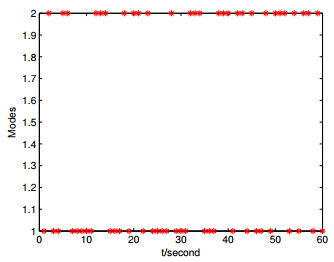
In this study, we establish a new inertial generalized viscosity approximation method and prove that the resulting sequence strongly converges to a common solution of a split generalized mixed equilibrium problem, fixed point problem for a finite family of nonexpansive mappings and hierarchical fixed point problem in real Hilbert spaces. As an application, we demonstrate the use of our main finding in compressed sensing in signal processing. Additionally, we include numerical examples to evaluate the efficiency of the suggested method and then conduct a comparative analysis of its efficiency with different methods. Our findings can be used in a variety of contexts to improve results.
Citation: Charu Batra, Renu Chugh, Mohammad Sajid, Nishu Gupta, Rajeev Kumar. Generalized viscosity approximation method for solving split generalized mixed equilibrium problem with application to compressed sensing[J]. AIMS Mathematics, 2024, 9(1): 1718-1754. doi: 10.3934/math.2024084
[1] | Yanting Xiao, Yifan Shi . Robust estimation for varying-coefficient partially nonlinear model with nonignorable missing response. AIMS Mathematics, 2023, 8(12): 29849-29871. doi: 10.3934/math.20231526 |
[2] | Heng Liu, Xia Cui . Adaptive estimation for spatially varying coefficient models. AIMS Mathematics, 2023, 8(6): 13923-13942. doi: 10.3934/math.2023713 |
[3] | Yanping Liu, Juliang Yin . B-spline estimation in varying coefficient models with correlated errors. AIMS Mathematics, 2022, 7(3): 3509-3523. doi: 10.3934/math.2022195 |
[4] | Anum Iftikhar, Hongbo Shi, Saddam Hussain, Ather Qayyum, M. El-Morshedy, Sanaa Al-Marzouki . Estimation of finite population mean in presence of maximum and minimum values under systematic sampling scheme. AIMS Mathematics, 2022, 7(6): 9825-9834. doi: 10.3934/math.2022547 |
[5] | Sanaa Al-Marzouki, Christophe Chesneau, Sohail Akhtar, Jamal Abdul Nasir, Sohaib Ahmad, Sardar Hussain, Farrukh Jamal, Mohammed Elgarhy, M. El-Morshedy . Estimation of finite population mean under PPS in presence of maximum and minimum values. AIMS Mathematics, 2021, 6(5): 5397-5409. doi: 10.3934/math.2021318 |
[6] | Jieqiong Lu, Peixin Zhao, Xiaoshuang Zhou . Orthogonality based modal empirical likelihood inferences for partially nonlinear models. AIMS Mathematics, 2024, 9(7): 18117-18133. doi: 10.3934/math.2024884 |
[7] | Zawar Hussain, Atif Akbar, Mohammed M. A. Almazah, A. Y. Al-Rezami, Fuad S. Al-Duais . Diagnostic power of some graphical methods in geometric regression model addressing cervical cancer data. AIMS Mathematics, 2024, 9(2): 4057-4075. doi: 10.3934/math.2024198 |
[8] | Peng Lai, Wenxin Tian, Yanqiu Zhou . Semi-supervised estimation for the varying coefficient regression model. AIMS Mathematics, 2024, 9(1): 55-72. doi: 10.3934/math.2024004 |
[9] | Dayang Dai, Dabuxilatu Wang . A generalized Liu-type estimator for logistic partial linear regression model with multicollinearity. AIMS Mathematics, 2023, 8(5): 11851-11874. doi: 10.3934/math.2023600 |
[10] | Abdullah Mohammed Alomair, Weineng Zhu, Usman Shahzad, Fawaz Khaled Alarfaj . Non-parametric calibration estimation of distribution function under stratified random sampling. AIMS Mathematics, 2025, 10(2): 4457-4472. doi: 10.3934/math.2025205 |
In this study, we establish a new inertial generalized viscosity approximation method and prove that the resulting sequence strongly converges to a common solution of a split generalized mixed equilibrium problem, fixed point problem for a finite family of nonexpansive mappings and hierarchical fixed point problem in real Hilbert spaces. As an application, we demonstrate the use of our main finding in compressed sensing in signal processing. Additionally, we include numerical examples to evaluate the efficiency of the suggested method and then conduct a comparative analysis of its efficiency with different methods. Our findings can be used in a variety of contexts to improve results.
Recently, neural networks such as the Hopfield neural network, cellular neural network, Cohen-Grossberg neural network and bidirectional associative neural network have attracted much attention due to their significant promise for many practical applications[1,2,3,4,5,6]. For example, in the field of signal processing, based on the theoretical results of discrete-time high-order switched neural networks synchronization, the encryption and decryption scheme of multi-channel audio signal design has good security [3]. In the field of fault diagnosis, by using deep parameter-free reconstruction-classification networks with parameter-free adaptively rectified linear units, the fault characteristics of vibration signals under the same fault state under different operating conditions can be better captured [5]. In the field of image processing, the theoretical results of random synchronization control framework based on semi-Markov switching quaternion-valued neural networks can be effectively applied to image encryption [6]. In particular, stability properties of the neural networks play a significant role in their design for solving practical problems. There are some different factors to influence the stability of neural networks. In particular, time delay is often unavoidable. Moreover, for the neural networks with time delay, there exists many techniques to reduce the conservatism of stability conditions, such as the improved bounding technique [7], free-weighting matrix theory [8], integral inequality technique [9] and so on.
