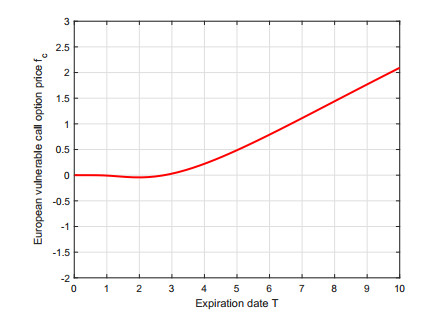
Taxi detour is a chronic problem in urban transport systems, which largely undermines passengers' riding experience and the city's image while unnecessarily worsening traffic congestion. Tourists unfamiliar with city roads often encounter detour problems. Therefore, it is important for regulatory authorities to develop a tool for detour behavior detection in order to discover or identify detours. This study proposes a detour trajectory detection model framework based on the trajectory data of taxis that can identify taxi driving detour fraud at the microscopic level and analyze the characteristics of detouring trajectories from the perspective of microscopic motion traits. The deviation from normal driving trajectories provides a framework for the automatic detection of detour trajectories for the off-site supervision platform of the taxis. Considering drawbacks of the isolation-Based Anomalous Trajectory (iBAT) algorithm, this paper made further improvements in trajectory anomaly detection. In this study, three methods including the iBAT, iBAT + Dynamic Time Warping (DTW), and iBAT + DTW algorithms considering the driving distance and time are compared using the relevant experimental data. The case studies verify that the proposed method outperforms the other methods. Verified by the experiments based on the trajectory data coming from Nanjing, the false positive rate of this framework is only 1.64%.
Citation: Jian Wan, Peiyun Yang, Wenbo Zhang, Yaxing Cheng, Runlin Cai, Zhiyuan Liu. A taxi detour trajectory detection model based on iBAT and DTW algorithm[J]. Electronic Research Archive, 2022, 30(12): 4507-4529. doi: 10.3934/era.2022229
[1] | Mona Alsulami . Existence theory for a third-order ordinary differential equation with non-separated multi-point and nonlocal Stieltjes boundary conditions. AIMS Mathematics, 2023, 8(6): 13572-13592. doi: 10.3934/math.2023689 |
[2] | Haixia Lu, Li Sun . Positive solutions to a semipositone superlinear elastic beam equation. AIMS Mathematics, 2021, 6(5): 4227-4237. doi: 10.3934/math.2021250 |
[3] | Ahmed Alsaedi, Bashir Ahmad, Afrah Assolami, Sotiris K. Ntouyas . On a nonlinear coupled system of differential equations involving Hilfer fractional derivative and Riemann-Liouville mixed operators with nonlocal integro-multi-point boundary conditions. AIMS Mathematics, 2022, 7(7): 12718-12741. doi: 10.3934/math.2022704 |
[4] | Min Jiang, Rengang Huang . Existence of solutions for q-fractional differential equations with nonlocal Erdélyi-Kober q-fractional integral condition. AIMS Mathematics, 2020, 5(6): 6537-6551. doi: 10.3934/math.2020421 |
[5] | Ayub Samadi, Chaiyod Kamthorncharoen, Sotiris K. Ntouyas, Jessada Tariboon . Mixed Erdélyi-Kober and Caputo fractional differential equations with nonlocal non-separated boundary conditions. AIMS Mathematics, 2024, 9(11): 32904-32920. doi: 10.3934/math.20241574 |
[6] | Weerawat Sudsutad, Chatthai Thaiprayoon, Sotiris K. Ntouyas . Existence and stability results for ψ-Hilfer fractional integro-differential equation with mixed nonlocal boundary conditions. AIMS Mathematics, 2021, 6(4): 4119-4141. doi: 10.3934/math.2021244 |
[7] | Bounmy Khaminsou, Weerawat Sudsutad, Jutarat Kongson, Somsiri Nontasawatsri, Adirek Vajrapatkul, Chatthai Thaiprayoon . Investigation of Caputo proportional fractional integro-differential equation with mixed nonlocal conditions with respect to another function. AIMS Mathematics, 2022, 7(6): 9549-9576. doi: 10.3934/math.2022531 |
[8] | Weerawat Sudsutad, Sotiris K. Ntouyas, Chatthai Thaiprayoon . Nonlocal coupled system for ψ-Hilfer fractional order Langevin equations. AIMS Mathematics, 2021, 6(9): 9731-9756. doi: 10.3934/math.2021566 |
[9] | Kishor D. Kucche, Sagar T. Sutar, Kottakkaran Sooppy Nisar . Analysis of nonlinear implicit fractional differential equations with the Atangana-Baleanu derivative via measure of non-compactness. AIMS Mathematics, 2024, 9(10): 27058-27079. doi: 10.3934/math.20241316 |
[10] | Sadam Hussain, Muhammad Sarwar, Kottakkaran Sooppy Nisar, Kamal Shah . Controllability of fractional differential evolution equation of order γ∈(1,2) with nonlocal conditions. AIMS Mathematics, 2023, 8(6): 14188-14206. doi: 10.3934/math.2023726 |
Taxi detour is a chronic problem in urban transport systems, which largely undermines passengers' riding experience and the city's image while unnecessarily worsening traffic congestion. Tourists unfamiliar with city roads often encounter detour problems. Therefore, it is important for regulatory authorities to develop a tool for detour behavior detection in order to discover or identify detours. This study proposes a detour trajectory detection model framework based on the trajectory data of taxis that can identify taxi driving detour fraud at the microscopic level and analyze the characteristics of detouring trajectories from the perspective of microscopic motion traits. The deviation from normal driving trajectories provides a framework for the automatic detection of detour trajectories for the off-site supervision platform of the taxis. Considering drawbacks of the isolation-Based Anomalous Trajectory (iBAT) algorithm, this paper made further improvements in trajectory anomaly detection. In this study, three methods including the iBAT, iBAT + Dynamic Time Warping (DTW), and iBAT + DTW algorithms considering the driving distance and time are compared using the relevant experimental data. The case studies verify that the proposed method outperforms the other methods. Verified by the experiments based on the trajectory data coming from Nanjing, the false positive rate of this framework is only 1.64%.
