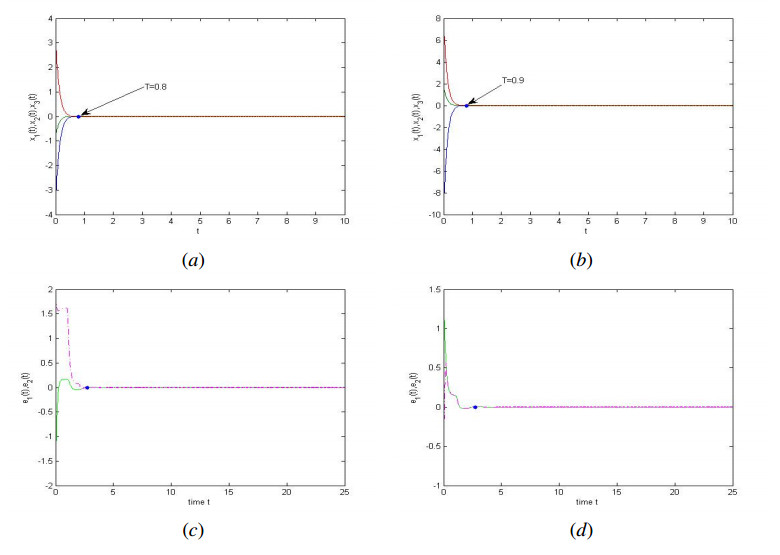
Proper water resource management is essential for maintaining a sustainable supply chain and meeting water demand. The urgent need to preserve river ecosystems by sustaining environmental flow (EF) in the realm of environmental management has been highlighted by the drastic changes to river ecosystems and upstream flow dynamics brought about by careless river exploitation in the last few decades. To optimize EF in river basin management, we present an integrated modeling approach. We focused on the Pir Khezran River basin. Our objective was to estimate EF and generalize the findings to adjacent rivers using modeling techniques, thus providing valuable insights for environmental management applications. The assessment and optimization of EF under uncertain conditions was achieved by combining physical habitat simulation (PHABSIM) modeling with advanced techniques like Adaptive Neuro-Fuzzy Inference Systems (ANFIS) and Multilayer Perceptron (MLP) neural networks. This integrated modeling approach contributes to sustainable solutions for river basin management and environmental conservation by effectively optimizing EF, as demonstrated by the results. This research, therefore, makes valuable contributions to environmental management in various areas such as ecological preservation, modeling and optimizing environmental systems, and policy considerations.
Citation: Seiran Haghgoo, Jamil Amanollahi, Barzan Bahrami Kamangar, Shahryar Sorooshian. Decision models enhancing environmental flow sustainability: A strategic approach to water resource management[J]. AIMS Environmental Science, 2024, 11(6): 900-917. doi: 10.3934/environsci.2024045
[1] | Huijun Xiong, Chao Yang, Wenhao Li . Fixed-time synchronization problem of coupled delayed discontinuous neural networks via indefinite derivative method. Electronic Research Archive, 2023, 31(3): 1625-1640. doi: 10.3934/era.2023084 |
[2] | Jun Guo, Yanchao Shi, Shengye Wang . Synchronization analysis of delayed quaternion-valued memristor-based neural networks by a direct analytical approach. Electronic Research Archive, 2024, 32(5): 3377-3395. doi: 10.3934/era.2024156 |
[3] | Shuang Liu, Tianwei Xu, Qingyun Wang . Effect analysis of pinning and impulsive selection for finite-time synchronization of delayed complex-valued neural networks. Electronic Research Archive, 2025, 33(3): 1792-1811. doi: 10.3934/era.2025081 |
[4] | Jun Guo, Yanchao Shi, Weihua Luo, Yanzhao Cheng, Shengye Wang . Exponential projective synchronization analysis for quaternion-valued memristor-based neural networks with time delays. Electronic Research Archive, 2023, 31(9): 5609-5631. doi: 10.3934/era.2023285 |
[5] | Xiong Jian, Zengyun Wang, Aitong Xin, Yujing Chen, Shujuan Xie . An improved finite-time stabilization of discontinuous non-autonomous IT2 T-S fuzzy interconnected complex-valued systems: A fuzzy switching state-feedback control method. Electronic Research Archive, 2023, 31(1): 273-298. doi: 10.3934/era.2023014 |
[6] | Janarthanan Ramadoss, Asma Alharbi, Karthikeyan Rajagopal, Salah Boulaaras . A fractional-order discrete memristor neuron model: Nodal and network dynamics. Electronic Research Archive, 2022, 30(11): 3977-3992. doi: 10.3934/era.2022202 |
[7] | Xingting Geng, Jianwen Feng, Yi Zhao, Na Li, Jingyi Wang . Fixed-time synchronization of nonlinear coupled memristive neural networks with time delays via sliding-mode control. Electronic Research Archive, 2023, 31(6): 3291-3308. doi: 10.3934/era.2023166 |
[8] | Yilin Li, Jianwen Feng, Jingyi Wang . Mean square synchronization for stochastic delayed neural networks via pinning impulsive control. Electronic Research Archive, 2022, 30(9): 3172-3192. doi: 10.3934/era.2022161 |
[9] | Jiaqi Chang, Xiangxin Yin, Caoyuan Ma, Donghua Zhao, Yongzheng Sun . Estimation of the time cost with pinning control for stochastic complex networks. Electronic Research Archive, 2022, 30(9): 3509-3526. doi: 10.3934/era.2022179 |
[10] | Yu-Jing Shi, Yan Ma . Finite/fixed-time synchronization for complex networks via quantized adaptive control. Electronic Research Archive, 2021, 29(2): 2047-2061. doi: 10.3934/era.2020104 |
Proper water resource management is essential for maintaining a sustainable supply chain and meeting water demand. The urgent need to preserve river ecosystems by sustaining environmental flow (EF) in the realm of environmental management has been highlighted by the drastic changes to river ecosystems and upstream flow dynamics brought about by careless river exploitation in the last few decades. To optimize EF in river basin management, we present an integrated modeling approach. We focused on the Pir Khezran River basin. Our objective was to estimate EF and generalize the findings to adjacent rivers using modeling techniques, thus providing valuable insights for environmental management applications. The assessment and optimization of EF under uncertain conditions was achieved by combining physical habitat simulation (PHABSIM) modeling with advanced techniques like Adaptive Neuro-Fuzzy Inference Systems (ANFIS) and Multilayer Perceptron (MLP) neural networks. This integrated modeling approach contributes to sustainable solutions for river basin management and environmental conservation by effectively optimizing EF, as demonstrated by the results. This research, therefore, makes valuable contributions to environmental management in various areas such as ecological preservation, modeling and optimizing environmental systems, and policy considerations.
With the emergence of large scientific devices in various scientific fields around the world, scientific discovery has entered the era of big data. Scientific discovery cannot completely rely on expert experience to find rare scientific events from massive data, and a large amount of historical data cannot be used effectively. At the same time, it is becoming more and more prominent in real-time and high precision. The mode of scientific events is rare, and the general algorithm is not suitable for the field of science. Therefore, the problem of intelligent discovery of scientific data came into being. Scientific data intelligent discovery aims to accelerate the discovery of scientific events by using data intelligent method. However, intelligent discovery of scientific data lacks the overall framework design, which is embodied in the lack of comprehensive analysis system of scientific data and efficient knowledge fusion mechanism of heterogeneous scientific data. From the perspective of data management, the long-term storage and mining of massive historical data is inefficient. This paper puts forward the framework and related challenges of scientific data intelligent discovery and management, in order to promote the progress of scientific discovery.
Another description form of neural network model is based on memristor. In 2008, the Hewlett-Packard(HP) laboratory research group in the United States first confirmed the existence of memristor in nanometre electronic components, and also described it in detail with mathematical and physical models [1]. As a new type of nanometre circuit element, the memristor has many good characteristics, such as low energy dissipation and powerful memory function. Memristor has attracted the attention of scientific research institutions in different fields all over the world. Its strong learning ability can consent memristor to replace the existing transistors in the future. If the resistance is used to replace the self-feedback connection weight, the Hopfield neural network system will have a simple memory storage function [2,3]. Similarly, further, if the memristor is used to replace the traditional resistor, a simple memristor model can be established. It also proves that memristor plays an important role in circuit theory, especially in the field of modeling and non-traditional signal processing. The connection weight is completely determined by the memristor in the artificial neural network. The memristor model based on neural networks can be established by simulating the structure of human brain which is very similar to the structure of neural networks (the structure diagram of four circuit elements of memristor). At the same time, due to the extremely rich dynamic behavior in the neural network system (especially the memristor system), the storage, reading and writing abilities of n-node neural network system with memristor are dozens of times that of the neural network system without memristor [4]. Compared with the traditional model, this kind of model has stronger computing power and information storage capacity. Another neural network model, recurrent neural network, has also received a lot of attention and has a wide range of applications, such as the four-tank benchmark problem, the nonlinear constrained optimization problem, the statistical model, the phase structure and the pattern recognition [5,6,7]. Therefore, combined with memristor and recurrent neural network model, considering its rich theoretical significance and practical achievements, many scholars are committed to the synchronization of recurrent neural network model with memristor. From the perspective of mathematical theory, this kind of new neural network model is a switched dynamic system model that depends on the initial state. In essence, it can be described by the discontinuous functional differential equation. Through the methods of set-valued analysis theory and functional differential equation, the mathematical theory and neural network engineering model are effectively combined. As a powerful analysis tool and an effective technical means, this paper makes a comprehensive discussion and dynamic analysis on the time-delay recurrent neural network model based on memristor, which not only improves the system of discontinuous functional differential equations at the right end of mathematics at the theoretical level, but also provides a more practical idea for the neural network model in engineering at the application level.
