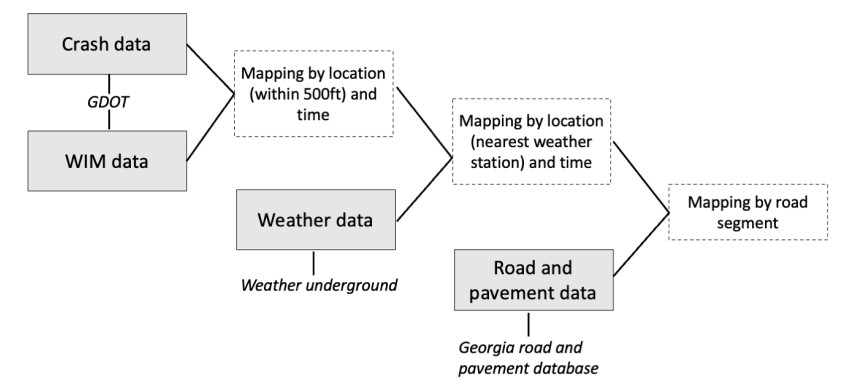
This paper presents a comprehensive study aimed at understanding the dynamics of single-vehicle and multi-vehicle crashes through a binary classification approach. By harnessing high-resolution, multi-source data, including high-resolution traffic profile data captured by weigh-in-motion stations, weather conditions, roadway attributes, and pavement properties, we delved into distinctive characteristics of the two crash types. Particularly, a meticulous data fusion approach was applied to integrate the diverse data sources, enabling a holistic investigation of influential factors. Framing it as a classification task, key factors differentiating between single-vehicle and multi-vehicle crashes were identified. The results of the study provide valuable insights into the underlying mechanisms of the two distinct crash types, supporting the development of targeted safety measures.
Citation: Hao Zhen, Oscar Lares, Jeffrey Cooper Fortson, Jidong J. Yang, Wei Li, Eric Conklin. Unraveling the dynamics of single-vehicle versus multi-vehicle crashes: a comparative analysis through binary classification[J]. Applied Computing and Intelligence, 2024, 4(2): 349-369. doi: 10.3934/aci.2024020
[1] | Oscar Lares, Hao Zhen, Jidong J. Yang . Feature group tabular transformer: a novel approach to traffic crash modeling and causality analysis. Applied Computing and Intelligence, 2025, 5(1): 29-56. doi: 10.3934/aci.2025003 |
[2] | Mark A. Seferian, Jidong J. Yang . Enhancing autonomous vehicle safety in rain: a data centric approach for clear vision. Applied Computing and Intelligence, 2024, 4(2): 282-299. doi: 10.3934/aci.2024017 |
[3] | Yunxiang Yang, Hao Zhen, Yongcan Huang, Jidong J. Yang . Enhancing nighttime vehicle detection with day-to-night style transfer and labeling-free augmentation. Applied Computing and Intelligence, 2025, 5(1): 14-28. doi: 10.3934/aci.2025002 |
[4] | Yongcan Huang, Jidong J. Yang . Semi-supervised multiscale dual-encoding method for faulty traffic data detection. Applied Computing and Intelligence, 2022, 2(2): 99-114. doi: 10.3934/aci.2022006 |
[5] | Francis Nweke, Abm Adnan Azmee, Md Abdullah Al Hafiz Khan, Yong Pei, Dominic Thomas, Monica Nandan . A transformer-driven framework for multi-label behavioral health classification in police narratives. Applied Computing and Intelligence, 2024, 4(2): 234-252. doi: 10.3934/aci.2024014 |
[6] | Sheyda Ghanbaralizadeh Bahnemiri, Mykola Pnomarenko, Karen Eguiazarian . Iterative transfer learning with large unlabeled datasets for no-reference image quality assessment. Applied Computing and Intelligence, 2024, 4(2): 107-124. doi: 10.3934/aci.2024007 |
[7] | Marko Niemelä, Mikaela von Bonsdorff, Sami Äyrämö, Tommi Kärkkäinen . Classification of dementia from spoken speech using feature selection and the bag of acoustic words model. Applied Computing and Intelligence, 2024, 4(1): 45-65. doi: 10.3934/aci.2024004 |
[8] | Youliang Zhang, Guowu Yuan, Hao Wu, Hao Zhou . MAE-GAN: a self-supervised learning-based classification model for cigarette appearance defects. Applied Computing and Intelligence, 2024, 4(2): 253-268. doi: 10.3934/aci.2024015 |
[9] | Hao Zhen, Yucheng Shi, Jidong J. Yang, Javad Mohammadpour Vehni . Co-supervised learning paradigm with conditional generative adversarial networks for sample-efficient classification. Applied Computing and Intelligence, 2023, 3(1): 13-26. doi: 10.3934/aci.2023002 |
[10] | Muhammad Amir Shafiq, Zhiling Long, Haibin Di, Ghassan AlRegib . A novel attention model for salient structure detection in seismic volumes. Applied Computing and Intelligence, 2021, 1(1): 31-45. doi: 10.3934/aci.2021002 |
This paper presents a comprehensive study aimed at understanding the dynamics of single-vehicle and multi-vehicle crashes through a binary classification approach. By harnessing high-resolution, multi-source data, including high-resolution traffic profile data captured by weigh-in-motion stations, weather conditions, roadway attributes, and pavement properties, we delved into distinctive characteristics of the two crash types. Particularly, a meticulous data fusion approach was applied to integrate the diverse data sources, enabling a holistic investigation of influential factors. Framing it as a classification task, key factors differentiating between single-vehicle and multi-vehicle crashes were identified. The results of the study provide valuable insights into the underlying mechanisms of the two distinct crash types, supporting the development of targeted safety measures.
The automobile has firmly established itself as the beloved and dominant mode of travel in the United States. With a staggering registration of approximately 283 million vehicles in 2022 [2], it is evident that cars play an integral role in people's daily life. However, this reliance on automobiles comes with a grave cost as evidenced by the tragic toll of motor vehicle crashes. According to the National Highway Traffic Safety Administration (NHTSA), an estimated 40,990 people died in motor vehicle traffic crashes in 2023 [14]. The urgency to investigate the patterns and root causes of these crashes cannot be overstated, as it is crucial for formulating and implementing effective safety measures and policies. By understanding the intricate dynamics of vehicle accidents, we not only safeguard lives but also pave the way for a safer and more sustainable transportation, benefiting the society as a whole.
Crash analysis, a cornerstone of traffic safety research, has examined contributing factors influencing crash outcomes, such as crash event features, roadway features, weather conditions, and traffic features. Among these, weather conditions and traffic-related features have emerged as critical areas of study, given their significant impact on crash likelihood and outcomes. For weather-related features, variables such as precipitation, visibility, and temperature have been shown to impact driver behavior and roadway conditions, thereby affecting the likelihood and severity of crashes [3,7,15]. Precipitation, in particular, influences crash risk in two different ways: it alters driver behavior and encourages cautious driving due to reduced visibility and wet roads while simultaneously decreasing road friction, thereby increasing the likelihood of skidding and necessitating longer stopping distances [23].
Moreover, traffic characteristics, especially truck-related traffic features, have been linked to increased crash severity due to the greater impact forces involved in truck-related collisions [20,22]. Research has also demonstrated that integrating traffic-related features such as speed and traffic flow, along with categorical weather data, can improve crash risk prediction modeling; also, the impact of traffic features on crash outcomes varies across different weather conditions [8,21]. Researchers also found that truck traffic significantly impacts crash risk and severity [4,5]. Due to their greater weights compared to passenger vehicles, crashes involving trucks tend to result in more severe outcomes because of higher impact forces and momentum involved. Weigh-in-motion (WIM) data, which includes truck volumes, vehicle weights, and the ratio of truck traffic to passenger cars, has been utilized to analyze the influence of truck traffic on crashes. Findings from these studies indicate that higher truck ratios and greater mean vehicle weights are correlated with increased injury and fatality risks in traffic crashes [20,22].
A crucial focus of traffic safety research is identifying factors that differentiate single-vehicle (SV) crashes from multi-vehicle (MV) crashes. SV crashes, often involving run-off-road incidents or collisions with stationary objects, differ markedly from MV crashes, which typically include head-on, rear-end, angle, and sideswipe collisions. While these two types of crashes may share some site-specific correlations, they differ substantially in contributing crash factors [9] and are often treated separately when conducting safety investigations. Extensive research has been devoted to analyzing MV crashes [1,6,12,17], which are most often caused by drivers' errors when interacting with other vehicles in dynamic traffic. In contrast, SV crashes usually result from the loss of vehicle control due to driver misbehavior or negligence when interacting with unfavorable environments (e.g., sharp curves and inclement weather). Thus, understanding the distinct factors that contribute to SV and MV crashes is essential for identifying the underlying dynamics that differentiate the two crash types. This knowledge can inform the development of targeted countermeasures and mitigation strategies.
Previous studies have examined various factors contributing to SV and MV crashes [10]. For example, Wang et al. [18] found that factors such as traffic volume, speed variance, and traffic operation characteristics had no significant influence on SV crashes, while the inverse was true for MV crashes. Similarly, Dong et al. [9], utilized a mixed logit model to investigate the contributing factors for different outcomes: non-crash events, SV crashes, and MV crashes, revealing that traffic, pavement characteristics, and road surface features play important roles in distinguishing among these outcomes. However, their dataset is still limited. For truck-related features with SV and MV, Wu et al. [19] employed mixed logit models and elasticity analysis to study SV and MV crashes on rural two-lane highways. Their findings revealed that the likelihood of drivers being fatally injured in a truck-involved MV crash is 270% higher. Similarly, Islam et al. [11] investigated the effect of truck at-fault SV and MV crashes in both rural and urban settings. Their results highlighted a strong correlation among single-unit trucks and increased injury severity in rural areas, while multi-unit truck crashes were found to be more severe in urban environments.
