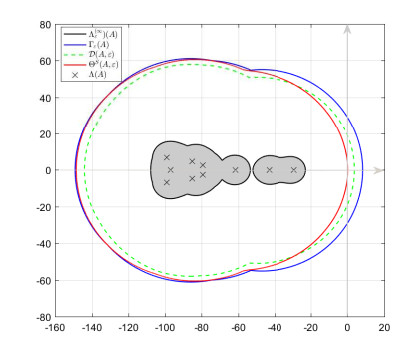
A new class of matrices called partially doubly strictly diagonally dominant (for shortly, PDSDD) matrices is introduced and proved to be a subclass of nonsingular H-matrices, which generalizes doubly strictly diagonally dominant matrices. As applications, a new eigenvalue localization set for matrices is given, and an upper bound for the infinity norm bound of the inverse of PDSDD matrices is presented. Based on this bound, a new pseudospectra localization for matrices is derived and a lower bound for distance to instability is obtained.
Citation: Yi Liu, Lei Gao, Tianxu Zhao. Partially doubly strictly diagonally dominant matrices with applications[J]. Electronic Research Archive, 2023, 31(5): 2994-3013. doi: 10.3934/era.2023151
[1] | Jiaqi Qi, Yaqiang Wang . Subdirect Sums of $ GSD{D_1} $ matrices. Electronic Research Archive, 2024, 32(6): 3989-4010. doi: 10.3934/era.2024179 |
[2] | Yan Li, Yaqiang Wang . Some new results for $ B_1 $-matrices. Electronic Research Archive, 2023, 31(8): 4773-4787. doi: 10.3934/era.2023244 |
[3] | Natália Bebiano, João da Providência, Wei-Ru Xu . Approximations for the von Neumann and Rényi entropies of graphs with circulant type Laplacians. Electronic Research Archive, 2022, 30(5): 1864-1880. doi: 10.3934/era.2022094 |
[4] | Zoran Pucanović, Marko Pešović . Analyzing Chebyshev polynomial-based geometric circulant matrices. Electronic Research Archive, 2024, 32(9): 5478-5495. doi: 10.3934/era.2024254 |
[5] | Jiachen Mu, Duanzhi Zhang . Multiplicity of symmetric brake orbits of asymptotically linear symmetric reversible Hamiltonian systems. Electronic Research Archive, 2022, 30(7): 2417-2427. doi: 10.3934/era.2022123 |
[6] | Xiuyun Guo, Xue Zhang . Determinants and invertibility of circulant matrices. Electronic Research Archive, 2024, 32(7): 4741-4752. doi: 10.3934/era.2024216 |
[7] | Yongge Tian . Characterizations of matrix equalities involving the sums and products of multiple matrices and their generalized inverse. Electronic Research Archive, 2023, 31(9): 5866-5893. doi: 10.3934/era.2023298 |
[8] | Daochang Zhang, Dijana Mosić, Liangyun Chen . On the Drazin inverse of anti-triangular block matrices. Electronic Research Archive, 2022, 30(7): 2428-2445. doi: 10.3934/era.2022124 |
[9] | Shunjie Bai, Caili Sang, Jianxing Zhao . Localization and calculation for C-eigenvalues of a piezoelectric-type tensor. Electronic Research Archive, 2022, 30(4): 1419-1441. doi: 10.3934/era.2022074 |
[10] | D. Mosić, P. S. Stanimirović, L. A. Kazakovtsev . The $ m $-weak group inverse for rectangular matrices. Electronic Research Archive, 2024, 32(3): 1822-1843. doi: 10.3934/era.2024083 |
A new class of matrices called partially doubly strictly diagonally dominant (for shortly, PDSDD) matrices is introduced and proved to be a subclass of nonsingular H-matrices, which generalizes doubly strictly diagonally dominant matrices. As applications, a new eigenvalue localization set for matrices is given, and an upper bound for the infinity norm bound of the inverse of PDSDD matrices is presented. Based on this bound, a new pseudospectra localization for matrices is derived and a lower bound for distance to instability is obtained.
A matrix A=[aij]∈Cn×n is called a strictly diagonally dominant (SDD) matrix if
|aii|>ri(A) | (1.1) |
for all i∈N:={1,…,n}, where ri(A)=∑j∈N∖{i}|aij|.
The concept of SDD originated from the well-known Lévy-Desplanques Theorem [1], which states that if condition (1.1) holds, then A is nonsingular, i.e., SDD matrices are nonsingular. It is well known that the class of SDD matrices has wide applications in many fields of scientific computing, such as the Schur complement problem [2,3], eigenvalue localizations [4,5,6,7,8,9,10], convergence analysis of the parallel-in-time iterative method [11], estimating the infinity norm for the inverse of H-matrices [12,13,14,15], error bound for linear complementarity problems [16,17], structure tensors [18,19], etc.
Some well-known matrices have been presented and studied [4,7,20] by breaking the diagonal dominance condition (1.1). For instance, by allowing at most one row to be non-SDD, Ostrowski introduced the class of Ostrowski matrices [20] (also known as doubly strictly diagonally dominant (DSDD) matrices). Here, a matrix A=[aij]∈Cn×n is an Ostrowski matrix [20] if for all j≠i,
|aii||ajj|>ri(A)rj(A). |
In addition, based on the partition-based approach that allows more than one row to be non-SDD, a well-known class of matrices called S-SDD matrices has been proposed and studied [7,21].
Definition 1.1. [7,21] Let A=[aij]∈Cn×n and S be a nonempty proper subset of N. Then, a matrix A is called an S-SDD matrix if
{|aii|>rSi(A),i∈S,(|aii|−rSi(A))(|ajj|−r¯Sj(A))>r¯Si(A)rSj(A),i∈S,j∈¯S, |
where rSi(A)=∑j∈S∖{i}|aij|.
Besides DSDD matrices and S-SDD matrices, there are many generalizations of SDD matrices, such as Nekrasov matrices [22,23], DZ-type matrices [24], CKV-type matrices [25] and so on.
Observe from Definition 1.1 that S-SDD matrices only consider the effect of "interaction" between i∈S and j∈¯S on the nonsingular. However, other "interaction, " such as the "constraint condition" between i∈S (i∈¯S) and j∈S (j∈¯S) with i≠j, might also affect the non-singularity of the matrix. Naturally, an interesting question arises: When we consider this "constraint condition, " can we get the non-singularity of the matrix? To answer this question, in this paper, we introduce a new class of nonsingular matrices arising from this "constraint condition" and show several benefits from this new class of matrices, which we will call partially doubly strictly diagonally dominant matrices.
This paper is organized as follows. In Section 2, we present a new class of matrices called PDSDD matrices and prove that it is a subclass of nonsingular H-matrices, which is similar to, but different from, the class of S-SDD matrices. Section 3 gives a new eigenvalue localization set for matrices and presents an infinity norm bound for the inverse of PDSDD matrices. It is proved that the obtained bound is better than the well-known Varah's bound for SDD matrices. Based on this infinity norm bound, we also obtain a new pseudospectra localization for matrices and apply it to measure the distance to instability. Finally, we give concluding remarks in Section 4.
