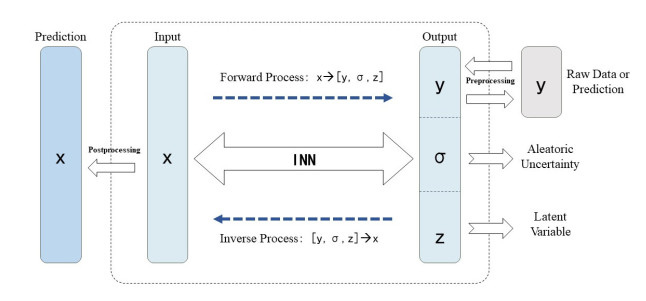
Invertible neural network (INN) is a promising tool for inverse design optimization. While generating forward predictions from given inputs to the system response, INN enables the inverse process without much extra cost. The inverse process of INN predicts the possible input parameters for the specified system response qualitatively. For the purpose of design space exploration and reasoning for critical engineering systems, accurate predictions from the inverse process are required. Moreover, INN predictions lack effective uncertainty quantification for regression tasks, which increases the challenges of decision making. This paper proposes the probabilistic invertible neural network (P-INN): the epistemic uncertainty and aleatoric uncertainty are integrated with INN. A new loss function is formulated to guide the training process with enhancement in the inverse process accuracy. Numerical evaluations have shown that the proposed P-INN has noticeable improvement on the inverse process accuracy and the prediction uncertainty is reliable.
Citation: Yiming Zhang, Zhiwei Pan, Shuyou Zhang, Na Qiu. Probabilistic invertible neural network for inverse design space exploration and reasoning[J]. Electronic Research Archive, 2023, 31(2): 860-881. doi: 10.3934/era.2023043
[1] | Zhiyuan Feng, Kai Qi, Bin Shi, Hao Mei, Qinghua Zheng, Hua Wei . Deep evidential learning in diffusion convolutional recurrent neural network. Electronic Research Archive, 2023, 31(4): 2252-2264. doi: 10.3934/era.2023115 |
[2] | Yao Sun, Lijuan He, Bo Chen . Application of neural networks to inverse elastic scattering problems with near-field measurements. Electronic Research Archive, 2023, 31(11): 7000-7020. doi: 10.3934/era.2023355 |
[3] | Xiangwen Yin . A review of dynamics analysis of neural networks and applications in creation psychology. Electronic Research Archive, 2023, 31(5): 2595-2625. doi: 10.3934/era.2023132 |
[4] | Pinchao Meng, Xinyu Wang, Weishi Yin . ODE-RU: a dynamical system view on recurrent neural networks. Electronic Research Archive, 2022, 30(1): 257-271. doi: 10.3934/era.2022014 |
[5] | Xiang Xu, Chuanqiang Huang, Chongchong Li, Gang Zhao, Xiaojie Li, Chao Ma . Uncertain design optimization of automobile structures: A survey. Electronic Research Archive, 2023, 31(3): 1212-1239. doi: 10.3934/era.2023062 |
[6] | Won-Ki Seo . Fredholm inversion around a singularity: Application to autoregressive time series in Banach space. Electronic Research Archive, 2023, 31(8): 4925-4950. doi: 10.3934/era.2023252 |
[7] | Xiaoping Zhao, Liwen Jiang, Adam Slowik, Zhenman Zhang, Yu Xue . Evolving blocks by segmentation for neural architecture search. Electronic Research Archive, 2024, 32(3): 2016-2032. doi: 10.3934/era.2024092 |
[8] | Ruyang Yin, Jiping Xing, Pengli Mo, Nan Zheng, Zhiyuan Liu . BO-B&B: A hybrid algorithm based on Bayesian optimization and branch-and-bound for discrete network design problems. Electronic Research Archive, 2022, 30(11): 3993-4014. doi: 10.3934/era.2022203 |
[9] | Yiyuan Qian, Kai Zhang, Jingzhi Li, Xiaoshen Wang . Adaptive neural network surrogate model for solving the implied volatility of time-dependent American option via Bayesian inference. Electronic Research Archive, 2022, 30(6): 2335-2355. doi: 10.3934/era.2022119 |
[10] | Weichi Liu, Gaifang Dong, Mingxin Zou . Satellite road extraction method based on RFDNet neural network. Electronic Research Archive, 2023, 31(8): 4362-4377. doi: 10.3934/era.2023223 |
Invertible neural network (INN) is a promising tool for inverse design optimization. While generating forward predictions from given inputs to the system response, INN enables the inverse process without much extra cost. The inverse process of INN predicts the possible input parameters for the specified system response qualitatively. For the purpose of design space exploration and reasoning for critical engineering systems, accurate predictions from the inverse process are required. Moreover, INN predictions lack effective uncertainty quantification for regression tasks, which increases the challenges of decision making. This paper proposes the probabilistic invertible neural network (P-INN): the epistemic uncertainty and aleatoric uncertainty are integrated with INN. A new loss function is formulated to guide the training process with enhancement in the inverse process accuracy. Numerical evaluations have shown that the proposed P-INN has noticeable improvement on the inverse process accuracy and the prediction uncertainty is reliable.
A variety of predictive algorithms have been developed to explain the forward mapping of input parameters x to measurable output parameters y. For some engineering applications, the inverse mapping from y to x is of paramount importance. Especially in the field of product design, inverse prediction based on specified output performance is required to obtain feasible design parameters. The design of industrial equipment represents a tricky and challenging task that encompasses multi-disciplinary knowledge. To optimize multiple performance objectives simultaneously, engineers need to perform hundreds of design iterations.
As a widely used power device in industry, the gas turbine incorporates principles from the disciplines of mechanics, materials, thermodynamics and aerodynamics which leads to a bulky and slow design process. This demands designers to comprehensively consider the performance of modules (e.g., combustors) as well as components (e.g., blades) to ensure overall stability and efficiency. Due to the fact that inverse design outputs the distribution of design parameters from the desired response, the interpretability and efficiency of the design space exploration could be improved comparing with that from only the forward design process. A range of inverse design methods have been developed which fall into two categories roughly: optimization-based methods and inverse process-based methods [1].
Optimization-based methods refer to the process searching for the solution that makes the target performance optimal in the design parameter space under the constraints. It is essentially a forward process, where the system response/performance obtained by simulations or experiments is used to adjust the design parameters reversely. Classical meta-heuristic algorithms including genetic algorithm and particle swarm algorithm are widely used in model update design of steam turbines and other equipment [2,3]. In special design cases, some deterministic algorithms [4,5] have been proven to achieve optimal solutions equivalent with heuristics in a much shorter time. These methods involve multiple iterations of the forward simulation process which might be computationally expensive. Although the simulation process can be replaced by a surrogate model to reduce computation time [6], these methods might fall into local optimum dilemmas readily. The most critical point is that there is a many-to-one mapping relationship between design parameters and performance parameters. Methods based on optimization cannot capture these possible design candidates, which is urgently required by engineers. Bayesian inference methods present the posterior distribution of parameters, thus achieving the goal of describing non-unique design parameters.
