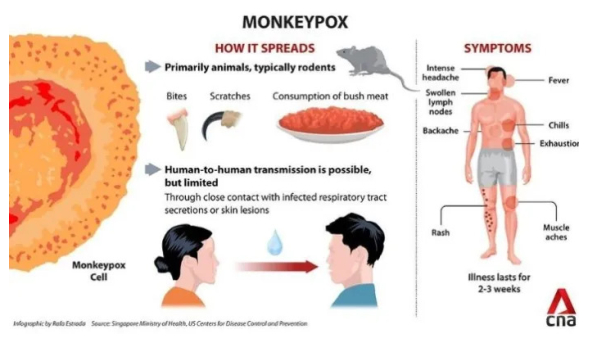
Monkeypox (MPX) is a zoonotic illness that is analogous to smallpox. Monkeypox infections have moved across the forests of Central Africa, where they were first discovered, to other parts of the world. It is transmitted by the monkeypox virus, which is a member of the Poxviridae species and belongs to the Orthopoxvirus genus. In this article, the monkeypox virus is investigated using a deterministic mathematical framework within the Atangana-Baleanu fractional derivative that depends on the generalized Mittag-Leffler (GML) kernel. The system's equilibrium conditions are investigated and examined for robustness. The global stability of the endemic equilibrium is addressed using Jacobian matrix techniques and the Routh-Hurwitz threshold. Furthermore, we also identify a criterion wherein the system's disease-free equilibrium is globally asymptotically stable. Also, we employ a new approach by combining the two-step Lagrange polynomial and the fundamental concept of fractional calculus. The numerical simulations for multiple fractional orders reveal that as the fractional order reduces from 1, the virus's transmission declines. The analysis results show that the proposed strategy is successful at reducing the number of occurrences in multiple groups. It is evident that the findings suggest that isolating affected people from the general community can assist in limiting the transmission of pathogens.
Citation: Maysaa Al Qurashi, Saima Rashid, Ahmed M. Alshehri, Fahd Jarad, Farhat Safdar. New numerical dynamics of the fractional monkeypox virus model transmission pertaining to nonsingular kernels[J]. Mathematical Biosciences and Engineering, 2023, 20(1): 402-436. doi: 10.3934/mbe.2023019
[1] | Emmanuel Addai, Mercy Ngungu, Musibau Abayomi Omoloye, Edmore Marinda . Modelling the impact of vaccination and environmental transmission on the dynamics of monkeypox virus under Caputo operator. Mathematical Biosciences and Engineering, 2023, 20(6): 10174-10199. doi: 10.3934/mbe.2023446 |
[2] | Asad Khan, Yassine Sabbar, Anwarud Din . Stochastic modeling of the Monkeypox 2022 epidemic with cross-infection hypothesis in a highly disturbed environment. Mathematical Biosciences and Engineering, 2022, 19(12): 13560-13581. doi: 10.3934/mbe.2022633 |
[3] | Alia M. Alzubaidi, Hakeem A. Othman, Saif Ullah, Nisar Ahmad, Mohammad Mahtab Alam . Analysis of Monkeypox viral infection with human to animal transmission via a fractional and Fractal-fractional operators with power law kernel. Mathematical Biosciences and Engineering, 2023, 20(4): 6666-6690. doi: 10.3934/mbe.2023287 |
[4] | H. M. Srivastava, Khaled M. Saad, J. F. Gómez-Aguilar, Abdulrhman A. Almadiy . Some new mathematical models of the fractional-order system of human immune against IAV infection. Mathematical Biosciences and Engineering, 2020, 17(5): 4942-4969. doi: 10.3934/mbe.2020268 |
[5] | Jutarat Kongson, Chatthai Thaiprayoon, Apichat Neamvonk, Jehad Alzabut, Weerawat Sudsutad . Investigation of fractal-fractional HIV infection by evaluating the drug therapy effect in the Atangana-Baleanu sense. Mathematical Biosciences and Engineering, 2022, 19(11): 10762-10808. doi: 10.3934/mbe.2022504 |
[6] | Maysaa Al Qurashi, Saima Rashid, Fahd Jarad . A computational study of a stochastic fractal-fractional hepatitis B virus infection incorporating delayed immune reactions via the exponential decay. Mathematical Biosciences and Engineering, 2022, 19(12): 12950-12980. doi: 10.3934/mbe.2022605 |
[7] | Maysaa Al Qurashi, Saima Rashid, Sobia Sultana, Hijaz Ahmad, Khaled A. Gepreel . New formulation for discrete dynamical type inequalities via h-discrete fractional operator pertaining to nonsingular kernel. Mathematical Biosciences and Engineering, 2021, 18(2): 1794-1812. doi: 10.3934/mbe.2021093 |
[8] | Saima Rashid, Rehana Ashraf, Qurat-Ul-Ain Asif, Fahd Jarad . Novel stochastic dynamics of a fractal-fractional immune effector response to viral infection via latently infectious tissues. Mathematical Biosciences and Engineering, 2022, 19(11): 11563-11594. doi: 10.3934/mbe.2022539 |
[9] | Tahir Khan, Roman Ullah, Gul Zaman, Jehad Alzabut . A mathematical model for the dynamics of SARS-CoV-2 virus using the Caputo-Fabrizio operator. Mathematical Biosciences and Engineering, 2021, 18(5): 6095-6116. doi: 10.3934/mbe.2021305 |
[10] | Yan Wang, Tingting Zhao, Jun Liu . Viral dynamics of an HIV stochastic model with cell-to-cell infection, CTL immune response and distributed delays. Mathematical Biosciences and Engineering, 2019, 16(6): 7126-7154. doi: 10.3934/mbe.2019358 |
Monkeypox (MPX) is a zoonotic illness that is analogous to smallpox. Monkeypox infections have moved across the forests of Central Africa, where they were first discovered, to other parts of the world. It is transmitted by the monkeypox virus, which is a member of the Poxviridae species and belongs to the Orthopoxvirus genus. In this article, the monkeypox virus is investigated using a deterministic mathematical framework within the Atangana-Baleanu fractional derivative that depends on the generalized Mittag-Leffler (GML) kernel. The system's equilibrium conditions are investigated and examined for robustness. The global stability of the endemic equilibrium is addressed using Jacobian matrix techniques and the Routh-Hurwitz threshold. Furthermore, we also identify a criterion wherein the system's disease-free equilibrium is globally asymptotically stable. Also, we employ a new approach by combining the two-step Lagrange polynomial and the fundamental concept of fractional calculus. The numerical simulations for multiple fractional orders reveal that as the fractional order reduces from 1, the virus's transmission declines. The analysis results show that the proposed strategy is successful at reducing the number of occurrences in multiple groups. It is evident that the findings suggest that isolating affected people from the general community can assist in limiting the transmission of pathogens.
Monkeypox (MPX) is a burgeoning contagious agent with a gradually increasing recurrence incidence and predicted breakout magnitude in human groups [1]. Inflammation, swollen glands and a dermatitis that produces blisters and then scabs over are among the symptoms (Figure 1). From the period of testing to the beginning of complications, it might take anywhere from 5 to 21 days. Symptoms last about 2 to 4 weeks on average. MPX infections have travelled beyond the woodlands of Central Africa, where they were first discovered, to various regions of the globe, where they are being transported. This mechanism of propagation is most probably connected to a global reduction in orthopoxvirus susceptibility following the termination of smallpox vaccination after the disease was declared eliminated in 1980. As a result, MPX could become the least common orthopoxvirus epidemic in human history [2]. The outbreak capability of MPX will tend to increase in a community exhibiting falling protective immunization versus orthopoxvirus organisms, according to mathematical techniques.