It is well known that many dynamical systems may switch in different unpredictable modes, such as random failures [10]. As an effective tool, the Markovian jump process can be used to model these switching systems. Until now, there existed many literatures to investigate the stability, stabilization and observation for Markovian jump systems. For example, the authors investigated the adaptive sliding mode control problem of nonlinear Markovian jump systems with partly unknown transition probabilities, and obtained some conditions to guarantee the stochastic stability of the closed-loop system in [11]. The authors in [12] discussed the realization of H∞ finite-time control for a class of uncertain stochastic time-delay systems with unmeasured states through sliding mode control, and obtained some conditions to guarantee the system state was stabilized within a limited time interval. In [13], the authors established some passivity analysis criteria for Markovian jump singularly perturbed systems with partially unknown probabilities by using the hidden Markov model, and presented a unified controller design method to ensure the passivity of the system. Much more literatures can be found in [14,15,16].
In recent years, sliding mode control has become an effective robust control method because it is insensitive to model uncertainties, parameter variations and external disturbances. So, the sliding mode control has been used for lots of physical systems, such as robot manipulators, automotive engines and power systems. There are some existing results for linear or nonlinear systems[17,18,19,20,21,22]. For example, the authors in [17] investigated the adaptive sliding mode control issue for switched nonlinear systems with matched and mismatched uncertainties, designed the switched adaptive sliding mode control law and estimated the upper bound parameters of the matched uncertainty. The authors in [18] considered the synchronization of delayed chaotic neural networks with unknown disturbance via the observer-based sliding mode control, where the sliding surface involves an integral structure and a discontinuous controller.
In addition, synchronization has received considerable attention from various research fields, such as secure communication [3], engineering [23] and chemistry [24]. Synchronization phenomenons can be observed in many real systems such as neural systems, lasers and electronic circuits [25,26,27,28]. For example, the authors in [25] dealt with chaos synchronization for master-slave piecewise linear systems and provided some new sufficient conditions by using a Lyapunov approach and the so-called S-procedure. In [26], the authors designed a proportional-derivative (PD) controller for the master-slave synchronization of chaotic Lurie systems and presented a new synchronization criterion based on Lyapunov functions with a quadratic form of states and nonlinear functions of the systems. In [27], the authors studied the finite-time lag synchronization issue of master-slave complex networks with unknown signal propagation delays by the linear and adaptive error state feedback approaches and discovered that the setting time was related to initial values.
Motivated by the above discussion, we will consider the master-slave synchronization for uncertain neural networks with time-delay by using the sliding mode control method. The main contributions of our paper are as follows: (1) The master-slave synchronization for uncertain Markov jump neural networks with time-delay is considered by using the sliding mode control method, (2) the effect of time-delay and uncertainty for the neural networks stability are considered, and the uncertain parts in the neural networks only need be bounded other than any structure condition and (3) the provided sliding mode controller is very general.
The rest of this letter is organized as follows. In Section 2, the considered neural network model and some preliminaries are given. In Section 3, some sufficient conditions are proposed by the sliding mode control. In Section 4, a numerical example is provided to illustrate the effectiveness of the method proposed. In the last section, conclusions are presented.
Notation:
Rn: the n-dimensional Euclidean space; Rn×m: the set of all n×m real matrices; ||⋅||: the two-norm of a vector; ||⋅||1: its one-norm; In: the n order unit matrix; λmin(H): the minimum eigenvalues of matrix H; the notation X>Y, where X,Y are symmetric matrices, meaning that X−Y is a positive definite symmetric matrix. For a given matrix A∈Rn×n, AT denotes its transpose. sign(⋅) is the sign function. ∗ in a symmetric matrix denotes the symmetric terms.
Let (Ω,F,Ft,P) be a probability space related to an increasing family {Ft}t≥0 of the σ-algebras Ft⊂F, where Ω is the sample space. F is the σ-algebras of the sample space and P is the probability measure defined on F.
Consider the uncertain time delay neural networks with the Markovian jump defined on the probability space (Ω,F,Ft,P) as follows:
M:{˙xm(t)=−A(r(t))xm(t)+[B(r(t))+ΔB(r(t))]f(xm(t))+[C(r(t))+ΔC(r(t))]g(xm(t−d(t)))+J,xm(t)=φ(t),t∈[−d,0], | (2.1) |
where xm(t)=(xm1(t),xm2(t),...,xmn(t))T∈Rn is the state of neuron networks and A(r(t))∈Rn×n and B(r(t))∈Rn×m and C(r(t))∈Rn×m are coefficient matrices. ΔB(r(t)) and ΔC(r(t))∈Rn×m denote the system's uncertain parts and satisfy
[ΔB(r(t))ΔC(r(t))]=M(r(t))W(t)[N1(r(t))N2(r(t))], |
where matrix W(t) satisfies WT(t)W(t)≤I, M(r(t)),N1(r(t)),N2(r(t)) are some known matrices with appropriate dimensions. d(t) represents time delay and satisfies 0≤d(t)≤d and ˙d(t)≤μ≤1. f(xm(t)) and g(xm(t−d(t))) are the neuron activation functions, and J∈Rn is a constant vector. {r(t),t≥0} is a finite state Markov jumping process and represents the switching process among different modes, which takes values in a state space L={1,2,...,l}, and l is the number of modes. Let ∏=[πij]l×l denote the transition rate matrix, where the mode transition probabilities are
Pr{r(t+Δt)=j|r(t)=i}={πijΔt+o(Δt),i≠j,1+πiiΔt+o(Δt),i=j, | (2.2) |
where Δt>0 and limΔt→0o(Δt)Δt=0, πij satisfies πij>0 with i≠j and πii=−∑lj=1,j≠iπij for each mode i.
