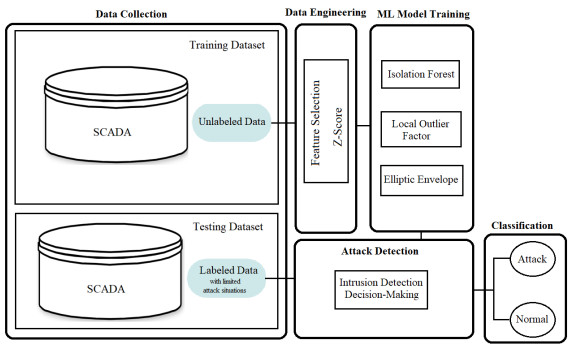
Anomaly detection strategies in industrial control systems mainly investigate the transmitting network traffic called network intrusion detection system. However, The measurement intrusion detection system inspects the sensors data integrated into the supervisory control and data acquisition center to find any abnormal behavior. An approach to detect anomalies in the measurement data is training supervised learning models that can learn to classify normal and abnormal data. But, a labeled dataset consisting of abnormal behavior, such as attacks, or malfunctions is extremely hard to achieve. Therefore, the unsupervised learning strategy that does not require labeled data for being trained can be helpful to tackle this problem. This study evaluates the performance of unsupervised learning strategies in anomaly detection using measurement data in control systems. The most accurate algorithms are selected to train unsupervised learning models, and the results show an accuracy of 98% in stealthy attack detection.
Citation: Sohrab Mokhtari, Kang K Yen. Measurement data intrusion detection in industrial control systems based on unsupervised learning[J]. Applied Computing and Intelligence, 2021, 1(1): 61-74. doi: 10.3934/aci.2021004
[1] | Yunxiang Yang, Hao Zhen, Yongcan Huang, Jidong J. Yang . Enhancing nighttime vehicle detection with day-to-night style transfer and labeling-free augmentation. Applied Computing and Intelligence, 2025, 5(1): 14-28. doi: 10.3934/aci.2025002 |
[2] | Mohammad Alkhalaf, Ping Yu, Jun Shen, Chao Deng . A review of the application of machine learning in adult obesity studies. Applied Computing and Intelligence, 2022, 2(1): 32-48. doi: 10.3934/aci.2022002 |
[3] | Lingju Kong, Ryan Z. Shi, Min Wang . A physics-informed neural network model for social media user growth. Applied Computing and Intelligence, 2024, 4(2): 195-208. doi: 10.3934/aci.2024012 |
[4] | Muhammad Amir Shafiq, Zhiling Long, Haibin Di, Ghassan AlRegib . A novel attention model for salient structure detection in seismic volumes. Applied Computing and Intelligence, 2021, 1(1): 31-45. doi: 10.3934/aci.2021002 |
[5] | Youliang Zhang, Guowu Yuan, Hao Wu, Hao Zhou . MAE-GAN: a self-supervised learning-based classification model for cigarette appearance defects. Applied Computing and Intelligence, 2024, 4(2): 253-268. doi: 10.3934/aci.2024015 |
[6] | Mohamed Wiem Mkaouer, Tarek Gaber, and Zaineb Chelly Dagdia . Effects of COVID-19 pandemic on computational intelligence and cybersecurity: Survey. Applied Computing and Intelligence, 2022, 2(2): 173-194. doi: 10.3934/aci.2022010 |
[7] | Yongcan Huang, Jidong J. Yang . Semi-supervised multiscale dual-encoding method for faulty traffic data detection. Applied Computing and Intelligence, 2022, 2(2): 99-114. doi: 10.3934/aci.2022006 |
[8] | Henri Tiittanen, Liisa Holm, Petri Törönen . Novel split quality measures for stratified multilabel cross validation with application to large and sparse gene ontology datasets. Applied Computing and Intelligence, 2022, 2(1): 49-62. doi: 10.3934/aci.2022003 |
[9] | Noah Gardner, John Paul Hellenbrand, Anthony Phan, Haige Zhu, Zhiling Long, Min Wang, Clint A. Penick, Chih-Cheng Hung . Investigation of ant cuticle dataset using image texture analysis. Applied Computing and Intelligence, 2022, 2(2): 133-151. doi: 10.3934/aci.2022008 |
[10] | Tinja Pitkämäki, Tapio Pahikkala, Ileana Montoya Perez, Parisa Movahedi, Valtteri Nieminen, Tom Southerington, Juho Vaiste, Mojtaba Jafaritadi, Muhammad Irfan Khan, Elina Kontio, Pertti Ranttila, Juha Pajula, Harri Pölönen, Aysen Degerli, Johan Plomp, Antti Airola . Finnish perspective on using synthetic health data to protect privacy: the PRIVASA project. Applied Computing and Intelligence, 2024, 4(2): 138-163. doi: 10.3934/aci.2024009 |
Anomaly detection strategies in industrial control systems mainly investigate the transmitting network traffic called network intrusion detection system. However, The measurement intrusion detection system inspects the sensors data integrated into the supervisory control and data acquisition center to find any abnormal behavior. An approach to detect anomalies in the measurement data is training supervised learning models that can learn to classify normal and abnormal data. But, a labeled dataset consisting of abnormal behavior, such as attacks, or malfunctions is extremely hard to achieve. Therefore, the unsupervised learning strategy that does not require labeled data for being trained can be helpful to tackle this problem. This study evaluates the performance of unsupervised learning strategies in anomaly detection using measurement data in control systems. The most accurate algorithms are selected to train unsupervised learning models, and the results show an accuracy of 98% in stealthy attack detection.