In order to better obtain the stability results on the neural network model, the way to realize stabilization is also divided into different categories. Among them, the popular stabilization methods include exponential stabilization [8], asymptotic stabilization [9], exponential adaptive stabilization [10], anti-stabilization [11], robust stabilization [12], finite-time stabilization and fixed-time synchronization. However, the previous results about asymptotic stabilization and exponential stabilization can be guaranteed only when the time is close to infinity. Since the service life of organisms and equipment is limited, the criteria of asymptotic stability and exponential stability are not applicable in practice. In order to improve convergence and efficiency, another finite-time stabilization has been widely studied in recent years [13,14,15,16,17,18,19,20]. Finite-time stabilization means that stabilization can reach the so-called stable time within the specified time. When the initial values are known, some effective controllers with adjustable parameters can be designed, in which the parameters considered can be stabilized before a given fixed time. Obviously, the efficiency of engineering such as image processing and secure communication will be greatly improved based on finite-time technology. Finite-time stabilization has better robustness and anti-interference ability than asymptotic stabilization. On the other hand, the stable time of finite-time stabilization depends on the selection of initial values. The stabilization stable settling time is not fixed for different initial values. In addition, considering the environmental constraints in practice and other factors, not all initial values are applicable in the system, so finite-time stabilization also has some limitations. Therefore, in recent years, a more suitable stabilization method for realizing finite-time stability, called fixed-time stabilization(FTS), was proposed by A. Polyakov et al. in [21,22]. Its advantage over finite-time stabilization method is that its stability time does not depend on the selection of the initial value. Under the framework of fixed-time technology, many scholars also made outstanding contributions to the finite-time stabilization problem. A unified control strategy is designed and it is revealed that a parameter value in the controller completely decides the memristors-based neural networks are stabilized whether in finite time or in fixed time, which is described in [23,24,25,26,27,28]. Meanwhile, the author designed a class of memristive neural networks with time-varying delays and general activation functions. They investigate the exponential stabilization problem of such systems in [29]. And Wen studied a new convolution algorithm: convolution kernel first operated (CKFO), which can solve the problem that the actual calculation is not reduced after pruning the weight of the convolution neural network [30].
However, in the intricate relationship within neurons, we must first consider the coupling network and understand the interaction between different neurons. In [31,32], the authors introduced an ordinary differential equation model with neuron nodes with mutual coupling relationship, and the following interesting dynamic behaviors will occur in the model: self-organization phenomenon, synchronization behavior, traveling wave solution, spatiotemporal chaos and defect propagation. In [33], a dynamic element was introduced as a neuron node. Considering the interaction and influence between neurons, the existing neural network models are considered from the static and dynamic levels respectively. At the same time, in practice, one can not control every neuron, so the introduction of a new containment control theory is very necessary. For the problem of non-strongly connected networks or undirected spanning trees, a lot of references have studied the stable traction control theory of complex networks [34,35,36,37,38,39]. The theory can be used for network topological features, such as strongly connected networks, undirected networks, scale-free networks and random networks, to realize global traction stable control under the stability framework. In addition, this brief considers a single system. If two drive response systems are considered, the finite-time stabilization problem can also be transformed into a variety of synchronization problems. Synchronization means that the state variables of these two systems are often consistent under control input. There have been many excellent works before, such as quasi-uniform synchronization [40,41], global synchronization for BAM delayed reaction-diffusion neural networks[42].
Based on the above statements, this brief presents a pinning control scheme for FTS of delayed CMNNs with nonlinear coupling. The main contributions of this paper are summarized as follows:
1) This paper comprehensively considers a class of complex systems with time-delayed and nonlinear coupling. Considering the interaction of internal neurons, the coupling function of neuron nodes is found through topological graph theory.
2) The Lyapunov method of the indefinite derivative is used in this paper. We consider the non-autonomous system with variable coefficients which is state-dependent, the stability of the system is better discussed according to the real-time state of neurons.
3) By designing a suitable pinning controller and considering the FTS problem of CMNNs. Compared with the previous research results, this paper can ensure the stability faster and the settling time shorter by controlling only a small number of neurons.
This brief mainly studies the fixed-time stabilization problem of CMNNs system based on the idea of the indefinite derivative, through the design of an appropriate pinning controller to make the system achieve finite-time stabilization as soon as possible. In the next section, we give the model description and some preliminaries. In Section 3, it is our main part. The main challenge of this section is to use the finite-time stability theory different from the traditional theory to stabilize the system as soon as possible. In the last section, two numerical simulation examples are given.
This part gives some basic definitions, lemmas and assumptions, which can be obtained from some basic references [43,44,45,46,47,48,49].
This section we introduce a mathematical model of MNNs as follows:
˙z(t)=−Dz(t)+A(z(t))f(z(t))+B(z(t))f(z(t−τ)), | (2.1) |
another expression of the above system (2.1) is:
dzi(t)dt=−dizi(t)+n∑j=1aij(zj(t))fj(zj(t))+n∑j=1bij(zj(t))fj(zj(t−τ)), | (2.2) |
where z(t)=(z1(t),z2(t),…,zn(t))T denotes the potential of capacitor ci, di>0 expresses the self-inhibition of the ith neuron; f(z(t)) expresses the activation function with fi(0)=0 for every i∈N; aij(⋅) and bij(⋅) are two measurable functions representing the weight of memristor, which can be shown as:
aij=WfijCi×SGNij, bij=WfijCi×SGNij, |
where Wfij indicate the memductances of memristors. Mfij stands for the memristors between the activation function fi(zi(t)) and zi(t), and Ci is the invariant capacitor when the memductances of memristors Wfij and Mfij respond to change in pinched hysteresis loops. Moreover,
SGNij={ 1, if i≠j, −1, if i=. |
According to the characteristics of memristor and the discussion in previous literature, we give the mathematical expression of the connection functions of memristor as follows:
¯co[aij(zj(t))]={ ˇaij, hi(z(t))≤ri, ˆaij, hi(z(t))>ri, ¯co[bij(zj(t))]={ ˇbij, hi(z(t))≤ri, ˆbij, hi(z(t))>ri, |
where hi:R→R are threshold level functions, ri∈R are threshold level, and ˇaij, ˇbij, ˆaij, ˆbij are constant numbers.
Through the idea of differential inclusion and Filippove theory, because aij and bij have discontinuous characteristics, we can get that dzi(t)dt∈−dizi(t)+n∑j=1¯co[aij(zj(t))]fj(zj(t))+n∑j=1¯co[bij(zj(t))]fj(zj(t−τ)), where
aij(zi(t))={ ˇaij, hi(z(t))<ri, [a_ij,¯aij], |hi(z(t))|=ri, ˆaij, hi(z(t))>ri, bij(zi(t))={ ˇbij, hi(z(t))>ri, [b_ij,¯bij], |hi(z(t))|=ri, ˆbij, hi(z(t))>ri, |
with a_ij=min{ˇaij,ˆaij}, ¯aij=max{ˇaij,ˆaij} and b_ij=min{ˇbij,ˆbij}, ¯bij=max{ˇbij,ˆbij}.
Then for a.e. t≥0 and i=1,2,…,n, there exist ˜aij(t)∈¯co[aij(zi)] and ˜bij(t)∈¯co[bij(zi)] such that
dzi(t)dt=−dizi(t)+n∑j=1˜aij(t)fj(zj(t))+n∑j=1˜bij(t)fj(zj(t−τ)), | (2.3) |
and the initial value of the system is definition as ψ(s)=(ψ1(s),ψ2(s),…,ψn(s))T∈C[(−τ,0),Rn].
Our goal is to gain the FTS issue of the above system. Before that, we need to make the basic assumption of the activation function as follow:
(Hypothesis 1). For every j∈N, the neuron activation fj are bounded and satisfy that
h−j≤fj(x)−fj(y)x−y≤h+j, |
where x≠y and h−j,h+j are constants.
Definition 2.1 (see [8]). The solution of system (2.1) is said to gain stabilized in a finite time, if there exists a settling time T∗(z0,t0) such that
limt→T∗‖ and \|z_{i}(t)-z_{j}(t)\|\equiv0 for all t > T^{*} and i, j = 1, 2, \ldots, n. |
Moreover, if the settling time T^{*}(z_{0}, t_{0}) is not depend on the initial values, the solution of system (2.1) is said to be fixed-time stabilized.
Definition 2.2 (see [8]). For the function \psi: {\mathbb R}_{+}\rightarrow {\mathbb R}_{+} , if it is continuous and strictly increasing with \psi(0) = 0 , \psi is called a \mathcal{K} -function and it can be expressed as \psi\in\mathcal{K} . Moreover, if \lim_{s\rightarrow +\infty}\psi(s) = +\infty holds, the function \psi is called a \mathcal{K}_{\infty} -function with \psi\in\mathcal{K}_{\infty} .
Lemma 2.3 (C-Regular see [48]). The function V: {\mathbb R}^{n}\rightarrow {\mathbb R}^{n} is C-Regular if and only if V(z) satisfies:
1) regularity in {\mathbb R}^{n} ;
2) V(z) > 0 for z\neq0 and V(0) = 0 ;
3) V(z)\rightarrow +\infty as \|z\|\rightarrow +\infty .
Lemma 2.4 (Chain Rule see [48]). V(z) is C-regular and for z(t) denotes absolute continuity function in [t_{0}, +\infty)\rightarrow {\mathbb R}^{n} , then V(z(t)) is differentiable for a.e. t\geq t_{0} , and \frac{\mathrm{d}V(z(t))}{\mathrm{d}t} = \langle\mathcal{L}(t), \frac{\mathrm{d}z(t)}{\mathrm{d}t}\rangle , where \mathcal{L}(t)\in\partial V(z(t)) .
Lemma 2.5 (See [24]). For an undirected graph \mathcal{G}(\mathcal{A}) , and the corresponding adjacency matrix and Laplace matrix are C = [c_{ij}] and L respectively, the following formula holds for arbitrary z = (z_{1}, z_{2}, \ldots, z_{n})\in {\mathbb R}^{n} :
\begin{equation*} z^{T}Lz = \frac{1}{2}\sum\limits^{n}_{i, j = 1}c_{ij}(z_{i}-z_{j})^{2}. \end{equation*} |
Moreover, if there exists an undirected and connected nonnegative column vector {\bf w} , L(\mathcal{A})+\mathrm{diag}({\bf w}) is positive definite.
