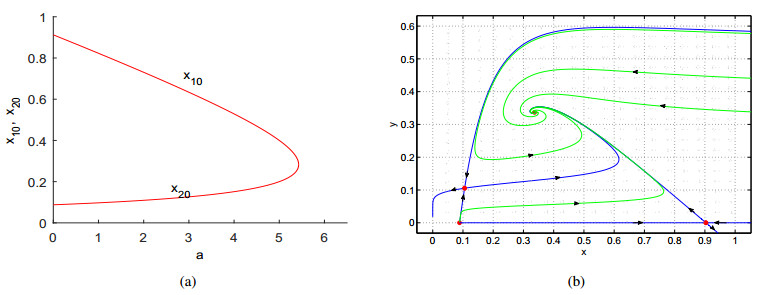
A Leslie-Gower predator-prey model with Smith growth and constant-yield harvesting is proposed in this paper. We show that the system admits at most two boundary equilibria, both of which are unstable. The degenerate positive equilibrium of the system is a cusp of codimension 2, and the system undergoes cusp-type Bogdanov-Takens bifurcation of codimension 2. Moreover, we prove that the system has a weak focus of order at most 3, and the system can undergo a degenerate Hopf bifurcation of codimension 3. Our results reveal that the constant-yield harvesting can lead to richer dynamic behaviors.
Citation: Mengxin He, Zhong Li. Dynamic behaviors of a Leslie-Gower predator-prey model with Smith growth and constant-yield harvesting[J]. Electronic Research Archive, 2024, 32(11): 6424-6442. doi: 10.3934/era.2024299
[1] | Yichao Shao, Hengguo Yu, Chenglei Jin, Jingzhe Fang, Min Zhao . Dynamics analysis of a predator-prey model with Allee effect and harvesting effort. Electronic Research Archive, 2024, 32(10): 5682-5716. doi: 10.3934/era.2024263 |
[2] | Jiani Jin, Haokun Qi, Bing Liu . Hopf bifurcation induced by fear: A Leslie-Gower reaction-diffusion predator-prey model. Electronic Research Archive, 2024, 32(12): 6503-6534. doi: 10.3934/era.2024304 |
[3] | Yuan Tian, Yang Liu, Kaibiao Sun . Complex dynamics of a predator-prey fishery model: The impact of the Allee effect and bilateral intervention. Electronic Research Archive, 2024, 32(11): 6379-6404. doi: 10.3934/era.2024297 |
[4] | Jiange Dong, Xianyi Li . Bifurcation of a discrete predator-prey model with increasing functional response and constant-yield prey harvesting. Electronic Research Archive, 2022, 30(10): 3930-3948. doi: 10.3934/era.2022200 |
[5] | Yifan Wu, Xiaohui Ai . Analysis of a stochastic Leslie-Gower predator-prey system with Beddington-DeAngelis and Ornstein–Uhlenbeck process. Electronic Research Archive, 2024, 32(1): 370-385. doi: 10.3934/era.2024018 |
[6] | Jialu Tian, Ping Liu . Global dynamics of a modified Leslie-Gower predator-prey model with Beddington-DeAngelis functional response and prey-taxis. Electronic Research Archive, 2022, 30(3): 929-942. doi: 10.3934/era.2022048 |
[7] | San-Xing Wu, Xin-You Meng . Hopf bifurcation analysis of a multiple delays stage-structure predator-prey model with refuge and cooperation. Electronic Research Archive, 2025, 33(2): 995-1036. doi: 10.3934/era.2025045 |
[8] | Fengrong Zhang, Ruining Chen . Spatiotemporal patterns of a delayed diffusive prey-predator model with prey-taxis. Electronic Research Archive, 2024, 32(7): 4723-4740. doi: 10.3934/era.2024215 |
[9] | Xin Du, Quansheng Liu, Yuanhong Bi . Bifurcation analysis of a two–dimensional p53 gene regulatory network without and with time delay. Electronic Research Archive, 2024, 32(1): 293-316. doi: 10.3934/era.2024014 |
[10] | Mengting Sui, Yanfei Du . Bifurcations, stability switches and chaos in a diffusive predator-prey model with fear response delay. Electronic Research Archive, 2023, 31(9): 5124-5150. doi: 10.3934/era.2023262 |
A Leslie-Gower predator-prey model with Smith growth and constant-yield harvesting is proposed in this paper. We show that the system admits at most two boundary equilibria, both of which are unstable. The degenerate positive equilibrium of the system is a cusp of codimension 2, and the system undergoes cusp-type Bogdanov-Takens bifurcation of codimension 2. Moreover, we prove that the system has a weak focus of order at most 3, and the system can undergo a degenerate Hopf bifurcation of codimension 3. Our results reveal that the constant-yield harvesting can lead to richer dynamic behaviors.
The traditional predator–prey model was built to explain the survival of marine fish, which has been extensively researched and improved to more accurately describe the development of populations. However, there is no upper limit to the growth rate of predators, which is not suitable for most populations in nature. Based on this fact, Leslie [1,2] considered that the growth rates of both predator and prey have upper limits by assuming that the "carrying capacity" of the predator's environment is proportional to the number of prey and proposed the following well-known Leslie–Gower model:
˙x=rx(1−xK)−βxy,˙y=δy(1−ynx), | (1.1) |
where ynx denotes the Leslie–Gower term and nx is the "carrying capacity" of the predator. Korobeinikov [3] successively verified that the fixed point of the Leslie–Gower model is globally stable. System (1.1) was modified by introducing different types of functional response, and the stability and bifurcations were analyzed [4,5,6,7,8,9,10].