Probability theory and uncertainty theory are two axiomatic mathematical systems that model uncertain phenomena. Probability theory is based on frequency. Traditionally, probability theory has been used to describe uncertain events, such as the price of the underlying asset in financial derivatives. If your distribution function is close enough to the frequency, using probability theory is fine. In fact, when we can only get small samples or no samples, the distribution function may deviate far from the real frequency. In this case, using probability theory may lead to counterintuitive results. The relevant content can be seen in Chapter 1 of [18]. Furthermore, as early as 1979, Kahneman and Tversky [12] pointed out that people always overestimate the probability of impossible events. Thus people always hope that experts in the field will give the belief degree of an event. In order to rationally deal with the belief degree, Liu [13] founded the uncertainty theory which is an axiomatic mathematical system based on normality, duality, subadditivity and product axioms in 2007. Nowadays uncertainty theory has become a branch of mathematics concerned with the analysis of belief degree. The forward development of a discipline is inseparable from the joint efforts of many scholars (see [4,13,14,15,16,28]).
In 2009, Liu [15] introduced the uncertainty theory into the financial field and proposed an uncertain stock model that follows uncertain differential equations driven by the geometric Liu process. Peng and Yao [22] gave a class of uncertain stock models with mean reversion. Dai et al. [8] gave a nonlinear uncertain stock model. In addition, Yu [29] studied uncertain stock models with jumps and Chen et al. [] also proposed an uncertain stock model with periodic dividends. Many option pricing formulas have been obtained using the above uncertain stock models.
In recent years, with the development of the financial market, hedging and avoiding financial risk have become more and more important, and various types of options have emerged. Default risk, also known as credit risk, comes from the possibility of the default of loan borrowers, bond issuers and derivative counterparties. In general, it has been assumed that options have no default risk when options are traded on an organized exchange. However, the rapid growth in the over-the-counter options has motivated increased attention to the implications of counterparty credit risk. The holders of over-the-counter options are exposed to potential credit risk due to the possibility of their counterparty being unable to make the necessary payments at the expiration date. In 1987, Johnson and Stulz [10] discussed the impact of credit default risk on option prices and called this option with credit risk a vulnerable option. In 2022, Xie and Deng [26] studied the pricing of European vulnerable options under the conditions of Heston stochastic volatility and a stochastic interest rate model. In 2021, Liang and Wang [21] proposed a closed-form hybrid credit risk model to price vulnerable options with stochastic volatility. In 2019, Zhou and Li [30] proposed a method to estimate the price of vulnerable options when the volatility of the underlying assets is within a small interval.
For Cox-Ingersoll-Ross(CIR) interest rate models, most of the related research is carried out in the field of randomization. In 2022, Zheng [31] priced European export-oriented barrier options by using a CIR model. In 2020, Lei [20] studied the asset liability management problem with a CIR interest rate by using a Heston model. In 2019, Sun et al. [23] studied the Euler grid difference method and convergence analysis of CIR interest rate models under the conditions of a fractional jump diffusion environment. In 2018, Chen and Hsu [3] studied barrier option pricing and hedging by using a Markov-modulated double exponential jump diffusion-CIR model. Wu [25] discussed the application of a CIR model in the Chinese market under the condition of a stochastic field in 2017. More references can be seen in [7,9,24]. Although the CIR interest rate model has many applications in the field of randomness, CIR interest rate models have few applications in the field of uncertainty. In this paper, we get the solution of the CIR interest rate model in terms of distribution. Based on the CIR interest rate model, the pricing formulas of the European vulnerable call option and European vulnerable put option are obtained, and the related risks are analyzed.
This paper gives the pricing formulas of vulnerable options as obtained by implementing the uncertain CIR interest rate model. The rest of the article is arranged as follows. In Section 2, we introduce some definitions and theorems that will be used in this article. In Section 3, the solution in terms of the distribution of an uncertain differential equation is given. Sections 4 and 5 give the pricing formulas of the European vulnerable call option and the European vulnerable put option based on a new uncertain vulnerable option pricing model, respectively. In Section 6, in order to obtain the numerical solution, two numerical algorithms are designed to calculate the prices of European vulnerable call options and European vulnerable put options, and numerical examples are given to verify the effectiveness of the numerical algorithm. Finally, a brief summary is given in Section 7.
Definition 2.1. [13] Let Γ be a nonempty set and L be a σ-algebra on Γ. The set function M is called an uncertain measure if it satisfies the following axioms:
Axiom 1. (Normality axiom) M{Γ}=1 for the universal set Γ.
Axiom 2. (Duality axiom) M{Λ}+M{Λc}=1 for any Λ∈L.
Axiom 3. (Subadditivity axiom) For every countable sequence Λ1,Λ2,⋯ in L, we have
M{∞⋃i=1Λi}≤∞∑i=1M{Λi}. |
The triplet (Γ,L,M) is called an uncertainty space. Moreover, the product uncertainty measure was defined by Liu [15] in 2009 as follows.
Axiom 4. (Product axiom) Let (Γk,Lk,Mk) be uncertainty spaces for k=1,2,⋯. The product uncertainty measure M is an uncertainty measure satisfying
M{∞∏k=1Λk}=∞⋀k=1Mk{Λk} |
where Λk denotes arbitrarily chosen events from Lk,k=1,2,⋯.
Definition 2.2. [13] Let ξ be a function from an uncertainty space (Γ,L,M) to the set of real numbers such that ξ∈B is an event for any Borel set B of real numbers; then, the function ξ is called an uncertain variable.
Theorem 2.1. [16] Let ξ1, ξ2, ⋯, ξn be independent uncertain variables with regular uncertainty distributions Φ1, Φ2, ⋯, Φn, respectively. If f(ξ1,ξ2,⋯,ξn) is a strictly increasing function with respect to ξ1, ξ2, ⋯, ξm and a strictly decreasing function with respect to ξm+1, ξm+2, ⋯, ξn, then ξ=f(ξ1,ξ2,⋯,ξn) has an inverse uncertainty distribution
Ψ−1(α)=f(Φ−11(α),⋯,Φ−1m(α),Φ−1m+1(1−α),⋯,Φ−1n(1−α)). |
Definition 2.3. [13] The expected value of an uncertain variable ξ is defined by
E[ξ]=∫+∞0M{ξ≥x}dx−∫0−∞M{ξ≤x}dx |
provided that at least one of the two integrals exists.
