can be made:
1) As the number of sampling increases, these two types of discrete EKM algorithms all converge to certain values. The absolute errors of RIEKM algorithms are always less than the EKM algorithms in these four examples (as the red error curves of RIEKM algorithms are under the corresponding blue error curves of EKM algorithms).
2) In example 1, the RIEKM algorithms can obtain the result that is closed to the benchmark value with the number of sampling of 150. In other three examples, the RIEKM algorithms can almost get the optimal value just at the number of sampling of 50 (starting number of sampling).
3) As N = 50:50:9000, the RIEKM algorithms can obtain smaller absolute errors sum than the EKM algorithms in all four examples. In addition, the total average absolute errors sum of RIEKM algorithms is less than the EKM algorithms.
4) According to the items one to three, it is evidence that the proposed RIEKM algorithms can obtain better calculation accuracy than the EKM algorithms.
For the sake of applying these algorithms, next we study the specific unrepeatable computation times that depend on the hardware and software environments. Here the computer simulation platform is a dual CPU desktop with the Microsoft Windows XP operating system, E5300@2.6GHz and 2.00GB memory. All the programs are performed by the Matlab 2013a. Then Figure 5 gives the computation times for four examples, and the unit of time is the second (s).
For the sake of applying these algorithms, next we study the specific unrepeatable computation times that depend on the hardware and software environments. Here the computer simulation platform is a dual CPU desktop with the Microsoft Windows XP operating system, E5300@2.6GHz and 2.00GB memory. All the programs are performed by the Matlab 2013a. Then Figure 5 gives the computation times for four examples, and the unit of time is the second (s).
As shown in Figure 5, except for some fluctuations, the computation times of two types of discrete EKM algorithms emerge linear variation. In most number of sampling, the computation times of EKM algorithms are slightly better than the RIEKM algorithms. In other words, the convergence speeds of RIEKM algorithms are faster than the EKM algorithms in general. When the number of sampling is fixed, the computation times of two types of EKM algorithms are as: RIEKM > EKM for all four examples. This may because the initialization of RIEKM algorithms is more complex than the simple EKM algorithms.
Here we should point out that the paper focuses on the theoretical performances of RIEKM algorithms compared with the EKM algorithms. Four computer simulation examples show the advantages of RIEKM algorithms on high computation accuracy requirement. However, if the requirement of calculation accuracy is not very high, the simple EKM algorithms will complete with slightly faster computation speeds.
This paper gives the fuzzy reasoning process of IT2 FLSs, and discusses the initializations of EKM algorithms. And the reasonable initialization EKM (RIEKM) algorithms are provided to perform the centroid TR and defuzzification of IT2 FLSs. For computing the defuzzified values of IT2 FLSs, the proposed RIEKM algorithms can obtain better absolute errors and faster convergence speeds than the EKM algorithms.
Many interesting works still lie ahead, including extending and weighting the RIEKM algorithms to perform the centroid TR [17,27,28,31,32,40,41,42,43,44,49,50] of general type-2 fuzzy logic systems, and studying the center-of-sets TR of T2 FLSs [33], and the relations between discrete and continuous TR algorithms [18,21,28,29,30]. Future studies will also be focused on designing and applying IT2 or GT2 FLSs based on intelligent optimization algorithms [3,7,8,9,10,11,12,34,35,36,37,38,39,45,46,47,48,53] for forecasting, control [51,52] and identification.
The paper is sponsored by the National Natural Science Foundation of China (No. 61973146, No. 61773188, No. 61903167, No. 61803189), the Liaoning Province Natural Science Foundation Guidance Project (No. 20180550056), and Talent Fund Project of Liaoning University of Technology (No. xr2020002). The author is very grateful for professor Jerry Mendel, who has given the author some valuable suggestions.
The authors declare that they have no conflict of interest.
[1] |
F. Yousef, M. Alquran, I. Jaradat, et al. Ternary-fractional differential transform schema: Theory and application, Adv. Differ. Equ., 2019 (2019), 1-13. doi: 10.1186/s13662-018-1939-6
![]() |
[2] | S. Bhatter, A. Mathur, D. Kumar, et al. A new analysis of fractional Drinfeld-Sokolov-Wilson model with exponential memory, Physica A, 537 (2020), 122578. |
[3] |
