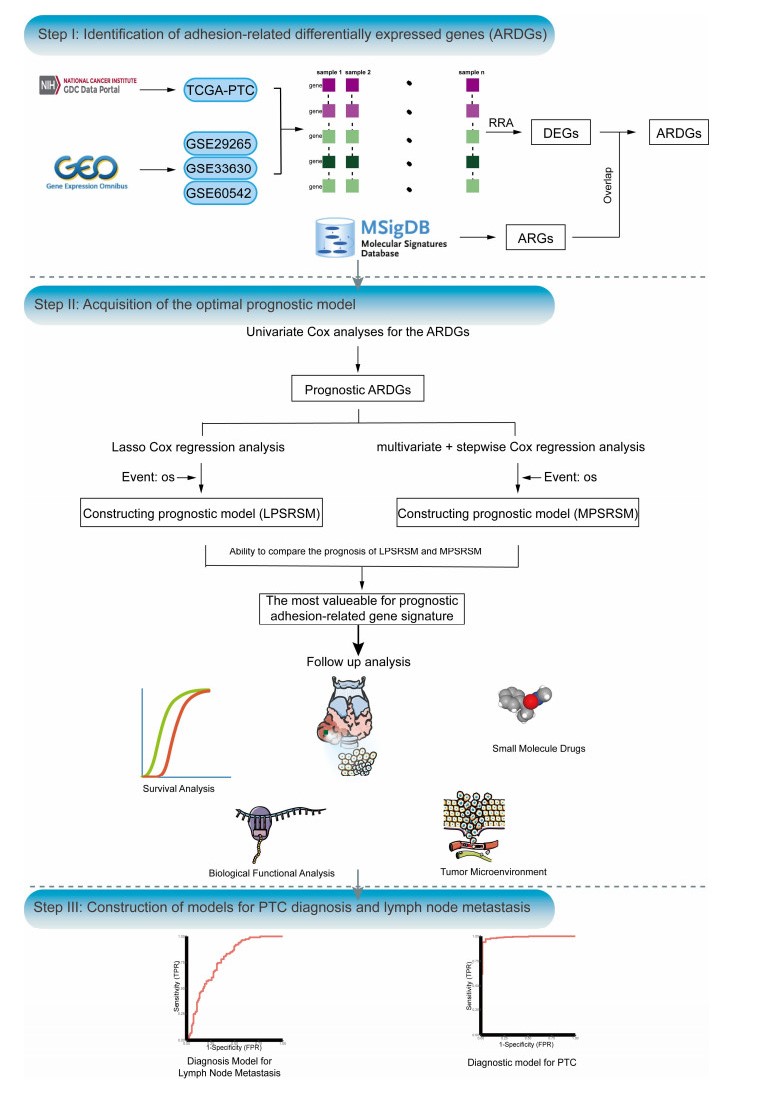
The association between adhesion function and papillary thyroid carcinoma (PTC) is increasingly recognized; however, the precise role of adhesion function in the pathogenesis and prognosis of PTC remains unclear. In this study, we employed the robust rank aggregation algorithm to identify 64 stable adhesion-related differentially expressed genes (ARDGs). Subsequently, using univariate Cox regression analysis, we identified 16 prognostic ARDGs. To construct PTC survival risk scoring models, we employed Lasso Cox and multivariate + stepwise Cox regression methods. Comparative analysis of these models revealed that the Lasso Cox regression model (LPSRSM) displayed superior performance. Further analyses identified age and LPSRSM as independent prognostic factors for PTC. Notably, patients classified as low-risk by LPSRSM exhibited significantly better prognosis, as demonstrated by Kaplan-Meier survival analyses. Additionally, we investigated the potential impact of adhesion feature on energy metabolism and inflammatory responses. Furthermore, leveraging the CMAP database, we screened 10 drugs that may improve prognosis. Finally, using Lasso regression analysis, we identified four genes for a diagnostic model of lymph node metastasis and three genes for a diagnostic model of tumor. These gene models hold promise for prognosis and disease diagnosis in PTC.
Citation: Shuo Sun, Xiaoni Cai, Jinhai Shao, Guimei Zhang, Shan Liu, Hongsheng Wang. Machine learning-based approach for efficient prediction of diagnosis, prognosis and lymph node metastasis of papillary thyroid carcinoma using adhesion signature selection[J]. Mathematical Biosciences and Engineering, 2023, 20(12): 20599-20623. doi: 10.3934/mbe.2023911
[1] | Dijian Shen, Youhua Jiang, Kaiyi Tao . Expression and clinical significance of p75NTR in esophageal squamous cell carcinoma. Mathematical Biosciences and Engineering, 2019, 16(6): 8060-8068. doi: 10.3934/mbe.2019405 |
[2] | Balamurugan Pandiyan, Stephen J. Merrill . A model of the cost of delaying treatment of Hashimotos thyroiditis: thyroid cancer initiation and growth. Mathematical Biosciences and Engineering, 2019, 16(6): 8069-8091. doi: 10.3934/mbe.2019406 |
[3] | Tongmeng Jiang, Pan Jin, Guoxiu Huang, Shi-Cheng Li . The function of guanylate binding protein 3 (GBP3) in human cancers by pan-cancer bioinformatics. Mathematical Biosciences and Engineering, 2023, 20(5): 9511-9529. doi: 10.3934/mbe.2023418 |
[4] | Yujia Xi, Liying Song, Shuang Wang, Haonan Zhou, Jieying Ren, Ran Zhang, Feifan Fu, Qian Yang, Guosheng Duan, Jingqi Wang . Identification of basement membrane-related prognostic signature for predicting prognosis, immune response and potential drug prediction in papillary renal cell carcinoma. Mathematical Biosciences and Engineering, 2023, 20(6): 10694-10724. doi: 10.3934/mbe.2023474 |
[5] | Jie Qiu, Maolin Sun, Chuanshan Zang, Liwei Jiang, Zuorong Qin, Yan Sun, Mingbo Liu, Wenwei Zhang . Five genes involved in circular RNA-associated competitive endogenous RNA network correlates with metastasis in papillary thyroid carcinoma. Mathematical Biosciences and Engineering, 2021, 18(6): 9016-9032. doi: 10.3934/mbe.2021444 |
[6] | Chenyang Jiang, Weidong Jiang . AGTR1, PLTP, and SCG2 associated with immune genes and immune cell infiltration in calcific aortic valve stenosis: analysis from integrated bioinformatics and machine learning. Mathematical Biosciences and Engineering, 2022, 19(4): 3787-3802. doi: 10.3934/mbe.2022174 |
[7] | Yong Luo, Qingbiao Chen, Jingbo Lin . Identification and validation of a tumor mutation burden-related signature combined with immune microenvironment infiltration in adrenocortical carcinoma. Mathematical Biosciences and Engineering, 2022, 19(7): 7055-7075. doi: 10.3934/mbe.2022333 |
[8] | Yu Jiang, Lijuan Lin, Huiming Lv, He Zhang, Lili Jiang, Fenfen Ma, Qiuyue Wang, Xue Ma, Shengjin Yu . Immune cell infiltration and immunotherapy in hepatocellular carcinoma. Mathematical Biosciences and Engineering, 2022, 19(7): 7178-7200. doi: 10.3934/mbe.2022339 |
[9] | Yan He, Nannan Cao, Yanan Tian, Xuelin Wang, Qiaohong Xiao, Xiaojuan Tang, Jiaolong Huang, Tingting Zhu, Chunhui Hu, Ying Zhang, Jie Deng, Han Yu, Peng Duan . Development and validation of two redox-related genes associated with prognosis and immune microenvironment in endometrial carcinoma. Mathematical Biosciences and Engineering, 2023, 20(6): 10339-10357. doi: 10.3934/mbe.2023453 |
[10] | Yuedan Wang, Jinke Huang, Jiaqi Zhang, Fengyun Wang, Xudong Tang . Identifying biomarkers associated with the diagnosis of ulcerative colitis via bioinformatics and machine learning. Mathematical Biosciences and Engineering, 2023, 20(6): 10741-10756. doi: 10.3934/mbe.2023476 |
The association between adhesion function and papillary thyroid carcinoma (PTC) is increasingly recognized; however, the precise role of adhesion function in the pathogenesis and prognosis of PTC remains unclear. In this study, we employed the robust rank aggregation algorithm to identify 64 stable adhesion-related differentially expressed genes (ARDGs). Subsequently, using univariate Cox regression analysis, we identified 16 prognostic ARDGs. To construct PTC survival risk scoring models, we employed Lasso Cox and multivariate + stepwise Cox regression methods. Comparative analysis of these models revealed that the Lasso Cox regression model (LPSRSM) displayed superior performance. Further analyses identified age and LPSRSM as independent prognostic factors for PTC. Notably, patients classified as low-risk by LPSRSM exhibited significantly better prognosis, as demonstrated by Kaplan-Meier survival analyses. Additionally, we investigated the potential impact of adhesion feature on energy metabolism and inflammatory responses. Furthermore, leveraging the CMAP database, we screened 10 drugs that may improve prognosis. Finally, using Lasso regression analysis, we identified four genes for a diagnostic model of lymph node metastasis and three genes for a diagnostic model of tumor. These gene models hold promise for prognosis and disease diagnosis in PTC.