Despite these advancements, significant gaps remain in comprehensively understanding the unique factors driving SV and MV crashes. A considerable limitation of many existing studies [9,11,13] lies in their reliance on datasets that lack the necessary breadth and depth to integrate the diverse range of features contributing to crash occurrences. In particular, the collection of detailed truck traffic data and fine-grained weather (temporal-wise) and pavement characteristics (spatial-wise) presents significant challenges, thereby constraining the scope of insights that can be derived. This limitation arises because the effectiveness and interpretability of crash severity models are highly dependent on the quality and completeness of the input data. When critical variables, such as real-time weather conditions or detailed pavement characteristics, are omitted, the ability of models to capture the underlying relationships between crash types and contributing factors is significantly constrained. Such data gaps can lead to biased parameter estimates or incorrect causal attributions, resulting in oversimplified models that fail to reflect the complex mechanisms underlying SV and MV crashes. For example, the absence of real-time weather data or granular pavement details may overlook essential interactions between environmental and traffic factors, thereby reducing the reliability and applicability of the resulting safety insights.
This paper addresses these gaps by integrating diverse datasets encompassing crash-related, road pavement-related, weather-related, and traffic-related variables. Our approach leverages high-resolution data from WIM systems, incorporating detailed traffic flow characteristics such as vehicle weights and truck ratios. While it is nearly impossible for any dataset to capture all the contributing factors in crash events, especially given the complexity of human behavior, aggregating a wide range of variables provides significant insights into the difference between SV and MV crashes. In this study, we aggregated four categories of data to explore the dynamics of SV and MV crashes through a comparative analysis: (1) accident-related data: vehicle direction and driver maneuver when the crash occurred; (2) road and pavement-related data: detailed pavement surface conditions where crashes occurred; (3) weather-related data: high-resolution weather conditions; and (4) traffic-related WIM data: WIM data capturing both macroscopic flow characteristics (e.g., vehicle counts and speed statistics) and refined flow details (e.g., average vehicle weights and truck ratios) when crashes occurred.
To investigate the contributing factors distinguishing SV and MV crashes using this fused dataset, a logistic regression model with norm was fit. Logistic regression was chosen for its robustness and interpretability, which make it well-suited for identifying and quantifying the relationships between crash outcomes and contributing factors. The norm regularization helps mitigate over-fitting by penalizing large coefficients, ensuring the model remains generalizable despite the diverse and high-dimensional nature of the input features. This approach balances model complexity with performance, enabling a clear understanding of how individual variables influence crash classification. The model achieved an accuracy of in classifying SV and MV crashes, demonstrating its effectiveness. More importantly, we conducted a detailed analysis of variables identified in each feature category and their influences on crash classification. For MV crashes, factors such as roadway locations (e.g., entrance/exit ramps), driver age, lane-changing maneuvers, wind speed, and speed variance showed strong correlations. In contrast, SV crashes were more strongly associated with factors like locations off the roadway or on the shoulder, average traffic speed, pavement cracking percentage, and UV radiation. A comprehensive discussion of these findings and their implications is provided in Sections 5–7.
In this section, we first introduce the data sources used and how they were fused together, followed by data processing for subsequent regularized logistic regression.
The Georgia Department of Transportation (GDOT) maintains a WIM system, a comprehensive repository of road and pavement characteristics for state roads, and an extensive crash database. Additionally, meteorological data are publicly accessible through the Weather Underground [16]. This study integrates data from these sources, depicted in Figure 1. First, crash records are filtered to include only accidents recorded during the years 2021 and 2022 that occurred within 500 feet of each WIM station. The reason we set the distance as 500 feet is to try to link actual traffic conditions when the crash happened. To match the WIM data with the crash data, traffic conditions are extracted within one hour when the crash happened. Then, the resultant dataset is further augmented with corresponding meteorological data obtained from the Weather Underground repository, by identifying the nearest weather station and synchronizing the observed atmospheric conditions at the time of the crash within a 5 minutes window. Finally, the integrated crash-traffic-weather dataset is further enriched with road and pavement characteristic data, also sourced from GDOT, by mapping each event to the corresponding road segment. The compiled multi-source dataset contains 448 crash occurrences with 124 SV crashes and 324 MV crashes. For the MV crashes, it includes rear-end collisions, angle collisions, and side-swipe collisions in the same and opposite directions. All the variables are summarized in Table 1, categorized into four groups: crash features, road and pavement characteristics, weather features, and WIM features.
Name | Variable | Description | Processing | |
Crash features | ||||
Time Period | X1 | 0 | Mid-day (9:00-15:00) | One-Hot |
1 | Early morning (0:00-6:00) | |||
2 | Early evening (15:00-18:00) | |||
3 | Evening (18:00-24:00) | |||
4 | Morning (6:00-9:00) | |||
Location of Impact Element | X2 | 5 | Median | One-Hot |
6 | On Roadway - Roadway Intersection | |||
7 | Off Roadway | |||
8 | On Roadway - Non-Intersection | |||
9 | Entrance/Exit Ramp | |||
10 | On Shoulder | |||
Light Element | X3 | 11 | Dark-Not Lighted | One-Hot |
12 | Dark-Lighted | |||
13 | Dawn | |||
14 | Dusk | |||
15 | Daylight | |||
Surface Element | X4 | 16 | Dry | One-Hot |
17 | Water (standing or moving) | |||
18 | Wet | |||
Driver Age Element | X5 | 19 | The age of the driver that crashed (years old) | Max-min |
Veh Type Element | X6 | 20 | Unknown | One-Hot |
21 | Passenger Car | |||
22 | Sports Utility Vehicle (SUV), Pickup Truck, Van | |||
23 | Tractor/Trailer | |||
Dir Veh1 Element | X7 | 24 | West | One-Hot |
25 | East | |||
26 | North | |||
27 | South | |||
Mnvr Veh1 Element | X8 | 28 | Changing Lanes | One-Hot |
29 | PIT | |||
30 | Negotiating A Curve | |||
31 | Passing | |||
32 | Other | |||
33 | Backing | |||
34 | Turning Right | |||
35 | Making U-turn | |||
36 | Straight | |||
37 | Turning Left | |||
38 | Stopped | |||
U1 Traffic Control Element | X9 | 39 | Yield Sign | One-Hot |
40 | Traffic Signal | |||
41 | Lanes | |||
42 | Other | |||
43 | Stop Sign | |||
44 | No Control Present | |||
Road and pavement characteristics | ||||
U1 Road Composition Element | X10 | 45 | Concrete | One-Hot |
46 | Black Top | |||
U1 Road Character Element | X11 | 47 | Straight and Level | One-Hot |
48 | Curve and Level | |||
49 | Straight on Grade | |||
50 | Curve on Grade | |||
51 | Straight on Hillcrest | |||
NumLanes | X12 | 52 | The number of lanes in the direction that the primary vehicle is driving | Max-min |
U1 Traffic Way Flow Element | X13 | 53 | One-Way trafficway | One-Hot |
54 | Two-Way trafficway with a physical barrier | |||
55 | Two-Way trafficway with a physical separation | |||
56 | Continuous turning Lane | |||
57 | Two-Way trafficway with no physical separation | |||
PrcntGrade | X14 | 58 | The grade classification expressed as a percentage | Max-min |
IRIAvg | X15 | 59 | The International Roughness Index average expressed in inch/mile | Max-min |
TexAvg | X16 | 60 | The variance of profile height on the left and right wheelpath averaged | Max-min |
RutAv | X17 | 61 | The surface depression depth on the right and left wheelpath averaged | Max-min |
FAUAvg3D | X18 | 62 | Faulting values that fall across the entire lane (Measured by inch/mile) | Max-min |
FAUAvg2D | X19 | 63 | Faulting values that fall within the minimum and maximum values | Max-min |
MacroText | X20 | 64 | The mean texture depth measure of the road measured in mm | Max-min |
Heading | X21 | 65 | Measurement from beginning to the end of a horizontal curvature | Max-min |
CS | X22 | 66 | Cross slope, which is a critical design element for pavement | Max-min |
CrackPerc | X23 | 67 | Percentage of pavement surface showing cracking (as a percentage) | Max-min |
Curve | X24 | 68 | Curves B | One-Hot |
69 | Curves C | |||
70 | Curves A | |||
71 | Curves E | |||
Weather features | ||||
Temp | X25 | 72 | The temperature outside at the given time (degrees Fahrenheit) | Max-min |
Dew Point | X26 | 73 | The dew point temperature outside at the given time (degrees Fahrenheit) | Max-min |
Humidity | X27 | 74 | Percentage of water vapor in the air (expressed as percent) | Max-min |
Wind Speed | X28 | 75 | Speed of the wind (in mph) | Max-min |
Pressure | X29 | 76 | The weight of the air (in) | Max-min |
Precip. Rate | X30 | 77 | The rate at which the precipitation is falling (in) | Max-min |
Precip. Accum. | X31 | 78 | Amount of precipitation accumulated to that point in the day (in) | Max-min |
Gust | X32 | 79 | A brief increase in the speed of the wind (mph) | Max-min |
UV | X33 | 80 | The measure of the amount of UV radiation (1-10) | Max-min |
WIM features | ||||
Avg. Speed (mph) | X34 | 81 | The average vehicle speed in the hour across lanes in the direction of the crash (mph) | Max-min |
Traffic Volume | X35 | 82 | The total traffic volume for the hour that the crash occurred | Max-min |
Avg. Headway (s) | X36 | 83 | The average amount of time between one vehicle arriving and the next in the crash hour | Max-min |
Truck/Car Ratio | X37 | 84 | The number of trucks divided by the number of passenger cars in the hour of the crash | Max-min |
Avg. Weight (kg) | X38 | 85 | The average weight of all vehicles that passed by in the hour of the crash | Max-min |
Avg. PC Weight (kg) | X39 | 86 | The average weight of passenger cars (less than class 3) in the hour of the crash | Max-min |
Avg. Truck Weight (kg) | X40 | 87 | The average weight of trucks (greater than class 3) in the hour of the crash | Max-min |
Speed (mph) Variance | X41 | 88 | The variance of the speeds in the hour of the crash () | Max-min |
The crash data extracted from the police reports includes details such as the location of impact, vehicle maneuver, type of the primary vehicle, roadway type, the direction of the primary vehicle, one or two-way street, presence of traffic control, surface conditions, pavement composition, light, and driver age. These descriptive crash attributes characterize the circumstances under which each crash occurred.