In the above system, the average growth rate ˙xx of the traditional logistic model is a linear function of the prey. However, in 1963, Smith [11] found that the average growth rate of large daphnia populations does not follow linear functions and demonstrated that food is needed for the maintenance and growth of the population during the growth stage but is only required for maintenance when the population reaches saturation. Then proposed the "limited food" model, sometimes called the "limited resources" model. When introducing Smith growth into system (1.1), the system takes the following form:
˙x=rx(K−xK+ax)−βxy,˙y=δy(1−ynx), | (1.2) |
where rx(K−xK+ax) is the Smith growth function. With the influence of environmental toxicants, Kumar [12] discussed the spatiotemporal dynamics and delay-induced instability of a Leslie-Gower prey-predator model with Smith growth rate. Li and He [13] studied the Hopf bifurcation of a delayed food-limited model with feedback control; Feng et al. [14] considered the stability and Hopf bifurcation of a modified Leslie-Gower predator–prey model with Smith growth rate and Beddington-DeAngelis functional response; Bai et al. [15] proposed a predator–prey model with a Smith growth function and the additive predation in prey and studied the stability and Hopf bifurcation of the system. For more results on dynamical behaviors of systems with Smith growth function, please see[16,17].
On the other hand, sustainable harvesting plays an important role in maintaining ecological balance, and its influence on population development has been extensively studied. Huang et al. [18] investigated the bifurcation of predator-prey models with constant-yield prey harvesting. Xu et al. [19] discussed the degenerate Bogdanov-Takens bifurcation of a Holling-Tanner predator-prey model with constant-yield prey harvesting. Wu et al. [20] studied the bifurcations such as the degenerate Bogdanov-Takens bifurcation and degenerate Hopf bifurcation of a Holling-Tanner model with generalist predator and constant-yield prey harvesting. Zhu and Lan [21] modified system (1.1) by introducing constant-yield prey harvesting
˙x=rx(1−xK)−βxy−H,˙y=δy(1−ynx), | (1.3) |
where H>0 is the constant-yield prey harvesting. The authors discussed the saddle node bifurcation, supercritical, and subcritical Hopf bifurcations of the system.
Motivated by systems (1.2) and (1.3), in this paper we propose the following system:
˙x=rx(K−xK+ax)−βxy−H,˙y=δy(1−ynx), | (1.4) |
where x(t) and y(t) are the densities of the prey and predator at time t, respectively; r>0 and δ>0 are the intrinsic growth rates of the prey and predator, respectively; K>0 is the carrying capacity of the prey; β>0 is the maximum rate of predation; H>0 is the constant-yield prey harvesting; ra with a>0 is the mass substitution rate of the population at K; n>0 is the quality of prey provided to the predator.
For simplicity, we make the following transformations:
¯x=xK, ¯y=ynK, ¯t=rt, |
and drop the bars, then system (1.4) can be rewritten as
˙x=x(1−x)1+ax−bxy−h,˙y=sy(1−yx), | (1.5) |
where
b=βnKr, h=HrK, s=δr. |
We can easily verify that all the solutions of system (1.5) are positive and bounded with positive initial conditions.
The rest of the paper is organized as follows: In Section 2, we will investigate the existence and stability of the boundary and positive equilibria of system (1.5). In Section 3, we will prove the Bogdanov–Takens bifurcation of codimension 2 and the degenerate Hopf bifurcation of codimension 3. In Section 4, we will present some bifurcation diagrams and phase portraits to support our theoretical results. We give a brief discussion in the last section.
In this section, we discuss types of the boundary and positive equilibria of system (1.5) in a positive invariant and bounded region
Ω={(x,y)|0<x<1, 0≤y<1}. |
For any nonnegative equilibrium E(x,y), the Jacobian matrix of system (1.5) is
JE=(1−2x−ax2(1+ax)2−by−bxsy2x2s(1−2yx)), |
and
Det(JE)=s(1−2x−ax2(1+ax)2−by)(1−2yx)+sby2x,Tr(JE)=1−2x−ax2(1+ax)2−by−2syx+s. |
Substituting y=0 into the first equation of (1.5), we have
f0(x)=x2+(ah−1)x+h, |
whose discriminant is
Δf0=(1−ah)2−4h. |
Denote
x10=1−ah−√Δf02, x20=1−ah+√Δf02 and ˉx∗=1−ah2. |
Then we have the following theorem.
Theorem 2.1. For the boundary equilibria of system (1.5), we have:
(1) If h<14 and 0<a<1−2√hh, then system (1.5) has two boundary equilibria: an unstable node E10(x10,0) and a saddle E20(x20,0);
(2) if h<14 and a=1−2√hh, then system (1.5) has a unique boundary equilibrium ˉE∗(ˉx∗,0), which is a saddle node, including an unstable parabolic sector in the left.
Proof. If ah<1 and Δf0>0, that is h<14 and 0<a<1−2√hh, f0(x) has two positive roots in the interval (0,1), which implies that system (1.5) has two boundary equilibria E10(x10,0) and E20(x20,0).
If ah<1 and Δf0=0, that is h<14 and a=1−2√hh, f0(x) has a unique positive root in the interval (0,1), which implies that system (1.5) has a unique boundary equilibrium ˉE∗(ˉx∗,0).
The Jacobian matrix of system (1.5) at E0(x,0) is
JE0=(1−2x−ax2(1+ax)2−bx0s). |
We can easily verify that
(1−2x−ax2)|x=x10>0, (1−2x−ax2)|x=x20<0, (1−2x−ax2)|x=ˉx∗=0. |
Hence, E10 is an unstable node and E20 is a saddle.
When a=1−2√hh, make the following transformations successively
x=x1+1−ah2, y=y1;x1=x2−b√hy2, y1=sy2, |
then system (1.5) becomes
˙x2=√hs(√h−1)x22−b(1+2hs(√h−1))x2y2+b(b√h−s+bh√h(√h−1)s)y22+o(|x2,y2|2),˙y2=y2−s√hy22+o(|x2,y2|2). | (2.1) |
By Theorem 7.1 in Chapter 2 of Zhang et al. [22], ˉE∗ is a saddle node, which includes an unstable parabolic sector in the left if h<14 and a=1−2√hh. The proof is completed.