A. Goswami, J. Singh, D. Kumar, et al. An efficient analytical approach for fractional equal width equations describing hydro-magnetic waves in cold plasma, Physica A, 524 (2019), 563-575. doi: 10.1016/j.physa.2019.04.058
![]() |
[4] | D. Kumar, F. Tchier, J. Singh, et al. An efficient computational technique for fractal vehicular traffic flow, Entropy, 20 (2018), 1-9. |
[5] |
D. Kumar, J. Singh, D. Baleanu, On the analysis of vibration equation involving a fractional derivative with Mittag-Leffler law, Math. Meth. Appl. Sci., 43 (2020), 443-457. doi: 10.1002/mma.5903
![]() |
[6] |
A. Yusuf, M. Inc, A. I. Aliyu, et al. Efficiency of the new fractional derivative with nonsingular Mittag-Leffler kernel to some nonlinear partial differential equations, Chaos Soliton Fract., 116 (2018), 220-226. doi: 10.1016/j.chaos.2018.09.036
![]() |
[7] |
D. Kumar, J. Singh, K. Tanwar, et al. A new fractional exothermic reactions model having constant heat source in porous media with power, exponential and Mittag-Leffler laws, Int. J. Heat Mass Tran., 138 (2019), 1222-1227. doi: 10.1016/j.ijheatmasstransfer.2019.04.094
![]() |
[8] | D. Kumar, J. Singh, S. D. Purohit, et al. A hybrid analytical algorithm for nonlinear fractional wave-like equations, Math. Model. Nat. Pheno., 14 (2019), 1-13. |
[9] | M. J. Ablowitz, M. A. Ablowitz, P. A. Clarkson, et al. Solitons, Nonlinear Evolution Equations and Inverse Scatterin, Cambridge university press, 1991. |
[10] | A. M. Yang, Y. Z. Zhang, C. Cattani, et al. Application of local fractional series expansion method to solve Klein-Gordon equations on Cantor sets, Abstr. Appl. Anal., 2014 (2014), 1-6. |
[11] |
A. M. Wazwaz, New travelling wave solutions to the Boussinesq and the Klein-Gordon equations, Commun. Nonlinear Sci., 13 (2008), 889-901. doi: 10.1016/j.cnsns.2006.08.005
![]() |
[12] | A. M. Wazwaz, The tanh and the sine-cosine methods for compact and noncompact solutions of the nonlinear Klein-Gordon equation, Appl. Math. Comput., 167 (2005), 1179-1195. |
[13] | A. S. V. R. Kanth, K. Aruna, Differential transform method for solving the linear and nonlinear Klein-Gordon equation, Comput. Phys. Commun., 180 (2018), 708-711. |
[14] |
K. Hosseini, P. Mayeli, R. Ansar, Modified Kudryashov method for solving the conformable timefractional Klein-Gordon equations with quadratic and cubic nonlinearities, Optik, 130 (2017), 737-742. doi: 10.1016/j.ijleo.2016.10.136
![]() |
[15] |
K. Hosseini, P. Mayeli, D. Kuma, New exact solutions of the coupled sine-Gordon equations in nonlinear optics using the modified Kudryashov method, J. Mod. Optic., 65 (2018), 361-364. doi: 10.1080/09500340.2017.1380857
![]() |
[16] |
K. Hosseini, P. Mayeli, R. Ansar, Bright and singular soliton solutions of the conformable timefractional Klein-Gordon equations with different nonlinearities, Wave. Random Complex, 28 (2018), 426-434. doi: 10.1080/17455030.2017.1362133
![]() |
[17] | K. Hosseini, Y. J. Xu, P. Mayeli, et al. A study on the conformable time-fractional Klein-Gordon equations with quadratic and cubic nonlinearitiesA study on the conformable time-fractional Klein-Gordon equations with quadratic and cubic nonlinearities, Optoelectron. Adv. Mat., 11 (2017), 423-429. |
[18] |
E. Yusufoğlu, The variational iteration method for studying the Klein-Gordon equation, Appl. Math. Lett., 21 (2008), 669-674. doi: 10.1016/j.aml.2007.07.023
![]() |
[19] | M. Alaroud, M. Al-Smadi, O. A. Arqub, et al. Numerical Solutions of Linear Time-fractional Klein-Gordon Equation by Using Power Series Approach, SSRN Electron. J., 2018 (2018), 1-6. |
[20] |
M. Kurulay, Solving the fractional nonlinear Klein-Gordon equation by means of the homotopy analysis method, Adv. Differ, Equ., 2012 (2012), 1-8. doi: 10.1186/1687-1847-2012-1
![]() |
[21] | K. A. Gepreel, M. S. Mohamed, Analytical approximate solution for nonlinear space-time fractional Klein-Gordon equation, Chinese Phys. B, 22 (2013), 010201. |
[22] |
A. K. Golmankhaneh, A. K. Golmankhaneh, D. Baleanu, On nonlinear fractional Klein-Gordon equation, Signal Process., 91 (2011), 446-451. doi: 10.1016/j.sigpro.2010.04.016
![]() |
[23] | E. A. B. Abdel-Salam, E. A. Yousif, Solution of nonlinear space-time fractional differential equations using the fractional Riccati expansion method, Math. Probl. Eng., 2013 (2013), 1-6. |
[24] |
D. Kumar, J. Singh, D. Baleanu, A hybrid computational approach for Klein-Gordon equations on Cantor sets, Nonlinear Dynam., 87 (2017), 511-517. doi: 10.1007/s11071-016-3057-x
![]() |
[25] |
A. Mohebbi, M. Abbaszadeh, M. Dehghan, High-order difference scheme for the solution of linear time fractional Klein-Gordon equations, Numer. Meth. Part. Differ. Equ., 30 (2014), 1234-1253. doi: 10.1002/num.21867
![]() |
[26] |
