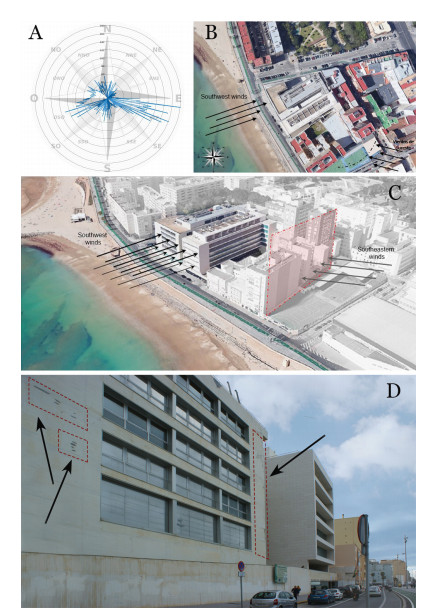
Alzheimer's disease (AD) is one of the most common neurodegenerative diseases and its onset is significantly associated with genetic factors. Being the capabilities of high specificity and accuracy, genetic testing has been considered as an important technique for AD diagnosis. In this paper, we presented an improved deep learning (DL) algorithm, namely differential genes screening TabNet (DGS-TabNet) for AD binary and multi-class classifications. For performance evaluation, our proposed approach was compared with three novel DLs of multi-layer perceptron (MLP), neural oblivious decision ensembles (NODE), TabNet as well as five classical machine learnings (MLs) including decision tree (DT), random forests (RF), gradient boosting decision tree (GBDT), light gradient boosting machine (LGBM) and support vector machine (SVM) on the public data set of gene expression omnibus (GEO). Moreover, the biological interpretability of global important genetic features implemented for AD classification was revealed by the Kyoto encyclopedia of genes and genomes (KEGG) and gene ontology (GO). The results demonstrated that our proposed DGS-TabNet achieved the best performance with an accuracy of 93.80% for binary classification, and with an accuracy of 88.27% for multi-class classification. Meanwhile, the gene pathway analyses demonstrated that there existed two most important global genetic features of AVIL and NDUFS4 and those obtained 22 feature genes were partially correlated with AD pathogenesis. It was concluded that the proposed DGS-TabNet could be used to detect AD-susceptible genes and the biological interpretability of susceptible genes also revealed the potential possibility of being AD biomarkers.
Citation: Yu Jin, Zhe Ren, Wenjie Wang, Yulei Zhang, Liang Zhou, Xufeng Yao, Tao Wu. Classification of Alzheimer's disease using robust TabNet neural networks on genetic data[J]. Mathematical Biosciences and Engineering, 2023, 20(5): 8358-8374. doi: 10.3934/mbe.2023366
[1] | M. Botros, E. A. A. Ziada, I. L. EL-Kalla . Semi-analytic solutions of nonlinear multidimensional fractional differential equations. Mathematical Biosciences and Engineering, 2022, 19(12): 13306-13320. doi: 10.3934/mbe.2022623 |
[2] | Jian Huang, Zhongdi Cen, Aimin Xu . An efficient numerical method for a time-fractional telegraph equation. Mathematical Biosciences and Engineering, 2022, 19(5): 4672-4689. doi: 10.3934/mbe.2022217 |
[3] | Yang Liu, Jie Liu, Tao Yu . Sharp conditions for a class of nonlinear Schrödinger equations. Mathematical Biosciences and Engineering, 2023, 20(2): 3721-3730. doi: 10.3934/mbe.2023174 |
[4] | Muhammad Nadeem, Ji-Huan He, Hamid. M. Sedighi . Numerical analysis of multi-dimensional time-fractional diffusion problems under the Atangana-Baleanu Caputo derivative. Mathematical Biosciences and Engineering, 2023, 20(5): 8190-8207. doi: 10.3934/mbe.2023356 |
[5] | Xiaomeng Ma, Zhanbing Bai, Sujing Sun . Stability and bifurcation control for a fractional-order chemostat model with time delays and incommensurate orders. Mathematical Biosciences and Engineering, 2023, 20(1): 437-455. doi: 10.3934/mbe.2023020 |
[6] | Noura Laksaci, Ahmed Boudaoui, Seham Mahyoub Al-Mekhlafi, Abdon Atangana . Mathematical analysis and numerical simulation for fractal-fractional cancer model. Mathematical Biosciences and Engineering, 2023, 20(10): 18083-18103. doi: 10.3934/mbe.2023803 |
[7] | Ilse Domínguez-Alemán, Itzel Domínguez-Alemán, Juan Carlos Hernández-Gómez, Francisco J. Ariza-Hernández . A predator-prey fractional model with disease in the prey species. Mathematical Biosciences and Engineering, 2024, 21(3): 3713-3741. doi: 10.3934/mbe.2024164 |
[8] | Swadesh Pal, Malay Banerjee, Vitaly Volpert . Spatio-temporal Bazykin’s model with space-time nonlocality. Mathematical Biosciences and Engineering, 2020, 17(5): 4801-4824. doi: 10.3934/mbe.2020262 |
[9] | Xian Zhang, Chen Huang . Existence, multiplicity and non-existence of solutions for modified Schrödinger-Poisson systems. Mathematical Biosciences and Engineering, 2023, 20(2): 3482-3503. doi: 10.3934/mbe.2023163 |
[10] | Debao Yan . Existence results of fractional differential equations with nonlocal double-integral boundary conditions. Mathematical Biosciences and Engineering, 2023, 20(3): 4437-4454. doi: 10.3934/mbe.2023206 |
Alzheimer's disease (AD) is one of the most common neurodegenerative diseases and its onset is significantly associated with genetic factors. Being the capabilities of high specificity and accuracy, genetic testing has been considered as an important technique for AD diagnosis. In this paper, we presented an improved deep learning (DL) algorithm, namely differential genes screening TabNet (DGS-TabNet) for AD binary and multi-class classifications. For performance evaluation, our proposed approach was compared with three novel DLs of multi-layer perceptron (MLP), neural oblivious decision ensembles (NODE), TabNet as well as five classical machine learnings (MLs) including decision tree (DT), random forests (RF), gradient boosting decision tree (GBDT), light gradient boosting machine (LGBM) and support vector machine (SVM) on the public data set of gene expression omnibus (GEO). Moreover, the biological interpretability of global important genetic features implemented for AD classification was revealed by the Kyoto encyclopedia of genes and genomes (KEGG) and gene ontology (GO). The results demonstrated that our proposed DGS-TabNet achieved the best performance with an accuracy of 93.80% for binary classification, and with an accuracy of 88.27% for multi-class classification. Meanwhile, the gene pathway analyses demonstrated that there existed two most important global genetic features of AVIL and NDUFS4 and those obtained 22 feature genes were partially correlated with AD pathogenesis. It was concluded that the proposed DGS-TabNet could be used to detect AD-susceptible genes and the biological interpretability of susceptible genes also revealed the potential possibility of being AD biomarkers.
