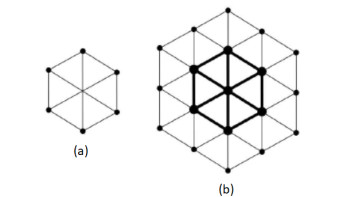
Coronavirus spread in Wuhan, China, in December 2019. A few weeks later, the virus was present in over 100 countries around the globe. Governments have adopted extreme measures to contain the spreading virus. Quarantine is considered the most effective way to control the spreading speed of COVID-19. In this study, a mathematical model is developed to explore the influence of quarantine and the latent period on the spatial spread of COVID-19. We use the mathematical model with quarantine, and delay to predict the spreading speed of the virus. In particular, we transform the model to a single integral equation and then apply the Laplace transform to find implicit equations for the spreading speeds. The basic reproduction number of COVID-19 is also found and calculated. Numerical simulations are performed to confirm our theoretical results. To validate the proposed model, we compare our outcomes with the actual reported data published by the National Health Commission of China and the Health Commission of local governments. The model demonstrates good qualitative agreement with the actual data reported. The results show that delay and quarantine highly influence the spreading speeds of COVID-19. Also, we can only contain the disease if we quarantine 75% of the infected people.
Citation: Khalaf M. Alanazi. The asymptotic spreading speeds of COVID-19 with the effect of delay and quarantine[J]. AIMS Mathematics, 2024, 9(7): 19397-19413. doi: 10.3934/math.2024945
[1] | Usman Babar, Haidar Ali, Shahid Hussain Arshad, Umber Sheikh . Multiplicative topological properties of graphs derived from honeycomb structure. AIMS Mathematics, 2020, 5(2): 1562-1587. doi: 10.3934/math.2020107 |
[2] | Ali Al Khabyah . Mathematical aspects and topological properties of two chemical networks. AIMS Mathematics, 2023, 8(2): 4666-4681. doi: 10.3934/math.2023230 |
[3] | R. Aguilar-Sánchez, J. A. Mendez-Bermudez, José M. Rodríguez, José M. Sigarreta . Multiplicative topological indices: Analytical properties and application to random networks. AIMS Mathematics, 2024, 9(2): 3646-3670. doi: 10.3934/math.2024179 |
[4] | Fei Yu, Hifza Iqbal, Saira Munir, Jia Bao Liu . M-polynomial and topological indices of some transformed networks. AIMS Mathematics, 2021, 6(12): 13887-13906. doi: 10.3934/math.2021804 |
[5] | Ali Al Khabyah, Haseeb Ahmad, Ali Ahmad, Ali N. A. Koam . A uniform interval-valued intuitionistic fuzzy environment: topological descriptors and their application in neural networks. AIMS Mathematics, 2024, 9(10): 28792-28812. doi: 10.3934/math.20241397 |
[6] | Sumiya Nasir, Nadeem ul Hassan Awan, Fozia Bashir Farooq, Saima Parveen . Topological indices of novel drugs used in blood cancer treatment and its QSPR modeling. AIMS Mathematics, 2022, 7(7): 11829-11850. doi: 10.3934/math.2022660 |
[7] | Fozia Bashir Farooq . Implementation of multi-criteria decision making for the ranking of drugs used to treat bone-cancer. AIMS Mathematics, 2024, 9(6): 15119-15131. doi: 10.3934/math.2024733 |
[8] | Jung-Chao Ban, Chih-Hung Chang . Entropy dimension of shifts of finite type on free groups. AIMS Mathematics, 2020, 5(5): 5121-5139. doi: 10.3934/math.2020329 |
[9] | Ali N. A. Koam, Ali Ahmad, Azeem Haider, Moin A. Ansari . Computation of eccentric topological indices of zero-divisor graphs based on their edges. AIMS Mathematics, 2022, 7(7): 11509-11518. doi: 10.3934/math.2022641 |
[10] | Yanjie Wang, Beibei Zhang, Bo Cao . On the number of zeros of Abelian integrals for a kind of quadratic reversible centers. AIMS Mathematics, 2023, 8(10): 23756-23770. doi: 10.3934/math.20231209 |
Coronavirus spread in Wuhan, China, in December 2019. A few weeks later, the virus was present in over 100 countries around the globe. Governments have adopted extreme measures to contain the spreading virus. Quarantine is considered the most effective way to control the spreading speed of COVID-19. In this study, a mathematical model is developed to explore the influence of quarantine and the latent period on the spatial spread of COVID-19. We use the mathematical model with quarantine, and delay to predict the spreading speed of the virus. In particular, we transform the model to a single integral equation and then apply the Laplace transform to find implicit equations for the spreading speeds. The basic reproduction number of COVID-19 is also found and calculated. Numerical simulations are performed to confirm our theoretical results. To validate the proposed model, we compare our outcomes with the actual reported data published by the National Health Commission of China and the Health Commission of local governments. The model demonstrates good qualitative agreement with the actual data reported. The results show that delay and quarantine highly influence the spreading speeds of COVID-19. Also, we can only contain the disease if we quarantine 75% of the infected people.
Graph theory has provided the researcher with various useful tools, such as graph labeling, locating numbers and topological indices. Graph theory subject has many applications and implementations in different research subjects like chemistry, medicine and engineering. A graph can be recognized by a numeric value, a polynomial, a sequence of numbers or a matrix. The representation of the chemical compound in terms of diagram, known as its molecular graph, in which its atoms and the chemical bonding between them represent the nodes and edges, respectively. Recently, a new subject caught attention of the researchers was introduced, which is the combination of chemistry, information science and mathematics is called Cheminformatics, which studies QSAR/QSPR relationship, bioactivity and characterization of chemical compounds [1].
The topological index is a numeric value related with chemical compositions maintaining the correlation of chemical structures with many physico-chemical properties, chemical reactivity or biological activity. Topological indices are prepared on the grounds of the transformation of a chemical network into a number that describes the topology of the chemical network. Some of the main types of topological indices of graphs are distance-based topological indices, degree-based topological indices, and counting-related topological indices. Recently, numerous researchers have found topological indices for the study of fundamental properties of molecular graph or network. These networks have very motivating topological properties which have been considered in different characteristics in [2,3,4,5,6,7,8,9].
