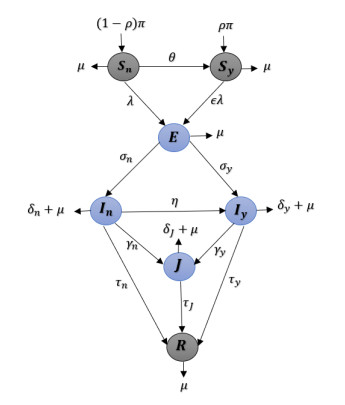
Monkeypox (mpox), a zoonotic viral disease caused by the monkeypox virus (mpoxv), is endemic in many countries in West Africa and is sometimes exported to other parts of the world. The recent outbreak of mpoxv in humans, in endemic and non-endemic countries, has created substantial public health concern worldwide. This research uses a mechanistic model to study the transmission dynamics of mpoxv epidemics in the USA. Our model describes the interaction between different categories of individuals represent various infection phases and hospitalization processes. The model also takes into account the extent of compliance with non-pharmaceutical intervention strategies (NPIs), such as using condoms during sexual contact, quarantine and avoiding large gatherings. The model's equilibria are analyzed, and results on asymptotic stability are obtained. Moreover, the basic reproductive number and other threshold quantities are used to establish the conditions for a forward or backward bifurcation. Our model accurately captures the incidence curves from mpox surveillance data for the USA, indicating that it can be used to explain mpoxv transmission and suggest some effective ways to enhance control efforts. In addition, numerical simulations are carried out to examine the influence of some parameters on the overall dynamics of the model. A partial rank correlation coefficient is adopted for the sensitivity analysis to determine the model most important parameters, which require close attention for effective mpoxv prevention and control. We conclude that it is especially important to ensure that NPIs are properly followed to mitigate mpoxv outbreaks effectively.
Citation: Rubayyi T. Alqahtani, Salihu S. Musa, Mustafa Inc. Modeling the role of public health intervention measures in halting the transmission of monkeypox virus[J]. AIMS Mathematics, 2023, 8(6): 14142-14166. doi: 10.3934/math.2023723
[1] | Ahmed Alshehri, Saif Ullah . Optimal control analysis of Monkeypox disease with the impact of environmental transmission. AIMS Mathematics, 2023, 8(7): 16926-16960. doi: 10.3934/math.2023865 |
[2] | Weerawat Sudsutad, Chatthai Thaiprayoon, Jutarat Kongson, Weerapan Sae-dan . A mathematical model for fractal-fractional monkeypox disease and its application to real data. AIMS Mathematics, 2024, 9(4): 8516-8563. doi: 10.3934/math.2024414 |
[3] | Jutarat Kongson, Chatthai Thaiprayoon, Weerawat Sudsutad . Analysis of a mathematical model for the spreading of the monkeypox virus with constant proportional-Caputo derivative operator. AIMS Mathematics, 2025, 10(2): 4000-4039. doi: 10.3934/math.2025187 |
[4] | Muhammad Altaf Khan, Sajjad Ullah, Saif Ullah, Muhammad Farhan . Fractional order SEIR model with generalized incidence rate. AIMS Mathematics, 2020, 5(4): 2843-2857. doi: 10.3934/math.2020182 |
[5] | Shah Hussain, Naveed Iqbal, Elissa Nadia Madi, Thoraya N. Alharthi, Ilyas Khan . Vaccination strategies in a stochastic SIVR epidemic model. AIMS Mathematics, 2025, 10(2): 4441-4456. doi: 10.3934/math.2025204 |
[6] | Khaled A. Aldwoah, Mohammed A. Almalahi, Kamal Shah, Muath Awadalla, Ria H. Egami . Dynamics analysis of dengue fever model with harmonic mean type under fractal-fractional derivative. AIMS Mathematics, 2024, 9(6): 13894-13926. doi: 10.3934/math.2024676 |
[7] | Yasir Ramzan, Aziz Ullah Awan, Muhammad Ozair, Takasar Hussain, Rahimah Mahat . Innovative strategies for Lassa fever epidemic control: a groundbreaking study. AIMS Mathematics, 2023, 8(12): 30790-30812. doi: 10.3934/math.20231574 |
[8] | Zafar Iqbal, Muhammad Aziz-ur Rehman, Muhammad Imran, Nauman Ahmed, Umbreen Fatima, Ali Akgül, Muhammad Rafiq, Ali Raza, Ali Asrorovich Djuraev, Fahd Jarad . A finite difference scheme to solve a fractional order epidemic model of computer virus. AIMS Mathematics, 2023, 8(1): 2337-2359. doi: 10.3934/math.2023121 |
[9] | Rahat Zarin, Amir Khan, Aurangzeb, Ali Akgül, Esra Karatas Akgül, Usa Wannasingha Humphries . Fractional modeling of COVID-19 pandemic model with real data from Pakistan under the ABC operator. AIMS Mathematics, 2022, 7(9): 15939-15964. doi: 10.3934/math.2022872 |
[10] | Abdul Qadeer Khan, Fakhra Bibi, Saud Fahad Aldosary . Bifurcation analysis and chaos in a discrete Hepatitis B virus model. AIMS Mathematics, 2024, 9(7): 19597-19625. doi: 10.3934/math.2024956 |
Monkeypox (mpox), a zoonotic viral disease caused by the monkeypox virus (mpoxv), is endemic in many countries in West Africa and is sometimes exported to other parts of the world. The recent outbreak of mpoxv in humans, in endemic and non-endemic countries, has created substantial public health concern worldwide. This research uses a mechanistic model to study the transmission dynamics of mpoxv epidemics in the USA. Our model describes the interaction between different categories of individuals represent various infection phases and hospitalization processes. The model also takes into account the extent of compliance with non-pharmaceutical intervention strategies (NPIs), such as using condoms during sexual contact, quarantine and avoiding large gatherings. The model's equilibria are analyzed, and results on asymptotic stability are obtained. Moreover, the basic reproductive number and other threshold quantities are used to establish the conditions for a forward or backward bifurcation. Our model accurately captures the incidence curves from mpox surveillance data for the USA, indicating that it can be used to explain mpoxv transmission and suggest some effective ways to enhance control efforts. In addition, numerical simulations are carried out to examine the influence of some parameters on the overall dynamics of the model. A partial rank correlation coefficient is adopted for the sensitivity analysis to determine the model most important parameters, which require close attention for effective mpoxv prevention and control. We conclude that it is especially important to ensure that NPIs are properly followed to mitigate mpoxv outbreaks effectively.
The outbreak of the monkeypox virus (mpoxv) across numerous non-endemic countries since May 2022 shows some distint clinical and epidemiological features from previous mpoxv outbreaks, most notably its discerned development and dominance amongst men who have sex with men (MSM) or those who identify as gay or bisexual [14]. The majority of recent mpoxv cases have no history of travel to endemic regions or proven contact with infected animals [14]. The geographic dispersion and rapid increase in cases indicate substantial person-to-person transmission rather than infection from an animal reservoir [14]. Additionally, this is the first significant outbreak of mpoxv that is MSM-dominated and has a significant sexually associated transmission route [14,34]. It has also been discovered in Nigeria that mpoxv is likely more predominant in young males and is also linked to genital lesions [14,21,35].
By March 2023, more than 86,000 people have been infected with mpoxv worldwide, among which more than 98% of infections have occurred in places that have not experienced transmission of this disease before. Over 100 people have died since the large outbreak of mpoxv in May 2022 [7]. In the USA, there have been over 30,000 cases (with 38 death cases), with the largest number of infections since the beginning of the large epidemics in 2022 [8]. The majority of those infected are MSM [14].
Mpox, a disease caused by mpoxv, spreads from person to person through close contact with respiratory secretions or skin sores from an infected individual [47]. Transmission via respiratory droplets requires prolonged face-to-face contact [47], which makes healthcare professionals, household members, and other close contacts of active cases especially vulnerable [47]. The longest documented chain of transmission has increased from 6 to 9 person-to-person infections. This could be attributed to a general decline in immunity due to the cessation of smallpox vaccination. Transmission can also occur from mother to fetus via the placenta or during close contact during or after birth [47]. The extent to which mpox can be transmitted via sexual contact is still unclear. More research is required to understand this risk fully. Mpoxv is a zoonotic virus that spreads to humans by direct contact with infected animals' blood, body fluids, or cutaneous or mucosal lesions [47]. The disease has an incubation period of two to four weeks [14,21,47].