We start with some preliminaries and definitions. Let Zn×n be the set of all matrices A=[aij]∈Rn×n with aij≤0 and i≠j. A matrix A∈Zn×n is called a nonsingular M-matrix if its inverse is nonnegative, i.e., A−1≥0 [26]. A matrix A=[aij]∈Cn×n is called a nonsingular H-matrix [26] if its comparison matrix M(A)=[mij]∈Rn×n defined by
mij={|aij|,i=j,−|aij|,i≠j, |
is a nonsingular M-matrix. Let |N| be the cardinality of set N.
In the following, we define a new class of matrices called PDSDD matrices.
Definition 2.1. Let S be a subset of N and ¯S be the complement of S. Given a matrix A=[aij]∈Cn×n, for each subset Δ∈{S,¯S}, denote Δ−:={i∈Δ|aii≤ri(A)} and Δ+:={i∈Δ|aii>ri(A)}. Then, a matrix A is called a partially doubly strictly diagonally dominant (PDSDD) matrix if for each Δ∈{S,¯S} either |Δ−|=0 or |Δ−|=1, and for i∈Δ−,
{|aii|>r¯Δi(A),(|aii|−r¯Δi(A))(|ajj|−rΔj(A)+|aji|)>rΔi(A)(r¯Δj(A)+|aji|),j∈Δ+, | (2.1) |
where rΔi(A)=∑j∈Δ∖{i}|aij|.
Note that the class of DSDD matrices allows at most one row to be non-SDD, whereas the class of matrices defined in Definition 2.1 allows at most one row to be non-SDD in each subset Δ∈{S,¯S}. For this reason, we call it the class of PDSDD matrices.
The following theorem provides that the class of PDSDD matrices is a subclass of nonsingular H-matrices.
Theorem 2.1. Every PDSDD matrix is a nonsingular H-matrix.
Proof. According to the well-known result that SDD matrices are nonsingular H-matrices, it is sufficient to consider the case that A has one or two non-SDD rows. Assume, on the contrary, that A is a singular matrix, and then there exists a nonzero eigenvector x=[x1,x2,…,xn]T corresponding to 0 eigenvalue such that
Ax=0. | (2.2) |
Let |xp|:=maxi∈N{|xi|}. Then, |xp|>0, and p∈Δ∪¯Δ, where Δ∈{S,¯S}. Without loss of generality, we assume that Δ=S. Next, we need only consider the case p∈S, and another case p∈¯S can be proved similarly. For p∈S, it follows from Definition 2.1 that p∈S−∪S+, where S−:={i∈S|aii≤ri(A)} and S+:={i∈S|aii>ri(A)}.
If p∈S−, then by Definition 2.1 we have
|app|>r¯Sp(A), | (2.3) |
and for all j∈S+,
(|app|−r¯Sp(A))(|ajj|−rSj(A)+|ajp|)>rSp(A)(r¯Sj(A)+|ajp|). | (2.4) |
Note that |S−|=1. Then, S∖{p}=S+. Let |xq|:=maxk∈S∖{p}{|xk|}. Considering the p-th equality of (2.2), we have
appxp=−∑k≠p,k∈Sapkxk−∑k≠p,k∈¯Sapkxk. |
Taking the modulus in the above equation and using the triangle inequality yields
|app||xp|≤∑k≠p,k∈S|apk||xk|+∑k≠p,k∈¯S|apk||xk|≤∑k≠p,k∈S|apk||xq|+∑k≠p,k∈¯S|apk||xp|=rSp(A)|xq|+r¯Sp(A)|xp|, |
which implies that
(|app|−r¯Sp(A))|xp|≤rSp(A)|xq|. | (2.5) |
If |xq|=0, then |app|−r¯Sp(A)≤0 as |xp|>0, which contradicts (2.3). If |xq|≠0, then from the q-th equality of (2.2), we obtain
|aqq||xq|≤∑k≠q,k∈S|aqk||xk|+∑k≠q,k∈¯S|aqk||xk|≤(∑k≠q,k∈S|aqk|−|aqp|)|xq|+(∑k≠q,k∈¯S|aqk|+|aqp|)|xp|=(rSq(A)−|aqp|)|xq|+(r¯Sq(A)+|aqp|)|xp|, |
i.e.,
(|aqq|−rSq(A)+|aqp|)|xq|≤(r¯Sq(A)+|aqp|)|xp|. | (2.6) |
Multiplying (2.6) with (2.5) and dividing by |xp||xq|>0, we have
(|app|−r¯Sp(A))(|aqq|−rSq(A)+|aqp|)≤rSp(A)(r¯Sq(A)+|aqp|), |
which contradicts (2.4).
If p∈S+, then |app|>rp(A). Considering the p-th equality of (2.2) and using the triangle inequality, we obtain
|app||xp|≤∑k≠p|apk||xk|≤rp(A)|xp|, |
i.e., |app|≤rp(A), which contradicts |app|>rp(A). Hence, we can conclude that 0 is not an eigenvalue of A, that is, A is a nonsingular matrix.
We next prove that A is a nonsingular H-matrix. For any ε≥0, let
Bε=M(A)+εI=[bij]. |
Note that bii=|aii|+ε, bij=−|aij|, and Bε∈Zn×n. Then, Δ−(Bε)⊆Δ−(A), and Δ+(A)⊆Δ+(Bε). If A is an SDD matrix, then Δ−(A)=∅, and thus Δ−(Bε)=∅, which implies that Bε is an SDD matrix. If A is not an SDD matrix, then |Δ−(A)|=1 for some Δ∈{S,¯S}. For this case, |Δ−(Bε)|=0 or |Δ−(Bε)|=1. If |Δ−(Bε)|=0 for each Δ∈{S,¯S}, then Bε is also an SDD matrix. If |Δ−(Bε)|=1 for some Δ∈{S,¯S}, then Δ−(Bε)=Δ−(A) and Δ+(Bε)=Δ+(A). Hence, for i∈Δ−(Bε),
|bii|−r¯Δi(Bε)=|aii|+ε−r¯Δi(A)>0, |
and for all j∈Δ+(Bε),
(|bii|−r¯Δi(Bε))(|bjj|−rΔj(Bε)+|bji|)=(|aii|+ε−r¯Δi(A))(|ajj|+ε−rΔj(A)+|aji|)≥(|aii|−r¯Δi(A))(|ajj|−rΔj(A)+|aji|)>rΔi(A)(r¯Δj(A)+|aji|)=r¯Δi(Bε)(rΔj(Bε)+|bji|). |
Therefore, Bε is a PDSDD matrix and thus nonsingular for each ε≥0. This implies that M(A) is a nonsingular M-matrix (see the condition (D15) of Theorem 2.3 in [26, Chapter 6]). Therefore, A is a nonsingular H-matrix. The proof is complete.
Proposition 2.1. Every DSDD matrix is a PDSDD matrix.