Abbreviations: ARDGs: adhesion-related differential expression genes; ARGs: adhesion-related genes; AUC: area under the curve; BP: biological processes; CC: cellular components; DEGs: differential expression genes; GO: gene ontology; GSVA: gene set variation analysis; HR: hazard ratio; KEGG: kyoto encyclopedia of genes and genomes; KM: kaplan-meier; LPSRSM: survival risk scoring model based on Lasso Cox regression analysis; MF: molecular functions; MPSRSM: survival risk scoring model based on multivariate Cox regression analysis; OS: overall survival; PTC: papillary thyroid carcinoma; ROC: receiver operating characteristic curve; RRA: robust rank aggregation; ssGSEA: single sample gene set enrichment analysis; TCGA: the Cancer Genome Atlas.
Thyroid cancer is a common endocrine tumor, with papillary thyroid carcinoma (PTC) being the most prevalent pathological type originating from the thyroid follicular epithelial cells, accounting for approximately 80 to 90% of all thyroid cancers [1]. Despite the well-differentiated nature and favorable prognosis of PTC tissue, 15 to 50% of PTC patients still experience neck lymph node metastasis, leading to a shortened survival period [2,3,4,5]. Lymph node metastasis is an important indicator of PTC severity and a risk factor for PTC recurrence and survival risk. It is the most common way for tumor metastasis, involving complex and diverse molecular mechanisms that entail interactions among multiple molecules and pathways. Increasing evidence suggests that adhesion molecules play a crucial role in tumor formation, basement membrane invasion, regional lymph node metastasis, and distant organ metastasis in PTC [6,7]. Previous studies have reported the involvement of adhesion molecules in PTC lymph node metastasis and extrathyroidal invasion, which profoundly alters the balance between adhesion molecules and the basement membrane [8]. However, the potential pathogenic mechanisms of adhesion molecules in PTC are still unclear, and further research is needed to explore adhesion-related potential diagnostic biomarkers and therapeutic targets.
Considering the significant role of lymph node metastasis in PTC patients' prognoses, it is crucial to pre-assess lymph node metastasis before PTC treatment begins. Ultrasound examination is the optimal imaging tool for evaluating thyroid nodules and detecting abnormal cervical lymph nodes, although some small metastatic lesions may go undetected through imaging examinations. Therefore, there is an urgent need for novel and sensitive diagnostic methods to identify PTC and assess the presence of lymph node metastasis. Stratifying PTC patients based on lymph node metastasis status is conducive to managing and monitoring disease progression and prognosis.
Tumor development is mediated by genetic mutations; thus, tumors are referred to as genetic diseases. The complex and intertwined pathological mechanisms of tumors are the result of the interaction between gene mutations and the environment. Based on this, genomic information has been widely used in the precision treatment of cancer. In recent years, the rapid development of high-throughput sequencing technologies has enriched multiple omics databases, such as genomics and proteomics. Data mining has ignited enthusiasm in cancer research, prompting researchers to effectively and objectively analyze data by utilizing techniques like bioinformatics and computer science to extract relevant field-specific data. Machine learning is an emerging field derived from bioinformatics and computer science that possesses the ability to handle large, complex, and heterogeneous data simultaneously [9,10]. However, currently, there is a lack of machine learning methods in the prognosis and survival research for identifying adhesion molecule-mediated lymph node metastasis in PTC. In this study, we will employ various machine learning methods combined with adhesion-related gene features and identification methods for gene expression features to establish classifiers for disease diagnosis, lymph node metastasis, and survival prognosis. Additionally, we will further explore the role of novel biomarkers in the pathogenesis of PTC and search for potential candidate therapeutic intervention factors.
The design of this study is shown in Figure 1. Transcriptome sequencing data (495 PTC samples and 59 adjacent cancer samples) and clinical pathology data were downloaded from The Cancer Genome Atlas (TCGA) public database (data release version: v36.0). Inclusion criteria were based on the pathology type "thyroid papillary carcinoma". Samples lacking survival time information were excluded. The TCGA transcriptome data were standardized and normalized using the normalizeQuantiles and Log2 transformation functions in the "limma" R package [11]. Additionally, series matrix files for GSE29265 (20 PTC samples and 20 normal thyroid samples; version: Jun 01, 2012) [unpublished], GSE33630 (49 PTC samples and 45 normal thyroid samples; version: Nov 09, 2012) [12], and GSE60542 (33 PTC samples and 30 normal thyroid samples; version: Sep 01, 2015) [13] were retrieved from the Gene Expression Omnibus (GEO) database using the search terms "papillary thyroid carcinoma" and "GPL570". Datasets with sample sizes less than 40 were excluded. A total of 1547 adhesion-related genes (ARGs) were collected and integrated from the Molecular Signatures Database (MSigDB, data version: v2023.1) (Supplementary Table S1) [14,15,16,17,18]. In this study, the robust rank aggregation (RRA) algorithm was employed to integrate adhesion-related differentially expressed genes (ARDGs) that exhibited stable expression across the datasets [19,20]. Prognostic ARDGs were identified through univariate Cox regression analysis, and PTC survival risk scoring models were constructed using Lasso Cox regression analysis (LPSRSM) and multivariate Cox regression analysis (MPSRSM), respectively. The optimal model, referred to as the LPSRSM, was determined by comparing the predictive abilities of the two models [21]. Subsequently, integration of risk scores with clinicopathologic features and identification of independent risk prognostic factors were performed using multivariate Cox regression analysis. Gene set variation analysis (GSVA) and the CIBERSORT algorithm were used to investigate variations in tumor mechanisms [22,23,24,25]. In addition, deep learning algorithms were utilized to simulate gene expression profiles based on large perturbation datasets to predict drugs that demonstrate efficacy in disease prognosis [26,27,28].
Differential expression analysis was conducted using the "limma" R package with the criteria |logFC| > 1 and adj.P < 0.05 in the TCGA cohort, GSE29265, GSE33630, and GSE60542 datasets. Afterwards, the "RobustRankAggreg" R package [29] was employed to perform the RRA algorithm to identify robust differentially expressed genes (DEGs) in PTC. This algorithm ranks the DEGs by logFC in each dataset and integrates them into a comprehensive ranking list, considering a threshold of Score < 0.05. Finally, the intersection of robust DEGs and ARGs was determined using the "ggVennDiagram" R package [30] to obtain ARDGs.
Metascape website (https://metascape.org/) [31] integrates over 40 bioinformatics databases, allowing for pathway enrichment, biological process annotation, gene-related protein network analysis, and drug analysis. In this study, we utilized Metascape website to enrich gene sets and annotate Gene Ontology (GO) terms and Kyoto Encyclopedia of Genes and Genomes (KEGG). GO terms consist of molecular function (MF), biological process (BP), and cellular component (CC). Analysis was performed with a threshold of P < 0.01, min overlap = 3, and min enrichment = 1.5, and enriched entries were grouped into clusters based on their similarity in hierarchical relationships. Hallmark gene sets (h.all.v2023.1.Hs.symbols.gmt, version: v2023.1) were downloaded from MSigDB, and unsupervised classification of biological states or processes activities was performed using the GSVA algorithm [32] to observe more stable and intuitive changes in biological activity. Additionally, the GSVA algorithm was employed to convert the collected adhesion data into feature scores.