A considerable number of epidemic models have been developed and employed to understand mpoxv transmission; see, for instance, [4,12,14,21,29,34,35,36,37,38,46,51,52,53] and the references therein. In particular, Yuan et al. [51] analyzed the transmission dynamics of mpoxv in metropolitan municipalities. Vaccination was incorporated into the model to examine its impact on mpoxv transmission at large gatherings [52]. In addition, Endo et al. [14] fitted a dynamic model to empirical sexual partnership and mpox data and revealed that the heavy-tailed sexual partnership distribution could be used to explain the expansion of current mpoxv outbreaks, especially among MSM. An SIR-based model was used by Peter et al. [36] to explore the transmission behavior of mpox. The model was extended by incorporating a fractional order approach [37] to gain more detailed insight into mpoxv transmission. Ward et al. [46] also used a statistical modelling framework taken advantage of contact tracing data to investigate the mpoxv dynamics in the UK. Their results suggested that the mpoxv epidemic peaked in the UK in early July and began to decline afterward. A similar technique was implemented by Musa et al. [29] to investigate the time-varying reproduction number of mpoxv in Nigeria. They found that mpoxv transmission reached an all-time peak in 2022.
Zumla et al. [56] investigated the dynamics of mpoxv transmission outside of endemic areas. They emphasized that effective control of mpoxv requires preventing widespread infection among frontline healthcare workers and the most vulnerable populations. Using a multi-group dynamic model, Yang et al. [49] examined the potential for mpox viral transmission from high-risk groups to the general population. They calculated the effective reproduction number (Reff) to be larger than 3, indicating that mpoxv is highly transmissible in MSM and that exposure to high-risk individuals must be minimized. They indicated that prevention of community transmission is vital to curb the spread of mpoxv. In a cross-sectional study, Hernaez et al. [22] discovered high viral loads in the saliva of most mpox patients using quantitative polymerase chain reaction (PCR), and found that they were associated with the severity of skin lesions and symptoms of systemic disease. To remove mpoxv particles from aerosols that can travel a long distance in the air, new nanofiber filters have been developed [22]. Following the discovery of highly viable viral loads in saliva in most mpox patients and mpoxv DNA in droplets and aerosols, the authors concluded that additional epidemiological studies are required to determine whether respiratory route of infection is relevant to the mpoxv outbreak in 2022. Americo et al.[3] studied the virulence variations of mpoxv clades Ⅰ, Ⅱa, and Ⅱb in a small animal model. They discovered that the clades in the order clade Ⅰ > clade Ⅱa > clade IIb in CAST/EiJ mice exhibit highly significant differences compared to the severity of clinical disease in humans.
Due to the sizable mpoxv outbreaks in non-endemic communities, recommendations from researchers and public health professionals are urgently required to help identify the primary factors causing the outbreaks and to formulate policies for effective control.
Epidemiological modeling has been used widely to study the transmission dynamics of reemerging diseases [1,2,13,15,17,19,20,24]. To better comprehend the factors behind the recent rapid spread of mpoxv, we used a dynamic model in this work to imitate the mpoxv outbreaks and analyze the transmission behaviour. The model extends earlier ones (such as [37]) by taking into account several stages of transmission, such as different infection phases, hospitalization, and individuals who do (or do not) adhere to non-pharmaceutical intervention measures (NPIs).
The remainder of this work is organized as follows. In Section 2, an epidemic model is presented. The model is theoretically analyzed in Section 3. We give numerical results in Section 4, and end the paper with a brief discussion and conclusions in Section 5.
We retrieved the daily epidemiological cases data of the mpoxv epidemic for the USA, as reported by the Centers for Disease Control Prevention (CDC) [7,8] from May 10 through December 14, 2022, following laboratory confirmation and case definition of mpoxv. We used the data to calculate the daily cumulative incidence and examined several mpoxv incidence scenarios for the USA.
This study uses a traditional SEIR-based model to examine the transmission dynamics and evaluate control measures for the mpoxv epidemic. It describes the epidemiological dynamics of mpoxv transmission. To more accurately analyze the transmission behavior of mpoxv, the proposed model accounts for both those who adhere to and do not adhere to NPI measures. The model explicitly distinguishes between the various susceptible and infectious stages to assess the overall transmission dynamics of mpoxv outbreaks. The parameter ρ represents the fraction of newly recruited individuals who comply with NPI measures, while 1−ρ represents those who do not comply. The model also considers isolation, which is a critical intervention strategy in mpox prevention and control. Several modeling studies have investigated the impact of isolation in suppressing disease transmission (in which infected people are taken into hospitals or isolation centers to prevent large community outbreaks); see, for example, [32,40], and the references therein.
Our model has the following notable features: (ⅰ) We only consider direct or human-to-human transmission routes since we aimed to access the mpoxv transmission dynamics in the USA, which has the highest cases since the re-emergence of mpox on May 2022. (ⅱ) Rodent populations are not incorporated into the model since it is believed that the mpoxv infections in the USA are due to human-to-human transmission. However, the situation is different from sub-Saharan Africa, where mpoxv is endemic, and most of the transmission is zoonotic (i.e., animal-to-human transmission). (ⅲ) Our model extends earlier ones (such as [37,53]) by taking into account several stages of transmission, such as different infection phases and hospitalization, as well as individuals who adhere (or do not adhere) to basic NPIs (described as high-risk and low-risk populations) to gain a better insight into the overall mpoxv transmission dynamics in the USA and beyond.
We separate the total population of humans at time t, denoted by N(t), into susceptible persons who do not comply with NPIs, Sn(t); susceptible persons who comply with NPIs, Sy(t); exposed persons, E(t); infectious persons who do not comply with NPIs, In(t); infectious persons who comply with NPIs, Iy(t); isolated persons, J(t); and recovered persons, R(t), so that N(t)=Sn(t)+Sy(t)+E(t)+In(t)+Iy(t)+J(t)+R(t).
Figure 1 shows an overview of the mpoxv model and Table 1 lists the state variables and parameters, which satisfy the following system of nonlinear ordinary differential equations:
dSndt=(1−ρ)π−λSn−(θ+μ)Sn,dSydt=ρπ+θSn−ϵλSy−μSy,dEdt=λ(Sn+ϵSy)−(σn+σy+μ)E,dIndt=σnE−(η+γn+τn+δn+μ)In,dIydt=σyE+ηIn−(γy+τy+δy+μ)Iy,dJdt=γnIn+γyIy−(τJ+δJ+μ)J,dRdt=τnIn+τyIy+τJJ−μR. | (1) |
The model's force of infection is formulated as follows:
λ=β(In+αIy)N. | (2) |
Note that 0<α<1 and 0<ϵ<1 represent alteration parameters for the reduction of infectiousness and efficacy for compliance of NPIs, respectively.
Variable | Description |
N | Total humans population |
Sn | Susceptible individuals who do not comply with NPIs |
Sy | Susceptible individuals who comply with NPIs |
E | Exposed individuals |
In | Symptomatically infectious individuals who do not comply with NPIs |
Iy | Symptomatically infectious individuals who do comply with NPIs |
J | Isolated individuals |
R | Recovered individuals |
parameter | |
π | Recruitment rate |
ρ | Fraction of newly recruited persons to a class of individuals who comply with NPIs measures |
μ | Natural death rate |
β | Transmission/contact rate |
α | Modification parameter for the reduction of infectiousness |
θ | Rate of NPIs compliance from Sn to Sy |
ϵ | Efficacy of NPIs compliance in preventing mpoxv infection |
σn, σy | Progression rates |
η | Rate of NPIs compliance from In to Iy |
γn, γy | Isolation/hospitalization rates |
τn, τy, τJ | Recovery rates |
δn, δy, δJ | Mpoxv induced death rates |
Since the model (1) examines the dynamics of mpoxv infection in humans, all of its state variables and parameters are presumptively positive. To study the fundamental analytic characteristics of model (1), we must first take into account the rate of change of the entire human population, N (N′(t), the prime here denotes the derivative with respect to time), which is calculated as follows.
dNdt=π−μN−δnIn−δyIy−δJIJ⩽π−μN. | (3) |
For the mpoxv model (1) to be biologically reasonable, all state variables must be positive at time t>0. In other words, solutions of model (1) with positive initial data will remain positive for all time t>0.
Lemma 2.1. Let the initial data Θ(0)≥0, where Θ(t)=(Sn,Sy,E,In,Iy,J,R). Then the solutions Υ(t) of model (1) are positive for all t>0.
Proof. Let t1=sup{t>0:Θ(t)>0∈[0,t]}. Thus, t1>0. Since π and 1−ρ are positive, it follows from the first equation of the model (1) that
dSndt≥−(λ(t)+θμ)Sn. |
Following the comparison theorem in conjunction with the separation of variables method [23,39,50], we have
Sn(t1)≥Sn(0)exp[−(∫t10λ(u)du+(θ+μ)t1)]>0. |
Hence, Sn(t)>0. Similarly, it can be shown that the remaining components of Θ(t), i.e., Sy,E,In,Iy,J,R are all positive for all t>0. Hence, Θ(t)>0 for all t>0.
The following biological feasible region is considered:
Ω={(Sn,Sy,E,In,Iy,J,R)∈R7+:N⩽πμ}. |
Since N is positive, to ensure that any solutions of the system that start in the region Ω remain in Ω for all non-negative time t, it is sufficient to look at solutions that are limited to Ω, which is positively-invariant. Therefore, the results for a normal existence, uniqueness, and continuity will be satisfied for model (1) per earlier works [23,39,50].