Proof. If A is an SDD matrix, then A is a PDSDD matrix. If A is not an SDD matrix, then by the assumptions it holds that there exists i0∈N such that |ai0i0|≤ri0(A) and
|ai0i0||ajj|>ri0(A)rj(A)for allj≠i0. |
Taking S=N, we have r¯Si(A)=0 and rSi(A)=ri(A) for all i∈N. This implies that for all j∈S∖{i0},
(|ai0i0|−r¯Si0(A))(|ajj|−rSj(A)+|aji0|)−rSi0(A)(r¯Sj(A)+|aji0|)=|ai0i0|(|ajj|−rj(A)+|aji0|)−ri0(A)|aji0|=|ai0i0||ajj|−|ai0i0|(rj(A)−|aji0|)−ri0(A)|aji0|≥|ai0i0||ajj|−ri0(A)(rj(A)−|aji0|+|aji0|)=|ai0i0||ajj|−ri0(A)rj(A)>0. |
Hence, by Definition 2.1, we conclude that A is a PDSDD matrix. The proof is complete.
Next, we give an example to show that neither PDSDD matrices nor S-SDD matrices are included in each other.
Example 2.1. Consider the following matrices:
A=[3−1−30−130−80−1300008],B=[300−3030−3003−30008]. |
By calculation, we know that A is a PDSDD matrix for S={1,3}, but it is not an S-SDD matrix for any nonempty proper subset S of N and thus not a DSDD matrix. Meanwhile, B is an S-SDD matrix for S={1,2,3}, but it is not a PDSDD matrix, because B has three non-SDD rows.
According to Theorem 2.1, Proposition 2.1 and Example 2.1, the relations among DSDD matrices, PDSDD matrices, S-SDD matrices and H-matrices can be depicted as follows:
{PDSDD}⊂{H},{PDSDD}⊄{S-SDD},{S-SDD}⊄{PDSDD}, |
and
{DSDD}⊂{PDSDD}∩{S-SDD}. |
It is well known that the non-singularity of matrices can generate the equivalent eigenvalue inclusion set in the complex plane [4,5,7,9,24]. By the non-singularity of PDSDD matrices, we in this section give a new eigenvalue localization set for matrices. Before that, an equivalent condition for the definition of PDSDD matrices is given, which can be proved immediately from Definition 2.1.
Lemma 3.1. Let A=[aij]∈Cn×n and {S,¯S} be a partition of the set N. A matrix A is called a PDSDD matrix if and only if S⋆ is not empty unless S is empty, and ¯S⋆ is not empty unless ¯S is empty, where
S⋆:={i∈S:|aii|>r¯Si(A),andforallj∈S∖{i},|ajj|>rj(A)and(|aii|−r¯Si(A))(|ajj|−rSj(A)+|aji|)>rSi(A)(r¯Sj(A)+|aji|)}, |
and
¯S⋆:={i∈¯S:|aii|>rSi(A),andforallj∈¯S∖{i},|ajj|>rj(A)and(|aii|−rSi(A))(|ajj|−r¯Sj(A)+|aji|)>r¯Si(A)(rSj(A)+|aji|)} |
with rSi(A)=∑j∈S∖{i}|aij|.
By Lemma 3.1, we can obtain the following theorem.
Theorem 3.1. Let A=[aij]∈Cn×n and S be any subset of N. Then,
σ(A)⊆ΘS(A):=θS(A)⋃θ¯S(A), |
where σ(A) is the set of all the eigenvalues of A,
θS(A)=⋂i∈S(Γ¯Si(A))⋃(⋃j∈S∖{i}(ˆV¯Sij(A)∪Γj(A))) |
and
θ¯S(A)=⋂i∈¯S(ΓSi(A))⋃(⋃j∈¯S∖{i}(ˆVSij(A)∪Γj(A))) |
with
ΓSi(A):={z∈C:|z−aii|≤rSi(A)},Γj(A):={z∈C:|z−ajj|≤rj(A)}, |
and
ˆVSij(A):={z∈C:(|z−aii|−rSi(A))(|z−ajj|−r¯Sj(A)+|aji|)≤r¯Si(A)(rSj(A)+|aji|)}. |
Proof. Without loss of generality, we assume that S is a nonempty subset of N. For the case of S=∅, we have ΘS(A)=θ¯S(A), and the conclusion can be proved similarly. Suppose, on the contrary, that there exists an eigenvalue λ of A such that λ∉ΘS(A), that is, λ∉θS(A) and λ∉θ¯S(A). For λ∉θS(A), there exists an index i∈S such that λ∉Γ¯Si(A), and for all j∈S∖{i}, λ∉Γj(A) and λ∉ˆV¯Sij(A), that is, |λ−aii|>r¯Si(A), |λ−ajj|>rj(A), and
(|λ−aii|−r¯Si(A))(|λ−ajj|−rSj(A)+|aji|)>rSi(A)(r¯Sj(A)+|aji|). |
Similarly, for λ∉θ¯S(A), there exists an index i∈¯S such that λ∉ΓSi(A), and for all j∈¯S∖{i}, λ∉Γj(A) and λ∉ˆVSij(A), that is, |λ−aii|>rSi(A), |λ−ajj|>rj(A), and
(|λ−aii|−rSi(A))(|λ−ajj|−r¯Sj(A)+|aji|)>r¯Si(A)(rSj(A)+|aji|). |
These imply that S⋆(λI−A) and ¯S⋆(λI−A) are not empty. It follows from Lemma 3.1 that λI−A is a PDSDD matrix. Then, by Theorem 2.1, λI−A is nonsingular, which contradicts that λ is an eigenvalue of A. Hence, λ∈ΘS(A). This completes the proof.
Remark 3.1. Take the intersection over all possible subsets S of N, and we can get a satisfactory eigenvalue localization although it has more computation costs:
σ(A)⊆Θ(A):=⋂S⊆NΘS(A). |
To compare our set Θ(A) with the Geršgorin disks Γ(A) in [8], Brauer's ovals of Cassini K(A) in [27] and the Cvetković-Kostić-Varga eigenvalue localization set C(A) in [7], }let us recall the definitions of Γ(A), K(A) and C(A) as follows.
Theorem 3.2. [8] Let A=[aij]∈Cn×n and σ(A) be the set of all eigenvalues of A. Then,
σ(A)⊆Γ(A):=⋃i∈NΓi(A), |
where Γi(A)={z∈C:|aii−z|≤ri(A)}.
Theorem 3.3. [27] Let A=[aij]∈Cn×n and σ(A) be the set of all eigenvalues of A. Then,
σ(A)⊆K(A):=⋃i,j∈N,i≠jKij(A), |
where Kij(A)={z∈C:|aii−z||ajj−z|≤ri(A)rj(A)}.