Quantification of tumor-infiltrating immune cells is helpful in elucidating the multifaceted role of the immune system in human cancer and its involvement in tumor escape mechanisms and response to treatment. We employed the "estimate" R package [33] to score stromal and immune gene sets in each sample of the tumor expression matrix, estimating the stromal and immune scores of the tumor samples, which represent the presence of stromal and immune cells. This process is based on the single sample Gene Set Enrichment Analysis (ssGSEA) algorithm. We also utilized the "CIBERSORT" R package [34] to quantify tumor-infiltrating immune cells from tumor RNA sequencing data. The basic principle of this approach is to deconvolve the expression matrix of human immune cell subtypes based on the linear support vector regression, and we performed 100 permutation tests in this process.
Survival analysis is the process of analyzing and inferring the life expectancy of a sample based on data. In this paper, we constructed the survival risk scoring models using Lasso Cox regression analysis and multivariable Cox regression analysis. First, we used univariate Cox regression analysis to screen for prognostic ARDGs significantly associated with overall survival (OS), using a significance level of P < 0.05 and hazard ratio (HR) ≠ 1 as criteria. Next, we built two survival risk scoring models in the TCGA tumor cohort. The first model utilized the Lasso regression algorithm for L1 regularization shrinkage penalty, penalizing the coefficients of variables with minimal contributions to survival and compressing the coefficients of non-important variables to zero, thereby reducing the number of covariates in the Cox regression. This process involved the use of the "glmnet" [35], "survival", and "survminer" R packages, along with 10-fold cross-validation. The second model employed multivariable Cox regression analysis, which is a survival analysis method to study the influence of multiple factors on survival time. We then used the step function to iteratively construct the optimal model, setting the direction to "both". This process utilized the "MASS", "survival", and "survminer" R packages. By linearly combining the regression coefficients and expression levels of each potential prognostic gene, we calculated the risk score: Risk Score = ExprGene1 × Coef1 + ExprGene2 × Coef2 +...+ ExprGeneN × CoefN, where Coef represents the regression coefficient of the gene and Expr represents the gene expression value. To evaluate the predictive ability of the model, we used the "timeROC" R package [36] to compute the receiver operating characteristic (ROC) curves for 1-, 2-, 3-, 5-, and 10-year survival, and assessed the model's performance using the area under curve (AUC). Based on the median of the survival risk scores, we categorized the tumor patients into high- and low-risk groups and evaluated the relationship between survival probability and OS using Kaplan-Meier (KM) survival curves and the log-rank test to compute the P. The optimal survival risk scoring model was determined based on the KM survival curves. Finally, we performed univariate Cox regression analysis and multivariable Cox regression analysis to determine independent prognostic factors, analyzing the relationship between the risk score, the clinical-pathological characteristics (total stage, N stage, M stage, T stage, sex, and age) of the TCGA tumor cohort and OS.
The CMAP database (https://clue.io) is a critical information resource that enables the investigation of the potential impact of existing drugs on diseases. We uploaded the top 100 DEGs upregulated genes from the high-risk group into the CMAP database, with the aim of identifying possible small-molecule drugs. Lead compounds with unclear or uncertain functional significance were excluded from consideration. Statistical significance was determined based on a P cutoff of < 0.05. The study screened for correlations between genes and drugs using an enrichment score that ranged from −1 to +1. Compounds with an enrichment score below zero indicated potential antagonistic effects in the high-risk population. Such compounds could have the potential to serve as therapy candidates by mitigating risk.
We constructed diagnostic models to predict tumors and lymph node metastasis using feature genes that affect prognosis. Both models were built based on Lasso and subjected to 10-fold cross-validation to select the optimal lambda value, followed by linear fitting. By combining the regression coefficients and expression levels of each potential important gene using linear regression, we created an index scoring model: Index = ExprGene1 * Coef1 + ExprGene2 * Coef2 +...+ ExprGeneN * CoefN, where Coef represents the gene regression coefficient, and Expr represents gene expression value. In the lymph node metastasis diagnostic model, we selected high-risk samples as the training set and three internal validation sets. We used the "caret" R package [37], specifically the createDataPartition function, to randomly divide the TCGA tumor samples into validation set 1 and validation set 2 at a 1:1 ratio. We then used the overall TCGA tumor samples as validation set 3. In the disease diagnostic model, we selected the TCGA cohort as the training set and GSE29265, GSE33630, and GSE60542 as external test sets. Finally, we evaluated the predictive ability by calculating the AUC value.
The Human Protein Atlas (HPA) database is a comprehensive resource that provides information on the expression patterns and localization of proteins in human tissues and cells. It is designed to map all proteins encoded by the human genome, providing detailed information about their functions, interactions, and involvement in disease. The database contains information on thousands of proteins, their expression levels in different tissues, and subcellular localization. It also provides information about the genes encoding these proteins, their regulatory elements, and their association with disease. The HPA database is organized into three sections: Cell, Tissue, and Pathology, showing how proteins are expressed in cells, normal tissues, and cancerous tissues. The Pathology section provides information on the pathology of 17 human cancers, including mRNA and protein expression data, as well as millions of in-house generated immunohistochemically stained tissue images. We use this database to look at adhesion feature gene expression in PTC tumor tissues [38].
We used R software (version 4.2.3) for all statistical analyses, and Adobe Illustrator (version 2022, v26.0.1) for image processing. For continuous variable data, The differences between the tumor and control groups, as well as the differences in hallmark gene set features between the high- and low-risk groups in the prognostic analysis, were analyzed using the limma function. The Wilcoxon test was employed for analyzing the differences in immune cell abundance, enabling effective elimination of the influence of outliers on the results. For the comparison of categorical variables, we used either Pearson's chi-squared test or the continuous correction chi-squared test. Pearson or Spearman correlation analysis was used for correlation analysis. We considered the results to have statistical significance if the probability level reached 0.05. P labeled with ns indicates P > 0.05; * indicates P < 0.05; ** indicates P < 0.01; *** indicates P < 0.001; and **** indicates P < 0.0001.
In the TCGA cohort, the GSE29265 dataset, the GSE33630 dataset, and the GSE60542 dataset, we identified a total of 1296 DEGs (with 582 genes downregulated and 714 genes upregulated), 803 DEGs (with 420 genes downregulated and 383 genes upregulated), 1072 DEGs (with 485 genes downregulated and 587 genes upregulated), and 956 DEGs (with 474 genes downregulated and 482 genes upregulated). The selection criteria were |logFC| > 1 and adj.P < 0.05. Volcano plots are shown in Figure 2A–D to visualize the genes with differential expression in the GSE29265, GSE33630, GSE60542 and TCGA cohorts. Subsequently, the RRA algorithm was employed to identify 1072 robust DEGs (with 148 genes downregulated and 169 genes upregulated) (Score < 0.05) that exhibited expression in PTC (Supplementary Table S2.). The top 30 upregulated and downregulated genes in PTC were visualized in heatmaps (Figure 2E–F). The numerical values in the heat maps represent the LogFC values of each gene in the respective dataset. Additionally, the Venn diagram (Figure 2G) illustrated the overlap between robust DEGs and ARGs, depicting 64 overlapping ARDGs (Supplementary Table S3).