WIM stations record lane-specific traffic and weight data at high temporal resolution. The WIM station locations are indicated by green rectangle markers in Figure 2. In reference to each crash, we extracted directional traffic by lanes and weight information from the corresponding WIM station for the specific hour in which the crash occurred. We further computed meaningful features to capture traffic dynamics, including average vehicle speed, average speed variance, range of speed, total traffic volume, average passenger car weight, average truck weight, average total weight, average headway, and the ratio of trucks to passenger cars as well as the proportions of each vehicle class type (Class 1 to 13).
For each WIM station, pavement condition data were obtained as well, including crack percentages, curves, faulting averages, rutting average, cross slope, percent grades, grades, macro-texture, the average texture, heading, and the average roughness index. These measurements characterize the roadway's geometry and surface conditions, which likely impact the risk of crashes and how they may occur.
Weather attributes are retrieved from the nearest available weather station for each crash occurrence, encompassing temperature, dew point, humidity, pressure, precipitation accumulation, precipitation rate, wind speed, wind gust, and UV index. These environmental conditions can influence roadway surface characteristics, driver perception and behavior, and overall driving risk.
All variables are pre-processed as shown in Table 1. Many of the features included in this study are categorical, for which one-hot encoding is applied. For numeric features, min-max scaling is used to normalize their value ranges to [0, 1].
Logistic regression is a widely used statistical method for binary classification tasks. In this study, we aim to investigate the features that help to distinguish between two distinct crash types: SV and MV crashes, which is essentially a binary classification task for which logistic regression is a natural choice.
The core of logistic regression lies in the assumption of the logistic distribution of the error term, denoted as follows:
(1) |
where is usually an affine transformation of input features and can be interpreted as the log-odds of the positive class.
The logistic function predicts the probability that a given instance belongs to the positive class (e.g., MV crash). The parametrized function can be written as
(2) |
where is the predicted probability that the instance belongs to the positive class, is model parameters (weights), and is the bias. Given the high dimensional feature space and relatively small sample size, we impose regularization to combat overfitting. For the model traning and testing, we randomly split the dataset into two subsets: 80% for training and 20% for testing.
The classification results of regularized logistic regression are summarized in Table 2, showing the model's overall effectiveness with an weighted average accuracy of 86% and weighted averaged f1-score 0.85 on the test dataset. For MV crashes, the model demonstrated a high f1-score of 0.90, precision of 0.84, and recall of 0.97. In comparison, for SV crashes, it achieves an f1-score of 0.73 and precision of 0.90, yet a lower recall of 0.62. Overall, the model demonstrated robust performance in identifying MV crashes but exhibited some limitations in accurately detecting SV crashes. This disparity may be attributed to the critical and complex role of driver behavior in SV crashes, which is challenging to model due to limited observational data on human behavior. Another possible reason is the imbalanced dataset with the majority of crashes being MV crashes. Despite these challenges, the model effectively handled the binary classification task, achieving a overall F1-score of 0.85.
precision | recall | f1-score | support | |
SV crash | 0.90 | 0.62 | 0.73 | 29 |
MV crash | 0.84 | 0.97 | 0.90 | 61 |
macro avg | 0.87 | 0.79 | 0.82 | 90 |
weighted avg | 0.86 | 0.86 | 0.85 | 90 |
accuracy | 0.86 | 90 |
In addition to the classification results, the coefficient and odds ratio for each variable are listed in Table 3. To highlight key predictors, the top 25 important variables, ranked by the magnitude of their coefficients, are summarized in Table 4. The coefficients quantify the change in the likelihood of a MV crash with respect to a one-unit change in each predictor variable. A positive coefficient signifies that an increase in the variable value raises the probability of a MV crash, while a negative coefficient indicates a decreased probability. Similarly, an odds ratio greater than 1 indicates a higher likelihood of a MV crash, whereas an odds ratio lower than 1 implies a greater likelihood of a SV crash.
Variable | Coefficients | Odds ratio | Variable | Coefficients | Odds ratio | ||
X1 | 0 | 0.1549 | 1.1675 | X10 | 45 | 0.1561 | 1.1690 |
1 | -0.2346 | 0.7909 | 46 | -0.1558 | 0.8557 | ||
2 | 0.0214 | 1.0216 | X11 | 47 | 0.4133 | 1.5118 | |
3 | -0.0692 | 0.9331 | 48 | -0.2845 | 0.7524 | ||
4 | 0.1278 | 1.1364 | 49 | 0.1785 | 1.1955 | ||
X2 | 5 | -0.3487 | 0.7056 | 50 | -0.3269 | 0.7211 | |
6 | 0.5809 | 1.7876 | 51 | 0.0199 | 1.0201 | ||
7 | -2.4101 | 0.0898 | X12 | 52 | -0.5226 | 0.5930 | |
8 | 1.7908 | 5.9944 | X13 | 53 | 0.1123 | 1.1189 | |
9 | 1.0359 | 2.8176 | 54 | -0.0621 | 0.9398 | ||
10 | -0.6485 | 0.5228 | 55 | 0.3570 | 1.4291 | ||
X3 | 11 | -0.0740 | 0.9286 | 56 | -0.3138 | 0.7307 | |
12 | -0.2425 | 0.7847 | 57 | -0.0932 | 0.9110 | ||
13 | -0.1274 | 0.8803 | X14 | 58 | -0.0672 | 0.9350 | |
14 | 0.3442 | 1.4109 | X15 | 59 | -0.0717 | 0.9308 | |
15 | 0.1000 | 1.1052 | X16 | 60 | -0.2329 | 0.7922 | |
X4 | 16 | 0.1520 | 1.1642 | X17 | 61 | 0.6428 | 1.9018 |
17 | 0.2921 | 1.3392 | X18 | 62 | -0.6190 | 0.5385 | |
18 | -0.4438 | 0.6416 | X19 | 63 | 0.0000 | 1.0000 | |
X5 | 19 | 0.8060 | 2.2389 | X20 | 64 | -0.5602 | 0.5711 |
X6 | 20 | -0.5584 | 0.5721 | X21 | 65 | 0.7518 | 2.1209 |
21 | 0.4964 | 1.6429 | X22 | 66 | 0.0333 | 1.0339 | |
22 | 0.3154 | 1.3708 | X23 | 67 | -1.3206 | 0.2670 | |
23 | -0.2532 | 0.7763 | X24 | 68 | 0.4618 | 1.5869 | |
X7 | 24 | 0.2807 | 1.3240 | 69 | -0.0625 | 0.9394 | |
25 | 0.2059 | 1.2287 | 70 | -0.1843 | 0.8317 | ||
26 | -0.0579 | 0.9438 | 71 | -0.2147 | 0.8068 | ||
27 | -0.4284 | 0.6515 | X25 | 72 | -0.1734 | 0.8408 | |
X8 | 28 | 0.7363 | 2.0881 | X26 | 73 | 0.1871 | 1.2058 |
29 | 0.0435 | 1.0445 | X27 | 74 | 0.0173 | 1.0175 | |
30 | -0.9183 | 0.3992 | X28 | 75 | 0.6781 | 1.9701 | |
31 | 0.0554 | 1.0569 | X29 | 76 | -0.1184 | 0.8883 | |
32 | 0.4451 | 1.5607 | X30 | 77 | -0.1342 | 0.8744 | |
33 | 0.3136 | 1.3684 | X31 | 78 | 0.1089 | 1.1151 | |
34 | 0.0168 | 1.0169 | X32 | 79 | -0.0945 | 0.9098 | |
35 | 0.0000 | 1.0000 | X33 | 80 | -0.6698 | 0.5118 | |
36 | -0.8274 | 0.4372 | X34 | 81 | -2.2176 | 0.1089 | |
37 | -0.0134 | 0.9867 | X35 | 82 | 0.2651 | 1.3035 | |
38 | 0.1487 | 1.1603 | X36 | 83 | -0.2415 | 0.7854 | |
X9 | 39 | 0.0168 | 1.0169 | X37 | 84 | 0.5763 | 1.7794 |
40 | 0.5597 | 1.7501 | X38 | 85 | 0.2904 | 1.3370 | |
41 | -0.5986 | 0.5496 | X39 | 86 | 0.2285 | 1.2567 | |
42 | 0.0637 | 1.0658 | X40 | 87 | 0.2302 | 1.2588 | |
43 | 0.0291 | 1.0295 | X41 | 88 | 0.5835 | 1.7923 | |
44 | -0.0703 | 0.9321 |
Variable name | Type | Variable | Coefficient | Odds ratio | |
Location (Off Roadway) | Crash | X2 | 7 | -2.4101 | 0.0898 |
Avg. Speed (mph) | WIM | X34 | 81 | -2.2176 | 0.1089 |
Location (On Roadway - Non-Intersection) | Crash | X2 | 8 | 1.7908 | 5.9944 |
CrackPerc | Pavement | X23 | 67 | -1.3206 | 0.2670 |
Location (Entrance/Exit Ramp) | Crash | X2 | 9 | 1.0359 | 2.8176 |
Mnvr Veh1 Element (Negotiating A Curve) | Crash | X8 | 30 | -0.9183 | 0.3992 |
Mnvr Veh1 Element (Straight) | Crash | X8 | 36 | -0.8274 | 0.4372 |
Driver Age Element | Crash | X5 | 19 | 0.8060 | 2.2389 |
Heading | Pavement | X21 | 65 | 0.7518 | 2.1209 |
Mnvr Veh1 Element (Changing Lanes) | Crash | X8 | 28 | 0.7363 | 2.0881 |
Wind Speed | Weather | X28 | 75 | 0.6781 | 1.9701 |
UV | Weather | X33 | 80 | -0.6698 | 0.5118 |
Location (On Shoulder) | Crash | X2 | 10 | -0.6485 | 0.5228 |
RutAv | Pavement | X17 | 61 | 0.6428 | 1.9018 |
FAUAvg3D | Pavement | X18 | 62 | -0.6190 | 0.5385 |
U1 Traffic Control Element (Lanes) | Crash | X9 | 41 | -0.5986 | 0.5496 |
Speed (mph) Variance | WIM | X41 | 88 | 0.5835 | 1.7923 |
Location (On Roadway - Roadway Intersection) | Crash | X2 | 6 | 0.5809 | 1.7876 |
Truck/Car Ratio | WIM | X37 | 84 | 0.5763 | 1.7794 |
MacroText | Pavement | X20 | 64 | -0.5602 | 0.5711 |
U1 Traffic Control Element (Traffic Signal) | Crash | X9 | 40 | 0.5597 | 1.7501 |