Industrial control system (ICS) is a general term used to describe various kinds of control systems and related instrumentation. ICSs consist of the equipment and networks employed to operate industrial processes such as manufacturing, energy, and transportation. These systems include different types of control systems, e.g., supervisory control and data acquisition (SCADA) and distributed control systems (DCS). In the last decades, the growing complexity of industrial and automated systems has made control designs more sophisticated. The entanglement of control designs increases the vulnerability of control systems against any kind of fault. For instance, it is possible that a very simple fault or a warning leads to a cascade failure in the system, and if it does not get detected and compensated, it will lead to a failure. Therefore, the reliability of control systems has attracted many research studies recently.
The reliability of a system can be increased by a multitude of methods such as fault-tolerant control (FTC) [1], network intrusion detection system (NIDS) [2], or measurement intrusion detection system (MIDS) [3]. Generally, in most of these methods, anomaly detection is considered the major step of the whole process. Anomaly detection means searching for any abnormal data in a system that does not conform to the expected behavior, and it can be caused by an attack or a malfunction [4]. Anomaly detection methods can be categorized into two main types: model-based and learning-based. The model-based anomaly detection uses the mathematical model of a control system to inspect the behavior and find any abnormal activity, while the learning-based methods, regardless of the mathematical model, just rely on the historical data obtained from the input/output of the system. The learning-based approach is handy when the system is complex, or the mathematical model is not available. This approach employs artificial intelligence (AI), particularly machine learning (ML), to find the patterns in the system's behavior and learns to distinguish outliers based on the achieved patterns.
The most significant step of building a machine learning model in the learning-based methods is to generate a reliable and accurate dataset. The dataset includes some inputs called features, and if possible, some outputs as the target data. The input data involve the historical log of the system consisting of sensors data or network traffic. Moreover, the output data could be any captured attacks or faults in the system. The supervised learning algorithms employ a targeted dataset to train an ML model for detecting anomalies [5]. It is clear that building a labeled/targeted dataset would not be easy due to the difficulties in collecting a large quantity of attacks data or faulty situations. One solution to tackle this problem is injecting attacks or faults into the system and collecting system data to generate a labeled dataset. But, in critical infrastructures, such as power plants, it is impossible to shut down the system to generate this kind of dataset. Even worse, injecting intentional attacks or faults into the system could cause irreversible damage to the system. In [3], we discussed the solution to address the dataset generation problem using hardware-in-the-loop (HIL) devices to simulate the system's critical parts. This device substitutes the sensitive components of the system to protect them from intentional attacks. For instance, the generator can be simulated using a HIL device to prevent damaging the dynamic components in a power plant. However, this technique is expensive and time-consuming. It can be sensible to be used when the system is critical infrastructure, and its reliability is extremely significant.
Nevertheless, it is possible to train an ML model using a dataset excluding any targeted data. This method is called an unsupervised learning approach for anomaly detection. The privilege of an unsupervised learning algorithm is the independence of this method from a targeted dataset. This means it is possible to train an ML model using the historical normal behavior data log. However, its accuracy in comparison with supervised learning is questionable and requires to be investigated. Many research studies focused on the unsupervised learning strategy to address the problem of anomaly detection in ICSs. Goh et al. leveraged unsupervised learning to detect faulty sensors in a water treatment system [6]. They employed recurrent neural networks (RNN) algorithm to investigate the behavior of incoming data and showed that their approach could effectively detect attacks. However, the accuracy of their method is not clarified in detail. In [7], Javaid et al. introduced a deep learning method to differentiate the abnormal and normal network traffic data. Their unsupervised algorithm consists of three layers of input, features, and output in which the output layer should classify the input data by adjusting the network parameters. Then, the trained model was used to classify the normal and abnormal data. However, their method could not reach satisfactory accuracy in anomaly detection. Choi et al. [8] developed a NIDS using an unsupervised learning autoencoders algorithm. Based on the main selected features, the original input data was reconstructed, and the normal and abnormal data were classified regarding the reconstruction error threshold. In their method, the network traffic data is used for generating the unlabeled dataset.