Bayesian inference methods mainly include the Markov chain Monte Carlo (McMC) sampling approach [7,8] and variational inference (VI) [9]. McMC obtains multiple sets of possible design parameters by sampling the posterior distribution function, while the mean and variance of these data are derived to describe the parameters' Probability Density Function (PDF). McMC is widely used [10,11,12] to approximate the empirical PDF for the parameters of interest. VI searches for simple distributions that approximate the target distribution (usually multi-modal) in a predefined family of distributions by optimizing the KL divergence. Attributed to the fact that VI is using optimization rather than random sampling, it improves the efficiency of solving the parameter distribution to some extent. In [13,14], VI significantly helps the design of circuits or communication systems. Still, their computational efficiency is not high enough.
Neural network-based methods facilitate real-time prediction of the inverse design. Neural networks (NNs) are flexible nonlinear mappings trained to efficiently predict outcomes regardless of the actual physical processes. Taking advantage of the rapidity of NNs, they are extensively used in inverse scattering problems [15]. The most typical ones are: [16] using fully connected neural networks (FCNN) to recover the shape parameters of unknown scatterers; [17] generating the obstacle appearances in reverse on the basis of two-layer long short-term memory (LSTM) neural networks; [18] proposing a parameter inversion model by a neural network (PIMNN), which captures the trajectory of moving point source to complete the subsequent predictions. Moreover, NNs also serve as surrogate models to achieve inverse design optimization [19,20,21]. However, these methods fail to provide an uncertain estimate, which means that NNs only seek one result for each input. In [22,23,24], multiple groups of NNs are trained simultaneously using Bayesian neural networks or dropout to estimate the uncertainty of input parameters, but they cannot acquire complex parametric distributions. Recently, various conditional generative models based on generative adversarial networks (GAN) have been widely used in inverse design [25,26,27,28]. These emerging models have achieved many promising effects, while the training process suffers from instable convergence.
To fully obtain the PDF of input parameters, the invertible neural network (INN) model is proposed [29]. INN is a bidirectional mapping network based on affine coupling blocks [30,31], considered as an excellent approach for solving inverse problems. The forward process of an INN predicts the output from the input while the inverse process derives the distribution of the input parameters. The key information of its inverse process is captured by the latent variables. INN shines not only in the field of computer vision [32,33], but also has distinctive achievements in the field of inverse design [1,34,35,36,37].
The forward process of the standard INN only gives the prediction result without presenting uncertainty. Moreover, its inverse process is usually acquired in a qualitative manner and not accurate enough for design purposes. To address this issue, we propose the P-INN with integrated epistemic uncertainty and aleatoric uncertainty. In this way, the outcome of the forward process carries uncertainty, while the posterior distribution of the inverse process incorporates epistemic uncertainty. We also design a bidirectional loss during training to improve the inverse prediction accuracy.
In the following sections, Section 2 describes the proposed method, including the architecture of P-INN and the realization of bidirectional prediction. Section 3 evaluates P-INN with two mathematical functions with varying dimensionality regarding prediction accuracy and reliability of prediction uncertainty. The case of inverse design of turbine blades in engineering is presented in Section 4. Section 5 concludes the work with major findings.
The proposed P-INN is shown in Figure 1. The main part of the framework is the INN, which consists of a series of affine coupling blocks. Model uncertainty includes epistemic uncertainty (EU) and aleatoric uncertainty (AU). Here, EU is obtained by the Monte Carlo dropout method [38] while AU is acquired by the additional noise channel, denoted as σ. In order to make the framework more general, it is necessary to add data preprocessing and postprocessing.
The INN architecture is shown in Figure 2, whose basic unit consists of an invertible block with two complementary affine coupling layers. In this work, we adopt a Real NVP architecture [31], which is superior for learning the distribution of data with stable convergence.
Denote the input vector of each invertible block as u, and divide u into two parts as u1 and u2. si and ti are trainable affine functions, where i∈{1,2}. The input vector group (u1, u2) is converted by them to obtain the corresponding output vector group (v1, v2). In this work, si and ti are assigned as fully connected neural networks with ReLU for the activation function, while these shallow neural networks will be considered as subnetworks of INN. v1 and v2 form the output vector v. The forward process of an invertible block is described as follows:
v1=u1☉exp(s1(u2))+t1(u2) |
v2=u2☉exp(s2(v1))+t2(v1) | (1) |
where ☉ represents the element product of the matrix. Likewise, the reverse process expressions are listed below:
u2=&(v2−t2(v1))☉exp(−s2(v1)) |
u1=&(v1−t1(u2))☉exp(−s1(u2)) | (2) |
It is worth noting that the "+" and "☉" operations in Eq (1) already provide the reversible effect of INN, thus the si and ti functions allow arbitrary mappings without requiring them to be invertible. An adequate volume of invertible blocks is connected in series to deepen the network; a certain level of zero vectors is replenished at the input and output to enhance the predictive capability of the network. Because each invertible block requires the same dimensionality of inputs and outputs, the input and output dimensions of INN also need to be consistent as shown in Figure 1 and Eq (3):
dim(inputs)=dim(x) |
dim(outputs)=dim(y)+dim(z)+dim(σ) | (3) |
dim(inputs)=dim(outputs) |
where x is the input parameter, y is the output parameter, σ denotes the AU corresponding to y, and z denotes the latent variable. To enhance the interaction between the dimensions of parameters, each invertible block is followed by a permutation block in this work, which is used to randomly shuffle the dimensions of the parameters.
The standard INN can generate an exhaustive posterior distribution of input parameters based on specific output parameters, which is extensively used for solving inverse problems [29]. However, INN has several problems: it may not converge for some regression tasks; results obtained by the forward process lack uncertainty; the distribution achieved by the inverse process is not accurate sufficiently. To address these issues, we propose the probabilistic invertible neural network (P-INN) framework shown in Figure 1, which provides uncertainty assessment while improving the bi-directional prediction accuracy.
We first review the knowledge about uncertainty [39]. Uncertainty is broadly divided into epistemic uncertainty (EU) and aleatoric uncertainty (AU) [40]. EU describes the uncertainty of model parameters, which is due to the fact that the prediction can be affected by factors such as poor training and insufficient training data. AU refers to the error caused by the intrinsic noise of data. [24] proposed that the AU may be predicted directly using the likelihood loss, which will be described in detail in Section 2.3. Common methods for EU acquisition include model fusion as well as Bayesian neural networks (BNN). The model fusion-based approach aims to train multiple network models on the same dataset; the predictions of these models are finally synthesized. The weight of each parameter in BNN is a distribution, so weights are sampled to get different results when predicting. The Monte Carlo dropout approach is a simple implementation, which we adopt in this work. The forward prediction distribution is drawn according to Eq (4):
q(y,z|x,D)=∫p(y,z|x,θ)q(θ,D)dθ | (4) |
where D is the training dataset, p(y,z|x,θ) is the likelihood function, and q(θ,D) is the posterior function of the weight parameters.