The forest plot in Figure 3A shows 16 prognostic ARDGs (DPP4, CLDN1, TENM1, CDH3, CLDN10, MPZL2, ADAMTS9, AMIGO2, ENTPD1, LGALS3, CHST2, NCAM1, FBLN1, APOD, RELN, and OMD) identified by univariate Cox regression analysis (Supplementary Table S4). These genes are consistently expressed in the TCGA cohort, GSE29265, GSE33630, and GSE60542 datasets (all P < 0.0001). Except for NCAM1, FBLN1, APOD, RELN, and OMD, the remaining genes exhibit high expression in tumor samples (Figure S1). The correlation network diagram (Figure 3B) illustrates the co-expression of these genes in tumor samples, where the Pearson correlation coefficients (Cor) are > 0.3 or < −0.3 (Supplementary Table S5). By setting the threshold at Cor > 0.8 and P < 0.05, the strongest correlations are observed between DPP4 and CLDN1 (Cor = 0.807, P < 0.0001), as well as OMD and FBLN1 (Cor = 0.882, P < 0.0001). Functional enrichment analysis (Figure 3C, D) reveals that these genes are involved in BP such as cell-cell adhesion via plasma-membrane adhesion molecules, regulation of leukocyte migration, negative regulation of cell motility, positive regulation of cellular component biogenesis, and positive regulation of cell migration. They are also enriched in the extracellular matrix at the CC level and have functions related to virus receptor activity and cell adhesion molecule binding at the MF level. Furthermore, we conducted a KEGG pathway analysis to understand the pathways in which the adhesion genes related to prognosis are enriched. The results indicated that these genes were enriched in cell adhesion molecules, and Leukocyte transendothelial migration. Hallmark Gene Sets analysis shows enrichment in HALLMARK KRAS SIGNALING UP.
We perform two methods to construct tumor prognostic risk score models. Lasso Cox regression analysis identified six genes significantly associated with prognosis, including DPP4, TENM1, ADAMTS9, AMIGO2, APOD, and RELN. The optimal lambda value determined through 10-fold cross-validation was 0.008 (Figure 4A and B). The LPSRSM was constructed using these six genes, with the formula: Risk score = ExprDPP4 × (−0.259) + ExprTENM1 × (−0.070) + ExprADAMTS9 × (−0.197) + ExprAMIGO2 × (−0.059) + ExprAPOD × 0.439 + ExprRELN × 0.238 (where Expr represents gene expression value). Another risk score model was constructed through multivariate and stepwise Cox regression analysis, which selected three major prognostic genes, including ADAMTS9, LGALS3, and APOD (Figure 4C and D). The multivariate Cox regression analysis PSRSM (MPSRSM) was then constructed using these three genes with the formula: Risk score = ExprADAMTS9 × (−0.572) + ExprLGALS3 × (−0.550) + ExprAPOD × 0.768 (where Expr represents gene expression value). Subsequently, tumor samples were divided into high-risk group and low-risk group based on the median risk score. Both the LPSRSM and MPSRSM showed a symmetric distribution of risk scores among patients (Figure S2A, C). In the LPSRSM, all genes except APOD and RELN demonstrated downregulated expression in high-risk group samples (all P < 0.0001) (Figure S2B). Similarly, in the MPSRSM, all genes except APOD showed downregulated expression in high-risk group samples (all P < 0.0001) (Figure S2D). KM survival analysis using LPSRSM (Figure 4G) demonstrated that the low-risk group had better prognosis than the high-risk group (P = 2.433 × 10−4). Similarly, KM survival analysis using MPSRSM (Figure 4H) demonstrated that the low-risk group had better prognosis than the high-risk group (P = 3.017 × 10−4). In the LPSRSM, the AUC values for predicting patient's 1-, 2-, 3-, 5-, and 10-year prognosis were 0.891, 0.903, 0.957, 0.847, and 0.848, respectively (Figure 4I).
In the MPSRSM, the AUC values for predicting patient's 1-, 2-, 3-, 5-, and 10-year prognosis were 0.876, 0.900, 0.952, 0.866, and 0.885, respectively (Figure 4J). By comparing the ability of the two models to predict OS using KM survival analysis, the LPSRSM showed a better correlation with OS, making it the optimal model for predicting PTC prognosis. Lastly, the LPSRSM was analyzed in relation to clinical and pathological features. Univariate independent prognostic analysis results showed that the total stage, age, and risk score were associated with OS (all P < 0.0001) (Figure 4E). Multivariate independent prognostic analysis showed that age and risk score could serve as independent prognostic factors for tumors (all P < 0.0001) (Figure 4F).
We performed differential analysis on the high-risk group and the low-risk group in the TCGA tumor cohort using the "limma" R package. Under the condition of adj.P < 0.05, a total of 33 terms showed differential expression (Figure 5A) (Supplementary Table S6). Compared to the low-risk group, all terms except PROTEIN SECRETION were upregulated in the high-risk group (all P < 0.05). We found significant associations between hallmark pathways related to energy metabolism, such as HYPOXIA, GLYCOLYSIS, and OXIDATIVE PHOSPHORYLATION in tumors (all P < 0.05). Interestingly, OXIDATIVE PHOSPHORYLATION (Cor = −0.64, P < 0.0001) showed a negative correlation with adhesion feature (Figure 5C, D). In addition, we observed upregulation of inflammatory responses in the high-risk group, which was significantly correlated with adhesion feature (Cor = 0.70, P < 0.0001) (Figure 5C, D). Finally, we compared the clinical and pathological characteristics between the high-risk group and the low-risk group using Pearson's chi-squared test for total stage, N stage, M stage, T stage, and age group (grouping by median). Comparison for M stage was performed using chi-squared test with continuity correction. The high-risk group had higher proportions of death, stage Ⅲ-Ⅳ, age > = 46, stage T3-4, stage N1, and stage M1 compared to the low-risk group. However, only the proportions of deaths (P < 0.01), stages Ⅲ-Ⅳ (P < 0.05), and age > = 46 (P < 0.01) showed statistical significance (Figure 5B) (Supplementary Table S7).
In the previous section of the analysis, we observed upregulation of the inflammatory response in the high-risk group. Additionally, we evaluated the expression levels of the tumor ImmuneScore and StromalScore, and found that both were significantly higher in the high-risk group (all P < 0.05) (Figure 6A, B). The ImmuneScore (Cor = 0.70, P < 0.0001) and StromalScore (Cor = 0.81, P < 0.0001) showed a positive correlation with adhesion feature (Figure 6C and D). Furthermore, we used the CIBERSORT algorithm to deconvolute the expression matrix of immune cell subtypes, allowing us to quantify the abundance of immune infiltrating cells. We then used the Wilcoxon Test to analyze the differences in immune infiltrating cell abundance (Figure 6E). Compared to the low-risk group, the high-risk group exhibited higher expression of memory B cells (P < 0.05), Monocytes (P < 0.01), and resting dendritic cells (P < 0.01) (Figure 6F, G, I). Meanwhile, the high-risk group showed lower expression levels of M0 macrophages (P < 0.05), and resting mast cells (P < 0.01) (Figure 6H, J).
We utilized the Cmap database to establish a correlation between small molecules and the expression of genes that are the top 100 DEGs upregulated genes from the high-risk group. A score close to 1 indicates a positive correlation between the gene and the drug, while a score approaching -1 indicates a negative correlation. In light of this, 10 small molecules (alclometasone, ATPA, azacitidine, fexofenadine, GW-3965, mephenytoin, phenolphthalein, sorafenib, thiostrepton, and UB-165) with a correlation Score < −0.7 and FDR < 2.22 × 10−16 were determined to have therapeutic potential for improving the prognosis of tumors. Finally, the molecular structures and formulas of these small molecule drugs were searched via the Pubchem database (https://pubchem.ncbi.nlm.nih.gov/) (Figure 7A–J).
We used Lasso regression analysis to build two models for predicting lymph node metastasis and tumor diagnosis. After 10-fold cross-validation, the optimal lambda value was determined to be 0.006. Accordingly, four genes, namely DPP4, TENM1, APOD and RELN, were identified as significant for prognosis and diagnosis of lymph node metastasis (Figure 8A–C). Using these four genes, a diagnostic scoring model was developed with the following formula: Index = ExprDPP4 × 0.313 + ExprTENM1 × 0.165 + ExprAPOD × 0.416 + ExprRELN × (−0.666) (where Expr indicates expression level). The accuracy of the lymph node metastasis prediction model was evaluated using ROC curves. The results showed AUC values of 0.767, 0.704, 0.698, and 0.700 for the training set, validation set 1, validation set 2, and validation set 3, respectively (Figure 8G–J). Furthermore, the optimal lambda value of 0.049 was obtained by 10-fold cross-validation, and three genes, DPP4, TENM1, and RELN, were identified as significant for prognosis and tumor diagnosis (Figure 8D–F). A diagnostic scoring model was developed using these three genes with the formula: Index = ExprDPP4 × 2.785 + ExprTENM1 × 0.206 + ExprRELN × (−1.654) (where Expr indicates expression level). The accuracy of the prediction model was evaluated using ROC curves, and the AUC values for the TCGA cohort, GSE29265, GSE33630, and GSE60542 were found to be 0.986, 0.933, 0.976, and 0.934, respectively (Figure 8K–N).
