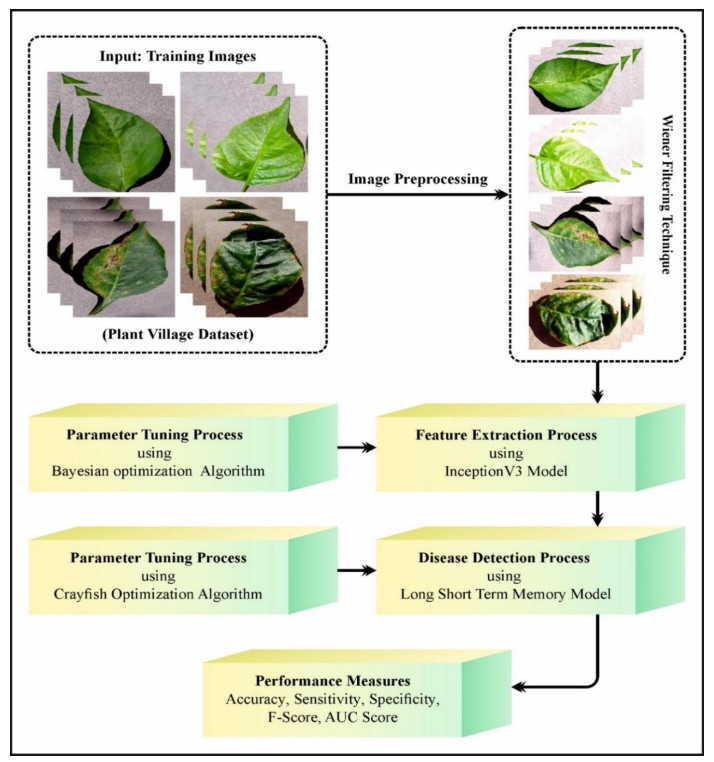
Agricultural decision-making involves a complex process of choosing strategies and options to enhance resource utilization, overall productivity, and farming practices. Agricultural stakeholders and farmers regularly make decisions at various levels of the farm cycle, ranging from crop selection and planting to harvesting and marketing. In agriculture, where crop health has played a central role in economic and yield outcomes, incorporating deep learning (DL) techniques has developed as a transformative force for the decision-making process. DL techniques, with their capability to discern subtle variations and complex patterns in plant health, empower agricultural experts and farmers to make informed decisions based on data-driven, real-time insights. Thus, we presented a Bayesian optimizer with deep learning based pepper leaf disease detection for decision making (BODL-PLDDM) approach in the agricultural sector. The BODL-PLDDM technique aimed to identify the healthy and bacterial spot pepper leaf disease. Primarily, the BODL-PLDDM technique involved a Wiener filtering (WF) approach for pre-processing. Besides, the complex and intrinsic feature patterns could be extracted using the Inception v3 model. Also, the Bayesian optimization (BO) algorithm was used for the optimal hyperparameter selection process. For disease detection, a crayfish optimization algorithm (COA) with a long short-term memory (LSTM) method was employed to identify the precise presence of pepper leaf diseases. The experimentation validation of the BODL-PLDDM system was verified using the Plant Village dataset. The obtained outcomes underlined the promising detection results of the BODL-PLDDM technique over other existing methods.
Citation: Asma A Alhashmi, Manal Abdullah Alohali, Nazir Ahmad Ijaz, Alaa O. Khadidos, Omar Alghushairy, Ahmed Sayed. Bayesian optimization with deep learning based pepper leaf disease detection for decision-making in the agricultural sector[J]. AIMS Mathematics, 2024, 9(7): 16826-16847. doi: 10.3934/math.2024816
[1] | Wahida Mansouri, Amal Alshardan, Nazir Ahmad, Nuha Alruwais . Deepfake image detection and classification model using Bayesian deep learning with coronavirus herd immunity optimizer. AIMS Mathematics, 2024, 9(10): 29107-29134. doi: 10.3934/math.20241412 |
[2] | S. Rama Sree, E Laxmi Lydia, C. S. S. Anupama, Ramya Nemani, Soojeong Lee, Gyanendra Prasad Joshi, Woong Cho . A battle royale optimization with feature fusion-based automated fruit disease grading and classification. AIMS Mathematics, 2024, 9(5): 11432-11451. doi: 10.3934/math.2024561 |
[3] | E Laxmi Lydia, Chukka Santhaiah, Mohammed Altaf Ahmed, K. Vijaya Kumar, Gyanendra Prasad Joshi, Woong Cho . An equilibrium optimizer with deep recurrent neural networks enabled intrusion detection in secure cyber-physical systems. AIMS Mathematics, 2024, 9(5): 11718-11734. doi: 10.3934/math.2024574 |
[4] | Hanan T. Halawani, Aisha M. Mashraqi, Yousef Asiri, Adwan A. Alanazi, Salem Alkhalaf, Gyanendra Prasad Joshi . Nature-Inspired Metaheuristic Algorithm with deep learning for Healthcare Data Analysis. AIMS Mathematics, 2024, 9(5): 12630-12649. doi: 10.3934/math.2024618 |
[5] | Suprawee Lertnaweephorn, Usa Wannasigha Humphries, Amir Khan . Stability analysis and optimal control for leaf brown spot disease of rice. AIMS Mathematics, 2023, 8(4): 9602-9623. doi: 10.3934/math.2023485 |
[6] | Fatma S. Alrayes, Latifah Almuqren, Abdullah Mohamed, Mohammed Rizwanullah . Image encryption with leveraging blockchain-based optimal deep learning for Secure Disease Detection and Classification in a smart healthcare environment. AIMS Mathematics, 2024, 9(6): 16093-16115. doi: 10.3934/math.2024779 |
[7] | Sultanah M. Alshammari, Nofe A. Alganmi, Mohammed H. Ba-Aoum, Sami Saeed Binyamin, Abdullah AL-Malaise AL-Ghamdi, Mahmoud Ragab . Hybrid arithmetic optimization algorithm with deep learning model for secure Unmanned Aerial Vehicle networks. AIMS Mathematics, 2024, 9(3): 7131-7151. doi: 10.3934/math.2024348 |
[8] | Hend Khalid Alkahtani, Nuha Alruwais, Asma Alshuhail, Nadhem NEMRI, Achraf Ben Miled, Ahmed Mahmud . Election-based optimization algorithm with deep learning-enabled false data injection attack detection in cyber-physical systems. AIMS Mathematics, 2024, 9(6): 15076-15096. doi: 10.3934/math.2024731 |
[9] | Nuha Alruwais, Hayam Alamro, Majdy M. Eltahir, Ahmed S. Salama, Mohammed Assiri, Noura Abdelaziz Ahmed . Modified arithmetic optimization algorithm with Deep Learning based data analytics for depression detection. AIMS Mathematics, 2023, 8(12): 30335-30352. doi: 10.3934/math.20231549 |
[10] | Mashael M Asiri, Abdelwahed Motwakel, Suhanda Drar . Robust sign language detection for hearing disabled persons by Improved Coyote Optimization Algorithm with deep learning. AIMS Mathematics, 2024, 9(6): 15911-15927. doi: 10.3934/math.2024769 |
Agricultural decision-making involves a complex process of choosing strategies and options to enhance resource utilization, overall productivity, and farming practices. Agricultural stakeholders and farmers regularly make decisions at various levels of the farm cycle, ranging from crop selection and planting to harvesting and marketing. In agriculture, where crop health has played a central role in economic and yield outcomes, incorporating deep learning (DL) techniques has developed as a transformative force for the decision-making process. DL techniques, with their capability to discern subtle variations and complex patterns in plant health, empower agricultural experts and farmers to make informed decisions based on data-driven, real-time insights. Thus, we presented a Bayesian optimizer with deep learning based pepper leaf disease detection for decision making (BODL-PLDDM) approach in the agricultural sector. The BODL-PLDDM technique aimed to identify the healthy and bacterial spot pepper leaf disease. Primarily, the BODL-PLDDM technique involved a Wiener filtering (WF) approach for pre-processing. Besides, the complex and intrinsic feature patterns could be extracted using the Inception v3 model. Also, the Bayesian optimization (BO) algorithm was used for the optimal hyperparameter selection process. For disease detection, a crayfish optimization algorithm (COA) with a long short-term memory (LSTM) method was employed to identify the precise presence of pepper leaf diseases. The experimentation validation of the BODL-PLDDM system was verified using the Plant Village dataset. The obtained outcomes underlined the promising detection results of the BODL-PLDDM technique over other existing methods.