Veh Type Element (Unknown) | Crash | X6 | 20 | -0.5584 | 0.5721 |
NumLanes | Pavement | X12 | 52 | -0.5226 | 0.5930 |
Veh Type Element (Passenger Car) | Crash | X6 | 21 | 0.4964 | 1.6429 |
CurveB | Pavement | X24 | 68 | 0.4618 | 1.5869 |
Table 4 shows that the top 25 important variables differentiating SV crashes and MV crashes span across various feature groups. Within the crash and traffic-related features, the most important variable is the crash location - off roadway, while the average speed (Avg. Speed (mph)) ranks highest in the traffic-related WIM features. The crack percentage (CrackPerc) emerges as the most influential variable in the road and pavement characteristics, and wind speed is the leading variable in the weather-related features. Detailed discussions of these findings are provided in the subsequent Sections 5–7.
In this section, we delve into important variables within the crash features and discuss their specific impacts on differentiating SV crashes and MV crashes.
The coefficients of five time periods are plotted in Figure 3, with the following definition: early morning (12:00 AM to 6:00 AM), morning (6:00 AM to 9:00 AM), mid-day (9:00 AM to 3:00 PM), early evening (3:00 PM to 6:00 PM), and evening (6:00 PM to 12:00 AM). This time segmentation partially captures variation in traffic characteristics, such as the typical AM and PM peak hours, represented by the morning and early evening periods. The results revealed that SV crashes are more likely to occur during the early morning and evening periods, when traffic is lighter, and less likely during the morning or mid-day periods.
Figure 4 illustrates the coefficients of various location elements, designated as Location Element (X2), including Entrance/Exit Ramp, Median, Off Roadway, On Shoulder, On Roadway-Non-Intersection, and On Roadway-Roadway Intersection. The results indicate that crashes at Off Roadway locations exhibit the strongest association with SV crashes. In contrast, crashes occurring On Roadway, both at intersections and non-intersections, are predominantly associated with MV crashes. Furthermore, the analysis reveals that MV crashes are more likely to occur on entrance and exit ramps compared to other locations.
Figure 5 presents the coefficients for various lighting conditions, designated as Light Element (X3), including Dusk, Daylight, Dark-Not Lighted, Dawn, and Dark-Lighted. The results indicate that crashes occurring during Dusk are more likely to involve multiple vehicles. Conversely, SV crashes are more commonly associated with Dark-Lighted and Dawn conditions.
These patterns highlight the influence of lighting conditions on crash types. The higher risk of MV crashes during Dusk may be attributed to reduced visibility from rapidly changing light levels and glare from the setting sun, compounded by high traffic volumes typical of evening commutes. In contrast, the association of SV crashes with Dark-Lighted and Dawn conditions could reflect challenges posed by low ambient light, which can impair a driver's ability to perceive road geometry or obstacles. Additionally, fatigue during early morning hours might contribute to the increased risk of SV crashes during Dawn. These findings underscore the importance of adaptive lighting solutions and visibility-enhancing measures, such as reflective road markings and targeted lighting improvements, to mitigate crash risks under varying lighting conditions.
Figure 6 illustrates the coefficients corresponding to road surface conditions, designated as Surface Element (X4) at the time of crashes, categorized as Water (standing or moving), Dry, and Wet. The results indicate that SV crashes are more likely to occur on Wet road surfaces, while MV crashes are predominantly associated with roadways featuring Water (standing or moving).
These findings highlight the distinct challenges posed by various road surface conditions. The increased likelihood of SV crashes on Wet surfaces likely results from reduced tire traction, increasing the risk of skidding or loss of control, particularly at higher speeds. Conversely, the strong association between MV crashes and Water (standing or moving) can be attributed to additional hazards such as hydroplaning or sudden braking in response to water-related obstacles, often leading to chain-reaction collisions involving multiple vehicles. These results underscore the importance of implementing proactive measures, including timely warnings about hazardous road conditions and the integration of computer vision techniques to support drivers in navigating adverse weather conditions effectively.
Figure 7 presents the coefficients for vehicle types, designated as Veh Type Element (X6) involved in crashes, categorized as Passenger Car, SUV, Pickup Truck, and Van, Tractor/Trailers, and Unknown. The results indicate that Passenger Car and SUV, Pickup Truck, and Van categories are more frequently associated with MV crashes, whereas larger vehicles, such as Tractor/Trailers, are more likely to be involved in SV crashes. This pattern suggests that the higher involvement of larger vehicles like Tractor/Trailers in SV crashes could be attributed to their reduced maneuverability and longer stopping distances, making them more prone to losing control in challenging situations.
Figure 8 depicts the coefficients for driving directions, designated as Dir Veh1 Element (X7) and categorized as South, North, West, and East. The results reveal that the risk of MV crashes increases when vehicles are traveling West or East. This phenomenon may be attributed to the adverse effects of sun glare during sunrise and sunset, which can significantly impair driver visibility. Additionally, sunrise and sunset periods often coincide with peak traffic volumes, further exacerbating the risk of MV crashes.
Figure 9 illustrates the coefficients for various vehicle maneuvers, designated as Mnvr Veh1 Element (X8), including Negotiating a Curve, Making a U-Turn, Other, Changing Lanes, Straight, Passing, Backing, PIT, Turning Left, Turning Right, and Stopped. The results indicate that SV crashes are more likely to occur when vehicles are Negotiating a Curve or traveling Straight. In contrast, MV crashes are more frequently associated with maneuvers such as Changing Lanes or Backing. This pattern highlights the differing dynamics of crash types based on vehicle maneuvers. The increased likelihood of SV crashes during Negotiating a Curve or traveling Straight may result from drivers losing control due to excessive speed, sharp turns, or adverse road conditions. Conversely, MV crashes being more common during maneuvers such as Changing Lanes or Backing likely reflects scenarios with higher vehicle interactions and limited visibility, increasing the risk of collisions.
Figure 10 presents the coefficients for various traffic control elements, designated as U1 Traffic Control Element (X9), including No Control Present, Yield Sign, Traffic Signal, Lanes, Stop Sign, and Other. The results indicate that SV crashes are more likely to occur on roads with Lanes control, which may reflect situations where lane markings are insufficient to guide drivers effectively, especially under adverse conditions. Conversely, the higher risk of MV crashes at locations with Traffic Signals likely stems from the increased vehicle interactions and traffic volumes at signalized intersections. These findings emphasize the need for targeted safety measures, such as improved lane markings for roads with Lanes control and enhanced signal management at high-traffic intersections, to mitigate crash risks.
This section examines key variables related to road and pavement characteristics, analyzing their impacts on distinguishing between SV and MV crashes.
Figure 11 plots the coefficients for the road characteristic elements, designated as U1 Road Character Element (X11) of the segments where crashes occurred, categorized as Curve and Level, Straight and Level, Straight on Hillcrest, Straight on Grade, and Curve on Grade. The results indicate that on straight road segments, whether level or on a grade, the probability of a MV crash is higher than that of a SV crash. Conversely, curved segments, whether level or on a grade, are more likely to be associated with SV crashes. This pattern likely reflects the greater challenges curves pose to vehicle control, particularly under adverse conditions, increasing the risk of losing control and resulting in SV crashes. In contrast, straight segments typically involve higher traffic volumes and interactions, which may lead to a greater likelihood of MV crashes.