T dropout samples are produced to approximate the distribution of weights, and use Monte-Carlo to estimate the first moment of the prediction function:
Eq(y|x,D)(y)≈1T∑Tt=1ŷ(x,Wt1,…,WtL) | (5) |
where Wti represents the parameter matrix of the i-th layer, and it is assumed the INN has a total of L layers. The second moment is solved in a similar way. In practice, we only need to activate dropout in forward process. EU is represented the calculated variance of T predictions.
The inverse process takes response y, predefined σ, and the sampled z as input, and derives the x. Set f=g−1, then f−1(y,z,σc;θ) is denoted as g(y,z;θ). The latent variable z is set to be the standard normal distribution z p(z)=N(0,I). Depending on the specific y each set of z is sampled from the distribution p(z) in order to derive the corresponding x. A one-to-many mapping of the inverse process (a set of y generates multiple sets of x) is implemented by sampling z multiple times. Define the complete distribution of results for inverse process as shown in Eq (6):
q(x|y)=p(z )det(∂g(y,z;θ )∂[y,z] ) | (6) |
where the denominator part is the Jacobian determinant embodied by the inverse process. The dropout of the fully connected layer is activated where the generated x is combined with EU.
The loss function is formulated with 6 terms from 2 categories. The loss terms in the first category aim for improving the forward prediction accuracy, including the mean square error (MSE) of output responses, the likelihood of output responses and the distribution term of latent variables. The loss terms in the second category are beneficial to improve the inverse prediction accuracy, including the distribution term of input parameters, reconstruction term and bidirectional term. Each loss term has a specific contribution as detailed in the following paragraphs.
MSE term of output response. The purpose of this loss term is to make the predicted output response and true value as identical as possible, which is the main goal of forward process. The MSE loss term is defined in Eq (7):
LMSE(θ)=1N∑Ni=1(ŷ(i)−y∗(i))2 | (7) |
where ŷ(i) is the forward predicted output response value, y∗(i) is the ground-truth response value, θ refers to the weight parameters that INN needs to learn, and N denotes the number of training samples.
Likelihood term of output response. This loss term is proposed to obtain the AU of the response. AU measures the noise within the data, which we characterize directly using an additional σ channel. In general, the input dimension is much larger than the output dimension. INN requires that the input dimension be consistent with the output dimension, which will be supplemented with the zero vector. The σ channel replaces part of the zero vectors. Thus, the loss function of this term is defined as follows:
Llikelihood(θ)=1N∑Ni=1((ŷ(i)−y∗(i))22(σ(i))2+12log((σ(i))2)) | (8) |
In Eq (8), the dimension of σ(i) is aligned with the dimension of the output response.
Distribution term of latent variable. The latent variable z can be arbitrarily distributed, and we take z N(0,1) to simplify the calculation. In the inverse process, the probability distribution of the input parameters is acquired with sampled z. The distribution term aims for measuring the difference between the distribution of forward predictions and the distribution of the training samples. The loss function is defined as follows:
Ldistributionz(θ)=MMD(q(ŷ, z;θ),p(y∗)p(z∗)) | (9) |
where z and z∗, respectively, represent the predicted and genuine value of the latent variable; q(ŷ, z;θ) is the distribution for the result in forward process; p(y∗) is the prior knowledge of output response from training samples; p(z∗) is the standard normal distribution. MMD refers to Maximum Mean Discrepancy, which is an effective way to compare differences between distributions.
Distribution term of input parameters. This term aims to narrow the difference between the inverse prediction distribution and the prior knowledge of input parameters from training samples, which can improve the accuracy of inverse prediction to a certain extent. The loss term is defined in Eq (10):
Ldistributionx(θ)=MMD(q( x;θ),p(x∗)) | (10) |
where x denotes the prediction result in inverse process; x∗ refers to the authentic input data; q( x;θ) is the distribution of the prediction result; and p(x∗) is the prior knowledge of inputs from training samples.
Reconstruction term. In the inverse process, a set of input parameters is generated for each set of z samples. Since the value of z is infinite, the result of INN backward prediction has a high degree of freedom for a given set of the output response. Design and reasoning of critical engineering systems require accurate posterior distribution of input parameters from a given system response. This loss term ensures that the same input parameters are predicted as identical as possible when sampling similar z. The loss term is defined in Eq (11):
Lreconstruction(θ)=1N∑Ni=1(f−1(y∗(i), z(i),σc;θ)−x∗(i))2 | (11) |
where f−1(y,z,σc;θ) refers to the inverse process, and σc takes a small constant because the given y is a ground truth value. Noise term is added to the predicted latent variable form increased robustness.
Bidirectional term. The bidirectional loss is proposed for further improvement of the inverse prediction accuracy. The distribution of input parameters is captured by the sampled latent variable z, but there is no guarantee that the distribution can precisely reflect the true input parameters. It is difficult to directly measure the gap between predicted values and the ground-truth values. We propose an indirect measurement for the distribution of inverse prediction. The predicted input parameters are first converted using the trained forward process h(x) where we then calculate the MSE between the transformed and true values. Here h(x) is set to be f(x;θ) and the loss term is defined as in Eq (12):
Lbidirectional(θ)=1N∑Ni=1(f(f−1(y∗(i),z(i),σc;θ)+noise;θ)−y∗(i))2 | (12) |
where f(x;θ) represents the forward process. Different from reconstruction loss term, z(i) is sampled from the standard normal distribution. The bidirectional loss forces all potential predicted input parameters to be reducible to the specified value.
Total loss. The total loss is the sum of all the above losses, as in Eq (13):
Ltotal=∑6i=1λiLi | (13) |
where λi represents the weight of each loss. After data preprocessing, y is normalized to be between 0 and 1. It is obvious that the MSE term, Likelihood term, and Bidirectional term all correlate directly or indirectly with y. Consequently, the order of magnitudes for these terms is the same. Meanwhile, the experiments indicate that the results of the two distribution terms are also appropriate. We consider that these five terms are balanced for model training, taking their weights as the one here. To adjust the Reconstruction term, its coefficient is the reciprocal of the square for the value range taken by the input data, which has a normalization-like effect.
In this section, two mathematical problems are adopted to evaluate the proposed method. Experiments show that the method has better results in multi-objective predictions and high-dimensional input situations.