Theorem 3.4. [7] Let S be any nonempty proper subset of N, and n≥2. Then, for any A=[aij]∈Cn×n, all the eigenvalues of A belong to set
CS(A)=(⋃i∈SΓSi(A))⋃(⋃i∈S,j∈¯SVSij(A)), |
and hence
σ(A)⊆C(A):=⋂S⊂N,S≠∅,S≠NCS(A), |
where ΓSi(A) is given by Theorem 3.1, and
VSij(A):={z∈C:(|z−aii|−rSi(A))(|z−ajj|−r¯Sj(A))≤r¯Si(A)rSj(A)}. |
Remark 3.2. Observe that the class of SDD matrices is a subclass of DSDD matrices, which is a subclass of of PDSDD matrices. Hence, the corresponding set Θ(A) will contain Brauer's ovals of Cassini K(A), which contains the Geršgorin disks Γ(A), that is,
Θ(A)⊆K(A)⊆Γ(A). |
In addition, because PDSDD class and S-SDD class do not contain each other, it follows that the relation between Θ(A) and C(A) is
Θ(A)⊄C(A)andC(A)⊄Θ(A). |
In this section, we consider the infinity norm bounds for the inverse of PDSDD matrices, since it might be used for the convergence analysis of matrix splitting and matrix multi-splitting iterative methods for solving large sparse systems of linear equations, linear complementarity problems and pseudospectra localizations.
Theorem 3.5. Let A=[aij]∈Cn×n be a PDSDD matrix. Then,
||A−1||∞≤max{mini∈S⋆max{1|aii|−r¯Si(A),φSi(A)},mini∈¯S⋆max{1|aii|−rSi(A),φ¯Si(A)}}, | (3.1) |
where S⋆ and ¯S⋆ are defined by Lemma 3.1,
φSi(A):=maxj∈S∖{i}{XSij(A),1|ajj|−rj(A)} |
with
XSij(A):=|ajj|−rSj(A)+|aji|+rSi(A)(|aii|−r¯Si(A))(|ajj|−rSj(A)+|aji|)−rSi(A)(r¯Sj(A)+|aji|). |
Proof. According to a well-known fact (see [10,30]),
||A−1||−1∞=infx≠0||Ax||∞||x||∞=min||x||∞=1||Ax||∞=||Ax∗||∞=maxi∈N|(Ax∗)i| |
for some x∗=[x∗1,x∗2,…,x∗n]T such that ||x||∞=1. Denote |x∗p|=||x||∞=1. It follows that
||A−1||−1∞≥|(Ax∗)p|. |
Consider the p-th row of Ax∗, and we have
(Ax∗)p=appx∗p+∑j≠papjx∗j. | (3.2) |
Since A is a PDSDD matrix, it follows from Lemma 3.1 that S⋆ is not empty unless S is empty, and ¯S⋆ is not empty unless ¯S is empty. We only consider the case both S and ¯S are not empty, and the case of S=∅ or ¯S=∅ can be proved similarly.
The first case. Suppose that either S or ¯S is a singleton set. We assume that S={k}. Then, ¯S=N∖{k}, and from Lemma 3.1 it holds that S⋆ is not empty and ¯S⋆ is not empty, that is,
|akk|>r¯Sk(A)=rk(A). |
For each i0∈¯S⋆, |ai0i0|>rSi0(A), and for all j∈¯S∖{i0},
(|ai0i0|−rSi0(A))(|ajj|−r¯Sj(A)+|aji0|)>r¯Si(A)(rSj(A)+|aji0|). | (3.3) |
Note that p∈S∪¯S. If p∈S={k}, then
|app|≤|(Ax∗)p|+rp(A), |
and
||A−1||−1∞≥|(Ax∗)p|≥|app|−rp(A)>0. |
Hence,
||A−1||∞≤1|app|−rp(A)=1|akk|−r¯Sk(A). | (3.4) |
If p∈¯S, then p=i0 or p≠i0, where i0∈¯S⋆. If p=i0, then let |x∗q|=maxj∈¯S∖{p}{|x∗j|}. By (3.2), it follows that
appx∗p=(Ax∗)p−∑j≠p,j∈Sapjx∗j−∑j≠p,j∈¯Sapjx∗j, |
and taking absolute values on both sides and using the triangle inequality, we get
|app||x∗p|≤|(Ax∗)p|+∑j≠p,j∈S|apj||x∗j|+∑j≠p,j∈¯S|apj||x∗j|≤|(Ax∗)p|+∑j≠p,j∈S|apj||x∗p|+∑j≠p,j∈¯S|apj||x∗q|≤||A−1||−1∞+rSp(A)|x∗p|+r¯Sp(A)|x∗q|, |
which implies that
|app|−||A−1||−1∞−rSp(A)≤r¯Sp(A)|x∗q|. | (3.5) |
Consider the q-th row of Ax∗, and we have
(Ax∗)q=aqqx∗q+∑j≠qaqjx∗j. |
It follows that
|aqq||x∗q|≤|(Ax∗)q|+(∑j≠q,j∈¯S|aqj|−|aqp|)|x∗q|+(∑j≠q,j∈S|aqj|+|aqp|)|x∗p|≤||A−1||−1∞+(r¯Sq(A)−|aqp|)|x∗q|+(rSq(A)+|aqp|), |
i.e.,
(|aqq|−r¯Sq(A)+|aqp|)|x∗q|≤||A−1||−1∞+(rSq(A)+|aqp|). | (3.6) |
Then, from (3.5) and (3.6), we get that
|app|−||A−1||−1∞−rSp(A)r¯Sp(A)≤|x∗q|≤||A−1||−1∞+rSq(A)+|aqp||aqq|−r¯Sq(A)+|aqp|, |
which implies that
||A−1||∞≤|aqq|−r¯Sq(A)+|aqp|+r¯Sp(A)(|app|−rSp(A))(|aqq|−r¯Sq(A)+|aqp|)−r¯Sp(A)(rSq(A)+|aqp|)≤maxj∈¯S∖{i0}|ajj|−r¯Sj(A)+|aji0|+r¯Si0(A)(|ai0i0|−rSi0(A))(|ajj|−r¯Sj(A)+|aji0|)−r¯Si0(A)(rSj(A)+|aji0|):=maxj∈¯S∖{i0}X¯Si0j(A). | (3.7) |
If p≠i0, then |app|>rp(A). Similarly to the proof of (3.4), we obtain
||A−1||∞≤1|app|−rp(A)≤maxj∈¯S∖{i0}1|ajj|−rj(A). |
Hence,
||A−1||∞≤maxj∈¯S∖{i0}{X¯Si0j(A),1|ajj|−rj(A)}. |
Since i0 is arbitrary in ¯S⋆, it follows that
||A−1||∞≤mini∈¯S⋆maxj∈¯S∖{i}{X¯Sij(A),1|ajj|−rj(A)}. | (3.8) |
By (3.4) and (3.8), it holds that
||A−1||∞≤max{mini∈S⋆1|aii|−r¯Si(A),mini∈¯S⋆maxj∈¯S∖{i}{X¯Sij(A),1|ajj|−rj(A)}}. |
If ¯S is a singleton set, then similar to the above case, we obtain
||A−1||∞≤max{mini∈S⋆maxj∈S∖{i}{XSij(A),1|ajj|−rj(A)},mini∈¯S⋆1|aii|−rSi(A)}. |
The second case. Suppose that both S and ¯S are singleton sets. By Lemma 3.1, it follows that S⋆ is not empty, and ¯S⋆ is not empty. Then, similar to the proof of the first case, we have
||A−1||∞≤mini∈S⋆maxj∈S∖{i}{XSij(A),1|ajj|−rj(A)}, |
and
||A−1||∞≤mini∈¯S⋆maxj∈¯S∖{i}{X¯Sij(A),1|ajj|−rj(A)}. |
Now, the conclusion follows from the above two cases.