Let G=(V,E) be a simple connected graph, with V be the vertex set and E be the edge set of graph G, with order |V|=p, size |E|=q. The number of edges incident with a vertex ω is known as the degree of ω, denoted by ζ(ω). The reverse vertex degree (R(ω)) was introduced by Kulli [10] defined as: R(ω)=1−ζ(ω)+Δ, where Δ denoted the maximum degree of the given graph. Let ER(ω),R(μ) represents the edge partition of the given graph based on reverse degree of end vertices of an edge ωμ∈E and |ER(ω),R(μ)| represents its cardinality. There are detailed variations of topological indices mainly distance-based and degree-based indices, see [11,12,13,14,15,16]. Milan Randic [30] was the first who defined the degree-based indices and its reverse Randic index is defined as:
RRα(G)=∑ωμ∈E(G)(R(ω)×R(μ))α,α=12,−12,1,−1. | (1.1) |
Estrada et al. presented the atom bond connectivity (ABC) index in [18] and the reverse atom bond connectivity (RABC) is defined as:
RABC(G)=∑ωμ∈E(G)√R(ω)+R(μ)−2R(ω)×R(μ) | (1.2) |
Vukicevic and Furtula defined the geometric arithmetic (GA) index in [19] and the reverse geometric arithmetic (RGA) is presented as:
RGA(G)=∑ωμ∈E(G)2√R(ω)×R(μ)R(ω)+R(μ) | (1.3) |
Gutman et al. [20,21] defined the first and second Zagreb and its reverse indices as:
RM1(G)=∑ωμ∈E(G)(R(ω)+R(μ)) | (1.4) |
RM2(G)=∑ωμ∈E(G)(R(ω)×R(μ)) | (1.5) |
Shirdel et al. [22] introduced hyper Zagreb index. We defined the reverse hyper Zagreb index as:
RHM(G)=∑ωμ∈E(G)(R(ω)+R(μ))2 | (1.6) |
Furtula and Gutman [23] accomplished the forgotten index and its reverse forgotten index as:
RF(G)=∑ωμ∈E(G)((R(ω))2+(R(μ))2) | (1.7) |
Augmented Zagreb index was introduced by Furtula et al. [24] and the reverse augmented Zagreb index as:
RAZI(G)=∑ωμ∈E(G)(R(ω)×R(μ)R(ω)+R(μ)−2)3 | (1.8) |
Ranjini et al. [25] introduced the first redefined, second redefined and third redefined Zagreb indices. The reverse first redefined, second redefined and third redefined Zagreb indices are defined as:
RReZ1(G)=∑ωμ∈E(G)R(ω)+R(μ)R(ω)×R(μ) | (1.9) |
RReZ2(G)=∑ωμ∈E(G)R(ω)×R(μ)R(ω)+R(μ) | (1.10) |
RReZ3(G)=∑ωμ∈E(G)(R(ω)+R(μ))(R(ω)×R(μ)) | (1.11) |
For latest results on topological indices see [26,27,28,29,31,32,33,34,35]. In this paper, we compute the exact results for all the above reverse indices.
With the help of complete graphs of order 3 (K3), Chen et al. [36] assembled a hexagonal mesh. In terms of chemistry, these K3 graphs are also called oxide graphs. The Figure 1 is obtained by joining these K3 graphs. Two dimensional mesh graph HX(2) (see Figure 1 (a)), is obtained by joining six K3 graphs and three dimensional mesh graph HX(3) (see Figure 1 (b)) is obtained by putting K3 graphs around all side of HX(2). Furthermore, repeating the same process by putting the t K3 graph around each hexagon, we obtained the tth hexagonal mesh. To be noted that the one dimensional hexagonal mesh graph does not exist.
Simonraj et al. [37] created the new network which is named as third type of hex-derived networks. The graphically construction algorithm for third type of hexagonal hex-derived network HHDN3(t) (see Figure 2), triangular hex-derived network THDN3(t) (see Figure 3) and rectangular hex-derived network RHDN3(t) (see Figure 4) are defined in [38,39] and they determined some topological indices of these new derived networks. Some networks such as hexagonal, honeycomb, and grid networks, for instance, endure closeness to atomic or molecular lattice configurations. Related research that applies this theory and which could get additional advantages from the visions of the new research is found in [40,41,42,43,44,45,46].
Let Γ1=HHDN3(t) be the third type of hexagonal hex-derived network which is shown in Figure 2, where t≥4. The graph Γ1 has 21t2−39t+19 vertices from which 18t2−36t+18 vertices of reverse degree 15, 4 vertices of reverse degree 12, 6t−12 vertices of reverse degree 9 and 3t2−9t+9 vertices of reverse degree 1. There are 63t2−123t+60 number of edges of Γ1 is partitioned into nine classes based on their reverse degrees which are given in Eq (3.1).
|ER(ω),R(μ)(Γ1)|={9t2−33t+30,for; R(ω)=1,R(μ)=112t−24,for; R(ω)=9,R(μ)=16t−18,for; R(ω)=9,R(μ)=96,for; R(ω)=12,R(μ)=112,for; R(ω)=12,R(μ)=936t2−108t+84,for; R(ω)=15,R(μ)=136t−72,for; R(ω)=15,R(μ)=924,for; R(ω)=15,R(μ)=1218t2−36t+18,for; R(ω)=15,R(μ)=15 | (3.1) |
In the next theorem, we will calculate the reverse Randic index, reverse Zagreb indices, reverse forgotten index for Γ1 graph.
Theorem 3.1. Let Γ1 be the third type of hexagonal hex-derived network, then
● RRα(Γ1)=[9+36(15)α+18(225)α]t2+[−33+12(9)α+6(81)α−108(15)α+36(135)α−36(225)α]t+30−24(9)α−18(81)α+6(12)α+12(108)α+84(15)α−72(135)α+24(180)α+18(225)α
● RM1(Γ1)=1134t2−1782t+630
● RM2(Γ1)=4599t2−4299t−366
● RHM(Γ1)=25452t2−36300t+11922
● RF(Γ1)=16254t2−27702t+12654
Proof. Let Γ1 be the third type of hexagonal hex-derived network which is shown in Figure 2. The order of hexagonal hex derived network Γ1 is p=|Γ1|=21t2−39t+19 and size is q=63t2−123t+60. The edge partitioned of Γ1 based on their reverse degrees are shown in Eq (3.1). Reverse Randic index can be calculated by using Eq (3.1). Thus, from Eq (1.1), it follows,
RRα(Γ1)=(1)α|E1,1(Γ1)|+(9)α|E9,1(Γ1)|+(81)α|E9,9(Γ1)|+(12)α|E12,1(Γ1)|+(108)α|E12,9(Γ1)|+(15)α|E15,1(Γ1)|+(135)α|E15,9(Γ1)|+(180)α|E15,12(Γ1)|+(225)α|E15,15(Γ1)|.
=(9t2−33t+30)+(9)α(12t−24)+(81)α(6t−18)+(12)α(6)+(108)α(12)+(15)α(36t2−108t+84)+(135)α(36t−72)+(180)α(24)+(225)α(18t2−36t+18).