Theorem 2.2. [13] Let ξ be an uncertain variable with the uncertainty distribution Φ. Then
E[ξ]=∫+∞0(1−Φ(x))dx−∫0−∞Φ(x)dx. |
Theorem 2.3. [16] Let ξ be an uncertain variable with the regular uncertainty distribution Φ. Then
E[ξ]=∫10Φ−1(α)dα |
where Φ−1(α) is the inverse uncertain distribution of the uncertain variable ξ.
Theorem 2.4. [19] Let ξ1, ξ2, ⋯, ξn be independent uncertain variables with regular uncertainty distributions Φ1, Φ2, ⋯, Φn, respectively. If f(x1,x2,⋯,xn) is a continuous, strictly increasing function with respect to x1, x2, ⋯, xm and a strictly decreasing function with respect to xm+1, xm+2, ⋯, xn, then the uncertain variable ξ=f(ξ1,ξ2,⋯,ξn) has an expected value of
E[ξ]=∫10f(Φ−11(α),⋯,Φ−1m(α),Φ−1m+1(1−α),⋯,Φ−1n(1−α))dα. |
Definition 2.4. [14] Let T be a totally ordered set (e.g. time) and let (Γ,L,M) be an uncertainty space. An uncertain process is a function Xt(γ) from T×(Γ,L,M) to the set of real numbers such that {Xt∈B} is an event for any Borel set B of real numbers at each time t.
Definition 2.5. [15] An uncertain process Ct is said to be a Liu process if
(1) C0=0 and almost all sample paths are Lipschitz continuous;
(2) Ct has stationary and independent increments;
(3) every increments Ct+s−Ct is a normal uncertain variable with an expected value 0 and variance t2.
It is very clear that a Liu process Ct∼N(0,t2), that is, the uncertainty distribution of Ct is
Φt(x)=(1+exp(−πx√3t))−1,x∈ℜ. |
Definition 2.6. [14] Uncertain processes X1t,X2t,⋯,Xnt are said to be independent if for any positive integer k and any times t1,t2,⋯,tk, the uncertain vectors
ξi=(Xit1,Xit2,⋯,Xitk),i=1,2,⋯,n |
are independent, i.e., for any Borel sets B1,B2,⋯,Bn of k-dimensional real vectors, we have
M{n⋂i=1(ξξi∈Bi)}=n⋀i=1M{ξξi∈Bi}. |
Definition 2.7. [17] The uncertainty distribution Φt(x) of an uncertain process Xt is defined by
Φt(x)=M{Xt≤x} |
for any time t and any real number x.
Definition 2.8. [17] Let Xt be an uncertain process with the regular uncertainty distribution Φt(x). Then the inverse function Φ−1t(α) is called the inverse uncertainty distribution of Xt.
Theorem 2.5. [27] Let Xt be a sample-continuous independent increment process with the regular uncertainty distribution Φt(x). Then for any time s>0, the time integral
Ys=∫s0Xtdt |
has an inverse uncertainty distribution
Ψ−1s(α)=∫s0Φ−1t(α)dt. |
Definition 2.9. [28] Let α be a number with 0<α<1. An uncertain differential equation
dXt=f(t,Xt)dt+g(t,Xt)dCt |
is said to have an α-path Xαt if it solves the corresponding ordinary differential equation
dXαt=f(t,Xαt)dt+|g(t,Xαt)|Φ−1(α)dt |
where Φ−1(α) is the inverse uncertainty distribution of standard normal uncertain variables, i.e.,
Φ−1(α)=√3πlnα1−α. |
Theorem 2.6. [28] Let Xt and Xαt be the solution and α-path of the uncertain differential equation
dXt=f(t,Xt)dt+g(t,Xt)dCt |
respectively. Then the solution Xt has an inverse uncertain distribution
Φ−1t(α)=Xαt. |
Theorem 2.7. [27] Let Xt and Xαt be the solution and α-path of the uncertain differential equation
dXt=f(t,Xt)dt+g(t,Xt)dCt |
respectively. Then for any time s>0 and a strictly increasing function J(x), the time integral ∫s0J(Xt)dt has an inverse uncertainty distribution Ψ−1s(α)=∫s0J(Xαt)dt.
In 1985, the well-known interest rate model, the CIR model was proposed for probability theory [6]
drt=(m−art)dt+σ√rtdWt | (3.1) |
where m>0, a≠0 and σ are constants, and Wt is Brownian motion. As its counterpart, Chen and Gao [2] proposed an uncertain interest model based on uncertainty theory in 2013. The interest rate rt follows the next uncertain differential equation driven by the Liu process
drt=(m−art)dt+σ√rtdC1t | (3.2) |
where m>0, a≠0 and σ are constants, and C1t is the Liu process.
Jiao and Yao [11] gave a numerical method of the uncertain interest model and obtained a formula to calculate the price of the zero coupon bond based on it.
Next we will discuss the solution in terms of uncertainty distribution for the expression (3.2).
Theorem 3.1. Let rt be the solution of the uncertain differential equation (3.2) with the inverse uncertainty distribution Ω−1t(α). Then Ω−1t(α) can be expressed in three forms with different values of Δ, where Δ=[σΦ−1(α)]2+4am.
Case I: Δ>0.
A1ln(√Ω−1t(α)−x1)+A2ln(√Ω−1t(α)−x2)=t+A1ln(√r0−x1)+A2ln(√r0−x2) |
where A1=−σΦ−1(α)+√Δ√Δ, A2=σΦ−1(α)+√Δ√Δ, x1=σΦ−1(α)−√Δ2a and x2=σΦ−1(α)+√Δ2a.
Case II: Δ=0.
ln(√Ω−1t(α)−σΦ−1(α)a)−σΦ−1(α)a√Ω−1t(α)−σΦ−1(α)=12t+ln(√r0−σΦ−1(α)a)−σΦ−1(α)a√r0−σΦ−1(α). |
Case III: Δ<0.
−1aln(u2+p)−σΦ−1(α)a2√parctanu√p=t−1aln[(√r0−σΦ−1(α)2a)2+p]−σΦ−1(α)a2√parctan√r0−σΦ−1(α)2a√p |
where p=Δ−4a2 and u=√Ω−1t(α)−σΦ−1(α)2a.
Proof. From Definition 2.9, the uncertain differential equation
drt=(m−art)dt+σ√rtdC1t |
has the α-path
drαt=(m−arαt)dt+σ√rαtΦ−1(α)dt. | (3.3) |
In order to obtain the solution of the differential equation (3.3), we divide it into three cases.