Most of the related studies used transmitting network traffic data packets, including internet protocols (IPs) and data packet headers, to investigate the behavior of the system. On the other hand, the measurement data obtained from the SCADA has the potential to be used as the input data for training an unsupervised model. In [3], we investigated the performance of supervised MIDS, and the effectiveness of this method was approved. But, the performance of unsupervised MIDS in fault detection requires to be evaluated. In this study, the unsupervised learning algorithms in detecting anomalies using the measurement data is investigated. Moreover, A real-world testbed dataset is used to approve the effectiveness of the proposed method.
Overall, this work has made the following contributions to the attack detection domain.
First, most existing studies detect anomalies from the network traffic as evidence of intrusion. Instead, we detect anomalies from the measurement data acquired from system sensors. This approach is not limited to detecting malicious activities from outside the system but is capable of detecting insider sabotage.
Second, existing methods are supervised and require a trained model with labeled ground truth data because the system log normally does not include a variety of abnormal behavior. Generating such labeled dataset, including attack cases, are potentially harmful to the system and can cause irreversible damages. We, therefore, focus on an unsupervised learning strategy that does not require labeled training data. Instead, unsupervised anomaly detection is applied for normal system logs without the need for labeled data.
Third, the proposed method is capable of detecting stealthy attacks. These cannot be detected from the network traffic directly because stealthy attacks imitate the normal behavior of the system. In this study, simulate the stealthy attacks and evaluate the performance of the selected anomaly detection approaches.
The remainder of this study is organized as follows. In Section 2, the methodology of building an unsupervised learning ML model is explained. Section 3 describes the testbed and dataset generation methods. The results of this study are shown in Section 4. Finally, Section 5 presents the conclusion and future work directions.
The unsupervised learning approach for anomaly detection in ICSs is illustrated in Fig. 1. This approach consists of four main steps: data collection, feature selection, model training, and decision-making. In the following, the concept of anomalies, feature selection in the training process, and ML models are described.
Anomaly detection in ICSs includes a wide range of research studies. It can be considered as intrusion detection, fault detection, or event detection in sensors network. Generally, any deviation from a standard behavior can be conformed as an anomaly. It can occur due to a multitude of reasons, such as insider sabotage, components malfunction, or a malicious activity known as a cyber-attack. The concept of an anomaly in this study refers to any fault or intrusion in the system. For instance, searching for a malfunction in system components is known as fault detection, while investigating any attempt to intrude the system's control center would be considered intrusion detection.
While the NIDS investigates the network traffic to find any anomaly in the transmitting data, the MIDS relies on the measurement data collected in the SCADA system obtained from the sensors. The MIDS approach could be more reliable if a stealthy intrusion is happening in the system. It means that an attacker is trying to intrude the system by imitating the normal behavior, aiming at changing set-points, manipulating the measured data, or turning off a vital component. For instance, in 2020, a state-of-the-art attack was detected at an international airport [9]. In this attack, a set of nonsensical commands were injected into the ICS communication network and forced the system to reboot or cause it to fail. Then, the attackers intruded into the system and spanned several days targeting different parts, such as the building management system (BMS). This kind of attack, known as a stealthy attack, can cause significant financial and reputational effects. For example, the BMS manages temperature, fire suppression system, lighting, and doors, which any malfunction would be disastrous. Therefore, if the NIDS cannot detect the attack, the IDS has to detect it using the MIDS. The privilege of MIDS is independent of data packages, and it only relies on the measurement data, making it extremely hard to be tricked by attackers. This study tries to evaluate the MIDS performance in stealthy attack detection.
In ICSs, the measured data from the system's sensors is collected in the SCADA and stored for a specific period of time. To build an unlabeled dataset, the measurement data from the SCADA can be employed. In an unsupervised approach, the dataset does not need to contain many quantities of abnormal behavior. Therefore, the problem of injecting attacks or faults into the system does not exist in an unsupervised learning anomaly detection approach. It should be mentioned that in real-life problems, the stored system log in the SCADA includes both normal and abnormal data. Usually, the portion of abnormal data would be far lower than the normal one, but the unsupervised learning mechanism does not require the abnormal data for the training process.