=[9+36(15)α+18(225)α]t2+[−33+12(9)α+6(81)α−108(15)α+36(135)α−36(225)α]t+30−24(9)α−18(81)α+6(12)α+12(108)α+84(15)α−72(135)α+24(180)α+18(225)α. Put α=1 and after some calculation, we get reverse second Zagreb index RM2(Γ1) as:
RM2(Γ1)=4599t2−4299t−366. | (3.2) |
Using the Eq (1.4), we can determine the reverse first Zagreb index RM1(Γ1) as:
RM1(Γ1)=2×|E1,1(Γ1)|+10×|E9,1(Γ1)|+18×|E9,9(Γ1)|+13×|E12,1(Γ1)|+21×|E12,9(Γ1)|+16×|E15,1(Γ1)|+24×|E15,9(Γ1)|+27×|E15,12(Γ1)|+30×|E15,15(Γ1)|.
By putting the values of from equation (3.1) and after simplification, we obtain:
RM1(Γ1)=1134t2−1782t+630. | (3.3) |
Using the Eq (1.6), we can determine the reverse hyper Zagreb index RHM(Γ1) as:
RHM(Γ1)=4×|E1,1(Γ1)|+100×|E9,1(Γ1)|+324×|E9,9(Γ1)|+169×|E12,1(Γ1)|+441×|E12,9(Γ1)|+256×|E15,1(Γ1)|+576×|E15,9(Γ1)|+729×|E15,12(Γ1)|+900×|E15,15(Γ1)|.
After simplification, we get
RHM(Γ1)=25452t2−36300t+11922 |
Using the Eq (1.7), we can determine the reverse forgotten index RF(Γ1)) as:
RF(Γ1))=2×|E1,1(Γ1)|+82×|E9,1(Γ1)|+162×|E9,9(Γ1)|+145×|E12,1(Γ1)|+225×|E12,9(Γ1)|+226×|E15,1(Γ1)|+306×|E15,9(Γ1)|+369×|E15,12(Γ1)|+450×|E15,15(Γ1)|.
After simplification, we get
RF(Γ1)=16254t2−27702t+12654 |
In the next theorem, we will calculate the reverse atom bond connectivity index, reverse geometric arithmetic index for Γ1 graph.
Theorem 3.2. Let Γ1 be the third type of hexagonal hex-derived network, then
● RABC(Γ1)=(12√2105+12√75)t2+(8√2+83−36√2105+4√3305−24√75)t−8−16√2+√33+2√573+28√2105−8√3305+4√5+12√75
● RGA(Γ1)=(27+9√152)t2+(−2795−9√152)t+785+792√391−15√152+32√53.
Proof. The reverse atom bond connectivity (RABC(Γ1)), can be determined by using Eq (1.2) and Eq (3.1), as follows:
RABC(Γ1)=0×|E1,1(Γ1)|+√89×|E9,1(Γ1)|+√1681×|E9,9(Γ1)|+√1112×|E12,1(Γ1)|+√19108×|E12,9(Γ1)|+√1415×|E15,1(Γ1)|+√22135×|E15,9(Γ1)|+√25180×|E15,12(Γ1)|+√28225×|E15,15(Γ1)|.
After some simplification, we get
RABC(Γ1)=(12√2105+12√75)t2+(8√2+83−36√2105+4√3305−24√75)t−8−16√2+√33+2√573+28√2105−8√3305+4√5+12√75.
The reverse geometric arithmetic (RGA(Γ1)), can be determined by using Eq (1.3) and Eq (3.1), as follows:
RGA(Γ1)=|E1,1(Γ1)|+2√910×|E9,1(Γ1)|+2√8118×|E9,9(Γ1)|+2√1213×|E12,1(Γ1)|+2√10821×|E12,9(Γ1)|+2√1516×|E15,1(Γ1)|+2√13524×|E15,9(Γ1)|+2√18027×|E15,12(Γ1)|+2√22530×|E15,15(Γ1)|.
After some simplification, we get
RGA(Γ1)=(27+9√152)t2+(−2795−9√152)t+785+792√391−15√152+32√53. In the next theorems, we will calculate the reverse redefined Zagreb indices for Γ1 graph.
Theorem 3.3. Let Γ1 be the third type of hexagonal hex-derived network, then
● RReZ1(Γ1)=294t25−2474t15+362930
● RReZ2(Γ1)=693t24−2949t20−939071820
● RReZ3(Γ1)=130158t2−142518t+24828
Proof. Reverse redefined Zagreb indices can be calculated by using Eq (3.1), the RReZ1(Γ1) by using Eq (1.9) as follows:
RReZ1(Γ1)=2×|E1,1(Γ1)|+109×|E9,1(Γ1)|+1881×|E9,9(Γ1)|+1312×|E12,1(Γ1)|+21108×|E12,9(Γ1)|+1615×|E15,1(Γ1)|+24135×|E15,9(Γ1)|+27108×|E15,12(Γ1)|+30225×|E15,15(Γ1)|.
After some simplification, we get
RReZ1(Γ1)=294t25−2474t15+362930. |
The RReZ2(Γ1) can be determined by using Eq (1.10) as follows:
RReZ2(Γ1)=12×|E1,1(Γ1)|+910×|E9,1(Γ1)|+8118×|E9,9(Γ1)|+1213×|E12,1(Γ1)|+10821×|E12,9(Γ1)|+1516×|E15,1(Γ1)|+13524×|E15,9(Γ1)|+10827×|E15,12(Γ1)|+22530×|E15,15(Γ1)|.
After some simplification, we get
RReZ2(Γ1)=693t24−2949t20−939071820. |
The RReZ3(Γ1) can be calculated by using Eq (1.11) as follows:
RReZ3(Γ1)=2×|E1,1(Γ1)|+90×|E9,1(Γ1)|+1458×|E9,9(Γ1)|+156×|E12,1(Γ1)|+2268×|E12,9(Γ1)|+240×|E15,1(Γ1)|+3240×|E15,9(Γ1)|+2916×|E15,12(Γ1)|+6750×|E15,15(Γ1)|.