M. Dehghan, M. Abbaszadeh, A. Mohebbi, An implicit RBF meshless approach for solving the time fractional nonlinear sine-Gordon and Klein-Gordon equations, Eng. Anal. Bound. Elem., 50 (2015), 412-434. doi: 10.1016/j.enganabound.2014.09.008
![]() |
[27] |
M. M. Khader, An efficient approximate method for solving linear fractional Klein-Gordon equation based on the generalized Laguerre polynomials, Int. J. Comput. Math., 90 (2013), 1853-1864. doi: 10.1080/00207160.2013.764994
![]() |
[28] | G. Hariharan, Wavelet method for a class of fractional Klein-Gordon equations, J. Comput. Nonlinear Dynam., 8 (2013), 1-6. |
[29] | H. Singh, D. Kumar, J. Singh, et al. A reliable numerical algorithm for the fractional klein-gordon equation, Eng. Trans., 67 (2019), 21-34. |
[30] | S. Vong, Z. Wang, A compact difference scheme for a two dimensional fractional Klein-Gordon equation with Neumann boundary conditions, Entropy, 274 (2014), 268-282. |
[31] |
M. Dehghan, A. Shokri, Numerical solution of the nonlinear Klein-Gordon equation using radial basis functions, J. Comput. Appl. Math., 230 (2009), 400-410. doi: 10.1016/j.cam.2008.12.011
![]() |
[32] | M. Dehghan, A. Shokri, A method of solution for certain problems of transient heat conduction, AIAA J., 8 (1968), 2004-2009. |
[33] |
G. J. Moridis, D. L. Reddell, The Laplace transform finite difference method for simulation of flow through porous media, Water Resour. Res., 27 (1991), 1873-1884. doi: 10.1029/91WR01190
![]() |
[34] | G. J. Moridis, D. L. Reddell, The Laplace transform boundary element (LTBE) method for the solution of diffusion-type equations, In: Boundary Elements XIII, Springer, Dordrecht, 1991, 83-97. |
[35] | G. J. Moridis, D. L. Reddell, The Laplace transform finite element (LTFE) numerical method for the solution of the ground water equations, paper H22C-4, AGU 91 Spring Meeting, Baltimore, May 28-31, 1991, EOS Trans. of the AGU, 72 (1991), 17. |
[36] |
E. A. Sudicky, R. G. McLaren, The Laplace Transform Galerkin Technique for large-scale simulation of mass transport in discretely fractured porous formations, Water Resour. Res., 28 (1992), 499-514. doi: 10.1029/91WR02560
![]() |
[37] | G. J. Moridis, E. J. Kansa, The Laplace transform multiquadric method: A highly accurate scheme for the numerical solution of partial differentia, J. Appl. Sci. Comput., (1993), 55910181. |
[38] |
Q. T. L. Gia, W. Mclean, Solving the heat equation on the unit sphere via Laplace transforms and radial basis functions, Adv. Comput. Math., 40 (2014), 353-375. doi: 10.1007/s10444-013-9311-6
![]() |
[39] |
W. McLean, V. Thomee, Time discretization of an evolution equation via Laplace transforms, IMA J. Numer. Anal., 24 (2004), 439-463. doi: 10.1093/imanum/24.3.439
![]() |
[40] |
M. L. Fernandez, C. Palencia, On the numerical inversion of the Laplace transform of certain holomorphic mappings, Appl. Numer. Math., 51 (2004), 289-303. doi: 10.1016/j.apnum.2004.06.015
![]() |
[41] |
W. McLean, V. Thomee, Numerical solution via Laplace transforms of a fractional order evolution equation, J. Integral Equ. Appl., 22 (2010), 57-94. doi: 10.1216/JIE-2010-22-1-57
![]() |
[42] |
J. A. C. Weideman, L. N. Trefethen, Parabolic and Hyperbolic contours for computing the Bromwich integral, Math. Comput., 76 (2007), 1341-1356. doi: 10.1090/S0025-5718-07-01945-X
![]() |
[43] |
D. Sheen, I. H. Shaon, V. Thomee, A parallel method for time discretization of parabolic equations based on Laplace transformation and quadrature, IMA J. Numer.l Anal., 23 (2003), 269-299. doi: 10.1093/imanum/23.2.269
![]() |
[44] |
Z. J. Fu, W. Chen, H. T. Yang, Boundary particle method for Laplace transformed time fractional diffusion equations, J. Comput. Phys., 235 (2013), 52-66. doi: 10.1016/j.jcp.2012.10.018
![]() |
[45] |
M. Uddin, A. Ali, On the approximation of time-fractional telegraph equations using localized kernel-based method, Adv. Differ. Equ., 2018 (2018), 1-14. doi: 10.1186/s13662-017-1452-3
![]() |
[46] |
M. Uddin, A. Ali, A localized transform-based meshless method for solving time fractional wave- diffusion equation, Eng. Anal. Bound. Elem., 92 (2018), 108-113. doi: 10.1016/j.enganabound.2017.10.021
![]() |
[47] | K. B. Oldham, J. Spanier, The fractional calculus theory and applications of differentiation and integration to arbitrary order, Academic Press, New York, London, 1974. |
[48] |
M. Uddin, On the selection of a good value of shape parameter in solving time dependent partial differential equations using RBF approximation method, Appl. Math. Model., 38 (2014), 135-144. doi: 10.1016/j.apm.2013.05.060
![]() |
[49] | R. E. Carlson, T. A. Foley, The parameter r2 in multiquadric interpolation, Comput. Math. Appl., 21 ((1991), 29-42. |
[50] | R. E. Carlson, T. A. Foley, Near optimal parameter selection for multiquadric interpolation, Manuscript, Computer Science and Engineering Department, Arizona State University, Tempe., 20 (1994). |