In order to be notional convenience, for the i-th mode, system (2.1) can be rewritten as
{˙xm(t)=−Aixm(t)+(Bi+ΔBi)f(xm(t))+(Ci+ΔCi)g(xm(t−d(t)))+J,xm(t)=φ(t),t∈[−d,0]. | (2.3) |
Let (2.3) be the master system, then the slave system is
S:{˙xs(t)=−A(r(t))xs(t)+B(r(t))f(xs(t))+C(r(t))g(xs(t−d(t)))+J+u(t),xs(t)=ψ(t),t∈[−d,0]. | (2.4) |
Definition 1. [29] Master system (2.3) and slave system (2.4) are said to be asymptotic synchronization if
limt→∞||xm(t)−xs(t)||=0 |
for any initial conditions.
In this paper, our objective is to design a suit controller u(t) such that the master system (2.3) and slave system (2.4) are in synchronization by using the sliding mode control method.
To the end, writing the state error e(t)=xs(t)−xm(t), and the corresponding state error system can be described by
{˙e(t)=−Aie(t)+BiF(e(t))+CiG(e(t−d(t)))−ΔBif(xm(t))−ΔCig(xm(t−d(t)))+u(t),e(t)=ψ(t)−φ(t),t∈[−d,0], | (2.5) |
where F(e(t))=f(xs(t))−f(xm(t)) and G(e(t−d(t)))=g(xs(t−d(t)))−g(xm(t−d(t))).
First, we take the sliding mode surface as
σi(t)=e(t)+∫t0[(Ai+Ki)e(θ)−BiF(e(θ))−CiG(e(θ−d(θ)))]dθ. | (2.6) |
Thus, the derivative of σi(t) is
˙σi(t)=˙e(t)+(Ai+Ki)e(t)−BiF(e(t))−CiG(e(t−d(t)))=Kie(t)−ΔBif(xm(t))−ΔCig(xm(t−d(t)))+u(t), | (2.7) |
where Ki(1≤i≤l) are some unknown matrices to be determined later. When the state trajectories reach the sliding mode surface, then ˙σi(t)=0 and σi(t)=0. So, we obtain the equivalent controller
ueq(t)=−Kie(t)+ΔBif(xm(t))+ΔCig(xm(t−d(t))). | (2.8) |
Substituting (2.8) into (2.5), we have
{˙e(t)=−(Ai+Ki)e(t)+BiF(e(t))+CiG(e(t−d(t))),e(t)=ψ(t)−φ(t),t∈[−d,0]. | (2.9) |
In this paper, the following assumptions for the neuron activation functions are needed.
Assumption 1. [30] Assume that each component of the nonlinear function f(⋅) and g(⋅) are continuous and bounded and satisfy
η−k≤fk(z1)−fk(z2)z1−z2≤η+k, |
θ−k≤gk(z1)−gk(z2)z1−z2≤θ+k,∀k=1,2,...,n, |
for any z1,z2∈R, where η−k>0,η+k>0,θ−k>0,θ+k>0 are some known positive constants.
From Assumption 1, it is easy to get the following inequalities
[eT(t)FT(e(t))][Θ1HΘ2H∗H][e(t)F(e(t))]≤0 | (2.10) |
and
[eT(t)GT(e(t−d(t)))][Θ3HΘ4H∗H][e(t)G(e(t−d(t)))]≤0, | (2.11) |
where H=diag{h1,h2,...,hn} is a positive definite diagonal matrix,
Θ1=diag{η−1η+1,η−2η+2,...,η−nη+n},Θ2=diag{−η−1+η+12,−η−2+η+22,...,−η−n+η+n2}, |
Θ3=diag{θ−1θ+1,θ−2θ+2,...,θ−nθ+n},Θ4=diag{−θ−1+θ+12,−θ−2+θ+22,...,−θ−n+θ+n2}. |
Assumption 2.[31] Assume that each component of the nonlinear functions f(⋅) and g(⋅) are bounded, which means that there exists positive scalars Bf and Bg such that
||fk(⋅)||≤Bf,||gk(⋅)||≤Bg |
for k=1,2,...,n.
Remark 1. In fact, the activation functions of neural networks are usually bounded. For example, the Logistic Sigmoid function h1(x)={1+e−ax}−1 and the threshold value function
h2(x)={1,x≥0,−1,x<0, |
and so on.
Throughout the paper, we need the following lemmas.
Lemma 1.[32] For any positive definite symmetric matrix W∈Rn×n and scalar τ>0, there is
∫tt−τxT(s)dsW∫tt−τx(s)ds≤τ∫tt−τxT(s)Wx(s)ds. |
Lemma 2.[33] The linear matrix inequality
[S11S12ST12S22]<0 |
is equivalent to the following condition
S22<0,S11−S12S−122ST12<0, |
where S11 and S22 are symmetric matrices.