After some simplification, we get
RReZ3(Γ1)=130158t2−142518t+24828. |
Let Γ2=THDN3(t) be the third type of triangular hex-derived network which is shown in Figure 3, where t≥4. The graph Γ2 has 7t2−11t+62 vertices. There are 21t2−39t+182 number of edges of Γ2 is partitioned into six classes based on their reverse degrees which are given in Eq (4.1). Now we calculated reverse degree based indices such as: reverse Randic index RRα, reverse atom bond connectivity index RABC, reverse geometric arithmetic index RGA, first reverse Zagreb index RM1, second reverse Zagreb index RM2, reverse forgotten index RF, reverse hyper Zagreb index RHM and reverse redefined Zagreb indices for Γ2 graph.
|ER(ω),R(μ)(Γ2)|={3t22−21t2+18,for; R(ω)=1,R(μ)=16t−18,for; R(ω)=9,R(μ)=13t−6,for; R(ω)=9,R(μ)=96t2−30t+36,for; R(ω)=15,R(μ)=118t−30,for; R(ω)=15,R(μ)=93t2−6t+9,for; R(ω)=15,R(μ)=15 | (4.1) |
In the next theorem, we will calculate the reverse Randic index, reverse Zagreb indices, reverse forgotten index for Γ2 graph.
Theorem 4.1. Let Γ2 be the third type of triangular hex-derived network, then
● RRα(Γ2)=(32+6(15)α+3(225)α)t2+(−212+6(9)α+3(81)α−30(15)α+18(135)α−6(225)α)t+18−18(9)α−6(81)α+36(15)α−30(135)α+9(225)α.
● RM1(Γ2)=189t2−135t−126
● RM2(Γ2)=1533t22+1833t2−2115
● RHM(Γ2)=4242t2−1182t−3636
● RF(Γ2)=2709t2−3015t+594
Proof. Let Γ2 be the third type of triangular hex-derived network which is shown in Figure 3. The order of triangular hex derived network Γ2 is p=|Γ2|=7t2−11t+62 and size is q=21t2−39t+182. The edge partitioned of Γ2 based on their reverse degrees are shown in Eq (4.1). Reverse Randic index can be calculated by using Eq (4.1). Thus, from Eq (1.1), it follows,
RRα(Γ2)=(1)α|E1,1(Γ2)|+(9)α|E9,1(Γ2)|+(81)α|E9,9(Γ2)|+(15)α|E15,1(Γ2)|+(135)α|E15,9(Γ2)|+(225)α|E15,15(Γ2)|.
After simplification, we get
RRα(Γ2)=(32+6(15)α+3(225)α)t2+(−212+6(9)α+3(81)α−30(15)α+18(135)α−6(225)α)t+18−18(9)α−6(81)α+36(15)α−30(135)α+9(225)α.
Put α=1 and after some calculation, we get reverse second Zagreb index RM2(Γ2) as:
RM2(Γ2)=1533t22+1833t2−2115 | (4.2) |
Using the Eq (1.4), we can determine the reverse first Zagreb index RM1(Γ2) as:
RM1(Γ2)=2×|E1,1(Γ1)|+10×|E9,1(Γ1)|+18×|E9,9(Γ1)|+16×|E15,1(Γ1)|+24×|E15,9(Γ1)|+30×|E15,15(Γ1)|.
By putting the values of from Eq (4.1) and after simplification, we obtain:
RM1(Γ2)=189t2−135t−126 | (4.3) |
Using the Eq (1.6), we can determine the reverse hyper Zagreb index RHM(Γ2) as:
RHM(Γ2)=4×|E1,1(Γ2)|+100×|E9,1(Γ2)|+324×|E9,9(Γ2)|+256×|E15,1(Γ2)|+576×|E15,9(Γ2)|+900×|E15,15(Γ2)|.
After simplification, we get
RHM(Γ2)=4242t2−1182t−3636. |
Using the Eq (1.7), we can determine the reverse forgotten index RF(Γ2)) as:
RF(Γ2))=2×|E1,1(Γ2)|+82×|E9,1(Γ2)|+162×|E9,9(Γ2)|+226×|E15,1(Γ2)|+306×|E15,9(Γ2)|+450×|E15,15(Γ2)|.
After simplification, we get
RF(Γ2)=2709t2−3015t+594. |
In the next theorem, we will calculate the reverse atom bond connectivity index, reverse geometric arithmetic index for Γ2 graph.
Theorem 4.2. Let Γ2 be the third type of triangular hex-derived network, then
● RABC(Γ2)=(2√2105+2√75)t2+(4√2+43−2√210+2√3305−4√75)t−83−12√2+12√2105−2√3303+6√75
● RGA(Γ2)=(92+3√154)t2+(−9910+3√154)t+515−3√15.
Proof. The reverse atom bond connectivity (RABC(Γ2)), can be determined by using Eq (1.2) and Eq (4.1), as follows:
RABC(Γ2)=0×|E1,1(Γ2)|+√89×|E9,1(Γ2)|+√1681×|E9,9(Γ2)|+√1415×|E15,1(Γ2)|+√22135×|E15,9(Γ2)|+√28225×|E15,15(Γ2)|.
After some simplification, we get
RABC(Γ2)=(2√2105+2√75)t2+(4√2+43−2√210+2√3305−4√75)t−83−12√2+12√2105−2√3303+6√75.
The reverse geometric arithmetic (RGA(Γ2)), can be determined by using Eq (1.3) and Eq (4.1), as follows:
RGA(Γ2)=|E1,1(Γ2)|+2√910×|E9,1(Γ2)|+2√8118×|E9,9(Γ2)|+2√1516×|E15,1(Γ2)|+2√13524×|E15,9(Γ2)|+2√22530×|E15,15(Γ2)|.
After some simplification, we get
RGA(Γ2)=(92+3√154)t2+(−9910+3√154)t+515−3√15. |
In the next theorems, we will calculate the reverse redefined Zagreb indices for Γ2 graph.
Theorem 4.3. Let Γ2 be the third type of triangular hex-derived network, then
● RReZ1(Γ2)=49t25−649t15+73415
● RReZ2(Γ2)=231t28+1671t40−101710
● RReZ3(Γ2)=21693t2+15513t−38142.
Proof. Reverse redefined Zagreb indices can be calculated by using Eq (4.1), the RReZ1(Γ2) by using Eq (1.9) as follows:
RReZ1(Γ2)=2×|E1,1(Γ2)|+109×|E9,1(Γ2)|+1881×|E9,9(Γ2)|+1615×|E15,1(Γ2)|+24135×|E15,9(Γ2)|+30225×|E15,15(Γ2)|.
After some simplification, we get
RReZ1(Γ2)=49t25−649t15+73415. |
The RReZ2(Γ2) can be determined by using Eq (1.10) as follows:
RReZ2(Γ2)=12×|E1,1(Γ2)|+910×|E9,1(Γ2)|+8118×|E9,9(Γ2)|+1516×|E15,1(Γ2)|+13524×|E15,9(Γ2)|+22530×|E15,15(Γ2)|.