[51] |
D. Kumar, F. Tchier, J. Singh, et al. Error estimates and condition numbers for radial basis function interpolation, Adv. Comput. Math., 3 (1995), 251-264. doi: 10.1007/BF02432002
![]() |
[52] | L. N. Trefethen, D. Bau, Numerical Linear Algebra, SIAM, 1997. |
1. | Liangying Miao, Man Xu, Zhiqian He, Existence and multiplicity of positive solutions for one-dimensional p -Laplacian problem with sign-changing weight, 2023, 31, 2688-1594, 3086, 10.3934/era.2023156 |
Step | CKM algorithm for {l_{\tilde B}}, {l_{\tilde B}} = \mathop {\min }\limits_{\forall \theta (y) \in [{{\underline \mu }_{\tilde B}}(y), {{\overline \mu }_{\tilde B}}(y)]} \frac{{\int_a^b {y\theta (y)dy} }}{{\int_a^b {\theta (y)dy} }} |
1 | Let \theta (y) = [{\underline \mu _{\tilde B}}(y) + {\overline \mu _{\tilde B}}(y)]/2, and compute \xi ' = \frac{{\int_a^b {y\theta (y)dy} }}{{\int_a^b {\theta (y)dy} }}. |
2 | Set \theta (y) = {\overline \mu _{\tilde B}}(y) when y \leqslant \xi ', and \theta (y) = {\underline \mu _{\tilde B}}(y) when y > \xi ', and compute {\xi _l} = \frac{{\int_a^b {y\theta (y)dy} }}{{\int_a^b {\theta (y)dy} }}. |
3 | Check if |\xi ' - {\xi _l}| \leqslant \varepsilon (\varepsilon is a given error bound), if yes, stop and set {l_{\tilde B}} = {\xi _l}, if no, go to Step 4. |
4 | Set \xi ' = {\xi _l} and go to Step 2. |
Step | CKM algorithm for {r_{\tilde B}}, {r_{\tilde B}} = \mathop {\max }\limits_{\forall \theta (y) \in [{{\underline \mu }_{\tilde B}}(y), {{\overline \mu }_{\tilde B}}(y)]} \frac{{\int_a^b {y\theta (y)dy} }}{{\int_a^b {\theta (y)dy} }} |
1 | Let \theta (y) = [{\underline \mu _{\tilde B}}(y) + {\overline \mu _{\tilde B}}(y)]/2, and compute \xi ' = \frac{{\int_a^b {y\theta (y)dy} }}{{\int_a^b {\theta (y)dy} }}. |
2 | Set \theta (y) = {\underline \mu _{\tilde B}}(y) when y \leqslant \xi ', and \theta (y) = {\overline \mu _{\tilde B}}(y) when y > \xi ', and compute {\xi _r} = \frac{{\int_a^b {y\theta (y)dy} }}{{\int_a^b {\theta (y)dy} }}. |
3 | Check if |\xi ' - {\xi _r}| \leqslant \varepsilon (\varepsilon is a given error bound), if yes, stop and set {r_{\tilde B}} = {\xi _r}, if no, go to Step 4. |
4 | Set \xi ' = {\xi _l} and go to Step 2. |
Step | CEKM algorithm for {l_{\tilde B}} |
1 | Set c = a + (b - a)/2.4, and compute \alpha = \int_a^c {y{{\overline \mu }_{\tilde B}}} (y)dy + \int_c^b {y{{\underline \mu }_{\tilde B}}} (y)dy, \beta = \int_a^c {{{\overline \mu }_{\tilde B}}} (y)dy + \int_c^b {{{\underline \mu }_{\tilde B}}} (y)dy, c' = \alpha /\beta . |
2 | Check if |c' - c| \leqslant \varepsilon , if yes, stop and set c' = {l_{\tilde B}}, if no, go to Step 4. |
3 | Compute s = sign(c' - c), \alpha ' = \alpha + s\int_{\min (c, c')}^{\max (c, c')} {y[{{\overline \mu }_{\tilde B}}(y) - {{\underline \mu }_{\tilde B}}(y)]dy} , \beta ' = \beta + s\int_{\min (c, c')}^{\max (c, c')} {[{{\overline \mu }_{\tilde B}}(y) - {{\underline \mu }_{\tilde B}}(y)]dy} , c'' = \alpha '/\beta '. |
4 | Set c = c', c' = c'', \alpha = \alpha ', \beta = \beta ' and go to Step 2. |
Step | CEKM algorithm for {r_{\tilde B}} |
1 | Set c = a + (b - a)/1.7, and compute \alpha = \int_a^c {y{{\underline \mu }_{\tilde B}}} (y)dy + \int_c^b {y{{\overline \mu }_{\tilde B}}} (y)dy, \beta = \int_a^c {{{\underline \mu }_{\tilde B}}} (y)dy + \int_c^b {{{\overline \mu }_{\tilde B}}} (y)dy, c' = \alpha /\beta . |
2 | Check if |c' - c| \leqslant \varepsilon , if yes, stop and set c' = {r_{\tilde B}}, if no, go to Step 4. |
3 | Compute s = sign(c' - c), \alpha ' = \alpha - s\int_{\min (c, c')}^{\max (c, c')} {y[{{\overline \mu }_{\tilde B}}(y) - {{\underline \mu }_{\tilde B}}(y)]dy} , \beta ' = \beta - s\int_{\min (c, c')}^{\max (c, c')} {[{{\overline \mu }_{\tilde B}}(y) - {{\underline \mu }_{\tilde B}}(y)]dy} , c'' = \alpha '/\beta '. |
4 | Set c = c', c' = c'', \alpha = \alpha ', \beta = \beta ' and go to Step 2. |
Step | KM algorithm for {l_{\tilde B}}, {l_{\tilde B}} = \mathop {\min }\limits_{\forall {\theta _i} \in [{{\underline \mu }_{\tilde B}}({y_i}), {{\overline \mu }_{\tilde B}}({y_i})]} (\sum\limits_{i = 1}^N {{y_i}{\theta _i}})/(\sum\limits_{i = 1}^N {{\theta _i}}) |
1 | Set \theta (i) = [{\underline \mu _{\tilde B}}({y_i}) + {\overline \mu _{\tilde B}}({y_i})]/2, i = 1, \cdots, N and compute c' = (\sum\limits_{i = 1}^N {{y_i}} {\theta _i})/(\sum\limits_{i = 1}^N {{\theta _i}}). |
2 | Find k'(1 \leqslant k' \leqslant N - 1) such that {y_{k'}} \leqslant c' < {y_{k' + 1}}. |
3 | Set {\theta _i} = {\overline \mu _{\tilde B}}({y_i}) when i \leqslant k', and {\theta _i} = {\underline \mu _{\tilde B}}({y_i}) when i > k', and compute {l_{\tilde B}}(k') = (\sum\limits_{i = 1}^N {{y_i}} {\theta _i})/(\sum\limits_{i = 1}^N {{\theta _i}}). |