Figure 12 plots the coefficients of different traffic way flow elements (X13) for the segments where crashes occurred. The traffic way flow elements include Two-Way Traffic-way with a Physical Separation, One-Way Traffic-way, Two-Way Traffic-way with a Physical Barrier, Continuous Turning Lane, and Two-Way Traffic-way with No Physical Separation. The results indicate that Continuous Turning Lanes are more likely to contribute to SV crashes, whereas Two-Way Traffic-ways with a Physical Separation are associated with a higher likelihood of MV crashes. This may reflect the unique dynamics of each road configuration. For example, continuous turning lanes can pose challenges for individual drivers, such as unexpected maneuvers or misjudging the lane's boundaries, leading to a higher risk of SV crashes.
Figure 13 illustrates the coefficients for various pavement variables, including the directional number of lanes (X12), PrcntGrade (X14), IRIAvg (X15), TexAvg (X16), RutAv (X17), FAUAvg3D (X18), FAUAvg2D (X19), MacroText (X20), Heading (X21), CS (X22), and CrackPerc (X23). The results show that increases in CrackPerc, MacroText, and FAUAvg2D are associated with a higher likelihood of SV crashes compared to MV crashes. Conversely, higher values of RutAv and Heading are linked to a greater likelihood of MV crashes. These findings suggest that deteriorated pavement conditions, such as increased cracking (CrackPerc) and macro-texture (MacroText), contribute to loss of control, making SV crashes more likely. On the other hand, higher RutAv values and variations in Heading could indicate areas with complex vehicle interactions or high traffic volumes, which may increase the risk of MV crashes.
This section explores key weather- and WIM-related variables, analyzing their impacts on distinguishing between SV and MV crashes.
Figure 14 presents the coefficients for weather-related variables, including Temp (X25), Dew Point (X26), Humidity (X27), Wind Speed (X28), Pressure (X29), Precip. Rate (X30), Precip. Accum. (X31), Gust (X32), and UV (X33). Among these variables, UV and Wind Speed stand out as relatively important features, with UV associated with a higher probability of SV crashes and Wind Speed linked to an increased likelihood of MV crashes. Higher UV levels could correlate with clear weather conditions, potentially encouraging higher speeds or riskier driving behavior that increases SV crash risks.
Figure 15 plots the coefficients of WIM-related variables, including Avg. Speed (X34), Traffic Volume (X35), Avg. Headway (X36), Truck/Car Ratio (X37), Avg. Weight (X38), Avg. Passenger Car Weight (X39), Avg. Truck Weight (X40), and Speed (mph) Variance (X41). The results indicate that higher Avg. Speed on the roadway increases the likelihood of SV crashes compared to MV crashes. A larger Truck/Car Ratio, reflecting a higher proportion of trucks in the traffic flow, is associated with an increased likelihood of MV crashes. Furthermore, greater Speed Variance across lanes is linked to a higher probability of MV crashes over SV crashes.
These findings highlight the interplay between traffic dynamics and crash types. Elevated Avg. Speed likely contributes to SV crashes due to reduced reaction time and greater difficulty in maintaining control at higher speeds. In contrast, a higher Truck/Car Ratio and Speed Variance create more complex traffic interactions, increasing the likelihood of MV crashes. These insights underscore the importance of managing traffic speed consistency and managing vehicle distributions on roadways or separate lanes at critical locations to enhance safety for all users.
This paper presents a comprehensive study aimed at understanding the dynamics of SV and MV crashes through a binary classification approach. By integrating high-resolution, multi-source data, we identified key factors that differentiate these two crash types. A data fusion approach was employed to integrate these diverse data sources, enabling a holistic investigation of the factors influencing crash outcomes. The regularized logistic regression was applied with the multi-source data for SV vs. MV crashes. The regularized logistic regression model demonstrated strong performance, achieving a weighted average accuracy of 86% and an f1-score of 0.85 on the test dataset.
Our findings reveal that for MV crashes, factors such as locations on the roadway, or entrance/exit ramps, driver age, maneuvers such as changing lanes, wind speed, and speed variance exhibit strong correlations. For SV crashes, factors like locations off the roadway or on the shoulder, average traffic speed, cracking percentage of pavement, or UV radiation show higher correlations. These insights highlight the specific factors influencing each crash type and pave the way for more efficient and targeted safety measures. By analyzing these specific factors, our research supports the development of tailored strategies to enhance road safety and reduce the frequency and severity of accidents. Ultimately, our study contributes to the advancement of evidence-based road safety practices, fostering a safer transportation landscape for all road users.
The authors declare they have not used Artificial Intelligence (AI) tools in the creation of this article.
The authors declare no conflict of interest.
[1] |
M. Abdel-Aty, N. Uddin, A. Pande, Split models for predicting multivehicle crashes during high-speed and low-speed operating conditions on freeways, Transport. Res. Rec., 1908 (2005), 51–58. https://doi.org/10.1177/0361198105190800107 doi: 10.1177/0361198105190800107
![]() |
[2] | Federal Highway Administration, Highway statistics series: motor vehicle registrations, Office of Highway Policy Information, 2022. Available from: https://www.fhwa.dot.gov/policyinformation/statistics/2022/mv1.cfm. |
[3] |
M. Ahmed, M. Abdel-Aty, R. Yu, Assessment of interaction of crash occurrence, mountainous freeway geometry, real-time weather, and traffic data, Transport. Res. Rec., 2280 (2012), 51–59. https://doi.org/10.3141/2280-06 doi: 10.3141/2280-06
![]() |
[4] |
M. Ahmed, R. Franke, K. Ksaibati, D. Shinstine, Effects of truck traffic on crash injury severity on rural highways in wyoming using bayesian binary logit models, Accident Anal. Prev., 117 (2018), 106–113. https://doi.org/10.1016/j.aap.2018.04.011 doi: 10.1016/j.aap.2018.04.011
![]() |
[5] |
N. Al-Bdairi, S. Hernandez, An empirical analysis of run-off-road injury severity crashes involving large trucks, Accident Anal. Prev., 102 (2017), 93–100. https://doi.org/10.1016/j.aap.2017.02.024 doi: 10.1016/j.aap.2017.02.024
![]() |
[6] |
F. Basso, L. Basso, F. Bravo, R. Pezoa, Real-time crash prediction in an urban expressway using disaggregated data, Transport. Res. C-Emer., 86 (2018), 202–219. https://doi.org/10.1016/j.trc.2017.11.014 doi: 10.1016/j.trc.2017.11.014
![]() |
[7] |
N. Becker, H. Rust, U. Ulbrich, Weather impacts on various types of road crashes: a quantitative analysis using generalized additive models, Eur. Transp. Res. Rev., 14 (2022), 37. https://doi.org/10.1186/s12544-022-00561-2 doi: 10.1186/s12544-022-00561-2
![]() |
[8] |
Z. Christoforou, S. Cohen, M. Karlaftis, Vehicle occupant injury severity on highways: an empirical investigation, Accident Anal. Prev., 42 (2010), 1606–1620. https://doi.org/10.1016/j.aap.2010.03.019 doi: 10.1016/j.aap.2010.03.019
![]() |
[9] |