In [15], an elegant upper bound for the inverse of SDD matrices is presented.
Theorem 3.6. [15] Let A=[aij]∈Cn×n be an SDD matrix. Then,
||A−1||∞≤maxi∈N1|aii|−ri(A). |
This bound is usually called Varah's bound and plays a critical role in numerical algebra [11,16,28,29]. As discussed before, an SDD matrix is a PDSDD matrix as well. Thus, Theorem 3.5 can be applied to SDD matrices. In the following, we show that bound (3.1) of Theorem 3.5 works better than Varah's bound of Theorem 3.6.
Theorem 3.7. Let A=[aij]∈Cn×n be an SDD matrix and S be any subset of N. Then,
||A−1||∞≤φS(A)≤maxi∈N1|aii|−ri(A), |
where
φS(A):=max{mini∈Smax{1|aii|−r¯Si(A),φSi(A)},mini∈¯Smax{1|aii|−rSi(A),φ¯Si(A)}} |
and φSi(A) is given by Theorem 3.5.
Proof. Since A is an SDD matrix, it follows from Lemma 3.1 that S⋆=S for any subset S of N. Hence, by Theorem 3.5, it follows that
||A−1||∞≤φS(A). |
We next prove that φS(A)≤maxi∈N1|aii|−ri(A). Define |ai0i0|−ri0(A):=mini∈N{|aii|−ri(A)}. Obviously, for each i∈N,
1|aii|−rSi(A)≤1|ai0i0|−ri0(A)and1|aii|−r¯Si(A)≤1|ai0i0|−ri0(A). |
Note that for each i∈S,
φSi(A):=maxj∈S∖{i}{XSij(A),1|ajj|−rj(A)} | (3.9) |
and
XSij(A):=|ajj|−rSj(A)+|aji|+rSi(A)(|aii|−r¯Si(A))(|ajj|−rSj(A)+|aji|)−rSi(A)(r¯Sj(A)+|aji|). |
If |aii|−ri(A)≥|ajj|−rj(A), then
(|aii|−r¯Si(A))(|ajj|−rSj(A)+|aji|)−rSi(A)(r¯Sj(A)+|aji|)=(|aii|−ri(A))(|ajj|−rSj(A)+|aji|)+rSi(A)(|ajj|−rj(A))≥(|ajj|−rj(A))(|ajj|−rSj(A)+|aji|)+rSi(A)(|ajj|−rj(A))=(|ajj|−rj(A))(|ajj|−rSj(A)+|aji|+rSi(A)), |
which implies that
XSij(A)≤1|ajj|−rj(A)≤1|ai0i0|−ri0(A). | (3.10) |
If |aii|−ri(A)<|ajj|−rj(A), then
(|aii|−r¯Si(A))(|ajj|−rSj(A)+|aji|)−rSi(A)(r¯Sj(A)+|aji|)=(|aii|−ri(A))(|ajj|−rSj(A)+|aji|)+rSi(A)(|ajj|−rj(A))>(|aii|−ri(A))(|ajj|−rSj(A)+|aji|)+rSi(A)(|aii|−ri(A))=(|aii|−ri(A))(|ajj|−rSj(A)+|aji|+rSi(A)), |
which implies that
1|ajj|−rj(A)<XSij(A)<1|aii|−ri(A)≤1|ai0i0|−ri0(A). | (3.11) |
By (3.9), (3.10) and (3.11), it follows that for each i∈S,
φSi(A)≤1|ai0i0|−ri0(A). |
Similarly, for each i∈¯S, we can see that
φ¯Si(A)≤1|ai0i0|−ri0(A). |
This completes the proof.
Remark 3.3. For SDD matrices, consider the intersection over all possible subsets S of N, and a tighter upper bound for ||A−1||∞ can be obtained from Theorem 3.7:
||A−1||∞≤minS⊆NφS(A)≤φS(A)≤maxi∈N1|aii|−ri(A). |
Besides SDD matrices, for other subclasses of H-matrices, such as DSDD matrices, S-SDD matrices and Nekrasov matrices, various infinity norm bounds for their inverse have also been derived. For details, see [12,23,28,29,30,31,32,33] and references therein. A numerical example is given to illustrate the advantage of the proposed bound in Theorem 3.6.
Example 3.1. Consider the matrix in Example 2.1:
A=[3−1−30−130−80−1300008]. |
By computation, we know that A is a PDSDD matrix for S={1,3} but neither SDD nor a Nekrasov matrix. Moreover, it is easy to verify that there is no nonempty proper subset S of N such that A is an S-SDD matrix, and so it is not a DSDD matrix. Therefore, neither of the existing bounds for SDD, DSDD, S-SDD, and Nekrasov matrices can be used to estimate ||A−1||∞. However, by our bound (3.1), we have
||A−1||∞≤2. |
The exact value of the infinity norm of the inverse of A is ||A−1||∞=1.
For a given ε>0, denoted by
Λε(A)={λ∈C:∃x∈Cn∖{0},E∈Cn×n,||E||≤εsuchthat(A+E)x=λx}, |
the ε-pseudospectrum of a matrix A consists of all eigenvalues of matrices [30], which is equivalent to
Λε(A)={z∈C:||(A−zI)−1||−1≤ε}, | (3.12) |
where the convention is ||A−1||−1=0 if A is singular [30]. This implies that the infinity norm bounds of the inverse of a given matrix could be used to generate new pseudospectra localizations. For details, see [13,25,30]. So, in this section, we shall give a new pseudospectra localization using the obtained bound in Section 3.2. Before that, a useful lemma is given that will be used later.