Now, we will analyze the synchronization condition and construct the sliding mode controller.
Theorem 1. Under Assumption 1, if there exists positive definite symmetric matrices Pi,Wi,Ri∈Rn×n such that
Φ1i=[Φ1i,1101dRiPiBi−Θ2HPiCi−Θ4H−ATiRi−KTiRi∗−(1−μ)Wi0000∗∗−1dRi000∗∗∗−H0BTiRi∗∗∗∗−HCTiRi∗∗∗∗∗−1dRi]<0 | (3.1) |
for i=1,2,...,l, then the master system (2.1) and slave system (2.4) are in synchronization, where
Φ1i,11=−PiAi−ATiPi−PiKi−KTiPi+l∑j=1,j≠iπij(Pj−Pi)+Wi−1dRi−Θ1H−Θ3H. |
Proof. Constructing the following Lyapunov function
V(t)=eT(t)Pie(t)+∫tt−d(t)eT(θ)Wie(θ)dθ+∫0−d∫tt+s˙eT(θ)Ri˙e(θ)dθds. |
The derivative of V(t) along with the trajectories of system (2.9) is
˙V(t)=2eT(t)Pi˙e(t)+eT(t)∑lj=1πijPje(t)+eT(t)Wie(t)−(1−˙d(t))eT(t−d(t))Wie(t−d(t))+d˙eT(t)Ri˙e(t)−∫tt−d˙eT(θ)Ri˙e(θ)dθ≤2eT(t)Pi[−(Ai+Ki)e(t)+BiF(e(t))+CiG(e(t−d(t)))]+eT(t)∑lj=1,j≠iπij(Pj−Pi)e(t)+eT(t)Wie(t)−(1−μ)eT(t−d(t))Wie(t−d(t))+d[−(Ai+Ki)e(t)+BiF(e(t))+CiG(e(t−d(t)))]TRi⋅[−(Ai+Ki)e(t)+BiF(e(t))+CiG(e(t−d(t)))]−1d[e(t)−e(t−d)]TRi[e(t)−e(t−d)]. |
Letting ξ(t)=(eT(t),eT(t−d(t)),eT(t−d),FT(e(t))andGT(e(t−d(t)))T, one yields
˙V(t)≤ξT(t)[Φ2i+dΨTRiΨ]ξ(t), | (3.2) |
where
Φ2i=[Φ2i,1101dRiPiBiPiCi∗−(1−μ)Wi000∗∗−1dRi00∗∗∗00∗∗∗∗0], |
Ψ=[−Ai−Ki00BiCi], |
Φ2i,11=−PiAi−ATiPi−PiKi−KTiPi+l∑j=1,j≠iπij(Pj−Pi)+Wi−1dRi. |
It is noted that
ξT(t)[Φ2i+dΨTRiΨ]ξ(t)−[eT(t)FT(e(t))][Θ1HΘ2H∗H][e(t)F(e(t))]−[eT(t)GT(e(t−d(t)))][Θ3HΘ4H∗H][e(t)G(e(t−d(t)))]=ξT(t)[Φ3i+dΨTRiΨ]ξ(t), | (3.3) |
where
Φ3i=[Φ1i,1101dRiPiBi−Θ2HPiCi−Θ4H∗−(1−μ)Wi000∗∗−1dRi00∗∗∗−H0∗∗∗∗−H]. |
By using Lemma 2, we know that Φ3i+dΨTRiΨ<0 is equivalent to Φ1i<0. Thus, one obtains ˙V(t)<0 and system (2.9) is asymptotically stable, which shows that the master system (2.1) and slave system (2.4) are in synchronization. The proof is completed.
Theorem 2. Under Assumption 2 and the action of controller
u(t)=−Kie(t)−ζ(t)sign(σi(t)), | (3.4) |
master system (2.1) and slave system (2.4) are in synchronization, where
ζ(t)=Bf⋅||Mi||⋅||N1i||+Bg⋅||Mi||⋅||N2i||+α, |
and α>0 is a positive scalar.
Proof. Constructing the following Lyapunov function
U(t)=12σTi(t)σi(t), |
then
˙U(t)=σTi(t)˙σi(t)=σTi(t)[Kie(t)−ΔBif(xm(t))−ΔCig(xm(t−d(t)))−Kie(t)−ζ(t)sign(σi(t))]≤||σi(t)||[||ΔBi||⋅||f(xm(t))||+||ΔCi||⋅||g(xm(t−d(t)))||]−ζ(t)||σi(t)||. | (3.5) |
Because of
||ΔBi||⋅||f(xm(t))||+||ΔCi||⋅||g(xm(t−d(t)))||≤||Mi||[Bf⋅||N1i||+Bg⋅||N2i||], |
then
˙U(t)≤−α||σi(t)||=−α√2U(t). |
Thus, the state trajectories can attain the sliding mode surface in the finite time interval [0,T∗], where T∗≤√2U(0)2α. The proof is completed.
Remark 2. Compared with the uncertain system in [15], we especially considered the effect of time delay. From Theorems 1 and 2, we see that the designed sliding mode controller can realize the master-slave synchronization of the Markov jump neural networks.