Atmospheric corrosion of steel embedded in reinforced concrete is the phenomenon that most influences the deterioration of structures, mainly those built in coastal areas due to the effect of marine spray. This is a worldwide problem that is very difficult to solve, and many researchers have raised the need to carry out studies that lead to prevent this phenomenon and its consequences. Real case studies are extremely important to indicate the factors of influence to be considered as variables in these models [1].
It must be taken into account that rebars embedded in concrete could be exposed not only to chlorides present in the concrete itself, but also to chlorides found in the environment that penetrate the concrete, being-in both cases-a durability problem for the building' service life. Additionally, carbonation of concrete or penetration of acidic gases into the concrete is other causes of reinforcement corrosion to consider [2].
The cost of global steel and concrete infrastructures is expected to exceed $300 billion by 2025 [3] and the National Association of Corrosion Engineers estimated the global cost of corrosion around $3 trillion per year, or 3.4% of global GDP [4], clearly demonstrating the need to address the problem scientifically.
As a guide, it should be noted that although the ambient temperature conditions are not particularly aggressive, the humidity and salinity conditions are deemed to be aggressive. The records obtained from Cádiz city observatory, provided by AEMET (State Meteorology Agency of Spain), show that annual temperatures range between 7.5 and 32.10 ℃, averaging 18.52 ℃, and relative humidity varies between 32 and 99%, with a relatively high average of 73.08%. In spite of these environmental conditions, the correct preservation of the reinforced concrete structures is guaranteed as long as the architectural project fulfils with quality and execution requirements indicated in the standards in force.
According to the limited data available (data from the original project could not be obtained), the concrete used is 25 N/mm2, manufactured with type-Ⅱ white cement with limestone addition whose compressive strength was 35 N/mm2. According to the legislation in force when the building was built [5], for concrete exposed to aerial marine environments, the cement content should have been 300 kg/m3, the water/cement ratio 0.55 and the reinforcements should have been arranged at a minimum depth of 40 mm. The knowledge of the thickness of the reinforcement coating is essential to determine the level of protection of the steel and identify potential causes of deterioration, since the barrier effect of the concrete protecting the reinforcement has a decisive impact in the deterioration processes over time.
The lack of coating would require an intervention on the façade that would cause the regeneration or strengthening of the protective barrier layer on the building as a whole. It is important to note that the aesthetic quality of the white concrete exposed to the open air is seriously affected by contamination due to the development of biological stains. In this case, the material has behaved as a low bioreceptivity concrete [6], since it does not present alterations due to colonization by biotic organisms.
In general, the specific conditions that allow chlorides to reach the limit state to initiate corrosion are not clearly established, since this depends on different circumstances, so it was not possible to introduce a single representative value in the models. Although numerous studies have been carried out to determine the critical level of chlorides in the reinforcement capable of initiating corrosion, the suggested range of variation is extremely broad, which makes it difficult to define a single value [7,8,9]. The chloride content in the concrete that reaches the reinforcement depends on the ion diffusivity-linked to the nature of the cement and the compactness of the concrete [10], and the solid geometry determined by the thickness of the reinforced concrete covering and the micro-cracking of the concrete [11].
The corrosion of the reinforcements usually involves two stages. During the first phase, two reactions take place simultaneously: the metal (Fe0) dissolves in certain areas, while at other points a cathodic reaction occurs, e.g., from hydroxyl ions (OH−) if oxygen content is high enough in interstitial water. In the second phase, the dissolved metal ion (Fe++) reacts with the anions in the water to form a precipitate that covers the steel. Observations show that if the concrete is sufficiently sound, i.e. if its pH remains high (pH > 11) and is not contaminated by substances such as chlorides, the coating product on the steel will be sufficiently watertight to prevent water from reaching the metallic surface, since the high alkalinity of the concrete protects the steel [12]. In the presence of chlorides, the passive layer of the steel can no longer be watertight; in this way, the steel corrodes due to dissolution, giving rise to corrosion if chloride content exceeds a certain threshold determined by the pH of the concrete [9].
Taking into account the studied characteristics of the concrete, an increase of w/c ratio contributes to lower chloride thresholds [13], due to the different conditions for the passivation of the reinforcement, higher moisture content and oxygen availability, lower binding capacity and lower hydroxide content in a porous network solution [8].
Taking as a reference the penetration models indicated in Annex 9 of the Code on Structural Concrete (EHE-2008), "Additional considerations on durability" [14], in case of corrosion the total time needed for a significant attack or degradation can be expressed as Eq 1:
tL=ti+tp | (1) |
tL: Total time required for the attack or degradation to become significant.
ti: Time taken by the penetration front of the aggressive agent to reach the reinforcement thereby causing the onset of corrosion.
tp: Corrosion propagation period until the structural elements suffers significant degradation.
Chloride concentration is expressed in % of concrete weight, using the cement content in kg/m3 and the actual density of the concrete. The critical chloride concentration, in accordance with current legislation, is a value of 0.6% of the cement weight for checking the limit state in relation to the corrosion of the passive reinforcements. The limit state concept implies that, if it is exceeded, a structural failure may occur because the project does not reach the end of its useful life as aggressive agents generate a degree of deterioration that prevents the structure from behaving in accordance with the requirements according to which it has been planned [14].
It is well known that carbon dioxide (CO2) contained in air penetrates over time through the porous system of the concrete, combining with calcium hydroxide to form calcium carbonate. This circumstance causes concrete pH to decrease from the outside inward, eventually causing the disappearance of the passivating oxide layer that naturally protects the steel reinforcement. The speed of the process depends on these variables: relative humidity, open porosity, cement dosage and age of the concrete [15]. Therefore, under the action of mainly ambient humidity and oxygen, the effect of corrosion can develop more quickly; even more in the presence of chlorides, as is the case of the marine spray that affects the building studied [16,17].
The object of this study was a building constructed in 1996 on the seafront of Cádiz, a city located in southern Spain, between 50 and 100 m from the sea. The material used in the building envelope was white concrete reinforced with conventional carbon steel reinforcement. This characteristic makes the building unique and represents an added maintenance difficulty since the degradation processes are more evident in white walls. The proximity of the sea and the subsequent action of marine spray and saline condensation on the surface has had an important impact on the evolution and conservation of the façade materials (Figure 1). Thus, undocumented repairs have been carried out in some areas consisting in the application of external mortar coating in highly localized areas.
Some evidence of concrete fragments becoming detached due to reinforcement corrosion (Figure 2), prompting the need for a general study of the building's façades to evaluate the condition of the concrete and the reinforcement making up the exterior construction elements in order to diagnose their current state, forecast the behaviour of the materials and report on the necessary rehabilitation actions.