Disease-free equilibrium (DFE) of model (1), denoted by Γ0, is obtained by setting all the equations of the right-hand side of model (1) to zero, that is dSndt=dSydt=dEdt=dIndt=dIydt=dJdt=dRdt=0. This yields S0n=(1−ρ)πθ+μ, S0y=ρπ+θS0nμ, E0=I0n=I0y=J∗=R0=0. The DFE for model (1) is given by
Γ0={S0n,S0y,E0,I0n,I0y,J0,R0}={(1−ρ)πθ+μ,π(ρμ+θ)μ(θ+μ),0,0,0,0,0}. | (4) |
Here, we use the next-generation matrix (NGM) method, which was introduced by van den Driessche & Watmough in [45], to calculate model's basic reproduction number R0, which is the number of secondary cases that a typical primary case would produce in a fully susceptible population during the infectious period [10,32,44,45]. Using the NGM technique, the matrices F, which stands for the new infection terms, and V, which stands for the other transfer terms are calculated and provided below. Note that the linear stability Γ0 is calculated using (4).
F=[λ0(S0n+ϵS0y)000] andV=[A1E0−σnE0+A2I0n−σyE0−ηI0n+A3I0y−γnI0n−γyI0y+A4J0], |
where A1=σn+σy+μ, A2=η+γn+τn+δn+μ, A3=γy+τy+δy+μ, and A4=τJ+δJ+μ. Consequently, the mpoxv infection and transition matrices are computed as follows:
F=[0B1B20000000000000] andV=[A1000−σnA200−σy−ηA300−γn−γyA4]. |
Therefore, the R0 is now computed as
R0=ρ(FV−1)=B1σnA3+B2(ησn+σyA2)A1A2A3, | (5) |
with ρ characterising the spectral radius of the NGM, B1=β(S0n+ϵS0y)N0, and B2=βα(S0n+ϵS0y)N0.
Therefore, based on the Theorem in [45], we established the following results about local stability of the DFE of model (1).
Theorem 3.1. The DFE of model (1) is locally-asymptotically stable whenever R0<1 and unstable if R0>1.
A value R0<1 indicates that a small number of mpoxv cases cannot lead to a large outbreak. For mpoxv containment measures in the epidemic model (1), making R0<1 necessary. Thus, whenever R0<1, mpoxv can be eradicated over time, while mpoxv persistence is continuous for R0>1. Therefore, adequate NPI measures are required to mitigate the disease effectively.
When mpoxv infiltrates a community, at least one infected compartment is not empty. Following some algebraic manuplulation, we obtain an endemic equilibrium (EE) state by setting the vector field of the system (1) to zero. Thus, the EE is now given by
Γ∗={S∗n,S∗y,E∗,I∗n,I∗y,J∗,R∗}. | (6) |
The following equations give the EE points in terms of λ∗.
S∗∗n=(1−ρ)πλ∗+μ,S∗∗y=π(((1−ρ))θ+λ∗ρ+μρ)(ϵλ∗+μ)(λ∗+μ),E∗∗=λ∗π(((1−ρ))ϵλ∗+((1−ρ))ϵθ+ϵλ∗ρ+ϵμρ+((1−ρ))μ)A1(λ∗+μ)(ϵλ∗+μ),I∗n=λ∗π(((1−ρ))ϵλ∗+((1−ρ))ϵθ+ϵλ∗ρ+ϵμρ+((1−ρ))μ)σnA1A2(λ∗+μ)(ϵλ∗+μ),I∗y=(ησn+A2σy)λ∗π(((1−ρ))ϵλ∗+((1−ρ))ϵθ+ϵλ∗ρ+ϵμρ+((1−ρ))μ)A3A1A2(λ∗+μ)(ϵλ∗+μ),J∗=(ηγyσn+A2γyσy+A3γnσn)λ∗π(((1−ρ))ϵλ∗+((1−ρ))ϵθ+ϵλ∗ρ+ϵμρ+((1−ρ))μ)A4A3A1A2(λ∗+μ)(ϵλ∗+μ),R∗=[λ∗π(((1−ρ))ϵλ∗+((1−ρ))ϵθ+ϵλ∗ρ+ϵμρ+((1−ρ))μ)μA4A3A1A2(λ∗+μ)(ϵλ∗+μ)]×(ηA4σnτy+ηγyσnτJ+A2A4σyτy+A2γyσyτJ+A3A4σnτn+A3γnσnτJ). | (7) |
Epidemiologically, the existence of the EE state indicates that at least one of the infected classes in the model is not empty, which shows that the mpoxv spreads and persists in the community.
In this section, we examine the model's interior feasible region, which converges to the singular EE, denoted by Γ∗ (6), whenever R0>1. The mpoxv will spread and remain in a population at Γ∗. By driving a Lyapunov function for a model, we demonstrate the overall stability of the EE. This method has been used extensively in earlier works [27,39,50].
Theorem 3.2. The EE Γ∗ is globally asymptotically stable (GAS) in the region Ω whenever R0>1 under a specific condition (see Appendix A).
Appendix A contains a proof of the aforementioned Theorem 3.2. In addition, in Appendix B, a bifurcation phenomenon is analyzed to further assess the dynamics of the model with respect to R0.
We obtained reported mpoxv outbreak data for the USA from the Centers for Disease Prevention and Control (CDC) [8] and fitted the mpoxv model(1) to the data using Pearson's Chi-square test and the least-squares framework, implemented in the R statistical software (version 4.1.1) [23,54].
In the numerical fitting results, the daily and cumulative numbers of mpoxv cases from May 10, 2022, through December 14, 2022, were used to fit the mpoxv model. As shown in Figure 2, the results revealed that the model (1) fitted the cumulative and the daily number of reported cases of mpoxv well, indicating that the model can be used to explain the transmission behavior of the mpoxv dynamics, since it reproduces the patterns of real data on the mpox situation in the USA. The parameter values used to fit the model are given in Table 2, with N(0)=3.35374570×108 million [9,48], Sn(0)=2.5×108, Sy(0)=0.8×108, E=800, In(0)=5, Iy(0)=1, J(0)=0, R(0)=0.
parameter | Baseline (Range) | Units | Sources |
N | 3.35374570×108(3×108 - 3.5×108) | persons | [48] |
π | 12,074(10,000 - 14,000) | persons per day | [9,48] |
ρ | 0.8(0 - 1) | per day | estimated [25] |
μ | 3.6×10−5(1.1×10−5 - 5.2×10−5) | per day | [9,48] |
β | 0.1565147(0.01 - 0.5) | per day | fitted |
α | 0.75(0 - 1) | per day | [51] |
θ | 0.025(0.01 - 0.75) | per day | estimated [26] |
ϵ | 0.72(0 - 1) | per day | assumed |
σn | 0.3204619(0.21 - 0.87) | per day | fitted |
σy | 0.6729062(0.21 - 0.87) | per day | fitted |
η | 0.03486687(0.01 - 0.05) | per day | fitted |
γn | 0.2(0.1 - 0.5) | per day | [51] |
γy | 0.04(0.03 - 0.05) | per day | [51] |
τn | 0.048(0.001 - 0.075) | per day | [51] |
τy | 0.05(0.001 - 0.075) | per day | [51] |
τJ | 0.056(0.001 - 0.075) | per day | [51] |
δn | 0.0171(0.001 - 0.025) | per day | [51] |
δy | 0.0011(0.001 - 0.025) | per day | [51] |
δJ | 0.001(0.001 - 0.025) | per day | [51] |
This section presents various numerical results for the proposed mpoxv model using parameters from Table 2 and different initial conditions for the model's state variables. The model (1) was simulated using the R statistical software to examine its dynamics by varying the values of the transmission rate parameter, which is one of the most crucial parameters in the model and needs to be minimized to control the spread of mpoxv effectively [14,15,17,21,26,33,42,50]. Figure 3(a)–(d) presents the simulation results. In Figure 3, we used the initial value of β, whereas, in Figure 3(c) and (d), we simulated the model by decreasing the values of β by 25%, 50%, and 75%, respectively, to examine the corresponding dynamics of In and Iy in each case.
In epidemiological modeling studies, the basic reproduction number R0 is a crucial parameter that determines whether the disease will continue to spread (persist) or die out in a community when an epidemic occurs [16]. In the current model, we used R0 and the infection attack rate as response functions to determine the partial rank correlation coefficient (PRCC) for sensitivity analysis to find the most sensitive biological parameters in the model that require maximum attention for mitigation and control [16,32,43,55]. Our sensitivity analysis results show that the parameters β (transmission/contact rate), α1, and ϵ are the most sensitive, followed by γn and τy. The PRCC of the basic reproduction number, R0, and the infection attack rate of the model (1) are presented with the estimated parameters in Figure 4.