Case I: Δ=[σΦ−1(α)]2+4am>0. For the convenience of presentation, let
A1=−σΦ−1(α)+√Δ√Δ,A2=σΦ−1(α)+√Δ√Δ,x1=σΦ−1(α)−√Δ2a,x2=σΦ−1(α)+√Δ2a. |
By solving Eq (3.3), we can get
A1ln(√rαt−x1)+A2ln(√rαt−x2)=t+c |
where c is an arbitrary constant. Substituting the initial conditions rα0=r0 into the above equation, we get
c=A1ln(√r0−x1)+A2ln(√r0−x2). |
Finally
A1ln(√rαt−x1)+A2ln(√rαt−x2)=12t+A1ln(√r0−x1)+A2ln(√r0−x2). |
By Theorem 2.6, Case I is true.
Case II: Δ=[σΦ−1(α)]2+4am=0. For the convenience of presentation, let A1=1, A2=x1=x2=σΦ−1(α)a.
The general solution of Eq (3.3) is
A1√rαt−x1+A2(√rαt−x1)2=12t+c |
where c is an arbitrary constant. From the initial value rα0=r0
c=A1ln(√r0−x1)−A21√r0−x1. |
Finally we get the special solution of Eq (3.3) as
A1ln(√rαt−x1)−A21√rαt−x1=12t+A1ln(√r0−x1)−A21√r0−x1. |
By Theorem 2.6, the result of Case II be proved.
Case III: Δ=[σΦ−1(α)]2+4am<0. For the convenience of presentation, let p=Δ−4a2, u=√rαt−σΦ−1(α)2a.
In this case, the general solution of Eq (3.3) is
−1aln(u2+p)−σΦ−1(α)a2√parctanu√p=t+c |
where c is an arbitrary constant. By applying the initial value rα0=r0 we get the special solution of Eq (3.3) as
−1aln(u2+p)−σΦ−1(α)a2√parctanu√p=t−1aln[(√r0−σΦ−1(α)a)2+p]−σΦ−1(α)a2√parctan√r0−σΦ−1(α)a√p. |
From Theorem 2.6, the result of Case III is true.
We have completed the proof of this theorem.
As is well known, the European call option is a contract which gives the holder the right rather than the obligation to buy stocks at an expiration date T for a strike price K. The European vulnerable call option supposes that the value of a company is Zt at the time t. Then the option seller promises to give (YT−K)+ to the option holder at the expiration date T. If the option seller cannot give (YT−K)+ to the holder at the expiration date T, the option holder will immediately take over the company. In other words, the final payoff of the option holder at time T is min((YT−K)+,ZT). We assume that the price of this European vulnerable call option is fc. According to the fair price principle, we can get the price of the European vulnerable call option
fc=E[exp(−∫T0rtdt)min((YT−K)+,ZT)]. |
In order to obtain the price of options, Liu [15] first modeled the change in financial asset price by using uncertain differential equations and proposed the Liu model, which is the counterpart of the famous B-S model [1]. From then on, based on the uncertainty theory, some scholars have put forward other uncertain financial derivatives models that are more suitable for market practice, such as the mean reversion stock model [22] and uncertain exponential Ornstein-Uhlenbeck model [8]. The above models always assume that the risk interest rate is a constant. However, in the real financial market, interest rates are often uncertain, so interest rates should follow an uncertain differential equation.
In this paper, let rt, Yt and Zt be the interest rate, stock price and the company value at time t of a European vulnerable option, respectively, and they follow the uncertain differential equations
{drt=(m−art)dt+σ√rtdC1tdYt=e1Ytdt+σ1YtdC2tdZt=e2Ztdt+σ2ZtdC3t | (4.1) |
where m>0, a≠0,e1,e2,σ,σ1 and σ2, are all constants and C1t, C2t and C3t are three independent Liu processes.
Theorem 4.1. Assume that a European vulnerable call option satisfies the model (4.1) with a strike price K and an expiration date T; then, the price of the European vulnerable call option is
fc=∫10Φ−11T(α)⋅(Υ−11T(α)∧Ψ−1T(α))dα | (4.2) |
where Φ−11T(α)=exp(−∫T0Ω−1t(1−α)dt), Υ−11T(α)=(Y0(e1T+√3σ1Tπlnα1−α)−K)+ and Ψ−1T(α)=Z0(e2T+√3σ2Tπlnα1−α).
Proof. The inverse uncertainty distribution Ω−1t(α) of rt has been obtained by Theorem 3.1.
From Theorem 2.5, we can get the inverse uncertainty distribution Φ−1T(α)=∫T0Ω−1t(α)dt of ∫T0rtdt. Since y=exp(−x) is a decreasing function of x, from Theorem 2.1,
exp(−∫T0rtdt) |
has an inverse uncertainty distribution
Φ−11T(α)=exp(−Φ−1T(1−α)). | (4.3) |
Let Yαt be an α-path of the uncertain differential equation
dYt=e1Ytdt+σ1YtdC2t. |
By solving the ordinary differential equation
dYαt=e1Yαtdt+σ1YαtΦ−1(α)dt, |
we can get
Yαt=Y0(e1t+√3σ1tπlnα1−α). |
It follows from Theorem 2.6 that YT has an inverse uncertainty distribution
Υ−1T(α)=YαT. | (4.4) |
Due to (YT−K)+ being an increasing function with respect to YT, by Theorem 2.1, (YT−K)+ has an inverse uncertainty distribution
Υ−11T(α)=(Υ−1T(α)−K)+. |
Similarly, we can get
Zαt=Z0(e2t+√3σ2tπlnα1−α). |
Thus from Theorem 2.6, ZT has an inverse uncertainty distribution
Ψ−1T(α)=ZαT. | (4.5) |
Following Theorem 2.1, the expression
exp(−∫T0rtdt)min((YT−K)+,ZT) |
has an inverse uncertainty distribution
Φ−11T(α)⋅(Υ−11T(α)∧Ψ−1T(α)). |
Thus according to Theorem 2.1 and Theorem 2.3, the price of the European vulnerable call option is shown as follows.
fc=E[exp(−∫T0rtdt)min((YT−K)+,ZT)]=∫10Φ−11T(α)⋅(Υ−11T(α)∧Ψ−1T(α))dα. |
We have completed the proof of this theorem.