B. Dong, X. Ma, F. Chen, S. Chen, Investigating the differences of single-vehicle and multivehicle accident probability using mixed logit model, J. Adv. Transport., 2018 (2018), 2702360. https://doi.org/10.1155/2018/2702360 doi: 10.1155/2018/2702360
![]() |
[10] |
S. Geedipally, D. Lord, Investigating the effect of modeling single-vehicle and multi-vehicle crashes separately on confidence intervals of poisson–-gamma models, Accident Anal. Prev., 42 (2010), 1273–1282. https://doi.org/10.1016/j.aap.2010.02.004 doi: 10.1016/j.aap.2010.02.004
![]() |
[11] |
S. Islam, S. Jones, D. Dye, Comprehensive analysis of single-and multi-vehicle large truck at-fault crashes on rural and urban roadways in alabama, Accident Anal. Prev., 67 (2014), 148–158. https://doi.org/10.1016/j.aap.2014.02.014 doi: 10.1016/j.aap.2014.02.014
![]() |
[12] |
C. Morris, J. Yang, Effectiveness of resampling methods in coping with imbalanced crash data: crash type analysis and predictive modeling, Accident Anal. Prev., 159 (2021), 106240. https://doi.org/10.1016/j.aap.2021.106240 doi: 10.1016/j.aap.2021.106240
![]() |
[13] |
B. Naik, L. Tung, S. Zhao, A. Khattak, Weather impacts on single-vehicle truck crash injury severity, J. Safety Res., 58 (2016), 57–65. https://doi.org/10.1016/j.jsr.2016.06.005 doi: 10.1016/j.jsr.2016.06.005
![]() |
[14] | National highway traffic safety administration, Early estimate of motor vehicle traffic fatalities in 2023, National Highway Traffic Safety Administration (NHTSA), 2023. Available from: https://crashstats.nhtsa.dot.gov/Api/Public/ViewPublication/813561. |
[15] |
M. Uddin, N. Huynh, Injury severity analysis of truck-involved crashes under different weather conditions, Accident Anal. Prev., 141 (2020), 105529. https://doi.org/10.1016/j.aap.2020.105529 doi: 10.1016/j.aap.2020.105529
![]() |
[16] | Weather Underground, Wundermap weather information, Weather Data APIs, 2023. Available from: https://www.wunderground.com/wundermap?lat=33.751&lon=-84.39. |
[17] |
D. Viano, C. Culver, L. Evans, M. Frick, R. Scott, Involvement of older drivers in multivehicle side-impact crashes, Accident Anal. Prev., 22 (1990), 177–188. https://doi.org/10.1016/0001-4575(90)90068-V doi: 10.1016/0001-4575(90)90068-V
![]() |
[18] |
X. Wang, M. Feng, Freeway single and multi-vehicle crash safety analysis: influencing factors and hotspots, Accident Anal. Prev., 132 (2019), 105268. https://doi.org/10.1016/j.aap.2019.105268 doi: 10.1016/j.aap.2019.105268
![]() |
[19] |
Q. Wu, F. Chen, G. Zhang, X. Liu, H. Wang, S. Bogus, Mixed logit model-based driver injury severity investigations in single-and multi-vehicle crashes on rural two-lane highways, Accident Anal. Prev., 72 (2014), 105–115. https://doi.org/10.1016/j.aap.2014.06.014 doi: 10.1016/j.aap.2014.06.014
![]() |
[20] |
K. Xie, K. Ozbay, H. Yang, A multivariate spatial approach to model crash counts by injury severity, Accident Anal. Prev., 122 (2019), 189–198. https://doi.org/10.1016/j.aap.2018.10.009 doi: 10.1016/j.aap.2018.10.009
![]() |
[21] |
C. Xu, W. Wang, P. Liu, Identifying crash-prone traffic conditions under different weather on freeways, J. Safety Res., 46 (2013), 135–144. https://doi.org/10.1016/j.jsr.2013.04.007 doi: 10.1016/j.jsr.2013.04.007
![]() |
[22] |
C. Xu, K. Ozbay, H. Liu, K. Xie, D. Yang, Exploring the impact of truck traffic on road segment-based severe crash proportion using extensive weigh-in-motion data, Safety Sci., 166 (2023), 106261. https://doi.org/10.1016/j.ssci.2023.106261 doi: 10.1016/j.ssci.2023.106261
![]() |
[23] |
Q. Zeng, W. Hao, J. Lee, F. Chen, Investigating the impacts of real-time weather conditions on freeway crash severity: a bayesian spatial analysis, Int. J. Environ. Res. Public Health, 17 (2020), 2768. https://doi.org/10.3390/ijerph17082768 doi: 10.3390/ijerph17082768
![]() |
Name | Variable | Description | Processing | |
Crash features | ||||
Time Period | X1 | 0 | Mid-day (9:00-15:00) | One-Hot |
1 | Early morning (0:00-6:00) | |||
2 | Early evening (15:00-18:00) | |||
3 | Evening (18:00-24:00) | |||
4 | Morning (6:00-9:00) | |||
Location of Impact Element | X2 | 5 | Median | One-Hot |
6 | On Roadway - Roadway Intersection | |||
7 | Off Roadway | |||
8 | On Roadway - Non-Intersection | |||
9 | Entrance/Exit Ramp | |||
10 | On Shoulder | |||
Light Element | X3 | 11 | Dark-Not Lighted | One-Hot |
12 | Dark-Lighted | |||
13 | Dawn | |||
14 | Dusk | |||
15 | Daylight | |||
Surface Element | X4 | 16 | Dry | One-Hot |
17 | Water (standing or moving) | |||
18 | Wet | |||
Driver Age Element | X5 | 19 | The age of the driver that crashed (years old) | Max-min |
Veh Type Element | X6 | 20 | Unknown | One-Hot |
21 | Passenger Car | |||
22 | Sports Utility Vehicle (SUV), Pickup Truck, Van | |||
23 | Tractor/Trailer | |||
Dir Veh1 Element | X7 | 24 | West | One-Hot |
25 | East | |||
26 | North | |||
27 | South | |||
Mnvr Veh1 Element | X8 | 28 | Changing Lanes | One-Hot |
29 | PIT | |||
30 | Negotiating A Curve | |||
31 | Passing | |||
32 | Other | |||
33 | Backing | |||
34 | Turning Right | |||
35 | Making U-turn | |||
36 | Straight | |||
37 | Turning Left | |||
38 | Stopped | |||
U1 Traffic Control Element | X9 | 39 | Yield Sign | One-Hot |
40 | Traffic Signal | |||
41 | Lanes | |||
42 | Other | |||
43 | Stop Sign | |||
44 | No Control Present | |||
Road and pavement characteristics | ||||
U1 Road Composition Element | X10 | 45 | Concrete | One-Hot |
46 | Black Top | |||
U1 Road Character Element | X11 | 47 | Straight and Level | One-Hot |
48 | Curve and Level | |||
49 | Straight on Grade | |||
50 | Curve on Grade | |||
51 | Straight on Hillcrest | |||
NumLanes | X12 | 52 | The number of lanes in the direction that the primary vehicle is driving | Max-min |
U1 Traffic Way Flow Element | X13 | 53 | One-Way trafficway | One-Hot |
54 | Two-Way trafficway with a physical barrier | |||
55 | Two-Way trafficway with a physical separation | |||
56 | Continuous turning Lane | |||
57 | Two-Way trafficway with no physical separation | |||
PrcntGrade | X14 | 58 | The grade classification expressed as a percentage | Max-min |
IRIAvg | X15 | 59 | The International Roughness Index average expressed in inch/mile | Max-min |
TexAvg | X16 | 60 | The variance of profile height on the left and right wheelpath averaged | Max-min |
RutAv | X17 | 61 | The surface depression depth on the right and left wheelpath averaged | Max-min |
FAUAvg3D | X18 | 62 | Faulting values that fall across the entire lane (Measured by inch/mile) | Max-min |
FAUAvg2D | X19 | 63 | Faulting values that fall within the minimum and maximum values | Max-min |
MacroText | X20 | 64 | The mean texture depth measure of the road measured in mm | Max-min |
Heading | X21 | 65 | Measurement from beginning to the end of a horizontal curvature | Max-min |
CS | X22 | 66 | Cross slope, which is a critical design element for pavement | Max-min |
CrackPerc | X23 | 67 | Percentage of pavement surface showing cracking (as a percentage) | Max-min |
Curve | X24 | 68 | Curves B | One-Hot |
69 | Curves C | |||
70 | Curves A | |||
71 | Curves E | |||
Weather features | ||||
Temp | X25 | 72 | The temperature outside at the given time (degrees Fahrenheit) | Max-min |
Dew Point | X26 | 73 | The dew point temperature outside at the given time (degrees Fahrenheit) | Max-min |
Humidity | X27 | 74 | Percentage of water vapor in the air (expressed as percent) | Max-min |
Wind Speed | X28 | 75 | Speed of the wind (in mph) | Max-min |
Pressure | X29 | 76 | The weight of the air (in) | Max-min |