Lemma 2.6 (Jesen inequality [49]). If a_{1}, a_{2}, \ldots, a_{n} are positive numbers and 0 < r < p , then
\begin{equation*} \Big(\sum\limits_{i = 1}^n a^{p}_{i}\Big)^{1/p}\leq\Big(\sum\limits_{i = 1}^n a^{r}_{i}\Big)^{1/r}, \end{equation*} |
and
\begin{equation*} \Big(\frac{1}{n}\sum\limits_{i = 1}^n a^{p}_{i}\Big)^{1/p}\geq\Big(\frac{1}{n}\sum\limits_{i = 1}^n a^{r}_{i}\Big)^{1/r}. \end{equation*} |
This section we introduce the FTS for N identical discontinuous MNNs (2.1), when consider the internal relationship of neurons and adding the nonlinear coupling relationship to the system, one can rewrite the system (2.3) as
\begin{align} \frac{\mathrm{d}x_{i}(t)}{\mathrm{d}t}& = -d_{i}x_{i}(t)+\widetilde{A}(x_{i}(t))f(x_{i}(t))+\widetilde{B}(x_{i}(t))f(x_{i}(t-\tau)) \\ &+\sum\limits^{N}_{j = 1}c_{ij}\phi_{\alpha+1}(x_{j}(t)-x_{i}(t))+\sum\limits^{N}_{j = 1}c_{ij}\phi_{\beta+1}(x_{j}(t)-x_{i}(t))+u_{i}(t), \end{align} | (3.1) |
where i = 1, 2, \cdots, N , and x_{i}(t) = (x_{i1}(t), x_{i2}(t), \cdots, x_{in}(t))^{T}\in {\mathbb R}^{n} is the state vector representing the state variables of node i at time t . f = f(x_{i}(t)): {\mathbb R}^{n}\rightarrow {\mathbb R}^{n} is a vector-value function standing for the activity of an individual subsystem. \widetilde{A} and \widetilde{B} express [\widetilde{a}_{ij}] and [\widetilde{b}_{ij}] , respectively. \phi is the nonlinear coupling function and \phi_{s+1}(z) = |z|^{s}\mathrm{sign}(z) . 0 < \alpha < 1 and \beta > 1 . Matrix C = [c_{ij}] denotes the adjacency matrix of subsystems, which corresponding Laplacian matrix is represented as L , and all of them are applicable to undirected weighted networks. u_{i}(t) is the controller.
In order to achieve the FTS and the convenience of engineering practice, it is generally impossible to control every neuron. Then we introduce the method of pinning control. In this paper, a random number \delta(\frac{1}{N} < \delta < 1) is selected, and let l = [\delta N] , that is, the control is applied to these l neurons. Without losing generality, we can select the first l neurons (which are also regarded as a random choice) as the control objects. Then the controller u_{i}(t) can be given as
\begin{equation} u_{i}(t) = \left\{\begin{split} \ &\frac{1}{2}Q(t)\mathrm{SIGN}(x_{i}(t))|x_{i}(t)|-p\mathrm{SIGN}(x_{i}(t))|x_{i}(t)|^{\alpha}-q\mathrm{SIGN}(x_{i}(t))|x_{i}(t)|^{\beta}, \ \ \ \ i = 1, 2, \ldots, l, \\ \ &0, \ \ \ \ i = l+1, \ldots, N. \end{split}\right. \end{equation} | (3.2) |
where Q(t) is a K_{\infty} -function p , q are nonnegative coefficients.
For the sake of convenience, we denote d^{\min} = \min\{d_{i}\} and h^{\max} = \max\{h_{j}^{-}, h_{j}^{+}\} for every i, j\in {\mathbb N} . Then the main results in this paper are given by the following theorems.
Theorem 3.1. If the activation function f of the system is assumed as the above Hypothesis. The coupled system (3.1) with novel controller (3.2) can be fixed-time stabilized if the following equal holds for every i\in {\mathbb N} and k, l = 1, 2, \ldots, n :
(A) d^{\min} > h^{\max}\cdot\max\limits_{k, l\in {\mathbb N}}\{\sup\limits_{t\in[t_{0}, +\infty)}|\widetilde{a}_{kl}(t)|\}+nh^{\max}\cdot\max\limits_{k, l\in {\mathbb N}}\{\sup\limits_{t\in[t_{0}, +\infty)}|\widetilde{b}_{kl}(t)|\} .
Proof. Define the Lyapunov function V(t, x) as follows:
\begin{equation*} V(t, x) = \frac{1}{2}\sum\limits^{N}_{i = 1}x^{T}_{i}(t)x_{i}(t) = \frac{1}{2}x^{T}(t)x(t). \end{equation*} |
Differentiating the derivative of V(t, x) along the trajectories of (3.1)
\begin{align} \dot{V}(t, x)& = \sum\limits^{N}_{i = 1}x^{T}_{i}(t)\dot{x}_{i}(t)\\ & = \sum\limits^{N}_{i = 1}x^{T}_{i}(t)\Big(-d_{i}x_{i}(t)+\sum\limits^{n}_{j = 1}\widetilde{a}_{ij}(t)f(x_{j}(t))+\sum\limits^{n}_{j = 1}\widetilde{b}_{ij}(t)f(x_{j}(t-\tau)) \\ &+\sum\limits^{N}_{j = 1}c_{ij}\phi_{\alpha+1}(x_{j}(t)-x_{i}(t))+\sum\limits^{N}_{j = 1}c_{ij}\phi_{\beta+1}(x_{j}(t)-x_{i}(t))\Big)+\sum\limits^{l}_{i = 1}x^{T}_{i}(t)u_{i}(t)\\ & = -\sum\limits^{N}_{i = 1}x^{T}_{i}(t)d_{i}x_{i}(t)+\sum\limits^{N}_{i = 1}x^{T}_{i}(t)\sum\limits^{n}_{j = 1}\widetilde{a}_{ij}(t)f(x_{j}(t))+\sum\limits^{N}_{i = 1}x^{T}_{i}(t)\sum\limits^{n}_{j = 1}\widetilde{b}_{ij}(t)f(x_{j}(t-\tau))\\ &+\sum\limits^{N}_{i = 1}x^{T}_{i}(t)\sum\limits^{N}_{j = 1}c_{ij}\phi_{\alpha+1}(x_{j}(t)-x_{i}(t))+\sum\limits^{N}_{i = 1}x^{T}_{i}(t)\sum\limits^{N}_{j = 1}c_{ij}\phi_{\beta+1}(x_{j}(t)-x_{i}(t))\\ &+\frac{1}{2}\sum\limits^{l}_{i = 1}x^{T}_{i}(t)Q(t)\mathrm{SIGN}(x_{i}(t))|x_{i}(t)|-\sum\limits^{l}_{i = 1}x^{T}_{i}(t)p\mathrm{SIGN}(x_{i}(t))|x_{i}(t)|^{\alpha}-\sum\limits^{l}_{i = 1}x^{T}_{i}(t)q\mathrm{SIGN}(x_{i}(t))|x_{i}(t)|^{\beta}. \end{align} | (3.3) |
From the above discussion of measurable functions \widetilde{a}_{ij}(t) , \widetilde{b}_{ij}(t) and the Hypothesis 1, one can obtain that