Worldwide crop losses are predicted to be in the billion dollars that can be allotted for plant diseases [1]. Various agricultural diseases block the development of farming. Agriculturalists identify diseases via noticeable signs in plants. It must be more understood and corrected in most instances. It causes the unauthorized usage of plant chemicals and pesticides [2]. The catastrophic impact of plant disease can produce loss and yield in a few complex cases. Black pepper is a therapeutic plant. It is a significant central element in India, and various foods have several medical and health advantages [3]. Diseases like yellowing, quick wilt, slow wilt, foot rot, and phytophthora have impacted black pepper plants. It is a native spice of Kerala, Tamil Nadu, and a few of northeast India's forest states. Hence, there is a critical requirement to make an accurate, quick, and suitable technique for identifying pepper leaf diseases [4]. To resolve the issue above, traditional ML methods like random forest (RF), artificial neural networks (ANN), K-nearest neighbour (KNN), and support vector machine (SVM) have been extensively employed in the field of crop leaf disease identification [5]. Although present research is supportive, it has limitations like suboptimum performance (computational efficiency, inaccuracy) and shortage of generalization ability.
Furthermore, the method becomes more complex while handling large leaf disease datasets and several categories of diseases, making the proper utilization and application of the methods more challenging [6]. With the expansion of the artificial intelligence (AI) concept, deep learning (DL) has been developed to resolve complex visible tasks. In the domain of agriculture, various DL methods, namely Long Short-Term Memory (LSTM), Gated Recurrent Units (GRU), and Convolutional Neural Networks (CNNs), were examined for the identification of the indications of central diseases that will affect the crops [7]. As one of the most promising methods, CNN has been effectively employed in crop leaf disease identification. Numerous CNN methods have been strongly associated with identifying crop leaf diseases. Later, the DL method was constructed, frequently requiring it to be transferred into an external model [8]. Consequently, when considering the detection accuracy, the difficulty of training must have a high potential to build the system into a lightweight one. However, accomplishing a higher identification rate often needs to improve the network's capacity, resulting in the complexity of the training task [9]. In addition, diverse regularization techniques, optimizers, and activation functions have varied impacts under model training. Thus, continuously balancing the training complexity and network depth and electing the suitable activation function and optimization for the network model will be a complex problem [10].
We present a Bayesian optimizer with deep learning based pepper leaf disease detection for decision making (BODL-PLDDM) approach in the agricultural sector. The BODL-PLDDM technique aims to identify the presence of healthy and bacterial spot pepper leaf disease. Primarily, the BODL-PLDDM technique involves a Wiener filtering (WF) approach to pre-processing the pepper leaf images. Besides, the complex and intrinsic feature patterns can be extracted using the Inception v3 model. Followed by the Bayesian optimization (BO) algorithm is used for the optimal hyperparameter selection process. A crayfish optimization algorithm (COA) with a long short-term memory (LSTM) method is employed for disease detection, which correctly perceives the presence of pepper leaf diseases. The experimentation validation of the BODL-PLDDM model is verified utilizing the Plant Village dataset:
● An intelligent BODL-PLDDM technique comprising of pre-processing, Inception v3 feature extractor, BO based hyperparameter tuning, and COA with LSTM model for pepper leaf disease detection and classification is presented. To the best of our knowledge, the BODL-PLDDM model has never been presented in the literature.
● Employing the Inception v3 technique for feature extraction enables the capture of complex and intrinsic patterns from pepper leaf images, enabling more robust disease recognition.
● The implementation of the BO automates the process of choosing the optimal hyperparameters for model training, paving the way to enhanced performance and effectiveness in disease classification.
● Incorporating COA and LSTM methodologies presents an innovative model for disease recognition in agricultural settings.
● The COA-LSTM framework efficiently learns temporal reliabilities in image sequences, improving the accuracy and reliability of disease detection.
The remaining sections of the article are arranged as follows: Section 2 offers the literature review, and Section 3 represents the proposed method. Then, Section 4 elaborates on the results evaluation, and Section 5 completes the work.
In [11], an innovative DL-based technique was developed, which can be proficient in identifying healthy and diseased leaves through various crops, while the system will not be trained in them. To effectively classify and leverage the superiority of the Inception system during disease identification, the method utilizes a minor Inception system that must be appropriate for processing minimum fields without diminished performance. In [12], a solution was developed by employing a DL-based technique through the image data of plant leaves. A model has been deployed for DL that is dependent upon a currently established CNN employing a supervised learning technique for identifying and detecting diverse tomato diseases through the Inception Net architecture in this exploration. Two recent semantic segmentation techniques are Adapted U-Net and U-Net for the detection and segmentation. In [13], a concatenated NN of the mined features of AlexNet and VGG16 frameworks was presented, and a classification of the pepper disease method was developed employing fully connected (FC) layers. The expansion of the developed CNN method comprises stages like classification, noise removal, feature extraction, segmentation, image pre-processing, and dataset collection. In conclusion, the developed integrated CNN method has been assessed.