Precip. Rate | X30 | 77 | The rate at which the precipitation is falling (in) | Max-min |
Precip. Accum. | X31 | 78 | Amount of precipitation accumulated to that point in the day (in) | Max-min |
Gust | X32 | 79 | A brief increase in the speed of the wind (mph) | Max-min |
UV | X33 | 80 | The measure of the amount of UV radiation (1-10) | Max-min |
WIM features | ||||
Avg. Speed (mph) | X34 | 81 | The average vehicle speed in the hour across lanes in the direction of the crash (mph) | Max-min |
Traffic Volume | X35 | 82 | The total traffic volume for the hour that the crash occurred | Max-min |
Avg. Headway (s) | X36 | 83 | The average amount of time between one vehicle arriving and the next in the crash hour | Max-min |
Truck/Car Ratio | X37 | 84 | The number of trucks divided by the number of passenger cars in the hour of the crash | Max-min |
Avg. Weight (kg) | X38 | 85 | The average weight of all vehicles that passed by in the hour of the crash | Max-min |
Avg. PC Weight (kg) | X39 | 86 | The average weight of passenger cars (less than class 3) in the hour of the crash | Max-min |
Avg. Truck Weight (kg) | X40 | 87 | The average weight of trucks (greater than class 3) in the hour of the crash | Max-min |
Speed (mph) Variance | X41 | 88 | The variance of the speeds in the hour of the crash () | Max-min |
precision | recall | f1-score | support | |
SV crash | 0.90 | 0.62 | 0.73 | 29 |
MV crash | 0.84 | 0.97 | 0.90 | 61 |
macro avg | 0.87 | 0.79 | 0.82 | 90 |
weighted avg | 0.86 | 0.86 | 0.85 | 90 |
accuracy | 0.86 | 90 |
Variable | Coefficients | Odds ratio | Variable | Coefficients | Odds ratio | ||
X1 | 0 | 0.1549 | 1.1675 | X10 | 45 | 0.1561 | 1.1690 |
1 | -0.2346 | 0.7909 | 46 | -0.1558 | 0.8557 | ||
2 | 0.0214 | 1.0216 | X11 | 47 | 0.4133 | 1.5118 | |
3 | -0.0692 | 0.9331 | 48 | -0.2845 | 0.7524 | ||
4 | 0.1278 | 1.1364 | 49 | 0.1785 | 1.1955 | ||
X2 | 5 | -0.3487 | 0.7056 | 50 | -0.3269 | 0.7211 | |
6 | 0.5809 | 1.7876 | 51 | 0.0199 | 1.0201 | ||
7 | -2.4101 | 0.0898 | X12 | 52 | -0.5226 | 0.5930 | |
8 | 1.7908 | 5.9944 | X13 | 53 | 0.1123 | 1.1189 | |
9 | 1.0359 | 2.8176 | 54 | -0.0621 | 0.9398 | ||
10 | -0.6485 | 0.5228 | 55 | 0.3570 | 1.4291 | ||
X3 | 11 | -0.0740 | 0.9286 | 56 | -0.3138 | 0.7307 | |
12 | -0.2425 | 0.7847 | 57 | -0.0932 | 0.9110 | ||
13 | -0.1274 | 0.8803 | X14 | 58 | -0.0672 | 0.9350 | |
14 | 0.3442 | 1.4109 | X15 | 59 | -0.0717 | 0.9308 | |
15 | 0.1000 | 1.1052 | X16 | 60 | -0.2329 | 0.7922 | |
X4 | 16 | 0.1520 | 1.1642 | X17 | 61 | 0.6428 | 1.9018 |
17 | 0.2921 | 1.3392 | X18 | 62 | -0.6190 | 0.5385 | |
18 | -0.4438 | 0.6416 | X19 | 63 | 0.0000 | 1.0000 | |
X5 | 19 | 0.8060 | 2.2389 | X20 | 64 | -0.5602 | 0.5711 |
X6 | 20 | -0.5584 | 0.5721 | X21 | 65 | 0.7518 | 2.1209 |
21 | 0.4964 | 1.6429 | X22 | 66 | 0.0333 | 1.0339 | |
22 | 0.3154 | 1.3708 | X23 | 67 | -1.3206 | 0.2670 | |
23 | -0.2532 | 0.7763 | X24 | 68 | 0.4618 | 1.5869 | |
X7 | 24 | 0.2807 | 1.3240 | 69 | -0.0625 | 0.9394 | |
25 | 0.2059 | 1.2287 | 70 | -0.1843 | 0.8317 | ||
26 | -0.0579 | 0.9438 | 71 | -0.2147 | 0.8068 | ||
27 | -0.4284 | 0.6515 | X25 | 72 | -0.1734 | 0.8408 | |
X8 | 28 | 0.7363 | 2.0881 | X26 | 73 | 0.1871 | 1.2058 |
29 | 0.0435 | 1.0445 | X27 | 74 | 0.0173 | 1.0175 | |
30 | -0.9183 | 0.3992 | X28 | 75 | 0.6781 | 1.9701 | |
31 | 0.0554 | 1.0569 | X29 | 76 | -0.1184 | 0.8883 | |
32 | 0.4451 | 1.5607 | X30 | 77 | -0.1342 | 0.8744 | |
33 | 0.3136 | 1.3684 | X31 | 78 | 0.1089 | 1.1151 | |
34 | 0.0168 | 1.0169 | X32 | 79 | -0.0945 | 0.9098 | |
35 | 0.0000 | 1.0000 | X33 | 80 | -0.6698 | 0.5118 | |
36 | -0.8274 | 0.4372 | X34 | 81 | -2.2176 | 0.1089 | |
37 | -0.0134 | 0.9867 | X35 | 82 | 0.2651 | 1.3035 | |
38 | 0.1487 | 1.1603 | X36 | 83 | -0.2415 | 0.7854 | |
X9 | 39 | 0.0168 | 1.0169 | X37 | 84 | 0.5763 | 1.7794 |
40 | 0.5597 | 1.7501 | X38 | 85 | 0.2904 | 1.3370 | |
41 | -0.5986 | 0.5496 | X39 | 86 | 0.2285 | 1.2567 | |
42 | 0.0637 | 1.0658 | X40 | 87 | 0.2302 | 1.2588 | |
43 | 0.0291 | 1.0295 | X41 | 88 | 0.5835 | 1.7923 | |
44 | -0.0703 | 0.9321 |
Variable name | Type | Variable | Coefficient | Odds ratio | |
Location (Off Roadway) | Crash | X2 | 7 | -2.4101 | 0.0898 |
Avg. Speed (mph) | WIM | X34 | 81 | -2.2176 | 0.1089 |
Location (On Roadway - Non-Intersection) | Crash | X2 | 8 | 1.7908 | 5.9944 |
CrackPerc | Pavement | X23 | 67 | -1.3206 | 0.2670 |
Location (Entrance/Exit Ramp) | Crash | X2 | 9 | 1.0359 | 2.8176 |
Mnvr Veh1 Element (Negotiating A Curve) | Crash | X8 | 30 | -0.9183 | 0.3992 |
Mnvr Veh1 Element (Straight) | Crash | X8 | 36 | -0.8274 | 0.4372 |
Driver Age Element | Crash | X5 | 19 | 0.8060 | 2.2389 |
Heading | Pavement | X21 | 65 | 0.7518 | 2.1209 |
Mnvr Veh1 Element (Changing Lanes) | Crash | X8 | 28 | 0.7363 | 2.0881 |
Wind Speed | Weather | X28 | 75 | 0.6781 | 1.9701 |
UV | Weather | X33 | 80 | -0.6698 | 0.5118 |
Location (On Shoulder) | Crash | X2 | 10 | -0.6485 | 0.5228 |
RutAv | Pavement | X17 | 61 | 0.6428 | 1.9018 |
FAUAvg3D | Pavement | X18 | 62 | -0.6190 | 0.5385 |
U1 Traffic Control Element (Lanes) | Crash | X9 | 41 | -0.5986 | 0.5496 |
Speed (mph) Variance | WIM | X41 | 88 | 0.5835 | 1.7923 |
Location (On Roadway - Roadway Intersection) | Crash | X2 | 6 | 0.5809 | 1.7876 |
Truck/Car Ratio | WIM | X37 | 84 | 0.5763 | 1.7794 |
MacroText | Pavement | X20 | 64 | -0.5602 | 0.5711 |
U1 Traffic Control Element (Traffic Signal) | Crash | X9 | 40 | 0.5597 | 1.7501 |
Veh Type Element (Unknown) | Crash | X6 | 20 | -0.5584 | 0.5721 |
NumLanes | Pavement | X12 | 52 | -0.5226 | 0.5930 |
Veh Type Element (Passenger Car) | Crash | X6 | 21 | 0.4964 | 1.6429 |
CurveB | Pavement | X24 | 68 | 0.4618 | 1.5869 |
Name | Variable | Description | Processing | |
Crash features | ||||
Time Period | X1 | 0 | Mid-day (9:00-15:00) | One-Hot |
1 | Early morning (0:00-6:00) | |||
2 | Early evening (15:00-18:00) | |||
3 | Evening (18:00-24:00) | |||
4 | Morning (6:00-9:00) | |||
Location of Impact Element | X2 | 5 | Median | One-Hot |
6 | On Roadway - Roadway Intersection | |||
7 | Off Roadway | |||
8 | On Roadway - Non-Intersection | |||
9 | Entrance/Exit Ramp | |||
10 | On Shoulder | |||
Light Element | X3 | 11 | Dark-Not Lighted | One-Hot |
12 | Dark-Lighted | |||
13 | Dawn | |||
14 | Dusk | |||
15 | Daylight | |||
Surface Element | X4 | 16 | Dry | One-Hot |
17 | Water (standing or moving) | |||
18 | Wet | |||
Driver Age Element | X5 | 19 | The age of the driver that crashed (years old) | Max-min |
Veh Type Element | X6 | 20 | Unknown | One-Hot |
21 | Passenger Car | |||
22 | Sports Utility Vehicle (SUV), Pickup Truck, Van | |||
23 | Tractor/Trailer | |||
Dir Veh1 Element | X7 | 24 | West | One-Hot |
25 | East | |||
26 | North | |||
27 | South | |||
Mnvr Veh1 Element | X8 | 28 | Changing Lanes | One-Hot |
29 | PIT | |||
30 | Negotiating A Curve | |||
31 | Passing | |||
32 | Other | |||
33 | Backing | |||
34 | Turning Right | |||
35 | Making U-turn | |||
36 | Straight | |||
37 | Turning Left | |||
38 | Stopped | |||
U1 Traffic Control Element | X9 | 39 | Yield Sign | One-Hot |
40 | Traffic Signal | |||
41 | Lanes | |||
42 | Other | |||
43 | Stop Sign | |||
44 | No Control Present | |||
Road and pavement characteristics | ||||
U1 Road Composition Element | X10 | 45 | Concrete | One-Hot |
46 | Black Top | |||
U1 Road Character Element | X11 | 47 | Straight and Level | One-Hot |
48 | Curve and Level | |||
49 | Straight on Grade | |||
50 | Curve on Grade | |||
51 | Straight on Hillcrest | |||
NumLanes | X12 | 52 | The number of lanes in the direction that the primary vehicle is driving | Max-min |