Nevertheless, in large-scale ICSs, a massive number of sensors are embedded in the system. Therefore, it is essential to select the optimum number of sensors (features) in the training process. Many techniques can be used to address the problem of feature selection, such as Pearson correlation [10], z-test [11], and principal component analysis (PCA) [12]. However, the feature selection method could be varied due to the individual features for pattern recognition. Furthermore, some feature data would show redundancy between sensor measurements. Hence, computation time could be increased because of having useless features, and the ML model classification suffers from overlapping data.
In methods like PCA employed for dimensionality reduction, the new generated features would not have the same characteristics as the original ones. Therefore, characterizing the reduced data space would not be simple. This could cause difficulties whenever required to find a specific faulty feature (sensor) in the system. Generally, unsupervised feature selection strategies that do not change the original feature data could be categorized as wrapper [13], filter [14], and embedded methods. The wrapper method requires a complex mathematical calculation leading to a very high computation cost. In the wrapper approach, for all possible feature combinations, a subset search is generated, and the selected learning method is used for each subset. Since a control system has a large number of sensors, wrappers are not a suitable strategy for feature selection due to the high computation cost.
In this study, the z-test method is employed to select the most significant features of the dataset. This method is a statistical test to figure out whether two population means are similar when the variances are calculated, and the dataset size is large. Moreover, Zscore represents the outcome result from the z-test. In this paper, the system logs are considered as the population of the z-test, and the limited sample of abnormal behaviors mentions the z-test samples. Also, the test would be a two-tailed z-test with a 0.01 level of significance and a corresponding critical value of 2.58. The Z critical value can be found regarding the significance level using Z-table. For more information associated with the critical value and Z-table, please visit [15]. In addition, in Fig. 2, a step-by-step flowchart of the method is described. If there is no difference between the two tails, the feature would be considered insignificant, and vice versa. The following equation shows the Zscore value computation:
Zscore=ˉx−μ√s2 | (1) |
where ˉx indicates the average of the abnormal samples, μ is the population mean, s2 represents the variance.
The reason for choosing z-test is two-folded: first, it is required to keep the sensors data untouched to find the origin of an occurred fault; therefore, PCA that changes the original features cannot be used; second, the z-test would perform more appropriately when the population of data is large. Hence, it is sensible to use this strategy in this specific problem, and the result implies the proper performance of this method.
Unsupervised anomaly detection based on the MIDS classifies the measurement data into two categories of outliers and inliers. Many unsupervised learning algorithms can be applied to the unlabeled dataset, but the most fitted ones are selected based on the obtained results in this study. Indeed, the unsupervised learning algorithm learns the system's normal behavior and tries to find any data that is behaving out of a defined normal zone.
The first algorithm is called isolation forest (IF), which identifies anomalies using isolation by recursively generating partitions on the data. This algorithm randomly selects an attribute, and then a split value for the attribute, between the min and max amounts allowed for the selected attribute. This algorithm performs quickly for high-dimensional datasets and could work even if no abnormal data is present in the training dataset. The key features of this algorithm can be described as follows [16]:
● It can build partial ML models and extract sub-sampling. This algorithm does not need to use a large part of the data for anomaly detection; therefore, a small number of samples would generate a better model due to the reduction of swamping and masking impacts.
● It does not measure any distance or density, which extremely reduces the calculation time.
● Its computation time increases linearly by growth in the complexity of the problem [17].
● It is capable of handling very large databases that have a wide range of irrelevant features.
These characteristics make the isolation forest algorithm a potential candidate for this study.
The second algorithm is known as the local outlier factor (LOF), which is based on the concept of local density. In this algorithm, the k-nearest neighbors are used to obtain the locality, and the distance between the data is employed for density estimation. Then, by comparing the local density of an outlier with the local densities of neighbor points, the similar density of the outlier can be identified and implies a substantially lower density than the neighbors [18]. The main step of this algorithm is computing the relative density as follow:
ζ=ρμ | (2) |
where ζ is the relative density of a data point X with k neighbors. ρ and μ are the density of X and the average density of neighbor data points. Moreover, the density of X can be calculated by inverting the mean distance of k nearest neighbors [19].
Finally, the third algorithm is the elliptic envelope (EE). This algorithm is based on the assumption that regular data comes from a specific distribution; then, it tries to find the shape of the data and defines the outliers that are far from the fitted shape [20], using the FAST-minimum covariance determinant (FAST-MCD) [21]. The FAST-MCD computes the mean and the covariance matrix of all features in non-intersecting subsets of data. Then, the algorithm calculates the Mahalanobis distance [22] to determine the outliers based on a specific threshold. The Mahalanobis distance, distMH, is defined as below:
distMH=√(A−mean)TCov−1(A−mean) | (3) |
where A is a multidimensional data vector, mean and Cov are the mean and covariance matrix, respectively. The distMH will be considered as Euclidean distance if the Cov is an identity matrix.