Begum and Syed [14] presented an innovative optimized DL method employing an efficient feature learning method that undergoes four main phases. The input images were primarily re-dimensioned and the ICLAHE method was presented. Subsequently, the Kernelized Gravity-based Density Clustering (KGDC) method was deployed for segmentation. A new osprey optimization algorithm (Os-OA) has also been given for tuning. Lee et al. [15] presented an enhanced crop disease diagnosis solution. The developed solution comprises two representative DL-based techniques like Object Recognition and Image Captioning. This system in the developed solution utilizes the Transformer system as a decoder and Inception v3 architecture as an encoder, whereas the Object Detection method of the developed solution utilizes the YOLOv5 framework. In [16], an ensemble stacked DL method was introduced to solve the issue of automated recognition of mango-leaf illnesses. Primarily, the images have been segmented in the region of interest (RoI) and input to many diverse DNN methods. The outcome of the DNN has been combined with an ML model for perceiving the leaf disease.
Ashwinkumar et al. [17] projected an automatic method for identifying and categorizing plant leaf diseases utilizing an optimum mobile network-based CNN (OMNCNN) approach. Moreover, the MobileNet method was implemented as the feature extraction algorithm wherein the hyperparameter has been enhanced through the emperor penguin optimization (EPO) method. In conclusion, an ELM-based technique was deployed for assigning suitable class labels. In [18], a Hierarchical DL-CNN (HDLCNN) was developed. Initially, a pre-processing stage was executed employing the Median Filtering (MF) technique. Next, an Intuitionistic Fuzzy Local Binary pattern (IFLBP) was presented, which can extract the features of the leaves. Afterward, the HDL-CNN was employed for identifying and categorizing the illness, and Decision Support Systems aided in executing proficient treatment schemes. Krishnamoorthy et al. [19] proposed a model utilizing InceptionResNetV2, a kind of CNN technique employed with a transfer learning approach to detect rice leaf image diseases. In [20], the application of VGG16, a CNN variant, is explored for the classification purpose of the infected and healthy leaves. The research also implements the Bayesian optimizing model for choosing optimal hyperparameters and examines the transfer learning model to improve the accomplishment and mitigate the training time.
We present a BODL-PLDDM approach to the agricultural sector. The technique's purpose is to identify the presence of healthy and bacterial spot pepper leaf disease. Figure 1 shows the work process of the presented BODL-PLDDM approach.
Primarily, the BODL-PLDDM technique involves the WF approach to preprocess the pepper leaf images. WF develops as a basis for pre-processing pepper leaf images, proposing a classy technique to moderate noise and improve image clarity [21]. In agricultural study, where precise image analysis is critical, WF offers a strong structure for removing significant data from noisy datasets.
By leveraging arithmetical models, WF efficiently separates noise and signal components, certifying the protection of vital details in pepper leaf imagery. This method optimally adjusts to the exclusive features of every image, allowing exact noise decrease while upholding reliability to the original content. Through spectral density assessment, WF builds an optimum filter, personalized to the precise noise profile of pepper leaf images, thus enabling enhanced image quality. Combining WF into the pre-processing pipeline allows researchers to attain more trustworthy and consistent outcomes in disease recognition and crop monitoring tasks.
The complex and intrinsic feature patterns can be extracted using the Inception v3 model in this work. IV3 is a form of CNN model developed for tasks including image identification and object classification [22]. This is presented as an enhanced form of the novel Inception framework, with higher practical usage and accuracy of computational resources. Assume, that X is the input image data that has the extent HxWxC, whereas W (breadth) and H (height), and C means the channels of images (for example, RGB images). The 1st layer of Inception v3 is a convolutional (Conv) layer with 32 filters, every size 3×3×3 (3×3 represents the filter size and 3 becomes the total quantity of input image channels). Consider F1 as the set of filters. The output is expressed below:
Z1=ReLU(Conv(X,F1)+b1). | (1) |
Here, b1 refers to the bias term, conv() describes the convolution process, and ReLu() represents rectified linear activation function. The following layer becomes a sequence of Inception components. An Inception component has a multi‐branch system that integrates the outputs of numerous Conv filters of various dimensions and diverse accessible domains. An Inception segment is an output of every branch. The mathematical equation for the Inception component will be denoted as given below:
z(inc)=ConCat([Conv(X,F1×1),Conv(X,F3×3,r),Conv(X,F5×5,r),Max−Pool(X,k),],axis=Channel), | (2) |
where the reduction parameters F1×1,F3×3,r, and F5×5,r define the set of filters with dimensions of 1×1,3×3 and 5×5, respectively.
The reduction parameter was utilized to decrease the amount of input channel to the 3×3 and 5×5 Conv, which will be the computational rate. k means the dimensions of the max pooling layer. The output of an Inception model will be provided via a batch normalization layer and ReLU can be given by
z(inc)=ReLU(Batch−Norm(Z(inc))). | (3) |
Next, the Inception components, the output is driven by applying a global average pooling layer for reducing the spatial sizes and then dual FC layers with 2048 neurons:
z(fc1)=ReLU((WFc1∗Global−Avg−Pool(Z(˙mc)))+bFc1), | (4) |
z(fc2)=ReLU((WFc2∗z(fc1))+bFc2), | (5) |
where WFc1andWFc2 represent the weight matrices that can be connected with the FC layers for the preceding layer, bFc1 andbFc2 describe the bias terms, and Global−Avg−Pool() denotes the function that averages the feature map through the spatial dimension. Then, the softmax layer output will be denoted as:
Y=SoftMax((WSM∗z(fc2))+bSM). | (6) |
WSM means the weight matrix connecting the softmax layer to the prior layer, and bSM represents the bias. The softmax standardizes the probability distribution output through the class labels. Figure 2 denotes the infrastructure of Inception v3.