Furthermore, the MPX infection, a species of the orthopoxvirus genera in the Poxviridae category, causes MPX. The variola virus (which causes smallpox), chicken pox infection and adenovirus bacterial infection are all members of this family. Smallpox and MPX have common physiological manifestations, with MPX exhibiting lymphadenopathy slightly earlier in the illness phase as a differentiating characteristic [2]. Cowpox contamination results in long-lasting immunization; chickenpox recurrence incidence is only approximately 1 in 1000 over 15–20 years [4]. A first vaccine against cowpox with attenuated virus produces long-lasting resistance with an 80–95 % effectiveness. Prospective researchers have reported that prevention can persist for a considerable time. The present recommendation for amendment was introduced a decade ago [5]. Vaccinia has also been shown to provide long-lasting protection against MPX, with an effectiveness of 85 % [6]. Additionally, investigations of immunogenicity to orthopoxvirus genera imply that cowpox and MPX have excellent meld.
There have never been any reports of smallpox and MPX outbreaks occurring at the same time. Smallpox is a deadly pathogen, whereas MPX is a zoonotic virus that is highly contagious to humans via an unexplained arthropod vector. Interactions between mammalian genera in northern and southern African jungles, particularly in the northern African countries, the democratic states of the Congo, Cameroon, and Nigeria, culminate in intermittent MPX invasions into living creature species. All MPX breakouts have been self-contained, having living organism infection networks stop before outbreaks could develop [2]. MPX looks to be emerging as the most prevalent viral illness in people following the elimination of smallpox (Figure 2). People are generally understood to be at low danger of an outbreak [7].
Recently, MPX has come to be known as complex and requires a mammalian repository at this time since MPX human-to-human dissemination networks are comparatively limited; the greatest quantity of iterations described in research is seven [9]. However, as the H1N1 pandemic (swine flu) demonstrated, certain viral modifications can improve infectious viability in humans [10]. They have longitudinal, dual DNA chromosomes ranging from 130 to 230 kbp, and so they evolve at a far more moderate pace than H1N1. Despite this, they can adjust quickly [11], and genomic manipulation and contemporary molecular genetics have successfully transformed a mousepox infection into a particularly dangerous species [12].
To fully comprehend the unintended consequences of bacterial contamination and transmission, etiologic variational systems have been developed [13, 14]. The structure for a mathematical equation for MPX has been roughly established, but previous incarnations have experienced flaws in trying to acknowledge several of the virus's conditions associated with their completeness. Despite the fact that Bhunu and Mushayabasa [15] established a fundamental SIR vector-borne variational framework between humans and primates, they dismiss the possibility of an invasive condition in people. Usman and Adamu [16] extended this paradigm by integrating an SVEIR dimensional ability to estimate the virus's persistence duration and vaccination efficacy.
Fractional calculus adds dimensions to the explanation of complex phenomena in physical processes involving memory impacts. However, researchers face significant challenges in defining such phenomena. This is because traditional fractional formulations have a unique kernel and therefore might not be equipped to adequately capture the non-locality of meaningful phenomena. Novel fractional derivatives featuring non-singular kernels have been proposed and implemented for pragmatic reasons in an attempt to accurately characterize nonlocal phenomena. The Mittag-Leffler (ML) [17] expressions are one of the leading contenders among known descriptions.
One of the most significant advantages of this novel derivative is that it exhibits innovative asymptotic behaviours that are distinct from those of fractional derivatives in their simplest rendition [18, 19, 20, 21]. Furthermore, authentic mechanisms are being employed to investigate the properties of these adaptations, and appropriate numerical techniques should be refined to enable them to be accessible in application. To put it differently, the Atangana–Baleanu operator in the Caputo interpretation has an ML form kernel that permits it to preserve the Riemann–Liouville and Caputo derivatives for subsequent duration but just the Caputo–Fabrizio derivative for earlier generations [22, 23, 24, 25]. The Atangana–Baleanu operator, more specifically, can encapsulate Brownian and unpredictable behavior, yielding crossover behavior. Careful analysis revealed that the Atangana–Baleanu operator can also represent strong predictive configurations, such as the multivariate Gaussian dispersion.
In new findings, it has been discovered that fractional-order formulations, in correlation with conventional ordinary formulae, have a stronger power to predict the non-local and unpredictable characteristics of diverse infections such as pneumonia-meningitis [26], diabetes [27], cholera [28], gastroenteritis [29], tuberculosis [30], hepatitis B virus [31], oncolytic virus [32], scabies [32] and several others [33, 34, 35]. Other scientific and mechanical frameworks [36, 37, 38] are advantageously adjusted in the domain of fractional derivatives because the discipline of fractional calculus includes the robust instruments of modeling a realistic world exhibiting significant memory consequences and irregularities [39, 40, 41].
The primary goal of this work was to analyze a novel fractional framework characterizing MPX and it was inspired by the preceding discussion and the work reported in [42, 43]. An extensive examination of the MPX model under fractional-order differential operators with the Atangana–Baleanu in the Caputo context was motivated by the flexibility of providing accessibility for clarity purposes. The Atangana-Baleanu fractional operator in the Caputo sense has been used to investigate the suggested system. An efficacious numerical approach proposed by the authors of [44] has been indeed adopted to handle these issues successfully. The following are the primary characteristics of the important milestones described in this article:
● The new technique just involves overcoming a simple recurrent mathematical expression for the proposed fractional operator. This research also includes an analysis of the indicated tool's existence-uniqueness that uses fixed point postulates. In comparison to other conventional systems, these features allow the suggested methodology to be inexpensive and simple to execute.
● The qualitative analysis of the MPX virus is discussed from a fractional standpoint.
● The stabilities of the disease-free and endemic equilibria are presented out in a detailed manner, taking into account the Routh-Hurwitz threshold.
● The modeling results show that emerging projections rely on a fractional operator and exhibit significantly more asymptomatic behavior than classical systems.
● The novel fractional form featuring ML kernels generates projections that are significantly correlated by using a handful of quantified evidence, according to numerical studies.
As a result, fractional calculus makes it possible to create increasingly comprehensive assessments of evolutionary phenomena, facilitating more revolutionary techniques of their complicated behaviours.
In this part, the Atangana-Baleanu-Caputo (ABC) fractional derivative form of the MPX epidemic mathematical systems is introduced. Let us just continue with a review of the ML kernels' notions and their concerning consequences.
Definition 2.1. ([17]) For ρ∈[0,1], c<d and F1∈H1(c,d), the ABC derivative of fractional-order for F1 is presented as
ABCDρF1(τ)=ABC(ρ)(1−ρ)τ∫cdF1dτEρ(ρρ−1(τ−ϱ)ρ)dϱ, | (2.1) |
where ABC(ρ) is a normalization mapping satisfying ABC(0)=ABC(1)=1 and the ML function signified by Eρ(z1) having the set of complex numbers C is defined as
Eρ(z1)=∞∑γ=0zγ1Γ(ργ+1),ρ,z1∈C,ℜ(ρ)>0. |
Definition 2.2. ([17]) For ρ∈[0,1], c<d and F1∈H1(c,d), the Atangana–Baleanu (AB) fractional integral of F1 is presented as
ABcIρτF1(τ)=(1−ρ)ABC(ρ)F1(τ)+ρΓ(ρ)ABC(ρ)τ∫cF1(ϱ)(τ−ϱ)ρ−1dϱ. | (2.2) |
Lemma 2.1. ([42]) (Newton-Leibniz identity) For F1∈C1(c,d), the ABC fractional derivative and integral for F1 holds:
ABcIρτ(ABCcDρτF1(τ))=F1(τ)−F1(c). | (2.3) |
Lemma 2.2. ([42]) For c<d,F1,F2∈H1(c,d), the ABC fractional derivative holds for the subsequent variant:
‖ABCcDρτF1(τ)−ABCcDρτF2(τ)‖≤H‖F1(τ)−F2(τ)‖. | (2.4) |
Our next result is the generalized mean-value theorem, which is mainly due to [42].
Lemma 2.3. ([42]) Assume there is a function h1(ϱ)∈C[c,d] and also suppose ABC0Dρτh1(ϱ)∈C[c,q2]andρ∈(0,1]. Then h1(ϱ)=h1(c)+1Γ(ρ)ABC0Dρτh1(χ)(ϱ−c)ρ,χ∈[0,ϱ].