The accuracy of ML models can be calculated by division of the number of true predicted normal data and the total number of predicted values. The ML model accuracy calculation is shown as below:
ηn=TPTn | (4) |
where ηn shows the accuracy of the ML model in the prediction of the normal situation, TP indicates the true predicted number of normal situations, and Tn is the total number of a normal population. Moreover, the accuracy of the abnormal condition can be obtained from the following equation:
ηa=TNTa | (5) |
where ηa is the accuracy of the ML model in the prediction of abnormal situations, TN shows the true predicted number of abnormal situations, and Ta represents the total number of abnormal populations. Due to the low number of abnormal cases in the system log, using a confusion matrix [23] for performance evaluation would lead to an unfair comparison of anomaly detection. Generally, less than 4% of cases in a dataset of measured data are associated with abnormal data. Therefore, it would be better to calculate the accuracy of normal and abnormal detection separately. However, it is possible to leverage balancing methods, such as SMOTE [24], to level the dataset. This would increase the computation cost and time, which is not suggested in unsupervised learning approaches. The SMOTE method is used for the supervised learning approach in [3].
The performance of unsupervised learning MIDS is evaluated on a power system with two generators [25]. This system consists of four primary processes: turbine, water treatment, boiler, and HIL simulator. Two distinct datasets are exploited from the system, in which one of them is used for the training of the ML algorithms, and the other one is applied for the accuracy testing process. The testbed framework is shown in Fig. 3.
In this power system, the boiler process has four controllers loop, including level controller, pressure controller, temperature controller, flow-rate controller, and is in charge of heating the water pumped from the main tank. In the turbine process, a motor speed controller is responsible for rotating the tribune. Also, two controllers are embedded to manage the water level using a pump and a valve. Finally, the HIL simulates two power generators that supply the demand.
This study provides two different datasets, including the system log collected for several days of the system's performance. The dataset with the larger size is used for the training process, and the other one is used as the testing dataset. These datasets consist of 59 sensor measurements that are sampled every one second during four days of operation. The datasets are generated and published by the affiliated institute of ETRI on Feb. 2020, which are available in [26]. For more information about the dataset, please see [27,28].
The system log includes some incidents data in real-life problems, such as faults, warnings, or attacks. This kind of data will not be used for the training of the ML model but can be used for the testing process. In this paper, the attack data is used to test the accuracy of the ML algorithms.
The proposed unsupervised MIDS approach is implemented on the introduced testbed, and the ML model's accuracy in the detection of anomalies is evaluated. The training and testing process is performed by Python, and some unsupervised machine learning algorithms are examined to reach the most accurate ones. These algorithms include isolation forest, elliptic envelope, local outlier factor, support vector machine, and k-nearest neighbor. Moreover, the most significant features are selected using the z-test method, and 49 sensors are chosen among the 59 available sensors in the system. This selection is based on the distribution of the incoming data (features).
Fig. 4 shows the distribution of normal and attack situations for 4 sensors of the testbed. This figure is a density plot made by integrating continuous curves at each individual data point. It means that for every data point, a Gaussian kernel curve is assumed, then all of the curves are added together to make the final density plot. The x-axis shows the measured value of the sensor, while the y-axis is the probability density function for the kernel density estimation. The difference between probability and probability density is that the probability density indicates the probability per unit on the x-axis. If the area under the curve of an interval on the x-axis is calculated, then the actual probability of that interval could be found. Therefore, the y-axis can have values greater than one. The density plot can relatively compare normal and abnormal data for a specific sensor on the y-axis.
Nevertheless, as shown in Fig. 4, the distribution of data in normal and attack cases are similar. This could make anomaly detection harder due to the similarity of the data values in stealthy attacks. Sometimes, the NIDS is blind to the changes in the data values, and the system requires a complementary IDS for detection of these kinds of attacks, such as MIDS. When the measured data are employed as the features of an ML model, the feature selection strategy plays a critical role in the whole system's performance. The z-test method tries to find the most related features to the attack cases regarding the distributions of the measurement data for normal and attack situations. In the z-test feature selection method, the system log would be considered as the population of the test, and the abnormal data would be the sample data. This test has two hypotheses, including H0:null hypothesis and H1: alternative hypothesis. The H0 assumes that the feature is redundant, while the H1 suppose that the feature is significant and independent from other features. To evaluate these two claims, first, a significance level should be selected by the designer. Then, a critical value for the corresponding level of significance can be found using the z-table. Finally, the true claim would be figured out by calculating the Zscore and comparing it with the critical value of Z. If the Zscore is greater than the critical value, then the H1 hypothesis would be accepted and vice versa. This process is shown step by step in Fig. 2.