Following the previous approach [17,33,39,42,50], we provide some numerical examples in this section to demonstrate the global asymptotic stability of the analytical results for the model (1) as provided in the appendix A. All the parameters have the same biological meaning as in Table 2. In Figure 5, we consider the case when the transmission rate parameter β=0.157, so that R0 = 0.78686606<1, while the other parameters have the same values as given in Table 2, with different initial conditions for the model's state variables. The dynamics of the model (1) with R0<1 are presented in Figure 5(a)–(d), which show that the system (1) has an EE, which is GAS whenever R0<1. In Figure 6, we consider the case when the transmission rate parameter β=0.257, so that R0=1.28805464>1, while the other parameters have the same values as given in Table 2, with different initial conditions for the model's state variables. The dynamics of the model (1) with R0>1 are presented in Figure 6(a)–(d) which show that the system (1) has an EE which is unstable whenever R0>1. These results support the theoretical result stated in Theorem 3.1.
Mpox is primarily a zoonotic viral disease that has caused recurrent outbreaks in West Africa and is occasionally transmitted to other regions [47]. It is transmitted to humans through contact with an infected individual or animal, respiratory droplets, or contaminated materials such as bedding [14]. The recent global outbreaks of mpoxv in non-endemic countries have caused substantial public health concern [7]. Although the vaccine used for smallpox is currently in use against mpoxv infection, due to inadequate vaccination levels, the public need to follow basic health precautions to protect themselves and others from mpoxv infection [47].
In this work, we developed a classical SEIR-based model to assess the transmission dynamics of mpoxv epidemics in the USA. The model was fitted successfully to the reported mpoxv cases and relevant demographic data in the USA. Thus, it can be used to explain the transmission of mpoxv and provide suggestions for optimal control and prevention of the disease. The theoretical and epidemiological outcomes of this work are summarized below.
i) Results on the basic reproduction number of the model were determined analytically and were used to assess the impact of mpoxv control strategies. In addition, subsequent mathematical analysis revealed that the DFE of the model is GAS whenever the R0 value is below or equal to unity and is unstable when the R0 is above unity. Further mathematical analysis showed that the EE is GAS whenever R0 is above unity, indicating the potential for mpoxv to spread and persist in a population. Moreover, we analyzed the forward bifurcation property of the model, which occurs under certain conditions (see Appendix B for more detail). Epidemiologically, the existence of forward bifurcation in the model indicates that the stability of the model exchanges between DFE and EE at R0=1.
Hence, the stability behavior of the model changes (from stable to unstable) around the endemic equilibrium when the bifurcation parameter βhh changes [11,17].
ii) The model prediction results provided in Section 4.1 using the daily number of reported mpoxv cases in the USA for the period from May 10 to December 14, 2022, show that the model fitted well to the mpoxv case data, highlighting the applicability of the model to the wave of mpoxv outbreaks.
iii) Numerical results were provided in Section 4.2 to examine the dynamics of the model (1) by decreasing the value of the transmission rate parameter β by 25%, 50%, and 75%, in turn, for each of the variables In and Iy. We chose the parameter β since it is one the most crucial parameters in the model and needs to be minimized to effectively control the spread of mpoxv. The results show that the transmission of mpoxv can be significantly reduced if the transmission rate parameter can be decreased as much as possible. This could be achieved if most members of the public complied with NPIs and if governments and other relevant organizations provided adequate medical resources, especially to the most vulnerable communities, to respond to epidemics effectively.
iv) The basic reproduction number and infection attack rates were used as response functions to perform sensitivity analysis of the model (presented in Section 4.3). Partial rank correlation coefficients (PRCC) were used for the sensitivity analysis, which showed that the top three PRCC ranked parameters are the transmission rate, the parameter representing the efficacy of compliance with NPIs in preventing mpoxv infection, and the modification parameter for the reduction of infectiousness. Other parameters with high PRCCs suggesting high priority (although not as high as the top three) include the isolation rate from In and recovery rate from Iy. Hence, this study has identified the parameters that should be targeted for effective mpoxv control and prevention.
v) Further numerical results were provided in Section 4.4 to investigate the global stability of the EE for the model (1). The results are illustrated in Figures 5 and 6 and support the theoretical results obtained in 3.2, which revealed that the EE is GAS when R0>1.
In summary, this work employed an SEIR-based model to study and theoretivcally analyze the mpoxv transmission dynamics in the US to shed light on the transmission of mpoxv epidemics, taking into account the roles of NPIs on the overall transmission dynamics. We revealed some vital epidemiological parameters that should be emphasized efforts to mitigate and control of mpoxv. Based on our results, we also observed that the transmission rate and other relevant parameters are crucial in controlling the spread of mpoxv outbreaks. The results in this paper could play an essential role in developing a long-term plan for controlling mpoxv epidemics, especially for researchers and policymakers. Moreover, our findings could provide an essential reference for policymakers to contain the virus's spread effectively. The model could be extended in the future by incorporating other control vital factors such as pharmaceuticals, human behavior, and seasonality to gain more insight into the transmission of mpoxv.
Part of this research was funded and supported by the Deanship of Scientific Research at Imam Mohammad Ibn Saud Islamic University (IMSIU) through Research Partnership Program with number: RP-21-09-07.