Followed by Lemma 2.3, for ρ∈(0,1], if h1(ϱ)∈[0,d],ABC0Dρτh1(ϱ)∈(0,d] and ABC0Dρτh1(ϱ)≥0 for all ϱ∈(0,d], then the mapping h1(ϱ) is increasing. Otherwise, h1(ϱ) is said to be decreasing for all ϱ∈[0,d].
We will now proceed to design the MPX model. The configuration diagram below (Figure 3) was utilized to construct the numerical structure for this analysis.
The explanatory features of the system are examined in this section. To streamline the approach, we divided the coherent model into several Differential equations (DEs), as shown in (2.5), that are estimations for (MPX).
The underlying formulations, which are established on the basis of the process, describe the numerical technique involving the mathematical model implemented in this research.
{˙Sℏ(τ)=χℏ−(η1Ir+η2Iℏ)SℏNℏ−υℏSℏ+σQℏ,˙Eℏ(τ)=(η1Ir+η2Iℏ)SℏNℏ−(δ1+δ2+υℏ)Eℏ,˙Iℏ(τ)=δ1Eℏ−(υℏ+φℏ+γ)Iℏ,˙Qℏ(τ)=δ2Eℏ−(σ+υℏ+φℏ+ψ)Qℏ,˙Rℏ(τ)=γIℏ+ψQℏ−υℏRℏ,˙Sr(τ)=χr−η3SrIrNr−υrSr,˙Er(τ)=η3SrIrNr−(υr+δ3)Er,˙Ir(τ)=δ3Er−(υr+φr)Ir. | (2.5) |
Here, we present a deterministic compartmental framework of MPX propagation and prevention, including two communities: individuals and rodents. Susceptible individuals Sℏ(t1), vulnerable individuals Eℏ(t1), infectious individuals Iℏ(t1), segregated individuals Qℏ(t1) and human restoration Rℏ(t1) are the five categories of the global community. Susceptible rodents Sr(t1), revealed rodents Er(t1) and infectious rodents Ir(t1) are the three categories of the rodent community. The proportion of enlistment into the global community is χℏ. η1 is the component of the efficacious interaction yield and the possibility of a sentient contracting the pathogen after coming into contact with a contaminated rodent, and η2 is the outcome of the impact correspondence yield and the plausibility of a sentient contracting the pathogen after coming into contact with a highly contagious person. The rate of reported cases who becoming extremely contaminated is δ2, while the population of individuals of becoming diseased is δ1. Several suspicious instances are validated after diagnostic testing, while the rest are not identified and are transferred to susceptibility populations at a pace σ. At an incidence of ψ, suspicious infections are diagnosed and shifted to the restored group. Adult recuperation capacity is increasing at a rate of γ. Spontaneous mortality happens at speeds of υℏ and υr in the adult and rodent populations, respectively. The appropriate connection incidence is based on η3, which is the possibility of a rodent being contaminated per encounter with an infectious rodent. Both the spontaneous fatality rate υr and the illness fatality rate φr reduced the diseased rodent community. The crossover between the several cohorts addressed in the system is depicted in Figure 3, and the system is controlled by the nonlinear differential equations listed below.
First we find the invariant region, which confirms that the solution is bounded. Therefore, we assume the overall human population is Nℏ=Sℏ+Eℏ+Iℏ+Qℏ+Rℏ and the rodent population is Nr=Sr+Er+Ir.
The fractional version of the biologically viable domain Φ=Φℏ×Φr for the MPX virus system (2.5), i.e., Φℏ⊂R5+ and Φr⊂R3+ is such that
Φℏ:={(Sℏ,Eℏ,Iℏ,Qℏ,rℏ)∈R5+:Nℏ≤χℏυℏ} |
and
Φr:={(Sr,Er,Ir)∈R3+:Nℏ≤χrυr}. |
Lemma 3.1. If there is an MPX virus system (2.5) having initial conditions (ICs) in Domain Φ is positively invariant.
Proof. Now, to illustrate the boundedness of the solutions of the MPX virus system (2.5), we proceed by accumulating all of the model's formulas, which yields
ABC0Dρt1Nℏ(t1)=χℏ−υℏSℏ−υℏEℏ−(υℏ+φℏ)Iℏ−(υℏ+φℏ)Qℏ−υℏRℏ=χℏ−υℏNℏ−φℏ(Iℏ+Qℏ)≤χℏ−υℏNℏ. |
Implementing the Laplace transform, we find that
L(ABC0Dρt1Nℏ(t1)+υℏNℏ(t1))≤L(χℏ). |
It follows that
L(Nℏ)≤(1−ℓ1ρ(1−ℓ1)(1−ρ)s−ρ1)−1{1−ρ(1−ℓ1)ABC(ρ)(1+ρ1−ρs−ρ1)χℏs1+Nℏ(0)1s1(1−ℓ1)}, |
where ℓ1=−υℏ(1−ρ)ABC(ρ).
By implementing the approach described in [35] and using the solution produced by employing the inverse Laplace transform, we have
Nℏ(t1)=χℏυℏ−χℏυℏ(1−ℓ1)ddt1t1∫0Eρ(ℓ1ρ(1−ℓ1)(1−ρ)(t1−x1)ρ)dx1+11−ℓ1Eρ(ℓ1ρ(1−ℓ1)(1−ρ)(t1)ρ)Nℏ(0). |
Repeating the analogous process for the rodent population, we have
Nr(t1)=χℏυr−χrυr(1−ℓ2)ddt1t1∫0Eρ(ℓ2ρ(1−ℓ2)(1−ρ)(t1−x1)ρ)dx1+11−ℓ2Eρ(ℓ2ρ(1−ℓ2)(1−ρ)(t1)ρ)Nr(0), |
where ℓ2=−υr(1−ρ)ABC(ρ).
Here, in both cases the ML function is denoted by Eν1,ν2. Based on the assumption that the ML function possesses asymptotic tendency, we have
Eν1,ν2≈w∑k=1z−k1/Γ(ν2−νk)+O(1/|z1|1+w),|z1|↦∞,ν1π/2<|Arg(z1)|≤π. |
Consequently, Nℏ(t1) and Nr(t1) converges for t1↦∞. Hence, the domain Φ is positively invariant.
Now, we demonstrated the accompanying result by applying a (Lemma 2.3 from [42]) and the fractional comparative criterion [48].
Assume that there is a solution (Sℏ,Eℏ,Iℏ,Qℏ,Rℏ,Sr,Er,Ir) involved in the ICs of R5+×R3+. Then, the R5+×R3+ domain is a positively invariant set of the system (2.5).
Based on the scheme described in [49], we intended to define the existence-uniqueness of the MPX virus dynamics (2.5); thus, we have
{ABCDρt1Sℏ(τ)|Sℏ=0=χℏ≥0,ABCDρt1Eℏ(τ)|Eℏ=0=0,ABCDρt1Iℏ(τ)|Iℏ=0=δ1Eℏ≥0,ABCDρt1Qℏ(τ)|Qℏ=0=δ2Eℏ≥0,ABCDρt1Rℏ(τ)|Rℏ=0=γIℏ+ψQℏ≥0,ABCDρt1Sr(τ)|Sr=0=χr≥0,ABCDρt1Er(τ)|Er=0=0,ABCDρt1Ir(τ)|Ir=0=δ3Er≥0. | (3.1) |
Observe that (3.1) shows that every solution of (2.5) is nonnegative and remains in Φ; we have that
0≤Nℏ(t1)≤Nℏ(0)exp(−υℏt1)+χℏυℏ(1−exp(−υℏt1)) | (3.2) |
and
0≤Nr(t1)≤Nr(0)exp(−(υr+χr)t1)+χℏυℏ(1−exp(−(υr+χr)t1)). | (3.3) |
This gives the desired estimates.
The disease-free phases are cohorts Sℏ,RℏandSr, in our developed framework (2.5), while the infectious category includes compartments Eℏ,Iℏ,Qℏ,ErandIr1.
As a result, the MPX-free equilibrium condition can be determined as E0=(χℏυℏ,0,0,0,0,χrυr,0,0).