\begin{align} \sum\limits^{N}_{i = 1}x^{T}_{i}(t)\sum\limits^{n}_{j = 1}\widetilde{a}_{ij}(t)f(x_{j}(t))& = \sum\limits^{N}_{i = 1}\sum\limits^{n}_{l = 1}\sum\limits^{n}_{k = 1}x_{ik}(t)\widetilde{a}_{kl}(t)f(x_{il}(t))\leq\sum\limits^{N}_{i = 1}\sum\limits^{n}_{l = 1}\sum\limits^{n}_{k = 1}|x_{ik}(t)||\widetilde{a}_{kl}(t)||f(x_{il}(t))| \\ &\leq\sum\limits^{N}_{i = 1}\sum\limits^{n}_{l = 1}\sum\limits^{n}_{k = 1}|x_{ik}(t)||\widetilde{a}_{kl}(t)|h^{+}_{l}\cdot|x_{il}(t)| \\ &\leq h^{\max}\cdot\max\limits_{k, l\in {\mathbb N}}\{\sup\limits_{t\in {\mathbb R}}|\widetilde{a}_{kl}(t)|\}\sum\limits^{N}_{i = 1}\sum\limits^{n}_{l = 1}\sum\limits^{n}_{k = 1}|x_{ik}(t)||x_{il}(t)| \end{align} | (3.4) |
\begin{align} \sum\limits^{N}_{i = 1}x^{T}_{i}(t)\sum\limits^{n}_{j = 1}\widetilde{b}_{ij}(t)f(x_{j}(t-\tau))& = \sum\limits^{N}_{i = 1}\sum\limits^{n}_{l = 1}\sum\limits^{n}_{k = 1}x_{ik}(t)\widetilde{b}_{kl}(t)f(x_{il}(t-\tau))\leq\sum\limits^{N}_{i = 1}\sum\limits^{n}_{l = 1}\sum\limits^{n}_{k = 1}|x_{ik}(t)||\widetilde{b}_{kl}(t)||f_{l}(x_{il}(t-\tau))| \\ &\leq\sum\limits^{N}_{i = 1}\sum\limits^{n}_{l = 1}\sum\limits^{n}_{k = 1}|x_{ik}(t)||\widetilde{b}_{kl}(t)|h^{+}_{l}\cdot|x_{il}(t-\tau)| \\ &\leq h^{\max}\cdot\max\limits_{k, l\in {\mathbb N}}\{\sup\limits_{t\in {\mathbb R}}|\widetilde{b}_{kl}(t)|\}\sum\limits^{N}_{i = 1}\sum\limits^{n}_{l = 1}\sum\limits^{n}_{k = 1}|x_{ik}(t)||x_{il}(t-\tau)| \\ &\leq h^{\max}\cdot\max\limits_{k, l\in {\mathbb N}}\{\sup\limits_{t\in {\mathbb R}}|\widetilde{b}_{kl}(t)|\}\sum\limits^{N}_{i = 1}\big(\frac{n}{2}\sum\limits^{n}_{k = 1}x^{2}_{ik}(t)+\frac{n}{2}\sum\limits^{n}_{l = 1}x^{2}_{il}(t-\tau)\big). \end{align} | (3.5) |
According to the hypothesis (A) of this theorem and two inequalities (Eqs 3.4 and 3.5), we can reduce the Eq (3.3) to
\begin{align} \dot{V}(t, x)&\leq\sum\limits^{N}_{i = 1}x^{T}_{i}(t)\sum\limits^{N}_{j = 1}c_{ij}\phi_{\alpha+1}(x_{j}(t)-x_{i}(t))+\sum\limits^{N}_{i = 1}x^{T}_{i}(t)\sum\limits^{N}_{j = 1}c_{ij}\phi_{\beta+1}(x_{j}(t)-x_{i}(t))\\ &+\frac{1}{2}Q(t)\sum\limits^{N}_{i = 1}x^{T}_{i}(t)x_{i}(t)-\sum\limits^{l}_{i = 1}p|x_{i}(t)|^{1+\alpha}-\sum\limits^{l}_{i = 1}q|x_{i}(t)|^{1+\beta}. \end{align} | (3.6) |
According the first Jesen inequality of Lemma 2.6, let p_{i} = p when i = 1, \ldots, l and p_{i} = 0 when i = l+1, \ldots, N and q_{i} = q when i = 1, \ldots, l and q_{i} = 0 when i = l+1, \ldots, N , one can reduce
\begin{align} &\sum\limits^{N}_{i = 1}x^{T}_{i}(t)\sum\limits^{N}_{j = 1}c_{ij}\phi_{\alpha+1}(x_{j}(t)-x_{i}(t))-\sum\limits^{l}_{i = 1}p|x_{i}(t)|^{1+\alpha}\\ & = -\frac{1}{2}\sum\limits^{N}_{i, j = 1}c_{ij}(x_{j}(t)-x_{i}(t))\phi_{\alpha+1}(x_{j}(t)-x_{i}(t))-\sum\limits^{N}_{i = 1}p_{i}|x_{i}(t)|^{1+\alpha}\\ & = -\frac{1}{2}\sum\limits^{N}_{i, j = 1}c_{ij}|x_{j}(t)-x_{i}(t)|^{1+\alpha}-\sum\limits^{N}_{i = 1}p_{i}|x_{i}(t)|^{1+\alpha}\\ & = -\frac{1}{2}\sum\limits^{N}_{i = 1}\Big[\sum\limits^{N}_{j = 1}c_{ij}|x_{j}(t)-x_{i}(t)|^{1+\alpha}+2p_{i}|x_{i}(t)|^{1+\alpha}\Big]\\ &\leq-\frac{1}{2}\Big\{\sum\limits^{N}_{i = 1}\Big[\sum\limits^{N}_{j = 1}c^{\frac{2}{\alpha+1}}_{ij}|x_{j}(t)-x_{i}(t)|^{2}+(2p_{i})^{\frac{2}{\alpha+1}}|x_{i}(t)|^{2}\Big\}^{\frac{1+\alpha}{2}}. \end{align} | (3.7) |
and by the second Jesen inequality of Lemma 2.6, similarly one can have
\begin{align} &\sum\limits^{N}_{i = 1}x^{T}_{i}(t)\sum\limits^{N}_{j = 1}c_{ij}\phi_{\beta+1}(x_{j}(t)-x_{i}(t))-\sum\limits^{l}_{i = 1}q|x_{i}(t)|^{1+\beta}\\ & = -\frac{N}{2}\sum\limits^{N}_{i = 1}\Big[\sum\limits^{N}_{j = 1}\frac{1}{N}c_{ij}|x_{j}(t)-x_{i}(t)|^{1+\beta}+\frac{1}{N}2q_{i}|x_{i}(t)|^{1+\beta}\Big]\\ &\leq-\frac{1}{2}N^{\frac{1-\beta}{2}}\Big(\sum\limits^{N}_{i, j = 1}c^{\frac{2}{\beta+1}}_{ij}|x_{j}(t)-x_{i}(t)|^{2}+\sum\limits^{N}_{i = 1}(2q_{i})^{\frac{2}{1+\beta}}|x_{i}(t)|^{2}\Big)^{\frac{1+\beta}{2}}. \end{align} | (3.8) |
Given two new matrices as C_{1} = (c^{\frac{2}{1+\alpha}}_{ij}) and C_{2} = (c^{\frac{2}{1+\beta}}_{ij}) . L_{C_{1}} and L_{C_{2}} denote the Laplacian matrices of the Graph \mathcal{G}(C_{1}) and \mathcal{G}(C_{2}) , respectively. Let D_{p} = \mathrm{diag}\big(\underbrace{(2p)^{\frac{2}{1+\alpha}}, \ldots, (2p)^{\frac{2}{1+\alpha}}}_{l}, \underbrace{0, \ldots, 0}_{N-l}\big) and D_{q} = \mathrm{diag}\big(\underbrace{(2q)^{\frac{2}{1+\alpha}}, \ldots, (2q)^{\frac{2}{1+\beta}}}_{l}, \underbrace{0, \ldots, 0}_{N-l}\big) . Recalling the Lemma 2.5, (3.7) and (3.8), the Eq (3.6) can be rewritten as
\begin{align} \dot{V}(t, x)&\leq \frac{1}{2}Q(t)\sum\limits^{N}_{i = 1}x^{T}_{i}(t)x_{i}(t)-\frac{1}{2}[x^{T}(2L_{C_{1}}+D_{p})x]^{\frac{1+\alpha}{2}}-\frac{1}{2}N^{\frac{1-\beta}{2}}[x^{T}(2L_{C_{2}}+D_{q})x]^{\frac{1+\beta}{2}}\\ &\leq Q(t)V(t, x)-\frac{1}{2}(\lambda_{1}x^{T}x)^{\frac{1+\alpha}{2}}-\frac{1}{2}N^{\frac{1-\beta}{2}}(\lambda_{2}x^{T}x)^{\frac{1+\beta}{2}}\\ &\leq Q(t)V(t, x)-2^{\frac{\alpha-1}{2}}\lambda^{\frac{1+\alpha}{2}}_{1}V^{\frac{1+\alpha}{2}}(t, x)-2^{\frac{\beta-1}{2}}N^{\frac{1-\beta}{2}}\lambda_{2}^{\frac{1+\beta}{2}}V^{\frac{1+\beta}{2}}(t, x), \end{align} | (3.9) |
where \lambda_{1} = \lambda_{\min}(2L_{C_{1}}+D_{p}) and \lambda_{2} = \lambda_{\min}(2L_{C_{2}}+D_{q}) .