The authors declare no conflict of interest.
In this section, we prove Theorem 3.2.
Proof. To prove Theorem 3.2, we adopted previous technique [39,41,50], and designed a Lyapunov function given below.
ℷ(t)=ι1(Sn−S∗n−S∗nlnSnS∗n)+ι2(Sy−S∗y−S∗ylnSyS∗y)+ι3(E−E∗−E∗lnEE∗)+ι4(In−I∗n−I∗nlnInI∗n)+ι5(Iy−I∗y−I∗ylnIyI∗y)+ι6(J−J∗−J∗lnJJ∗). | (A-1) |
Therefore, the derivative of the Lyapunov function (A-1) calculated along solutions of the model (1) is given by
˙ℷ(t)=ι1(1−S∗nSn)˙Sn+ι2(1−S∗ySy)˙Sy+ι3(1−E∗E)˙E+ι4(1−I∗nIn)˙In+ι5(1−I∗yIy)˙Iy+ι6(1−J∗J)˙J. | (A-2) |
By direct computation from Eq (A-2), we have
ι1(1−S∗nSn)˙Sn=ι1(1−S∗nSn)(π(1−ρ)−λSn−A5Sn)=ι1(1−S∗nSn)(λ∗S∗n+A5S∗n−λSn−A5Sn)=ι1λ∗S∗n(1−S∗nSn)(1−λSnλ∗S∗n)−ι1A5(Sn−S∗n)2Sn≤ι1λ∗S∗n(1−λSnλ∗S∗n−S∗nSn+λλ∗), | (A-3) |
and,
ι2(1−S∗ySy)˙Sy=ι2(1−S∗ySy)(πρ+θSn−ϵλSy−μhSy)=ι2(1−S∗ySy)(ϵλ∗S∗y+μhS∗y−ϵλSy−μhSy)=ι2ϵλ∗S∗y(1−S∗ySy)(1−λSyλ∗S∗y)−ι2μh(Sy−S∗y)2Sy≤ι2ϵλ∗S∗y(1−λSyλ∗S∗y−S∗ySy+λλ∗), | (A-4) |
and,
ι3(1−E∗E)˙E=ι3(1−E∗E)(λSn+ϵλSy−A1E)=ι3(1−E∗E)(λSn+ϵλSy−(λ∗S∗n+ϵλ∗S∗y)EE∗)=ι3λ∗S∗n(1−E∗E)(λSnλ∗S∗n−EE∗)+ι3ϵλ∗S∗y(1−E∗E)(λSyλ∗S∗y−EE∗)=ι3λ∗S∗n(λSnλ∗S∗n−EE∗−λSnE∗λ∗S∗nE+1)+ι3ϵλ∗S∗y(λSyλ∗S∗y−EE∗−λSyE∗λ∗S∗yE+1), | (A-5) |
and,
ι4(1−I∗nIn)˙In=ι4(1−I∗nIn)(σnE−A2In)=ι4(1−I∗nIn)(σnE−σnE∗InI∗n)=ι4σnE∗(1−I∗nIn)(EE∗−InI∗n)=ι4σnE∗(EE∗−InI∗n−I∗nEInE∗+1), | (A-6) |
similarly,
ι5(1−I∗yIy)˙Iy==ι5σyE∗(EE∗−IyI∗y−E∗IyEI∗y+1)+ι5ηI∗n(InI∗n−IyI∗y−InI∗yI∗nIy+1), | (A-7) |
and,
ι6(1−J∗J)˙J==ι6γnI∗n(InI∗n−JJ∗−InJ∗I∗nJ+1)+ι6γyI∗y(IyI∗y−JJ∗−IyJ∗I∗yJ+1), | (A-8) |
Substituting ι1=ι2=ι3=ι5=ι6=1, ι4=λ∗S∗n+ϵλ∗Sy∗σnE∗ and Eqs (A-3)–(A-8) into Eq (A-2), we have
˙ℷ(t)≤λ∗S∗n(2−S∗nSn−EE∗−λSnE∗λ∗S∗nE+λλ∗)+ϵλ∗S∗y(2−S∗ySy−EE∗−λSyE∗λ∗S∗yE+λλ∗)+λ∗S∗n(EE∗−InI∗n−I∗nEInE∗+1)+ϵλ∗S∗y(EE∗−InI∗n−I∗nEInE∗+1)+σyE∗(EE∗−IyI∗y−EI∗yE∗Iy+1)+ηI∗n(InI∗n−IyI∗y−InI∗yI∗nIy+1)+γnI∗n(InI∗n−JJ∗−InJ∗I∗nJ+1)+γyI∗y(IyI∗y−JJ∗−IyJ∗I∗yJ+1). | (A-9) |
Suppose, a function is define as u(x)=1−x+lnx, then, if x>0 it leads to u(x)≤0. Also, if x=1, then u(x)=0. Implies that x−1≥ln(x) for any x>0 [28,50]. By using the above definition, direct calculation from Eq (A-8), and conditions (ⅰ) and (ⅱ), we have
(2−S∗nSn−EE∗−λSnE∗λ∗S∗nE+λλ∗)=(−(1−λλ∗)(1−Inλ∗I∗nλ)+3−S∗nSn−λSnE∗λ∗S∗nE−Inλ∗I∗nλ−EE∗+InI∗n)≤(−(S∗nSn−1)−(λSnE∗λ∗S∗nE−1)−(Inλ∗I∗nλ−1)−EE∗+InI∗n)≤(−ln(S∗nSnλSnE∗λ∗S∗nEInλ∗I∗nλ)−EE∗+InI∗n)=(InI∗n−ln(InI∗n)+ln(EE∗)−EE∗). | (A-10) |
Similarly
(2−S∗ySy−EE∗−λSyE∗λ∗S∗yE+λλ∗)≤(InI∗n−ln(InI∗n)+ln(EE∗)−EE∗). | (A-11) |
Also from Eq (A-7), we have
EE∗−InI∗n−I∗nEInE∗+1=(u(I∗nEInE∗)+EE∗−ln(EE∗)−InI∗n+ln(InI∗n))≤EE∗−ln(EE∗)+ln(InI∗n)−InI∗n. | (A-12) |
Similarly,
EE∗+IyI∗y−I∗yEIyE∗+1≤−IyI∗y+ln(IyI∗y)+ln(EE∗)−EE∗. | (A-13) |
InI∗n+IyI∗y−I∗yInIyI∗n+1≤−IyI∗y+ln(IyI∗y)+ln(InI∗n)−InI∗n. | (A-14) |
InI∗n+JJ∗−J∗InJI∗n+1≤−JJ∗+ln(JJ∗)+ln(InI∗n)−InI∗n. | (A-15) |
IyI∗y+JJ∗−J∗IyJI∗y+1≤−JJ∗+ln(JJ∗)+ln(IyI∗y)−IyI∗y. | (A-16) |
Hence,
˙ℷ(t)≤λ∗S∗n(InI∗n−ln(InI∗n)+ln(EE∗)−EE∗)+ϵλ∗S∗y(InI∗n−ln(InI∗n)+ln(EE∗)−EE∗)+λ∗S∗n(EE∗−ln(EE∗)+ln(InI∗n)−InI∗n)+ϵλ∗S∗y(EE∗−ln(EE∗)+ln(InI∗n)−InI∗n)+σyE∗(−IyI∗y+ln(IyI∗y)+ln(EE∗)−EE∗)+ηI∗n(−IyI∗y+ln(IyI∗y)+ln(InI∗n)−InI∗n)+γ∗nI∗n(−JJ∗+ln(JJ∗)+ln(InI∗n)−InI∗n)+γ∗yI∗y(−JJ∗+ln(JJ∗)+ln(IyI∗y)−IyI∗y). | (A-17) |
Equations (A-3)–(A-17) and conditions (ⅰ) (1−λλ∗)(1−Inλ∗I∗nλ)≥0, (ⅱ) IyI∗y+EE∗≥ln(IyI∗y)+ln(EE∗), IyI∗y+InI∗n≥ln(IyI∗y)+ln(InI∗n), JJ∗+InI∗n≥ln(JJ∗)+ln(InI∗n), and JJ∗+IyI∗y≥ln(JJ∗)+ln(IyI∗y), ensure that ˙ℷ(t)≤0. Further, the equality dℷdt=0 holds only if Sn=S∗n, Sy=S∗y E=E∗, In=I∗n, Iy=I∗y, and J=J∗. Thus, the EE state (B-1), is the only positive invariant set to the system (1) contained entirely in {(Sn,Sy,E,In,Iy,J)∈Ω:Sn=S∗n,Sy=S∗y,E=E∗,In=I∗n,Iy=I∗y,J=J∗}. Hence, it follows from LaSalle's invariance principle [27] that every solution to the Eq (1) with initial conditions in Ω converge to EE points, Γ∗, as t→∞. Thus, the positive EE is globally asymptotically stable.
The bifurcation analysis is carried out using center manifold theory (CMT), as presented by Castillo-Chavez and Song [6]. The conditions for the parameter values in the model (1) that result in forward or backward bifurcation are investigated. This approach has been widely used in earlier works, for example, [5,6,18,31,45]. When R0 crosses unity from below, there is a forward bifurcation, which results in the loss of stability of the DFE and the appearance of a small positive asymptotically stable equilibrium [6].
Theorem 5.1. The mpoxv model (1) undergoes forward bifurcation at R0=1 whenever the bifurcation coefficients, A and B, are negative and positive, respectively.
Proof. Following [5,6,30,45], we employ CMT. First, we let dxdt=f(x,Ξ), where Ξ is the bifurcation parameter, and f is continuously differentiable at least twice with respect to both x and Ξ. The DFE (Γ0) is the point (x0=0,Ξ=0) and the local stability of Γ0 changes at the point (x0,0) [45]. We define a nontrivial equilibrium near the bifurcation point (x0,Ξ).
Suppose that β=β∗ is chosen as a bifurcation parameter and we let R0=1 from (3). Then, by Theorem 5.1, the DFE Γ0, is locally stable when β<β∗ and unstable when β>β∗. Here β=β∗ is a bifurcation value.
For convenience, let Sn=x1, Sy=x2, E=x3, In=x4, Iy=x5, J=x6 and R=x7, so that N=x1+x2+x3+x4+x5+x6+x7. Further, by adopting the same vector notation with x=(x1,x2,...,x7)T, the model (1) can be written as dxdt=f(x) where f=(f1,f2,...,f7)T is as follows:
f1=(1−ρ)π−λx1−A5x1,f2=πρ+θx1−ϵλx2−μx2,f3=λ(x1+ϵx2)−A1x3,f4=σnx3−A2x4,f5=σyx3+ηx4−A3x5,f6=γnx4+γyx5−A4x6,f7=τnx4+τyx5+τJx6−μx7, | (B-1) |
where the associated forces of infection are respectively given by
λ=β(x4+αx5)7∑i=1xi. | (B-2) |
The Jacobian matrix of the system (B-2) (computed from the relation J(Γ0)=F−V, where F and V are infection and transition matrices, respectively), evaluated at the DFE (Γ0) with β=β∗, is given by
J(Γ0)=[−A000−c1−c400θ−μ0−c2−c50000−A1c3c60000σn−A200000σyη−A300000γnγy−A40000τnτyτj−μ], | (B-3) |
where
c1=A5βμμ3ρ+θ(2ρ+1)μ2+((ρ+2)θ2+A5)μ+θ3, c2=ϵ(μρ+θ)(θ+μ)2βμ3ρ+θ(2ρ+1)μ2+((ρ+2)θ2+A5)μ+θ3,
c3=β(ϵπ(μρ+θ)(θ+μ)μ+πA5θ+μ)(πA5θ+μ+π(μρ+θ)(θ+μ)μ)−1, c4=A5βαμμ3ρ+θ(2ρ+1)μ2+((ρ+2)θ2+A5)μ+θ3,
c5=ϵ(μρ+θ)(θ+μ)2βαμ3ρ+θ(2ρ+1)μ2+((ρ+2)θ2+A5)μ+θ3, and c6=βα(ϵπ(μρ+θ)(θ+μ)μ+πA5θ+μ)(πA5θ+μ+π(μρ+θ)(θ+μ)μ)−1.
The Jacobian matrix J(Γ0) of the system (B-2) has a simple zero eigenvalue (other eigenvalues have negative real parts). Hence, the CMT [6,45] can be employed to analyse the dynamics of the system (B-2) located at β=β∗ [6]. The subsequent computations are carried out using similar notations as in [6].