One of the major considerations for analyzing an epidemic's protracted dynamics is the basic reproduction number. It is the number of additional occurrences created by a single infectious person over the course of their pathogenic agent's lifetime. To obtain the formulation of the reproducing number R0, we used the next-generation matrix procedure described in [50]. It was initially mentioned in [51], which goes into great length about how to estimate R0 using this approach. There are also several publications on this research wherein the authors describe a next-generation matrix approach to determine the basic reproduction number representation.
The matrix F corresponds to transmissions and the matrix V to transitions. In this paper, we include death in the transition matrix to keep the notation simple (in contrast with Diekmann et al. [50]). Hence, all epidemiological events that lead to new infections are incorporated into the model via F, and all other events via V. Progress to either death or immunity guarantees that V is invertible. Thus, the MPX can be expressed as
F=[0η1Ir+η2IℏNℏSℏ0000000]andV=[−χℏ+(η1Ir+η2Iℏ)SℏNℏ+υℏSℏ−σQℏ(δ1+δ2+υℏ)Eℏ−δ1Eℏ+(υℏ+φℏ+γ)Iℏ−δ2Eℏ+(σ+υℏ+φℏ+ψ)Qℏ−γIℏ−ψQℏ+υℏRℏ−χr−η3SrIrNr+υrSr−η3SrIrNr+(υr+δ3)Er−δ3Er+(υr+φr)Ir]. |
The progression of contaminated individuals from Eℏ to Eℏ or Qℏ is not considered as the emergence of a new virus, but rather the evolution of contaminated individuals across multiple cohorts. So, we have a linearized system at a disease-free state:
F=[0η20η1000000000000]andV=[(δ1+δ2+υℏ)000−δ1υℏ+φℏ+γ00−δ20σ+ψ+φℏ+υℏ0000υr+φr], |
where F and V are 4×4 matrices, computed as F=∂Fȷ∂xȷ and V=∂Vȷ∂xȷ. For the sake of convenience, assume b1=δ1+δ2+υℏ,b2=υℏ+φℏ+γ,b3=σ+ψ+φℏ+υℏ and b4=υr+φr.
Furthermore, the next-generation matrix is presented as:
FV−1=1b1b2b3b4[η2δ1b3b400η1b1b2b3000000000000]. |
Thus, the reproductive number for System (2.5) can be calculated as
R0=Ψ(FV−1)=η2δ1b3b4b1b2b3b4, |
or equivalently,
R0=η2δ1(δ1+δ2+υℏ)(υℏ+φℏ+γ). |
Theorem 3.1. Suppose there is two non-negative integers ϑ1,ϑ2 with gcd(ϑ1,ϑ2)=1,σ=ϑ1/ϑ2 and K=ϑ1; then, the model (3.1) is locally asymptotically stable if |Arg(λ)|>π2K for all roots of the concerning equation det(diag(λKσ)−J(E0))=0.
Proof. The Jacobian matrix of system (3.1) at E0 implies,
(J0)E0=[−υℏ0−η20000−η10−B1η20000η10δ1−B2000000δ20−B3000000γψ−υℏ00000000−υr00000000−B4η3000000η3−B5] | (3.4) |
Suppose the concerned eigenvalues are ϖ=(ϖ1,ϖ2,ϖ3,ϖ4,ϖ5,ϖ6,ϖ7,ϖ8). This can be achieved by simple computation:
−υℏϖ1−η2ϖ3−η1η8=0,−B1ϖ2+η2ϖ3+η1ϖ8=0,δ1ϖ2−B2ϖ3=0,δ2ϖ2−B3ϖ4=0,γϖ3+ψϖ4−υℏϖ5=0,υrϖ6−η3ϖ8=0,−B4ϖ7+η3ϖ8=0,δ3ϖ7−B5ϖ8=0. | (3.5) |
Clearly, ϖȷ,ȷ=1,2,...,8 are positive if R0<1. Moreover, the argument is
arg(ϖȷ)=πϑ1+ȷ2πϑ1>πK>π2K,ȷ=0,1,2,...(ϑ1−1). |
The arguments of the other roots can be acquired in a similar way and are all greater than π2K if R0<1. So, the DFE is locally asymptotically stable for R0<1.
Further, we adopted the methodology proposed by Castillo-Chavez and Song [52] to determine the requirements for global stability (GS) for E0, which stipulates that the model scheme be stated in the appropriate pattern:
˙X1=F1(X1,Z1),˙Z1=G1(X1,Z1),G1(X1,0)=0. | (3.6) |
Now, X1∈Rn represents the unexposed persons and Z1∈Rm states the infectious people. Using this terminology, the DFE is calculated by H0=(X10,0). The GS of the DFE is now guaranteed by the underlying two requirements:
a)For˙X1=F1(X1,0),X10 is asymptotically GS.
b)G1=(X1,Z1)=A1Z1−~G1(X1,Z1), where ~G1(X1,Z1)≥0 for X1,Z1∈Υ.
Since A1=DZ1G1(X10,0) is an M-matrix and the viability of the system is presented by Υ. The GS of E0 is then determined by the accompanying lemma.
Lemma 3.2. Suppose there is an equilibrium point H0=(X10,0) that is asymptotically GS when R0<1 admits the assertions (a) and (b).
Proof. Firstly, we intend to verify a) as:
F1(X1,0)=[χℏ−υℏSℏ−υℏRℏχr−υrSr−(υr+δ3)Er]. |
The characteristic polynomial of F1(X1,0) implies that λ1=λ2=−υℏ,λ3=−υrandλ4=−υr−δ3.
Therefore, X1=X10 is asymptotically GS.
Furthermore, we have
G1(X1,Z1)=A1Z1−~G1(X1,Z1)=[−b1η2S0ℏNℏ0η1S0ℏNℏδ1−b200δ20−b30000b4][EℏIℏQℏIr]−[η2(S0ℏ−Sℏ)+η1(S0ℏ−Sℏ)NℏEℏ00δ3Er]. |
As a result, it is clear that A1 satisfies all of the criteria stated in b).
The EEP happens when the illness continues to spread among the community, as indicated by
E∗0=(S∗ℏ,E∗ℏ,I∗ℏ,Q∗ℏ,R∗ℏ,S∗r,E∗r,I∗r). |
Thus, we have
E∗0=(b1b3χℏυℏb1b3−δ2σb6+b1b3b6,b6b3χℏυℏb1b3−δ2σb6+b1b3b6,δ1b3b6χℏb2(υℏb1b3−δ3σb6+b1b3b6),δ2b6χℏυℏb1b3−δ3σb6+b1b3b6,(δ1γb3+δ2b2ψ)b6χℏυℏb2(υℏb1b3−δ3σb6+b1b3b6),χrυr+b7,χrb5(υr+b7),b7δ−3χrb4b5(υr+b7)), | (3.7) |
where b5=υr+δ3,b6=η1I∗r+η2I∗ℏNℏandb7=η3I∗rNr
Here, the Routh–Hurwitz threshold [53] will be employed to demonstrate the endemic equilibrium's (EE's) local stability. The criteria whereby the EE is locally asymptotically stable will be determined by the Jacobian matrix as
J=[ϱ110ϱ13ϱ14000ϱ18ϱ21ϱ22ϱ230000ϱ280ϱ32ϱ33000000ϱ420ϱ44000000ϱ53ϱ54ϱ5500000000ϱ660ϱ6800000ϱ76ϱ77ϱ78000000ϱ87ϱ88], | (3.8) |
where ϱ11=−η1Ir+η2IℏNℏ, ϱ13=−η2SℏNℏ, ϱ14=ϕ, ϱ18=−η1SℏNℏ, ϱ21=−η1Ir+η2IℏNℏ, ϱ22=−b1, ϱ23=η2SℏNℏ, ϱ28=η1IℏNℏ, ϱ32=δ1, ϱ33=−(υℏ+φℏ+γ), ϱ42=δ2, ϱ44=−b2, ϱ53=γ, ϱ54=ψ, ϱ55=−υℏ, ϱ66=−(υr+η3IrNr), ϱ68=−η3SrNr, ϱ76=η3IrNr, ϱ77=−b5, ϱ78=η3SrNr, ϱ87=δ3andϱ88=−b4. The characteristic equation yields
y81+B1y71+B2y61+B3y51+B4y41+B5y31+B6y21+B7y1+B8=0, | (3.9) |
where Bȷ,ȷ=0,1,2,...,8 are the coefficients of yȷ1 after the polynomial has been converted to the simplified form.