Lemma 3.2. Let A be an arbitrary matrix and S be any subset of N. Then,
||A−1||−1∞≥μ(A):=min{f(A),g(A)}, |
where
f(A):=maxi∈Smin{|aii|−r¯Si(A),minj∈S∖{i}{μSij(A),|ajj|−rj(A)}} |
and
g(A):=maxi∈¯Smin{|aii|−rSi(A),minj∈¯S∖{i}{μ¯Sij(A),|ajj|−rj(A)}} |
with the convention ||A−1||−1∞ if A is singular, and μSij(A)=0 if |ajj|−rSj(A)+|aji|+rSi(A)=0; otherwise,
μSij(A)=(|aii|−r¯Si(A))(|ajj|−rSj(A)+|aji|)−rSi(A)(r¯Sj(A)+|aji|)|ajj|−rSj(A)+|aji|+rSi(A). |
Proof. For any given subset S of N, if A is a PDSDD matrix, then it follows from Lemma 3.1 and Theorem 3.5 that
||A−1||−1∞≥min{maxi∈S⋆min{|aii|−r¯Si(A),minj∈S∖{i}{μSij(A),|ajj|−rj(A)}},maxi∈¯S⋆min{|aii|−rSi(A),minj∈¯S∖{i}{μ¯Sij(A),|ajj|−rj(A)}}}=μ(A). |
If A is not a PDSDD matrix, then it follows from Lemma 3.1 that at least one of the following conditions holds: (i) |aii|≤r¯Si(A) for all i∈S; (ii) |aii|>r¯Si(A) for some i∈S; but for some j∈S∖{i}, |ajj|≤rj(A) or
(|aii|−r¯Si(A))(|ajj|−rSj(A)+|aji|)≤rSi(A)(r¯Sj(A)+|aji|); |
(iii) |aii|≤rSi(A) for all i∈¯S; (iv) |aii|>rSi(A) for some i∈¯S; but for some j∈¯S∖{i}, |ajj|≤rj(A) or
(|aii|−rSi(A))(|ajj|−r¯Sj(A)+|aji|)≤r¯Si(A)(rSj(A)+|aji|). |
This implies that μ(A)≤0≤||A−1||−1∞. The proof is complete.
Now, a new pseudospectra localization for matrices is given based on Lemma 3.2.
Theorem 3.8. (ε-pseudo PDSDD set) Let A=[aij]∈Cn×n and S be any subset of N. Then,
Λε(A)⊆ΘS(A,ε):=θS(A,ε)⋃θ¯S(A,ε), |
where
θS(A,ε):=⋂i∈S(Γ¯Si(A,ε)⋃(⋃j∈S∖{i}(ˆV¯Sij(A,ε)∪Γj(A,ε)))) |
and
θ¯S(A,ε):=⋂i∈¯S(ΓSi(A,ε)⋃(⋃j∈¯S∖{i}(ˆVSij(A,ε)∪Γj(A,ε)))) |
with
ΓSi(A,ε):={z∈C:|z−aii|≤rSi(A)+ε}, |
Γj(A,ε):={z∈C:|z−ajj|≤rj(A)+ε}, |
and
ˆVSij(A,ε):={z∈C:(|z−aii|−rSi(A)−ε)(|z−ajj|−r¯Sj(A)+|aji|)≤r¯Si(A)(rSj(A)+|aji|+ε)}. |
Proof. From Lemma 3.2 and (3.12), we immediately get
Λε(A)={z∈C:||(A−zI)−1||−1≤ε}⊆{z∈C:μ(A−zI)≤ε}, | (3.13) |
where μ(A−zI) is defined as in Lemma 3.2. Note that rSi(A−zI)=rSi(A) and r¯Si(A−zI)=r¯Si(A). Therefore, for any λ∈Λε(A), it follows from (3.13) that
f(A−λI)≤εorg(A−λI)≤ε, |
where f(A−λI) and g(A−λI) are given by Lemma 3.2.
Case Ⅰ. If f(A−λI)≤ε, then for all i∈S, |aii−λ|≤r¯Si(A)+ε or |aii−λ|>r¯Si(A)+ε for some i∈S; but for some j∈S∖{i}, |ajj−λ|≤rj(A)+ε or μSij(A−λI)≤ε, i.e.,
(|aii−λ|−r¯Si(A))(|ajj−λ|−rSj(A)+|aji|)−rSi(A)(r¯Sj(A)+|aji|)|ajj−λ|−rSj(A)+|aji|+rSi(A)≤ε. | (3.14) |
If |ajj−λ|−rSj(A)>0, then it follows from (3.14) that
(|aii−λ|−r¯Si(A)−ε)(|ajj−λ|−rSj(A)+|aji|)≤rSi(A)(r¯Sj(A)+|aji|+ε). |
If |ajj−λ|−rSj(A)≤0, then |ajj−λ|≤rSj(A)+ε≤rj(A)+ε. These imply that λ∈θS(A,ε).
Case Ⅱ. If g(A−λI)≤ε, then for all i∈¯S, |aii−λ|≤rSi(A)+ε or |aii−λ|>rSi(A)+ε for some i∈¯S; but for some j∈¯S∖{i}, |ajj−λ|≤rj(A)+ε or μ¯Sij(A−λI)≤ε, i.e.,
(|aii−λ|−rSi(A))(|ajj−λ|−r¯Sj(A)+|aji|)−r¯Si(A)(rSj(A)+|aji|)|ajj−λ|−r¯Sj(A)+|aji|+r¯Si(A)≤ε. | (3.15) |
If |ajj−λ|−r¯Sj(A)>0, then it follows from (3.15) that
(|aii−λ|−rSi(A)−ε)(|ajj−λ|−r¯Sj(A)+|aji|)≤r¯Si(A)(rSj(A)+|aji|+ε). |
If |ajj−λ|−r¯Sj(A)≤0, then |ajj−λ|≤r¯Sj(A)+ε≤rj(A)+ε. These imply that λ∈θ¯S(A,ε).
From Case Ⅰ and Case Ⅱ, the conclusion follows.
As an application, using Theorem 3.8, we next give a lower bound for distance to instability. Denote by Red(A)∈Rn×n the real matrix associated with a given matrix A=[aij]∈Cn×n in the following way:
(Red(A))ij={Re(aii),j=i,|aij|,j≠i. |
Theorem 3.9. Consider A=[aij]∈Cn×n, such that Red(A) is a PDSDD matrix with all diagonal elements negative. Then, μ(Red(A))>0 and
Λε(A)⊆ΘS(A,ε)⊂C−forall0<ε<μ(Red(A)), | (3.16) |
where C− is the open left half plane of C, μ(Red(A)) is defined as in Lemma 3.2, and Λε(A) denotes the infinity norm ε-pseudospectrum of A.
Proof. Since Red(A) is a PDSDD matrix, it follows from Lemma 3.2 that μ(Red(A))>0. To prove (3.16), for 0<ε<μ(Red(A)), it suffices to show that Re(z)<0 for each z∈ΘS(A,ε). It follows from Theorem 3.8 that z∈θS(A,ε) or z∈θ¯S(A,ε). We only consider the case of z∈θS(A,ε), and the case of z∈θ¯S(A,ε) can be proved similarly. Since z∈θS(A,ε), it follows that for all i∈S, either (i) |aii−z|≤r¯Si(A)+ε or (ii) |aii−z|>r¯Si(A)+ε for some i∈S; but for some j∈S∖{i}, |ajj−z|≤rj(A)+ε or
(|aii−z|−r¯Si(A)−ε)(|ajj−z|−rSj(A)+|aji|)≤rSi(A)(r¯Sj(A)+|aji|+ε). | (3.17) |
Note that
μ(Red(A))=min{f(Red(A)),g(Red(A))}, |
where
f(Red(A)):=maxi∈Smin{|Re(aii)|−r¯Si(Red(A)),minj∈S∖{i}{μSij(Red(A)),|Re(ajj)|−rj(Red(A))}} |
and
g(Red(A)):=maxi∈¯Smin{|Re(aii)|−rSi(Red(A)),minj∈¯S∖{i}{μ¯Sij(Red(A)),|Re(ajj)|−rj(Red(A))}} |
with μSij(Red(A)) defined as in Lemma 3.2.