After some simplification, we get
RReZ2(Γ2)=231t28+1671t40−101710. |
The RReZ3(Γ2) can be calculated by using Eq (1.11) as follows:
RReZ3(Γ2)=2×|E1,1(Γ2)|+90×|E9,1(Γ2)|+1458×|E9,9(Γ2)|+240×|E15,1(Γ2)|+3240×|E15,9(Γ2)|+6750×|E15,15(Γ2)|.
After some simplification, we get
RReZ3(Γ2)=21693t2+15513t−38142. |
In this section, we calculate certain reverse degree based topological indices of the third type of rectangular hex-derived network, RHDN3(t,w) of dimension t=w. Now we calculated reverse degree based indices such as: reverse Randic index RRα, reverse atom bond connectivity index RABC, reverse geometric arithmetic index RGA, first reverse Zagreb index RM1, second reverse Zagreb index RM2, reverse forgotten index RF, reverse augmented Zagreb index RAZI, reverse hyper Zagreb index RHM and reverse redefined Zagreb indices for Γ3 graph.
|ER(ω),R(μ)(Γ3)|={3t2−16t+21,for; R(ω)=1,R(μ)=18t−20,for; R(ω)=9,R(μ)=14t−10,for; R(ω)=9,R(μ)=92,for; R(ω)=12,R(μ)=14,for; R(ω)=12,R(μ)=912t2−48t+48,for; R(ω)=15,R(μ)=124t−44,for; R(ω)=15,R(μ)=98,for; R(ω)=15,R(μ)=126t2−12t+10,for; R(ω)=15,R(μ)=15 | (5.1) |
In the next theorem, we will calculate the reverse Randic index, reverse Zagreb indices, reverse forgotten index for Γ3 graph.
Theorem 5.1. Let Γ3 be the third type of rectangular hex-derived network, then
● RRα(Γ3)=(3+12(15)α+6(225)α)t2+(−16+8(9)α+4(81)α−48(15)α+24(135)α−12(225)α)t+21−20(9)α−10(81)α+2(12)α+4(108)α+48(15)α−44(135)α+8(180)α+10(225)α
● RM1(Γ3)=378t2−432t
● RM2(Γ3)=1533t2+200t−2043
● RHM(Γ3)=8484t2−7232t−1278
● RF(Γ3)=5418t2−7632t+2808.
Proof. Let Γ3 be the third type of rectangular hex-derived network which is shown in Figure 4. The order of hexagonal hex derived network Γ3 is p=|Γ1|=7t2−12t+6 and size is q=21t2−40t+19. The edge partitioned of Γ3 based on their reverse degrees are shown in Eq (5.1). Reverse Randic index can be calculated by using Eq (5.1). Thus, from Eq (1.1), it follows,
RRα(Γ3)=(1)α|E1,1(Γ3)|+(9)α|E9,1(Γ3)|+(81)α|E9,9(Γ3)|+(12)α|E12,1(Γ3)|+(108)α|E12,9(Γ3)|+(15)α|E15,1(Γ3)|+(135)α|E15,9(Γ3)|+(180)α|E15,12(Γ3)|+(225)α|E15,15(Γ3)|.
After Simplification, we get
RRα(Γ3)=(3+12(15)α+6(225)α)t2+(−16+8(9)α+4(81)α−48(15)α+24(135)α−12(225)α)t+21−20(9)α−10(81)α+2(12)α+4(108)α+48(15)α−44(135)α+8(180)α+10(225)α.
Put α=1 and after some calculation, we get reverse second Zagreb index RM2(Γ3) as:
RM2(Γ3)=1533t2+200t−2043. | (5.2) |
Using the Eq (1.4), we can determine the reverse first Zagreb index RM1(Γ3) as:
RM1(Γ3)=2×|E1,1(Γ3)|+10×|E9,1(Γ3)|+18×|E9,9(Γ3)|+13×|E12,1(Γ3)|+21×|E12,9(Γ3)|+16×|E15,1(Γ3)|+24×|E15,9(Γ3)|+27×|E15,12(Γ3)|+30×|E15,15(Γ3)|.
By putting the values of from Eq (5.1) and after simplification, we obtain:
RM1(Γ3)=378t2−432t. | (5.3) |
Using the Eq (1.6), we can determine the reverse hyper Zagreb index RHM(Γ3) as:
RHM(Γ3)=4×|E1,1(Γ3)|+100×|E9,1(Γ3)|+324×|E9,9(Γ3)|+169×|E12,1(Γ3)|+441×|E12,9(Γ3)|+256×|E15,1(Γ3)|+576×|E15,9(Γ3)|+729×|E15,12(Γ3)|+900×|E15,15(Γ3)|.
After simplification, we get
RHM(Γ3)=8484t2−7232t−1278. |
Using the Eq (1.7), we can determine the reverse forgotten index RF(Γ3)) as:
RF(Γ3))=2×|E1,1(Γ3)|+82×|E9,1(Γ3)|+162×|E9,9(Γ3)|+145×|E12,1(Γ3)|+225×|E12,9(Γ3)|+226×|E15,1(Γ3)|+306×|E15,9(Γ3)|+369×|E15,12(Γ3)|+450×|E15,15(Γ3)|.
After simplification, we get
RF(Γ3)=5418t2−7632t+2808. |
In the next theorem, we will calculate the reverse atom bond connectivity index, reverse geometric arithmetic index for Γ3 graph.
Theorem 5.2. Let Γ3 be the third type of rectangular hex-derived network, then
● RABC(Γ3)=(4√2105+4√75)t2+(16√23+169−16√2105+8√33015−8√75)t−409−40√23+√333+2√579+16√2105−44√33045+4√53+4√73.
● RGA(Γ3)=(9+3√152)t2−96t5+9+264√391−5√15+32√59.
Proof. The reverse atom bond connectivity (RABC(Γ3)), can be determined by using Eq (1.2) and Eq (5.1), as follows:
RABC(Γ3)=0×|E1,1(Γ3)|+√89×|E9,1(Γ3)|+√1681×|E9,9(Γ3)|+√1112×|E12,1(Γ3)|+√19108×|E12,9(Γ3)|+√1415×|E15,1(Γ3)|+√22135×|E15,9(Γ3)|+√25180×|E15,12(Γ3)|+√28225×|E15,15(Γ3)|.
After some simplification, we get
RABC(Γ3)=(4√2105+4√75)t2+(16√23+169−16√2105+8√33015−8√75)t−409−40√23+√333+2√579+16√2105−44√33045+4√53+4√73.
The reverse geometric arithmetic (RGA(Γ3)), can be determined by using Eq (1.3) and Eq (5.1), as follows:
RGA(Γ3)=|E1,1(Γ3)|+2√910×|E9,1(Γ3)|+2√8118×|E9,9(Γ3)|+2√1213×|E12,1(Γ3)|+2√10821×|E12,9(Γ3)|+2√1516×|E15,1(Γ3)|+2√13524×|E15,9(Γ3)|+2√18027×|E15,12(Γ3)|+2√22530×|E15,15(Γ3)|.