We shall use the appropriate adjustment to achieve the EE stability requirements:
P=B1B2−B0B3B1,Q=B1B4−B0B5B1,R=B1B6−B0B7B1,S=B8,P∗=PB3−QB1P,Q∗=PB5−RB1P,R∗=PB7−SB1P,M=P∗Q−PQ∗P∗,N=P∗R−PR∗P∗,T=P∗SP∗,M∗=MQ∗−NP∗M,N∗=MR∗−TP∗M,X∗=NM∗−MN∗M∗ | (3.10) |
Therefore, the Hurwitz assumptions concerning the characteristic equation are
B1>0,B1B2>B3,B1B2B3+B0B1B5>B0B23+B4B21,P∗Q>PQ∗,Q∗M>NP∗,M∗N>MN∗,N∗X>TM∗. | (3.11) |
Hence, the EEP is locally asymptotic stable.
The combination of the DEs depicts the intricate framework (2.5), which includes the assumptions, the saturation contact pattern, and the schematic diagram shown in Figure 3, as well as analyses of the concept (2.5) using the ABC fractional derivative.
{ABC0DρτSℏ(τ)=Ω1(τ,Sℏ),ABC0DρτEℏ(τ)=Ω2(τ,Eℏ),ABC0DρτIℏ(τ)=Ω3(t3,Iℏ),ABC0DρτQℏ(τ)=Ω4(τ,Qℏ),ABC0DρτRℏ(τ)=Ω5(τ,Rℏ),ABC0DρτSr(τ)=Ω6(τ,Sr),ABC0DρτEr(τ)=Ω7(τ,Qℏ),ABC0DρτIr(τ)=Ω8(τ,Qℏ), | (3.12) |
where kernels are configured as shown in:
{Ω1(τ,Sℏ)=χℏ−(η1Ir+η2Iℏ)SℏNℏ−υℏSℏ+σQℏ,Ω2(τ,Eℏ)=(η1Ir+η2Iℏ)SℏNℏ−(δ1+δ2+υℏ)Eℏ,Ω3(τ,Iℏ)=δ1Eℏ−(υℏ+φℏ+γ)Iℏ,Ω4(τ,Qℏ)=δ2Eℏ−(σ+υℏ+φℏ+ψ)Qℏ,Ω5(τ,Rℏ)=γIℏ+ψQℏ−υℏRℏ,Ω6(τ,Sr)=χr−η3SrIrNr−υrSr,Ω7(τ,Er)=η3SrIrNr−(υr+δ3)Er,Ω8(τ,Ir)=δ3Er−(υr+φr)Ir, | (3.13) |
which are subject the following ICs: Sℏ(0)=Sℏ0, Eℏ(0)=Eℏ0, Iℏ(0)=Iℏ0, Qℏ(0)=Qℏ0, Rℏ(0)=Rℏ0, Sr(0)=Sr0, Er(0)=Er0 and Ir(0)=Ir0.
Here, we have dN/dτ=χℏ−φℏIℏ−υℏNℏ in the occurrence of human infectious and rodent dN/dτ=Sr+Er+Ir, illustrating that the size of the communities is not constant. The parameters that were evaluated in the investigation (2.5) are listed in Table 1.
Symbols | Explanations | Values | References | |
χℏ | Proportion of humans acquisition | 0.029 | [45] | |
χr | Proportion of rodents acquisition | 0.2 | [45] | |
η1 | Rodent-human interaction rate | 0.00025 | [46] | |
η2 | Rate of human-to-human interaction | 0.00006 | [46] | |
η3 | Rate of rodent-to-rodent interaction | 0.027 | [46] | |
δ1 | Rate of exposed people to infectious people | 0.2 | Supposed | |
δ2 | Rate of confirmed reported incidents | 2.0 | Estimated | |
σ | Rate of untreated after screening | 2.0 | Estimated | |
ψ | Transition from the separated to the restored group | 0.52 | Supposed | |
γ | Humans' rate of recuperation | 0.83 | [45] | |
υℏ | Natural mortality rate for people | 1.5 | [46] | |
υr | Natural mortality rate for rodents | 0.002 | [46] | |
φℏ | Proportion of rodents dying as a result of disease | 0.5 | Supposed | |
φr | Proportion of humans dying as a result of disease | 0.2 | [47] |
The Banach fixed point ˜fp assumption for contraction mapping is used to demonstrate the existence–uniqueness of the result for the ABC fractional framework stated in (3.12). It is vital to understand the two new theories preceding the progress on [43].
To establish the system's existence-uniqueness, we proceed as follows. While implementing the Atangana-Baleanu fractional integral, we can obtain System (3.12):
{Sℏ(τ)−Sℏ(0)=1−ρABC(ρ)Ω1(τ,Sℏ)+ρABC(ρ)Γ(ρ)∫τ0Ω1(ς,Sℏ)(τ−ς)ρ−1dς,Eℏ(τ)−Eℏ(0)=1−ρABC(ρ)Ω2(τ,Eℏ)+ρABC(ρ)Γ(ρ)∫τ0Ω2(ς,Eℏ)(τ−ς)ρ−1dς,Iℏ(τ)−Iℏ(0)=1−ρABC(ρ)Ω3(τ,Iℏ)+ρABC(ρ)Γ(ρ)∫τ0Ω3(ς,Iℏ)(τ−ς)ρ−1dς,Qℏ(τ)−Qℏ(0)=1−ρABC(ρ)Ω4(τ,Qℏ)+ρABC(ρ)Γ(ρ)∫τ0Ω4(ς,Qℏ)(τ−ς)ρ−1dς,Rℏ(τ)−Rℏ(0)=1−ρABC(ρ)Ω5(τ,Rℏ)+ρABC(ρ)Γ(ρ)∫τ0Ω5(ς,Rℏ)(τ−ς)ρ−1dς,Sr(τ)−Sr(0)=1−ρABC(ρ)Ω6(τ,Rℏ)+ρABC(ρ)Γ(ρ)∫τ0Ω6(ς,Rℏ)(τ−ς)ρ−1dς,Er(τ)−Er(0)=1−ρABC(ρ)Ω7(τ,Er)+ρABC(ρ)Γ(ρ)∫τ0Ω7(ς,Er)(τ−ς)ρ−1dς,Ir(τ)−Ir(0)=1−ρABC(ρ)Ω8(τ,Ir)+ρABC(ρ)Γ(ρ)∫τ0Ω8(ς,Ir)(τ−ς)ρ−1dς. | (3.14) |
Assume that the collection B=Λ(J)×Λ(J)×Λ(J)×Λ(J)×Λ(J)×Λ(J)×Λ(J)×Λ(J), where Λ(J)=C[0,ˉT] refers to real-valued continuous functions for the B on J=[0,ˉT], taking into account the established norm ‖(Sℏ,Eℏ,Iℏ,Qℏ,Rℏ,Sr,Er,Ir)‖=‖Sℏ‖+‖Eℏ‖+‖Iℏ‖+‖Qℏ‖+‖Rℏ‖+‖Sr‖+‖Er‖+‖Ir‖, where ‖Sℏ‖=supτ∈J|Sℏ(τ)|, ‖Eℏ‖=supτ∈J|Eℏ(τ)|, ‖Iℏ‖=supτ∈J|Iℏ(τ)|, ‖Qℏ‖=supτ∈J|Qℏ(τ)|, ‖Rℏ‖=supτ∈J|Rℏ(τ)|, ‖Sr‖=supτ∈J|Sr(τ)|, ‖Er‖=supτ∈J|Er(τ)| and ‖Ir‖=supτ∈J|Ir(τ)|.
The accompanying result is established on the basis of the contraction and the Lipschitz supposition.