Remark 3. The control gain Ki can be obtained from matrix inequality (3.1). However, (3.1) is not a linear matrix inequality. In order to solve it, we can take Pi=Ri.
From Theorem 1, we can obtain the following useful corollary as r(t)=1, which means that system (2.1) only has one mode.
Corollary 1. Under Assumption 1, if there exist positive definite symmetric matrices P,W,R∈Rn×n such that
Φ1=[Φ1,1101dRPB−Θ2HPC−Θ4H−ATR−KTR∗−(1−μ)W0000∗∗−1dR000∗∗∗−H0BTR∗∗∗∗−HCTR∗∗∗∗∗−1dR]<0, | (3.6) |
then the master system
{˙xm(t)=−Axm(t)+(B+ΔB)f(xm(t))+(C+ΔC)g(xm(t−d(t)))+J,xm(t)=φ(t),t∈[−d,0] | (3.7) |
and slave system
{˙xs(t)=−Axs(t)+Bf(xs(t))+Cg(xs(t−d(t)))+J+u(t),xs(t)=ψ(t),t∈[−d,0] | (3.8) |
are in synchronization, where
Φ1,11=−PA−ATP−PK−KTP+W−1dR−Θ1H−Θ3H. |
Consider master system (2.1) and slave system (2.4) with the following parameters
A1=[42105−20−26],A2=[5120700−18],B1=[0.20.60.20.2−0.200.2−0.1−0.1], |
B2=[0.20.50.30.2−0.400.3−0.1−0.2],C1=[0.50.4−0.30.50.10.10.50.10.5],C2=[0.8−0.30.30.10.20.2−0.60.30.2], |
Π=[−221−1],M1=[−0.20.50.7],M2=[0.30.8−0.4], |
N11=[0.1−0.2−0.1],N12=[0.05−0.1−0.03], |
N21=[−0.40.10.3],N22=[−0.10.020.07],μ=0.5,d=2, |
f(x(t))=[tanh(0.5x1(t)),tanh(0.4x2(t)),tanh(0.6x3(t))]T, |
g(x(t))=[tanh(0.3x1(t)),tanh(0.4x2(t)),tanh(0.2x3(t))]T, |
J1=0.5I3,J2=0.3I3,Θ1=Θ3=I3,Θ2=Θ4=−I3,w(t)=sin(t). |
By using the linear matrix inequality (LMI) toolbox in the MATLAB, we obtain the following solutions of inequality (3.1):
P1=[23.4405−7.37377.0525−7.373765.8658−20.94927.0525−20.949240.9154],P2=[21.2128−6.29397.4587−6.293961.5282−19.50707.4587−19.507038.4827], |
W1=[10.4452−3.21871.4795−3.218724.3005−7.12561.4795−7.125615.5240],W2=[5.7913−1.22002.4543−1.220016.4749−4.94762.4543−4.947610.7346], |
K1=[0.13660.0093−0.01880.00930.05500.0266−0.01880.02660.0902],K2=[0.13660.0093−0.01880.00930.05500.0266−0.01880.02660.0902], |
H=[76.637400059.824500045.2654]. |
For the initial values xm(0)=(−25,5,25)T and xs(0)=(30,−5,−25)T, Figure 1 is the Markovian jump process in different modes. Figure 2 is the state trajectories of error system (2.5), which shows that the error system is convergent and the master system synchronizes with the slave system. Figure 3 is the curve of the sliding mode surface, which shows that the state trajectories can arrive at the surface in a finite time interval.
In this paper, the master-slave synchronization for uncertain neural networks with time delay by using the sliding mode control method has been studied. An integral sliding mode surface and sliding mode controller was designed. Moreover, the state trajectories of the neural networks can reach the sliding mode surface in finite time under the action of the controller. Sufficient conditions in terms of linear matrix inequalities were presented to guarantee the neural networks asymptotical stability. Finally, an example was provided to illustrate the validity of the proposed design method. In the future, we will consider how to solve some physical problems by applying the obtained theoretical results.
The authors declare they have not used Artificial Intelligence (AI) tools in the creation of this article.
This work is supported by the National Natural Science Foundation (NNSF) of China under Grant 11602134.
The authors declare that they have no conflicts of interest.