Choose (h,b,s)=(0.08,1.2,0.1), and we present the relationship between the boundary equilibria of system (1.5) and a, as well as the phase portrait when a=0.1, shown in Figure 1. Figure 1(a) shows that when 0<a<5.428932188, system (1.5) has two boundary equilibria; when a≐5.428932188, system (1.5) has a unique boundary equilibrium; and when a>5.428932188, system (1.5) has no boundary equilibrium. Figure 1(b) shows that system (1.5) admits two boundary equilibria, where E10 is an unstable node and E20 is a saddle. Further, when the initial values lie inside the region to the right of the stable manifold of the saddle (the positive equilibrium), both populations will coexist in fixed sizes; otherwise, both populations will not coexist.
Note that any positive equilibrium of system (1.5) satisfies
x(1−x)1+ax−bxy−h=0,1−yx=0. | (2.2) |
From Eq (2.2), we denote
f(x)=abx3+(b+1)x2+(ah−1)x+h, |
then
f′(x)=3abx2+2(b+1)x+ah−1, | (2.3) |
whose discriminant
Δ=4(b+1)2−12ab(ah−1)>0, when ah<1. |
Obviously, in the interval (0,1), f′(x)=0 has a unique positive root
x∗=−2(b+1)+√Δ6ab. |
For any positive equilibrium E(x,y), from f(x)=0, we obtain
h=−x(abx2+bx+x−1)ax+1. |
Substituting this into Det(JE) and f′(x), there is
Det(JE)=s1+axf′(x). | (2.4) |
Theorem 2.2. For the positive equilibria of system (1.5), the following conclusions hold:
(1) If ah<1 and f(x∗)<0, then system (1.5) has two positive equilibria: a hyperbolic saddle E11(x11,x11), and an elementary and antisaddle equilibrium E12(x12,x12), with x11<x∗<x12;
(2) if ah<1 and f(x∗)=0, then system (1.5) has a unique positive degenerate equilibrium E∗(x∗,x∗);
(3) if ah≥1 or f(x∗)>0, then system (1.5) has no positive equilibrium.
Proof. From (2.3) and (2.4), it is obvious that Det(JE11)<0, Det(JE12)>0 and Det(JE∗)=0. Thus E11 is a hyperbolic saddle, E12 is an elementary and antisaddle equilibrium, and E∗ is a degenerate equilibrium. The proof is completed.
Next, we consider case (2) of Theorem 2.2. From f(x∗)=f′(x∗)=0, we can express
b=b∗=1−ax2∗−2x∗2x∗(ax∗+1)2, h=h∗=(1−ax2∗+2ax∗)x∗2(ax∗+1)2, |
furthermore, we let
s∗=1−ax2∗−2x∗2(ax∗+1)2. |
Theorem 2.3. Assume that b=b∗, h=h∗ and a<1−2x∗x2∗ with x∗<12, then system (1.5) admits a degenerate positive equilibrium E∗(x∗,x∗). Moreover,
(1) If s>s∗(or 0<s<s∗), then E∗ is a saddle node, which includes a stable (or an unstable) parabolic sector in the left;
(2) If s=s∗, then E∗ is a cusp of codimension 2.
Proof. (1) When s≠s∗, make the following transformation
x=x1+x∗, y=y1+x∗, |
then system (1.5) can be written as
˙x1=s∗x1−s∗y1−a+1(1+ax∗)3x21−bx1y1+o(|x1,y1|2),˙y1=sx1−sy1−sx∗x21+2sx∗x1y1−sx∗y21+o(|x1,y1|2). | (2.5) |
In order to translate the linear part of this system to Jordan form, we make the following transformation:
x1=−s∗(x2−y2), y1=−s∗x2+sy2, dτ=(s∗−s)dt. |
Then system (2.5) becomes
˙x2=a20x22+a11x2y2+a02y22+o(|x2,y2|2),˙y2=y2+b20x22+b11x2y1+b02y22+o(|x2,y2|2), |
where
a20=−sA1A2A3, a11=−sA1(2(1+ax∗)3s+2(a+1)x∗−A2)A3,a02=s(4s2(1+ax∗)5+6s(1+ax∗)3A1+A1A2)A3, b20=−A1a202s(1+ax∗)2,b11=−A1a112s(1+ax∗)2, b02=4s3(1+ax∗)7+4s2(1+ax∗)5A1−(a+1)x∗A21(1+ax∗)2A3, |
with
A1=ax2∗+2x∗−1, A2=a2x3∗+3ax2∗−3ax∗−1,A3=x∗(1+ax∗)(2a2sx2∗+ax2∗+4asx∗+2x∗+2s−1)2. | (2.6) |
Note that when a<1−2x∗x2∗ with x∗<12, A1<0, A2<0 and A3>0, thus a20<0. By Theorem 7.1 in Chapter 2 of Zhang et al. [22], E∗ is a saddle node, which includes a stable (or an unstable) parabolic sector in the left if s>s∗( or 0<s<s∗).