The protein expressions of DPP4, TENM1, ADAMTS9, AMIGO2, and APOD based on IHC were analyzed using the HPA database (Figure 9A–E). The results showed that the expression of DPP4 (staining = high), TENM1 (staining = high), ADAMTS9 (staining = medium), AMIGO2 (staining = medium), and APOD (staining = not detected) were assessed in PTC tumor tissues. It is worth noting that the protein expression of RELN was not included in the HPA database.
Thyroid cancer is considered the most prevalent endocrine malignancy globally, and its incidence is rapidly increasing. While many patients with PTC have a favorable prognosis, there are some who experience adverse outcomes, including recurrence, distant metastasis, and other factors that affect survival [2,3,4,5]. Therefore, it is essential to manage and stratify the risks for PTC patients to optimize treatment. Adhesion, which plays a role in tumor proliferation, metastasis, and is closely linked to the tumor microenvironment (TME) and tumor immunity, has been identified as a key factor [8]. In this study, we developed a model based on adhesion feature to predict prognosis, lymph node metastasis, and tumor diagnosis in PTC, while also exploring the biological functions of adhesion in PTC.
Due to the significant increase in data sets from various sequencing platforms, facilitated by advancements in high-throughput microarray technology and bioinformatics methods, inconsistencies and biases exist among different data sets. In this study, we employed the RRA algorithm to address the noise and inconsistency in gene sequencing for the TCGA cohort, as well as the GSE29265, GSE33630, and GSE60542 datasets. By implementing the RRA algorithm, we identified 317 DEGs, comprising 169 upregulated genes and 148 downregulated genes. Among these DEGs, 64 genes overlapped with ARGs. Univariate Cox regression analysis revealed 16 prognostic ARDGs. Functional enrichment analysis of these genes indicated associations with biological processes such as cell-cell adhesion via plasma-membrane adhesion molecules, regulation of leukocyte migration, and calcium-independent cell-cell adhesion through plasma membrane cell-adhesion molecules. Subsequently, we constructed a PTC prognosis model using two methods: Lasso Cox regression analysis and multivariable Cox regression analysis. The results demonstrated that the Lasso Cox regression model slightly outperformed the multivariable Cox regression analysis when predicting OS at 1, 2, and 3 years. However, for predicting OS at 5 and 10 years, the multivariable Cox regression model showed slight superiority. It has been determined that the model constructed using Lasso Cox regression is slightly superior through comparison of Kaplan-Meier survival analysis. The Lasso Cox regression model identified six feature genes for prognosis, namely DPP4, TENM1, ADAMTS9, AMIGO2, APOD, and RELN. In thyroid cancer, the significantly higher expression of DPP4 has been observed compared to adjacent noncancerous tissue [39]. The correlation between DPP4 expression and lymph node metastasis as well as BRAFV600E mutation has been demonstrated in various studies. Moreover, the metastasis of thyroid cancer cells is promoted by DPP4 through the signaling pathway of integrins/FAK/AKT/c-Jun/TGF-β1 [40]. The inhibition of PTC cell proliferation, EMT, and promotion of cell apoptosis are observed when the DPP4 gene is silenced, which is evidenced by the suppression of the MAPK pathway as discovered by Hu et al [41]. TENM1 has emerged as a potential marker for PTC progression, with highly upregulated expression in cancerous tissue and negative expression in benign thyroid tissue [42]. Furthermore, TENM1 is associated with extrathyroidal invasion, BRAF V600E mutation, and advanced stage [43]. ADAMTS9, known for its involvement in proteoglycans degradation, organ shape control during development, and inhibition of angiogenesis, is frequently silenced by promoter hypermethylation [44]. The loss of ADAMTS9 expression promotes cell proliferation and tumor growth through the activation of the oncogenic PI3K/AKT signaling pathway. Methylation of ADAMTS9 is identified as an independent prognostic factor in gastric cancer [45,46]. AMIGO2, implicated in tumor development and metastasis, has been shown to facilitate the adhesion of tumor cells to hepatic endothelial cells, leading to liver metastasis in gastric and colorectal cancer [47,48]. In prostate cancer, high AMIGO2 expression is correlated with unfavorable prognosis and tumor progression. Additionally, AMIGO2 is involved in tumor cell proliferation, invasion, metastasis, and possibly tumor progression through the induction of EMT [49]. APOD, a protein involved in lipid metabolism and prevalent in the central and peripheral nervous systems, exhibits increased expression in certain neurodegenerative diseases and spinal cord injuries [50,51]. Overexpressing APOD impedes human umbilical vein endothelial cell formation, making it a potential therapeutic target for tumor angiogenesis [52]. RELN, an extracellular glycoprotein vital for neuronal migration, is often silenced due to abnormal promoter hypermethylation in gastric cancer, and its loss of expression is significantly associated with advanced stages of gastric cancer [53,54]. Additionally, mutations in the RELN gene may serve as potential biomarkers in melanoma and non-small cell lung cancer [55]. Using these six genes, a prognostic diagnostic model was constructed, yielding AUC values of 0.891, 0.903, 0.957, 0.847, and 0.848 for predicting the 1-, 2-, 3-, 5-, and 10-year prognosis of patients, respectively. Moreover, through multivariate Cox regression analysis of the prognostic model and PTC clinical pathological features, the independent prognostic factor status of the prognostic survival model was confirmed.
In this paper, the PTC patients were divided into high-risk and low-risk groups based on our prognostic model. Within the high-risk group, downregulation of HALLMARK PROTEIN SECRETION biological function activity was observed, while the biological function activities of HALLMARK GLYCOLYSIS, HALLMARK FATTY ACID METABOLISM, HALLMARK HYPOXIA, HALLMARK OXIDATIVE PHOSPHORYLATION, and HALLMARK INFLAMMATORY RESPONSE, which are related to energy metabolism and immunity, were upregulated. A significant association between adhesion biological function and these energy metabolism and immunity-related biological functions in PTC was found in this study. Tissue formation and structural integrity heavily rely on adhesion's crucial role. Throughout different stages of cancer progression, the adhesion between cancer cells and their attachment to the extracellular matrix undergo constant changes. Adhesion biological features are closely intertwined with tumorigenesis and tumor development, necessitating a thorough exploration of adhesion characteristics' mechanisms in PTC. The protein secretion pathway is a vital system that connects tumor cells with one another and with the microenvironment, wherein the secretion pathway and its products play crucial roles in the survival of eukaryotic organisms [56]. However, dysregulation of the secretion mechanism may support detrimental processes, such as tumor formation [57]. Cellular metabolism encompasses a complex biochemical network that converts nutrients into various small molecules [6]. Among these molecules, ATP generation is essential for maintaining cellular homeostasis and relies on glycolysis in the cytoplasm and oxidative phosphorylation in the mitochondria, which can occur through the pentose phosphate pathway and the TCA cycle [58,59]. Additionally, cellular metabolism produces biomolecules required for protein, DNA/RNA, and membrane synthesis, such as amino acids, nucleotides, and fatty acids [60,61]. These processes are vital for maintaining normal cell growth and function. Metabolic changes significantly contribute to the metastasis and formation of cancer cells [59]. The multi-step process of metastasis is closely tied to metabolic reprogramming, while the adhesion between cancer cells and their attachment to the extracellular matrix continuously changes throughout different stages of cancer progression [62]. Adhesion modifications that promote cancer have been shown to induce or be induced by signal pathways closely linked to metabolic changes. This discovery further emphasizes the importance of metabolic alterations in the process of cancer cell metastasis and formation, as they have the potential to promote tumor development by influencing cell adhesion [6]. Tumor cells acquire and maintain many of these characteristics through interactions with each other and neighboring "normal" cells, collectively forming the tumor microenvironment alongside cancer cells [63]. In our research, we identified upregulation of the inflammatory response biological function in the high-risk group, along with increased matrix score and immune score, all of which were associated with adhesion characteristics. Analysis of the tumor tissue microenvironment demonstrated upregulation of memory B cells, monocytes, and resting dendritic cells within the high-risk group. Furthermore, using the prognostic model, we identified small molecular drugs in the CMAP database that were closely related to gene expression. Through the analysis of the link between gene expression and drug molecules, we identified ten potential small molecular drugs that could improve PTC prognosis: alclometasone, ATPA, azacitidine, fexofenadine, GW-3965, mephenytoin, phenolphthalein, sorafenib, thiostrepton, and UB-165.