The BO is used for the optimum hyperparameter selection process in this stage. BO is a sample effective plan for a worldwide optimizer of black boxes, which is costly and has multi‐external functions [23], and is usually controlled over a box‐bound searching space Ω:
minθϵΩg(θ). | (7) |
To resolve the problem of (1), BO utilizes dual major modules such as a probabilistic replacement method of an acquisition function nfill criterion or utility function, and the objective function g(θ) depends upon the present estimation of g(θ). The acquisition function's optimizer permits the choice of the subsequent capable θ' used to assess the objective function. The experimental value, g(θ') (or g(θ')+ϵ that the objective function is also loud), is next utilized to upgrade the probabilistic method resembling g(θ), and the procedure is repeated until assumed conclusion norms are attained (e.g., a maximal amount of function estimations).
A Gaussian Process (GP) is usually selected for the probabilistic replacement method. A substitute is produced by RF, an ensemble learning model which, opposite to GP, can handle complex search spaces Ω, covered by assorted, definite, and uncertain modules of (θ). Uncertain means that the value of solution vector θ[i] is based on the smallest modulevalueθ[j], with i≠j.
Its exact execution and the main goal of the probabilistic replacement technique is to offer an estimation of g(θ),∀θ∈Ω, beside by a scale of uncertainty regarding such estimation. These dual units are namely the standard deviation and mean of the forecast delivered by the probabilistic surrogate method signified by μ(θ) and σ(θ), respectively.
The acquisition function is intended to drive the collection of the following θ' to be assessed on the objective function, balancing among exploitation, that is selecting θ' whose related forecast is not inferior to the finest function value and exploration is picking θ' whose forecast is chiefly indefinite. The exploitation and exploration are related to local and global search, respectively. The primary and secondary are expressively compelled by μ(θ) and σ(θ),respectively. Many functions of acquisition have developed a summary is delivered. Each one provides a dissimilar device to stabilize the exploration and exploitation trade-off. The commonly utilized acquisition functions are lower confidence bound (LCB), maximal probability of improvement (MPI), and expected improvement (EI).
LCB controls both exploration and exploitation by positive in the aspect of uncertainty:
LCB(θ)=μ(θ)−ξσ(θ), | (8) |
where μ(x) denotes the mean value, σ(x) signifies the standard deviation. ξ≥0 refers to the parameter to control the trade-off between exploitation and exploration. Exactly, ξ=0 is for clean exploitation, and high values of ξ highlight exploration. A plan of ξ is planned with converge proof.
EI processes the probability of the development on g(θ) with esteem to an analytical distribution.
EI(θ)={(g(θ+)−μ(θ)−ξ)Φ(Z)+σ(θ)φ(Z) if σ(θ)>0,0 if σ(θ)=0. | (9) |
g(θ+) denotes the finest value, ξ is employed to stable among exploitation and exploration, φ(Z) and Φ(Z) refer to the prospect and increasing dispersal of the uniform normal, correspondingly by Z definite as below:
Z={g(θ+)−μ(θ)σ(θ) if σ(θ)>0,0 if σ(θ)=0. | (10) |
MPI is the primary acquisition function projected. As it is essentially inclined near exploitation, it is adapted with a parameter ξ to permit a superior exploitation and exploration trade-off:
MPI(θ)=P(g(θ)≤g(θ+)+ξ)=Φ(g(θ+)+μ(θ)+ξσ(θ)). | (11) |
At last, picking θ' needs to resolve an auxiliary optimizer problem on the similar searching space Ω but is more inexpensive than one, which minimizes LCB(θ) or maximizes EI(θ) or MPI(θ).
Signify by D1:n a set of initial solutions. For instance, experimented by employing the Latin Hypercube Sampling (LHS) model. The component Di is (θi,g(θi)), by i=1, n (i.e., we consider the set of noise‐free deprived of generalization loss. Besides, reflect N as the maximal number of function estimates.
The COA with LSTM model is applied for disease detection, which accurately detects the presence of pepper leaf diseases. The COA is a new optimization metaheuristics approach stimulated by crayfish's foraging, avoidance, and social behaviors [24]. Using three different operating stages, this technique influences principles from the biological field to address optimizer issues in other areas. This phase establishes an equilibrium of exploitation and exploration. Initially, COA concentrates on discovering promising solutions. Afterward, the "foraging" and "competition" phase mimic the exploitation stage. Transitions among both phases were impacted by temperature regulation. High temperature prompts crayfish to compete for shelter or search for shelter, whereas optimum temperature dictates foraging strategy based on food size. Temperature control bolsters the global optimization abilities and improves the randomness level. The subsequent equation describes the COA functioning:
X=[X1,X2,⋯,XN]=[X1,1⋯X1,j⋯X1,dim⋮⋯⋮⋯⋮Xi,1⋯Xi,j⋯Xi,dim⋮⋯⋮⋯⋮XN,1⋯XN,j⋯XN,dim]. | (12) |
Here, N is the population limit, P indicates the population, andk is the problem's dimensionality, Xi,j denotes the agent's location in ith and jth coordinates. An agent is arbitrarily disseminated through the search range:
Xi,j=lbj+(ubj−lbj)×rand, | (13) |
where ll and ul are the lower and upper limits and rnd denotes the random number.
temp=rand×15+20. | (14) |
When the temperature exceeds 30, agents select to find an excellent place to break and restart foraging at a suitable climate. Agent intakes are almost assumed to be uniformly distributed, and it is defined as follows:
p=C1×(1√2×π×σ)×exp(−(temp−μ)22σ2)). | (15) |
Equation (15), μ indicates the optimum agent temperature, and σ and C are the control parameters. Crayfish fight for cave space. This is based on the randomly generated event with a 0.5 probability of arising when the temperatures surpass 30 as:
Xt+1i,j=Xti,j−Xtz,j+Xshade, | (16) |
where z denotes a random agent. The position is adjusted according to other competing agents.
The agent position is updated as:
Xt+1i,j=Xti,j+Xfood×p×(cos(2×π×rand)−sin(2×π×rand)). | (17) |
COA progresses toward the best solution during the foraging stage, which bolsters the capability of the algorithm to exploit resources and ensure strong convergence ability.
The fitness function (FF) is the significant factor prompting the performance of COA. The hyperparameter range procedure includes the solution encoder technique to assess the efficacy of the candidate solution. In this work, the COA reveals that accuracy is the leading standard to project the FF conveyed below.
Fitness=max(P), | (18) |
P=TPTP+FP. | (19) |
Here, FP represents the false positive value and TP signifies the true positive.
LSTM is the elaborate variant of the RNN model that can be a recursive neural network method [25]. It is implanted for the modeling of successive data. The main feature of RNN is the network delay recursion that allows it to define the dynamic effectiveness of models. Nevertheless, it is generally complex to train RNNs to learn the extended dependences from time sequence data because of the blast and vanishing gradient complexity. Both difficulties have been produced by RNN, whose incline will be equivalent to the matrix of recurrent weight and then increased to a higher supremacy.