(2) When s=s∗, by the following transformations successively
x=x1+x∗, y=y1+x∗;x1=−s∗x2, y1=−s∗x2+y2, |
system (1.5) can be rewritten as
˙x2=y2+A1A24x∗(1+ax∗)5x22+A12x∗(1+ax∗)2x2y2+o(|x2,y2|2),˙y2=−A21A28x∗(1+ax∗)7x22−A214x∗(1+ax∗)4x2y2+A12x∗(1+ax∗)2y22+o(|x2,y2|2). | (2.7) |
By Lemma 3.1 of [18], system (2.7) near the origin is equivalent to
˙X=Y+o(|X,Y|3),˙Y=DX2+ˇEXY+o(|X,Y|3), |
where
D=−A21A28x∗(1+ax∗)7, ˇE=A1(a2x3∗+3ax2∗−5ax∗−2x∗−1)4x∗(1+ax∗)5. |
It is obvious that when a<1−2x∗x2∗ with x∗<12, we have D>0 and ˇE<0, thus E∗ is a cusp of codimension 2. The proof is completed.
In this section, we investigate the Bogdanov-Takens bifurcation of codimension 2 and the degenerate Hopf bifurcation of codimension 3.
According to Theorem 2.3 (2), system (1.5) may have a Bogdanov-Takens bifurcation of codimension 2 around E∗. Now we choose a and h as bifurcation parameters and obtain the following unfolding system
˙x=x(1−x)1+(a+λ1)x−b∗xy−(h∗+λ2),˙y=s∗y(1−yx), | (3.1) |
where λ=(λ1,λ2) is a parameter vector in a small neighborhood of (0,0).
Theorem 3.1. If the conditions of Theorem 2.3(2) hold, then system (1.5) undergoes a Bogdanov–Takens bifurcation of codimension 2 around E∗.
Proof. In order to move E∗ to the origin, we make a transformation
x=x1+x∗, y=y1+x∗, |
then system (3.1) becomes
˙x1=c00+c10x1−s∗y1+c20x21−b∗x1y1+P1(x1,y1,λ),˙y1=s∗x1−s∗y1−b∗x21+2b∗x1y1−b∗y21+P2(x1,y1,λ), | (3.2) |
where P1(x1,y1,λ) and P2(x1,y1,λ) are C∞ functions of at least third order with respect to (x1,y1), and
c00=(x∗−1)x2∗λ1(1+ax∗)(ax∗+x∗λ1+1)−λ2,c10=−(ax2∗+2x∗−1)(ax∗−x∗λ1+1)2(ax∗+x∗λ1+1)(1+ax∗)2+x∗(x∗−1)λ1(ax∗+x∗λ1+1)2(1+ax∗),c20=−a+λ1+1(ax∗+x∗λ1+1)3. |
By the following transformation
x2=x1,y2=c00+c10x1−s∗y1+c20x21−b∗x1y1+P1(x1,y1,λ), |
system (3.2) can be rewritten as
˙x2=y2,˙y2=d00+d10x2+d01y2+d20x22+d11x2y2+2b∗s∗y22+P3(x2,y2,λ), | (3.3) |
where P3(x2,y2,λ) is a C∞ function of at least third order with respect to (x2,y2), and
d00=c00(c00b∗+s2∗)s∗,d10=−c200b2∗−2c00s∗(c10−s∗)b∗−s3∗(c10−s∗)s2∗,d01=−3c00b∗−c10s∗+s2∗s∗,d20=−c200b3∗−2c00c10b2∗s∗+2c00c20b∗s2∗+c210b∗s2∗−2c10b∗s3∗+c20s4∗s3∗,d11=−3c00b2∗−3c10b∗s∗+2c20s2∗+2b∗s2∗s2∗. |
Make a transformation
x3=x2, y3=(1−2b∗s∗x2)y2, dt=(1−2b∗s∗x2)dτ, |
still denoting τ as t, then system (3.3) becomes
˙x3=y3,˙y3=d1+d2x3+d3y3+d4x23+d5x3y3+P4(x3,y3,λ), | (3.4) |
where P4(x3,y3,λ) is a C∞ function of at least third order with respect to (x3,y3), and
d1=d00, d2=d10−4b∗s∗d00, d3=d01,d4=4b2∗d00−4b∗d10s∗+d20s2∗s2∗, d5=d11s∗−2b∗d01s∗. |
Note that when λ1 and λ2 are small enough,
d4=−A1A24x∗(1+ax∗)5<0, |
where A1 and A2 are given in (2.6).
Let
x4=x3, y4=y3√−d4, dτ=√−d4dt, |
still denoting τ as t, then we can rewrite system (3.4) as
˙x4=y4,˙y4=−d1d4−d2d4x4+d3√−d4y4−x24+d5√−d4x4y4+P5(x4,y4,λ), |
where P5(x4,y4,λ) is a C∞ function of at least third order with respect to (x4,y4).
It is obvious that when λ1 and λ2 are small enough,
d5=a2x3∗+3ax2∗−5ax∗−2x∗−12x∗(1+ax∗)3<0. |
Making the following transformations successively
x5=x4+d22d4, y5=y4;x6=d25d4x5, y6=d35(−d4)3/2y5, dτ=−√−d4d5dt, |
we obtain the following versal unfolding of system (3.1)
˙x6=y6,˙y6=μ1+μ2y6+x26+x6y6+P6(x6,y6,λ), |
where P6(x6,y6,λ) is a C∞ function of at least third order with respect to (x6,y6), and
μ1=(4d1d4−d22)d454d44, μ2=(2d3d4−d2d5)d52d24. |
By Maple software, we can obtain
|∂(μ1,μ2)∂(λ1,λ2)|λ=0=−2(a2x3∗+3ax2∗−5ax∗−2x∗−1)5(ax2∗+3x∗−2)(1+ax∗)(ax2∗+2x∗−1)2(a2x3∗+3ax2∗−3ax∗−1)5>0. |
By the results of [23], system (3.1) is the versal unfolding of Bogdanov–Takens sigularity (cusp case) of codimension 2, when λ=(λ1,λ2) is a parameter vector in a small neighborhood of (0,0). Hence, system (1.5) undergoes a Bogdanov-Takens bifurcation of codimension 2. The proof is completed.