The purpose of this work was to determine the state of the materials comprising the exposed concrete façade of the building studied by extracting cores from different orientations of the building, determining their density and open porosity, the concrete coatings of the reinforcements, the depth of carbonation and chloride penetration, in order to evaluate the development of corrosion processes, as well as their origin and intensity. This study serves as a useful tool for the technicians entrusted with the maintenance and rehabilitation of the building, supporting decision-making in areas already damaged and in other areas where there are not yet external signs of deterioration.
A general prior analysis of the reinforcement was carried out in the studied areas using a GPR (ground penetrating radar) model GP8800 manufactured by PROCEQ with a defined frequency range between 400–6000 MHz and a penetration capacity for measuring up to 65 cm. The data were processed using Proceq GPR Live software enabling results to be read in situ. GPR is a non-destructive method that uses UHF (Ultra High Frequency) electromagnetic waves designed to detect the internal characteristics of a specific medium and basically responds to the principle of wave reflection in dielectric discontinuities [18]. In this case, it was used to detect the rebar location in the reinforced concrete, areas of discontinuity in reinforcement, lack of reinforcing bars or overlapping reinforcements. Horizontal and vertical façade readings were taken and 3D analyses were performed to obtain a simulated image of the layout and state of the reinforcement [19].
The measurements taken with the GPR were complemented with measurements taken on the surface with the Proetisa Elcometer 331 BH detector to accurately confirm the position and depth of the steel bars and determine average coating thicknesses in the sampled areas and surrounding areas.
To carry out the investigation, in agreement with the technicians responsible for the conservation and maintenance of the building, a total of six cylindrical cores measuring 10 cm in diameter by 15–18 cm in depth were extracted, analysed and tested (Figure 3). They were obtained and stored properly in accordance with the criteria and measures required by the UNE-EN 12504-1 standard [20]. Three two-centimetre-thick slices were cut from each core, from the outside to the inside, in order to characterize the chloride penetration front. The cuts were carried out in a dry state to avoid altering the concentration of soluble salts in the samples [21].
Real density, apparent density and open porosity of the concrete in vacuum conditions were determined in accordance with the UNE-EN 83980 standard [22]. From the extracted cores, samples were taken from the slices cut at different depths (2, 4 and 6 cm), analysing the penetration profile in each sample and chloride concentration by cement weight (Figure 4).
Chloride analysis was performed by ion chromatography on a Metrohm 930 Compact IC Flex (Herisau, Switzerland). For the calculation of the percentage of chlorides in cement weight, "Chlorides in Concrete" CTI Technical Note C2 (September 2002) [23,24] was used as a reference, which considered real density calculated in the porosity test. In accordance with this methodology, the samples were prepared by grinding 50 g of the respective samples to a size of less than 125 µm. These were then quartered to extract an amount of 20 g, which was stirred for 60 min in deionized water at room temperature and subsequently filtered until get a volume of 250 mL.
Mineralogical analysis was carried out by x-ray diffraction (XRD) in a Bruker-AXS model D8I-A25 diffractometer, equipped with a Cu Kα copper filament (λ = 1.5405 Ǻ), with Bragg-Brentano θ-θ configuration, filter nickel and Lynxeye linear detector, using the powder technique; and thermogravimetry (TGA) on a TA Instruments Q600 SDT Differential Scanning Calorimeter.
Finally, the carbonation front of the cores was measured using the phenolphthalein test, in accordance with the UNE 112011 standard [25], and carried out inside the specimens-cores, one for each extraction, previously broken by the generatrix using the indirect traction test, in accordance with the UNE-EN 12390-6 standard [26]. The pH assessment of the cores produces a magenta colour on the concrete if its pH is greater than 8.5 and is colourless if the pH is less than 8. The test was carried out in the laboratory on samples that were packed immediately after extraction and isolated to avoid their superficial carbonation (Figure 5).
Table 1 shows the average coating thicknesses measured in the sampling areas.
Sample | Location | Average thickness (mm) |
1. | Inner courtyard façade (east) | ≥45 |
2. | Main façade (west) | 15–20 |
3. | Main façade (south) | 30–40 |
4. | Exterior ramp façade (west) | 15–20 |
5. | Roof façade (east) | ≥50 |
6. | Roof façade (west) | 15–20 |
The minimum coating value recorded on the construction date of the building was 40 mm [1] Therefore, it is evident that, generally speaking, this parameter has not been fulfilled. These measurements may be one of the potential causes of the alterations present.
The readings taken with the GPR corroborated that the external reinforcement generally consisted of horizontal rebars arranged every 20 cm and vertical rebars every 20–25 cm. However, areas of discontinuity in the horizontal reinforcement were detected due to the lack of reinforcing bars between the different sections of wall executed during construction works. The GPR also allow to identify areas with defective bonding, thus voids were observed in the radargrams. These voids were mainly found on the south-west façade -exposed to greater action of the marine spray- and some of these areas presented an imminent risk of detachment. In addition, approximate measurements of the existing coatings were obtained in each reading, being < 3 cm in most of the areas studied which is less than recommended by national standards at the time of construction (EHE-2008) (Table 1) (Figures 6 and 7).
Figure 6 presents the readings of the vertical radargrams in four areas, showing the following items: (ⅰ) the horizontal rebars from the top reinforcement (hyperbolas with higher contrast) and the bottom reinforcement, (ⅱ) their poor coating, (ⅲ) the wall thickness and (ⅳ) significant alterations like voids (Figure 6B, C) and lack of reinforcement in specific areas (Figure 6D). Additionally, it could be seeing the location of sample 4 (Figure 6B) and sample 2 (Figure 6D), due to the different concrete used to replace the horizontal coring previously extracted.
In the case of Figure 7, it is shown the vertical reinforcement and clearly identify differences in the coatings thickness-this fact is obvious, in the distance between rebars from the same wall section, voids and even double reinforcements, probably due to previous reinforcement interventions.
All the extracted samples could be classified as porous and permeable concretes, unsuitable for exposure to a marine environment as all exceeded 15% porosity. This high porosity may have its origin in a high w/c ratio and poor execution due to insufficient compaction [27,28]. The results obtained for the physical properties of the elements tested are shown in Table 2.
Sample | Real density (g/cm3) | Apparent density (g/cm3) | Open porosity (%) |
1. | 2.65 | 2.15 | 18.8 |
2. | 2.65 | 2.15 | 18.9 |
3. | 2.66 | 2.16 | 19.0 |
4. | 2.77 | 2.22 | 19.7 |
5. | 2.64 | 2.20 | 16.5 |
6. | 2.63 | 2.12 | 19.3 |
It should be noted that the density results obtained are fully consistent values, since the actual density of calcite is 2.71 g/cm3 and concrete is mainly composed of crushed limestone aggregate.