In this section, we will give the pricing formulas of the European vulnerable put option. Note that rt, Yt and Zt are the same as in Theorem 4.1.
The European put option is a contract which gives the holder the right rather than the obligation to sell stocks at an expiration date T for a strike price K.
For the European vulnerable put option, the option seller promises to give (K−YT)+ to the option holder at the expiration date T. If the option seller cannot give (K−YT)+ to the holder at the expiration date T, the option holder will immediately take over the company. In other words, the final payoff of the option holder at time T is min((K−YT)+,ZT). We assume that the price of this European vulnerable put option is fp.
According to the fair price principle, we can get the price of the European vulnerable put option
fp=E[exp(−∫T0rtdt)min((K−YT)+,ZT)]. |
Theorem 5.1. Assume that a European vulnerable put option satisfies the uncertain stock model (4.1) with a strick K and an expiration date T; then, the price of the European vulnerable put option is
fp=∫10Φ−11T(α)⋅(Υ−12T(α)∧Ψ−1T(α))dα | (5.1) |
where Φ−11T(α)=exp(−∫T0Ω−1t(1−α)dt), Υ−12T(α)=(K−Y0(e1T+√3σ1Tπln1−αα))+ and Ψ−1T(α)=Z0(e2T+√3σ2Tπlnα1−α).
Proof. Similar to the proof of Eqs (4.3)–(4.5) in Theorem 4.1, the inverse uncertainty distributions of exp(−∫T0rtdt), YT and ZT are exp(−Φ−1T(1−α))≜Φ−11T(α), YαT≜Υ−1T(α) and ZαT≜Ψ−1T(α), respectively. Due to (K−YT)+ being a decreasing function with respect to YT, by Theorem 2.1, (K−YT)+ has an inverse uncertainty distribution
Υ−12T(α)=(K−Υ−1T(1−α))+. |
Following Theorem 2.1, the expression
exp(−∫T0rtdt)min((K−YT)+,ZT) |
has an inverse uncertainty distribution
Φ−11T(α)⋅(Υ−12T(α)∧Ψ−1T(α)). |
Thus according to Theorem 2.1 and Theorem 2.3, the price of the European vulnerable put option is shown as follows.
fp=E[exp(−∫T0rtdt)min((K−YT)+,ZT)]=∫10Φ−11T(α)⋅(Υ−12T(α)∧Ψ−1T(α))dα. |
We have completed the proof of this theorem.
In this section, we design numerical algorithms to calculate the numerical solutions of the European vulnerable call option fc and European vulnerable put option fp, respectively.
Step 0: Set the values of the corresponding parameters a,m,σ,Y0,e1,σ1,Z0,e2,σ2,T and K.
Step 1: Choose two appropriate N and M values according to the required accuracy; set αi=i/N and tj=jT/M, i=1,2,⋯N−1, j=1,2,⋯,M.
Step 2: Set i=0.
Step 3: Set i=i+1.
Step 4: Set j=0.
Step 5: Set j=j+1.
Step 6: For each αi, determine the value of Δ=(√3σπlnαi1−αi)2+4am.
If Δ>0, solve the following ordinary differential equation
A1ln(√Ω−1tj(1−αi)−x1)+A2ln(√Ω−1tj(1−αi)−x2)=tj+A1ln(√r0−x1)+A2ln(√r0−x2) |
where
A1=−σΦ−1(1−αi)+√[σΦ−1(1−αi)]2+4am√[σΦ−1(1−αi)]2+4am, A2=σΦ−1(1−αi)+√[σΦ−1(1−αi)]2+4am√[σΦ−1(1−αi)]2+4am, x1=σΦ−1(1−αi)−√[σΦ−1(1−αi)]2+4am2a, x2=σΦ−1(1−αi)+√[σΦ−1(1−αi)]2+4am2a.
Else if Δ=0, solve the following ordinary differential equation
ln(√Ω−1tj(1−αi)−σΦ−1(1−αi)a)−σΦ−1(1−αi)a√Ω−1tj(1−αi)−σΦ−1(1−αi)=12tj+ln(√r0−σΦ−1(1−αi)a)−σΦ−1(1−αi)a√r0−σΦ−1(1−αi). |
Else if Δ<0, solve the following ordinary differential equation
−1aln(u2+p)−σΦ−1(1−αi)a2√parctanu√p=tj−1aln[(√r0−σΦ−1(1−αi)2a)2+p]−σΦ−1(1−αi)a2√parctan√r0−σΦ−1(1−αi)2a√p |
where p=[σΦ−1(1−αi)]2+4am−4a2 and u=√Ω−1tj(1−αi)−σΦ−1(1−αi)2a.
If j<M, return to Step 5.
Step 7: Calculate the discount rate
exp(−∫T0Ω−1s(1−αi)ds)→exp(−TMM∑j=1Ω−1tj(1−αi)) |
and
Υ−11T(αi)=(Υ−1T(αi)−K)+=max(Y0(e1T+√3σ1Tπlnαi1−αi)−K,0). |
Step 8: It is obvious that
Ψ−1T(αi)=Z0(e2T+√3σ2Tπlnαi1−αi). |
Step 9: Calculate
exp(−∫T0Ω−1s(1−αi)ds)min(Υ−11T(αi),Ψ−1T(αi)); |
if i<N−1, return Step 3.
Step 10: fc→1N−1∑N−1i=1exp(−∫T0Ω−1s(1−αi)ds)min(Υ−11T(αi),Ψ−1T(αi)).
Example 6.1. For the European vulnerable call option pricing formula (6), we assume the M=100,N=100,a=0.001,m=0.002,σ=5∗10−5,Y0=5,e1=0.1,σ1=π10√3,Z0=10,e2=0.2,σ2=π8√3,T=5 and K=3.6. Thus the European vulnerable call option price is fc=0.49944278. When the parameters aside from the parameter expiration date T remain unchanged, the relationship between the price of the European vulnerable call option fc and the expiration date T is as shown in Figure 1. When all parameters except for the parameter volatility σ1 remain unchanged, the relationship between the price of the European vulnerable call option fc and volatility σ1 is as shown in Figure 2. When all parameters except for the parameter log-drift e1 remain unchanged, the relationship between the price of the European vulnerable call option fc and the log-drift e1 is as shown in Figure 3.