For case (i), i.e., |aii−z|≤r¯Si(A)+ε for all i∈S, we have
Re(z)−Re(aii)≤|Re(z)−Re(aii)|=|Re(z−aii)|≤|z−aii|≤r¯Si(A)+ε<r¯Si(A)+|Re(aii)|−r¯Si(A)=−Re(aii), |
which implies that Re(z)<0.
For case (ii), i.e., |z−aii|>r¯Si(A)+ε for some i∈S, if |ajj−z|≤rj(A)+ε, then
Re(z)−Re(ajj)≤|Re(z)−Re(ajj)|=|Re(z−ajj)|≤|z−ajj|≤rj(A)+ε<rj(A)+|Re(ajj)|−rj(A)=−Re(ajj), |
which implies that Re(z)<0. If (3.17) holds, then
Re(z)−Re(ajj)≤|Re(z)−Re(ajj)|=|Re(z−ajj)|≤|z−ajj|≤r¯Si(A)(rSj(A)+|aji|+ε)|z−aii|−rSi(A)−ε+rSj(A)−|aji|. | (3.18) |
{If |z−aii|<|Re(aii)|, then Re(z)−Re(aii)≤|z−aii|<|Re(aii)|, which leads to Re(z)<0. Otherwise, since}
0<ε<(|Re(aii)|−r¯Si(A))(|Re(ajj)|−rSj(A)+|aji|)−rSi(A)(r¯Sj(A)+|aji|)|Re(ajj)|−rSj(A)+|aji|+rSi(A)≤(|z−aii|−r¯Si(A))(|Re(ajj)|−rSj(A)+|aji|)−rSi(A)(r¯Sj(A)+|aji|)|Re(ajj)|−rSj(A)+|aji|+rSi(A), |
it follows that
r¯Si(A)(rSj(A)+|aji|+ε)|z−aii|−rSi(A)−ε+rSj(A)−|aji|<|Re(ajj)|, |
which together with (3.18) yields that
Re(z)−Re(ajj)<|Re(ajj)|=−Re(ajj), |
and thus Re(z)<0. This completes the proof.
The following example shows that the bound μ(Red(A)) in Theorem 3.9 is better than those of [13] and [30] in some cases.
Example 3.2. Consider the matrix A∈R10×10 in [13], where
A=[−78−710510835174−959−335355244−58681−3446978−87256−88302−42−901726710936−3−808269−965487−86711079177−34−93113108443564−45−54221099210−3−47]. |
It follows from [13] that A is a DZ-type matrix, and ε=μ(Red(A))=0.66. On the other hand, it is easy to verify that A is also a PDSDD matrix for S={1,2,3,4,9}. Hence, from Theorem 3.9, we can get a new lower bound for distance to instability ε=μ(Red(A))=4.17 and plot the corresponding pseudospectrum as shown in Figure 1, where Γε(A), D(A,ε), ΘS(A,ε), the pseudospectrum Λε(A) and the eigenvalues of A are represented by a blue solid boundary, a green dotted boundary, a red solid boundary, a gray area, and a black "×, " respectively.
As can be seen from Figure 1, the sets Γε(A) of [30] and D(A,ε) of [13] propagate far into the right half-plane of C, but the localization set ΘS(A,ε) touches the y-axis. This implies that we cannot use Γε(A) and D(A,ε) to determine the stability of A. However, using the localization set ΘS(A,ε), we can determine that A is a stable matrix.
This paper proposes a new class of nonsingular H-matrices called PDSDD matrices, which is similar to but different from S-SDD matrices. By its non-singularity, a new eigenvalue localization set for matrices is presented, which improves some existing results in [8] and [27]. Furthermore, an infinity norm bound for the inverse of PDSDD matrices is obtained, which improves the well-known Varah's bound for strictly diagonally dominant matrices. Meanwhile, utilizing the proposed infinity norm bound, a new pseudospectra localization for matrices is given, and a lower bound for distance to instability is provided as well. In addition, applying the proposed infinity norm bound to explore the error bounds for linear complementarity problems of PDSDD matrices is also an interesting problem. It is worth studying in the future.
The authors would like to thank the editor and the anonymous referees for their valuable suggestions and comments. This work was partly supported by the National Natural Science Foundations of China (61962059 and 31600299), the Young Science and Technology Nova Program of Shaanxi Province (2022KJXX-01), the Science and Technology Project of Yan'an (2022SLGYGG-007), the Scientific Research Program Funded by Yunnan Provincial Education Department (2022J0949).
The authors declare there are no conflicts of interest.
[1] | L. Lévy, Sur le possibilitédu l'equibre électrique, C. R. Acad. Sci. Paris, 93 (1881), 706–708. |
[2] |
B. Li, M. Tsatsomeros, Doubly diagonally dominant matrices, Linear Algebra Appl., 261 (1997), 221–235. https://doi.org/10.1016/S0024-3795(96)00406-5 doi: 10.1016/S0024-3795(96)00406-5
![]() |
[3] |
J. Z. Liu, F.Z. Zhang, Disc separation of the Schur complements of diagonally dominant matrices and determinantal bounds, SIAM J. Matrix Anal. Appl., 27 (2005), 665–674. https://doi.org/10.1137/040620369 doi: 10.1137/040620369
![]() |
[4] |
L. Cvetković, H-matrix theory vs. eigenvalue localization, Numer. Algorithms, 42 (2006), 229–245. https://doi.org/10.1007/s11075-006-9029-3 doi: 10.1007/s11075-006-9029-3
![]() |
[5] |
L. Cvetković, M. Erić, J. M. Peña, Eventually SDD matrices and eigenvalue localization, Appl. Math. Comput., 252 (2015), 535–540. https://doi.org/10.1016/j.amc.2014.12.012 doi: 10.1016/j.amc.2014.12.012
![]() |
[6] |
L. Cvetković, V. Kostić, R. Bru, F. Pedroche, A simple generalization of Geršgorin's theorem, Adv. Comput. Math., 35 (2011), 271–280. https://doi.org/10.1007/s10444-009-9143-6 doi: 10.1007/s10444-009-9143-6
![]() |
[7] | L. Cvetković, V. Kostić, R. Varga, A new Geršgorin-type eigenvalue inclusion area, Electron. Trans. Numer. Anal., 18 (2004), 73–80. |
[8] | S. Geršgorin, Über die Abgrenzung der Eigenwerte einer Matrix, Izv. Akad. Nauk SSSR Ser. Mat., 1 (1931), 749–754. |
[9] |
Q. Liu, Z.B. Li, C.Q. Li, A note on eventually SDD matrices and eigenvalue localization, Appl. Math. Comput., 311 (2017), 19–21. https://doi.org/10.1016/j.amc.2017.05.011 doi: 10.1016/j.amc.2017.05.011