The available data makes impossible to estimate the times indicated in Eq 1 in this building. Although the EHE-08 Code indicates that the critical concentration of chlorides in the reinforcement area is 0.6% by cement weight for passive reinforcements [14]. If this threshold value is taken as a reference, the data obtained in the analysis carried out are clearly well below that value. The results for chloride concentration obtained are shown in Table 3.
Cores | Sample location | Chloride concentration (%) |
1 | 1.1 External sample | 0.039 |
1.2 Intermediate sample | 0.023 | |
1.3 Interior sample | 0.023 | |
2 | 2.1 External sample | 0.144 |
2.2 Intermediate sample | 0.061 | |
2.3 Interior sample | 0.019 | |
3 | 3.1 External sample | 0.250 |
3.2 Intermediate sample | 0.032 | |
3.3 Interior sample | 0.019 | |
4 | 4.1 External sample | 0.181 |
4.2 Intermediate sample | 0.095 | |
4.3 Interior sample | 0.079 | |
5 | 5.1 External sample | 0.058 |
5.2 Intermediate sample | 0.023 | |
5.3 Interior sample | 0.020 | |
6 | 6.1 External sample | 0.081 |
6.2 Intermediate sample | 0.095 | |
6.3 Interior sample | 0.043 |
It is important to highlight that the samples from the south-west and south-east façades had higher chloride contents than the rest. The south-east façade is in a cul-de-sac that favours condensation. While the south-west façade is directly facing the sea, and both are subject to the prevailing winds at this location (Figure 1).
The diffractograms obtained for the samples taken from the exterior and interior areas of the cores did not allow the identification of any mineral phase that can be associated with chlorides (Figure 8), thus making it possible to consider that the soluble salts which have penetrated through the porous system of the material may have reacted with tricalcium aluminate, but not enough chloroaluminate has been produced to develop visible peaks in the XRD spectra.
In this case, it was very important to determine the penetration depth of the carbonation front to confirm if the area where the metal reinforcements were located had been reached. This fact occurs in all samples except in 1. and 5. leading to their depassivation and their subsequent oxidation and expansion due to the formation of iron hydroxides. It was observed that this process is causing cracking in these elements and detachment of fragments of this material, due to the expansion of the products resulting from the corrosion process. The results of the carbonation depth and tests are shown in Table 4.
Sample | Carbonation depth (mm) | Reinforcement coating (mm) |
1. | 20–25 | 40–50 |
2. | 10–15 | 15–20 |
3. | 30 | 30–40 |
4. | 10–15 | 15–20 |
5. | 15–20 | ≥50 |
6. | 20 | 15–20 |
As described by Sanjuan et al. (2015) [29] and considering the characteristic of the concrete used in this case study (CEM Ⅱ cement and a w/c ratio of 0.5), the estimated chloride penetration coefficient (KCl) was 8.05 mm2/year. The most widely used model to evaluate the degree of penetration of chlorine salts in concrete consists in the application of Fick's second law of diffusion of aggressive chlorides through the coating. In this regard, the Eq 2 retrieved from the Annex 9 of the EHE-2008 expresses in a simplified way the relationship between the depth reached by a given concentration of aggressive agent over time and permits calculating the initiation period:
d=KCl⋅√t | (2) |
Equation 2 indicates the time required for critical chloride concentration to be reached at a given distance from the surface of the element by taking into account the chloride diffusion coefficient. According to this value, based on the current age of the building (23 years).The depth reached by the penetration front of Cl− would be 38.6 mm.
Considering in accordance with Annex 9 of the EHE-2008, a limit value of 0.6% in cement weight to check the limit state in relation to the corrosion of passive reinforcements, it can be observed that this value has not been reached in the walls analysed in this building. However, an additional circumstance has seriously conditioned the behaviour of the reinforcements and concrete: the resulting carbonation and the consequent depassivation of reinforcements placed at an insufficient distance from the surface.
If the values shown in Table 4 are analysed, it can be observed that in samples 2, 3, 4 and 6 the carbonation front has gone beyond the position of the reinforcements or limits with said position. Given this circumstance, it may be concluded that. Although chloride concentration values never exceed the service limit state value [30], chloride concentration is sufficient for significant corrosion of the reinforcements in those areas where carbonation has caused depassivation and the consequent lack of protection of the steel elements. Reinforcements were even observed where chloride action has triggered pitting corrosion processes in non-carbonated areas (Figure 9).
Previous studies on the structural concrete cores of the building's façade have revealed the start principle of carbonation, depassivation on the reinforcements and their subsequent corrosion as the main causes of deterioration. These processes derive from, among other factors, the environmental conditions to which the building is exposed, aggravated by the physical characteristics of the concrete and the insufficient thickness of the reinforcement coating in certain areas. It should be noted that the problem detected in this building stems not only from the marine environment, but also from poor execution and poor emplacement of the steel reinforcement bars. So, it is essential in these cases a careful execution following the technical specifications and standards to guarantee the maximum durability of the constructive elements, particularly in the façades because they are more exposed.
The comparative test of properties such as density and porosity allowed us to evaluate their suitability to provide adequate protection, based on our estimates of the factors responsible for the damage. It is important to highlight that it was possible to demonstrate that non-compliance with the requirements stipulated in current regulations has negatively influenced the behaviour and durability of the reinforced concrete elements.
In reinforced concrete structures close to the coast, the action of marine spray is often assumed to be the cause of corrosion of rebars, and carbonation processes are not adequately considered. It has been shown that carbonation depassivation phenomena in concrete greatly accelerate the aggressive action of chlorides. It was confirmed that the action of chlorides -by itself- has not been a determining factor in the degradation of the building's façade. The depassivation of the steel reinforcements, due to the carbonation of the concrete, was confirmed as a necessary factor for the start of the alteration of the reinforcements due to chloride activity.
The study of the carbonation front and the thickness of the coating is an essential tool for technicians that make it easier to establish intervention criteria for the repair of the façade. Based on the scientific and technical results obtained, a proposal for the protection of the façade surfaces can be defined and a waterproofing agent for its application on concrete elements can be selected. These measurements would prevent the humidity penetration and condensation processes, that favour the migration of a greater quantity of chlorides towards the interior. Additionally, a palliative treatment to inhibit corrosion should be considered. Finally, attention must be drawn to the importance of considering a periodic protective maintenance programme, considering the complex environmental conditions to which the building is exposed. In accordance with the products selected for protecting of the façade and the durability indicated by the supplier, the stakeholders and technicians responsible for the retrofitting must establish a maintenance programme that guarantees the effectiveness of the executed work over time.
This research has been carried out thanks to the financing of project PGC2018-093470-B-I00 of the Ministry of Science, Innovation and Universities of the Government of Spain. Authors would like to thank the Ⅵ PPIT-2021-I.3 from the University of Seville and the Research, Technology and Innovation Centre (CITIUS) of the University of Seville, in whose laboratories the characterization analyses were carried out.