Let \breve{p} = 2^{\frac{\alpha-1}{2}}\lambda^{\frac{1+\alpha}{2}}_{1} and \breve{q} = 2^{\frac{\beta-1}{2}}N^{\frac{1-\beta}{2}}\lambda_{2}^{\frac{1+\beta}{2}} , because 0 < \frac{1+\alpha}{2} < 1 and \frac{1+\beta}{2} > 1 , multiplying both sides of (3.9) by V^{-\frac{1+\alpha}{2}}(t, x) , one can have
\begin{align} \frac{2}{1-\alpha}\frac{\mathrm{d}V^{\frac{1-\alpha}{2}}(t, x)}{\mathrm{d}t} = V^{-\frac{1+\alpha}{2}}(t, x)\frac{\mathrm{d}V(t, x)}{\mathrm{d}t}&\leq Q(t)V^{\frac{1-\alpha}{2}}(t, x)-\breve{p}-\breve{q}V^{\frac{\beta-\alpha}{2}}(t, x), \end{align} | (3.10) |
let W(t, x) = V^{\frac{1-\alpha}{2}}(t, x) , then we can expand the inequality (Eq 3.10) as
\begin{align} \frac{\mathrm{d}W(t, x)}{\mathrm{d}t}\leq \frac{1-\alpha}{2}Q(t)W(t, x)-\frac{1-\alpha}{2}\breve{p}\leq Q(t)W(t, x)-\frac{1-\alpha}{2}\breve{p}, \end{align} | (3.11) |
multiplying both sides of (3.11) by e^{-\int^{t}_{t^{*}}Q(\varsigma)\mathrm{d}\varsigma} , for a.e. t\geq t^{*} we get
\begin{align} \frac{\mathrm{d}\big[W(t, x)e^{-\int^{t}_{t^{*}}Q(\varsigma)\mathrm{d}\varsigma}\big]}{\mathrm{d}t}\leq -\frac{1-\alpha}{2}\breve{p}\cdot e^{-\int^{t}_{t^{*}}Q(\varsigma)\mathrm{d}\varsigma}. \end{align} | (3.12) |
Integrate both sides of (3.14) from t^{*} to t and according to the definition of K_{\infty} -function, which implies \int^{t}_{t_{0}}Q(s)\mathrm{d}s\leq -\lambda(t-t_{0})+M , then one can get
\begin{align} W(t, x(t))e^{-\int^{t}_{t^{*}}Q(\varsigma)\mathrm{d}\varsigma}&\leq W(t^{*}, x(t^{*}))-\frac{1-\alpha}{2}\breve{p}\int^{t}_{t^{*}}e^{-\int^{\varsigma}_{t^{*}}Q(s)\mathrm{d}s}\mathrm{d}\varsigma\\ &\leq W(t^{*}, x(t^{*}))-\frac{1-\alpha}{2}\breve{p}\int^{t}_{t^{*}}e^{\lambda(\varsigma-t^{*})-M}\mathrm{d}\varsigma\\ & = W(t^{*}, x(t^{*}))-\frac{(1-\alpha)\breve{p}}{2\lambda e^{M}}e^{\lambda(\varsigma-t^{*})}\Big|^{t}_{t^{*}}\\ & = W(t^{*}, x(t^{*}))-\frac{(1-\alpha)\breve{p}}{2\lambda e^{M}}\Big(e^{\lambda(t-t^{*})}-1\Big)\\ & = W(t^{*}, x(t^{*}))+\frac{(1-\alpha)\breve{p}}{2\lambda e^{M}}-\frac{(1-\alpha)\breve{p}}{2\lambda e^{M}}e^{\lambda(t-t^{*})}, \end{align} | (3.13) |
which implies
\begin{align} V^{\frac{1-\alpha}{2}}(t, x)\leq e^{\int^{t}_{t^{*}}Q(\varsigma)\mathrm{d}\varsigma}\Big[V^{\frac{1-\alpha}{2}}(t^{*}, x(t^{*}))+\frac{(1-\alpha)\breve{p}}{2\lambda e^{M}}-\frac{(1-\alpha)\breve{p}}{2\lambda e^{M}}e^{\lambda(t-t^{*})}\Big]. \end{align} | (3.14) |
Moreover, from (3.9) it's easily to see that \frac{\mathrm{d}V(t, x)}{\mathrm{d}t}\leq Q(t)V(t, x) , which yields for t^{*} > t_{0}
\begin{align} V^{\frac{1-\alpha}{2}}(t^{*}, x(t^{*}))\leq V^{\frac{1-\alpha}{2}}(t_{0}, x_{0})e^{\frac{(1-\alpha)M}{2}}\triangleq \Upsilon. \end{align} | (3.15) |
Combined with (3.14) and (3.15), it is easy to see V(t, x(t))\equiv0 if the following inequality holds:
\begin{align} \Upsilon+\frac{(1-\alpha)\breve{p}}{2\lambda e^{M}}-\frac{(1-\alpha)\breve{p}}{2\lambda e^{M}}e^{\lambda(t-t^{*})}\leq0, \end{align} | (3.16) |
which yield
\begin{align} t\geq t^{*}+\frac{1}{\lambda}\ln\Big(1+\frac{2\lambda e^{M}\Upsilon}{(1-\alpha)\breve{p}}\Big), \end{align} | (3.17) |
where t^{*} is a priori condition to make inequality (Eq 3.15) true, it is not difficult to find that when t_{0} and x_{0} are determined, one can find the corresponding t^{*} , then according to (3.14)–(3.17) and the fact e^{\int^{t}_{t^{*}}Q(\varsigma)\mathrm{d}\varsigma} > 0 , when t\geq T^{*}(t_{0}, x_{0}) = t^{*}+\frac{1}{\lambda}\ln\Big(1+\frac{2\lambda e^{M}\Upsilon}{(1-\alpha)\breve{p}}\Big) , one can obtain V(t, x(t))\equiv0 . Then the FTS can be finally realized and the settling time can be estimated by T^{*}(t_{0}, x_{0}) .
Remark 3.2. Many previous literatures [21,22,23,24] have used Polyakov's classical finite/fixed-time theorem. In this paper, a term Q(t) is added to the controller u_{i} , and the method of indefinite V -function derivative is used to further popularize Polyakov's finite/fixed-time theorem. We can see that fewer conditions are required in our model and the stable settling time is faster. Our results generalize the previous conclusion.
The corresponding adaptive controller of (3.2)can be designed as
\begin{eqnarray} u_{i}(t) = \left\{\begin{split} \ &Q(t)\mathrm{SIGN}(x_{i}(t))|x_{i}(t)|-\Delta_{i}\mathrm{SIGN}(x_{i}(t))|x_{i}(t)|^{\alpha}-\Pi_{i}\mathrm{SIGN}(x_{i}(t))|x_{i}(t)|^{\beta}, i = 1, 2, \ldots, l, \\ \ &0, \ \ \ \ i = l+1, \ldots, N. \end{split}\right. \end{eqnarray} | (3.18) |
where \Delta_{i} = \mathrm{diag}(\zeta_{i1}, \zeta_{i2}, \ldots, \zeta_{in}) , \Pi_{i} = \mathrm{diag}(\pi_{i1}, \pi_{i2}, \ldots, \pi_{in}) , and for k = 1, 2, \ldots, n the controller rules of \zeta_{ik} , \pi_{ik} satisfy:
\begin{align*} \dot{\zeta}_{ik} = p_{ik}|x_{ik}(t)|^{1+\alpha} \ \mathrm{and} \ \dot{\pi}_{ik} = q_{ik}|x_{ik}(t)|^{1+\beta}, \end{align*} |
where p_{ik} , q_{ik} are adaptive coefficients need to be determined.
Theorem 3.3. If the condition (A) of Theorem 3.1 holds and the activation function f of the system is assumed as the above Hypothesis. The coupled system (3.1) can be fixed-time stabilized by adding the adaptive controller (3.18) and choosing the suitable adaptive coefficients.
Proof. We construct the following Lyapunov function:
\begin{align*} &V(t, x(t)) = \frac{1}{2}\sum\limits^{N}_{i = 1}x^{T}_{i}(t)x_{i}(t)+\sum\limits^{l}_{i = 1}\sum\limits^{n}_{k = 1}\frac{1}{2p_{ik}}\big(\zeta_{ik}-p_{ik}\big)^{2} +\sum\limits^{l}_{i = 1}\sum\limits^{n}_{k = 1}\frac{1}{2q_{ik}}\big(\pi_{ik}-q_{ik}\big)^{2} \end{align*} |
Taking the derivative of V(t, x) along the trajectories of (3.2)
\begin{align} \dot{V}(t, x)& = \sum\limits^{N}_{i = 1}x^{T}_{i}(t)\dot{x}_{i}(t)+\sum\limits^{l}_{i = 1}\sum\limits^{n}_{k = 1}\big(\zeta_{ik}-p_{ik}\big)|x_{ik}(t)|^{1+\alpha} +\sum\limits^{l}_{i = 1}\sum\limits^{n}_{k = 1}\big(\pi_{ik}-q_{ik}\big)|x_{ik}(t)|^{1+\beta}\\ & = \sum\limits^{N}_{i = 1}x^{T}_{i}(t)\Big(-d_{i}x_{i}(t)+\sum\limits^{n}_{j = 1}\widetilde{a}_{ij}(t)f(x_{j}(t))+\sum\limits^{n}_{j = 1}\widetilde{b}_{ij}(t)f(x_{j}(t-\tau)) +\sum\limits^{N}_{j = 1}c_{ij}\phi_{\alpha+1}(x_{j}(t)-x_{i}(t))\\ &+\sum\limits^{N}_{j = 1}c_{ij}\phi_{\beta+1}(x_{j}(t)-x_{i}(t))\Big)+\sum\limits^{N}_{i = 1}x^{T}_{i}(t)u_{i}(t)+\sum\limits^{l}_{i = 1}\sum\limits^{n}_{k = 1}\big(\zeta_{ik}-p_{ik}\big)|x_{ik}(t)|^{1+\alpha} +\sum\limits^{l}_{i = 1}\sum\limits^{n}_{k = 1}\big(\pi_{ik}-q_{ik}\big)|x_{ik}(t)|^{1+\beta} \end{align} | (3.19) |
Through the hypothesis (A) of Theorem 3.1 and recalling the previous discussion (3.4) and (3.5), one can have
\begin{align} \dot{V}(t, x)&\leq\sum\limits^{N}_{j = 1}c_{ij}\phi_{\alpha+1}(x_{j}(t)-x_{i}(t))+\sum\limits^{N}_{j = 1}c_{ij}\phi_{\beta+1}(x_{j}(t)-x_{i}(t))-\sum\limits^{l}_{i = 1}\sum\limits^{n}_{k = 1}p_{ik}|x_{ik}(t)|^{1+\alpha} -\sum\limits^{l}_{i = 1}\sum\limits^{n}_{k = 1}q_{ik}|x_{ik}(t)|^{1+\beta} \end{align} | (3.20) |
Then we return to the evolution in Eq (3.6) in Theorem 3.1, and we can see that the FTS of the coupled system can be achieved by adding the pinning adaptive controller. Moreover, the settling time T(x_{0}, t_{0}) can be estimated by (3.17), the corresponding parameters of (3.17) in this theorem can be adjusted adaptively with the coefficients of the controller (3.18).
Remark 3.4. In fact, in this paper, it is a classical fixed stability problem when Q(t) is equal to 0. This paper breaks the conventional restrictions and uses inequality skill to deduce derivative term of indefinite function. Compared with the previous articles[26,27], the FTS problem is obtained from the two aspects of pinning state controller and pinning adaptive controller. In particular, the coefficient of adaptive adjustment is added to make the parameters more flexible to adapt to the system.
Remark 3.5. In this paper, a novel coupling parameter is introduced to study the fixed time problem, which combines the parameters \alpha , \beta in the controller. Based on Lemma 2.5, the FTS problem can be simplified by converting fewer conditional requirements. In addition, if \breve{q} = 0 in (3.9), we can find that it is the global asymptotic stability or finite-time criteria problem of complex network systems in [9,10,18,19,20]. This method of the indefinite derivative is also applicable. This means that our results can generalize the previous conclusion.
Remark 3.6. In this brief, the finite-time stabilization of the CDMMs is studied mainly by choosing the suitable pinning controller. Compared with other methods, this brief has a wider scope of application and more advantages: 1) The controller only randomly controls a part of nodes. 2) In the proof, the derivative of V function is not necessarily strictly negative. It has an indefinite term, and the calculation is more complex. Meanwhile, this article also has some shortcomings. If we want to achieve finite/fixed-time stabilization, although the convergence speed is faster, it will consume more components, that is, the cost will be higher. Moreover, compared with the traditional Polyakov finite-time theorem [21] used by the V function, when the derivative of the V function has an indefinite term, the calculation is more complex, and it is more difficult to achieve a fixed-time stabilization.