![]() |
[10] | R.S. Varga, Geršgorin and His Circles, Springer-Verlag, Berlin, 2004. |
[11] |
X. M. Gu, S. L. Wu, A parallel-in-time iterative algorithm for Volterra partial integro-differential problems with weakly singular kernel, J. Comput. Phys., 417 (2020), 109576. https://doi.org/10.1016/j.jcp.2020.109576 doi: 10.1016/j.jcp.2020.109576
![]() |
[12] |
W. Li, The infinity norm bound for the inverse of nonsingular diagonal dominant matrices, Appl. Math. Lett., 21 (2008), 258–263. https://doi.org/10.1016/j.aml.2007.03.018 doi: 10.1016/j.aml.2007.03.018
![]() |
[13] |
C. Q. Li, L. Cvetković, Y. M. Wei, J. X. Zhao, An infinity norm bound for the inverse of Dashnic-Zusmanovich type matrices with applications, Linear Algebra Appl., 565 (2019), 99–122. https://doi.org/10.1016/j.laa.2018.12.013 doi: 10.1016/j.laa.2018.12.013
![]() |
[14] |
C. Q. Li, Schur complement-based infinity norm bounds for the inverse of SDD matrices, Bull. Malays. Math. Sci. Soc., 43 (2020), 3829–3845. https://doi.org/10.1007/s40840-020-00895-x doi: 10.1007/s40840-020-00895-x
![]() |
[15] |
J. M. Varah, A lower bound for the smallest singular value of a matrix, Linear Algebra Appl., 11 (1975), 3–5. https://doi.org/10.1016/0024-3795(75)90112-3 doi: 10.1016/0024-3795(75)90112-3
![]() |
[16] |
C. Q. Li, Y. T. Li, Note on error bounds for linear complementarity problems for B-matrices, Appl. Math. Lett., 57 (2016), 108–113. https://doi.org/10.1016/j.aml.2016.01.013 doi: 10.1016/j.aml.2016.01.013
![]() |
[17] |
C. Q. Li, Y. T. Li, Weakly chained diagonally dominant B-matrices and error bounds for linear complementarity problems, Numer. Algorithms, 73 (2016), 985–998. https://doi.org/10.1007/s11075-016-0125-8 doi: 10.1007/s11075-016-0125-8
![]() |
[18] |
C. Q. Li, Y. T. Li, Double B-tensors and quasi-double B-tensors, Linear Algebra Appl., 466 (2015), 343–356. https://doi.org/10.1016/j.laa.2014.10.027 doi: 10.1016/j.laa.2014.10.027
![]() |
[19] |
Q. L. Liu, Y. T. Li, p-Norm SDD tensors and eigenvalue localization, J. Inequal. Appl., 2016 (2016), 178. https://doi.org/10.1186/s13660-016-1119-8 doi: 10.1186/s13660-016-1119-8
![]() |
[20] | A. M. Ostrowski, Über die Determinanten mit iiberwiegender Hauptdiagonale, Comment. Math. Helvetici., 10 (1937), 69–96. |
[21] |
Y. M. Gao, H. W. Xiao, Criteria for generalized diagonally dominant matrices and M-matrices, Linear Algebra Appl., 169 (1992), 257–268. https://doi.org/10.1016/0024-3795(92)90182-A doi: 10.1016/0024-3795(92)90182-A
![]() |
[22] |
T. Szulc, Some remarks on a theorem of Gudkov, Linear Algebra Appl., 225 (1995), 221–235. https://doi.org/10.1016/0024-3795(95)00343-P doi: 10.1016/0024-3795(95)00343-P
![]() |
[23] |
W. Li, On Nekrasov matrices, Linear Algebra Appl., 281 (1998), 87–96. https://doi.org/10.1016/S0024-3795(98)10031-9 doi: 10.1016/S0024-3795(98)10031-9
![]() |
[24] |
J. X. Zhao, Q. L. Liu, C. Q. Li, Y. T. Li, Dashnic-Zusmanovich type matrices: A new subclass of nonsingular H-matrices, Linear Algebra Appl., 552 (2018), 277–287. https://doi.org/10.1016/j.laa.2018.04.028 doi: 10.1016/j.laa.2018.04.028
![]() |
[25] |
D. L. Cvetković, L. Cvetković, C. Q. Li, CKV-type matrices with applications, Linear Algebra Appl., 608 (2021), 158–184. https://doi.org/10.1016/j.laa.2020.08.028 doi: 10.1016/j.laa.2020.08.028
![]() |
[26] | A. Berman, R. J. Plemmons, Nonnegative Matrices in the Mathematical Sciences, SIAM, Philadelphia, PA, 1994. |
[27] |
A. Brauer, Limits for the characteristic roots of a matrix Ⅱ, Duke Math. J., 14 (1947), 21–26. https://doi.org/10.1215/S0012-7094-47-01403-8 doi: 10.1215/S0012-7094-47-01403-8
![]() |
[28] |
L. Cvetković, P. F. Dai, K. Doroslovački, Y. T. Li, Infinity norm bounds for the inverse of Nekrasov matrices, Appl. Math. Comput., 219 (2013), 5020–5024. https://doi.org/10.1016/j.amc.2012.11.056 doi: 10.1016/j.amc.2012.11.056
![]() |
[29] |
C. Q. Li, H. Pei, A. N. Gao, Y. T. Li, Improvements on the infinity norm bound for the inverse of Nekrasov matrices, Numer. Algorithms, 71 (2016), 613–630. https://doi.org/10.1007/s11075-015-0012-8 doi: 10.1007/s11075-015-0012-8
![]() |
[30] |
V. R. Kostić, L. Cvetković, D. L. Cvetković, Pseudospectra localizations and their applications, Numer. Linear Algebra Appl., 23 (2016), 356–372. https://doi.org/10.1002/nla.2028 doi: 10.1002/nla.2028
![]() |
[31] |
L. Y. Kolotilina, On bounding inverse to Nekrasov matrices in the infinity norm, J. Math. Sci., 199 (2014), 432–437. https://doi.org/10.1007/s10958-014-1870-7 doi: 10.1007/s10958-014-1870-7
![]() |
[32] |
N. Morača, Upper bounds for the infinity norm of the inverse of SDD and S-SDD matrices, J. Comput. Appl. Math., 206 (2007), 666–678. https://doi.org/10.1016/j.cam.2006.08.013 doi: 10.1016/j.cam.2006.08.013
![]() |
[33] |
S. Z. Pan, S. C. Chen, An upper bound for ||A−1||∞ of strictly doubly diagonally dominant matrices, J. Fuzhou Univ. Nat. Sci. Ed., 36 (2008), 639–642. https://doi.org/10.3724/SP.J.1047.2008.00026 doi: 10.3724/SP.J.1047.2008.00026
![]() |
1. | Fude Zhang, Lanlan Liu, Deshu Sun, Subdirect sums of partially doubly strictly diagonally matrices, 2025, 199, 00074497, 103571, 10.1016/j.bulsci.2024.103571 |