All authors declare no conflicts of interest in this paper.
[1] |
X. Liu, D. Hou, F. Lin, J. Luo, J. Xie, Y. Wang, et al., The role of neurovascular unit damage in the occurrence and development of Alzheimer's disease, Rev. Neurosci., 30 (2019), 477–484. https://doi.org/10.1515/revneuro-2018-0056 doi: 10.1515/revneuro-2018-0056
![]() |
[2] |
F. Falahati, E. Westman, A. Simmons, Multivariate data analysis and machine learning in Alzheimer's disease with a focus on structural magnetic resonance imaging, J. Alzheimers Dis., 41 (2014), 685–708. https://doi.org/10.3233/JAD-131928 doi: 10.3233/JAD-131928
![]() |
[3] |
A. B. Sallim, A. A. Sayampanathan, A. Cuttilan, R. Chun-Man Ho, Prevalence of mental health disorders among caregivers of patients with Alzheimer disease, J. Am. Med. Dir. Assoc., 16 (2015), 1034–1041. https://doi.org/10.1016/j.jamda.2015.09.007 doi: 10.1016/j.jamda.2015.09.007
![]() |
[4] |
E. L. G. E. Koedam, V. Lauffer, A. E. van der Vlies, W. M. van der Flier, P. Scheltens, Y. A. L. Pijnenburg, Early-versus late-onset Alzheimer's disease: More than age alone, J. Alzheimers Dis., 19 (2010), 1401–1408. https://doi.org/10.3233/JAD-2010-1337 doi: 10.3233/JAD-2010-1337
![]() |
[5] |
Y. Freudenberg-Hua, W. Li, P. Davies, The role of genetics in advancing precision medicine for Alzheimer's disease—a narrative review, Front. Med., 5 (2018), 108. https://doi.org/10.3389/fmed.2018.00108 doi: 10.3389/fmed.2018.00108
![]() |
[6] |
E. Giacobini, G. Gold, Alzheimer disease therapy—moving from amyloid-β to tau, Nat. Rev. Neurol., 9 (2013), 677–686. https://doi.org/10.1038/nrneurol.2013.223 doi: 10.1038/nrneurol.2013.223
![]() |
[7] |
R. J. Jutten, S. A. M. Sikkes, R. E. Amariglio, R. F. Buckley, M. J. Properzi, G. A. Marshall, et al., Identifying sensitive measures of cognitive decline at different clinical stages of Alzheimer's disease, J. Int. Neuropsychol. Soc., 27 (2021), 426–438. https://doi.org/10.1017/S1355617720000934 doi: 10.1017/S1355617720000934
![]() |
[8] |
D. A. McGrowder, F. Miller, K. Vaz, C. Nwokocha, C. Wilson-Clarke, M. Anderson-Cross, et al., Cerebrospinal fluid biomarkers of Alzheimer's disease: Current evidence and future perspectives, Brain Sci., 11 (2021), 215. https://doi.org/10.3390/brainsci11020215 doi: 10.3390/brainsci11020215
![]() |
[9] |
R. L. Cazzato, J. Garnon, B. Shaygi, G. Koch, G. Tsoumakidou, J. Caudrelier, et al., PET/CT-guided interventions: Indications, advantages, disadvantages and the state of the art, Minimally Invasive Ther. Allied Technol., 27 (2018), 27–32. https://doi.org/10.1080/13645706.2017.1399280 doi: 10.1080/13645706.2017.1399280
![]() |
[10] |
M. Amini, M. M. Pedram, A. Moradi, M. Jamshidi, M. Ouchani, Single and combined neuroimaging techniques for Alzheimer's disease detection, Comput. Intell. Neurosci., 2021 (2021), 9523039. https://doi.org/10.1155/2021/9523039 doi: 10.1155/2021/9523039
![]() |
[11] |
C. E. Wierenga, M. W. Bondi, Use of functional magnetic resonance imaging in the early identification of Alzheimer's disease, Neuropsychol. Rev., 17 (2007), 127–143. https://doi.org/10.1007/s11065-007-9025-y doi: 10.1007/s11065-007-9025-y
![]() |
[12] |
N. J. Gong, C. C. Chan, L. M. Leung, C. S. Wong, R. Dibb, C. Liu, Differential microstructural and morphological abnormalities in mild cognitive impairment and Alzheimer's disease: Evidence from cortical and deep gray matter, Hum. Brain Mapp., 38 (2017), 2495–2508. https://doi.org/10.1002/hbm.23535 doi: 10.1002/hbm.23535
![]() |
[13] |
C. Van Cauwenberghe, C. Van Broeckhoven, K. Sleegers, The genetic landscape of Alzheimer disease: Clinical implications and perspectives, Genet. Med., 18 (2016), 421–430. https://doi.org/10.1038/gim.2015.117 doi: 10.1038/gim.2015.117
![]() |
[14] |
B. L. Romero-Rosales, J. G. Tamez-Pena, H. Nicolini, M. G. Moreno-Treviño, V. Trevino, Improving predictive models for Alzheimer's disease using GWAS data by incorporating misclassified samples modeling, PLoS One, 15 (2020). https://doi.org/10.1371/journal.pone.0232103 doi: 10.1371/journal.pone.0232103
![]() |
[15] |
T. Jo, K. Nho, A. J. Saykin, Deep learning in Alzheimer's disease: diagnostic classification and prognostic prediction using neuroimaging data, Front. Aging Neurosci., 11 (2019). https://doi.org/10.3389/fnagi.2019.00220 doi: 10.3389/fnagi.2019.00220
![]() |
[16] |
J. Ha, MDMF: predicting miRNA-disease association based on matrix factorization with disease similarity constraint, J. Pers. Med., 12 (2022). https://doi.org/10.3390/jpm12060885 doi: 10.3390/jpm12060885
![]() |
[17] |
J. Ha, SMAP: Similarity-based matrix factorization framework for inferring miRNA-disease association, Knowl-Based Syst., 263 (2023). https://doi.org/10.1016/j.knosys.2023.110295 doi: 10.1016/j.knosys.2023.110295
![]() |
[18] |
J. De. Velasco Oriol, E. E. Vallejo, K. Estrada, Benchmarking machine learning models for late-onset Alzheimer's disease prediction from genomic data, BMC Bioinf., 20 (2019), 1–17. https://doi.org/10.1186/s12859-019-3158-x doi: 10.1186/s12859-018-2565-8
![]() |
[19] |
L. Xu, G. Liang, C. Liao, G. D. Chen, C. C. Chang, An efficient classifier for Alzheimer's disease genes identification, Molecules, 23 (2018), 3140. https://doi.org/10.3390/molecules23123140 doi: 10.3390/molecules23123140
![]() |