Then, three unsupervised learning algorithms performing more accurately in this problem are selected among tested algorithms and trained to predict the anomalies in the system. Isolation forest, local outlier factor, and elliptic envelope are the three employed algorithms in this study. There are two factors in the selection of these algorithms: first, the accuracy and the performance of them in the anomaly detection task; second, the time of computation, especially in the prediction process. The performance of these three models are compared in Table 1.
Isolation forest | Local outlier factor | Elliptic envelope | |
Normal detection accuracy | 0.7436 | 0.9624 | 0.8367 |
Abnormal detection accuracy | 0.9021 | 0.8801 | 0.9848 |
Training time [s] | 8.58 | 25.7 | 371.4 |
Prediction time [s] | 6.83 | 14.3 | 0.291 |
Based on the results in Table 1, the LOF algorithm has the most accurate rate of anomaly detection for the normal behavior of the system by the accuracy of 96%. While, in the anomaly detection task, EE is performing remarkably better than other algorithms by the rate of 98% accuracy in detecting attack situations. Although the IF requires the least time for the training process, its accuracy cannot compete with other algorithms. Furthermore, while the LOF has a lower computation time for the training process, it consumes a longer time in the prediction in comparison with the EE algorithm. In anomaly detection problems, the essential process is the prediction step. In this step, the high accuracy of the detection system is vital, but the required time for computing and classifying the incoming data has an extremely high impact on selecting an ML algorithm. On the other hand, the required training time for building an ML model does not significantly impact the selection of algorithms. This is due to the importance of the real-time performance of the detection system during operation. In other words, the training computation would be performed only once to build the ML model of the MIDS, and it could take several days in some vast datasets. But, the prediction process is constantly performed during the system operation in real-time. In this paper, the EE algorithm shows an astonishing performance in the pace of attack detection, while it requires a considerable amount of time for building the ML model. Also, this algorithm shows a reliable accuracy in attack detection with a rate of 98%, which is higher than the other mentioned algorithms. However, the LOF indicates better performance in normal cases detection compared to the EE algorithm.
In this paper, the unsupervised learning mechanism for anomaly detection in ICSs based on measurement data is studied, and machine learning models are trained based on unlabeled datasets to detect abnormal behaviors in the system. The unsupervised MIDS learns from historical data acquired from the SCADA system to find anomalies, especially stealthy attacks, in an ICS. Due to the difficulties of generating a labeled dataset for training a supervised machine learning model, the unsupervised learning approach has the privilege of performing with the regular system log without dependency on any targeted data in the dataset. Many unsupervised learning algorithms were examined to reach the most accurate ones in anomaly detection. In this paper, isolation forest, local outlier factor, and elliptic envelope are the most accurate unsupervised learning algorithms for anomaly detection of ICSs.
Moreover, to select the most significant features in the process of ML models training, the z-test mechanism is applied. This method uses the distribution of each feature and investigates its relationship with abnormal behaviors. Therefore, if a feature does not show a significant relationship with the abnormal behavior would be removed. In this way, the ML model would operate more accurately and faster. The results show that the elliptic envelope algorithm has the most accuracy in detecting anomalies, especially stealthy attacks, compared to other algorithms. Also, this algorithm consumes a significantly lower time to classify the normal/abnormal cases. However, it requires a long time to get trained, but the training process does not require to be fast because it would be performed once before embedding the unsupervised MIDS in the system for real-time operation.
In future studies, we would like to investigate the possibility of identifying the attack type based on measurement data. Since the fault/attack compensation in an ICS requires the best-fitted controller, it is essential to identify which kind of attack has happened in the system. Therefore, if the type of attack is known to the intrusion detection system, it can trigger the most fitted controller to compensate for the occurred fault and prevent a potential failure in the ICS.
All authors declare no conflicts of interest in this paper.