Eigenvectors of J(Γ0)β=β∗: For the case when R0=1, it can be shown that the J(Γ0) has a right eigenvector (corresponding to the zero eigenvalues), given by Ψ=(Ψ1,Ψ2,...,Ψ7)T, where
Ψ1=A3c1σn+c5A2σy+n5ησnA0A2A3Ψ3,Ψ2=θA3c1σn+θc5A2σy+θc5ησn+A0A3c2σn+A0c5A2σy+A0c5ησnμA0A2A3Ψ3,Ψ3>0,Ψ4=σnA2Ψ3,Ψ5=A2σy+ησnA2A3Ψ3,Ψ6=A3γnσn+γyA2σy+γyησnA2A3A4Ψ3,Ψ7=A3A4τnσn+A4τyA2σy+A4τyησn+τJA3γnσn+τJγyA2σy+τJγyησnμA2A3A4Ψ3. | (B-4) |
Similarly, the components of the left eigenvector of J(Γ0) (corresponding to the zero eigenvalue), denoted by Φ=(Φ1,Φ2,...,Φ7), are given by
Φ1=0,Φ2=0,Φ3>0,Φ4=c3(σnη−A2σy)−η(σnc3−A1A2)A2(σnη−A2σy)Φ3,Φ5=(σnc3−A1A2)σnη−A2σyΦ3,Φ6=0,Φ7=0. | (B-5) |
Note that the free components (entry) are chosen to be Φ3=1 and Ψ3=1A1+A2 respectively, where
A1=1+σnA2+(c3(σnη−A2σy)−η(σnc3−A1A2)A2(σnη−A2σy))andA2=σnc3−A1A2σnη−A2σyA2σy+ησnA2A3, | (B-6) |
so that, v⋅w=1 (in line with [6]).
It can be shown, by computing the non-zero partial derivatives of fi(i=1,...,7), that the associated bifurcation coefficients, A and B, are given, respectively, by
A=7∑k,i,j=1ΦkΨiΨj∂2fk(0,0)∂xi∂xj=−2A0[ρU1μ3+2U1(ρ+12)θμ2+((ρ+2)U1θ2−A5U2)μ+U1θ3](αΨ5+Ψ4)μβΦ3π(μ3ρ+θ(2ρ+1)μ2+((ρ+2)θ2+A5)μ+θ3)2, | (B-7) |
B=7∑k,i=1ΦkΨi∂2fk(0,0)∂xi∂β=(Ψ4+αΨ5)(ϵμ3ρ+2ϵμ2ρθ+ϵμρθ2+ϵμ2θ+2ϵμθ2+ϵθ3+μA5)Φ3μ3ρ+2μ2ρθ+μρθ2+μ2θ+2μθ2+θ3+μA5, | (B-8) |
where U1=(Ψ1+Ψ3+Ψ4+Ψ5+Ψ6+Ψ7)ϵ−Ψ1 and U2=ϵΨ2−Ψ2−Ψ3−Ψ4−Ψ5−Ψ6−Ψ7. Now, since Φ3>0, Ψ3>0, Ψ4>0, and Ψ5>0, indicating that B≥0, therefore, forward bifurcation occurs if and only if A<0 else we have backward bifurcation (i.e. when A>0). To verify the above results numerically, we computed the expression using the parameter values in Table 2 and found that A (−651.5562000) and B (5.585740031) were negative and positive, respectively. As a result, forward bifurcation is likely for the mpoxv model (1) at R0=1. Furthermore, an EE will not exist if R0<1, leaving DFE as the only local attractor. However, if R0>1, the EE exists. Therefore, a forward bifurcation likely exists because disease prevalence is an increasing function of R0 in the vicinity of the bifurcation point.
[1] |
N. Ahmed, A. Elsonbaty, A. Raza, M. Rafiq, W. Adel, Numerical simulation and stability analysis of a novel reaction-diffusion COVID-19 model, Nonlinear Dyn., 106 (2021), 1293–1310. http://dx.doi.org/10.1007/s11071-021-06623-9 doi: 10.1007/s11071-021-06623-9
![]() |
[2] |
N. Ahmed, M. Rafiq, W. Adel, H. Rezazadeh, I. Khan, K. Nisar, Structure preserving numerical analysis of HIV and CD4+ T-cells reaction diffusion model in two space dimensions, Chaos Soliton. Fract., 139 (2020), 110307. http://dx.doi.org/10.1016/j.chaos.2020.110307 doi: 10.1016/j.chaos.2020.110307
![]() |
[3] |
J. Americo, P. Earl, B. Moss, Virulence differences of mpox (monkeypox) virus clades Ⅰ, Ⅱa, and Ⅱb. 1 in a small animal model, PNAS, 120 (2023), 2220415120. http://dx.doi.org/10.1073/pnas.2220415120 doi: 10.1073/pnas.2220415120
![]() |
[4] |
S. Bankuru, S. Kossol, W. Hou, P. Mahmoudi, J. Rychtář, D. Taylor, A game-theoretic model of Monkeypox to assess vaccination strategies, PeerJ, 8 (2020), 9272. http://dx.doi.org/10.7717/peerj.9272 doi: 10.7717/peerj.9272
![]() |
[5] | J. Carr, Applications of centre manifold theory, New York: Springer, 1981. http://dx.doi.org/10.1007/978-1-4612-5929-9 |
[6] |
C. Castillo-Chavez, B. Song, Dynamical models of tuberculosis and their applications, Math. Biosci. Eng., 1 (2004), 361–404. http://dx.doi.org/10.3934/mbe.2004.1.361 doi: 10.3934/mbe.2004.1.361
![]() |
[7] | 2022 Mpox outbreak global map, Centers for Disease Control and Prevention, 2023. Available from: https://www.cdc.gov/poxvirus/monkeypox/response/2022/world-map.html |
[8] | 2022 outbreak cases and data, Centers for Disease Control and Prevention, 2023. Available from: https://www.cdc.gov/poxvirus/monkeypox/response/2022/index.html |
[9] | Life expectancy in the US dropped for the second year in a row in 2021, Centers for Disease Control and Prevention, 2022. Available from: https://www.cdc.gov/nchs/pressroom/nchs_press_releases/2022/20220831.htm |
[10] |
O. Diekmann, J. Heesterbeek, J. Metz, On the definition and the computation of the basic reproduction ratio R0 in models for infectious diseases in heterogeneous populations, J. Math. Biol., 28 (1990), 365–382. http://dx.doi.org/10.1007/BF00178324 doi: 10.1007/BF00178324
![]() |
[11] | B. Dubey, P. Dubey, U. Dubey, Dynamics of an SIR model with nonlinear incidence and treatment rate, Appl. Appl. Math., 10 (2015), 5. |
[12] |
A. El-Mesady, A. Elsonbaty, W. Adel, On nonlinear dynamics of a fractional order monkeypox virus model, Chaos Soliton. Fract., 164 (2022), 112716. http://dx.doi.org/10.1016/j.chaos.2022.112716 doi: 10.1016/j.chaos.2022.112716
![]() |
[13] |
A. Elsonbaty, Z. Sabir, R. Ramaswamy, W. Adel, Dynamical analysis of a novel discrete fractional SITRS model for COVID-19, Fractals, 29 (2021), 2140035. http://dx.doi.org/10.1142/S0218348X21400351 doi: 10.1142/S0218348X21400351
![]() |
[14] |
A. Endo, H. Murayama, S. Abbott, R. Ratnayake, C. Pearson, W. Edmunds, et al., Heavy-tailed sexual contact networks and monkeypox epidemiology in the global outbreak, Science, 378 (2022), 90–94. http://dx.doi.org/10.1126/science.add4507 doi: 10.1126/science.add4507
![]() |
[15] |
S. Garba, A. Gumel, M. Bakar, Backward bifurcations in dengue transmission dynamics, Math. Biosci., 215 (2008), 11–25. http://dx.doi.org/10.1016/j.mbs.2008.05.002 doi: 10.1016/j.mbs.2008.05.002
![]() |
[16] |
D. Gao, Y. Lou, D. He, T. Porco, Y. Kuang, G. Chowell, et al., Prevention and control of Zika as a mosquito-borne and sexually transmitted disease: a mathematical modeling analysis, Sci. Rep., 6 (2016), 28070. http://dx.doi.org/10.1038/srep28070 doi: 10.1038/srep28070
![]() |
[17] |
I. Ghosh, P. Tiwari, J. Chattopadhyay, Effect of active case finding on dengue control: implications from a mathematical model, J. Theor. Biol., 464 (2019), 50–62. http://dx.doi.org/10.1016/j.jtbi.2018.12.027 doi: 10.1016/j.jtbi.2018.12.027
![]() |
[18] |
A. Gumel, Causes of backward bifurcations in some epidemiological models, J. Math. Anal. Appl., 395 (2012), 355–365. http://dx.doi.org/10.1016/j.jmaa.2012.04.077 doi: 10.1016/j.jmaa.2012.04.077
![]() |
[19] | H. Gunerhan, H. Rezazadeh, W. Adel, M. Hatami, K. Sagayam, H. Emadifar, et al., Analytical approximate solution of fractional order smoking epidemic model, Adv. Mech. Eng., in press. http://dx.doi.org/10.1177/16878132221123888 |
[20] |
H. Günerhan, H. Dutta, M. Dokuyucu, W. Adel, Analysis of a fractional HIV model with Caputo and constant proportional Caputo operators, Chaos Soliton. Fract., 139 (2020), 110053. http://dx.doi.org/10.1016/j.chaos.2020.110053 doi: 10.1016/j.chaos.2020.110053
![]() |
[21] |