[20] |
D. Castillo-Barnes, L. Su, J. Ramírez, D. Salas-Gonzalez, F. J. Martinez-Murcia, I. A. Illan, et al., Autosomal dominantly inherited Alzheimer disease: Analysis of genetic subgroups by machine learning, Inf. Fusion, 58 (2020), 153–167. https://doi.org/10.1016/j.inffus.2020.01.001 doi: 10.1016/j.inffus.2020.01.001
![]() |
[21] |
N. Voyle, A. Keohane, S. Newhouse, K. Lunnon, C. Johnston, H. Soininen, et al., A pathway based classification method for analyzing gene expression for Alzheimer's disease diagnosis, J. Alzheimers Dis., 49 (2016), 659–669. https://doi.org/10.3233/JAD-150440 doi: 10.3233/JAD-150440
![]() |
[22] |
E. Moradi, M. Marttinen, T. Häkkinen, M. Hiltunen, M. Nykter, Supervised pathway analysis of blood gene expression profiles in Alzheimer's disease, Neurobiol. Aging, 84 (2019), 98–108. https://doi.org/10.1016/j.neurobiolaging.2019.07.004 doi: 10.1016/j.neurobiolaging.2019.07.004
![]() |
[23] | D. Cheng, M. Liu, Classification of Alzheimer's disease by cascaded convolutional neural networks using PET images, in Machine Leaening in Medical Imaging, Springer, (2017), 106–113. https://doi.org/10.1007/978-3-319-67389-9_13 |
[24] |
M. Grassi, G. Perna, D. Caldirola, K. Schruers, R. Duara, D. A. Loewenstein, A clinically-translatable machine learning algorithm for the prediction of Alzheimer's disease conversion in individuals with mild and premild cognitive impairment, J. Alzheimers Dis., 61 (2018), 1555–1573. https://doi.org/10.3233/JAD-170547 doi: 10.3233/JAD-170547
![]() |
[25] |
S. M. Plis, D. R. Hjelm, R. Salakhutdinov, E. A. Allen, H. J. Bockholt, J. D. Long, et al., Deep learning for neuroimaging: a validation study, Front. Neurosci., 8 (2014). https://doi.org/10.3389/fnins.2014.00229 doi: 10.3389/fnins.2014.00229
![]() |
[26] | S. Wang, H. Wang, Y. Shen, X. Wang, Automatic recognition of mild cognitive impairment and Alzheimers disease using ensemble based 3D densely connected convolutional networks, in 17th IEEE International Conference on Machine Learning and Applications (ICMLA), (2018), 517–523. https://doi.org/10.1109/icmla.2018.00083 |
[27] |
W. Yu, B. Lei, M. K. Ng, A. C. Cheung, Y. Shen, S. Wang, Tensorizing GAN with high-order pooling for Alzheimer's disease assessment, IEEE Trans. Neural Networks Learn. Syst., 33 (2022), 4945–4959. https://doi.org/10.1109/TNNLS.2021.3063516 doi: 10.1109/TNNLS.2021.3063516
![]() |
[28] |
W. Yu, B. Lei, S. Wang, Y. Liu, Z. Feng, Y. Hu, et al., Morphological feature visualization of Alzheimer's disease via multidirectional perception GAN, IEEE Trans. Neural Networks Learn. Syst., 2022 (2022), 1–15. https://doi.org/10.1109/TNNLS.2021.3118369 doi: 10.1109/TNNLS.2021.3118369
![]() |
[29] |
T. Lee, H. Lee, Prediction of Alzheimer's disease using blood gene expression data, Sci. Rep., 10 (2020), 3485. https://doi.org/10.1038/s41598-020-60595-1 doi: 10.1038/s41598-020-60595-1
![]() |
[30] |
N. Mahendran, P. Durai Raj Vincent, A deep learning framework with an embedded-based feature selection approach for the early detection of the Alzheimer's disease, Comput. Biol. Med., 141 (2022), 105056. https://doi.org/10.1016/j.compbiomed.2021.105056 doi: 10.1016/j.compbiomed.2021.105056
![]() |
[31] |
C. Park, J. Ha, S. Park, Prediction of Alzheimer's disease based on deep neural network by integrating gene expression and DNA methylation dataset, Expert Syst. Appl., 140 (2020). https://doi.org/10.1016/j.eswa.2019.112873 doi: 10.1016/j.eswa.2019.112873
![]() |
[32] |
Y. Liu, Z. Li, Q. Ge, N. Lin, M. Xiong, Deep feature selection and causal analysis of Alzheimer's disease, Front. Neurosci., 13 (2019). https://doi.org/10.3389/fnins.2019.01198 doi: 10.3389/fnins.2019.01198
![]() |
[33] |
S. Spasov, L. Passamonti, A. Duggento, P. Liò, N. Toschi, A parameter-efficient deep learning approach to predict conversion from mild cognitive impairment to Alzheimer's disease, NeuroImage, 189 (2019), 276–287. https://doi.org/10.1016/j.neuroimage.2019.01.031 doi: 10.1016/j.neuroimage.2019.01.031
![]() |
[34] |
S. Gauthier, B. Reisberg, M. Zaudig, R. C. Petersen, K. Ritchie, K. Broich, et al., Mild cognitive impairment, Lancet, 367 (2006), 1262–1270. https://doi.org/10.1016/S0140-6736(06)68542-5 doi: 10.1016/S0140-6736(06)68542-5
![]() |
[35] |
M. Grundman, R. C. Petersen, S. H. Ferris, R. G. Thomas, P. S. Aisen, D. A. Bennett, et al., Mild cognitive impairment can be distinguished from Alzheimer disease and normal aging for clinical trials, Arch. Neurol., 61 (2004), 59–66. https://doi.org/10.1001/archneur.61.1.59 doi: 10.1001/archneur.61.1.59
![]() |
[36] | A. Kadra, M. Lindauer, F. Hutter, J. Grabocka, Well-tuned simple nets excel on tabular datasets, 2021. |
[37] | S. Popov, S. Morozov, A. Babenko, Neural oblivious decision ensembles for deep learning on tabular data, arXiv preprint, 2019, arXiv: 1909.06312. https://doi.org/10.48550/arXiv.1909.06312 |
[38] |
C. Shah, Q. Du, Y. Xu, Enhanced TabNet: attentive interpretable tabular learning for hyperspectral image classification, Remote Sens., 14 (2022), 716. https://doi.org/10.3390/rs14030716 doi: 10.3390/rs14030716
![]() |
[39] | Y. Y. Song, Y. Lu, Decision tree methods: applications for classification and prediction, Shanghai Arch Psychiatry, 27 (2015), 130–135. |
[40] |