U1 Traffic Way Flow Element | X13 | 53 | One-Way trafficway | One-Hot |
54 | Two-Way trafficway with a physical barrier | |||
55 | Two-Way trafficway with a physical separation | |||
56 | Continuous turning Lane | |||
57 | Two-Way trafficway with no physical separation | |||
PrcntGrade | X14 | 58 | The grade classification expressed as a percentage | Max-min |
IRIAvg | X15 | 59 | The International Roughness Index average expressed in inch/mile | Max-min |
TexAvg | X16 | 60 | The variance of profile height on the left and right wheelpath averaged | Max-min |
RutAv | X17 | 61 | The surface depression depth on the right and left wheelpath averaged | Max-min |
FAUAvg3D | X18 | 62 | Faulting values that fall across the entire lane (Measured by inch/mile) | Max-min |
FAUAvg2D | X19 | 63 | Faulting values that fall within the minimum and maximum values | Max-min |
MacroText | X20 | 64 | The mean texture depth measure of the road measured in mm | Max-min |
Heading | X21 | 65 | Measurement from beginning to the end of a horizontal curvature | Max-min |
CS | X22 | 66 | Cross slope, which is a critical design element for pavement | Max-min |
CrackPerc | X23 | 67 | Percentage of pavement surface showing cracking (as a percentage) | Max-min |
Curve | X24 | 68 | Curves B | One-Hot |
69 | Curves C | |||
70 | Curves A | |||
71 | Curves E | |||
Weather features | ||||
Temp | X25 | 72 | The temperature outside at the given time (degrees Fahrenheit) | Max-min |
Dew Point | X26 | 73 | The dew point temperature outside at the given time (degrees Fahrenheit) | Max-min |
Humidity | X27 | 74 | Percentage of water vapor in the air (expressed as percent) | Max-min |
Wind Speed | X28 | 75 | Speed of the wind (in mph) | Max-min |
Pressure | X29 | 76 | The weight of the air (in) | Max-min |
Precip. Rate | X30 | 77 | The rate at which the precipitation is falling (in) | Max-min |
Precip. Accum. | X31 | 78 | Amount of precipitation accumulated to that point in the day (in) | Max-min |
Gust | X32 | 79 | A brief increase in the speed of the wind (mph) | Max-min |
UV | X33 | 80 | The measure of the amount of UV radiation (1-10) | Max-min |
WIM features | ||||
Avg. Speed (mph) | X34 | 81 | The average vehicle speed in the hour across lanes in the direction of the crash (mph) | Max-min |
Traffic Volume | X35 | 82 | The total traffic volume for the hour that the crash occurred | Max-min |
Avg. Headway (s) | X36 | 83 | The average amount of time between one vehicle arriving and the next in the crash hour | Max-min |
Truck/Car Ratio | X37 | 84 | The number of trucks divided by the number of passenger cars in the hour of the crash | Max-min |
Avg. Weight (kg) | X38 | 85 | The average weight of all vehicles that passed by in the hour of the crash | Max-min |
Avg. PC Weight (kg) | X39 | 86 | The average weight of passenger cars (less than class 3) in the hour of the crash | Max-min |
Avg. Truck Weight (kg) | X40 | 87 | The average weight of trucks (greater than class 3) in the hour of the crash | Max-min |
Speed (mph) Variance | X41 | 88 | The variance of the speeds in the hour of the crash () | Max-min |
precision | recall | f1-score | support | |
SV crash | 0.90 | 0.62 | 0.73 | 29 |
MV crash | 0.84 | 0.97 | 0.90 | 61 |
macro avg | 0.87 | 0.79 | 0.82 | 90 |
weighted avg | 0.86 | 0.86 | 0.85 | 90 |
accuracy | 0.86 | 90 |
Variable | Coefficients | Odds ratio | Variable | Coefficients | Odds ratio | ||
X1 | 0 | 0.1549 | 1.1675 | X10 | 45 | 0.1561 | 1.1690 |
1 | -0.2346 | 0.7909 | 46 | -0.1558 | 0.8557 | ||
2 | 0.0214 | 1.0216 | X11 | 47 | 0.4133 | 1.5118 | |
3 | -0.0692 | 0.9331 | 48 | -0.2845 | 0.7524 | ||
4 | 0.1278 | 1.1364 | 49 | 0.1785 | 1.1955 | ||
X2 | 5 | -0.3487 | 0.7056 | 50 | -0.3269 | 0.7211 | |
6 | 0.5809 | 1.7876 | 51 | 0.0199 | 1.0201 | ||
7 | -2.4101 | 0.0898 | X12 | 52 | -0.5226 | 0.5930 | |
8 | 1.7908 | 5.9944 | X13 | 53 | 0.1123 | 1.1189 | |
9 | 1.0359 | 2.8176 | 54 | -0.0621 | 0.9398 | ||
10 | -0.6485 | 0.5228 | 55 | 0.3570 | 1.4291 | ||
X3 | 11 | -0.0740 | 0.9286 | 56 | -0.3138 | 0.7307 | |
12 | -0.2425 | 0.7847 | 57 | -0.0932 | 0.9110 | ||
13 | -0.1274 | 0.8803 | X14 | 58 | -0.0672 | 0.9350 | |
14 | 0.3442 | 1.4109 | X15 | 59 | -0.0717 | 0.9308 | |
15 | 0.1000 | 1.1052 | X16 | 60 | -0.2329 | 0.7922 | |
X4 | 16 | 0.1520 | 1.1642 | X17 | 61 | 0.6428 | 1.9018 |
17 | 0.2921 | 1.3392 | X18 | 62 | -0.6190 | 0.5385 | |
18 | -0.4438 | 0.6416 | X19 | 63 | 0.0000 | 1.0000 | |
X5 | 19 | 0.8060 | 2.2389 | X20 | 64 | -0.5602 | 0.5711 |
X6 | 20 | -0.5584 | 0.5721 | X21 | 65 | 0.7518 | 2.1209 |
21 | 0.4964 | 1.6429 | X22 | 66 | 0.0333 | 1.0339 | |
22 | 0.3154 | 1.3708 | X23 | 67 | -1.3206 | 0.2670 | |
23 | -0.2532 | 0.7763 | X24 | 68 | 0.4618 | 1.5869 | |
X7 | 24 | 0.2807 | 1.3240 | 69 | -0.0625 | 0.9394 | |
25 | 0.2059 | 1.2287 | 70 | -0.1843 | 0.8317 | ||
26 | -0.0579 | 0.9438 | 71 | -0.2147 | 0.8068 | ||
27 | -0.4284 | 0.6515 | X25 | 72 | -0.1734 | 0.8408 | |
X8 | 28 | 0.7363 | 2.0881 | X26 | 73 | 0.1871 | 1.2058 |
29 | 0.0435 | 1.0445 | X27 | 74 | 0.0173 | 1.0175 | |
30 | -0.9183 | 0.3992 | X28 | 75 | 0.6781 | 1.9701 | |
31 | 0.0554 | 1.0569 | X29 | 76 | -0.1184 | 0.8883 | |
32 | 0.4451 | 1.5607 | X30 | 77 | -0.1342 | 0.8744 | |
33 | 0.3136 | 1.3684 | X31 | 78 | 0.1089 | 1.1151 | |
34 | 0.0168 | 1.0169 | X32 | 79 | -0.0945 | 0.9098 | |
35 | 0.0000 | 1.0000 | X33 | 80 | -0.6698 | 0.5118 | |
36 | -0.8274 | 0.4372 | X34 | 81 | -2.2176 | 0.1089 | |
37 | -0.0134 | 0.9867 | X35 | 82 | 0.2651 | 1.3035 | |
38 | 0.1487 | 1.1603 | X36 | 83 | -0.2415 | 0.7854 | |
X9 | 39 | 0.0168 | 1.0169 | X37 | 84 | 0.5763 | 1.7794 |
40 | 0.5597 | 1.7501 | X38 | 85 | 0.2904 | 1.3370 | |
41 | -0.5986 | 0.5496 | X39 | 86 | 0.2285 | 1.2567 | |
42 | 0.0637 | 1.0658 | X40 | 87 | 0.2302 | 1.2588 | |
43 | 0.0291 | 1.0295 | X41 | 88 | 0.5835 | 1.7923 | |
44 | -0.0703 | 0.9321 |
Variable name | Type | Variable | Coefficient | Odds ratio | |
Location (Off Roadway) | Crash | X2 | 7 | -2.4101 | 0.0898 |
Avg. Speed (mph) | WIM | X34 | 81 | -2.2176 | 0.1089 |
Location (On Roadway - Non-Intersection) | Crash | X2 | 8 | 1.7908 | 5.9944 |
CrackPerc | Pavement | X23 | 67 | -1.3206 | 0.2670 |
Location (Entrance/Exit Ramp) | Crash | X2 | 9 | 1.0359 | 2.8176 |
Mnvr Veh1 Element (Negotiating A Curve) | Crash | X8 | 30 | -0.9183 | 0.3992 |
Mnvr Veh1 Element (Straight) | Crash | X8 | 36 | -0.8274 | 0.4372 |
Driver Age Element | Crash | X5 | 19 | 0.8060 | 2.2389 |
Heading | Pavement | X21 | 65 | 0.7518 | 2.1209 |
Mnvr Veh1 Element (Changing Lanes) | Crash | X8 | 28 | 0.7363 | 2.0881 |
Wind Speed | Weather | X28 | 75 | 0.6781 | 1.9701 |
UV | Weather | X33 | 80 | -0.6698 | 0.5118 |
Location (On Shoulder) | Crash | X2 | 10 | -0.6485 | 0.5228 |
RutAv | Pavement | X17 | 61 | 0.6428 | 1.9018 |
FAUAvg3D | Pavement | X18 | 62 | -0.6190 | 0.5385 |
U1 Traffic Control Element (Lanes) | Crash | X9 | 41 | -0.5986 | 0.5496 |
Speed (mph) Variance | WIM | X41 | 88 | 0.5835 | 1.7923 |
Location (On Roadway - Roadway Intersection) | Crash | X2 | 6 | 0.5809 | 1.7876 |
Truck/Car Ratio | WIM | X37 | 84 | 0.5763 | 1.7794 |
MacroText | Pavement | X20 | 64 | -0.5602 | 0.5711 |
U1 Traffic Control Element (Traffic Signal) | Crash | X9 | 40 | 0.5597 | 1.7501 |
Veh Type Element (Unknown) | Crash | X6 | 20 | -0.5584 | 0.5721 |
NumLanes | Pavement | X12 | 52 | -0.5226 | 0.5930 |
Veh Type Element (Passenger Car) | Crash | X6 | 21 | 0.4964 | 1.6429 |
CurveB | Pavement | X24 | 68 | 0.4618 | 1.5869 |