[1] |
Y. Censor, T. Bortfeld, B. Martin, A. Trofimov, A unified approach for inversion problems in intensity-modulated radiation therapy, Phys. Med. Biol., 51 (2006), 2353–2365. https://doi.org/10.1088/0031-9155/51/10/001 doi: 10.1088/0031-9155/51/10/001
![]() |
[2] |
Y. Censor, A. Segal, The split common fixed point problem for directed operators, J. Convex Anal., 26 (2010), 55007. https://doi.org/10.1088/0266-5611/26/5/055007 doi: 10.1088/0266-5611/26/5/055007
![]() |
[3] | K. Fan, A minimax inequality and applications, In: Inequality III, New York: Academic Press, 1972,103–113. |
[4] | E. Blum, W. Oettli, From optimization and variational inequalities to equilibrium problems, Mathematics Student, 63 (1994), 123–145. |
[5] |
S. Suantai, S. Kesornprom, P. Cholamjiak, A new hybrid CQ algorithm for the split feasibility problem in Hilbert spaces and its applications to compressed sensing, Mathematics, 7 (2019), 789. https://doi.org/10.3390/math7090789 doi: 10.3390/math7090789
![]() |
[6] |
R. Chugh, R. Kumar, C. Batra, A novel inertial Tseng's method for solving generalized variational inequality problem, J. Appl. Math. Comput., 2023 (2023), 1–27. https://doi.org/10.1007/s12190-023-01942-z doi: 10.1007/s12190-023-01942-z
![]() |
[7] |
J. W. Peng, J. C. Yao, A new hybrid-extragradient method for generalized mixed equilibrium problems, fixed point problems and variational inequality problems, Taiwanese J. Math., 12 (2008), 1401–1432. https://doi.org/10.11650/twjm/1500405033 doi: 10.11650/twjm/1500405033
![]() |
[8] | S. Chaiyasil, S. Suantai, Aproximation method for generalized mixed equilibrium problems and fixed point problems for a countable family of nonexpansive mappings, Journal of Nonlinear Analysis and Optimization: Theory and Applications, 2 (2011), 337–353. |
[9] |
I. Inchan, Extragradient method for generalized mixed equilibrium problems and fixed point problems of finite family of nonexpansive mapping, Appl. Math. Comput., 219 (2012), 2949–2959. https://doi.org/10.1016/j.amc.2012.09.020 doi: 10.1016/j.amc.2012.09.020
![]() |
[10] |
F. U. Ogbuisi, O. T. Mewomo, On split generalised mixed equilibrium problems and fixed-point problems with no prior knowledge of operator norm, J. Fixed Point Theory Appl., 19 (2017), 2109–2128. https://doi.org/10.1007/s11784-016-0397-6 doi: 10.1007/s11784-016-0397-6
![]() |
[11] | O. K. Oyewole, O. T. Mewomo, A new inertial-projection method for solving split generalized mixed equilibrium and hierarchical fixed point problems, Kragujev. J. Math., 48 (2024), 199–223. |
[12] |
A. Moudafi, P. E. Mainge, Towards viscosity approximations of hierarchical fixed point problems, Fixed Point Theory Appl., 2006 (2006), 95453. https://doi.org/10.1155/FPTA/2006/95453 doi: 10.1155/FPTA/2006/95453
![]() |
[13] |
Y. Yao, Y. C. Liou, J. C. Yao, Iterative algorithms for the split variational inequality and fixed point problems under nonlinear transformations, J. Nonlinear Sci. Appl., 10 (2017), 843–854. https://doi.org/10.22436/jnsa.010.02.43 doi: 10.22436/jnsa.010.02.43
![]() |
[14] |
A. Kangtunyakarn, S. Suantai, A new mapping for finding common solutions of equilibrium problems and fixed point problems of finite family of nonexpansive mappings, Nonlinear Anal. Theor., 71 (2009), 4448–4460. https://doi.org/10.1016/j.na.2009.03.003 doi: 10.1016/j.na.2009.03.003
![]() |
[15] |
Anjali, R. Chugh, C. Batra, Fixed point theorems of enriched Ciric's type and enriched Hardy-Rogers contractions, Numer. Algebr. Control, 2023 (2023), 022. https://doi.org/10.3934/naco.2023022 doi: 10.3934/naco.2023022
![]() |
[16] |
A. Gangwar, A. Tomar, M. Sajid, R. C. Dimri, Common fixed points and convergence results for α-Krasnosel'skii mappings, AIMS Mathematics, 8 (2023), 9911–9923. https://doi.org/10.3934/math.2023501 doi: 10.3934/math.2023501
![]() |
[17] |
C. Batra, N. Gupta, R. Chugh, R. Kumar, Generalized viscosity extragradient algorithm for pseudomonotone equilibrium and fixed point problems for finite family of demicontractive operators, J. Appl. Math. Comput., 68 (2022), 4195–4222. https://doi.org/10.1007/s12190-022-01699-x doi: 10.1007/s12190-022-01699-x
![]() |
[18] |
R. Chugh, N. Gupta, Strong convergence of new split general system of monotone variational inclusion problem, Appl. Anal., 2023 (2023), 2178423. https://doi.org/10.1080/00036811.2023.2178423 doi: 10.1080/00036811.2023.2178423
![]() |
[19] |
H. ur Rehman, W. Kumam, K. Sombut, Inertial modification using self-adaptive subgradient extragradient techniques for equilibrium programming applied to variational inequalities and fixed-point problems, Mathematics, 10 (2022), 1751. https://doi.org/10.3390/math10101751 doi: 10.3390/math10101751
![]() |