Lymph node metastasis and extrathyroidal invasion profoundly alter the equilibrium between adhesive molecules and the basement membrane in PTC. Currently, there is no effective method for predicting lymph node metastasis, with most cases being detected through imaging examinations. In this study, four adhesive feature genes (DPP4, TENM1, APOD, and RELN) were identified using lasso regression analysis. A lymph node metastasis model based on these four genes achieved an accuracy of 0.767 in the training set, and accuracies of 0.704, 0.698, and 0.700 in validation sets 1, 2, and 3, respectively. Additionally, a disease diagnosis model based on three adhesive feature genes (DPP4, TENM1, and RELN) achieved an accuracy of 0.986 in the training set, and accuracies of 0.933, 0.976, and 0.934 in GSE29265, GSE33630, and GSE60542 dataset, respectively. The lymph node metastasis model and tumor diagnosis model constructed in this study exhibited stability and accuracy in prediction.
Our study was novel in that it integrated multiple machine learning algorithms to construct models for diagnosing PTC, lymph node metastasis, and prognosis. Additionally, we investigated the adhesion functional mechanisms, which served as a new topic in this study. However, there are still some limitations to our research. Firstly, during the process of merging datasets and eliminating multicollinearity, many genes were excluded, resulting in the loss of some important genes. However, in order to validate the models in independent datasets, we had to ensure that the genes used for model construction were available in the test set. Secondly, some clinical and molecular features were not adequately provided in public datasets, which limited our ability to further uncover the potential links between diagnostic genes and certain traits. Lastly, although our study provided a framework for early tumor diagnosis, lymph node metastasis prediction, and prognosis evaluation by evaluating specific genes, the results are still in the stage of analysis and speculation without experimental validation. Future research could explore the integration of our findings into clinical practice to improve their usability and effectiveness. Additionally, further research is needed to investigate the combined therapeutic value of these ten small molecule drugs at the cellular and animal levels.
In our study, we employed machine learning signature selection methods to identify six prognostic feature genes, four lymph node metastasis feature genes, and three tumor diagnosis feature genes from PTC tumor tissues. These feature genes can be used to construct models with high predictive value, effectively predicting tumor, lymph node metastasis, and prognosis. The selected signature revealed that energy metabolism and immune response may be key mechanisms for PTC prognosis. Finally, drugs targeting prognostic genes will provide new insights into targeted therapy.
The authors declare they have not used Artificial Intelligence (AI) tools in the creation of this article.
All authors listed have made a substantial, direct, and intellectual contribution to the work and approved it for publication. S. S. analyzed the data and drafted the manuscript. X. C. and J. S. downloaded the data and prepared figures. G. Z., S. L. and H. W. designed the research and edited the manuscript. All authors reviewed, revised, commented on and approved the final manuscript. This study was supported by Doctor of excellence program (DEP), The First Hospital of Jilin University (JDYY-DEP-2022015).
The authors declare there is no conflict of interest.
Analyses were conducted using RStudio. The programming code is available upon request by contacting the corresponding author.
[1] |
K. R. Joseph, S. Edirimanne, G. D. Eslick, Multifocality as a prognostic factor in thyroid cancer: A meta-analysis, Int. J. Surg., 50 (2018), 121–125. http://.doi.org/10.1016/j.ijsu.2017.12.035 doi: 10.1016/j.ijsu.2017.12.035
![]() |
[2] |
A. Arianpoor, M. Asadi, E. Amini, A. Ziaeemehr, Investigating the prevalence of risk factors of papillary thyroid carcinoma recurrence and disease-free survival after thyroidectomy and central neck dissection in Iranian patients, Acta Chir. Belg., 120 (2020), 173–178. http://.doi.org/10.1080/00015458.2019.1576447 doi: 10.1080/00015458.2019.1576447
![]() |
[3] |
V. Zaydfudim, I. D. Feurer, M. R. Griffin, J. E. Phay, The impact of lymph node involvement on survival in patients with papillary and follicular thyroid carcinoma, Surgery, 144 (2008), 1077–1078. http://.doi.org/10.1016/j.surg.2008.08.034 doi: 10.1016/j.surg.2008.08.034
![]() |
[4] |
I. M. Boschin, M. R. Pelizzo, F. Giammarile, D. Rubello, P. Colletti, Lymphoscintigraphy in differentiated thyroid cancer, Clin. Nucl. Med., 40 (2015), e343–350. http://.doi.org/10.1097/RLU.0000000000000825 doi: 10.1097/RLU.0000000000000825
![]() |
[5] |
D. Hou, H. Xu, B. Yuan, J. Liu, Y. Lu, M. Liu, Effects of active localization and vascular preservation of inferior parathyroid glands in central neck dissection for papillary thyroid carcinoma, World J. Surg. Oncol., 18 (2020), 95. http://.doi.org/10.1186/s12957-020-01867-y doi: 10.1186/s12957-020-01867-y
![]() |
[6] |
I. Elia, G. Doglioni, S. M. Fendt, Metabolic hallmarks of metastasis formation, Trends Cell Biol., 28 (2018), 673–684. http://.doi.org/10.1016/j.tcb.2018.04.002 doi: 10.1016/j.tcb.2018.04.002
![]() |
[7] |
H. Harjunpää, M. Llort Asens, C. Guenther, S. C. Fagerholm, Cell adhesion molecules and their roles and regulation in the immune and tumor microenvironment, Front. Immunol., 10 (2019), 1078. http://.doi.org/10.3389/fimmu.2019.01078 doi: 10.3389/fimmu.2019.01078
![]() |
[8] |
L. Mautone, C. Ferravante, A. Tortora, Higher integrin alpha 3 beta1 expression in papillary thyroid cancer is associated with worst outcome, Cancers (Basel), 13 (2021), 2937.http://.doi.org/10.3390/cancers13122937 doi: 10.3390/cancers13122937
![]() |
[9] | J. Weiss, F. Kuusisto, K. Boyd, Machine learning for treatment assignment: Improving individualized risk attribution, AMIA Annu. Symp. Proc., 2015 (2015), 1306–1315. |
[10] | J. C. Weiss, D. Page, P. L. Peissig, Statistical Relational Learning to predict primary myocardial infarction from electronic health records, Proc. Innov. Appl. Artif. Intell. Conf., 2012 (2012), 2341–2347. |
[11] |
M. E. Ritchie, B. Phipson, D. Wu, Limma powers differential expression analyses for RNA-sequencing and microarray studies, Nucleic Acids Res., 43 (2015), e47.http://.doi.org/10.1093/nar/gkv007 doi: 10.1093/nar/gkv007