In this section, we introduce two examples and simulations to prove our results' effectiveness. The dimension of the system (3.1) in the example is three-dimensional, and its model and parameters are defined as follows:
\begin{align} \frac{\mathrm{d}x_{i}(t)}{\mathrm{d}t}& = -d_{i}x_{i}(t)+\sum\limits^{n}_{j = 1}\widetilde{a}_{ij}(t)f(x_{j}(t))+\sum\limits^{n}_{j = 1}\widetilde{b}_{ij}(t)f(x_{j}(t-\tau)) \\ &+\sum\limits^{N}_{j = 1}c_{ij}\phi_{\alpha+1}(x_{j}(t)-x_{i}(t))+\sum\limits^{N}_{j = 1}c_{ij}\phi_{\beta+1}(x_{j}(t)-x_{i}(t))+u_{i}(t), \end{align} | (4.1) |
where d_{1} = d_{2} = d_{3} = 9 ; f(\cdot) = \begin{pmatrix} \tanh(\cdot) \\ \tanh(\cdot) \\ \tanh(\cdot) \end{pmatrix} . Moreover, A and B are expressed as follows:
A = \begin{pmatrix} a_{11} & 0.3 & 0.5 \\ 0.2 & a_{22} & 0.3 \\ 0.1 & 0.5 & a_{33} \end{pmatrix} ; B = \begin{pmatrix} b_{11} & 0.1 & 0.8 \\ -0.1 & b_{22} & 0.8 \\ 0.2 & 1 & b_{33} \end{pmatrix} ;
where
\begin{equation*} a_{11}(t) = \left\{\begin{split} \ &1.6256, \ h_{1}(x(t)) < r_{1} \\ \ &[1.4325, 1.6256], \ |h_{1}(x(t))| = r_{1} \\ \ &1.4325, \ h_{1}(x(t)) > r_{1} \end{split}\right. \ \ \ \ b_{11}(t) = \left\{\begin{split} \ &-1.1236, \ h_{1}(x(t)) < r_{1} \\ \ &[-1.1236, 1.8265], \ |h_{1}(x(t))| = r_{1} \\ \ &1.8265, \ h_{1}(x(t)) > r_{1} \end{split}\right. \end{equation*} |
\begin{equation*} a_{22}(t) = \left\{\begin{split} \ &2.1234, \ h_{2}(x(t)) < r_{2} \\ \ &[1.2345, 2.1234], \ |h_{2}(x(t))| = r_{2} \\ \ &1.2345, \ h_{2}(x(t)) > r_{2} \end{split}\right. \ \ \ \ b_{22}(t) = \left\{\begin{split} \ &-1.2266, \ h_{2}(x(t)) < r_{2} \\ \ &[-2.1245, -1.2266], \ |h_{2}(x(t))| = r_{2} \\ \ &-2.1245, \ h_{2}(x(t)) > r_{2} \end{split}\right. \end{equation*} |
\begin{equation*} a_{33}(t) = \left\{\begin{split} \ &0.1745, \ h_{3}(x(t)) < r_{3} \\ \ &[0.1745, 0.8992], \ |h_{3}(x(t))| = r_{3} \\ \ &0.8325, \ h_{3}(x(t)) > r_{3} \end{split}\right. \ \ \ \ b_{33}(t) = \left\{\begin{split} \ &-1.2148, \ h_{3}(x(t)) < r_{3} \\ \ &[-1.2148, 1.7462], \ |h_{3}(x(t))| = r_{3} \\ \ &1.6999, \ h_{3}(x(t)) > r_{3} \end{split}\right. \end{equation*} |
Example 4.1. Consider the model (4.1) i.e., it is easy to check that the neuron function satisfies the Hypothesis 1 with h^{\max} = 1 . Moreover, let N = 6 , we randomly select a relationship between different neurons of coupling network, and its topology rule and the corresponding adjacency matrix C can be shown as follows:
![]() |
and the corresponding Laplace is L , one can see that \lambda_{\min}(L) = 0 . Then we choose the control rule by u_{i}(t) = Q(t)\mathrm{SIGN}(x_{i}(t))|x_{i}(t)|-p_{i}\mathrm{SIGN}(x_{i}(t))|x_{i}(t)|^{\alpha}-q_{i}\mathrm{SIGN}(x_{i}(t))|x_{i}(t)|^{\beta} with Q(t) = \frac{1}{1+t^{2}} , p_{i} = q_{i} = 1 for i = 1, 2 , and \alpha = 0.5 , \beta = 2 , one can check that:
\begin{align*} d^{\min} = 9 > 8.49 = h^{\max}\cdot\max\limits_{k, l\in {\mathbb N}}\{\sup\limits_{t\in {\mathbb R}}|\widetilde{a}_{kl}(t)|\}+nh^{\max}\cdot\max\limits_{k, l\in {\mathbb N}}\{\sup\limits_{t\in {\mathbb R}}|\widetilde{b}_{kl}(t)|\}, \end{align*} |
then Hypothesis (A) holds. By using Theorem 3.1, the coupled system (4.1) can be fixed-time stabilized by randomly choosing 3 groups of initial values 2.5, -0.5 and -3. The simulation results are described by Figure 1(a). Moreover, the settling time can be estimated as T^{*}(t_{0}, x^{0}) = 0.8 .
Example 4.2. Recalling (4.1) again, let N = 4 , and the topological connection of the coupling neurons network and the corresponding configuration matrix are illustrated by the following structure:
![]() |
which easily have \lambda_{\min}(L) = 0 .
Then we choose the control rule u_{i}(t) in (3.18) and only control the first three nodes. The same as Example 4.1, we can see that the Hypothesis ({\bf A}) is true. By using Theorem 3.3, the coupled system (4.1) can be fixed-time stabilized by randomly choosing 3 groups of initial values 0.8, 6.2 and -8. The simulation results are described by Figure 1(b), the settling time can be estimated as T^{*}(t_{0}, x^{0}) = 0.9 .
Remark 4.3. Through the above two examples, we can find that the number of control nodes in the example is randomly selected, and the initial value is also arbitrary. According to the simulation image, it can be seen that the stability of the system can be achieved quickly, and the stability of the system has nothing to do with the selection of the initial value and the number of nodes. This fully demonstrates the advanced nature and effectiveness of our results. We can see from Figure 1(c) and Figure 1(d) that when the controller is cancelled, the system settling time T_{0} is obviously longer than that with the controller.
This paper introduces the FTS problem of CMNNs system. The main method is to establish a novel state-dependent pinning controller and the corresponding adaptive pinning controller in the form of vector based on Lyapunov functional and undirected topological graph theory. In addition, we use the method of the indefinite derivative to solve the FTS issue of CMNNs with nonlinear coupling, which is independent of the initial value. The limit that the conventional V -function must be negative definite is broken. Finally, through experimental analysis and numerical simulation, it is verified that the experimental and theoretical methods in this brief are effective. Moreover, the control method and the calculation technology of the indefinite derivative established in this brief are relatively novel and can be extended to many fields, such as recurrent neural networks [4,5], statistical language modeling [6], stochastic memristive chaotic systems [10,35,36,37], muti-agent systems [14], fuzzy neural networks [38], chaotic systems [39] and so on.
This work is supported by the National Natural Science Foundation of China (11801042, 12171056 and 11671011), Training Program for Excellent Young Innovators of Changsha (kq20106072) and Scientific Research Foundation of Hunan Provincial Education Department (21B0771).
The authors declare there is no conflicts of interest.
Data sharing allows researchers to verify the results of the article, replicate the analysis, and conduct secondary analyses. The data used to support the findings of this study are available from the corresponding author upon request.