Theorem 3.2. For kernels Ωℓ,ℓ=1,2,...,8 in (3.12), there exists Lℓ>0,ℓ=1,2,...8, such that
{‖Ω1(τ,Sℏ)−Ω1(τ,Sℏ1)‖≤L1‖Sℏ(τ)−Sℏ1(τ)‖,‖Ω2(τ,Eℏ)−Ω2(τ,Eℏ1)‖≤L2‖Eℏ(τ)−Eℏ1(τ)‖,‖Ω3(τ,Iℏ)−Ω3(τ,Iℏ1)‖≤L3‖Iℏ(τ)−Iℏ1(τ)‖,‖Ω4(τ,Qℏ)−Ω4(τ,Qℏ1)‖≤L4‖Qℏ(τ)−Qℏ1(τ)‖,‖Ω5(τ,Rℏ)−Ω5(τ,Rℏ1)‖≤L5‖Rℏ(τ)−Rℏ1(τ)‖,‖Ω6(τ,Sr)−Ω6(τ,Sm11)‖≤L6‖Sr(τ)−Sm11(τ)‖,‖Ω7(τ,Er)−Ω7(τ,Er1)‖≤L7‖Er(τ)−Er1(τ)‖,‖Ω8(τ,Ir)−Ω8(τ,Ir1)‖≤L8‖Ir(τ)−Ir1(τ)‖, | (3.15) |
which are contractions for Lℓ∈[0,1),ℓ=1,2,...,8.
Proof. To achieve Lipschitz's requirements, we have
‖Ω1(τ,Sℏ)−Ω1(τ,Sℏ1)‖=‖χℏ−(η1Ir+η2Iℏ)SℏNℏ−υℏSℏ+σQℏ−(χℏ−(η1Ir+η2Iℏ)Sℏ1Nℏ−υℏSℏ1+σQℏ)‖=‖−((η1Ir+η2Iℏ)Nℏ+υℏ)(Sℏ−Sℏ1)‖≤((η1Ir+η2Iℏ)Nℏ+υℏ)‖Sℏ−Sℏ1‖≤L1‖Sℏ−Sℏ1‖, | (3.16) |
where L1=η1(K8+η2K3)N, ‖Sℏ‖=supτ∈J|Sℏ(τ)|=K1, ‖Eℏ‖=supτ∈J|Eℏ(τ)|=K2, ‖Iℏ‖=supτ∈J|Iℏ(τ)|=K3, ‖Qℏ‖=supτ∈J|Qℏ(τ)|=K4, ‖Rℏ‖=supτ∈J|Rℏ(τ)|=K5, ‖Sr‖=supτ∈J|Sr(τ)|=K6, and ‖Er‖=supτ∈J|Er(τ)|=K7, and ‖Ir‖=supτ∈J|Ir(τ)|=K8.
It is significant to mention that Ω1(τ,Sℏ1) admits the Lipschitz requirement involving the Lipschitz constant L1=η1(K8+η2K3)N. Also, if L1∈[0,1), then Ω1(τ,Sℏ1) is verified to be a contraction.
Accordingly, we can investigate the significance of the existence of Lℓ,ℓ=2,3,...,8 and the contraction condition for Ω2(τ,Eℏ),Ω3(τ,Iℏ),Ω4(τ,Qℏ),Ω5(τ,Rℏ),Ω6(τ,Sr) and Ω7(τ,Er) for Lℓ∈[0,1),ℓ=2,3,...,8.
At τ=τm,m=1,2,..., presenting the recurrent form that follows from (3.14) gives
{Sℏm(τ)=1−ρABC(ρ)Ω1(τ,Sℏm−1)+ρABC(ρ)Γ(ρ)∫τ0Ω1(ς,Sℏm−1)(τ−ς)ρ−1dς,Eℏm(τ)=1−ρABC(ρ)Ω2(τ,Eℏm−1)+ρABC(ρ)Γ(ρ)∫τ0Ω2(ς,Eℏm−1)(τ−ς)ρ−1dς,Iℏm(τ)=1−ρABC(ρ)Ω3(τ,Iℏm−1)+ρABC(ρ)Γ(ρ)∫τ0Ω3(ς,ℏmm−1)(τ−ς)ρ−1dς,Qℏm(τ)=1−ρABC(ρ)Ω4(τ,Qℏm−1)+ρABC(ρ)Γ(ρ)∫τ0Ω4(ς,Qℏm−1)(τ−ς)ρ−1dς,Rℏm(τ)=1−ρABC(ρ)Ω5(τ,Rℏm−1)+ρABC(ρ)Γ(ρ)∫τ0Ω5(ς,Rℏm−1)(τ−ς)ρ−1dς,Srm(τ)=1−ρABC(ρ)Ω6(τ,Rℏm−1)+ρABC(ρ)Γ(ρ)∫τ0Ω6(ς,Srm−1)(τ−ς)ρ−1dς,Erm(τ)=1−ρABC(ρ)Ω7(τ,Erm−1)+ρABC(ρ)Γ(ρ)∫τ0Ω7(ς,Erm−1)(τ−ς)ρ−1dς,Irm(τ)=1−ρABC(ρ)Ω8(τ,Irm−1)+ρABC(ρ)Γ(ρ)∫τ0Ω8(ς,Irm−1)(τ−ς)ρ−1dς | (3.17) |
in the presence of the ICs Sℏ(0)=Sℏ0, Eℏ(0)=Eℏ0 Iℏ(0)=Iℏ0 Qℏ(0)=Qℏ0 Rℏ(0)=Rℏ0 Sr(0)=Sr0, Er(0)=Er0 and Ir(0)=Ir0.
In (3.17), the differences of successive terms are expressed in the following terms:
Ψ1m(τ)=Sℏm(τ)−Sℏm−1(τ)=1−ρABC(ρ)(Ω1(τ,Sℏm−1)−Ω1(τ,Sℏm−2))+ρΓ(ρ)ABC(ρ)∫τ0(Ω1(ς,Sℏm−1)−Ω1(ς,Sℏm−2))(τ−ς)ρ−1dς,Ψ2m(τ)=Eℏm(τ)−Eℏm−1(τ)=1−ρABC(ρ)(Ω2(τ,Eℏm−1)−Ω2(τ,Eℏm−2))+ρΓ(ρ)ABC(ρ)∫τ0(Ω2(ς,Eℏm−1)−Ω2(ς,Eℏm−2))(τ−ς)ρ−1dς,Ψ3m(τ)=Iℏm(τ)−Iℏm−1(τ)=1−ρABC(ρ)(Ω3(τ,Iℏm−1)−Ω3(τ,Iℏm−2))+ρΓ(ρ)ABC(ρ)∫τ0(Ω3(ς,Iℏm−1)−Ω3(ς,Iℏm−2))(τ−ς)ρ−1dς,Ψ4m(τ)=Qℏm(τ)−Qℏm−1(τ)=1−ρABC(ρ)(Ω4(τ,Qℏm−1)−Ω4(τ,Qℏm−2))+ρΓ(ρ)ABC(ρ)∫τ0(Ω4(ς,Qℏm−1)−Ω4(ς,Qℏm−2))(τ−ς)ρ−1dς,Ψ5m(τ)=Rℏm(τ)−Rℏm−1(τ)=1−ρABC(ρ)(Ω5(τ,Rℏm−1)−Ω5(τ,Rℏm−2))+ρΓ(ρ)ABC(ρ)∫τ0(Ω5(ς,Rℏm−1)−Ω5(ς,Rℏm−2))(τ−ς)ρ−1dς,Ψ6m(τ)=Srm(τ)−Srm−1(τ)=1−ρABC(ρ)(Ω2(τ,Srm−1)−Ω6(τ,Srm−2))+ρΓ(ρ)ABC(ρ)∫τ0(Ω6(ς,Srm−1)−Ω6(ς,Srm−2))(τ−ς)ρ−1dς,Ψ7m(τ)=Erm(τ)−Erm−1(τ)=1−ρABC(ρ)(Ω7(τ,Erm−1)−Ω7(τ,Erm−2))+ρΓ(ρ)ABC(ρ)∫τ0(Ω7(ς,Srm−1)−Ω7(ς,Erm−2))(τ−ς)ρ−1dς,Ψ8m(τ)=Irm(τ)−Irm−1(τ)=1−ρABC(ρ)(Ω8(τ,Irm−1)−Ω8(τ,Irm−2))+ρΓ(ρ)ABC(ρ)∫τ0(Ω8(ς,Irm−1)−Ω8(ς,Irm−2))(τ−ς)ρ−1dς. | (3.18) |