It can be seen in Figure 1 that with the increase of the expiration date T, the price of European vulnerable call options shows an overall upward trend. When the expiration date T is in the range of [0, 3], the price changes are not obvious, and the price shows a slow growth trend in the range of [3, 10]. Although the direction of time is deterministic, the trend of expiration T is still a focus of attention. As can be seen in Figure 2, with the increase of volatility σ1, the price of European vulnerable call options shows an overall upward trend. When the volatility is in the range of [0, 0.04], the option price is negative, indicating that the power of the sellers is far greater than that of the buyers. At this time, the investor should carefully consider the future prospect of this option according to their own risk preference. In the later period, with the increase of volatility σ1, the option price shows an upward trend. The slope of the figure represents the vega of the option price, which is the ratio of the change in the corresponding option price to the change in the volatility of the underlying asset. It can be seen in the figure that the vega presents a trend of first increasing and then decreasing under the conditions of this uncertain model. This indicates that the sensitivity of the European vulnerable call option price to volatility changes increases first and then decreases. At this time, it is best to frist buy and then sell the corresponding stock in the form of dynamic hedging, so as to ensure risk neutrality. It can be seen in Figure 3 that with the increase of log-drift e1, the price of European vulnerable call options shows an overall upward trend. When investing, investors should combine log-drift e1 and other indicators to reasonably choose the time to buy and sell.
The first six steps of the numerical algorithm for European vulnerable put options are the same as those for the European vulnerable call option.
Step 7: Calculate the discount rate
exp(−∫T0Ω−1s(1−αi)ds)→exp(−TMM∑j=1Ω−1tj(1−αi)) |
and
Υ−12T(αi)=(K−Υ−1T(1−αi))+=max(K−Y0(e1T+√3σ1Tπln1−αiαi),0). |
Step 8: It is obvious that
Ψ−1T(αi)=Z0(e2T+√3σ2Tπlnαi1−αi). |
Step 9: Calculate
exp(−∫T0Ω−1s(1−αi)ds)min(Υ−12T(αi),Ψ−1T(αi)); |
if i<N−1, return Step 3.
Step 10: fp→1N−1∑N−1i=1exp(−∫T0Ω−1s(1−αi)ds)min(Υ−12T(αi),Ψ−1T(αi)).
Example 6.2. For the European vulnerable put option pricing formula (7), we assume that M=100,N=100,a=0.001,m=0.002,σ=5∗10−5,Y0=5,e1=0.1,σ1=π10√3,Z0=10,e2=0.2,σ2=π8√3,T=5 and K=3.6. Thus the European vulnerable put option price is fp=1.3576985. When all parameters except for the parameter expiration date T remain unchanged, the relationship between the price of the European vulnerable put option fp and the expiration date T is as shown in Figure 4. When all parameters except for the parameter volatility σ1 remain unchanged, the relationship between the price of the European vulnerable put option fp and volatility σ1 is as shown in Figure 5. When all parameters except for the parameter log-drift e1 remain unchanged, the relationship between the price of the European vulnerable put option fp and the log-drift e1 is as shown in Figure 6.
It can be seen in Figure 4 that with the increase of the expiration date T, the price of the European vulnerable put option generally increases first and then decreases. When the expiration date T is in the range of [0, 1.6], the price gradually increases, and in the range of [1.6, 10], the price gradually decreases. As can be seen in Figure 5, with the increase of volatility σ1, the price of European vulnerable put options shows an overall upward trend. When the volatility is in the range of [0, 0.04], option prices are negative. In the range of [0.04, 0.45], with the increase of volatility σ1, the option prices show an upward trend. At this time, the vega is not very sensitive to changes in the current underlying asset, so there is no need to make significant adjustments. It can be seen in Figure 6 that with the increase of log-drift e1, the price of the European vulnerable put option shows a downward trend. When investing, investors should combine log-drift e1 and other indicators to reasonably choose the time to buy and sell.
In this paper, first, we obtained the solution in terms of the distribution of the uncertain CIR interest rate model. Second, we obtained the pricing formulas of the European vulnerable call option and European vulnerable put option in the model. Finally, according to the the above pricing formulas, the corresponding numerical algorithms and numerical examples were given to verify them.
This work was supported by the Colleges and Universities in Hebei Province Science and Technology Research Project (ZD2019047) and the National Natural Science Foundation (61806133).
The authors declare that they have no conflicts of interest.
[1] |
Q. Cheng, Z. Liu, Y. Lin, X. S. Zhou, An s-shaped three-parameter (S3) traffic stream model with consistent car following relationship, Transp. Res. Part B Methodol., 153 (2021), 246-271. https://doi.org/10.1016/j.trb.2021.09.004 doi: 10.1016/j.trb.2021.09.004
![]() |
[2] |
H. Wang, Transportation-enabled urban services: A brief discussion, Mutimodal Transp., 1 (2022), 100007. https://doi.org/10.1016/j.multra.2022.100007 doi: 10.1016/j.multra.2022.100007
![]() |
[3] | M. Ester, H. P. Kriegel, J. Sander, X. Xu, A density-based algorithm for discovering clusters in large spatial databases with noise, in Kdd, AAAI, 96 (1996), 226-231. |