[1] |
Pandey CL (2021) Managing urban water security: challenges and prospects in Nepal. Environ Dev Sustain 23: 241–257. https://doi.org/10.1007/s10668-019-00577-0 doi: 10.1007/s10668-019-00577-0
![]() |
[2] | IUCN (World Conservation Union) (2000). Vision for Water and Nature. A World Strategy for conservation and sustainable management of water resources in the 21st Century. IUCN, Gland, Switzerland and Cambridge, UK, p 52. |
[3] |
Perez-Blanco CD, Gil-Garcia L, Saiz-Santiago (2021) An actionable hydroeconomic descision support system for the assessment of water reallocations in irrigated agriculture. Astudy of minimum environmental flows in the Douro River Basin, Spain. J Environ Managet 298: 113432. https://doi.org/10.1016/j.jenvman.2021.113432 doi: 10.1016/j.jenvman.2021.113432
![]() |
[4] |
Senent-Aparicio J, George C, Srinivasan R (2021) Introducing a new post-processing tool for the SWAT+model to evaluate environmental flows. Environ Modell Softw 136: 104944. https://doi.org/10.1016/j.envsoft.2020.104944 doi: 10.1016/j.envsoft.2020.104944
![]() |
[5] |
Zolfagharpour F, Saghafian B, Delavar M (2021) Adapting reservoir operation rules to hydrological drought state and environmental flow requirements. J Hydrol 660: 126581. https://doi.org/10.1016/j.jhydrol.2021.126581 doi: 10.1016/j.jhydrol.2021.126581
![]() |
[6] |
Sedighkia M, Datta B (2023) Analyzing environmental flow supply in the semi-arid area through integrating drought analysis and optimal operation of reservoir. J Arid Land 15: 1439–1454. https://doi.org/10.1007/s40333-023-0035-2 doi: 10.1007/s40333-023-0035-2
![]() |
[7] |
Aazami J, Motevalli A, Savabieasfahani M (2022) Correction to: Evaluation of three environmental flow techniques in Shoor wetland of Golpayegan, Iran. Int J Environ Sci Technol 19: 7899. https://doi.org/10.1007/s13762-022-04289-3 doi: 10.1007/s13762-022-04289-3
![]() |
[8] | Wang H, Cong P, Zhu Z, et al. (2022) Analysis of environmental dispersion in wetland flows with floating vegetation islands. J Hydrol 606: 127359. https://doi.org/10.1016/j.jhydrol.2021.127359 |
[9] |
Arthington ÁH, Naiman RJ, Mcclain ME, et al. (2010). Preserving the biodiversity and ecological services of rivers: new challenges and research opportunities. Freshwater Biol 55: 1–16. https://doi.org/10.1111/j.1365-2427.2009.02340.x doi: 10.1111/j.1365-2427.2009.02340.x
![]() |
[10] |
Chilton D, Hamilton DP, Nagelkerken I, et al. (2021). Environmental flow requirements of estuaries: providing resilience to current and future climate and direct anthropogenic changes. Front Environ Sci 9: 764218. https://doi.org/10.3389/fenvs.2021.764218 doi: 10.3389/fenvs.2021.764218
![]() |
[11] |
Espinoza T, Burke CL, Carpenter-Bundhoo L, et al. (2021) Quantifying movement of multiple threatened species to inform adaptive management of environmental flows. J Environ Managet 295: 113067. https://doi.org/10.1016/j.jenvman.2021.113067 doi: 10.1016/j.jenvman.2021.113067
![]() |
[12] |
Zhang Y, Yu H, Yu H, et al. (2020) Optimization of environmental variables in habitat suitability modeling for mantis shrimp Oratosquilla oratoria in the Haizhou Bay and adjacent waters. Acta Oceanol Sin 39: 36–47. https://doi.org/10.1007/s13131-020-1546-8 doi: 10.1007/s13131-020-1546-8
![]() |
[13] |
Vivian LM, Godfree RC, Colloff MJ, et al. (2014) Wetland plant growth under contrasting water regimes associated with river regulation and drought: implications for environmental water management. Plant Ecol 215: 997–1011. https://doi.org/10.1007/s11258-014-0357-4 doi: 10.1007/s11258-014-0357-4
![]() |
[14] |
Gain AK, Apel H, Renaud FG, et al. (2013) Thresholds of hydrologic flow regime of a river and investigation of climate change impact—the case of the Lower Brahmaputra river Basin. Climatic Change 120: 463–475. https://doi.org/10.1007/s10584-013-0800-x doi: 10.1007/s10584-013-0800-x
![]() |
[15] |
Belmar O, Velasco J, Martinez-Capel F (2011) Hydrological Classification of Natural Flow Regimes to Support Environmental Flow Assessments in Intensively Regulated Mediterranean Rivers, Segura River Basin (Spain). Environ Manage 47: 992–1004. https://doi.org/10.1007/s00267-011-9661-0 doi: 10.1007/s00267-011-9661-0
![]() |
[16] |
Dumitriu D (2020) Sediment flux during flood events along the Trotuș River channel: hydrogeomorphological approach. J Soils Sediments 20: 4083–4102. https://doi.org/10.1007/s11368-020-02763-4 doi: 10.1007/s11368-020-02763-4
![]() |
[17] |
Bouska K (2020) Regime change in a large-floodplain river ecosystem: patterns in body-size and functional biomass indicate a shift in fish communities. Biol Invasions 22: 3371–3389. https://doi.org/10.1007/s10530-020-02330-5 doi: 10.1007/s10530-020-02330-5
![]() |
[18] |
Poff NL, Allan JD, Bain MB, et al. (1997). The natural flow regime. Bio Science 47: 769–784. https://doi.org/10.2307/1313099 doi: 10.2307/1313099
![]() |
[19] | Nasiri Khiavi A, Mostafazadeh R, Ghanbari Talouki F (2024) Using game theory algorithm to identify critical watersheds based on environmental flow components and hydrological indicators. Environ Dev Sustain https://doi-org.access.semantak.com/10.1007/s10668-023-04390-8 |
[20] |
Sedighkia M, Abdoli A (2023) Design of optimal environmental flow regime at downstream of multireservoir systems by a coupled SWAT-reservoir operation optimization method. Environ Dev Sustain 25: 834–854. https://doi.org/10.1007/s10668-021-02081-w doi: 10.1007/s10668-021-02081-w
![]() |
[21] |
Yu L, Wu X, Wu S, et al. (2021) Multi-objective optimal operation of cascade hydropower plants considering ecological flow under different ecological conditions. J Hydrol 601: 126599. https://doi.org/10.1016/j.jhydrol.2021.126599 doi: 10.1016/j.jhydrol.2021.126599
![]() |
[22] |
Mlynski D, Operacz A, Walega A (2020) Sensitivity of methods for calculating environmental flows based on hydrological characteristics of watercourses regarding te hydropower potential of rivers. J Clean Pro 250: 119527. https://doi.org/10.1016/j.jclepro.2019.119527 doi: 10.1016/j.jclepro.2019.119527
![]() |
[23] |
Bejarano MD, Sord-Ward A, Gabriel-Martin I, et al. (2019) Tradeoff between economic and environmental costs and benefits of hydropower production at run-of-river-diversion schemes under different environmental flows scenarios. J Hydrol 572: 790–804. https://doi.org/10.1016/j.jhydrol.2019.03.048 doi: 10.1016/j.jhydrol.2019.03.048
![]() |
[24] | Bovee KD (1982) Instream flow methodology. US Fish and Wildlife Service. FWS/OBS, 82, 26 |
[25] |
Stucchi L. Bocchiola D (2023) Environmental Flow Assessment using multiple criteria: A case study in the Kumbih river, West Sumatra (Indonesia). Sci Total Environ 901: 166516. https://doi.org/10.1016/j.scitotenv.2023.166516 doi: 10.1016/j.scitotenv.2023.166516
![]() |
[26] |
Mwamila TB, Kimwaga RJ, Mtalo FW (2008) Eco-hydrology of the Pangani River downstream of Nyumba ya Mungu reservoir, Tanzania. Phys Chem Earth 33: 695–700. https://doi.org/10.1016/j.pce.2008.06.054 doi: 10.1016/j.pce.2008.06.054
![]() |
[27] |
Nagaya T, Shiraishi Y, Onitsuka K, et al (2008) Evaluation of suitable hydraulic conditions for spawning of ayu with horizontal 2D numerical simulation and PHABSIM. Ecol Modell 215: 133–143. https://doi.org/10.1016/j.ecolmodel.2008.02.043 doi: 10.1016/j.ecolmodel.2008.02.043
![]() |
[28] |
Nikghalb S, Shokoohi A, Singh VP et al (2016) Ecological Regime versus Minimum Environmental Flow:Comparison of Results for a River in a Semi Mediterranean Region. Water Resour Manage 30: 4969–4984. https://doi.org/10.1007/s11269-016-1488-2 doi: 10.1007/s11269-016-1488-2
![]() |
[29] |
Spence R, Hickley P (2000) The use of PHABSIM in the management of water resources and fisheries in England and Wales. Ecol Eng 16: 153–158. https://doi.org/10.1016/S0925-8574(00)00099-9 doi: 10.1016/S0925-8574(00)00099-9
![]() |
[30] |
Miao Y, Li J, Feng P, et al. (2020) Effects of land use changes on the ecological operation of the Panjiakou-Daheiting Reservoir system, China Ecol Eng 152: 10585. https://doi.org/10.1016/j.ecoleng.2020.105851 doi: 10.1016/j.ecoleng.2020.105851
![]() |
[31] |
Dana K, Jana C, Tomáš V (2017) Analysis of environmental flow requirements for macroinvertebrates in a creek affected by urban drainage (Prague metropolitan area, Czech Republic). Urban Ecosyst 20: 785–797. https://doi.org/10.1007/s11252-017-0649-2 doi: 10.1007/s11252-017-0649-2
![]() |
[32] |
Weng X, Jiang C, Yuan M, et al. (2021) An ecologically dispatch strategy using environmental flows for a cascade multi-sluice system: A case study of the Yongjiang River Basin, China Ecol Indic 121: 107053. https://doi.org/10.1016/j.ecolind.2020.107053 doi: 10.1016/j.ecolind.2020.107053
![]() |
[33] |
Knack IM, Huang F, Shen HT (2020) Modeling fish habitat condition in ice affected rivers. Cold Reg Sci Technol 176: 103086. https://doi.org/10.1016/j.coldregions.2020.103086 doi: 10.1016/j.coldregions.2020.103086
![]() |
[34] |
Peng L, Sun L (2016) Minimum instream flow requirement for the water-reduction section of diversion-type hydropower station: a case study of the Zagunao River, China. Environ Earth Sci 75: 1210. https://doi.org/10.1007/s12665-016-6019-1 doi: 10.1007/s12665-016-6019-1