After some simplification, we get
RGA(Γ3)=(9+3√152)t2−96t5+9+264√391−5√15+32√59.
In the next theorems, we will calculate the reverse redefined Zagreb indices for Γ3 graph.
Theorem 5.3. Let Γ3 be the third type of rectangular hex-derived network, then
● RReZ1(Γ3)=98t25−3184t45+597790
● RReZ2(Γ3)=231t24+86t5−28460273
● RReZ3(Γ3)=43386t2−8240t−31614.
Proof. Reverse redefined Zagreb indices can be calculated by using Eq (5.1), the RReZ1(Γ3) by using Eq (1.9) as follows:
RReZ1(Γ3)=2×|E1,1(Γ3)|+109×|E9,1(Γ3)|+1881×|E9,9(Γ3)|+1312×|E12,1(Γ3)|+21108×|E12,9(Γ3)|+1615×|E15,1(Γ3)|+24135×|E15,9(Γ3)|+27108×|E15,12(Γ3)|+30225×|E15,15(Γ3)|.
After some simplification, we get
RReZ1(Γ3)=98t25−3184t45+597790. |
The RReZ2(Γ1) can be determined by using equation (1.10) as follows:
RReZ2(Γ3)=12×|E1,1(Γ3)|+910×|E9,1(Γ3)|+8118×|E9,9(Γ3)|+1213×|E12,1(Γ3)|+10821×|E12,9(Γ3)|+1516×|E15,1(Γ3)|+13524×|E15,9(Γ3)|+10827×|E15,12(Γ3)|+22530×|E15,15(Γ3)|.
After some simplification, we get
RReZ2(Γ3)=231t24+86t5−28460273. |
The RReZ3(Γ3) can be calculated by using Eq (1.11) as follows:
RReZ3(Γ3)=2×|E1,1(Γ3)|+90×|E9,1(Γ3)|+1458×|E9,9(Γ3)|+156×|E12,1(Γ3)|+2268×|E12,9(Γ3)|+240×|E15,1(Γ3)|+3240×|E15,9(Γ3)|+2916×|E15,12(Γ3)|+6750×|E15,15(Γ3)|.
After some simplification, we get
RReZ3(Γ3)=43386t2−8240t−31614. |
In this article, we have calculated the exact solutions of reverse degree-based topological descriptors for hex-derived networks of third type. Hex-derived network has a variety of useful applications in pharmacy, electronics, and networking. We obtained the reverse degree-based indices such as reverse Randic index, reverse atom bond connectivity index, reverse geometric arithmetic index, reverse Zagreb indices, reverse redefined Zagreb indices for hex derived networks. These results may be helpful for people working in computer science and chemistry who encounter hex-derived networks.
The authors declare that there is no conflict of financial interests regarding the publication of this paper.
[1] |
K. M. Alanazi, Modeling and simulating an epidemic in two dimensions with an application regarding COVID-19, Computation, 12 (2024), 34. https://doi.org/10.3390/computation12020034 doi: 10.3390/computation12020034
![]() |
[2] |
K. M. Alanazi, Z. Jackiewicz, H. R. Thieme, Numerical simulations of spread of rabies in a spatially distributed fox population, Math. Comput. Simul., 159 (2019), 161–182. https://doi.org/10.1016/j.matcom.2018.11.010 doi: 10.1016/j.matcom.2018.11.010
![]() |
[3] |
K. M. Alanazi, Z. Jackiewicz, H. R. Thieme, Numerical simulations of the spread of rabies in two-dimensional space, Appl. Numer. Math., 135 (2019), 87–98. https://doi.org/10.1016/j.apnum.2018.08.009 doi: 10.1016/j.apnum.2018.08.009
![]() |
[4] |
K. M. Alanazi, Z. Jackiewicz, H. R. Thieme, Spreading speeds of rabies with territorial and diffusing rabid foxes, Discr. Cont. Dyn. Syst. Ser. B, 25 (2020), 2143. https://doi.org/10.3934/dcdsb.2019222 doi: 10.3934/dcdsb.2019222
![]() |
[5] |
C. Anastassopoulou, L. Russo, L. Tsakris, C. Siettos, Modelling and forecasting of the COVID-19 outbreak, PLoS One, 15 (2020), e0230405. https://doi.org/10.1371/journal.pone.0230405 doi: 10.1371/journal.pone.0230405
![]() |
[6] | J. Arino, S. Portet, A simple model for COVID-19, Infect. Dis. Model., 5 (2020), 309–315. |
[7] | D. G. Aronson, The asymptotic speed of propagation of a simple epidemic, Nonlinear Diffus., 14, (1977), 1–23. |
[8] | D. G. Aronson, H. F. Weinberger, Nonlinear diffusion in population genetics, combustion, and nerve pulse propagation, Partial Differ. Equ. Related Topics, 446, (1975), 5–49. |
[9] |
D. G. Aronson, H. F. Weinberger, Multidimensional nonlinear diffusion arising in population genetics, Adv. Math., 30 (1978), 33–76. https://doi.org/10.1016/0001-8708(78)90130-5 doi: 10.1016/0001-8708(78)90130-5
![]() |
[10] |
A. Babaei, M. Ahmadi, H. Jafari, A. Liya, A mathematical model to examine the effect of quarantine on the spread of coronavirus, Chaos Solitons Fract., 142 (2021), 110418. https://doi.org/10.1016/j.chaos.2020.110418 doi: 10.1016/j.chaos.2020.110418
![]() |
[11] |
B. Batista, Minimizing disease spread on a quarantined cruise ship: a model of COVID-19 with asymptomatic infections, Math. Biosci., 329 (2020), 108442. https://doi.org/10.1016/j.mbs.2020.108442 doi: 10.1016/j.mbs.2020.108442
![]() |
[12] |
H. Berestycki, J. M. Roquejoffre, L. Rossi, Propagation of epidemics along lines with fast diffusion, Bull. Math. Biol., 83 (2021), 2. https://doi.org/10.1007/s11538-020-00826-8 doi: 10.1007/s11538-020-00826-8
![]() |
[13] |
J. Chen, Pathogenicity and transmissibility of 2019-nCoV-a quick overview and comparison with other emerging viruses, Microbes Infect., 22 (2020), 69–71. https://doi.org/10.1016/j.micinf.2020.01.004 doi: 10.1016/j.micinf.2020.01.004
![]() |
[14] |
Q. Cui, Z. Hu, Y. Li, J. Han, Z. Teng, J. Qian, Dynamic variations of the COVID-19 disease at different quarantine strategies in Wuhan and mainland China, J. Infect. Publ. Heal., 13 (2020), 849–855. https://doi.org/10.1016/j.jiph.2020.05.014 doi: 10.1016/j.jiph.2020.05.014