![]() |
[12] |
G. Tomas, M. Tarabichi, D. Gacquer, A general method to derive robust organ-specific gene expression-based differentiation indices: application to thyroid cancer diagnostic, Oncogene, 31 (2012), 4490–4498.http://.doi.org/10.1038/onc.2011.626 doi: 10.1038/onc.2011.626
![]() |
[13] |
M. Tarabichi, M. Saiselet, C. Tresallet, Revisiting the transcriptional analysis of primary tumours and associated nodal metastases with enhanced biological and statistical controls: application to thyroid cancer, Br. J. Cancer, 112 (2015), 1665–1674.http://.doi.org/10.1038/bjc.2014.665 doi: 10.1038/bjc.2014.665
![]() |
[14] |
A. Subramanian, P. Tamayo, V. K. Mootha, Gene set enrichment analysis: a knowledge-based approach for interpreting genome-wide expression profiles, Proc. Natl. Acad. Sci. U. S. A., 102 (2005), 15545–15550. http://.doi.org/10.1073/pnas.0506580102 doi: 10.1073/pnas.0506580102
![]() |
[15] |
A. Liberzon, A. Subramanian, R. Pinchback, Molecular signatures database (MSigDB) 3.0, Bioinformatics, 27 (2011), 1739–1740. http://.doi.org/10.1093/bioinformatics/btr260 doi: 10.1093/bioinformatics/btr260
![]() |
[16] |
A. Liberzon, C. Birger, H. Thorvaldsdottir, The Molecular Signatures Database (MSigDB) hallmark gene set collection, Cell Syst., 1 (2015), 417–425. http://.doi.org/10.1016/j.cels.2015.12.004 doi: 10.1016/j.cels.2015.12.004
![]() |
[17] |
G. Huang, X. Xu, C. Ju, Identification and validation of autophagy-related gene expression for predicting prognosis in patients with idiopathic pulmonary fibrosis, Front. Immunol., 13 (2022), 997138. http://.doi.org/10.3389/fimmu.2022.997138 doi: 10.3389/fimmu.2022.997138
![]() |
[18] |
X. Sun, Z. Zhang, Z. Wang, The role of Angiogenesis and remodeling (AR) associated signature for predicting prognosis and clinical outcome of immunotherapy in pan-cancer, Front. Immunol., 13 (2022), 1033967. http://.doi.org/10.3389/fimmu.2022.1033967 doi: 10.3389/fimmu.2022.1033967
![]() |
[19] |
J. Ruan, S. Xu, R. Chen, EMLI-ICC: an ensemble machine learning-based integration algorithm for metastasis prediction and risk stratification in intrahepatic cholangiocarcinoma, Brief. Bioinform., 23 (2022), bbac450. http://.doi.org/10.1093/bib/bbac450 doi: 10.1093/bib/bbac450
![]() |
[20] |
X. Wang, L. Yang, C. Yu, An integrated computational strategy to predict personalized cancer drug combinations by reversing drug resistance signatures, Comput. Biol. Med., 163 (2023), 107230. http://.doi.org/10.1016/j.compbiomed.2023.107230 doi: 10.1016/j.compbiomed.2023.107230
![]() |
[21] |
H. Zhang, P. Xia, J. Liu, ATIC inhibits autophagy in hepatocellular cancer through the AKT/FOXO3 pathway and serves as a prognostic signature for modeling patient survival, Int. J. Biol. Sci., 17 (2021), 4442–4458. http://.doi.org/10.7150/ijbs.65669 doi: 10.7150/ijbs.65669
![]() |
[22] |
X. Bao, J. Chi, Y. Zhu, High FAAP24 expression reveals poor prognosis and an immunosuppressive microenvironment shaping in AML, Cancer Cell Int., 23 (2023), 117. http://.doi.org/10.1186/s12935-023-02937-3 doi: 10.1186/s12935-023-02937-3
![]() |
[23] |
B. Cheng, C. Tang, J. Xie, Cuproptosis illustrates tumor micro-environment features and predicts prostate cancer therapeutic sensitivity and prognosis, Life Sci., 325 (2023), 121659. http://.doi.org/10.1016/j.lfs.2023.121659 doi: 10.1016/j.lfs.2023.121659
![]() |
[24] |
S. He, Y. Ding, Z. Ji, HOPX is a tumor-suppressive biomarker that corresponds to T cell infiltration in skin cutaneous melanoma, Cancer Cell Int., 23 (2023), 122. http://.doi.org/10.1186/s12935-023-02962-2 doi: 10.1186/s12935-023-02962-2
![]() |
[25] |
Z. Liu, L. Liu, S. Weng, Machine learning-based integration develops an immune-derived lncRNA signature for improving outcomes in colorectal cancer, Nat. Commun., 13 (2022), 816. http://.doi.org/10.1038/s41467-022-28421-6 doi: 10.1038/s41467-022-28421-6
![]() |
[26] |
Y. Chen, Y. Pan, H. Gao, Mechanistic insights into super-enhancer-driven genes as prognostic signatures in patients with glioblastoma, J. Cancer Res. Clin. Oncol., 149 (2023), 12315–12332. http://.doi.org/10.1007/s00432-023-05121-2 doi: 10.1007/s00432-023-05121-2
![]() |
[27] |
A. Huang, L. Li, X. Liu, Hedgehog signaling is a potential therapeutic target for vascular calcification, Gene, 872 (2023), 147457. http://.doi.org/10.1016/j.gene.2023.147457 doi: 10.1016/j.gene.2023.147457
![]() |
[28] |
P. Zhou, J. Shen, X. Ge, Classification and characterisation of extracellular vesicles-related tuberculosis subgroups and immune cell profiles, J. Cell. Mol. Med., 27 (2023), 2482–2494. http://.doi.org/10.1111/jcmm.17836 doi: 10.1111/jcmm.17836
![]() |
[29] |
R. Kolde, S. Laur, P. Adler, Robust rank aggregation for gene list integration and meta-analysis, Bioinformatics, 28 (2012), 573–580. http://.doi.org/10.1093/bioinformatics/btr709 doi: 10.1093/bioinformatics/btr709
![]() |
[30] |
C. H. Gao, G. Yu, P. Cai, ggVennDiagram: An intuitive, easy-to-use, and highly customizable R package to generate Venn Diagram, Front. Genet., 12 (2021), 706907. http://.doi.org/10.3389/fgene.2021.706907 doi: 10.3389/fgene.2021.706907
![]() |
[31] |
Y. Zhou, B. Zhou, L. Pache, Metascape provides a biologist-oriented resource for the analysis of systems-level datasets, Nat. Commun., 10 (2019), 1523. http://.doi.org/10.1038/s41467-019-09234-6 doi: 10.1038/s41467-019-09234-6
![]() |
[32] |
S. Hanzelmann, R. Castelo, J. Guinney, GSVA: gene set variation analysis for microarray and RNA-seq data, BMC Bioinf., 14 (2013), 7. http://.doi.org/10.1186/1471-2105-14-7 doi: 10.1186/1471-2105-14-7
![]() |
[33] |
K. Yoshihara, M. Shahmoradgoli, E. Martinez, Inferring tumour purity and stromal and immune cell admixture from expression data, Nat. Commun., 4 (2013), 2612. http://.doi.org/10.1038/ncomms3612 doi: 10.1038/ncomms3612
![]() |
[34] |
A. M. Newman, C. L. Liu, M. R. Green, Robust enumeration of cell subsets from tissue expression profiles, Nat. Methods, 12 (2015), 453–457. http://.doi.org/10.1038/nmeth.3337 doi: 10.1038/nmeth.3337
![]() |
[35] |
J. H. Friedman, T. Hastie, R. Tibshirani, Regularization paths for generalized linear models via coordinate descent, J. Stat. Software, 33 (2010), 1–22. http://.doi.org/10.18637/jss.v033.i01 doi: 10.18637/jss.v033.i01
![]() |
[36] |
P. Blanche, J. F. Dartigues, H. Jacqmin-Gadda, Estimating and comparing time-dependent areas under receiver operating characteristic curves for censored event times with competing risks, Stat. Med., 32 (2013), 5381–5397. http://.doi.org/10.1002/sim.5958 doi: 10.1002/sim.5958
![]() |
[37] |