Mathematical settings. To test the performance of P-INN on the inverse problem, we design a multi-objective regression task. Consider an example of two-dimensional input and two-dimensional output. The input x is written as [x1, x2], and the output y is written as [y1, y2]. The input domains are Ωx1ϵ[−5,10], Ωx2ϵ[0,15] which equipped with a uniform probability density p(xi). The two-objective functions y1 and y2 are modified from the branin and hyperbolic paraboloid function. Moreover, the curved surfaces are shown in Figure 3a, b respectively, where the outputs have been normalized. The function expressions are shown in Eq (14):
y1=a(x2−bx21+cx1−r)2+s(1−t)cos(x1)+s |
y2=(x1−u)2m−(x2−v)2n | (14) |
where a=1, b=5.14π2, c=5π, r=6, s=10, t=18π; u=2, v=7, m=2.25, n=400. Branin function is a commonly used complex surface for optimization problems, while hyperbolic paraboloid function is a universal surface in structural design, so we select them as the test functions. We train the P-INN with 6 invertble blocks and the si and ti functions of the invertble block both use a 5-layer NN. All hidden layers are followed by a dropout layer with a dropout coefficient of 0.1. Train on datasets of size 50,100 and 150 while optimizing for 3000 iterations using the Adam optimizer.
Benchmarks. To evaluate the superiority of the proposed method, we compare it with the standard INN. In fairness, the standard INN has the same number of reversible blocks, and the si and ti of reversible blocks are also consistent with P-INN. We also train a forward 5-layer DNN to compare the prediction accuracy of the proposed method.
Evaluation metrics. To intuitively evaluate the performance of P-INN, we use two types of metrics to measure the quality. The first type measures the prediction accuracy, including the root mean square error (RMSE). However, since the reverse prediction has multiple unknown truth values, the inverse RMSE cannot be calculated. We use an indirect approach: convert the obtained input parameters through the ground-truth functions; then compute the RMSE between transformed values and true output parameters. The second type measures uncertainty interval includes Prediction Interval Coverage Probability (PICP) and PI Normalized Averaged Width (PINAW). PICP evaluates the ability of the uncertainty interval for capturing the true value, as follows:
PICP=1N∑Nj=1Cj | (15) |
where Cj=1 if the true value is in the uncertainty interval, otherwise Cj=0. PICP ranges from 0% to 100%. For a specified probability of 100(1−α)%, the uncertainty can be considered reliable if PICP≈100(1−α)%. PINAW evaluates the width of the uncertainty, as a percentage of the target range. The following definitions are given:
PINAW=1RN∑Nj=1Lj | (16) |
where R is the range of the target variable and Lj is the range of the uncertainty interval. The predicted uncertainty behaves better with a lower PINAW. Similarly, since the true value of the inverse is difficult to determine, we transform the inverse predicted value into the output space through the ground-truth functions, and then perform uncertainty evaluation.
Forward discussion. The prediction accuracy and uncertainty estimates of the forward process are analyzed from Tables 1 and 2, where the data that perform outstanding are bolded. When the training dataset size is small (such as size 50), the prediction accuracy of P-INN is lower, but still more accurate than INN. If trained on a sufficient dataset, the proposed method will even outperform DNNs. It is intuitive that the forward RMSE of P-INN is only 0.0199 and 0.0338 with 150 sets of training data while the forward RMSE of INN is 0.2021 and 0.2861, which is even considered as a fitting failure. The surfaces acquired by the proposed method are shown in Figure 3c, d while the surfaces derived by DNN are shown in Figure 3e, f. The surfaces fitted by INN are poor so we don't show them. Obviously, although the surfaces obtained by the proposed method are not smooth, they fit the real surface better than DNN. We find that in the case of few data, the PICP of the forward process can still be close to 1 (assuming a reliability of 0.95), but the PINAW is a bit large. This means that the uncertainty interval covers almost all true values, but it is wide so the uncertainty is not entirely convincing. With an appropriate increase in training data (such as a size of 100), PINAW drops below 0.5.
Data size | Objective 1 | Objective 2 | ||||
50 | 100 | 150 | 50 | 100 | 150 | |
P-INN | 0.1235 | 0.0325 | 0.0199 | 0.1855 | 0.0251 | 0.0338 |
INN | 0.2281 | 0.4173 | 0.2021 | 0.9457 | 0.1896 | 0.2861 |
DNN | 0.0648 | 0.0629 | 0.0572 | 0.1073 | 0.0700 | 0.0588 |
Data size | Objective 1 | Objective 2 | ||||
50 | 100 | 150 | 50 | 100 | 150 | |
PICP | 1 | 1 | 1 | 0.9833 | 1 | 1 |
PINAW | 1.0489 | 0.4032 | 0.2855 | 0.8948 | 0.3304 | 0.7166 |
Inverse discussion. Tables 3 and 4 demonstrate the superiority of P-INN in reverse process. Because DNN does not have the capability of inverse prediction, only the proposed method is compared with INN. Experiments show that the inverse prediction accuracy of P-INN is often much higher than that of INN. PICP remains above 0.95 when the training dataset size is greater than 50. Therefore, the obtained uncertainty is considered to be reliable. Meanwhile, PINAW stays under 0.5, which means that the uncertainty interval is narrow enough.
Data size | Objective 1 | Objective 2 | ||||
50 | 100 | 150 | 50 | 100 | 150 | |
P-INN | 0.1749 | 0.1392 | 0.1200 | 0.2353 | 0.1579 | 0.1832 |
INN | 0.1595 | 0.2412 | 0.2330 | 0.2982 | 0.2551 | 0.2753 |
DNN | × | × | × | × | × | × |
Data size | Objective 1 | Objective 2 | ||||
50 | 100 | 150 | 50 | 100 | 150 | |
PICP | 0.8417 | 0.9667 | 0.9750 | 0.6750 | 1 | 0.9917 |
PINAW | 0.2971 | 0.3557 | 0.3248 | 0.3287 | 0.4456 | 0.4481 |
Mathematical settings. We design a high-dimensional input example to show that P-INN is effective in general applications. The weight of an airplane's wing is related to a number of factors that designers must take into account when designing a wing. Therefore, we take the classical wing weight function, which has 10-dimensional inputs, as follows:
y=0.036S0.758wW0.0035fw(Acos2(Λ))0.6q0.006λ0.04(100tccos(Λ))−0.3(NzWdg)0.49+SwWp | (17) |
Where Sw refers to the wing area, Swϵ[150,200]; Wfw represents the fuel weight of the aircraft, Wfwϵ[220,300]; A denotes the aspect ratio of the wing, Aϵ[6,10]; Λ denotes quarter-chord sweep, Λϵ[6,10]; q denotes dynamic pressure at cruise, qϵ[16,45]; λ denotes taper ratio, λϵ[0.5,1]; tc denotes aerofoil thickness to chord ratio, tcϵ[0.08,0.18]; Nz denotes ultimate load factor, Nzϵ[2.5,6]; Wdg refers to flight design gross weight, Wdgϵ[1700,2500]; Wp denotes paint weight, Wpϵ[0.025,0.08]. Each input variable is uniformly sampled in its domain. The training parameters are similar to Section 3.1. It should be noted that the order of magnitude difference between the input variables is significant, thus the reconstruction loss must be assigned weights related to the range of input variables. The reverse process is followed by data post-processing, which is implemented by limiting the predicted value within the corresponding domain. Figure 4 presents the loss curves for a given training data set of 100. As this is a high-dimension input problem, the output requires complementary zero vectors to guarantee dimensional alignment, which will contribute to greater values of MSE term and likelihood term at the early stage of training. In the meantime, it can be seen from Eq (8) that the value of likelihood term may be less than 0 when the σ(i) is small. Due to the above reasons, we add a constant of 10 to the loss results and then logarithmically process them to obtain better visualization. As shown in Eq (12), the bidirectional term relies on the exact forward process, hence we incorporate this loss after the 400th epoch. It is noticed that all six losses converge and tend to stabilize after the 450th epoch.