[1] |
A. Abbaspour, S. Mokhtari, A. Sargolzaei, K. K. Yen, A survey on active fault-tolerant control systems, Electronics, 9 (2021), 1513. doi: 10.3390/electronics9091513 doi: 10.3390/electronics9091513
![]() |
[2] |
N. Sultana, N. Chilamkurti, W. Peng, R. Alhadad, Survey on SDN based network intrusion detection system using machine learning approaches, Peer-to-Peer Netw. Appl., 12 (2019), 493–501. doi: 10.1007/s12083-017-0630-0 doi: 10.1007/s12083-017-0630-0
![]() |
[3] |
S. Mokhtari, A. Abbaspour, K. K. Yen, A. Sargolzaei, A machine learning approach for anomaly detection in industrial control systems based on measurement data, Electronics, 10 (2021), 407. doi: 10.3390/electronics10040407 doi: 10.3390/electronics10040407
![]() |
[4] |
V. Chandola, A. Banerjee, V. Kumar, Anomaly detection: A survey, ACM comput. surv. (CSUR), 41 (2009), 1–58. doi: 10.1145/1541880.1541882 doi: 10.1145/1541880.1541882
![]() |
[5] |
K. Paridari, N. O'Mahony, A. Mady, R. Chabukswar, M. Boubekeur, H. Sandberg, A framework for attack-resilient industrial control systems: Attack detection and controller reconfiguration, P. IEEE, 106 (2017), 113–128. doi: 10.1109/JPROC.2017.2725482 doi: 10.1109/JPROC.2017.2725482
![]() |
[6] |
J. Goh, S. Adepu, M. Tan, Z. S. Lee, Anomaly detection in cyber physical systems using recurrent neural networks, 2017 IEEE 18th International Symposium on High Assurance Systems Engineering (HASE), (2017), 140–145. doi: 10.1109/HASE.2017.36 doi: 10.1109/HASE.2017.36
![]() |
[7] |
A. Javaid, Q. Niyaz, W. Sun, M. Alam, A deep learning approach for network intrusion detection system, Eai Endorsed Transactions on Security and Safety, 3 (2016). doi: 10.4108/eai.3-12-2015.2262516 doi: 10.4108/eai.3-12-2015.2262516
![]() |
[8] |
H. Choi, M. Kim, G. Lee, W. Kim, Unsupervised learning approach for network intrusion detection system using autoencoders, The Journal of Supercomputing, 75 (2019), 5597–5621. doi: 10.1007/s11227-019-02805-w doi: 10.1007/s11227-019-02805-w
![]() |
[9] | M. Masson, Darktrace OT threat finds: Detecting an advanced ICS attack targeting an international airport, Aug., 2007. Available from: http://shorturl.at/nuJ19. |
[10] |
Y. Liu, Y. Mu, K. Chen, Y. Li, J. Guo, Daily activity feature selection in smart homes based on pearson correlation coefficient, Neural Process. Lett., (2020), 1–17. doi: 10.1007/s11063-019-10185-8 doi: 10.1007/s11063-019-10185-8
![]() |
[11] |
S. Bornelöv, J. Komorowski, Selection of significant features using Monte Carlo feature selection, Challenges in Computational Statistics and Data Mining, (2016), 25–38. doi: 10.1007/978-3-319-18781-5_2 doi: 10.1007/978-3-319-18781-5_2
![]() |
[12] |
Q. Guo, W. Wu, D. L. Massart, C. Boucon, S. De Jong, Feature selection in principal component analysis of analytical data, Chemometr. Intell. Lab., 61 (2002), 123–132. doi: 10.1016/S0169-7439(01)00203-9 doi: 10.1016/S0169-7439(01)00203-9
![]() |
[13] |
J. G. Dy, C. E. Brodley, Feature selection for unsupervised learning, J. Mach. Learn. Res., 5 (2004), 845–889. doi: 10.5555/1005332.1016787 doi: 10.5555/1005332.1016787
![]() |
[14] |
Y. Li, B. Lu, Z. Wu, Hierarchical fuzzy filter method for unsupervised feature selection, J. Intell. Fuzzy Syst., 18 (2007), 157–169. doi: 10.5555/1368376.1368381 doi: 10.5555/1368376.1368381
![]() |
[15] | Z table website provides all required information for using Z-test. Aug., 2021. Available from: https://www.ztable.net. |
[16] |
F. T. Liu, K. M. Ting, Z. Zhou, Isolation forest, 2008 eighth ieee international conference on data mining, (2008), 413–422. doi: 10.1109/ICDM.2008.17 doi: 10.1109/ICDM.2008.17
![]() |
[17] |
M. Wu, C. Jermaine, Outlier detection by sampling with accuracy guarantees, Proceedings of the 12th ACM SIGKDD international conference on Knowledge discovery and data mining, (2006), 767–772. doi: 10.1145/1150402.1150501 doi: 10.1021/ie302042c