4 | Check if {l_{\tilde B}}(k') = c', if yes, stop and set {l_{\tilde B}}(k') = {l_{\tilde B}} and k' = L, if no, go to Step 5. |
5 | Set c' = {l_{\tilde B}}(k') and go to Step 2. |
Step | KM algorithm for {r_{\tilde B}}, {r_{\tilde B}} = \mathop {\max }\limits_{\forall {\theta _i} \in [{{\underline \mu }_{\tilde B}}({y_i}), {{\overline \mu }_{\tilde B}}({y_i})]} (\sum\limits_{i = 1}^N {{y_i}{\theta _i}})/(\sum\limits_{i = 1}^N {{\theta _i}}) |
1 | Set \theta (i) = [{\underline \mu _{\tilde B}}({y_i}) + {\overline \mu _{\tilde B}}({y_i})]/2, i = 1, \cdots, N and compute c' = (\sum\limits_{i = 1}^N {{y_i}} {\theta _i})/(\sum\limits_{i = 1}^N {{\theta _i}}). |
2 | Find k'(1 \leqslant k' \leqslant N - 1) such that {y_{k'}} \leqslant c' < {y_{k' + 1}}. |
3 | Set {\theta _i} = {\underline \mu _{\tilde B}}({y_i}) when i \leqslant k', and {\theta _i} = {\overline \mu _{\tilde B}}({y_i}) when i > k', and compute {r_{\tilde B}}(k') = (\sum\limits_{i = 1}^N {{y_i}} {\theta _i})/(\sum\limits_{i = 1}^N {{\theta _i}}). |
4 | Check if {r_{\tilde B}}(k') = c', if yes, stop and set {r_{\tilde B}}(k') = {r_{\tilde B}} and k' = R, if no, go to Step 5. |
5 | Set c' = {r_{\tilde B}}(k') and go to Step 2. |
Step | EKM algorithm for {l_{\tilde B}} |
1 | Set k = [N/2.4] (the nearest integer to N/2.4) and compute\alpha = \sum\limits_{i = 1}^k {{y_i}} {\overline \mu _{\tilde B}}({y_i}) + \sum\limits_{i = k + 1}^N {{y_i}} {\underline \mu _{\tilde B}}({y_i}), \beta = \sum\limits_{i = 1}^k {{{\overline \mu }_{\tilde B}}({y_i})} + \sum\limits_{i = k + 1}^N {{{\underline \mu }_{\tilde B}}({y_i})} , c' = \alpha /\beta . |
2 | Find k'(1 \leqslant k' < N - 1) such that {y_{k'}} \leqslant c' < {y_{k' + 1}}. |
3 | Check if k' = k, if yes, stop and set c' = {l_{\tilde B}} and k = L, if no, go to Step 4. |
4 | Compute s = sign(k' - k), \alpha ' = \alpha + s\sum\limits_{i = \min (k, k') + 1}^{\max (k, k')} {{y_i}} [{\overline \mu _{\tilde B}}({y_i}) - {\underline \mu _{\tilde B}}({y_i})], \beta ' = \beta + s\sum\limits_{i = \min (k, k') + 1}^{\max (k, k')} {[{{\overline \mu }_{\tilde B}}({y_i}) - {{\underline \mu }_{\tilde B}}({y_i})]} , c'' = \alpha '/\beta '. |
5 | Set c' = c'', \alpha = \alpha ', \beta = \beta ', k = k' and go to Step 2. |
Step | EKM algorithm for {r_{\tilde B}} |
1 | Set k = [N/1.7] and compute \alpha = \sum\limits_{i = 1}^k {{y_i}} {\underline \mu _{\tilde B}}({y_i}) + \sum\limits_{i = k + 1}^N {{y_i}} {\overline \mu _{\tilde B}}({y_i}), \beta = \sum\limits_{i = 1}^k {{{\underline \mu }_{\tilde B}}({y_i})} + \sum\limits_{i = k + 1}^N {{{\overline \mu }_{\tilde B}}({y_i})} , c' = \alpha /\beta . |
2 | Find k'(1 \leqslant k' < N - 1) such that {y_{k'}} \leqslant c' < {y_{k' + 1}}. |
3 | Check if k' = k, if yes, stop and set c' = {r_{\tilde B}} and k = R, if no, go to Step 4. |
4 | Compute s = sign(k' - k), \alpha ' = \alpha - s\sum\limits_{i = \min (k, k') + 1}^{\max (k, k')} {{y_i}} [{\overline \mu _{\tilde B}}({y_i}) - {\underline \mu _{\tilde B}}({y_i})], \beta ' = \beta - s\sum\limits_{i = \min (k, k') + 1}^{\max (k, k')} {[{{\overline \mu }_{\tilde B}}({y_i}) - {{\underline \mu }_{\tilde B}}({y_i})]} , c'' = \alpha '/\beta '. |
5 | Set c' = c'', \alpha = \alpha ', \beta = \beta ', k = k' and go to Step 2. |
Step | RIEKM algorithm for {l_{\tilde B}} |
1 | Set k = [N/(1 + \sqrt \rho)] (\rho = [\sum\limits_{i = 1}^N {{{\overline \mu }_{\tilde B}}(y)]/[\sum\limits_{i = 1}^N {{{\underline \mu }_{\tilde B}}(y)]} } , which is depend on the specific examples) and compute\alpha = \sum\limits_{i = 1}^k {{y_i}} {\overline \mu _{\tilde B}}({y_i}) + \sum\limits_{i = k + 1}^N {{y_i}} {\underline \mu _{\tilde B}}({y_i}), \beta = \sum\limits_{i = 1}^k {{{\overline \mu }_{\tilde B}}({y_i})} + \sum\limits_{i = k + 1}^N {{{\underline \mu }_{\tilde B}}({y_i})} , c' = \alpha /\beta . |
2 | Find k'(1 \leqslant k' < N - 1) such that {y_{k'}} \leqslant c' < {y_{k' + 1}}. |
3 | Check if k' = k, if yes, stop and set c' = {l_{\tilde B}} and k = L, if no, go to Step 4. |
4 | Compute s = sign(k' - k), \alpha ' = \alpha + s\sum\limits_{i = \min (k, k') + 1}^{\max (k, k')} {{y_i}} [{\overline \mu _{\tilde B}}({y_i}) - {\underline \mu _{\tilde B}}({y_i})], \beta ' = \beta + s\sum\limits_{i = \min (k, k') + 1}^{\max (k, k')} {[{{\overline \mu }_{\tilde B}}({y_i}) - {{\underline \mu }_{\tilde B}}({y_i})]} , c'' = \alpha '/\beta '. |
5 | Set c' = c'', \alpha = \alpha ', \beta = \beta ', k = k' and return to Step 2. |
Step | RIEKM algorithm for {r_{\tilde B}} |
1 | Set k = [N/(1 + \sqrt {1/\rho })] and compute \alpha = \sum\limits_{i = 1}^k {{y_i}} {\underline \mu _{\tilde B}}({y_i}) + \sum\limits_{i = k + 1}^N {{y_i}} {\overline \mu _{\tilde B}}({y_i}), \beta = \sum\limits_{i = 1}^k {{{\underline \mu }_{\tilde B}}({y_i})} + \sum\limits_{i = k + 1}^N {{{\overline \mu }_{\tilde B}}({y_i})} , c' = \alpha /\beta |