Applying the norm to the specified framework (3.18), we have
‖Ψ1m(τ)‖=‖Sℏm(τ)−Sℏm−1(τ)‖=1−ρABC(ρ)‖Ω1(τ,Sℏm−1)−Ω1(τ,Sℏm−2)‖+ρΓ(ρ)ABC(ρ)∫τ0‖Ω1(ς,Sℏm−1)−Ω1(ς,Sℏm−2)‖(τ−ς)ρ−1dς,‖Ψ2m(τ)‖=‖Eℏm(τ)−Eℏm−1(τ)‖=1−ρABC(ρ)‖Ω2(τ,Eℏm−1)−Ω2(τ,Eℏm−2)‖+ρΓ(ρ)ABC(ρ)∫τ0‖Ω2(ς,Eℏm−1)−Ω2(ς,Eℏm−2)‖(τ−ς)ρ−1dς,‖Ψ3m(τ)‖=‖Iℏm(τ)−Iℏm−1(τ)‖=1−ρABC(ρ)‖Ω3(τ,Iℏm−1)−Ω3(τ,Iℏm−2)‖+ρΓ(ρ)ABC(ρ)∫τ0‖Ω3(ς,Iℏm−1)−Ω3(ς,Iℏm−2)‖(τ−ς)ρ−1dς,‖Ψ4m(τ)‖=‖Qℏm(τ)−Qℏm−1(τ)‖=1−ρABC(ρ)‖Ω4(τ,Qℏm−1)−Ω4(τ,Qℏm−2)‖+ρΓ(ρ)ABC(ρ)∫τ0‖Ω4(ς,Qℏm−1)−Ω4(ς,Qℏm−2)‖(τ−ς)ρ−1dς,‖Ψ5m(τ)‖=‖Rℏm(τ)−Rℏm−1(τ)‖=1−ρABC(ρ)‖Ω5(τ,Rℏm−1)−Ω5(τ,Rℏm−2)‖+ρΓ(ρ)ABC(ρ)∫τ0‖Ω5(ς,Rℏm−1)−Ω5(ς,Rℏm−2)‖(τ−ς)ρ−1dς,‖Ψ6m(τ)‖=‖Srm(τ)−Srm−1(τ)‖=1−ρABC(ρ)‖Ω6(τ,Srm−1)−Ω6(τ,Srm−2)‖+ρΓ(ρ)ABC(ρ)∫τ0‖Ω6(ς,Srm−1)−Ω6(ς,Srm−2)‖(τ−ς)ρ−1dς,‖Ψ7m(τ)‖=‖Erm(τ)−Erm−1(τ)‖=1−ρABC(ρ)‖Ω7(τ,Erm−1)−Ω7(τ,Erm−2)‖+ρΓ(ρ)ABC(ρ)∫τ0‖Ω7(ς,Erm−1)−Ω7(ς,Erm−2)‖(τ−ς)ρ−1dς,‖Ψ8m(τ)‖=‖Irm(τ)−Irm−1(τ)‖=1−ρABC(ρ)‖Ω8(τ,Irm−1)−Ω8(τ,Irm−2)‖+ρΓ(ρ)ABC(ρ)∫τ0‖Ω8(ς,Irm−1)−Ω8(ς,Irm−2)‖(τ−ς)ρ−1dς. | (3.19) |
Furthermore, the first equation in (3.19) can be converted to the following characterizations:
‖Ψ1m(τ)‖=‖Sℏm(τ)−Sℏm−1(τ)‖=1−ρABC(ρ)‖Ω1(τ,Sℏm−1)−Ω1(τ,Sℏm−2)‖+ρΓ(ρ)ABC(ρ)∫τ0‖Ω1(ς,Sℏm−1)−Ω1(ς,Sℏm−2)‖(τ−ς)ρ−1dς≤L11−ρABC(ρ)‖Sℏm−1−Sℏm−2‖+ρL1Γ(ρ)ABC(ρ)∫τ0‖Sℏm−1−Sℏm−2‖(τ−ς)ρ−1dς≤L1‖Ψ1(m−1)(τ)‖|1−ρABC(ρ)+τρABC(ρ)|. |
Ultimately, we have
‖Ψ1m(τ)‖≤L1‖Ψ1(m−1)(τ)‖|1−ρABC(ρ)+τρABC(ρ)|. | (3.20) |
By a similar argument, the following terms of (3.19) can be computed as
‖Ψ2m(τ)‖≤L2‖Ψ2(m−1)(τ)‖|1−ρABC(ρ)+τρABC(ρ)|,‖Ψ3m(τ)‖≤L3‖Ψ3(m−1)(τ)‖|1−ρABC(ρ)+τρABC(ρ)|,‖Ψ4m(τ)‖≤L4‖Ψ4(m−1)(τ)‖|1−ρABC(ρ)+τρABC(ρ)|,‖Ψ5m(τ)‖≤L5‖Ψ5(m−1)(τ)‖|1−ρABC(ρ)+τρABC(ρ)|,‖Ψ6m(τ)‖≤L6‖Ψ6(m−1)(τ)‖|1−ρABC(ρ)+τρABC(ρ)|,‖Ψ7m(τ)‖≤L7‖Ψ7(m−1)(τ)‖|1−ρABC(ρ)+τρABC(ρ)|,‖Ψ8m(τ)‖≤L8‖Ψ8(m−1)(τ)‖|1−ρABC(ρ)+τρABC(ρ)|. | (3.21) |
Theorem 3.3. There is a fractional MPX model defined in (3.12) that has a solution if U0 admits the variant
(1−ρABC(ρ)+Uρ0ABC(ρ)Γ(ρ))Lℓ<1,ℓ=1,2,...,8. | (3.22) |
Proof. Utilizing the hypothesis stated in (3.20) and (3.23), one obtains
‖Ψ1m(τ)‖≤‖Sℏ(0)‖{L1(1−ρABC(ρ)+τρABC(ρ))}m,‖Ψ2m(τ)‖≤‖Eℏ(0)‖{L2(1−ρABC(ρ)+τρABC(ρ))}m,‖Ψ3m(τ)‖≤‖Iℏ(0)‖{L3(1−ρABC(ρ)+τρABC(ρ))}m,‖Ψ4m(τ)‖≤‖Qℏ(0)‖{L4(1−ρABC(ρ)+τρABC(ρ))}m,‖Ψ5m(τ)‖≤‖Rℏ(0)‖{L5(1−ρABC(ρ)+τρABC(ρ))}m,‖Ψ6m(τ)‖≤‖Sr(0)‖{L6(1−ρABC(ρ)+τρABC(ρ))}m,‖Ψ7m(τ)‖≤‖Er(0)‖{L7(1−ρABC(ρ)+τρABC(ρ))}m,‖Ψ8m(τ)‖≤‖Ir(0)‖{L8(1−ρABC(ρ)+τρABC(ρ))}m. | (3.23) |
Theorem 3.2 verifies the validity of the solution (the existence of a ˜fp) and and which shows that the mappings Sℏ(τ), Eℏ(τ), Iℏ(τ), Qℏ(τ), Rℏ(τ), Sr(τ) and Er(τ), are solution of the model (3.12).