![]() |
[15] | O. Diekmann, Limiting behaviour in an epidemic model, Nonlinear Anal., 1 (1977), 459–470. |
[16] |
O. Diekmann, Thresholds and travelling waves for the geographical spread of infection, J. Math. Biol., 6 (1978), 109–130. https://doi.org/10.1007/BF02450783 doi: 10.1007/BF02450783
![]() |
[17] |
O. Diekmann, Run for your life. A note on the asymptotic speed of propagation of an epidemic, J. Diff. Equ., 33 (1979), 5873. https://doi.org/10.1016/0022-0396(79)90080-9 doi: 10.1016/0022-0396(79)90080-9
![]() |
[18] |
R. Engbert, M. M. Rabe, R. Kliegl, S. Reich, Sequential data assimilation of the stochastic SEIR epidemic model for regional COVID-19 dynamics, Bull. Math. Biol., 83 (2021), 1. https://doi.org/10.1007/s11538-020-00834-8 doi: 10.1007/s11538-020-00834-8
![]() |
[19] |
Y. Feng, Q. Li, X. Tong, R. Wang, S. Zhai, C. Gao, et al., Spatiotemporal spread pattern of the COVID-19 cases in China, PLoS One, 15 (2020), e0244351. https://doi.org/10.1371/journal.pone.0244351 doi: 10.1371/journal.pone.0244351
![]() |
[20] |
M. Gatto, E. Bertuzzo, L. Mari, S. Miccoli, L. Carraro, R. Casagrandi, et al., Spread and dynamics of the COVID-19 epidemic in Italy: effects of emergency containment measures, Proc. Natl. Academy Sci., 117 (2020), 10484–10491. https://doi.org/10.1073/pnas.2004978117 doi: 10.1073/pnas.2004978117
![]() |
[21] |
Y. Guo, T. Li, Modeling the competitive transmission of the Omicron strain and Delta strain of COVID-19, J. Math. Anal. Appl., 526 (2023), 127283. https://doi.org/10.1016/j.jmaa.2023.127283 doi: 10.1016/j.jmaa.2023.127283
![]() |
[22] |
J. Hellewell, S. Abbott, A. Gimma, N. I. Bosse, C. I Jarvis, T. W. Russell, et al., Feasibility of controlling COVID-19 outbreaks by isolation of cases and contacts, Lancet Global Heal., 8 (2020), e488–e496. https://doi.org/10.1016/S2214-109X(20)30074-7 doi: 10.1016/S2214-109X(20)30074-7
![]() |
[23] |
C. Hou, J. Chen, Y. Zhou, L. Hua, J. Yuan, S. He, et al., The effectiveness of quarantine of Wuhan city against the Corona Virus Disease 2019 (COVID‐19): a well‐mixed SEIR model analysis, J. Medical Virol., 92 (2020), 841–848. https://doi.org/10.1002/jmv.25827 doi: 10.1002/jmv.25827
![]() |
[24] |
K. K. Hwang, C. J. Edholm, O. Saucedo, L. J. S. Allen, N. Shakiba, A hybrid epidemic model to explore stochasticity in COVID-19 dynamics, Bull. Math. Biol., 84 (2022), 91. https://doi.org/10.1007/s11538-022-01030-6 doi: 10.1007/s11538-022-01030-6
![]() |
[25] |
E. Iboi, O. O. Sharomi, C. Ngonghala, A. B. Gumel, Mathematical modeling and analysis of COVID-19 pandemic in Nigeria, Math. Biosci. Eng., 17 (2020), 7192–7220. https://doi.org/10.3934/mbe.2020369 doi: 10.3934/mbe.2020369
![]() |
[26] |
A. Kouidere, L. E. Youssoufi, H. Ferjouchia, O. Balatif, M. Rachik, Optimal control of mathematical modeling of the spread of the COVID-19 pandemic with highlighting the negative impact of quarantine on diabetics people with cost-effectiveness, Chaos Solitons Fract., 145 (2021), 110777. https://doi.org/10.1016/j.chaos.2021.110777 doi: 10.1016/j.chaos.2021.110777
![]() |
[27] |
A. J. Kucharski, T. W. Russell, C. Diamond, Y. Liu, J. Edmunds, S. Funk, et al., Early dynamics of transmission and control of COVID-19: a mathematical modelling study, Lancet Infect. Dis., 20 (2020), 553–558. https://doi.org/10.1016/S1473-3099(20)30144-4 doi: 10.1016/S1473-3099(20)30144-4
![]() |
[28] |
Q. Li, X. Guan, P. Wu, X. Wang, L. Zhou, Y. Tong, et al., Early transmission dynamics in Wuhan, China, of novel coronavirus–infected pneumonia, New England J. Medic., 382 (2020), 1199–1207. https://doi.org/10.1056/NEJMoa2001316 doi: 10.1056/NEJMoa2001316
![]() |
[29] |
T. Li, Y. Guo, Modeling and optimal control of mutated COVID-19 (Delta strain) with imperfect vaccination, Chaos Solitons Fract., 156 (2022), 111825. https://doi.org/10.1016/j.chaos.2022.111825 doi: 10.1016/j.chaos.2022.111825
![]() |
[30] |
Q. Lin, S. Zhao, D. Gao, Y. Lou, S. Yang, S. S. Musa, et al., A conceptual model for the coronavirus disease 2019 (COVID-19) outbreak in Wuhan, China with individual reaction and governmental action, Int. J. Infect. Dis., 93 (2020), 211–216. https://doi.org/10.1016/j.ijid.2020.02.058 doi: 10.1016/j.ijid.2020.02.058
![]() |
[31] |
J. M. Read, J. R. Bridgen, D. A. Cummings, A. Ho, C. P. Jewell, Novel coronavirus 2019-nCoV (COVID-19): early estimation of epidemiological parameters and epidemic size estimates, Philos. T. Royal Soc. B, 376 (2021), 20200265. https://doi.org/10.1098/rstb.2020.0265 doi: 10.1098/rstb.2020.0265
![]() |
[32] | A. Remuzzi, G. Remuzzi, COVID-19 and Italy: what next? Lancet, 395 (2020), 1225–1228. https://doi.org/10.1016/S0140-6736(20)30627-9 |
[33] | S. Ruan, Spatial-Temporal Dynamics in Nonlocal Epidemiological Models, Berlin: Springer, 2007. |
[34] |
M. A. Safi, A. B. Gumel, Dynamics of a model with quarantine-adjusted incidence and quarantine of susceptible individuals, J. Math. Anal. Appl., 399 (2013), 565–575. https://doi.org/10.1016/j.jmaa.2012.10.015 doi: 10.1016/j.jmaa.2012.10.015