Benchmarks and metrics. INN and DNN are provided to compare the prediction results in bidirectional, where their network parameters are analogous to those in the multi-objective problem. The evaluation metric of prediction accuracy is RMSE, while the evaluation metric of uncertainty interval is PICP and PINAW.
Discussion. The comparison of prediction accuracy of different methods is shown in Table 5. The proposed method excels on high-dimensional input examples: the forward RMSE is already the lowest with a dataset size of 50, while the forward process of INN behaves terribly. The forward RMSE and reverse RMSE of the proposed method are 0.0839 and 0.1309 with an increase in training set size to 150. The inverse uncertainty of P-INN is calculated, as shown in Table 6. The final PICP is 0.95 and the PINAW is 0.357, both indicating that the uncertainty is certainly convincing.
Forward RMSE | Inverse RMSE | |||||
Data size | 50 | 100 | 150 | 50 | 100 | 150 |
P-INN | 0.1521 | 0.1115 | 0.0839 | 0.3574 | 0.1286 | 0.1309 |
INN | 3.6367 | 3.9584 | 3.0569 | 0.5253 | 0.4706 | 0.2788 |
DNN | 0.1715 | 0.1662 | 0.1609 | × | × | × |
Data size | PICP | PINAW |
50 | 0.5250 | 0.4321 |
100 | 1 | 0.4803 |
150 | 0.9500 | 0.3570 |
Visualization. The input dimension in this example is 10, which means that the performance of the model is unable to be visualized. We use principal component analysis (PCA) to reduce dimensionality of the input. The main idea of PCA is to map n-dimensional features to k-dimensions, which are completely new orthogonal features. The new features are reconstructed on the basis of the original n-dimensional features. The calculation shows that the cumulative contribution rate of the first two eigenvalues is 0.9943, so the original input features can be replaced with new two-dimensional features. The eigenvectors corresponding to the eigenvalues indicate the contribution of the original features to the new features, and the results show that Wdg plays an important role. Figure 5 shows the mapping relationship between Wdg and output (all other input parameters are fixed; here the average of each parameter is taken). The generated training data is added with noise that satisfies the standard normal distribution to simulate the real situation, and some training points are shown in Figure 5a. The curve derived by P-INN almost coincides with the real curve, while the curve obtained by INN is unacceptable. The curve fitted by DNN is also not quite satisfactory.
Jet engine produces thrust by jetting backwards to achieve forward movement. Gas turbine is a vital component of jet engine because of its light weight, compact structure, and high combustion efficiency [41]. Gas turbine engines are divided into four categories: turbojet, turbofan, turboprop, and turboshaft. When a jet engine works, the gas drawn in is compressed by a compressor to the smallest possible optimal volume. The compressed gas pressure is almost 30 times the original, while the temperature rises to 1000℃. It is then sent to the combustion chamber for combustion. The turbine is responsible for extracting energy from the high temperature and pressure gases produced by the combustion chamber. Due to the long-term extreme working environment, turbine blade materials are usually chosen from high-strength, high-melting nickel alloys. Turbine blades expand when heated, creating significant stress at their joints resulting in strains of several millimeters, which can cause friction and even damage between the blade tip and the casing. Therefore, designers must consider the stress and strain of the blade in the normal working state when designing the turbine. It is natural for us to apply P-INN to the inverse design of the blade.
The simulated 3D structure of the turbine blade is shown in Figure 6. In reality, the stresses and strains of the blade are affected by a combination of pressure and thermal effects. We first calculate the stress and strain that are only affected by the pressure while ignoring the thermal effect; then analyze the stress and strain that only has the thermal effect while ignoring the pressure; finally, the simulation model with the combined effect of the two factors is obtained. The stress or temperature heat maps of the three processes are shown in Figure 7. The pressure model parameters include Young's modulus E, Poisson's ratio nu, the thermal expansion coefficient CTE of the nickel-chromium alloy, and the pressures p1 and p2 on both sides of the blade; the thermal effect model parameters include the thermal conductivity kapp of the nickel-chromium alloy.
Here, kapp is fixed and the remaining 5 variables are taken as input. Each variable ranges from 80% to 120% of the recommended value, sampling 100 sets of input data evenly. The simulated stress is used as output. 20 sets of data were randomly selected as the test set. The prediction accuracy of P-INN is compared with INN and DNN.
The prediction accuracy of different methods is shown in Table 7. It can be found that even if the training set is small, the forward accuracy of the proposed method is already ahead of other algorithms; when given more data to train, the forward accuracy and inverse accuracy of P-INN have reached a satisfactory level (respectively 0.0634 and 0.1052). After sufficient training, INN's forward accuracy is poor but inverse accuracy is acceptable. DNN does not perform well in this case.
Data size | Forward RMSE | Inverse RMSE | ||||
20 | 40 | 80 | 20 | 40 | 80 | |
P-INN | 0.2017 | 0.1327 | 0.0634 | 0.2894 | 0.2057 | 0.1052 |
INN | 5.0359 | 0.7367 | 0.2194 | 0.4048 | 0.2652 | 0.1564 |
DNN | 0.2110 | 0.2014 | 0.2002 | × | × | × |
The inverse uncertainty predicted by the proposed method is shown in Table 8. The PICP has reached 1 with a training set size of 40, which indicates that the uncertainty interval fully covers the true value. PINAW remains around 0.5 with adequate training datasets. Figure 8 visualizes the uncertainty intervals of the inverse forecast, where the results for P-INN and INN are represented by green histograms and orange histograms respectively. Three stress values were randomly selected from the test set (we take y = 942.46,720.85, 1000.37). After that 100 sets of input parameters were generated by P-INN and INN. Since the real input parameters are not available to obtain, we use simulation to convert the reverse predicted values. In this way, the input parameters distribution is referred to by the output parameters distribution. The true stress value is marked with a red dashed line. It can be found that both P-INN and INN cover the ground truth, but the distribution of our proposed method is more concentrated, which means that our inverse process is more reliable and convincing. For a given specific stress, the solution generated by P-INN has a higher probability of manifesting the desired performance compared to the solution generated by INN. In Figure 8c and Figure 8e, although the probability of the interval in which the true value falls is not the maximum, the interval adjoins the maximum probability interval. Moreover, the truth interval probability of P-INN is larger than that of INN.