M. Kuhn, Building Predictive models in R using the caret package, J. Stat. Software, 28 (2008), 1–26. http://.doi.org/10.18637/jss.v028.i05 doi: 10.18637/jss.v028.i05
![]() |
[38] |
M. Uhlen, C. Zhang, S. Lee, A pathology atlas of the human cancer transcriptome, Science, 357 (2017), eaan2507. http://.doi.org/10.1126/science.aan2507 doi: 10.1126/science.aan2507
![]() |
[39] |
N. Enz, G. Vliegen, I. De Meester, CD26/DPP4-a potential biomarker and target for cancer therapy, Pharmacol Ther, 198 (2019), 135–159. http://.doi.org/10.1016/j.pharmthera.2019.02.015 doi: 10.1016/j.pharmthera.2019.02.015
![]() |
[40] |
Q. He, H. Cao, Y. Zhao, Dipeptidyl peptidase-4 stabilizes integrin alpha4beta1 complex to promote thyroid cancer cell metastasis by activating transforming growth factor-beta signaling pathway, Thyroid, 32 (2022), 1411–1422. http://.doi.org/10.1089/thy.2022.0317 doi: 10.1089/thy.2022.0317
![]() |
[41] |
X. Hu, S. Chen, C. Xie, DPP4 gene silencing inhibits proliferation and epithelial-mesenchymal transition of papillary thyroid carcinoma cells through suppression of the MAPK pathway, J. Endocrinol. Invest., 44 (2021), 1609–1623. http://.doi.org/10.1007/s40618-020-01455-7 doi: 10.1007/s40618-020-01455-7
![]() |
[42] |
G. Peppino, R. Ruiu, M. Arigoni, Teneurins: Role in cancer and potential role as diagnostic biomarkers and targets for therapy, Int. J. Mol. Sci., 22 (2021), 2321. http://.doi.org/10.3390/ijms22052321 doi: 10.3390/ijms22052321
![]() |
[43] |
S. P. Cheng, M. J. Chen, M. N. Chien, Overexpression of teneurin transmembrane protein 1 is a potential marker of disease progression in papillary thyroid carcinoma, Clin. Exper. Med., 17 (2017), 555–564. http://.doi.org/10.1007/s10238-016-0445-y doi: 10.1007/s10238-016-0445-y
![]() |
[44] |
S. Lemarchant, M. Pruvost, J. Montaner, ADAMTS proteoglycanases in the physiological and pathological central nervous system, J. Neuroinflamm., 10 (2013), 133. http://.doi.org/10.1186/1742-2094-10-133 doi: 10.1186/1742-2094-10-133
![]() |
[45] |
W. Sun, G. Ma, L. Zhang, DNMT3A-mediated silence in ADAMTS9 expression is restored by RNF180 to inhibit viability and motility in gastric cancer cells, Cell Death Dis., 12 (2021), 428. http://.doi.org/10.1038/s41419-021-03628-5 doi: 10.1038/s41419-021-03628-5
![]() |
[46] |
N. Wang, X. Huo, B. Zhang, METTL3-Mediated ADAMTS9 Suppression facilitates angiogenesis and carcinogenesis in gastric cancer, Front. Oncol., 12 (2022), 861807. http://.doi.org/10.3389/fonc.2022.861807 doi: 10.3389/fonc.2022.861807
![]() |
[47] |
K. Goto, M. Morimoto, M. Osaki, The impact of AMIGO2 on prognosis and hepatic metastasis in gastric cancer patients, BMC Cancer, 22 (2022), 280. http://.doi.org/10.1186/s12885-022-09339-0 doi: 10.1186/s12885-022-09339-0
![]() |
[48] |
R. Izutsu, M. Osaki, J. P. Jehun, Liver metastasis formation is defined by AMIGO2 expression via adhesion to hepatic endothelial cells in human gastric and colorectal cancer cells, Pathol. Res. Pract., 237 (2022), 154015. http://.doi.org/10.1016/j.prp.2022.154015 doi: 10.1016/j.prp.2022.154015
![]() |
[49] |
Z. Han, Y. Feng, Y. Deng, Integrated analysis reveals prognostic value and progression-related role of AMIGO2 in prostate cancer, Transl. Androl. Urol., 11 (2022), 914–928. http://.doi.org/10.21037/tau-21-1148 doi: 10.21037/tau-21-1148
![]() |
[50] |
E. Rassart, F. Desmarais, O. Najyb, Apolipoprotein D, Gene, 756 (2020), 144874. http://.doi.org/10.1016/j.gene.2020.144874 doi: 10.1016/j.gene.2020.144874
![]() |
[51] |
F. Desmarais, V. Herve, K. F. Bergeron, Cerebral apolipoprotein D exits the brain and accumulates in peripheral tissues, Int. J. Mol. Sci., 22 (2021), 4118. http://.doi.org/10.3390/ijms22084118 doi: 10.3390/ijms22084118
![]() |
[52] |
C. J. Lai, H. C. Cheng, C. Y. Lin, Activation of liver X receptor suppresses angiogenesis via induction of ApoD, Faseb J., 31 (2017), 5568–5576. http://.doi.org/10.1096/fj.201700374R doi: 10.1096/fj.201700374R
![]() |
[53] |
M. Schulze, C. Violonchi, S. Swoboda, RELN signaling modulates glioblastoma growth and substrate-dependent migration, Brain Pathol., 28 (2018), 695–709. http://.doi.org/10.1111/bpa.12584 doi: 10.1111/bpa.12584
![]() |
[54] | O. Dohi, H. Takada, N. Wakabayashi, Epigenetic silencing of RELN in gastric cancer, Int. J. Oncol., 36 (2010), 85–92. http://doi.org/10.3892/ijo_00000478 |
[55] |
Z. Li, X. Wang, Y. Yang, Identification and validation of RELN mutation as a response indicator for immune checkpoint inhibitor therapy in melanoma and non-small cell lung cancer, Cells, 11 (2022), 3841. http://.doi.org/10.3390/cells11233841 doi: 10.3390/cells11233841
![]() |
[56] |
N. Rufo, A. D. Garg, P. Agostinis, The unfolded protein response in immunogenic cell death and cancer immunotherapy, Trends Cancer, 3 (2017), 643–658. http://.doi.org/10.1016/j.trecan.2017.07.002 doi: 10.1016/j.trecan.2017.07.002
![]() |
[57] |
R. Saghaleyni, A. Sheikh Muhammad, P. Bangalore, Machine learning-based investigation of the cancer protein secretory pathway, PLoS Comput. Biol., 17 (2021), e1008898. http://.doi.org/10.1371/journal.pcbi.1008898 doi: 10.1371/journal.pcbi.1008898
![]() |
[58] |
C. T. Walsh, B. P. Tu, Y. Tang, Eight kinetically stable but thermodynamically activated molecules that power cell metabolism, Chem. Rev., 118 (2018), 1460–1494. http://.doi.org/10.1021/acs.chemrev.7b00510 doi: 10.1021/acs.chemrev.7b00510
![]() |
[59] |
S. Y. Lunt, S. M. Fendt, Metabolism – A cornerstone of cancer initiation, progression, immune evasion and treatment response, Curr. Opin. Syst. Biol., 8 (2018), 67–72. https://doi.org/10.1016/j.coisb.2017.12.006 doi: 10.1016/j.coisb.2017.12.006
![]() |
[60] |
V. Friand, G. David, P. Zimmermann, Syntenin and syndecan in the biogenesis of exosomes, Biol. Cell., 107 (2015), 331–341. http://.doi.org/10.1111/boc.201500010 doi: 10.1111/boc.201500010
![]() |
[61] |
S. Y. Lunt, M. G. Vander Heiden, Aerobic glycolysis: meeting the metabolic requirements of cell proliferation, Annu. Rev. Cell Dev. Biol., 27 (2011), 441–464. http://.doi.org/10.1146/annurev-cellbio-092910-154237 doi: 10.1146/annurev-cellbio-092910-154237
![]() |
[62] |
B. Sousa, J. Pereira, J. Paredes, The crosstalk between cell adhesion and cancer metabolism, Int. J. Mol. Sci., 20 (2019), 1933. http://.doi.org/10.3390/ijms20081933 doi: 10.3390/ijms20081933
![]() |
[63] |
D. Hanahan, L. M. Coussens, Accessories to the crime: functions of cells recruited to the tumor microenvironment, Cancer Cell, 21 (2012), 309–322. http://.doi.org/10.1016/j.ccr.2012.02.022 doi: 10.1016/j.ccr.2012.02.022
![]() |
![]() |
![]() |