2 | Find k'(1 \leqslant k' < N - 1) such that {y_{k'}} \leqslant c' < {y_{k' + 1}}. |
3 | Check if k' = k, if yes, stop and set c' = {r_{\tilde B}} and k = R, if no, go to Step 4. |
4 | Compute s = sign(k' - k), \alpha ' = \alpha - s\sum\limits_{i = \min (k, k') + 1}^{\max (k, k')} {{y_i}} [{\overline \mu _{\tilde B}}({y_i}) - {\underline \mu _{\tilde B}}({y_i})], \beta ' = \beta - s\sum\limits_{i = \min (k, k') + 1}^{\max (k, k')} {[{{\overline \mu }_{\tilde B}}({y_i}) - {{\underline \mu }_{\tilde B}}({y_i})]} , c'' = \alpha '/\beta '. |
5 | Set c' = c'', \alpha = \alpha ', \beta = \beta ', k = k' and return to Step 2. |
Num | Expressions |
1 | {\underline \mu _{{{\tilde A}_1}}}(x) = \max \{ \left[\begin{gathered} \frac{{x - 1}}{6}, {\rm{ 1}} \leqslant x \leqslant 4 \\ \frac{{7 - x}}{6}, {\rm{ }}4 < x \leqslant 7 \\ 0, {\rm{ otherwise}} \\ \end{gathered} \right], \left[\begin{gathered} \frac{{x - 3}}{6}, {\rm{ 3}} \leqslant x \leqslant 5 \\ \frac{{8 - x}}{9}, {\rm{ }}5 < x \leqslant 8 \\ 0, {\rm{ otherwise}} \\ \end{gathered} \right]\} {\overline \mu _{{{\tilde A}_1}}}(x) = \max \{ \left[\begin{gathered} \frac{{x - 1}}{2}, {\rm{ }}1 \leqslant x \leqslant 3 \\ \frac{{7 - x}}{4}, {\rm{ }}3 < x \leqslant 7 \\ 0, {\rm{ otherwise}} \\ \end{gathered} \right], \left[\begin{gathered} \frac{{x - 2}}{5}, {\rm{ 2}} \leqslant x \leqslant 6 \\ \frac{{16 - 2x}}{5}, {\rm{ }}6 < x \leqslant 8 \\ 0, {\rm{ otherwise}} \\ \end{gathered} \right]\} |
2 | {\underline \mu _{{{\tilde A}_2}}}(x) = \left\{ \begin{gathered} \frac{{0.6(x + 5)}}{{19}}, - 5 \leqslant x \leqslant 2.6 \\ \frac{{0.4(14 - x)}}{{19}}, 2.6 < x \leqslant 14 \\ \end{gathered} \right.{\overline \mu _{{{\tilde A}_2}}}(x) = \left\{ \begin{gathered} \exp [- \frac{1}{2}{(\frac{{x -2}}{5})^2}], - 5 \leqslant x \leqslant 7.185 \\ \exp [- \frac{1}{2}{(\frac{{x -9}}{{1.75}})^2}], 7.185 < x \leqslant 14 \\ \end{gathered} \right. |
3 | {\underline \mu _{{{\tilde A}_3}}}(x) = \max \{ 0.5\exp [- \frac{{{{(x -3)}^2}}}{2}], 0.4\exp [- \frac{{{{(x -6)}^2}}}{2}]\} {\overline \mu _{{{\tilde A}_3}}}(x) = \max \{ \exp [- 0.5\frac{{{{(x -3)}^2}}}{4}], 0.8\exp [- 0.5\frac{{{{(x -6)}^2}}}{4}]\} |
4 | {\underline \mu _{{{\tilde A}_4}}}(x) = \exp [- \frac{1}{2}{(\frac{{x -3}}{{0.25}})^2}], {\overline \mu _{{{\tilde A}_4}}}(x) = \exp [- \frac{1}{2}{(\frac{{x -3}}{{1.75}})^2}] |
Algorithms | EKM | RIEKM |
Example 1 | 0.932160 | 0.889015 |
Example 2 | 15.595056 | 14.933244 |
Example 3 | 3.577131 | 3.546304 |
Example 4 | 0.027159 | 0.000251 |
Total average | 5.032877 | 4.842204 |
Step | CKM algorithm for {l_{\tilde B}}, {l_{\tilde B}} = \mathop {\min }\limits_{\forall \theta (y) \in [{{\underline \mu }_{\tilde B}}(y), {{\overline \mu }_{\tilde B}}(y)]} \frac{{\int_a^b {y\theta (y)dy} }}{{\int_a^b {\theta (y)dy} }} |
1 | Let \theta (y) = [{\underline \mu _{\tilde B}}(y) + {\overline \mu _{\tilde B}}(y)]/2, and compute \xi ' = \frac{{\int_a^b {y\theta (y)dy} }}{{\int_a^b {\theta (y)dy} }}. |
2 | Set \theta (y) = {\overline \mu _{\tilde B}}(y) when y \leqslant \xi ', and \theta (y) = {\underline \mu _{\tilde B}}(y) when y > \xi ', and compute {\xi _l} = \frac{{\int_a^b {y\theta (y)dy} }}{{\int_a^b {\theta (y)dy} }}. |
3 | Check if |\xi ' - {\xi _l}| \leqslant \varepsilon (\varepsilon is a given error bound), if yes, stop and set {l_{\tilde B}} = {\xi _l}, if no, go to Step 4. |
4 | Set \xi ' = {\xi _l} and go to Step 2. |
Step | CKM algorithm for {r_{\tilde B}}, {r_{\tilde B}} = \mathop {\max }\limits_{\forall \theta (y) \in [{{\underline \mu }_{\tilde B}}(y), {{\overline \mu }_{\tilde B}}(y)]} \frac{{\int_a^b {y\theta (y)dy} }}{{\int_a^b {\theta (y)dy} }} |
1 | Let \theta (y) = [{\underline \mu _{\tilde B}}(y) + {\overline \mu _{\tilde B}}(y)]/2, and compute \xi ' = \frac{{\int_a^b {y\theta (y)dy} }}{{\int_a^b {\theta (y)dy} }}. |
2 | Set \theta (y) = {\underline \mu _{\tilde B}}(y) when y \leqslant \xi ', and \theta (y) = {\overline \mu _{\tilde B}}(y) when y > \xi ', and compute {\xi _r} = \frac{{\int_a^b {y\theta (y)dy} }}{{\int_a^b {\theta (y)dy} }}. |
3 | Check if |\xi ' - {\xi _r}| \leqslant \varepsilon (\varepsilon is a given error bound), if yes, stop and set {r_{\tilde B}} = {\xi _r}, if no, go to Step 4. |
4 | Set \xi ' = {\xi _l} and go to Step 2. |
Step | CEKM algorithm for {l_{\tilde B}} |
1 | Set c = a + (b - a)/2.4, and compute \alpha = \int_a^c {y{{\overline \mu }_{\tilde B}}} (y)dy + \int_c^b {y{{\underline \mu }_{\tilde B}}} (y)dy, \beta = \int_a^c {{{\overline \mu }_{\tilde B}}} (y)dy + \int_c^b {{{\underline \mu }_{\tilde B}}} (y)dy, c' = \alpha /\beta . |
2 | Check if |c' - c| \leqslant \varepsilon , if yes, stop and set c' = {l_{\tilde B}}, if no, go to Step 4. |