![]() |
[35] |
Gholami V, Khalili A, Sahour H, et al. (2020) Assessment of environmental water requirement for rivers of the Miankaleh wetland drainage basin. Appl Water Sci 10: 233. https://doi.org/10.1007/s13201-020-01319-8 doi: 10.1007/s13201-020-01319-8
![]() |
[36] |
Im D, Choi SU, Choi B (2018) Physical habitat simulation for a fish community using the ANFIS method. Ecol Inform 43: 73-83. https://doi.org/10.1016/j.ecoinf.2017.09.001 doi: 10.1016/j.ecoinf.2017.09.001
![]() |
[37] |
Zamanzad-Ghavidel S, Fazeli S, Mozaffari S, et al. (2023) Estimating of aqueduct water withdrawal via a wavelet-hybrid soft-computing approach under uniform and non-uniform climatic conditions. Environ Dev Sustain 25: 5283–5314. https://doi.org/10.1007/s10668-022-02265-y doi: 10.1007/s10668-022-02265-y
![]() |
[38] | Emadi A, Sobhani R, Ahmadi H, et al. Multivariate modeling of agricultural river water abstraction via novel integrated-wavelet methods in various climatic conditions. Environ Dev Sustain 24: 4845–4871. https://doi.org/10.1007/s10668-021-01637-0 |
[39] | Amanollahi J, Ausati Sh (2020a) PM2.5 concentration forecasting using ANFIS, EEMD-GRNN, MLP, and MLR models: A case study of Tehran, Iran. Air Qual Atmos Health 13: 161–17. https://doi.org/10.1007/s11869-019-00779-5 |
[40] |
Ghasemi A, Amanollahi J (2019) Integration of ANFIS model and forward selection method for air quality forecasting. Air Qual Atmos Health 12: 59–72. https://doi.org/10.1007/s11869-018-0630-0 doi: 10.1007/s11869-018-0630-0
![]() |
[41] |
Kaboodvandpour S, Amanollahi J, Qhavami S, et al. (2015) Assessing the accuracy of multiple regressions, ANFIS, and ANN models in predicting dust storm occurrences in Sanandaj. Iran Nat Hazards 78: 879–893. https://doi.org/10.1007/s11069-015-1748-0 doi: 10.1007/s11069-015-1748-0
![]() |
[42] |
Bice CM, Gehrig SL, Zampatti BP, et al. (2014) Flow-induced alterations to fish assemblages, habitat and fish–habitat associations in a regulated lowland river. Hydrobiologia 722: 205–222. https://doi.org/10.1007/s10750-013-1701-8 doi: 10.1007/s10750-013-1701-8
![]() |
[43] |
Kim HJ, Kim JH, Ji U, et al. (2020) Effect of Probability Distribution-Based Physical Habitat Suitability Index on Environmental-Flow Estimation. KSCE. J Civ Eng 24: 2393–2402. https://doi.org/10.1007/s12205-020-1923-z doi: 10.1007/s12205-020-1923-z
![]() |
[44] | EPA, 2015. Fish Field Sampling. SESDPROC-512-R4. |
[45] | Standard Methods for the Examination of Water and Wastewater, 1999. 20th Edition, Part 10600 B, Electrofishing, P 2206. |
[46] |
Van der Lee GEM, Van der Molen DT, Van den Boogaard HFP, et al. (2006) Uncertainty analysis of a spatial habitat suitability model and implications for ecological management of water bodies. Landscape Ecol 21: 1019–1032. https://doi.org/10.1007/s10980-006-6587-7 doi: 10.1007/s10980-006-6587-7
![]() |
[47] |
Sekine M, Wang J, Yamamoto K, et al. (2020) Fish habitat evaluation based on width-to-depth ratio and eco-environmental diversity index in small rivers. Environ Sci Pollut Res 27: 34781–34795. https://doi.org/10.1007/s11356-020-08691-7 doi: 10.1007/s11356-020-08691-7
![]() |
[48] |
Wen X, Lv Y, Liu Z, et al. (2021) Operation chart optimization of multi-hydropower system incorporating the long- and short-term fish habitat requirements. J Clen Pro 121: 107053. https://doi.org/10.1016/j.jclepro.2020.125292 doi: 10.1016/j.jclepro.2020.125292
![]() |
[49] |
Jung SH, Choi S-Uk (2015) Prediction of composite suitability index for physical habitatsimulations using the ANFIS method. Appl Soft Comput 34: 502–512. https://doi.org/10.1016/j.asoc.2015.05.028 doi: 10.1016/j.asoc.2015.05.028
![]() |
[50] |
Fraternali P, Castelletti A, Soncini-Sessa R, et al. (2012) Putting humans in the loop: social computing for Water Resources Management. Environ Modell Softw 37: 68–77. https://doi.org/10.1016/j.envsoft.2012.03.002 doi: 10.1016/j.envsoft.2012.03.002
![]() |
[51] |
Rinderknecht SL, Borsuk ME, Reichert P (2012) Bridging uncertain and ambiguous knowledge with imprecise probabilities. Environ Modell Softw 36: 122–130. https://doi.org/10.1016/j.envsoft.2011.07.022 doi: 10.1016/j.envsoft.2011.07.022
![]() |
[52] |
Fukuda S (2009) Consideration of fuzziness: Is it necessary in modelling fish habitat preference of Japanese medaka (Oryzias latipes)? Ecol Model 220: 2877–2884. https://doi.org/10.1016/j.ecolmodel.2008.12.025 doi: 10.1016/j.ecolmodel.2008.12.025
![]() |
[53] |
Fukuda S, Hiramatsu B (2008) Prediction ability and sensitivity of artificial intelligence-based habitat preference models for predicting spatial distribution of Japanese medaka (Oryzias latipes). Ecol Model 215: 301–313. https://doi.org/10.1016/j.ecolmodel.2008.03.022 doi: 10.1016/j.ecolmodel.2008.03.022
![]() |
[54] |
Dawson, CW, Wilby R L (2001) Hydrological modelling using artificial neural networks. Progr Phys Geogr 25: 80–108. https://doi.org/10.1177/030913330102500104 doi: 10.1177/030913330102500104
![]() |
[55] |
Sykes AO (1993) An Introduction to Regression Analysis. Am Stat 61: 101. https://doi.org/10.1198/tas.2007.s74 doi: 10.1198/tas.2007.s74
![]() |
[56] | Kayes I, Shahriar SA, Hasan K, et al. (2019) The relationships between meteorological parameters and air pollutants in an urban environment. Global J Environ Sci Manag 5: 265–278. |
[57] |
Ceylan Ζ, Bulkan S (2018) Forecasting PM10 levels using ANN and MLR: A case study for Sakarya City. Global Nest J 20: 281–290. https://doi.org/10.30955/gnj.002522 doi: 10.30955/gnj.002522
![]() |
[58] |
Karatzas K, Katsifarakis N, Orlowski C, et al. (2018) Revisiting urban air quality forecasting: a regression approach. Vietnam J Comput Sci 5: 177–184. https://doi.org/10.1007/s40595-018-0113-0 doi: 10.1007/s40595-018-0113-0
![]() |
[59] |
Mishra D, Goyal P (2015) Development of artificial intelligence based NO2 forecasting models at Taj Mahal, Agra. Atmos Pollut Res 6: 99–106. https://doi.org/10.5094/APR.2015.012 doi: 10.5094/APR.2015.012
![]() |
[60] |
Mishra D, Goyal P (2016) Neuro-Fuzzy approach to forecasting ozone episodes over the urban area of Delhi, India. Environ Technol Inno 5: 83–94. https://doi.org/10.1016/j.eti.2016.01.001 doi: 10.1016/j.eti.2016.01.001
![]() |
[61] |
Rahimi A (2017) Short-term prediction of NO 2 and NO x concentrations using multilayer perceptron neural network: a case study of Tabriz, Iran. Ecol Process 6: 4. https://doi.org/10.1186/s13717-016-0069-x doi: 10.1186/s13717-016-0069-x
![]() |
[62] |
Abba S, Hadi SJ, Abdullahi J (2017) River water modelling prediction using multi linear regression, artificial neural network, and adaptive neuro-fuzzy inference system techniques. Procedia Comput Sci 120: 75–82. https://doi.org/10.1016/j.procs.2017.11.212 doi: 10.1016/j.procs.2017.11.212
![]() |
[63] |
Abba SI, Elkirn G (2017) Effluent prediction of chemical oxygen demand from the astewater treatment plant using artificial neural network application. Procedia Comput Sci 120: 156–163. https://doi.org/10.1016/j.procs.2017.11.223 doi: 10.1016/j.procs.2017.11.223
![]() |
[64] |
Mohammadi S, Naseri F, Abri R (2019) Simulating soil loss rate in Ekbatan Dam watershed using experimental and statistical approaches. Int J Sediment Res 34: 226–239. https://doi.org/10.1016/j.ijsrc.2018.10.013 doi: 10.1016/j.ijsrc.2018.10.013
![]() |
[65] |
Amanollahi J, Ausati Sh (2020b). Validation of linear, nonlinear, and hybrid models for predicting particulate matter concentration in Tehran. Iran. Theor Appl Climatol 140: 709–717. https://doi.org/10.1007/s00704-020-03115-5 doi: 10.1007/s00704-020-03115-5
![]() |
[66] | Jorde K, Schneider M, Peter A, et al. (2001) Fuzzy based Models for the Evaluation of Fish Habitat Quality and Instream Flow Assessment. Proceedings of the 2001 International Symposium on Environmental Hydraulics Copyright © 2001, ISEH. |
[67] |
Fukuda Sh, Hiramatsu K, Mori M (2006) Fuzzy neural network model for habitat prediction and HEP for habitat quality estimation focusing on 61 Japanese medaka (Oryzias latipes) in agricultural canals. Paddy Water Environ 4: 119–124. https://doi.org/10.1007/s10333-006-0039-5 doi: 10.1007/s10333-006-0039-5
![]() |
[68] |
Marsili-Libelli S, Giusti E, Nocita A (2012) A new instream flow assessment method based on fuzzy habitat suitability and large scale river modelling. Environ Modell Softw 41: 27–38. https://doi.org/10.1016/j.envsoft.2012.10.005 doi: 10.1016/j.envsoft.2012.10.005
![]() |
[69] |
Junga SH, Choib S-Uk (2015) Prediction of composite suitability index for physical habitatsimulations using the ANFIS method. Appl Soft Comput 34: 502–512. https://doi.org/10.1016/j.asoc.2015.05.028 doi: 10.1016/j.asoc.2015.05.028
![]() |
[70] |
Im D, Choi S-U, Choi B (2017) Physical habitat simulation for a fish community using the ANFIS method. Ecol Inform 43: 73–83. https://doi.org/10.1016/j.ecoinf.2017.09.001 doi: 10.1016/j.ecoinf.2017.09.001
![]() |
[71] | Elkiran G, Nourani V, Abba1 SI, et al. (2018) Artificial intelligence-based approaches for multi-station modelling of dissolve oxygen in river. Global J Environ Sci Manage 4: 439–450. |