Data size | PICP | PINAW |
20 | 0.5000 | 0.4711 |
40 | 1 | 0.6461 |
80 | 1 | 0.4620 |
Based on the above analysis, we use P-INN to generate thorough and reliable turbine blade design solutions, given the stress conditions. Specifically, the distance between the blade and the case is specified in advance, while the stress and strain during operation are known; possible design solutions (including design parameters such as blade material) are available through P-INN as a way to avoid collisions between the blade and the chassis. Our proposed method indeed contributes to the inverse design of turbine blades.
This work proposes the P-INN framework incorporating both epistemic and aleatory uncertainty for better inverse design and reasoning. The bi-directional loss is developed to enhance the inverse prediction accuracy. We applied the method to two mathematical problems and turbine blade design, as demonstrated by comparing the results with standard INN and DNN. Experiments show that P-INN provides not only more accurate forward predictions, but also generates more concentrated and reliable inverse posterior distributions. After training, the P-INN can generate possible inverse solutions corresponding to the target system response within a second, which is beneficial for the fast design space exploration and reasoning of critical engineering systems. Although promising results have been observed with P-INN, further study is needed regarding the down-sampling of design candidates and the screening of optimal solutions.
This work has been supported by the National Key R & D Program of China (2018YFB1700700), the National Natural Science Foundation of China (52165029), Hainan Key Research and Development program (ZDYF2021GXJS022).
The authors declare there is no conflict of interest.
[1] |
S. Ghosh, G. A. Padmanabha, C. Peng, V. Andreoli, S. Atkinson, P. Pandita, et al., Inverse aerodynamic design of Gas turbine blades using probabilistic machine learning, J. Mech. Des., 144 (2022), 021706. https://doi.org/10.1115/1.4052301 doi: 10.1115/1.4052301
![]() |
[2] |
S. Obayashi, S Takanashi, Genetic optimization of target pressure distributions for inverse design methods, AIAA J., 34 (1996), 881–886. https://doi.org/10.2514/3.13163 doi: 10.2514/3.13163
![]() |
[3] |
P. Boselli, M. Zangeneh, An inverse design based methodology for rapid 3D multi-objective/multidisciplinary optimization of axial turbines, ASME J. Turbomach., 7 (2011), 1459–1468. https://doi.org/10.1115/GT2011-46729 doi: 10.1115/GT2011-46729
![]() |
[4] |
A. Nickless, P. J. Rayner, B. Erni, R. J. Scholes, Comparison of the genetic algorithm and incremental optimisation routines for a Bayesian inverse modelling based network design, Inverse Probl., 34 (2018), 055006. https://doi.org/10.1088/1361-6420/aab46c doi: 10.1088/1361-6420/aab46c
![]() |
[5] |
B. Hofmeister, M. Bruns, R. Rolfes, Finite element model updating using deterministic optimisation: a global pattern search approach, Eng. Struct., 195 (2019), 373–381. https://doi.org/10.1016/j.engstruct.2019.05.047 doi: 10.1016/j.engstruct.2019.05.047
![]() |
[6] | S. S. Kadre, V. K. Tripathi, Advanced surrogate models for design optimization, Int. J. Eng. Sci., 9 (2016), 66–73. |
[7] | C. P. Robert, G. Casella, Monte Carlo Statistical Methods, Springer New York, 2004. https://doi.org/10.1007/978-1-4757-4145-2 |
[8] | J. Jiang, Large Sample Techniques for Statistics, Cham, Springer International Publishing, 2022. https://doi.org/10.1007/978-3-030-91695-4 |
[9] |
D. M. Blei, A. Kucukelbir, J. D. McAuliffe, Variational inference: a review for statisticians, J. Am. Stat. Assoc., 112 (2017), 859–877. https://doi.org/10.1080/01621459.2017.1285773 doi: 10.1080/01621459.2017.1285773
![]() |
[10] |
L. Wu, W. Ji, S. M. AbouRizk, Bayesian inference with markov chain monte carlo–based numerical approach for input model updating, J. Comput. Civ. Eng., 34 (2020), 04019043. https://doi.org/10.1061/(ASCE)CP.1943-5487.0000862 doi: 10.1061/(ASCE)CP.1943-5487.0000862
![]() |
[11] |
J. J. Xu, W. G. Chen, C. Demartino, T. Y. Xie, Y. Yu, C. F. Fang, et al., A Bayesian model updating approach applied to mechanical properties of recycled aggregate concrete under uniaxial or triaxial compression, Constr. Build. Mater., 301 (2021), 124274. https://doi.org/10.1016/j.conbuildmat.2021.124274 doi: 10.1016/j.conbuildmat.2021.124274
![]() |
[12] |
Y. Yin, W. Yin, P. Meng, H. Liu, On a hybrid approach for recovering multiple obstacles, Commun. Comput. Phys., 31 (2022), 869–892. https://doi.org/10.4208/cicp.OA-2021-0124 doi: 10.4208/cicp.OA-2021-0124
![]() |
[13] | N. C. Laurenciu, S. D. Cotofana, Probability density function based reliability evaluation of large-scale ICs, in Proceedings of the 2014 IEEE/ACM International Symposium on Nanoscale Architectures, (2014), 157–162. https://doi.org/10.1145/2770287.2770326 |
[14] |
V. Raj, S. Kalyani, Design of communication systems using deep learning: a variational inference perspective, IEEE Trans. Cognit. Commun. Networking, 6 (2020), 1320–1334. https://doi.org/10.1109/TCCN.2020.2985371 doi: 10.1109/TCCN.2020.2985371