![]() |
[35] |
B. Tang, F. Xia, S. Tang, N. L. Bragazzi, Q. Li, X. Sun, et al., The effectiveness of quarantine and isolation determine the trend of the COVID-19 epidemic in the final phase of the current outbreak in China, Int. J. Infect. Dis., 96 (2020), 288–293. https://doi.org/10.1016/j.ijid.2020.05.113 doi: 10.1016/j.ijid.2020.05.113
![]() |
[36] | J. Tanimoto, Sociophysics Approach to Epidemics, Singapore: Springer, 2021. |
[37] |
H. R. Thieme, A model for the spatial spread of an epidemic, J. Math. Biol., 4 (1977), 337–351. https://doi.org/10.1007/BF00275082 doi: 10.1007/BF00275082
![]() |
[38] |
H. R. Thieme, Asymptotic estimates of the solutions of nonlinear integral equations and asymptotic speeds for the spread of populations, J. Reine Angew. Math., 306 (1979), 94–121. https://doi.org/10.1515/crll.1979.306.94 doi: 10.1515/crll.1979.306.94
![]() |
[39] | H. R. Thieme, Mathematics in Population Biology, Princeton: Princeton University Press, 2003. |
[40] |
H. R. Thieme, X. Q. Zhao, Asymptotic speeds of spread and traveling waves for integral equations and delayed reaction-diffusion models, J. Diff. Equ., 195 (2003), 430–470. https://doi.org/10.1016/S0022-0396(03)00175-X doi: 10.1016/S0022-0396(03)00175-X
![]() |
[41] |
A. Viguerie, G. Lorenzo, F. Auricchio, D. Baroli, T. J. Hughes, A. Patton, et al., Simulating the spread of COVID-19 via a spatially-resolved susceptible-exposed-infected-recovered-deceased (SEIRD) model with heterogeneous diffusion, Appl. Math. Lett., 111 (2021), 106617. https://doi.org/10.1016/j.aml.2020.106617 doi: 10.1016/j.aml.2020.106617
![]() |
[42] |
Y. Wang, Y. Wang, Y. Chen, Q. Qin, Unique epidemiological and clinical features of the emerging 2019 novel coronavirus pneumonia (COVID‐19) implicate special control measures, J. Medical Virol., 92 (2020), 568–576. https://doi.org/10.1002/jmv.25748 doi: 10.1002/jmv.25748
![]() |
[43] |
F. Wei, R. Zhou, Z. Jin, S. Huang, Z. Peng, J. Wang, et al., COVID-19 transmission driven by age-group mathematical model in Shijiazhuang City of China, Infect. Dis. Model., 8 (2023), 1050–1062. https://doi.org/10.1016/j.idm.2023.08.004 doi: 10.1016/j.idm.2023.08.004
![]() |
[44] |
J. T. Wu, K. Leung, G. M. Leung, Nowcasting and forecasting the potential domestic and international spread of the 2019-nCoV outbreak originating in Wuhan, China: a modelling study, Lancet, 395 (2020), 689–697. https://doi.org/10.1016/S0140-6736(20)30260-9 doi: 10.1016/S0140-6736(20)30260-9
![]() |
[45] |
R. Xu, H. Rahmandad, M. Gupta, C. DiGennaro, N. Ghaffarzadegan, H. Amini, et al., Weather, air pollution, and SARS-CoV-2 transmission: a global analysis, Lancet Planetary Heal., 5 (2021), e671–e680. https://doi.org/10.1016/S2542-5196(21)00202-3 doi: 10.1016/S2542-5196(21)00202-3
![]() |
[46] |
S. Zhao, Q. Lin, J. Ran, S. S. Musa, G. Yang, W. Wang, et al., Preliminary estimation of the basic reproduction number of novel coronavirus (2019-nCoV) in China, from 2019 to 2020: a data-driven analysis in the early phase of the outbreak, Int. J. Infect. Dis., 92 (2020), 214–217. https://doi.org/10.1016/j.ijid.2020.01.050 doi: 10.1016/j.ijid.2020.01.050
![]() |
[47] |
C. C. Zhu, J. Zhu, Dynamic analysis of a delayed COVID-19 epidemic with home quarantine in temporal-spatial heterogeneous via global exponential attractor method, Chaos Solitons Fract., 143 (2021), 110546. https://doi.org/10.1016/j.chaos.2020.110546 doi: 10.1016/j.chaos.2020.110546
![]() |
1. | Saima Parveen, Fozia Bashir Farooq, Nadeem Ul Hassan Awan, Rakotondrajao Fanja, Muhammad Farooq Choudhary, Muhammad Kamran Jamil, Topological Indices of Drugs Used in Rheumatoid Arthritis Treatment and Its QSPR Modeling, 2022, 2022, 2314-4785, 1, 10.1155/2022/1562125 | |
2. | Ali N.A. Koam, Moin A. Ansari, Azeem Haider, Ali Ahmad, Muhammad Azeem, Topological properties of reverse-degree-based indices for sodalite materials network, 2022, 15, 18785352, 104160, 10.1016/j.arabjc.2022.104160 | |
3. | Ali N. A. Koam, Ali Ahmad, Ashfaq Ahmed Qummer, Gohar Ali, On the Study of Reverse Degree-Based Topological Properties for the Third Type of p th Chain Hex-Derived Network, 2021, 2021, 2314-4785, 1, 10.1155/2021/4540276 | |
4. | Usman Babar, Asim Naseem, Hani Shaker, Mian Muhammad Zobair, Haidar Ali, Andrea Penoni, Eccentricity-Based Topological Descriptors of First Type of Hex-Derived Network, 2022, 2022, 2090-9071, 1, 10.1155/2022/3340057 | |
5. | Vignesh Ravi, QSPR analysis of drugs used for treatment of hepatitis via reduced reverse degree-based topological descriptors, 2024, 99, 0031-8949, 105236, 10.1088/1402-4896/ad729d | |
6. | Muhammad Mudassar Hassan, Topological Descriptors of Molecular Networks via Reverse Degree, 2023, 1040-6638, 1, 10.1080/10406638.2023.2274473 | |
7. | Qasem M. Tawhari, Muhammad Naeem, Abdul Rauf, Muhammad Kamran Siddiqui, Oladele Oyelakin, Modeling and estimation of physiochemical properties of cancer drugs using entropy measures, 2025, 15, 2045-2322, 10.1038/s41598-025-87755-5 |