[20] | C. Batra, R. Chugh, R. Kumar, Variational inequality problem with application to convex minimization problem, Mathematics in Engineering, Science and Aerospace, 14 (2023), 71–82. |
[21] |
N. Gupta, L. O. Jolaoso, A. Nandal, R. Chugh, Convergence analysis of multiple-sets split equality common fixed point problem with applications, Numer. Algebr. Control, 2023 (2023), 012. https://doi.org/10.3934/naco.2023012 doi: 10.3934/naco.2023012
![]() |
[22] |
N. Gupta, M. Postolache, A. Nandal, R. Chugh, A cyclic iterative algorithm for multiple-sets split common fixed point problem of demicontractive mappings without prior knowledge of operator norm, Mathematics, 9 (2021), 372. https://doi.org/10.3390/math9040372 doi: 10.3390/math9040372
![]() |
[23] |
K. R. Kazmi, R. Ali, M. Furkan, Krasnosel'skii-Mann type iterative method for hierarchical fixed point problem and split mixed equilibrium problem, Numer. Algor., 77 (2018), 289–308. https://doi.org/10.1007/s11075-017-0316-y doi: 10.1007/s11075-017-0316-y
![]() |
[24] |
P. Majee, C. Nahak, A hybrid viscosity iterative method with averaged mappings for split equilibrium problems and fixed point problems, Numer. Algor., 74 (2017), 609–635. https://doi.org/10.1007/s11075-016-0164-1 doi: 10.1007/s11075-016-0164-1
![]() |
[25] |
P. Majee, C. Nahak, A modified iterative method for capturing a common solution of split generalized equilibrium problem and fixed point problem, Racsam. Rev. R. Acad. A., 112 (2018), 1327–1348. https://doi.org/10.1007/s13398-017-0428-1 doi: 10.1007/s13398-017-0428-1
![]() |
[26] |
J. K. Kim, P. Majee, Modified Krasnosel'skii-Mann iterative method for hierarchical fixed point problem and split mixed equilibrium problem, J. Inequal. Appl., 2020 (2020), 227. https://doi.org/10.1186/s13661–250-020-02493-8 doi: 10.1186/s13661–250-020-02493-8
![]() |
[27] |
M. Yazdi, S. H. Sababe, A viscosity approximation method for solving general system of variational inequalities, generalized mixed equilibrium problems and fixed point problems, Symmetry, 14 (2022), 1507. https://doi.org/10.3390/sym14081507 doi: 10.3390/sym14081507
![]() |
[28] |
K. Zhao, Solvability, approximation and stability of periodic boundary value problem for a nonlinear Hadamard fractional differential equation with p-Laplacian, Axioms, 12 (2023), 733. https://doi.org/10.3390/axioms12080733 doi: 10.3390/axioms12080733
![]() |
[29] |
K. Zhao, Generalized UH-stability of a nonlinear fractional coupling (p1,p2)-Laplacian system concerned with nonsingular Atangana-Baleanu fractional calculus, J. Inequal. Appl., 2023 (2023), 96. https://doi.org/10.1186/s13660-023-03010-3 doi: 10.1186/s13660-023-03010-3
![]() |
[30] |
K. Zhao, Stability of a nonlinear Langevin system of ML-type fractional derivative affected by time-varying delays and differential feedback control, Fractal Fract., 6 (2022), 725. https://doi.org/10.3390/fractalfract6120725 doi: 10.3390/fractalfract6120725
![]() |
[31] |
K. Zhao, Stability of a nonlinear fractional Langevin system with nonsingular exponential kernel and delay control, Discrete Dyn. Nat. Soc., 2022 (2022), 9169185. https://doi.org/10.1155/2022/9169185 doi: 10.1155/2022/9169185
![]() |
[32] | K. Zhao, Global asymptotic stability for a classical controlled nonlinear periodic commensalism AG-ecosystem with distributed lags on time scales, Filomat, 37 (2023), 9899–9911. |
[33] | R. Chugh, C. Batra, G. Biban, A. Ahuja, New four step iteration process for approximating fixed point of contraction mappings, Mathematics in Engineering, Science and Aerospace, 13 (2022), 1071–1087. |
[34] |
T. Jin, X. Yang, Monotonicity theorem for the uncertain fractional differential equation and application to uncertain financial market, Math. Comput. Simulat., 190 (2021), 203–221. https://doi.org/10.1016/j.matcom.2021.05.018 doi: 10.1016/j.matcom.2021.05.018
![]() |
[35] |
T. Jin, H. Xia, Lookback option pricing models based on the uncertain fractional-order differential equation with Caputo type, J. Ambient Intell. Human. Comput., 14 (2021), 6435–6448. https://doi.org/10.1007/s12652-021-03516-y doi: 10.1007/s12652-021-03516-y
![]() |
[36] |
B. T. Polyak, Some methods of speeding up the convergence of iterative methods, Comp. Math. Math. Phys. 4 (1964), 1–17. https://doi.org/10.1016/0041-5553(64)90137-5 doi: 10.1016/0041-5553(64)90137-5
![]() |
[37] |
P. Chuasuk, A. Kaewcharoen, Krasnosel'skii-Mann-type inertial method for solving split generalized mixed equilibrium and hierarchical fixed point problems, J. Inequal. Appl., 2021 (2021), 94. https://doi.org/10.1186/s13660-021-02632-9 doi: 10.1186/s13660-021-02632-9
![]() |
[38] |