3 | Compute s = sign(c' - c), \alpha ' = \alpha + s\int_{\min (c, c')}^{\max (c, c')} {y[{{\overline \mu }_{\tilde B}}(y) - {{\underline \mu }_{\tilde B}}(y)]dy} , \beta ' = \beta + s\int_{\min (c, c')}^{\max (c, c')} {[{{\overline \mu }_{\tilde B}}(y) - {{\underline \mu }_{\tilde B}}(y)]dy} , c'' = \alpha '/\beta '. |
4 | Set c = c', c' = c'', \alpha = \alpha ', \beta = \beta ' and go to Step 2. |
Step | CEKM algorithm for {r_{\tilde B}} |
1 | Set c = a + (b - a)/1.7, and compute \alpha = \int_a^c {y{{\underline \mu }_{\tilde B}}} (y)dy + \int_c^b {y{{\overline \mu }_{\tilde B}}} (y)dy, \beta = \int_a^c {{{\underline \mu }_{\tilde B}}} (y)dy + \int_c^b {{{\overline \mu }_{\tilde B}}} (y)dy, c' = \alpha /\beta . |
2 | Check if |c' - c| \leqslant \varepsilon , if yes, stop and set c' = {r_{\tilde B}}, if no, go to Step 4. |
3 | Compute s = sign(c' - c), \alpha ' = \alpha - s\int_{\min (c, c')}^{\max (c, c')} {y[{{\overline \mu }_{\tilde B}}(y) - {{\underline \mu }_{\tilde B}}(y)]dy} , \beta ' = \beta - s\int_{\min (c, c')}^{\max (c, c')} {[{{\overline \mu }_{\tilde B}}(y) - {{\underline \mu }_{\tilde B}}(y)]dy} , c'' = \alpha '/\beta '. |
4 | Set c = c', c' = c'', \alpha = \alpha ', \beta = \beta ' and go to Step 2. |
Step | KM algorithm for {l_{\tilde B}}, {l_{\tilde B}} = \mathop {\min }\limits_{\forall {\theta _i} \in [{{\underline \mu }_{\tilde B}}({y_i}), {{\overline \mu }_{\tilde B}}({y_i})]} (\sum\limits_{i = 1}^N {{y_i}{\theta _i}})/(\sum\limits_{i = 1}^N {{\theta _i}}) |
1 | Set \theta (i) = [{\underline \mu _{\tilde B}}({y_i}) + {\overline \mu _{\tilde B}}({y_i})]/2, i = 1, \cdots, N and compute c' = (\sum\limits_{i = 1}^N {{y_i}} {\theta _i})/(\sum\limits_{i = 1}^N {{\theta _i}}). |
2 | Find k'(1 \leqslant k' \leqslant N - 1) such that {y_{k'}} \leqslant c' < {y_{k' + 1}}. |
3 | Set {\theta _i} = {\overline \mu _{\tilde B}}({y_i}) when i \leqslant k', and {\theta _i} = {\underline \mu _{\tilde B}}({y_i}) when i > k', and compute {l_{\tilde B}}(k') = (\sum\limits_{i = 1}^N {{y_i}} {\theta _i})/(\sum\limits_{i = 1}^N {{\theta _i}}). |
4 | Check if {l_{\tilde B}}(k') = c', if yes, stop and set {l_{\tilde B}}(k') = {l_{\tilde B}} and k' = L, if no, go to Step 5. |
5 | Set c' = {l_{\tilde B}}(k') and go to Step 2. |
Step | KM algorithm for {r_{\tilde B}}, {r_{\tilde B}} = \mathop {\max }\limits_{\forall {\theta _i} \in [{{\underline \mu }_{\tilde B}}({y_i}), {{\overline \mu }_{\tilde B}}({y_i})]} (\sum\limits_{i = 1}^N {{y_i}{\theta _i}})/(\sum\limits_{i = 1}^N {{\theta _i}}) |
1 | Set \theta (i) = [{\underline \mu _{\tilde B}}({y_i}) + {\overline \mu _{\tilde B}}({y_i})]/2, i = 1, \cdots, N and compute c' = (\sum\limits_{i = 1}^N {{y_i}} {\theta _i})/(\sum\limits_{i = 1}^N {{\theta _i}}). |
2 | Find k'(1 \leqslant k' \leqslant N - 1) such that {y_{k'}} \leqslant c' < {y_{k' + 1}}. |
3 | Set {\theta _i} = {\underline \mu _{\tilde B}}({y_i}) when i \leqslant k', and {\theta _i} = {\overline \mu _{\tilde B}}({y_i}) when i > k', and compute {r_{\tilde B}}(k') = (\sum\limits_{i = 1}^N {{y_i}} {\theta _i})/(\sum\limits_{i = 1}^N {{\theta _i}}). |
4 | Check if {r_{\tilde B}}(k') = c', if yes, stop and set {r_{\tilde B}}(k') = {r_{\tilde B}} and k' = R, if no, go to Step 5. |
5 | Set c' = {r_{\tilde B}}(k') and go to Step 2. |
Step | EKM algorithm for {l_{\tilde B}} |
1 | Set k = [N/2.4] (the nearest integer to N/2.4) and compute\alpha = \sum\limits_{i = 1}^k {{y_i}} {\overline \mu _{\tilde B}}({y_i}) + \sum\limits_{i = k + 1}^N {{y_i}} {\underline \mu _{\tilde B}}({y_i}), \beta = \sum\limits_{i = 1}^k {{{\overline \mu }_{\tilde B}}({y_i})} + \sum\limits_{i = k + 1}^N {{{\underline \mu }_{\tilde B}}({y_i})} , c' = \alpha /\beta . |
2 | Find k'(1 \leqslant k' < N - 1) such that {y_{k'}} \leqslant c' < {y_{k' + 1}}. |
3 | Check if k' = k, if yes, stop and set c' = {l_{\tilde B}} and k = L, if no, go to Step 4. |
4 | Compute s = sign(k' - k), \alpha ' = \alpha + s\sum\limits_{i = \min (k, k') + 1}^{\max (k, k')} {{y_i}} [{\overline \mu _{\tilde B}}({y_i}) - {\underline \mu _{\tilde B}}({y_i})], \beta ' = \beta + s\sum\limits_{i = \min (k, k') + 1}^{\max (k, k')} {[{{\overline \mu }_{\tilde B}}({y_i}) - {{\underline \mu }_{\tilde B}}({y_i})]} , c'' = \alpha '/\beta '. |
5 | Set c' = c'', \alpha = \alpha ', \beta = \beta ', k = k' and go to Step 2. |
Step | EKM algorithm for {r_{\tilde B}} |
1 | Set k = [N/1.7] and compute \alpha = \sum\limits_{i = 1}^k {{y_i}} {\underline \mu _{\tilde B}}({y_i}) + \sum\limits_{i = k + 1}^N {{y_i}} {\overline \mu _{\tilde B}}({y_i}), \beta = \sum\limits_{i = 1}^k {{{\underline \mu }_{\tilde B}}({y_i})} + \sum\limits_{i = k + 1}^N {{{\overline \mu }_{\tilde B}}({y_i})} , c' = \alpha /\beta . |