The proof of Theorem 2.2 shows that Det(JE12)>0, that is, system (1.5) may undergo a Hopf bifurcation around E12 when Tr(JE12)=0. For simplicity, we denote x12 by z. From f(z)=0 and Tr(JEz)=0, we obtain
b=1−2z−az2−s(1+az)2z(1+az)2, h=z(s(1+az)2+z(a+1))(1+az)2. | (3.5) |
By b>0 and Det(JEz)>0, we denote
M:={(a,s,z)∈R3+|0<s<1−2z−az2(1+az)2, 0<a<1−2zz2, 0<z<12}. | (3.6) |
To obtain the focal values around E12(z,z+k), we make the following transformations successively
(i)dt=x(1+ax)dτ0;(ii)x=x1+z, y=y1+z;(iii)x1=x2+√Dzs(1+az)y2, y1=x2, dτ=√Ddτ0, |
where
D=−sz2(2a2sz2+4asz+az2+2s+2z−1)>0, for (a,s,z)∈M. |
Still denoting τ by t, system (1.5) can be rewritten as
˙x2=y2+ˉa11x2y2+ˉa02y22+ˉa21x22y2+ˉa12x2y22,˙y2=−x2+ˉb20x22+ˉb11x2y2+ˉb02y22+ˉb30x32+ˉb21x22y2+ˉb12x2y22+ˉb03y32+ˉb40x42+ˉb31x32y2+ˉb22x22y22+ˉb13x2y32, |
where
ˉa11=2az+1z(az+1), ˉa02=a√Dzs(az+1)2, ˉa21=az(az+1), ˉa12=a√Dz2s(az+1)2,ˉb20=szB1(1+az)D, ˉb11=B1−z(a+1)(1+az)2D, ˉb02=B1−B2−B3−z(a+1)sz(az+1)3,ˉb30=sB2(az+1)D, ˉb21=2B2−z(a+1)z(az+1)2√D, ˉb12=B1−2B3+az2−2az−1sz2(az+1)3,ˉb03=(az2+2z−1−2B3)(aB3−a2z2−2az−1)(az+1)4s√D, ˉb40=asB3(az+1)D,ˉb31=3aB3z(az+1)2√D, ˉb22=3aB3(az+1)3sz2, ˉb13=aB3√Dz3s2(az+1)4,B1=5a3sz3+13a2sz2+3a2z3+11asz+8az2−4az+3s+3z−2,B2=4a3sz3+9a2sz2+3a2z3+6asz+7az2−4az+s+z−1,B3=a2sz2+2asz+az2+s+2z−1. |
The first three Lyapunov coefficients [22] at E12, respectively, are
L1=−zB3f14(az+1)5D3/2,L2=−zB3f224s(az+1)11D5/2,L3=z3B3f31152s(az+1)17D9/2, |
where
f1=(az+1)6s2−z(a+1)(az−5)(az+1)2s−z(a+1)(2a2z3+3az2−4az−4z+1), |
and f2, f3 are polynomials with respect to a,s and z, whose expressions are omitted.
For the polynomials f,g and hi(i=1,2,⋯,n), let V(h1,h2,⋯,hn) be the set of common zeros of hi(i=1,2,⋯,n), res(f,g,x) be the Sylvester resultant of f and g with respect to x, and lcoeff(f,x) be the leading coefficient of f with respect to x. By Maple software, we can obtain
r12=res(f1,f2,s)=z3(a+1)3(az+1)22P1P2P3g1,r13=res(fl,f3,s)=−4z4(a+1)4(az+1)39P1P2P3g2,r23=res(f2,f3,s)=−3z12(a+1)9(az2+2z−1)3(az+1)92P1P2P3g3,ˉr12=res(g1,g2,a)=c1z391(2z−5)(25z2+20z−94)(z−1)107P4h1h2,ˉr13=res(g1,g3,a)=c2z995(2z−5)(z−1)267P4h1h3, |
where
P1=a2z3+3az2−3az−1, P2=2a2z3+3az2−4az−4z+1,P3=a2z3+3az2−5az−2z−1, P4=113z3−153z2+46z−22,c1=3259867590634045802246936985600,c2=81561880369203584840887625576610808536760320000000, |
gi (1=1,2,3) are polynomials with respect to a and z, hi (1=1,2,3) are polynomials with respect to z, and their expressions are omitted.
Let
l1=lcoeff(f1,s)=(az+1)6>0,l2=lcoeff(f2,s)=3(3az+5)(4az+3)(az+1)12>0,l3=lcoeff(g1,a)=8z16>0. |
Theorem 3.2. Assume that (3.5) and (3.6) hold, then Ez is a weak focus of order at most 3.
Proof. Note that B3<0 in M, thus
V(L1,L2,L3)∩M=V(f1,f2,f3)∩M. |
We can easily verify that P1<0, P3<0, P4<0 and 25z2+20z−94<0 in M. Then by Lemma 2 in Chen and Zhang [24], we obtain the following decomposition
V(f1,f2,f3)∩M=(V1∪V2∪V3)∩M, |
where
V1=V(f1,f2,f3,P2), V2=V(f1,f2,f3,g1,g2,g3,h1), V3=V(f1,f2,f3,g1,g2,g3,h2,h3). |
Next, we prove V(L1,L2,L3)∩M=∅ by three steps.
Step 1. Proving that V1∩M=∅. We can easily verify that P2<0 when 14≤z<12, and when 0<z<14, in M, P2=0 has a unique positive root
a1=4−3z−√41z2−32z+164z2. |
Note that 5−a1z>0 when 0<z<14, which leads to
f1=(az+1)6s2−z(a+1)(az−5)(az+1)2s−z(a+1)P2>0, for a=a1. |
Thus, V1∩M=∅.