![]() |
[15] |
H. Liu, On local and global structures of transmission eigenfunctions and beyond, J. Inverse Ill-Posed Probl., 30 (2020), 287–305. https://doi.org/10.1515/jiip-2020-0099 doi: 10.1515/jiip-2020-0099
![]() |
[16] |
Y. Gao, H. Liu, X. Wang, K. Zhang, On an artificial neural network for inverse scattering problems, J. Comput. Phys., 448 (2022), 110771. https://doi.org/10.1016/j.jcp.2021.110771 doi: 10.1016/j.jcp.2021.110771
![]() |
[17] |
W. Yin, W. Yang, H. Liu, A neural network scheme for recovering scattering obstacles with limited phaseless far-field data, J. Comput. Phys., 417 (2020), 109594. https://doi.org/10.1016/j.jcp.2020.109594 doi: 10.1016/j.jcp.2020.109594
![]() |
[18] |
P. Zhang, P. Meng, W. Yin, H. Liu, A neural network method for time-dependent inverse source problem with limited-aperture data, J. Comput. Appl. Math., 421 (2023), 114842. https://doi.org/10.1016/j.cam.2022.114842 doi: 10.1016/j.cam.2022.114842
![]() |
[19] |
Y. Lu, Z. Tu, A two-level neural network approach for dynamic FE model updating including damping, J. Sound Vib., 275 (2004), 931–952. https://doi.org/10.1016/S0022-460X(03)00796-X doi: 10.1016/S0022-460X(03)00796-X
![]() |
[20] | H. Sung, S. Chang, M. Cho, Reduction method based structural model updating method via neural networks, 2020. https://doi.org/10.2514/6.2020-1445 |
[21] |
H. Sung, S. Chang, M. Cho, Efficient model updating method for system identification using a convolutional neural network, AIAAJ, 59 (2021), 3480–3489. https://doi.org/10.2514/1.J059964 doi: 10.2514/1.J059964
![]() |
[22] |
T. Yin, H. Zhu, An efficient algorithm for architecture design of Bayesian neural network in structural model updating, Comput.-Aided Civ. Infrastruct. Eng., 35 (2020), 354–372. https://doi.org/10.1111/mice.12492 doi: 10.1111/mice.12492
![]() |
[23] | D. P. Kingma, T. Salimans, M. Welling, Variational dropout and the local reparameterization trick, in Advances in Neural Information Processing Systems, 28 (2015). Available from: https://proceedings.neurips.cc/paper/2015/file/bc7316929fe1545bf0b98d114ee3ecb8-Paper.pdf. |
[24] | A. Kendall, Y. Gal, What uncertainties do we need in bayesian deep learning for computer vision? in Advances in Neural Information Processing Systems, 30 (2017). Available from: https://proceedings.neurips.cc/paper/2017/file/2650d6089a6d640c5e85b2b88265dc2b-Paper.pdf. |
[25] |
E. Yilmaz, B. German, Conditional generative adversarial network framework for airfoil inverse design, AIAA, 2020 (2020). https://doi.org/10.2514/6.2020-3185 doi: 10.2514/6.2020-3185
![]() |
[26] | J. A. Hodge, K. V. Mishra, A. I. Zaghloul, Joint multi-layer GAN-based design of tensorial RF metasurfaces, in 2019 IEEE 29th International Workshop on Machine Learning for Signal Processing (MLSP), (2019), 1–6. https://doi.org/10.1109/MLSP.2019.8918860 |
[27] | A. H. Nobari, W. Chen, F. Ahmed, PcDGAN: A continuous conditional diverse generative adversarial network for inverse design, preprint, arXiv: 2106.03620. |
[28] | A. H. Nobari, W. Chen, F. Ahmed, Range-GAN: Range-constrained generative adversarial network for conditioned design synthesis, in Proceedings of the ASME 2021 International Design Engineering Technical Conferences and Computers and Information in Engineering Conference, 3B (2021), V03BT03A039. https://doi.org/10.1115/DETC2021-69963 |
[29] | L. Ardizzone, J. Kruse, S. Wirkert, D. Rahner, E. W. Pellegrini, R. S. Klessen, et al., Analyzing inverse problems with invertible neural networks, preprint, arXiv: 1808.04730. |
[30] | L. Dinh, D. Krueger, Y. Bengio, NICE: Non-linear independent components estimation, preprint, arXiv: 1410.8516. |
[31] | L. Dinh, J. Sohl-Dickstein, S. Bengio, Density estimation using real NVP, preprint, arXiv: 1605.08803. |
[32] | Z. Guan, J. Jing, X. Deng, M. Xu, L. Jiang, Z. Zhang, et al., DeepMIH: Deep invertible network for multiple image hiding, IEEE Trans. Pattern Anal. Mach. Intell., 2022 (2022). https://doi.org/10.1109/TPAMI.2022.3141725 |
[33] | Y. Liu, Z. Qin, S. Anwar, P. Ji, D. Kim, S. Caldwell, et al., Invertible denoising network: a light solution for real noise removal, in 2021 IEEE/CVF Conference on Computer Vision and Pattern Recognition (CVPR), (2021), 13360–13369. https://doi.org/10.1109/CVPR46437.2021.01316 |
[34] |
M. Oddiraju, A. Behjat, M. Nouh, S. Chowdhury, Inverse design framework with invertible neural networks for passive vibration suppression in phononic structures, J. Mech. Des., 144 (2022), 021707. https://doi.org/10.1115/1.4052300 doi: 10.1115/1.4052300
![]() |
[35] |
V. Fung, J. Zhang, G. Hu, P. Ganesh, B. G. Sumpter, Inverse design of two-dimensional materials with invertible neural networks, npj Comput. Mater., 7 (2021), 200. https://doi.org/10.1038/s41524-021-00670-x doi: 10.1038/s41524-021-00670-x
![]() |
[36] |
P. Noever-Castelos, L. Ardizzone, C. Balzani, Model updating of wind turbine blade cross sections with invertible neural networks, Wind Energy, 25 (2022), 573–599. https://doi.org/10.1002/we.2687 doi: 10.1002/we.2687