G. Biau, E. Scornet, A random forest guided tour, TEST, 25 (2016), 197–227. https://doi.org/10.1007/s11749-016-0481-7 doi: 10.1007/s11749-016-0481-7
![]() |
[41] |
C. Zhang, C. Liu, X. Zhang, G. Almpanidis, An up-to-date comparison of state-of-the-art classification algorithms, Expert Syst. Appl., 82 (2017), 128–150. https://doi.org/10.1016/j.eswa.2017.04.003 doi: 10.1016/j.eswa.2017.04.003
![]() |
[42] | G. Ke, Q. Meng, T. Finley, T. Wang, W. Chen, W. Ma, et al., Lightgbm: A highly efficient gradient boosting decision tree, 2017. |
[43] |
M. Pirooznia, J. Y. Yang, M. Q. Yang, Y. Deng, A comparative study of different machine learning methods on microarray gene expression data, BMC Genomics, 9 (2008). https://doi.org/10.1186/1471-2164-9-S1-S13 doi: 10.1186/1471-2164-9-S1-S13
![]() |
[44] |
Q. S. Zhang, S. C. Zhu, Visual interpretability for deep learning: a survey, Front. Inf. Technol. Electron. Eng., 19 (2018), 27–39. https://doi.org/10.1631/FITEE.1700808 doi: 10.1631/FITEE.1700808
![]() |
[45] |
S. Lovestone, P. Francis, I. Kloszewska, P. Mecocci, A. Simmons, H. Soininen, et al., AddNeuroMed-the european collaboration for the discovery of novel biomarkers for Alzheimer's disease, Ann. N. Y. Acad. Sci., 1180 (2009), 36–46. https://doi.org/10.1111/j.1749-6632.2009.05064.x doi: 10.1111/j.1749-6632.2009.05064.x
![]() |
[46] |
S. Sood, I. J. Gallagher, K. Lunnon, E. Rullman, A. Keohane, H. Crossland, et al., A novel multi-tissue RNA diagnostic of healthy ageing relates to cognitive health status, Genome Biol., 16 (2015), 185. https://doi.org/10.1186/s13059-015-0750-x doi: 10.1186/s13059-015-0750-x
![]() |
[47] |
S. Davis, P. S. Meltzer, GEOquery: a bridge between the Gene Expression Omnibus (GEO) and BioConductor, Bioinformatics, 23 (2007), 1846–1847. https://doi.org/10.1093/bioinformatics/btm254 doi: 10.1093/bioinformatics/btm254
![]() |
[48] |
X. Li, H. Wang, J. Long, G. Pan, T. He, O. Anichtchik, et al., Systematic analysis and biomarker study for Alzheimer's disease, Sci. Rep., 8 (2018), 17394. https://doi.org/10.1038/s41598-018-35789-3 doi: 10.1038/s41598-018-35789-3
![]() |
[49] |
A. Antonell, A. Llado, J. Altirriba, T. Botta-Orfila, M. Balasa, M. Fernandez, et al., A preliminary study of the whole-genome expression profile of sporadic and monogenic early-onset Alzheimer's disease, Neurobiol. Aging, 34 (2013), 1772–1778. https://doi.org/10.1016/j.neurobiolaging.2012.12.026 doi: 10.1016/j.neurobiolaging.2012.12.026
![]() |
[50] | S. Arık, T. Pfister, TabNet: Attentive interpretable tabular learning, in Proceedings of the AAAI Conference on Artificial Intelligence, 35 (2021), 6679–6687. https://doi.org/10.1609/aaai.v35i8.16826 |
[51] |
N. N. Parikshak, M. J. Gandal, D. H. Geschwind, Systems biology and gene networks in neurodevelopmental and neurodegenerative disorders, Nat. Rev. Genet., 16 (2015), 441–458. https://doi.org/10.1038/nrg3934 doi: 10.1038/nrg3934
![]() |
[52] | G. K. Smyth, limma: Linear models for microarray data, in Bioinformatics and Computational Biology Solutions Using R and Bioconductor, Springer, (2005), 397–420. https://doi.org/10.1007/0-387-29362-0_23 |
[53] |
C. Garbin, X. Zhu, O. Marques, Dropout vs. batch normalization: an empirical study of their impact to deep learning, Multimedia Tools Appl., 79 (2020), 12777–12815. https://doi.org/10.1007/s11042-019-08453-9 doi: 10.1007/s11042-019-08453-9
![]() |
[54] | Y. N. Dauphin, A. Fan, M. Auli, D. Grangier, Language modeling with gated convolutional networks, in Conference on Machine Learning, (2017), 933–941. |
[55] | J. Gehring, M. Auli, D. Grangier, D. Yarats, Y. N. Dauphin, Convolutional sequence to sequence learning, in Proceedings of the 34th International Conference on Machine Learning, 70 (2017), 1243–1252. |
[56] | A. Martins, R. Astudillo, From softmax to sparsemax: a sparse model of attention and multi-label classification, in Proceedings of the 33rd International Conference on Machine Learning, 48 (2016), 1614–1623. |
[57] |
N. Deepa, S. P. Chokkalingam, Optimization of VGG16 utilizing the Arithmetic Optimization Algorithm for early detection of Alzheimer's disease, Biomed. Signal Process. Control, 74 (2022), 103455. https://doi.org/10.1016/j.bspc.2021.103455 doi: 10.1016/j.bspc.2021.103455
![]() |
[58] |
M. B. Kursa, W. R. Rudnicki, Feature selection with the Boruta package, J. Stat. Software, 36 (2010), 1–13. https://doi.org/10.18637/jss.v036.i11 doi: 10.18637/jss.v036.i11
![]() |
[59] | A. Kulshrestha, O. Farooq, Seizure prediction using fybrid features, in IEEE 7th Uttar Pradesh Section International Conference on Electrical, Electronics and Computer Engineering (UPCON), (2020), 1–6. https://doi.org/10.1109/upcon50219.2020.9376552 |
[60] | R. Martinez-Cantin, Bayesian optimization with adaptive kernels for robot control, in IEEE International Conference on Robotics and Automation (ICRA), (2017), 3350–3356. https://doi.org/10.1109/ICRA.2017.7989380 |
[61] |
T. Wu, E. Hu, S. Xu, M. Chen, P. Guo, Z. Dai, et al., ClusterProfiler 4.0: A universal enrichment tool for interpreting omics data, Innovation, 2 (2021), 100141. https://doi.org/10.1016/j.xinn.2021.100141 doi: 10.1016/j.xinn.2021.100141
![]() |
[62] |
The Gene Ontology Consortium, The gene ontology resource: 20 years and still GOing strong, Nucleic Acids Res., 47 (2019), 330–338. https://doi.org/10.1093/nar/gky1055 doi: 10.1093/nar/gky1055