2 | Find k'(1 \leqslant k' < N - 1) such that {y_{k'}} \leqslant c' < {y_{k' + 1}}. |
3 | Check if k' = k, if yes, stop and set c' = {r_{\tilde B}} and k = R, if no, go to Step 4. |
4 | Compute s = sign(k' - k), \alpha ' = \alpha - s\sum\limits_{i = \min (k, k') + 1}^{\max (k, k')} {{y_i}} [{\overline \mu _{\tilde B}}({y_i}) - {\underline \mu _{\tilde B}}({y_i})], \beta ' = \beta - s\sum\limits_{i = \min (k, k') + 1}^{\max (k, k')} {[{{\overline \mu }_{\tilde B}}({y_i}) - {{\underline \mu }_{\tilde B}}({y_i})]} , c'' = \alpha '/\beta '. |
5 | Set c' = c'', \alpha = \alpha ', \beta = \beta ', k = k' and go to Step 2. |
Step | RIEKM algorithm for {l_{\tilde B}} |
1 | Set k = [N/(1 + \sqrt \rho)] (\rho = [\sum\limits_{i = 1}^N {{{\overline \mu }_{\tilde B}}(y)]/[\sum\limits_{i = 1}^N {{{\underline \mu }_{\tilde B}}(y)]} } , which is depend on the specific examples) and compute\alpha = \sum\limits_{i = 1}^k {{y_i}} {\overline \mu _{\tilde B}}({y_i}) + \sum\limits_{i = k + 1}^N {{y_i}} {\underline \mu _{\tilde B}}({y_i}), \beta = \sum\limits_{i = 1}^k {{{\overline \mu }_{\tilde B}}({y_i})} + \sum\limits_{i = k + 1}^N {{{\underline \mu }_{\tilde B}}({y_i})} , c' = \alpha /\beta . |
2 | Find k'(1 \leqslant k' < N - 1) such that {y_{k'}} \leqslant c' < {y_{k' + 1}}. |
3 | Check if k' = k, if yes, stop and set c' = {l_{\tilde B}} and k = L, if no, go to Step 4. |
4 | Compute s = sign(k' - k), \alpha ' = \alpha + s\sum\limits_{i = \min (k, k') + 1}^{\max (k, k')} {{y_i}} [{\overline \mu _{\tilde B}}({y_i}) - {\underline \mu _{\tilde B}}({y_i})], \beta ' = \beta + s\sum\limits_{i = \min (k, k') + 1}^{\max (k, k')} {[{{\overline \mu }_{\tilde B}}({y_i}) - {{\underline \mu }_{\tilde B}}({y_i})]} , c'' = \alpha '/\beta '. |
5 | Set c' = c'', \alpha = \alpha ', \beta = \beta ', k = k' and return to Step 2. |
Step | RIEKM algorithm for {r_{\tilde B}} |
1 | Set k = [N/(1 + \sqrt {1/\rho })] and compute \alpha = \sum\limits_{i = 1}^k {{y_i}} {\underline \mu _{\tilde B}}({y_i}) + \sum\limits_{i = k + 1}^N {{y_i}} {\overline \mu _{\tilde B}}({y_i}), \beta = \sum\limits_{i = 1}^k {{{\underline \mu }_{\tilde B}}({y_i})} + \sum\limits_{i = k + 1}^N {{{\overline \mu }_{\tilde B}}({y_i})} , c' = \alpha /\beta |
2 | Find k'(1 \leqslant k' < N - 1) such that {y_{k'}} \leqslant c' < {y_{k' + 1}}. |
3 | Check if k' = k, if yes, stop and set c' = {r_{\tilde B}} and k = R, if no, go to Step 4. |
4 | Compute s = sign(k' - k), \alpha ' = \alpha - s\sum\limits_{i = \min (k, k') + 1}^{\max (k, k')} {{y_i}} [{\overline \mu _{\tilde B}}({y_i}) - {\underline \mu _{\tilde B}}({y_i})], \beta ' = \beta - s\sum\limits_{i = \min (k, k') + 1}^{\max (k, k')} {[{{\overline \mu }_{\tilde B}}({y_i}) - {{\underline \mu }_{\tilde B}}({y_i})]} , c'' = \alpha '/\beta '. |
5 | Set c' = c'', \alpha = \alpha ', \beta = \beta ', k = k' and return to Step 2. |
Num | Expressions |
1 | {\underline \mu _{{{\tilde A}_1}}}(x) = \max \{ \left[\begin{gathered} \frac{{x - 1}}{6}, {\rm{ 1}} \leqslant x \leqslant 4 \\ \frac{{7 - x}}{6}, {\rm{ }}4 < x \leqslant 7 \\ 0, {\rm{ otherwise}} \\ \end{gathered} \right], \left[\begin{gathered} \frac{{x - 3}}{6}, {\rm{ 3}} \leqslant x \leqslant 5 \\ \frac{{8 - x}}{9}, {\rm{ }}5 < x \leqslant 8 \\ 0, {\rm{ otherwise}} \\ \end{gathered} \right]\} {\overline \mu _{{{\tilde A}_1}}}(x) = \max \{ \left[\begin{gathered} \frac{{x - 1}}{2}, {\rm{ }}1 \leqslant x \leqslant 3 \\ \frac{{7 - x}}{4}, {\rm{ }}3 < x \leqslant 7 \\ 0, {\rm{ otherwise}} \\ \end{gathered} \right], \left[\begin{gathered} \frac{{x - 2}}{5}, {\rm{ 2}} \leqslant x \leqslant 6 \\ \frac{{16 - 2x}}{5}, {\rm{ }}6 < x \leqslant 8 \\ 0, {\rm{ otherwise}} \\ \end{gathered} \right]\} |
2 | {\underline \mu _{{{\tilde A}_2}}}(x) = \left\{ \begin{gathered} \frac{{0.6(x + 5)}}{{19}}, - 5 \leqslant x \leqslant 2.6 \\ \frac{{0.4(14 - x)}}{{19}}, 2.6 < x \leqslant 14 \\ \end{gathered} \right.{\overline \mu _{{{\tilde A}_2}}}(x) = \left\{ \begin{gathered} \exp [- \frac{1}{2}{(\frac{{x -2}}{5})^2}], - 5 \leqslant x \leqslant 7.185 \\ \exp [- \frac{1}{2}{(\frac{{x -9}}{{1.75}})^2}], 7.185 < x \leqslant 14 \\ \end{gathered} \right. |
3 | {\underline \mu _{{{\tilde A}_3}}}(x) = \max \{ 0.5\exp [- \frac{{{{(x -3)}^2}}}{2}], 0.4\exp [- \frac{{{{(x -6)}^2}}}{2}]\} {\overline \mu _{{{\tilde A}_3}}}(x) = \max \{ \exp [- 0.5\frac{{{{(x -3)}^2}}}{4}], 0.8\exp [- 0.5\frac{{{{(x -6)}^2}}}{4}]\} |
4 | {\underline \mu _{{{\tilde A}_4}}}(x) = \exp [- \frac{1}{2}{(\frac{{x -3}}{{0.25}})^2}], {\overline \mu _{{{\tilde A}_4}}}(x) = \exp [- \frac{1}{2}{(\frac{{x -3}}{{1.75}})^2}] |
Algorithms | EKM | RIEKM |
Example 1 | 0.932160 | 0.889015 |
Example 2 | 15.595056 | 14.933244 |
Example 3 | 3.577131 | 3.546304 |
Example 4 | 0.027159 | 0.000251 |
Total average | 5.032877 | 4.842204 |