![]() |
[37] | S. Ghosh, G. A. Padmanabha, C. Peng, S. Atkinson, V. Andreoli, P. Pandita, et al., Pro-ML IDeAS: A probabilistic framework for explicit inverse design using invertible neural network, AIAA, 2021 (2021). https://doi.org/10.2514/6.2021-0465 |
[38] | Y. Gal, Z. Ghahramani, Dropout as a bayesian approximation: representing model uncertainty in deep learning, in Proceedings of 33rd International Conference on Machine Learning, 48 (2016), 1050–1059. Available from: http://proceedings.mlr.press/v48/gal16.html?ref=https://githubhelp.com. |
[39] |
M. Abdar, F. Pourpanah, S. Hussain, D. Rezazadegan, L. Liu, M. Ghavamzadeh, et al., A review of uncertainty quantification in deep learning: techniques, applications and challenges, Inf. Fusion, 76 (2021), 243–297. https://doi.org/10.1016/j.inffus.2021.05.008 doi: 10.1016/j.inffus.2021.05.008
![]() |
[40] |
E. Hüllermeier, W. Waegeman, Aleatoric and epistemic uncertainty in machine learning: an introduction to concepts and methods, Mach. Learn., 110 (2021), 457–506. https://doi.org/10.1007/s10994-021-05946-3 doi: 10.1007/s10994-021-05946-3
![]() |
[41] |
M. Yadav, A. Misra, A. Malhotra, N. Kumar, Design and analysis of a high-pressure turbine blade in a jet engine using advanced materials, Mater. Today:. Proc., 25 (2020), 639–645. https://doi.org/10.1016/j.matpr.2019.07.530 doi: 10.1016/j.matpr.2019.07.530
![]() |
1. | Chao Ma, Hong Fu, Pengcheng Lu, Hongpeng Lu, Multi-objective crashworthiness design optimization of a rollover protective structure by an improved constraint-handling technique, 2023, 31, 2688-1594, 4278, 10.3934/era.2023218 | |
2. | Zhiwei Pan, Yiming Zhang, Xiaojian Liu, Yang Wang, Shuyou Zhang, 2024, Chapter 87, 978-981-97-0921-2, 1369, 10.1007/978-981-97-0922-9_87 | |
3. | S. V. Bezzateev, G. A. Zhemelev, S. G. Fomicheva, Researching the Performance of AutoML Platforms in Confidential Computing, 2024, 58, 0146-4116, 1373, 10.3103/S0146411624701049 |
Data size | Objective 1 | Objective 2 | ||||
50 | 100 | 150 | 50 | 100 | 150 | |
P-INN | 0.1235 | 0.0325 | 0.0199 | 0.1855 | 0.0251 | 0.0338 |
INN | 0.2281 | 0.4173 | 0.2021 | 0.9457 | 0.1896 | 0.2861 |
DNN | 0.0648 | 0.0629 | 0.0572 | 0.1073 | 0.0700 | 0.0588 |
Data size | Objective 1 | Objective 2 | ||||
50 | 100 | 150 | 50 | 100 | 150 | |
PICP | 1 | 1 | 1 | 0.9833 | 1 | 1 |
PINAW | 1.0489 | 0.4032 | 0.2855 | 0.8948 | 0.3304 | 0.7166 |
Data size | Objective 1 | Objective 2 | ||||
50 | 100 | 150 | 50 | 100 | 150 | |
P-INN | 0.1749 | 0.1392 | 0.1200 | 0.2353 | 0.1579 | 0.1832 |
INN | 0.1595 | 0.2412 | 0.2330 | 0.2982 | 0.2551 | 0.2753 |
DNN | × | × | × | × | × | × |
Data size | Objective 1 | Objective 2 | ||||
50 | 100 | 150 | 50 | 100 | 150 | |
PICP | 0.8417 | 0.9667 | 0.9750 | 0.6750 | 1 | 0.9917 |
PINAW | 0.2971 | 0.3557 | 0.3248 | 0.3287 | 0.4456 | 0.4481 |
Forward RMSE | Inverse RMSE | |||||
Data size | 50 | 100 | 150 | 50 | 100 | 150 |
P-INN | 0.1521 | 0.1115 | 0.0839 | 0.3574 | 0.1286 | 0.1309 |
INN | 3.6367 | 3.9584 | 3.0569 | 0.5253 | 0.4706 | 0.2788 |
DNN | 0.1715 | 0.1662 | 0.1609 | × | × | × |
Data size | PICP | PINAW |
50 | 0.5250 | 0.4321 |
100 | 1 | 0.4803 |
150 | 0.9500 | 0.3570 |
Data size | Forward RMSE | Inverse RMSE | ||||
20 | 40 | 80 | 20 | 40 | 80 | |
P-INN | 0.2017 | 0.1327 | 0.0634 | 0.2894 | 0.2057 | 0.1052 |
INN | 5.0359 | 0.7367 | 0.2194 | 0.4048 | 0.2652 | 0.1564 |
DNN | 0.2110 | 0.2014 | 0.2002 | × | × | × |
Data size | PICP | PINAW |
20 | 0.5000 | 0.4711 |
40 | 1 | 0.6461 |
80 | 1 | 0.4620 |
Data size | Objective 1 | Objective 2 | ||||
50 | 100 | 150 | 50 | 100 | 150 | |
P-INN | 0.1235 | 0.0325 | 0.0199 | 0.1855 | 0.0251 | 0.0338 |
INN | 0.2281 | 0.4173 | 0.2021 | 0.9457 | 0.1896 | 0.2861 |
DNN | 0.0648 | 0.0629 | 0.0572 | 0.1073 | 0.0700 | 0.0588 |
Data size | Objective 1 | Objective 2 | ||||
50 | 100 | 150 | 50 | 100 | 150 | |
PICP | 1 | 1 | 1 | 0.9833 | 1 | 1 |
PINAW | 1.0489 | 0.4032 | 0.2855 | 0.8948 | 0.3304 | 0.7166 |
Data size | Objective 1 | Objective 2 | ||||
50 | 100 | 150 | 50 | 100 | 150 | |
P-INN | 0.1749 | 0.1392 | 0.1200 | 0.2353 | 0.1579 | 0.1832 |
INN | 0.1595 | 0.2412 | 0.2330 | 0.2982 | 0.2551 | 0.2753 |
DNN | × | × | × | × | × | × |
Data size | Objective 1 | Objective 2 | ||||
50 | 100 | 150 | 50 | 100 | 150 | |
PICP | 0.8417 | 0.9667 | 0.9750 | 0.6750 | 1 | 0.9917 |
PINAW | 0.2971 | 0.3557 | 0.3248 | 0.3287 | 0.4456 | 0.4481 |
Forward RMSE | Inverse RMSE | |||||
Data size | 50 | 100 | 150 | 50 | 100 | 150 |
P-INN | 0.1521 | 0.1115 | 0.0839 | 0.3574 | 0.1286 | 0.1309 |
INN | 3.6367 | 3.9584 | 3.0569 | 0.5253 | 0.4706 | 0.2788 |
DNN | 0.1715 | 0.1662 | 0.1609 | × | × | × |
Data size | PICP | PINAW |
50 | 0.5250 | 0.4321 |
100 | 1 | 0.4803 |
150 | 0.9500 | 0.3570 |
Data size | Forward RMSE | Inverse RMSE | ||||
20 | 40 | 80 | 20 | 40 | 80 | |
P-INN | 0.2017 | 0.1327 | 0.0634 | 0.2894 | 0.2057 | 0.1052 |
INN | 5.0359 | 0.7367 | 0.2194 | 0.4048 | 0.2652 | 0.1564 |
DNN | 0.2110 | 0.2014 | 0.2002 | × | × | × |
Data size | PICP | PINAW |
20 | 0.5000 | 0.4711 |
40 | 1 | 0.6461 |
80 | 1 | 0.4620 |