![]() |
[18] |
H. Ma, Y. Hu, H. Shi, Fault detection and identification based on the neighborhood standardized local outlier factor method, Ind. Eng. Chem. Res., 52 (2013), 2389–2402. doi: 10.1021/ie302042c doi: 10.1021/ie302042c
![]() |
[19] |
V. Kotu, B. Deshpande, Chapter 13 - Anomaly Detection, Data Science (Second Edition), (2019), 447–465. doi: 10.1016/B978-0-12-814761-0.00013-7 doi: 10.1016/B978-0-12-814761-0.00013-7
![]() |
[20] |
M. Ashrafuzzaman, S. Das, A. A. Jillepalli, Y. Chakhchoukh, F. T. Sheldon, Elliptic Envelope Based Detection of Stealthy False Data Injection Attacks in Smart Grid Control Systems, 2020 IEEE Symposium Series on Computational Intelligence (SSCI), (2020), 1131–1137. doi: 10.1109/SSCI47803.2020.9308523 doi: 10.1109/SSCI47803.2020.9308523
![]() |
[21] |
P. J. Rousseeuw, K. V. Driessen, A fast algorithm for the minimum covariance determinant estimator, Technometrics, 41 (1999), 212–223. doi: 10.1080/00401706.1999.10485670 doi: 10.1080/00401706.1999.10485670
![]() |
[22] |
P. C. Mahalanobis, On the generalized distance in statistics, National Institute of Science of India, 1936. doi: 10.1007/s13171-019-00164-5 doi: 10.1007/s13171-019-00164-5
![]() |
[23] |
M. Sokolova, G. Lapalme, A systematic analysis of performance measures for classification tasks, Inform. process. manag., 45 (2009), 427–437. doi: 10.1016/j.ipm.2009.03.002 doi: 10.1016/j.ipm.2009.03.002
![]() |
[24] |
N. V. Chawla, K. W. Bowyer, L. O. Hall, W. P. Kegelmeyer, SMOTE: synthetic minority over-sampling technique, J. artif. intell. res., 16 (2002), 321–357. doi: 10.5555/1622407.1622416 doi: 10.5555/1622407.1622416
![]() |
[25] |
H. Shin, W. Lee, J. Yun, H. Kim, HAI 1.0: HIL-based Augmented ICS Security Dataset, 13th USENIX Workshop on Cyber Security Experimentation and Test (CSET 20), 2020. doi: 10.5555/3485754.3485755 doi: 10.5555/3485754.3485755
![]() |
[26] | S. Choi, HIL-based Augmented ICS (HAI) Security Dataset, The Affiliated Institute of ETRI, South Korea, 2020. Available from: https://github.com/icsdataset/hai. |
[27] |
H. Shin, W. Lee, J. Yun, H. Kim, Implementation of programmable CPS testbed for anomaly detection, 12th USENIX Workshop on Cyber Security Experimentation and Test (CSET 19), 2019. doi: 10.5555/3359012.3359014 doi: 10.5555/3359012.3359014
![]() |
[28] |
W. Hwang, J. Yun, J. Kim, H. Kim, Time-series aware precision and recall for anomaly detection: considering variety of detection result and addressing ambiguous labeling, Proceedings of the 28th ACM International Conference on Information and Knowledge Management, (2019), 2241–2244. doi: 10.1145/3357384.3358118 doi: 10.1145/3357384.3358118
![]() |
1. | Konrad Wolsing, Lea Thiemt, Christian van Sloun, Eric Wagner, Klaus Wehrle, Martin Henze, 2022, Chapter 28, 978-3-031-17142-0, 574, 10.1007/978-3-031-17143-7_28 | |
2. | Vincenzo Gattulli, Donato Impedovo, Lucia Sarcinella, 2024, Chapter 33, 978-3-031-45650-3, 330, 10.1007/978-3-031-45651-0_33 | |
3. | Sohrab Mokhtari, Kang K. Yen, False Data Injection Attack Detection, Isolation, and Identification in Industrial Control Systems Based on Machine Learning: Application in Load Frequency Control, 2024, 13, 2079-9292, 3239, 10.3390/electronics13163239 | |
4. | Pasi Fränti, Jun Shen, Chih-Cheng Hung, Applied Computing and Intelligence: A new open access journal, 2024, 4, 2771-392X, 19, 10.3934/aci.2024002 |
Isolation forest | Local outlier factor | Elliptic envelope | |
Normal detection accuracy | 0.7436 | 0.9624 | 0.8367 |
Abnormal detection accuracy | 0.9021 | 0.8801 | 0.9848 |
Training time [s] | 8.58 | 25.7 | 371.4 |
Prediction time [s] | 6.83 | 14.3 | 0.291 |