C. Happi, I. Adetifa, P. Mbala, R. Njouom, E. Nakoune, A. Happi, et al., Urgent need for a non-discriminatory and non-stigmatizing nomenclature for monkeypox virus, PLoS Biol., 20 (2022), 3001769. http://dx.doi.org/10.1371/journal.pbio.3001769 doi: 10.1371/journal.pbio.3001769
![]() |
[22] |
B. Hernaez, A. Muñoz-Gómez, A. Sanchiz, E. Orviz, A. Valls-Carbo, I. Sagastagoitia, et al., Monitoring monkeypox virus in saliva and air samples in Spain: a cross-sectional study, The Lancet Microbe, 4 (2023), 21–28. http://dx.doi.org/10.1016/S2666-5247(22)00291-9 doi: 10.1016/S2666-5247(22)00291-9
![]() |
[23] |
N. Hussaini, K. Okuneye, A. Gumel, Mathematical analysis of a model for zoonotic visceral leishmaniasis, Infect. Dis. Model., 2 (2017), 455–474. http://dx.doi.org/10.1016/j.idm.2017.12.002 doi: 10.1016/j.idm.2017.12.002
![]() |
[24] |
M. Izadi, S. Yüzbaşı, W. Adel, Accurate and efficient matrix techniques for solving the fractional Lotka-Volterra population model, Physica A, 600 (2022), 127558. http://dx.doi.org/10.1016/j.physa.2022.127558 doi: 10.1016/j.physa.2022.127558
![]() |
[25] |
A. Khan, M. Naveed, M. Dur-e-Ahmad, M. Imran, Estimating the basic reproductive ratio for the Ebola outbreak in Liberia and Sierra Leone, Infect. Dis. Poverty, 4 (2015), 13. http://dx.doi.org/10.1186/s40249-015-0043-3 doi: 10.1186/s40249-015-0043-3
![]() |
[26] |
N. Hussaini, M. Winter, A. Gumel, Qualitative assessment of the role of public health education program on HIV transmission dynamics, Math. Med. Biol., 28 (2011), 245–270. http://dx.doi.org/10.1093/imammb/dqq009 doi: 10.1093/imammb/dqq009
![]() |
[27] | J. La Salle, The stability of dynamical systems, Philadelphia: SIAM, 1976. |
[28] |
Q. Lin, S. Musa, S. Zhao, D. He, Modeling the 2014–2015 Ebola virus disease outbreaks in Sierra Leone, Guinea, and Liberia with effect of high-and low-risk susceptible individuals, Bull. Math. Biol., 82 (2020), 102. http://dx.doi.org/10.1007/s11538-020-00779-y doi: 10.1007/s11538-020-00779-y
![]() |
[29] |
S. Musa, Z. Abdullahi, S. Zhao, U. Bello, N. Hussaini, A. Habib, et al., Transmission dynamics of Monkeypox virus in Nigeria during the current COVID-19 pandemic and estimation of effective reproduction number, Vaccines, 10 (2022), 2153. http://dx.doi.org/10.3390/vaccines10122153 doi: 10.3390/vaccines10122153
![]() |
[30] |
S. Musa, N. Hussaini, S. Zhao, D. He, Dynamical analysis of chikungunya and dengue co-infection model, Discrete Cont. Dyn.-B, 25 (2020), 1907–1933. http://dx.doi.org/10.3934/dcdsb.2020009 doi: 10.3934/dcdsb.2020009
![]() |
[31] |
S. Musa, A. Yusuf, E. Bakare, Z. Abdullahi, L. Adamu, U. Mustapha, et al., Unravelling the dynamics of Lassa fever transmission with differential infectivity: Modeling analysis and control strategies, Math. Biosci. Eng., 19 (2022), 13114–13136. http://dx.doi.org/10.3934/mbe.2022613 doi: 10.3934/mbe.2022613
![]() |
[32] |
S. Musa, S. Zhao, D. Gao, Q. Lin, G. Chowell, D. He, Mechanistic modelling of the large-scale Lassa fever epidemics in Nigeria from 2016 to 2019, J. Theor. Biol., 493 (2020), 110209. http://dx.doi.org/10.1016/j.jtbi.2020.110209 doi: 10.1016/j.jtbi.2020.110209
![]() |
[33] |
L. Nkamba, T. Manga, F. Agouanet, M. Mann Manyombe, Mathematical model to assess vaccination and effective contact rate impact in the spread of tuberculosis, J. Biol. Dyn., 13 (2019), 26–42. http://dx.doi.org/10.1080/17513758.2018.1563218 doi: 10.1080/17513758.2018.1563218
![]() |
[34] |
D. Ogoina, M. Iroezindu, H. James, R. Oladokun, A. Yinka-Ogunleye, P. Wakama, et al., Clinical course and outcome of human Monkeypox in Nigeria, Clin. Infect. Dis., 71 (2020), 210–214. http://dx.doi.org/10.1093/cid/ciaa143 doi: 10.1093/cid/ciaa143
![]() |
[35] |
D. Ogoina, J. Izibewule, A. Ogunleye, E. Ederiane, U. Anebonam, A. Neni, et al., The 2017 human monkeypox outbreak in Nigeria-report of outbreak experience and response in the Niger Delta University Teaching Hospital, Bayelsa State, Nigeria, PLoS. One, 14 (2019), 0214229. http://dx.doi.org/10.1371/journal.pone.0214229 doi: 10.1371/journal.pone.0214229
![]() |
[36] |
O. Peter, S. Kumar, N. Kumari, F. Oguntolu, K. Oshinubi, R. Musa, Transmission dynamics of Monkeypox virus: a mathematical modelling approach, Model. Earth Syst. Environ., 8 (2022), 3423–3434. http://dx.doi.org/10.1007/s40808-021-01313-2 doi: 10.1007/s40808-021-01313-2
![]() |
[37] |
O. Peter, F. Oguntolu, M. Ojo, A. Oyeniyi, R. Jan, I. Khan, Fractional order mathematical model of monkeypox transmission dynamics, Phys. Scr., 97 (2022), 084005. http://dx.doi.org/10.1088/1402-4896/ac7ebc doi: 10.1088/1402-4896/ac7ebc
![]() |
[38] |
J. Riopelle, V. Munster, J. Port, Atypical and unique transmission of monkeypox virus during the 2022 outbreak: an overview of the current state of knowledge, Viruses, 14 (2022), 2012. http://dx.doi.org/10.3390/v14092012 doi: 10.3390/v14092012
![]() |
[39] |
P. Roop-O, W. Chinviriyasit, S. Chinviriyasit, The effect of incidence function in backward bifurcation for malaria model with temporary immunity, Math. Biosci., 265 (2015), 47–64. http://dx.doi.org/10.1016/j.mbs.2015.04.008 doi: 10.1016/j.mbs.2015.04.008
![]() |
[40] |
M. Safi, A. Gumel, Qualitative study of a quarantine/isolation model with multiple disease stages, Appl. Math. Comput., 218 (2011), 1941–1961. http://dx.doi.org/10.1016/j.amc.2011.07.007 doi: 10.1016/j.amc.2011.07.007
![]() |
[41] |
Z. Shuai, P. van den Driessche, Global stability of infectious disease models using Lyapunov functions, SIAM J. Appl. Math., 73 (2013), 1513–1532. http://dx.doi.org/10.1137/120876642 doi: 10.1137/120876642
![]() |
[42] |
G. Sun, J. Xie, S. Huang, Z. Jin, M. Li, L. Liu, Transmission dynamics of cholera: mathematical modeling and control strategies, Commun. Nonlinear Sci., 45 (2017), 235–244. http://dx.doi.org/10.1016/j.cnsns.2016.10.007 doi: 10.1016/j.cnsns.2016.10.007
![]() |
[43] |
C. Trotter, N. Gay, W. Edmunds, Dynamic models of meningococcal carriage, disease, and the impact of serogroup C conjugate vaccination. Am. J. Epidemiol., 162 (2005), 89–100. http://dx.doi.org/10.1093/aje/kwi160 doi: 10.1093/aje/kwi160
![]() |
[44] |
P. van den Driessche, Reproduction numbers of infectious disease models, Infectious Disease Modelling, 2 (2017), 288–303. http://dx.doi.org/10.1016/j.idm.2017.06.002 doi: 10.1016/j.idm.2017.06.002
![]() |
[45] |
P. van den Driessche, J. Watmough, Reproduction numbers and sub-threshold endemic equilibria for compartmental models of disease transmission, Math. Biosci., 180 (2002), 29–48. http://dx.doi.org/10.1016/S0025-5564(02)00108-6 doi: 10.1016/S0025-5564(02)00108-6
![]() |
[46] |
T. Ward, R. Christie, R. Paton, F. Cumming, C. Overton, Transmission dynamics of monkeypox in the United Kingdom: contact tracing study, BMJ, 379 (2022), 073153. http://dx.doi.org/10.1136/bmj-2022-073153 doi: 10.1136/bmj-2022-073153
![]() |