Step 2. Proving that V2∩M=∅. Note that
V2=V(f1,g1,h1)∩V(f2,f3,g2,g3). |
By the command "realroot(h1,10−30)", h1(z) exists only one positive real root isolation interval [z_,¯z]⊂(0,12), where
z_=536102070554666748154056775040140564819207303340847894502572032, |
¯z=268051035277333374077028387520120282409603651670423947251286016. |
For the real root interval [z_,¯z] of h1(z), using the real root isolation algorithm of multivariate polynomial systems [25], we can obtain a unique positive real root isolation interval [a_,¯a] in M, where
a_=1201057613194270064910343638323310141204801825835211973625643008,¯a=60052880659713503245517181916195070602400912917605986812821504. |
Note that
az−5≤ˉaˉz−5<0,2a2z3+3az2−4az−4z+1≤2ˉa2ˉz3+3ˉaˉz2−4a_z_−4z_+1<0. |
This means f1(s,a,z)>0 in the real root isolation interval [z_,¯z]×[a_,¯a] of {h1,g1}. Thus V2⊆V(f1,g1,h1)=∅, which implies V2∩M=∅.
Step 3. Proving that V3∩M=∅. By Maple software, there is
res(h2,h3,z)<0, |
which implies V(h2,h3)=∅. Therefore, V3∩M=∅.
To sum up, we show that V(f1,f2,f3)∩M=∅. Thus Ez is a weak focus of order at most 3. The proof is completed.
Define
M1:={(a,s,z)∈M:f1(a,s,z)≠0},M2:={(a,s,z)∈M:f1(a,s,z)=0, f2(a,s,z)≠0},M3:={(a,s,z)∈M:f1(a,s,z)=0, f2(a,s,z)=0}. |
From Theorem 3.2, we have the following theorem.
Theorem 3.3. Assume that (3.5) and (3.6) hold, then Ez is a weak focus of order up to 3. Moreover,
(1) if (a,s,z)∈M1, then Ez is a weak focus of order 1;
(2) if (a,s,z)∈M2, then Ez is a weak focus of order 2;
(3) if (a,s,z)∈M3, then Ez is a weak focus of order 3.
To verify M3≠∅, we let z=120. Again from f(z)=0 and Tr(JEz)=0, we have
b=ˉb:=−20(a2s+40as+a+400s−360)(a+20)2, h=ˉh:=a2s+40as+20a+400s+2020(a+20)2. | (3.7) |
By b>0, h>0 and Det(JEz)>0, we define
M∗:={(a,s)∈R2+|0<s<360−a(a+20)2, 0<a<360}. | (3.8) |
We can obtain the following theorem.
Theorem 3.4. When z=120, assume that (3.7) and (3.8) hold, and there exist a0∈[a_0,¯a0] and s0∈[s_,¯s] given respectively in (3.9) and (3.10), then Ez is a weak focus of order 3 and system (1.5) can undergo a degenerate Hopf bifurcation of codimension 3 near Ez.
Proof. When z=120, by the command "realroot(g1,10−30)", g1(a,12) exists a positive real root isolation interval [a_0,¯a0] in M∗, where
a_0=587483542755771983299666810929340564819207303340847894502572032,¯a0=293741771377885991649833405464720282409603651670423947251286016. | (3.9) |
For the real root interval [a_0,¯a0] of g1(a,12), using the real root isolation algorithm of multivariate polynomial systems [25], we can obtain a unique positive real root isolation interval [s_,¯s] in M∗, where
s_=1636665719497057001254010951755406743278485434514351496577676626844588240573268701473812127674924007424,¯s=6546662877988228005016043807021803030843922323864795986310706507378352962293074805895248510699696029696. | (3.10) |
Hence, under the conditions (3.7) and (3.8), when z=120, there exist a0∈[a_0,¯a0] and s0∈[s_,¯s] such that f1(a0,s0,120)=f2(a0,s0,120)=0. Therefore, according to Theorem 3.2, Ez is a weak focus of order exactly 3.
Finally,
|∂(Tr(JEz),L1,L2)∂(a,s,z)|z=120=−100000(a2s+40as+a+400s−360)J(a,s)3(a+20)19s6(2a2s+80as+a+800s−360)5, |
where the expression of J(a,s) is too long and is omitted here.
For the polynomial f(x,y), let f+(x,y) and f−(x,y) be the summations of the positive and negative terms in f(x,y), respectively ([25]).
According to Theorem 2.2 in [25], we have
J(a,s)<J+(¯a0,¯s)+J−(a_0,s_)≈−1.739971526×1032<0. |
Note that a2s+40as+a+400s−360≠0, thus |∂(Tr(JEz),L1,L2)∂(a,s,z)|≠0, for (a,s,z)=(a0,s0,120). Therefore, system (1.5) can undergo a degenerate Hopf bifurcation of codimension 3 near Ez. The proof is completed.
In this section, we give some numerical simulations by Matcont to support the bifurcation phenomena of system (1.5).
Choose a=0.142, b=14.2, and s=0.1, and present the bifurcation diagram in the (h,x)-plane by Matcont. Figure 2 shows that when 0<h<0.0088222644, the positive equilibrium E12 is stable and system (1.5) has no limit cycle; when 0.0088222644<h<0.0088313334, system (1.5) admits an unstable limit cycle around E12; when 0.0088313334<h<0.0088955378, system (1.5) has two limit cycles, where the inner one is stable and the outer one is unstable; and when h>0.0088955378, the positive equilibrium E12 is unstable and system (1.5) has no limit cycle. It is shown that when the constant-yield prey harvesting h is relatively small, the constant-yield prey harvesting does not change the dynamic behaviors of the system, that is, both populations will coexist in fixed sizes E12; as h increases, system (1.5) first generates an unstable limit cycle and then two limit cycles (the inner one is stable); but when h is relatively large, the limit cycles will disappear first and then the positive equilibria, and finally there will be no positive equilibrium point.