[4] |
Q. Cheng, Z. Liu, J. Guo, X. Wu, R. Pendyala, B. Belezamo, et al., Estimating key traffic state parameters through parsimonious spatial queue models, Transp. Res. Part C Emerging Technol., 137 (2022), 103596. https://doi.org/10.1016/j.trc.2022.103596 doi: 10.1016/j.trc.2022.103596
![]() |
[5] |
Z. Liu, Y. Wang, Q. Cheng, H. Yang, Analysis of the information entropy on traffic flows, IEEE Trans. Intell. Transp. Syst., 2022 (2022), 1-12. https://doi.org/10.1109/TITS.2022.3155933 doi: 10.1109/TITS.2022.3155933
![]() |
[6] |
D. Huang, J. Xing, Z. Liu, Q. An, A multi-stage stochastic optimization approach to the stop-skipping and bus lane reservation schemes, Transportmetrica A Transp. Sci., 17 (2021), 1272-1304. https://doi.org/10.1080/23249935.2020.1858206 doi: 10.1080/23249935.2020.1858206
![]() |
[7] | F. Giannotti, M. Nanni, F. Pinelli, D. Pedreschi, Trajectory pattern mining, in Proceedings of the 13th ACM SIGKDD International Conference on Knowledge Discovery and Data Mining, ACM, San Jose, USA, (2007), 330-339. https://doi.org/10.1145/1281192.1281230 |
[8] | I. Syarif, A. Prugel-Bennett, G. Wills, Data mining approaches for network intrusion detection: from dimensionality reduction to misuse and anomaly detection, J. Inf. Technol. Rev., 3 (2012), 70-83. |
[9] |
Y. Yue, H. D. Wang, B. Hu, Q. Li, Y. G. Li, A. G. Yeh, Exploratory calibration of a spatial interaction model using taxi GPS trajectories, Comput. Environ. Urban Syst., 36 (2012), 140-153. https://doi.org/10.1016/j.compenvurbsys.2011.09.002 doi: 10.1016/j.compenvurbsys.2011.09.002
![]() |
[10] |
Q. Cheng, Y. Chen, Z. Liu, A bi-level programming model for the optimal lane reservation problem, Expert Syst. Appl., 189 (2022), 116147. https://doi.org/10.1016/j.eswa.2021.116147 doi: 10.1016/j.eswa.2021.116147
![]() |
[11] | G. Münz, S. Li, G. Carle, Traffic anomaly detection using k-means clustering, in GI/ITG Workshop MMBnet, 7 (2007), 9. |
[12] | I. N. Junejo, O. Javed, M. Shah, Multi feature path modeling for video surveillance, in Proceedings of the 17th International Conference on Pattern Recognition, IEEE, Cambridge, UK, 2 (2004), 716-719. https://doi.org/10.1109/ICPR.2004.1334359 |
[13] |
Q. Meng, P. Liu, Z. Liu, Integrating multimodal transportation research, J. Multimodal Transport., 1 (2022), 100001. https://doi.org/10.1016/j.multra.2022.100001 doi: 10.1016/j.multra.2022.100001
![]() |
[14] |
Y. Zheng, Trajectory data mining: an overview, ACM Trans. Intell. Syst. Technol. (TIST), 6 (2015), 1-41. https://doi.org/10.1145/2743025 doi: 10.1145/2743025
![]() |
[15] |
Z. Feng, Y. Zhu, A survey on trajectory data mining: techniques and applications, IEEE Access, 4 (2016), 2056-2067. https://doi.org/10.1109/ACCESS.2016.2553681 doi: 10.1109/ACCESS.2016.2553681
![]() |
[16] |
N. Paragios, R. Deriche, Geodesic active regions: a new framework to deal with frame partition problems in computer vision, J. Visual Commun. Image Represent., 13 (2002), 249-268. https://doi.org/10.1006/jvci.2001.0475 doi: 10.1006/jvci.2001.0475
![]() |
[17] | C. Stauffer, W. E. Grimson, Adaptive background mixture models for real-time tracking, in 1999 IEEE Computer Society Conference on Computer Vision and Pattern Recognition (Cat. No PR00149), IEEE, Collins, USA, 2 (1999), 246-252. https://doi.org/10.1109/CVPR.1999.784637 |
[18] |
S. Coşar, G. Donatiello, V. Bogorny, C. Garate, L. O. Alvares, F. Bremond, Toward abnormal trajectory and event detection in video surveillance, IEEE Trans. Circuits Syst. Video Technol., 27 (2016), 683-695. https://doi.org/10.1109/TCSVT.2016.2589859 doi: 10.1109/TCSVT.2016.2589859
![]() |
[19] |
J. Huo, X. Fu, Z. Liu, Q. Zhang, Short-term estimation and prediction of pedestrian density in urban hot spots based on mobile phone data, IEEE Trans. Intell. Transp. Syst., 23 (2022), 10827-10838. https://doi.org/10.1109/TITS.2021.3096274 doi: 10.1109/TITS.2021.3096274
![]() |
[20] |
D. Huang, Y. Wang, S. Jia, Z. Liu, A Lagrangian relaxation approach for the electric bus charging scheduling optimisation problem, Transportmetrica A Transp. Sci., 2022 (2022), 1-24. https://doi.org/10.1080/23249935.2021.2023690 doi: 10.1080/23249935.2021.2023690
![]() |
[21] |
J. Simon, Remote supply revisited: the jeep problem with costly transfer points, Multimodal Transp., 1 (2022), 100019. https://doi.org/10.1016/j.multra.2022.100019 doi: 10.1016/j.multra.2022.100019
![]() |
[22] |
J. Qiu, K. Huang, J. Hawkins, The taxi sharing practices: matching, routing and pricing methods, Multimodal Transp., 1 (2022), 100003. https://doi.org/10.1016/j.multra.2022.100003 doi: 10.1016/j.multra.2022.100003
![]() |
[23] | A. T. Palma, V. Bogorny, B. Kuijpers, L. O. Alvares, A clustering-based approach for discovering interesting places in trajectories, in Proceedings of the 2008 ACM Symposium on Applied Computing, ACM, Fortaleza, Brazil, (2008), 863-868. https://doi.org/10.1145/1363686.1363886 |
[24] |
L. Grady, E. L. Schwartz, Isoperimetric graph partitioning for image segmentation, IEEE Trans. Pattern Anal. Mach. Intell., 28 (2006), 469-475. https://doi.org/10.1109/TPAMI.2006.57 doi: 10.1109/TPAMI.2006.57
![]() |
[25] | L. Zhao, G. Shi, J. Yang, An adaptive hierarchical clustering method for ship trajectory data based on DBSCAN algorithm, in 2017 IEEE 2nd International Conference on Big Data Analysis (ICBDA), IEEE, Beijing, China, (2017), 329-336. https://doi.org/10.1109/ICBDA.2017.8078834 |