On the other hand, it was proved that the exploding gradient complexity is comparatively easy to handle by employing a method called gradient clipping, which merely decreases the gradients whose standards surpass a threshold. A significant benefit of the gradient clipping method is maintained as the gradient is smaller than a few levels for maximum learning time. In contrast, the learning or convergence could be affected once the gradient is decreased. Alternatively, the problems of the vanishing gradient are enormously complex due to the gradient's module in the trends concerning extended dependencies being smaller. Furthermore, it affects the gradient element in the trends, which provides short‐term dependencies to be more significant. Therefore, the RNN will absorb the short‐term dependencies simply; however, it undergoes extended dependencies.
The development of the LSTM creates efficient outcomes for competition in the vanishing gradient complexity of the RNN. This employs a memory cell proficient in signifying the continuing dependencies in sequential information. The LSTM memory cell includes 4 gates (or components): The self‐recurrent neuron, forget gate, output gate, and input gate. Such gates can be accountable for controlling the connections between various memory modules. Remarkably, the input gate manages whether the input signal will change the condition of the memory cell or not, while the output gate manages if it could alter the condition of the alternative memory unit. The forget gate must be selected to forget (or recollect) its prior status. The hidden output, gates, and cell status are signified as given below:
ft=σ(XtUf+St−1Wf+bf), | (20) |
it=σ(XtUi+St−1Wi+bi), | (21) |
ot=σ(XtUo+St−1Wo+bo), | (22) |
Ct=tanh(XtUc+St−1Wc+bc), | (23) |
Ct=Ct−1⊗ft⊕it⊗ Ct, | (24) |
St=ot⊗tanh(Ct). | (25) |
Here, Ct,St, andXt are cell states, hidden and input at time step t, individually, (Wf,Wj,Wo,Wc), (Uf,Uj,Uo,Uc),and (bf,bj,bo,bc) describe recurrent weights, input weights, and biases, correspondingly. St−1 and Ct−1 indicate the cell and hidden status at time step t−1, σ ⊗, and ⊕ denote sigmoid activation, pointwise multiplication, and pointwise addition, respectively.
It is verified that several efforts exist to address the vanishing gradient complexity of RNN, but the LSTM is a significant and effective effort. The LSTM resolves such an issue by shortening the gradients at the system, wherein it is inoffensive to perform, thereby applying the constant error flows via carousels with distinct multiplication components. Such particular non‐linear modules are acquired to alter the continual error flow.
The experimental validation of the BODL-PLDDM model is verified using the Plant Village dataset [26]. The dataset contains 2475 samples under two classes as demonstrated in Table 1. Figure 3 illustrates the sample images. The suggested technique is simulated using Python 3.6.5 tool on PC i5-8600k, 250GB SSD, GeForce 1050Ti 4GB, 16GB RAM, and 1TB HDD. The parameters settings are provided: Learning rate: 0.01, activation: ReLU, epoch count: 50, dropout: 0.5, and batch size: 5.
Classes | No. of Instances |
Bacterial Spot | 997 |
Healthy | 1478 |
Total Instances | 2475 |
Figure 4 demonstrates the confusion matrices formed by the BODL-PLDDM approach under 80:20 and 70:30 of TRAS/TSES. The results indicate that the BODL-PLDDM approach has effectual recognition in two classes: Bacterial spot and healthy.
Table 2 and Figure 5 show a leaf disease recognition analysis of the BODL-PLDDM approach under 80%TRAS and 20%TESS. The experimentation value concluded that the BODL-PLDDM methodology has recognized two classes under bacterial spot and healthy. With 80%TRAS, the BODL-PLDDM method gains an average accuy of 98.48%, precn of 98.50%, recal of 98.42%, F1score of 98.42%, and MCC of 96.84%. In addition, with 20%TESS, the BODL-PLDDM system gains an average accuy of 99.60%, precn of 99.52%, recal of 99.65%, F1score of 99.58%, and MCC of 99.17%.
Classes | Accuy | Precn | Recal | F1Score | MCC |
TRAS (80%) | |||||
Bacterial Spot | 98.48 | 98.60 | 97.60 | 98.09 | 96.84 |
Healthy | 98.48 | 98.41 | 99.07 | 98.74 | 96.84 |
Average | 98.48 | 98.50 | 98.34 | 98.42 | 96.84 |
TESS (20%) | |||||
Bacterial Spot | 99.60 | 99.04 | 100.00 | 99.52 | 99.17 |
Healthy | 99.60 | 100.00 | 99.31 | 99.65 | 99.17 |
Average | 99.60 | 99.52 | 99.65 | 99.58 | 99.17 |
The performance of the BODL-PLDDM system is graphically offered in Figure 6 in the method of training accuracy (TRAA) and validation accuracy (VALA) curves below 80%TRAS and 20%TESS. The figure shows a beneficial interpretation into the behaviour of the BODL-PLDDM technique over many epoch counts, signifying its learning procedure and generalization skills. Remarkably, the figure infers a steady improvement in the TRAA and VALA with a growth in epochs. It certifies the adaptive nature of the BODL-PLDDM technique in the pattern detection procedure on both TRA and TES data. The emerging trend in VALA summarizes the BODL-PLDDM technique's ability to adjust to the TRA data and also provides exact classification of unseen data, pointing out the robust generalization abilities.
Figure 7 demonstrates a broad representation of the training loss (TRLA) and validation loss (VALL) outcomes of the BODL-PLDDM system over different epochs below 80%TRAS and 20%TESS. The progressive reduction in TRLA highlights the BODL-PLDDM system enhancing the weights and minimalizing the classification error on the TRA and TES data. The figure specifies a clean understanding into the BODL-PLDDM model's association with the TRA data, emphasizing its ability to take patterns within both datasets. Remarkably, the BODL-PLDDM technique continually enhances its parameters in decreasing the changes among the forecast and actual TRA class labels.
The results of inspecting the precision recall (PR) curve, as shown in Figure 8, confirmed that the BODL-PLDDM system gradually achieves improved PR values over every class below 80%TRAS and 20%TESS. It confirms the boosted capabilities of the BODL-PLDDM technique in the classification of dissimilar classes, displaying the ability to recognize courses.