In Figure 3, we present the corresponding phase portraits of system (1.5) with a=0.142, b=14.2, and s=0.1, as h varies. When h=0.008, from Figure 3(a), the positive equilibrium E12 is stable, that is, when the initial values (i.e. initial densities of both populations) lie inside the region to the right of the stable manifold of the saddle E11, both populations will coexist in fixed sizes E12; when h=0.00883, from Figure 3(b), system (1.5) admits a unstable limit cycle, that is, when the initial values lie inside the unstable limit cycle, both populations will coexist in fixed sizes E12; when h=0.00888, from Figure 3(c), system (1.5) admits two limit cycles (the inner one is stable), that is, when the initial values lie inside the outer limit cycle, both populations will coexist in the stable limit cycle; otherwise, both populations will not coexist in the above three figures. When h=0.0098, from Figure 3(d), all the nonnegative equilibria are unstable, that is, both populations will not coexist. Hence the relatively small harvesting can make both populations coexist in fixed sizes or in a periodic orbit; that is, the small harvesting can affect the populations of both species to some extent; however, the large harvesting is detrimental to the dynamic behaviors of the system.
In this paper, we discussed the stability and bifurcation of a Leslie–Gower predator–prey model with Smith growth and constant-yield harvesting. We proved that system (1.5) admits at most two boundary equilibria, both of which are unstable. For system (1.5) without Smith growth, that is system (1.3), Zhu and Lan [21] investigated the stability of equilibria. Also, system (1.3) undergoes the saddle-node bifurcation and supercritical and subcritical Hopf bifurcations. Compared with the results in [21], we presented some different dynamics of the system, and found that both the Smith growth and constant-yield harvesting play important roles in the dynamics of system (1.5), more precisely, we proved that the degenerate positive equilibrium of system (1.5) is a cusp of codimension 2 and system (1.5) undergoes cusp-type Bogdanov–Takens bifurcation of codimension 2. Further, using the resultant elimination method and the real root isolation algorithm of multivariate polynomial systems [25], we proved that system (1.5) has a weak focus of order at most 3 and undergoes a degenerate Hopf bifurcation of codimension 3. By numerical simulation, we illustrated that system (1.5) has two limit cycles (the inner one is stable). Also, we showed that the small harvesting is conducive to the survival of prey and predators, but the large harvesting is detrimental to the coexistence of the populations. Therefore, our results revealed that Smith growth and constant-yield harvesting can lead to richer dynamic behaviors.
Motivated by Lampart and Zapomˇel [26], whether there are some new numerical methods to derive some new dynamical behaviors. Also, we can regard the presence of more complex phenomena such as intermittency (see [27]) of the system in the future.
The authors declare they have not used Artificial Intelligence (AI) tools in the creation of this article.
This work was supported by the Natural Science Foundation of Fujian Province (2021J01613, 2021J011032, 2023J05250) and the Scientific Research Foundation of Minjiang University (MJY22027).
The authors declare there is no conflict of interest.
[1] |
P. H. Leslie, Some further notes on the use of matrices in population mathematics, Biometrika, 35 (1948), 213–245. https://doi.org/10.2307/2332342 doi: 10.2307/2332342
![]() |
[2] |
P. H. Leslie, A stochastic model for studying the properties of certain biological systems by numerical methods, Biometrika, 45 (1958), 16–31. https://doi.org/10.2307/2333042 doi: 10.2307/2333042
![]() |
[3] |
A. Korobeinikov, A Lyapunov function for Leslie–Gower predator–prey models, Appl. Math. Lett., 14 (2001), 697–699. https://doi.org/10.1016/s0893-9659(01)80029-x doi: 10.1016/s0893-9659(01)80029-x
![]() |
[4] |
S. B. Hsu, T. W. Huang, Global stability for a class of predator–prey system, SIAM J. Appl. Math., 55 (1995), 763–783. https://doi.org/10.1137/s0036139993253201 doi: 10.1137/s0036139993253201
![]() |
[5] |
J. C. Huang, S. G. Ruan, J. Song, Bifurcations in a predator–prey system of Leslie type with generalized Holling type Ⅲ functional response, J. Differ. Equations, 257 (2014), 1721–1752. https://doi.org/10.1016/j.jde.2014.04.024 doi: 10.1016/j.jde.2014.04.024
![]() |
[6] |
Y. F. Dai, Y. L. Zhao, B. Sang, Four limit cycles in a predator–prey system of Leslie type with generalized Holling type Ⅲ functional response, Nonlinear Anal. Real World Appl., 50 (2019), 218–239. https://doi.org/10.1016/j.nonrwa.2019.04.003 doi: 10.1016/j.nonrwa.2019.04.003
![]() |
[7] |
M. X. He, Z. Li, Global dynamics of a Leslie-Gower predator-prey model with square root response function, Appl. Math. Lett., 140 (2023), 108561. https://doi.org/10.1016/j.aml.2022.108561 doi: 10.1016/j.aml.2022.108561
![]() |
[8] |