[26] |
Y. Xi, D. Huang, Y. Yuan, Z. Liu, K. Anish, N. Zheng, Improved dynamic time warping algorithm for bus route trajectory curve fitting, J. Transp. Eng., 147 (2021), 04021044. https://doi.org/10.1061/JTEPBS.0000544 doi: 10.1061/JTEPBS.0000544
![]() |
[27] | R. F. Ibrahim, A recommendation system based on clustering and classification for optimal trajectory analysis, PhD thesis, Carleton University, 2019. https://doi.org/10.22215/etd/2019-13400 |
[28] |
M. Khashei, M. Bijari, A novel hybridization of artificial neural networks and ARIMA models for time series forecasting, Appl. Soft Comput., 11 (2011), 2664-2675. https://doi.org/10.1016/j.asoc.2010.10.015 doi: 10.1016/j.asoc.2010.10.015
![]() |
[29] |
Y. Yuan, W. Zhang, X. Yang, Y. Liu, Z. Liu, W. Wang, Traffic state classification and prediction based on trajectory data, J. Intell. Transp. Syst., 2021 (2021), 1-15. https://doi.org/10.1080/15472450.2021.1955210 doi: 10.1080/15472450.2021.1955210
![]() |
[30] |
V. Hodge, J. Austin, A survey of outlier detection methodologies, Artif. Intell. Rev., 22 (2004), 85-126. https://doi.org/10.1023/B:AIRE.0000045502.10941.a9 doi: 10.1023/B:AIRE.0000045502.10941.a9
![]() |
[31] | S. Y. Huang, Y. N. Huang, Network traffic anomaly detection based on growing hierarchical SOM, in 2013 43rd Annual IEEE/IFIP International Conference on Dependable Systems and Networks (DSN), IEEE, Budapest, Hungary, (2013), 1-2. https://doi.org/10.1109/DSN.2013.6575338 |
[32] | A. S. da Silva, J. A. Wickboldt, L. Z. Granville, A. Schaeffer-Filho, ATLANTIC: A framework for anomaly traffic detection, classification, and mitigation in SDN, in NOMS 2016-2016 IEEE/IFIP Network Operations and Management Symposium, IEEE, Istanbul, Turkey, (2016), 27-35. https://doi.org/10.1109/NOMS.2016.7502793 |
[33] |
K. K. Santhosh, D. P. Dogra, P. P. Roy, Anomaly detection in road traffic using visual surveillance: A survey, ACM Comput. Surv. (CSUR), 53 (2020), 1-26. https://doi.org/10.1145/3417989 doi: 10.1145/3417989
![]() |
[34] | S. Chawla, Y. Zheng, J. Hu, Inferring the root cause in road traffic anomalies, in 2012 IEEE 12th International Conference on Data Mining, IEEE, Brussels, Belgium, (2012), 141-150. https://doi.org/10.1109/ICDM.2012.104 |
[35] |
P. R. Lei, A framework for anomaly detection in maritime trajectory behavior, Knowl. Inf. Syst., 47 (2016), 189-214. https://doi.org/10.1007/s10115-015-0845-4 doi: 10.1007/s10115-015-0845-4
![]() |
[36] |
J. Wang, I. C. Paschalidis, Statistical traffic anomaly detection in time-varying communication networks, IEEE Trans. Control Network Syst., 2 (2014), 100-111. https://doi.org/10.1109/TCNS.2014.2378631 doi: 10.1109/TCNS.2014.2378631
![]() |
[37] |
E. M. Knorr, R. T. Ng, V. Tucakov, Distance-based outliers: Algorithms and applications, VLDB J., 8 (2000), 237-253. https://doi.org/10.1007/s007780050006 doi: 10.1007/s007780050006
![]() |
[38] | E. M. Knorr, R.T. Ng, Finding intensional knowledge of distance-based outliers, in Vldb, 99 (1999), 211-222. |
[39] | J. G. Lee, J. Han, X. Li, Trajectory outlier detection: A partition-and-detect framework, in 2008 IEEE 24th International Conference on Data Engineering, IEEE, Cancun, Mexico, (2008), 140-149. https://doi.org/10.1109/ICDE.2008.4497422 |
[40] |
S. A. Ahmed, D. P. Dogra, S. Kar, P. P. Roy, Surveillance scene representation and trajectory abnormality detection using aggregation of multiple concepts, Expert Syst. Appl., 101 (2018), 43-55. https://doi.org/10.1016/j.eswa.2018.02.013 doi: 10.1016/j.eswa.2018.02.013
![]() |
[41] | Y. Ge, H. Xiong, Z. Zhou, H. Ozdemir, J. Yu, K. C. Lee, Top-eye: top-k evolving trajectory outlier detection, in Proceedings of the 19th ACM International Conference on Information and Knowledge Management, ACM, Toronto, Canada, (2010), 1733-1736. https://doi.org/10.1145/1871437.1871716 |
[42] | W. Qin, J. Tang, C. Lu, S. Lao, A trajectory abnormal detection method based on segmentation and clustering, in Journal of Physics: Conference Series, 2010 (2021), 012188. https://doi.org/10.1088/1742-6596/2010/1/012188 |
[43] |
X. Zhao, Y. Rao, J. Cai, W. Ma, Abnormal trajectory detection based on a sparse subgraph, IEEE Access, 8 (2020), 29987-30000. https://doi.org/10.1109/ACCESS.2020.2972299 doi: 10.1109/ACCESS.2020.2972299
![]() |
[44] |
Z. Ding, M. Fei, An anomaly detection approach based on isolation forest algorithm for streaming data using sliding window, IFAC Proc. Vol., 46 (2013), 12-17. https://doi.org/10.3182/20130902-3-CN-3020.00044 doi: 10.3182/20130902-3-CN-3020.00044
![]() |
[45] | D. Xu, Y. Wang, Y. Meng, Z. Zhang, An improved data anomaly detection method based on isolation forest, in 2017 10th International Symposium on Computational Intelligence and Design (ISCID), IEEE, Hangzhou, China, 2 (2017), 287-291. https://doi.org/10.1109/ISCID.2017.202 |
[46] | Z. Cheng, C. Zou, J. Dong, Outlier detection using isolation forest and local outlier factor, in Proceedings of the Conference on Research in Adaptive and Convergent Systems, ACM, Chongqing, China, (2019), 161-168. https://doi.org/10.1145/3338840.3355641 |
1. | 云康 杜, Pricing of Lookback Options under the Time-Fractional CIR Model, 2024, 14, 2161-0967, 1539, 10.12677/FIN.2024.144159 | |
2. | Junkee Jeon, Geonwoo Kim, Valuation of Commodity-Linked Bond with Stochastic Convenience Yield, Stochastic Volatility, and Credit Risk in an Intensity-Based Model, 2023, 11, 2227-7390, 4969, 10.3390/math11244969 |