Also, in Figure 9, ROC curves formed by the BODL-PLDDM system outperformed in classifying discrete labels under 80%TRAS and 20%TESS. It offers comprehensive understanding of the tradeoff among TPR and FRP over distinct detection threshold values and epoch counts. The figure underlined the improved classifier outcomes of the BODL-PLDDM approach under all classes, outlining the efficiency in addressing frequent classification issues.
Table 3 and Figure 10 show a leaf disease recognition analysis of BODL-PLDDM method below 70%TRAS and 30%TESS. The experimental value inferred that the BODL-PLDDM system has recognition dual classes below bacterial spot and healthy. With 70 %TRAS, the BODL-PLDDM system gains an average accuy of 99.21%, precn of 99.35%, recal of 99.21%, F1score of 99.28%, and MCC of 98.56%. Furthermore, with 30%TESS, the BODL-PLDDM technique gain average accuy of 98.64%, precn of 98.82%, recal of 98.64%, F1score of 98.73%, and MCC of 97.46%.
Classes | Accuy | Precn | Recal | F1Score | MCC |
TRAS (70%) | |||||
Bacterial Spot | 98.72 | 99.57 | 98.72 | 99.14 | 98.56 |
Healthy | 99.71 | 99.13 | 99.71 | 99.42 | 98.56 |
Average | 99.21 | 99.35 | 99.21 | 99.28 | 98.56 |
TESS (30%) | |||||
Bacterial Spot | 97.95 | 98.97 | 97.95 | 98.46 | 97.46 |
Healthy | 99.33 | 98.68 | 99.33 | 99.00 | 97.46 |
Average | 98.64 | 98.82 | 98.64 | 98.73 | 97.46 |
The performance of the BODL-PLDDM method is graphically offered in Figure 11 in the procedure of TRAA and VALA curves below 70%TRAS and 30%TESS. The figure displays valuable clarification into the behavior of the BODL-PLDDM system over numerous epoch counts, indicating its learning procedure and generalization skills. Remarkably, the figure infers a steady improvement in the TRAA and VALA with a progress in epochs. It safeguards the adaptive nature of the BODL-PLDDM technique in the pattern detection procedure on both TRA and TES data. The rising trend in VALA outlines the BODL-PLDDM system's ability to adjust to the TRA data. Also, it shines in proposing accurate identification of unseen data, indicating the strong generalization capacities.
Figure 12 reveals a comprehensive representation of the TRLA and VALL outcomes of the BODL-PLDDM system over different epochs under 70%TRAS and 30%TESS. The advanced reduction in TRLA highlights the BODL-PLDDM system enhancing the weights and diminishing the TRA and TES data classification error. The figure specifies a clean understanding into the BODL-PLDDM model's link with the TRA data, emphasizing its ability to take patterns within both datasets. Remarkably, the BODL-PLDDM technique repeatedly enhances its parameters in decreasing the variances between the forecast and actual TRA class labels.
Inspecting the PR curve revealed in Figure 13, the outcomes certified that the BODL-PLDDM method gradually accomplishes greater PR values over every class under 70%TRAS and 30%TESS. It confirms the improved skills of the BODL-PLDDM technique in classifying distinct classes, displaying the ability to detect class labels.
Furthermore, in Figure 14, ROC curves offered by the BODL-PLDDM model outperformed in identifying labels below 70%TRAS and 30%TESS. It comprehensively explains the tradeoff between TPR and FRP over different detection threshold values and epoch counts. The figure shows the superior classifier outcomes of the BODL-PLDDM technique below all classes, outlining the efficiency in addressing many classification problems.
Table 4 provides an overall comparative examination of the BODL-PLDDM approach [14]. Figure 15 represents the accuy analysis of the BODL-PLDDM approach with other recent algorithms. The simulation values implied that the BODL-PLDDM approach has efficient performances. Based on accuy, the BODL-PLDDM approach has a higher accuy of 99.60% while the VGG16, VGGNet, CNN, DL-IBPDD, RPLD-CV, and GSAtt-CMNetV3 PLDC approaches have lesser accuy of 99.00%, 96.78%, 99.00%, 99.30%, 99.10%, and 99.30%, respectively.
Technology | Accuy | Precn | Recal | F1Score |
VGG-16 | 99.00 | 99.00 | 99.00 | 99.00 |
VGGNet | 96.78 | 98.09 | 98.31 | 98.58 |
CNN | 99.00 | 97.63 | 97.65 | 98.17 |
DL-IBPDD | 99.30 | 97.26 | 97.66 | 97.77 |
RPLD-CV | 99.10 | 95.70 | 95.70 | 95.40 |
GSAtt-CMNetV3 PLDC | 99.30 | 98.73 | 99.03 | 99.00 |
BODL-PLDDM | 99.60 | 99.52 | 99.65 | 99.58 |
Figure 16 signifies the precn, recal, and F1score study of the BODL-PLDDM technique with other current algorithms. The simulation values implied that the BODL-PLDDM system has effective performance. Based on precn, the BODL-PLDDM methodology has developed precn of 99.52%, whereas the VGG16, VGGNet, CNN, DL-IBPDD, RPLD-CV, and GSAtt-CMNetV3 PLDC techniques have smaller precn of 99.00%, 98.09%, 97.63%, 97.26%, 95.70%, and 98.73%, respectively. Additionally, based on F1score, the BODL-PLDDM method has a higher F1score of 99.58% while the VGG16, VGGNet, CNN, DL-IBPDD, RPLD-CV, and GSAtt-CMNetV3 PLDC methodologies have reduced F1score of 99.00%, 98.58%, 98.17%, 97.77%, 95.40%, and 99.00%, respectively.
In this paper, a BODL-PLDDM technique is proposed for the agricultural sector. The BODL-PLDDM technique aims to identify the presence of healthy and bacterial spot pepper leaf disease. Primarily, the BODL-PLDDM technique involves the WF approach to preprocess the pepper leaf images. Moreover, the complex and intrinsic feature patterns can be extracted using the Inception v3 model. Following this, the BO algorithm is used for the optimal hyperparameter selection process. For disease detection, COA with an LSTM model is applied, which accurately detects the presence of pepper leaf diseases. The experimentation validation of the BODL-PLDDM model is verified using the Plant Village database. The obtained results underlined the promising detection results of the BODL-PLDDM technique over other existing models. The limitations of the BODL-PLDDM technique comprise potential threats in adjusting to larger datasets and generalizing across various environmental settings. Future works may be on incorporating more image augmenting model for improving the robustness, exploring ensemble learning models for enhanced accomplishment, and performing free trials for validating the technique's efficiency in real-time agricultural situations.