Y. F. Wu, X. H. Ai, Analysis of a stochastic Leslie-Gower predator–prey system with Beddington-DeAngelis and Ornstein-Uhlenbeck process, Electron. Res. Arch., 32 (2024), 370-385. https://doi.org/10.3934/era.2024018 doi: 10.3934/era.2024018
![]() |
[9] |
J. Xu, Y. Tian, H. J. Guo, X. Y. Song, Dynamical analysis of a pest management Leslie-Gower model with ratio-dependent functional response, Nonlinear Dyn., 93 (2018), 507-720. https://doi.10.1007/s11071-018-4219-9 doi: 10.1007/s11071-018-4219-9
![]() |
[10] |
Y. Tian, X. R. Yan, K. B. Sun, Dual effects of additional food supply and threshold control on the dynamics of a Leslie-Gower model with pest herd behavior, Chaos Solitons Fractals, 185 (2024), 115163. https://doi.org/10.1016/j.chaos.2024.115163 doi: 10.1016/j.chaos.2024.115163
![]() |
[11] |
F. E. Smith, Population dynamics in Daphnia magna and a new model for population growth, Ecology, 44 (1963), 651–663. https://doi.org/10.2307/1933011 doi: 10.2307/1933011
![]() |
[12] |
V. Kumar, Pattern formation and delay-induced instability in a Leslie-Gower type prey–predator system with Smith growth function, Math. Comput. Simulat., 225 (2024), 78–97. https://doi.org/10.1016/j.matcom.2024.05.004 doi: 10.1016/j.matcom.2024.05.004
![]() |
[13] |
Z. Li, M. X. He, Hopf bifurcation in a delayed food-limited model with feedback control, Nonlinear Dyn., 76 (2014), 1215–1224. https://doi.org/10.1007/s11071-013-1205-0 doi: 10.1007/s11071-013-1205-0
![]() |
[14] |
X. Z. Feng, X. Liu, C. Sun, Y. L. Jiang, Stability and Hopf bifurcation of a modified Leslie-Gower predator–prey model with Smith growth rate and B-D functional response, Chaos Solitons Fractals, 174 (2023), 113794. https://doi.org/10.1016/j.chaos.2023.113794 doi: 10.1016/j.chaos.2023.113794
![]() |
[15] |
D. Bai, J. Zheng, Y. Kang, Global dynamics of a predator-prey model with a Smith growth function and the additive predation in prey, Discrete Continuous Dyn. Syst. Ser. B, 29 (2024), 1923–1960. https://doi.org/10.3934/dcdsb.2023161 doi: 10.3934/dcdsb.2023161
![]() |
[16] |
X. L. Han, C. Y. Lei, Bifurcation and turing instability analysis for a space- and time-discrete predator-prey system with Smith growth function, Chaos Solitons Fractals, 166 (2023), 112910. https://doi.org/10.1016/j.chaos.2022.112910 doi: 10.1016/j.chaos.2022.112910
![]() |
[17] |
H. Guo, Y. Tian, K. B. Sun, X. Y. Song, Dynamic analysis of two fishery capture models with a variable search rate and fuzzy biological parameters, Math. Biosci. Eng., 20 (2023), 21049–21074. https://doi.org/10.3934/mbe.2023931 doi: 10.3934/mbe.2023931
![]() |
[18] |
J. C. Huang, Y. J. Gong, S. G. Ruan, Bifurcations analysis in a predator-prey model with constant-yield predator harvesting, Discrete Continuous Dyn. Syst. Ser. B, 18 (2013), 2101–2121. https://doi.org/10.3934/dcdsb.2013.18.2101 doi: 10.3934/dcdsb.2013.18.2101
![]() |
[19] |
Y. C. Xu, Y. Yang, F. W. Meng, S. G. Ruan, Degenerate codimension-2 cusp of limit cycles in a Holling-Tanner model with harvesting and anti-predator behavior, Nonlinear Anal. Real World Appl., 76 (2024), 103995. https://doi.org/10.1016/j.nonrwa.2023.103995 doi: 10.1016/j.nonrwa.2023.103995
![]() |
[20] |
H. Wu, Z. Li, M. X. He, Bifurcation analysis of a Holling-Tanner model with generalist predator and constant-yield harvesting, Int. J. Bifurcation Chaos, 34 (2024), 2450076. https://doi.org/10.1142/s0218127424500767 doi: 10.1142/s0218127424500767
![]() |
[21] |
C. R. Zhu, K. Q. Lan, Phase portraits, Hopf bifurcations and limit cycles of Leslie–Gower predator-prey systems with harvesting rates, Discrete Continuous Dyn. Syst. Ser. B, 14 (2010), 289–306. https://doi.org/10.3934/dcdsb.2010.14.289 doi: 10.3934/dcdsb.2010.14.289
![]() |
[22] | Z. F. Zhang, T. R. Ding, W. Z. Huang, Z. X. Dong, Qualitative Theory of Differential Equations, Translations of Mathematical Monographs, American Mathematical Society, 1992. https://doi.org/10.1090/mmono/101 |
[23] | L. Perko, Differential Equations and Dynamical Systems, Springer, New York, 1996. https://doi.org/10.1007/978-1-4684-0392-3 |
[24] |
X. W. Chen, W. N. Zhang, Decomposition of algebraic sets and applications to weak centers of cubic systems, J. Comput. Appl. Math., 23 (2009), 565–581. https://doi.org/10.1016/j.cam.2009.06.029 doi: 10.1016/j.cam.2009.06.029
![]() |
[25] |
Z. Lu, B. He, Y. Lou, L. Pan, An algorithm of real root isolation for polynomial systems with application to the construction of limit cycles, Symb. Numer. Comput., 232 (2007), 131–147. https://doi.org/10.1007/978-3-7643-7984-1_9 doi: 10.1007/978-3-7643-7984-1_9
![]() |
[26] |
M. Lampart, J. Zapomˇel, The disturbance influence on vibration of a belt device driven by a crank mechanism, Chaos Solitons Fractals, 173 (2023), 113634. https://doi.org/10.1016/j.chaos.2023.113634 doi: 10.1016/j.chaos.2023.113634
![]() |
[27] |
A. Lampartovˊa, M. Lampart, Exploring diverse trajectory patterns in nonlinear dynamic systems, Chaos Solitons Fractals, 182 (2024), 114863. https://doi.org/10.1016/j.chaos.2024.114863 doi: 10.1016/j.chaos.2024.114863
![]() |