G. C. Ugwunnadi, L. Y. Haruna, M. H. Harbau, Accelerated Krasnoselski-Mann type algorithm for hierarchical fixed point and split monotone variational inclusion problems in Hilbert spaces, Carpathian Math. Publ., 15 (2023), 158–179. https://doi.org/10.15330/cmp.15.1.158-179 doi: 10.15330/cmp.15.1.158-179
![]() |
[39] |
A. Moudafi, P. E. Mainge, Towards viscosity approximations of hierarchical fixed point problems, Fixed Point Theory Appl., 2006 (2006), 95453. https://doi.org/10.1155/FPTA/2006/95453 doi: 10.1155/FPTA/2006/95453
![]() |
[40] | K. Goebel, S. Reich, Uniform convexity, hyperbolic geometry, and nonexpansive mappings, New York: Dekker, 1983. |
[41] |
H. H. Bauschke, J. M. Borwein, On projection algorithms for solving convex feasibility problems, SIAM Rev., 38 (1996), 367–426. https://doi.org/10.1137/S0036144593251710 doi: 10.1137/S0036144593251710
![]() |
[42] |
M. Bianchi, S. Schaible, Generalized monotone bifunctions and equilibrium problems, J. Optimiz. Theory App., 90 (1996), 31–43. https://doi.org/10.1007/BF02192244 doi: 10.1007/BF02192244
![]() |
[43] |
H. U. Rehman, P. Kumam, Q. L. Dong, Y. Peng, W. Deebani, A new Popov' subgradient extragradient method for two classes of equilibrium programming in a real Hilbert space, Optimization, 70 (2021), 2675–2710. https://doi.org/10.1080/02331934.2020.1797026 doi: 10.1080/02331934.2020.1797026
![]() |
[44] |
H. A. Abass, C. Izuchukwu, O. T. Mewomo, Viscosity approximation method for modified split generalized equilibrium and fixed point problems, Rev. Union Mat. Argent., 61 (2020), 389–411. https://doi.org/10.33044/revuma.v61n2a13 doi: 10.33044/revuma.v61n2a13
![]() |
[45] |
G. Marino, H. K. Xu, A general iterative method for nonexpansive mapping in Hilbert spaces, J. Math. Anal. Appl., 318 (2006), 43–52. https://doi.org/10.1016/j.jmaa.2005.05.028 doi: 10.1016/j.jmaa.2005.05.028
![]() |
[46] |
B. V. Dinh, D. S. Kim, Projection algorithms for solving nonmonotone equilibrium problems in Hilbert space, J. Comput. Appl. Math., 302 (2016), 106–117. https://doi.org/10.1016/j.cam.2016.01.054 doi: 10.1016/j.cam.2016.01.054
![]() |
[47] |
A. Moudafi, Split monotone variational inclusions, J. Optim. Theory Appl., 150 (2011), 275–283. https://doi.org/10.1007/s10957-011-9814-6 doi: 10.1007/s10957-011-9814-6
![]() |
[48] | H. H. Bauschke, P. L. Combettes, Convex analysis and monotone operator theory in Hilbert space, Cham: Springer, 2017. https://doi.org/10.1007/978-3-319-48311-5 |
[49] |
H. Zhou, Convergence theorems of fixed points for k-strict pseudo-contractions in Hilbert spaces, Nonlinear Anal. Theor., 69 (2008), 456–462. https://doi.org/10.1016/j.na.2007.05.032 doi: 10.1016/j.na.2007.05.032
![]() |
[50] |
P. E. Maingè, Strong convergence of projected subgradient methods for nonsmooth and nonstrictly convex minimization, Set-Valued Analysis, 16 (2008), 899–912. https://doi.org/10.1007/s11228-008-0102-z doi: 10.1007/s11228-008-0102-z
![]() |
[51] |
S. Li, L. Li L. Cao, X. He, X. Yue, Hybrid extragradient method for generalized mixed equilibrium problem and fixed point problems in Hilbert space, Fixed Point Theory Appl., 2013 (2013), 240. https://doi.org/10.1186/1687-1812-2013-240 doi: 10.1186/1687-1812-2013-240
![]() |
[52] | P. L. Combettes, S. A. Hirstoaga, Equilibrium programming in Hilbert spaces, J. Nonlinear Convex A., 6 (2005), 117–136. |
[53] |
T. Suzuki, Strong convergence of Krasnosel'skiii and Mann's type sequences for one parameter nonexpansive semigroups without Bochner integrals, J. Math. Anal. Appl., 305 (2005), 227–239. https://doi.org/10.1016/j.jmaa.2004.11.017 doi: 10.1016/j.jmaa.2004.11.017
![]() |
[54] |
A. Kangtunyakar, S. Suantai, A new mapping for finding common solutions of equilibrium problems and fixed point problems of finite family of nonexpansive mappings, Nonlinear Anal. Theor., 71 (2009), 4448–4460. https://doi.org/10.1016/j.na.2009.03.003 doi: 10.1016/j.na.2009.03.003
![]() |
[55] | K. Goebel, W. A. Kirk, Topics in metric fixed point theory, Cambridge: Cambridge University Press, 1990. https://doi.org/10.1017/CBO9780511526152 |
[56] |
S. H. Rizvi, A strong convergence theorem for split mixed equilibrium and fixed point problems for nonexpansive mappings, J. Fixed Point Theory Appl., 20 (2018), 8. https://doi.org/10.1007/s11784-018-0487-8 doi: 10.1007/s11784-018-0487-8
![]() |
[57] |
S. Husain, N. Singh, A hybrid iterative algorithm for a split mixed equilibrium problem and a hierarchical fixed point problem, Appl. Set-Valued Anal. Optim., 1 (2019), 149–169. https://doi.org/10.23952/asvao.1.2019.2.05 doi: 10.23952/asvao.1.2019.2.05
![]() |
[58] |
R. Tibshirani, Regression shrinkage and selection via the Lasso, J. R. Stat. Soc. B, 58 (1996), 267–288. https://doi.org/10.1111/j.2517-6161.1996.tb02080.x doi: 10.1111/j.2517-6161.1996.tb02080.x
![]() |