Asma A. Alhashmi: Conceptualization, Investigation, Data curation, Writing-original draft, Funding acquisition; Manal Abdullah Alohali: Conceptualization, Validation, Writing-original draft, Writing-review & editing, Project administration, Funding acquisition; Nazir Ahmad Ijaz: Software, Validation, Visualization; Alaa O. Khadidos: Methodology, Writing-original draft, Writing-review & editing; Omar Alghushairy: Writing-original draft; Ahmed Sayed: Writing-review & editing. All authors have read and approved the final version of the manuscript for publication.
The authors declare that they have not used Artificial Intelligence (AI) tools in the creation of this article.
The authors extend their appreciation to the Deanship of Research and Graduate Studies at King Khalid University for funding this work through large group Research Project under grant number (RGP2/51/45). Princess Nourah bint Abdulrahman University Researchers Supporting Project number (PNURSP2024R330), Princess Nourah bint Abdulrahman University, Riyadh, Saudi Arabia. The authors extend their appreciation to the Deanship of Scientific Research at Northern Border University, Arar, KSA for funding this research work through the project number "NBU-FPEJ-2024- 2913-02". This study is partially funded by the Future University in Egypt (FUE).
The authors declare that they have no conflict of interest.
This article does not contain any studies with human participants performed by any of the authors.
[1] | R. Sharma, K. Vinay, D. Bordoloi, Deep learning meets agriculture: A faster RCNN based approach to pepper leaf blight disease detection and multi-classification, In: 2023 4th International Conference for Emerging Technology (INCET), 2023. |
[2] | M. B. Devi, K. Amarendra, Machine learning-based application to detect pepper leaf diseases using histgradientboosting classifier with fused HOG and LBP features, In: Smart Technologies in Data Science and Communication: Proceedings of SMART-DSC, Singapore: Springer, 2021. |
[3] | T. H. H. Aldhyani, A. Hasan, R. J. Eunice, D. J. Hemanth, Leaf pathology detection in potato and pepper bell plant using convolutional neural networks, In: 2022 7th International Conference on Communication and Electronics Systems (ICCES), 2022. |
[4] | C. H. Kim, M. N. R. Samsuzzaman, K. Y. Lee, M. R. Ali, Deep learning-based identification of Pepper (Capsicum annuum L.) diseases: A review, Precis. Agric., 5 (2023), 68. |
[5] | A. S. Kini, K. V. Prema, S. N. Pai, State of the art deep learning implementation for multiclass classification of black pepper leaf diseases, 2023. https://doi.org/10.21203/rs.3.rs-3272019/v1 |
[6] | I. Haque, M. A. Islam, K. Roy, M. M. Rahaman, A. A. Shohan, I. Md Saiful, Classifying pepper disease based on transfer learning: A deep learning approach, In: 2022 International Conference on Applied Artificial Intelligence and Computing (ICAAIC), 2022. http://doi.org/10.1109/ICAAIC53929.2022.9793178 |
[7] | K. Andersson, M. S. Hoassain, Bell pepper leaf disease classification using convolutional neural network, In: Intelligent Computing & Optimization: Proceedings of the 5th International Conference on Intelligent Computing and Optimization 2022 (ICO2022), Springer Nature, 569 (2022). |
[8] | Y. Akhalifi, A. Subekti, Bell pepper leaf disease classification using fine-tuned transfer learning, J. Elektronikadan Telekomunikasi, 23 (2023), 55–61. |
[9] | P. Thakur, C. Anuradha, A. P. Singh, Plant disease detection of bell pepper plant using transfer learning over different models, In: 2021 8th International Conference on Signal Processing and Integrated Networks (SPIN), 2021,384–389. |
[10] | C. Y. Khew, Y. Q. Teow, E. T. Lau, S. S. Hwang, C. H. Bong, N. K. Lee, Evaluation of deep learning for image-based black pepper disease and nutrient deficiency classification, In: 2021 2nd International Conference on Artificial Intelligence and Data Sciences (AiDAS), 2021, 1–6. http://doi.org/10.1109/AiDAS53897.2021.9574346 |
[11] | I. Bouacida, B. Farou, L. Djakhdjakha, H. Seridi, M. Kurulay, Innovative deep learning approach for cross-crop plant disease detection: A generalized method for identifying unhealthy leaves, Inform. Process. Agricul., 2024. |
[12] | M. Shoaib, T. Hussain, B. Shah, I. Ullah, S. M. Shah, F. Ali, et al., Deep learning-based segmentation and classification of leaf images for detection of tomato plant disease, Front. Plant Sci., 13 (2022), 1031748. |
[13] | Y. A. Bezabih, A. O. Salau, B. M. Abuhayi, A. A. Mussa, A. M. Ayalew, CPD-CCNN: Classification of pepper disease using a concatenation of convolutional neural network models, Sci. Rep., 13 (2023), 15581. |
[14] | S. S. A. Begum, H. Syed, GSAtt-CMNetV3: Pepper leaf disease classification using osprey optimization, IEEE Access, 2024. |
[15] | D. I. Lee, J. H. Lee, S. H. Jang, S. J. Oh, I. C. Doo, Crop disease diagnosis with deep learning-based image captioning and object detection, Appl. Sci., 13 (2023), 3148. |
[16] | V. Gautam, R. K. Ranjan, P. Dahiya, A. Kumar, ESDNN: A novel ensembled stack deep neural network for mango leaf disease classification and detection, Multimed. Tools Appl., 83 (2024), 10989–11015. |