![]() |
[63] |
J. Krawczuk, T. Łukaszuk, The feature selection bias problem in relation to high-dimensional gene data, Artif. Intell. Med., 66 (2016), 63–71. https://doi.org/10.1016/j.artmed.2015.11.001 doi: 10.1016/j.artmed.2015.11.001
![]() |
[64] |
S. S. Mehta, N. S. Lingayat, Development of SVM based classification techniques for the delineation of wave components in 12-lead electrocardiogram, Biomed. Signal Process. Control, 3 (2008), 341–349. https://doi.org/10.1016/j.bspc.2008.04.002 doi: 10.1016/j.bspc.2008.04.002
![]() |
[65] |
Z. Tümer, P. J. P. Croucher, L. R. Jensen, J. Hampe, C. Hansen, V. Kalscheuer, et al., Genomic structure, chromosome mapping and expression analysis of the human AVIL gene, and its exclusion as a candidate for locus for inflammatory bowel disease at 12q13–14 (IBD2), Gene, 288 (2002), 179–185. https://doi.org/10.1016/S0378-1119(02)00478-X doi: 10.1016/S0378-1119(02)00478-X
![]() |
[66] |
S. Hong, V. F. Beja-Glasser, B. M. Nfonoyim, A. Frouin, S. Li, S. Ramakrishnan, et al., Complement and microglia mediate early synapse loss in Alzheimer mouse models, Science, 352 (2016), 712–716. https://doi.org/10.1126/science.aad8373 doi: 10.1126/science.aad8373
![]() |
[67] |
A. Quintana, S. E. Kruse, R. P. Kapur, E. Sanz, R. D. Palmiter, Complex I deficiency due to loss of Ndufs4 in the brain results in progressive encephalopathy resembling Leigh syndrome, Proc. Natl. Acad. Sci., 107 (2010), 10996–11001. https://doi.org/10.1073/pnas.1006214107 doi: 10.1073/pnas.1006214107
![]() |
[68] |
D. F. F. Silva, A. R. Esteves, C. R. Oliveira, S. M. Cardoso, Mitochondria: the common upstream driver of amyloid-β and tau pathology in Alzheimer's disease, Curr. Alzheimer Res., 8 (2011), 563–572. https://doi.org/10.2174/156720511796391872 doi: 10.2174/156720511796391872
![]() |
[69] |
M. Calabrò, C. Rinaldi, G. Santoro, C. Crisafulli, The biological pathways of Alzheimer disease: a review, AIMS Neurosci., 8 (2021), 86–132. https://doi.org/10.3934/Neuroscience.2021005 doi: 10.3934/Neuroscience.2021005
![]() |
1. | Yasin Asghari, Mostafa Eslami, Mashallah Matinfar, Hadi Rezazadeh, Novel soliton solution of discrete nonlinear Schrödinger system in nonlinear optical fiber, 2024, 90, 11100168, 7, 10.1016/j.aej.2024.01.024 | |
2. | Bo Liu, Zhou-Bo Duan, Li-Fang Niu, Soliton solutions of the TWPA-SNAIL transmission line circuit equation under continuum approximation via the Jacobi elliptic function expansion method, 2024, 98, 0973-7111, 10.1007/s12043-024-02791-6 |
Sample | Location | Average thickness (mm) |
1. | Inner courtyard façade (east) | ≥45 |
2. | Main façade (west) | 15–20 |
3. | Main façade (south) | 30–40 |
4. | Exterior ramp façade (west) | 15–20 |
5. | Roof façade (east) | ≥50 |
6. | Roof façade (west) | 15–20 |
Sample | Real density (g/cm3) | Apparent density (g/cm3) | Open porosity (%) |
1. | 2.65 | 2.15 | 18.8 |
2. | 2.65 | 2.15 | 18.9 |
3. | 2.66 | 2.16 | 19.0 |
4. | 2.77 | 2.22 | 19.7 |
5. | 2.64 | 2.20 | 16.5 |
6. | 2.63 | 2.12 | 19.3 |
Cores | Sample location | Chloride concentration (%) |
1 | 1.1 External sample | 0.039 |
1.2 Intermediate sample | 0.023 | |
1.3 Interior sample | 0.023 | |
2 | 2.1 External sample | 0.144 |
2.2 Intermediate sample | 0.061 | |
2.3 Interior sample | 0.019 | |
3 | 3.1 External sample | 0.250 |
3.2 Intermediate sample | 0.032 | |
3.3 Interior sample | 0.019 | |
4 | 4.1 External sample | 0.181 |
4.2 Intermediate sample | 0.095 | |
4.3 Interior sample | 0.079 | |
5 | 5.1 External sample | 0.058 |
5.2 Intermediate sample | 0.023 | |
5.3 Interior sample | 0.020 | |
6 | 6.1 External sample | 0.081 |
6.2 Intermediate sample | 0.095 | |
6.3 Interior sample | 0.043 |
Sample | Carbonation depth (mm) | Reinforcement coating (mm) |
1. | 20–25 | 40–50 |
2. | 10–15 | 15–20 |
3. | 30 | 30–40 |
4. | 10–15 | 15–20 |
5. | 15–20 | ≥50 |
6. | 20 | 15–20 |
Sample | Location | Average thickness (mm) |
1. | Inner courtyard façade (east) | ≥45 |
2. | Main façade (west) | 15–20 |
3. | Main façade (south) | 30–40 |
4. | Exterior ramp façade (west) | 15–20 |
5. | Roof façade (east) | ≥50 |
6. | Roof façade (west) | 15–20 |
Sample | Real density (g/cm3) | Apparent density (g/cm3) | Open porosity (%) |
1. | 2.65 | 2.15 | 18.8 |
2. | 2.65 | 2.15 | 18.9 |
3. | 2.66 | 2.16 | 19.0 |
4. | 2.77 | 2.22 | 19.7 |
5. | 2.64 | 2.20 | 16.5 |
6. | 2.63 | 2.12 | 19.3 |
Cores | Sample location | Chloride concentration (%) |
1 | 1.1 External sample | 0.039 |
1.2 Intermediate sample | 0.023 | |
1.3 Interior sample | 0.023 | |
2 | 2.1 External sample | 0.144 |
2.2 Intermediate sample | 0.061 | |
2.3 Interior sample | 0.019 | |
3 | 3.1 External sample | 0.250 |
3.2 Intermediate sample | 0.032 | |
3.3 Interior sample | 0.019 | |
4 | 4.1 External sample | 0.181 |
4.2 Intermediate sample | 0.095 | |
4.3 Interior sample | 0.079 | |
5 | 5.1 External sample | 0.058 |
5.2 Intermediate sample | 0.023 | |
5.3 Interior sample | 0.020 | |
6 | 6.1 External sample | 0.081 |
6.2 Intermediate sample | 0.095 | |
6.3 Interior sample | 0.043 |
Sample | Carbonation depth (mm) | Reinforcement coating (mm) |
1. | 20–25 | 40–50 |
2. | 10–15 | 15–20 |
3. | 30 | 30–40 |
4. | 10–15 | 15–20 |
5. | 15–20 | ≥50 |
6. | 20 | 15–20 |