[47] | Monkeypox, World Health Organization, 2022. Available from: https://www.who.int/news-room/fact-sheets/detail/monkeypox?gclid = CjwKCAjws–ZBhAXEiwAv-RNL3T7SFlwhw8Ew8-flekFNnaO9DZaJgz0ybxgg7xwzsIRYi_VAYCb1hoC1OIQAvD_BwE |
[48] | Population of the United States (2020 and historical), Worldometer, 2022. Available from: https://www.worldometers.info/world-population/us-population/. |
[49] |
S. Yang, X. Guo, Z. Zhao, B. Abudunaibi, Y. Zhao, J. Rui, et al., Possibility of mpox viral transmission and control from high-risk to the general population: a modeling study, BMC Infect. Dis., 23 (2023), 119. http://dx.doi.org/10.1186/s12879-023-08083-5 doi: 10.1186/s12879-023-08083-5
![]() |
[50] |
C. Yang, X. Wang, D. Gao, J. Wang, Impact of awareness programs on cholera dynamics: two modeling approaches, Bull. Math. Biol., 79 (2017), 2109–2131. http://dx.doi.org/10.1007/s11538-017-0322-1 doi: 10.1007/s11538-017-0322-1
![]() |
[51] |
P. Yuan, Y. Tan, L. Yang, E. Aruffo, N. Ogden, J. Bélair, et al., Assessing transmission risks and control strategy for monkeypox as an emerging zoonosis in a metropolitan area, J. Med. Virol., 95 (2023), 28137. http://dx.doi.org/10.1002/jmv.28137 doi: 10.1002/jmv.28137
![]() |
[52] |
P. Yuan, Y. Tan, L. Yang, E. Aruffo, N. Ogden, J. Belair, et al., Modelling vaccination and control strategies for outbreaks of monkeypox at gatherings, Front. Public Health, 10 (2022), 1026489. http://dx.doi.org/10.3389/fpubh.2022.1026489 doi: 10.3389/fpubh.2022.1026489
![]() |
[53] |
M. Al-Shomrani, S. Musa, A. Yusuf, Unfolding the transmission dynamics of monkeypox virus: an epidemiological modelling analysis, Mathematics, 11 (2023), 1121. http://dx.doi.org/10.3390/math11051121 doi: 10.3390/math11051121
![]() |
[54] |
Z. Yuan, S. Musa, S. Hsu, C. Cheung, D. He, Post pandemic fatigue: what are effective strategies? Sci. Rep., 12 (2022), 9706. http://dx.doi.org/10.1038/s41598-022-13597-0 doi: 10.1038/s41598-022-13597-0
![]() |
[55] |
S. Zhao, L. Stone, D. Gao, D. He, Modelling the large-scale yellow fever outbreak in Luanda, Angola, and the impact of vaccination, PLoS Neglect. Trop. Dis., 12 (2018), 0006158. http://dx.doi.org/10.1371/journal.pntd.0006158 doi: 10.1371/journal.pntd.0006158
![]() |
[56] |
A. Zumla, S. Valdoleiros, N. Haider, D. Asogun, F. Ntoumi, E. Petersen, et al., Monkeypox outbreaks outside endemic regions: scientific and social priorities, Lancet Infect. Dis., 22 (2022), 929–931. http://dx.doi.org/10.1016/S1473-3099(22)00354-1 doi: 10.1016/S1473-3099(22)00354-1
![]() |
1. | Wilfredo Meza Cuba, Juan Carlos Huaman Alfaro, Hasnain Iftikhar, Javier Linkolk López-Gonzales, Modeling and Analysis of Monkeypox Outbreak Using a New Time Series Ensemble Technique, 2024, 13, 2075-1680, 554, 10.3390/axioms13080554 | |
2. | Walter Okongo, Jeconia Okelo Abonyo, Duncan Kioi, Stephen Edward Moore, Stanley Nnaemeka Aguegboh, Mathematical modeling and optimal control analysis of Monkeypox virus in contaminated environment, 2024, 10, 2363-6203, 3969, 10.1007/s40808-024-01987-4 |
Variable | Description |
N | Total humans population |
Sn | Susceptible individuals who do not comply with NPIs |
Sy | Susceptible individuals who comply with NPIs |
E | Exposed individuals |
In | Symptomatically infectious individuals who do not comply with NPIs |
Iy | Symptomatically infectious individuals who do comply with NPIs |
J | Isolated individuals |
R | Recovered individuals |
parameter | |
π | Recruitment rate |
ρ | Fraction of newly recruited persons to a class of individuals who comply with NPIs measures |
μ | Natural death rate |
β | Transmission/contact rate |
α | Modification parameter for the reduction of infectiousness |
θ | Rate of NPIs compliance from Sn to Sy |
ϵ | Efficacy of NPIs compliance in preventing mpoxv infection |
σn, σy | Progression rates |
η | Rate of NPIs compliance from In to Iy |
γn, γy | Isolation/hospitalization rates |
τn, τy, τJ | Recovery rates |
δn, δy, δJ | Mpoxv induced death rates |
parameter | Baseline (Range) | Units | Sources |
N | 3.35374570×108(3×108 - 3.5×108) | persons | [48] |
π | 12,074(10,000 - 14,000) | persons per day | [9,48] |
ρ | 0.8(0 - 1) | per day | estimated [25] |
μ | 3.6×10−5(1.1×10−5 - 5.2×10−5) | per day | [9,48] |
β | 0.1565147(0.01 - 0.5) | per day | fitted |
α | 0.75(0 - 1) | per day | [51] |
θ | 0.025(0.01 - 0.75) | per day | estimated [26] |
ϵ | 0.72(0 - 1) | per day | assumed |
σn | 0.3204619(0.21 - 0.87) | per day | fitted |
σy | 0.6729062(0.21 - 0.87) | per day | fitted |
η | 0.03486687(0.01 - 0.05) | per day | fitted |
γn | 0.2(0.1 - 0.5) | per day | [51] |
γy | 0.04(0.03 - 0.05) | per day | [51] |
τn | 0.048(0.001 - 0.075) | per day | [51] |
τy | 0.05(0.001 - 0.075) | per day | [51] |
τJ | 0.056(0.001 - 0.075) | per day | [51] |
δn | 0.0171(0.001 - 0.025) | per day | [51] |
δy | 0.0011(0.001 - 0.025) | per day | [51] |
δJ | 0.001(0.001 - 0.025) | per day | [51] |
Variable | Description |
N | Total humans population |
Sn | Susceptible individuals who do not comply with NPIs |
Sy | Susceptible individuals who comply with NPIs |
E | Exposed individuals |
In | Symptomatically infectious individuals who do not comply with NPIs |
Iy | Symptomatically infectious individuals who do comply with NPIs |
J | Isolated individuals |
R | Recovered individuals |
parameter | |
π | Recruitment rate |
ρ | Fraction of newly recruited persons to a class of individuals who comply with NPIs measures |
μ | Natural death rate |
β | Transmission/contact rate |
α | Modification parameter for the reduction of infectiousness |
θ | Rate of NPIs compliance from Sn to Sy |
ϵ | Efficacy of NPIs compliance in preventing mpoxv infection |
σn, σy | Progression rates |
η | Rate of NPIs compliance from In to Iy |
γn, γy | Isolation/hospitalization rates |
τn, τy, τJ | Recovery rates |
δn, δy, δJ | Mpoxv induced death rates |
parameter | Baseline (Range) | Units | Sources |
N | 3.35374570×108(3×108 - 3.5×108) | persons | [48] |
π | 12,074(10,000 - 14,000) | persons per day | [9,48] |
ρ | 0.8(0 - 1) | per day | estimated [25] |
μ | 3.6×10−5(1.1×10−5 - 5.2×10−5) | per day | [9,48] |
β | 0.1565147(0.01 - 0.5) | per day | fitted |
α | 0.75(0 - 1) | per day | [51] |
θ | 0.025(0.01 - 0.75) | per day | estimated [26] |
ϵ | 0.72(0 - 1) | per day | assumed |
σn | 0.3204619(0.21 - 0.87) | per day | fitted |
σy | 0.6729062(0.21 - 0.87) | per day | fitted |
η | 0.03486687(0.01 - 0.05) | per day | fitted |
γn | 0.2(0.1 - 0.5) | per day | [51] |
γy | 0.04(0.03 - 0.05) | per day | [51] |
τn | 0.048(0.001 - 0.075) | per day | [51] |
τy | 0.05(0.001 - 0.075) | per day | [51] |
τJ | 0.056(0.001 - 0.075) | per day | [51] |
δn | 0.0171(0.001 - 0.025) | per day | [51] |
δy | 0.0011(0.001 - 0.025) | per day | [51] |
δJ | 0.001(0.001 - 0.025) | per day | [51] |