[17] | S. Ashwinkumar, S. Rajagopal, V. Manimaran, B. Jegajothi, Automated plant leaf disease detection and classification using optimal MobileNet based convolutional neural networks, Mater. Today Proceed., 51 (2022), 480–487. |
[18] | A. Kumar, V. K. Patel, Classification and identification of disease in potato leaf using hierarchical based deep learning convolutional neural network, Multimed. Tools Appl., 2023, 1–27. |
[19] | N. Krishnamoorthy, L. N. Prasad, C. P. Kumar, B. Subedi, H. B. Abraha, V. E. Sathishkumar, Rice leaf diseases prediction using deep neural networks with transfer learning, Environ. Res., 198 (2021), 111275. |
[20] |
M. Subramanian, N. P. Lv, S. VE, Hyperparameter optimization for transfer learning of VGG16 for disease identification in corn leaves using Bayesian optimization, Big Data, 10 (2022), 215–229. https://doi.org/10.1089/big.2021.021 doi: 10.1089/big.2021.021
![]() |
[21] |
J. V. Valls, D. Vivet, E. Chaumette, F. Vincent, P. Closas, Recursive linearly constrained Wiener filter for robust multi-channel signal processing, Signal Process., 167 (2020), 107291. https://doi.org/10.1016/j.sigpro.2019.107291 doi: 10.1016/j.sigpro.2019.107291
![]() |
[22] |
K. S. Rao, P. V. Terlapu, D. Jayaram, K. K. Raju, G. K. Kumar, R. Pemula, et al., Intelligent ultrasound imaging for enhanced breast cancer diagnosis: Ensemble transfer learning strategies, IEEE Access, 2024. http://doi.org/10.1109/ACCESS.2024.3358448 doi: 10.1109/ACCESS.2024.3358448
![]() |
[23] |
A. Riboni, N. Ghioldi, A. Candelieri, M. Borrotti, Bayesian optimization and deep learning for steering wheel angle prediction, Sci. Rep., 12 (2022), 8739. https://doi.org/10.1038/s41598-022-12509-6 doi: 10.1038/s41598-022-12509-6
![]() |
[24] |
A. Cuk, T. Bezdan, L. Jovanovic, M. Antonijevic, M. Stankovic, V. Simic, et al., Tuning attention based long-short term memory neural networks for Parkinson's disease detection using modified metaheuristics, Sci. Rep., 14 (2024), 4309. https://doi.org/10.1038/s41598-024-54680-y doi: 10.1038/s41598-024-54680-y
![]() |
[25] |
A. Sagheer, M. Kotb, Unsupervised pre-training of a deep LSTM-based stacked autoencoder for multivariate time series forecasting problems, Sci. Rep., 9 (2019), 19038. https://doi.org/10.1038/s41598-019-55320-6 doi: 10.1038/s41598-019-55320-6
![]() |
[26] | Plant Village dataset. Available from: https://www.kaggle.com/datasets/adilmubashirchaudhry/plant-village-dataset. |
1. | SANA ALAZWARI, SAMAH AL ZANIN, JAWHARA ALJABRI, AMANI A. ALNEIL, ENHANCING PEPPER LEAF DISEASE DETECTION USING DEEP TRANSFER LEARNING FOR SUSTAINABLE AGRICULTURAL SECTOR IN KSA, 2024, 32, 0218-348X, 10.1142/S0218348X25400286 |
Classes | No. of Instances |
Bacterial Spot | 997 |
Healthy | 1478 |
Total Instances | 2475 |
Classes | Accuy | Precn | Recal | F1Score | MCC |
TRAS (80%) | |||||
Bacterial Spot | 98.48 | 98.60 | 97.60 | 98.09 | 96.84 |
Healthy | 98.48 | 98.41 | 99.07 | 98.74 | 96.84 |
Average | 98.48 | 98.50 | 98.34 | 98.42 | 96.84 |
TESS (20%) | |||||
Bacterial Spot | 99.60 | 99.04 | 100.00 | 99.52 | 99.17 |
Healthy | 99.60 | 100.00 | 99.31 | 99.65 | 99.17 |
Average | 99.60 | 99.52 | 99.65 | 99.58 | 99.17 |
Classes | Accuy | Precn | Recal | F1Score | MCC |
TRAS (70%) | |||||
Bacterial Spot | 98.72 | 99.57 | 98.72 | 99.14 | 98.56 |
Healthy | 99.71 | 99.13 | 99.71 | 99.42 | 98.56 |
Average | 99.21 | 99.35 | 99.21 | 99.28 | 98.56 |
TESS (30%) | |||||
Bacterial Spot | 97.95 | 98.97 | 97.95 | 98.46 | 97.46 |
Healthy | 99.33 | 98.68 | 99.33 | 99.00 | 97.46 |
Average | 98.64 | 98.82 | 98.64 | 98.73 | 97.46 |
Technology | Accuy | Precn | Recal | F1Score |
VGG-16 | 99.00 | 99.00 | 99.00 | 99.00 |
VGGNet | 96.78 | 98.09 | 98.31 | 98.58 |
CNN | 99.00 | 97.63 | 97.65 | 98.17 |
DL-IBPDD | 99.30 | 97.26 | 97.66 | 97.77 |
RPLD-CV | 99.10 | 95.70 | 95.70 | 95.40 |
GSAtt-CMNetV3 PLDC | 99.30 | 98.73 | 99.03 | 99.00 |
BODL-PLDDM | 99.60 | 99.52 | 99.65 | 99.58 |
Classes | No. of Instances |
Bacterial Spot | 997 |
Healthy | 1478 |
Total Instances | 2475 |
Classes | Accuy | Precn | Recal | F1Score | MCC |
TRAS (80%) | |||||
Bacterial Spot | 98.48 | 98.60 | 97.60 | 98.09 | 96.84 |
Healthy | 98.48 | 98.41 | 99.07 | 98.74 | 96.84 |
Average | 98.48 | 98.50 | 98.34 | 98.42 | 96.84 |
TESS (20%) | |||||
Bacterial Spot | 99.60 | 99.04 | 100.00 | 99.52 | 99.17 |
Healthy | 99.60 | 100.00 | 99.31 | 99.65 | 99.17 |
Average | 99.60 | 99.52 | 99.65 | 99.58 | 99.17 |
Classes | Accuy | Precn | Recal | F1Score | MCC |
TRAS (70%) | |||||
Bacterial Spot | 98.72 | 99.57 | 98.72 | 99.14 | 98.56 |
Healthy | 99.71 | 99.13 | 99.71 | 99.42 | 98.56 |
Average | 99.21 | 99.35 | 99.21 | 99.28 | 98.56 |
TESS (30%) | |||||
Bacterial Spot | 97.95 | 98.97 | 97.95 | 98.46 | 97.46 |
Healthy | 99.33 | 98.68 | 99.33 | 99.00 | 97.46 |
Average | 98.64 | 98.82 | 98.64 | 98.73 | 97.46 |
Technology | Accuy | Precn | Recal | F1Score |
VGG-16 | 99.00 | 99.00 | 99.00 | 99.00 |
VGGNet | 96.78 | 98.09 | 98.31 | 98.58 |
CNN | 99.00 | 97.63 | 97.65 | 98.17 |
DL-IBPDD | 99.30 | 97.26 | 97.66 | 97.77 |
RPLD-CV | 99.10 | 95.70 | 95.70 | 95.40 |
GSAtt-CMNetV3 PLDC | 99.30 | 98.73 | 99.03 | 99.00 |
BODL-PLDDM | 99.60 | 99.52 | 99.65 | 99.58 |