Let us commence by identifying which criteria are fulfilled:
{Sℏ(τ)−Sℏ(0)=Sℏm−~B1m(τ),Eℏ(τ)−Eℏ(0)=Eℏm−~B2m(τ),Iℏ(τ)−Iℏ(0)=Iℏm−~B3m(τ),Qℏ(τ)−Qℏ(0)=Qℏm−~B4m(τ),Rℏ(τ)−Rℏ(0)=Rℏm−~B5m(τ),Sr(τ)−Sr(0)=Srm−~B6m(τ),Er(τ)−Er(0)=Erm−~B7m(τ),Ir(τ)−Ir(0)=Irm−~B8m(τ). |
By making the use of (3.24), we have
‖B1m(τ)‖≤1−ρABC(ρ)‖Ω1(τ,Sℏm)−Ω1(τ,Sℏm−1)‖+ρΓ(ρ)ABC(ρ)∫τ0‖Ω1(ς,Sℏm)−Ω1(ς,Sℏm−1)‖(τ−ς)ρ−1dς≤L11−ρABC(ρ)‖Sℏm−Sℏm−1‖+ρmL1Γ(ρ)ABC(ρ)‖Sℏm−Sℏm−1‖. |
Recursively conducting the procedure yields
‖B1m(τ)‖≤Lm1{1−ρABC(ρ)+Lm1τρΓ(ρ)ABC(ρ)}m+1‖Sℏm−Sℏm−1‖m. |
Then, τ=Uρ0 generates
‖B1m(τ)‖≤Lm1{1−ρABC(ρ)+τρΓ(ρ)ABC(ρ)}m+1‖Sℏm−Sℏm−1‖m. | (3.24) |
This is because
‖B1m(τ)‖↦0. |
Let us apply the following limit to (3.24) as m↦∞. Obviously, we have that ‖B1m(τ)‖↦0 for (1−ρABC(ρ)+τρΓ(ρ)ABC(ρ))L1<1.
In an analogous manner, we can obtain ‖Bℓm(τ)‖↦0,forℓ=2,3,...,7; then,
(1−ρABC(ρ)+τρΓ(ρ)ABC(ρ))Lℓ<1,ℓ=1,2,...,8.
This gives the immediate consequence.
Furthermore, the Banach ˜fp assumptions assure the existence of the system solution for (3.12) by Theorem 3.2 and Theorem 3.3. Theorem 3.4 confirms the system's uniqueness.
Theorem 3.4. A fractional MPX system (3.12) has unique solution if
(1−ρABC(ρ)+τρΓ(ρ)ABC(ρ))Lℓ<1,ℓ=1,2,...,8. |
Proof. Suppose that Sℏ1,Eℏ1,Iℏ1,Qℏ1,Rℏ1,Sr1,Er1 and Ir are another solution to the fractional MPX system (3.12). Then
S(τ)−S1(τ)=1−ρABC(ρ)(Ω1(τ,Sℏ)−Ω1(τ,Sℏ1))+ρABC(ρ)Γ(ρ)∫τ0(Ω1(ς,Sℏ)−Ω1(ς,Sℏ1))(τ−ς)ρ−1dς. |
Considering the norm to the above identity, gives
‖S(τ)−S1(τ)‖≤1−ρABC(ρ)‖Sℏ−Sℏ1‖L1+τρABC(ρ)Γ(ρ)‖Sℏ−Sℏ1‖. |
Since (1−(1−ρABC(ρ)+τρΓ(ρ)ABC(ρ))L1)>0, we acquire ‖Sℏ−Sℏ1‖=0. Finally, we have Sℏ=Sℏ1. by the same argument, we can verify that Eℏ=Ip1,Iℏ=Ir,Qℏ=Ipr,Rℏ=Rp1,Sr=Rr and Er=Rpr. This yields the immediate consequence.
In this part, we implement the Toufik–Atangana [44] method to produce a comprehensive formulation for the framework (3.12).
When we investigate the first factor of (3.12), we obtain
{ABC0DρτSℏ(τ)=Θ1(τ,Sℏ(τ)),Sℏ(0)=Sℏ0. | (3.25) |
Analyzing (3.16), ones can estimate for (3.25) in the formulation stated in (3.26):
Sℏ(τ)=Sℏ(0)+1−ρABC(ρ)Θ1(τ,Sℏ(τ))+ρABC(ρ)Γ(ρ)∫τ0Θ1(ς,Sℏ(ς))(τ−ς)ρ−1dς. | (3.26) |
Considering Lagrange's interpolating polynomial approach on [τq,τq+1], gives
Sℏq≈1h1[(w−τq−1)Θ1(τq,Sℏ(τq),Eℏ(τq),Iℏ(τq),Qℏ(τq),Rℏ(τq),Sr(τq),Er(τq),Ir(τq))−(w−τq)Θ1(τq−1,Sℏ(τq−1),Eℏ(τq−1),Iℏ(τq−1),Qℏ(τq−1),Rℏ(τq−1),Sr(τq−1),Er(τq−1),Er(τq−1))], | (3.27) |
where h1=τq−τq−1.
Substituting (3.27) into (3.26), one can obtain
Sℏ(τm+1)=Sℏ(0)+1−ρABC(ρ)Θ1(τq,Sℏ(τq),Eℏ(τq),Iℏ(τq),Qℏ(τq),Rℏ(τq),Sr(τq),Er(τq),Ir(τq))+ρABC(ρ)Γ(ρ)m∑ℓ=1{Θ1(τℓ,Sℏ(τℓ),Eℏ(τℓ),Iℏ(τℓ),Qℏ(τℓ),Rℏ(τℓ),Sr(τℓ),Er(τℓ),Ir(τℓ))ℏ×∫τℓ+1τℓ(w−τℓ−1)(τm+1−w)ρ−1dw−Θ1(τℓ−1,Sℏ(τℓ−1),Eℏ(τℓ−1),Iℏ(τℓ−1),Qℏ(τℓ−1),Rℏ(τℓ−1),Sr(τℓ−1),Er(τℓ−1),Ir(τℓ−1))ℏ×∫τℓ+1τℓ(w−τℓ−1)(τm+1−w)ρ−1dw=Sℏ(0)+1−ρABC(ρ)Θ1(τm,Sℏ(τm),Eℏ(τm),Iℏ(τm),Qℏ(τm),Rℏ(τm),Sr(τm),Er(τm),Ir(τm))+ρABC(ρ)Γ(ρ)m∑ℓ=1({Θ1(τℓ,Sℏ(τℓ),Eℏ(τℓ),Iℏ(τℓ),Qℏ(τℓ),Rℏ(τℓ),Sr(τℓ),Er(τℓ),Ir(τℓ))h1ℑℓ−1−Θ1(τℓ−1,Sℏ(τℓ−1),Eℏ(τℓ−1),Iℏ(τℓ−1),Qℏ(τℓ−1),Rℏ(τℓ−1),Sr(τℓ−1),Er(τℓ−1),Ir(τℓ−1))h1ℑℓ), |
where
ℑℓ−1=∫τℓ+1τℓ(w−τℓ−1)(τm+1−w)ρ−1dw=−1ρ{(τℓ+1−τℓ−1)(τm+1−τℓ+1)ρ−(τℓ−τℓ−1)(τm+1−τℓ)ρ}−1ρ(ρ+1){(τm+1−τℓ+1)ρ+1(τm+1−τℓ+1)ρ−(τm+1−τℓ)ρ+1}, | (3.28) |
and
ℑℓ=∫τℓ+1τℓ(w−τℓ−1)(τm+1−w)ρ−1dw=−1ρ{(τℓ+1−τℓ−1)(τm+1−τℓ+1)ρ}−1ρ(ρ+1){(τm+1−τℓ+1)ρ+1−(τm+1−τℓ)ρ+1}. | (3.29) |
Moreover, employing τℓ=ℓℏ in (3.28) and (3.29) describes
ℑℓ−1=ℏρ+1ρ(ρ+1){(m+1−ℓ)ρ(m−ℓ+2+ρ)−(m−ℓ)ρ(m−ℓ+2+2ρ)} | (3.30) |
and
ℑℓ=ℏρ+1ρ(ρ+1){(m+1−ℓ)ρ+1−(m−ℓ)ρ(m−ℓ+1+ρ)}. | (3.31) |
Consequently, we can express (3.32) in the expression of (3.30) and (3.31) as follows:
Sℏ(τm+1)=Sℏ(τ0)+1−ρABC(ρ)Θ1(τm,Sℏ(τm),Eℏ(τm),Iℏ(τm),Qℏ(τm),Rℏ(τm),Sr(τm),Er(τm),Ir(τm))+ρABC(ρ)Γ(ρ)m∑ℓ=1({Θ1(τℓ,Sℏ(τℓ),Eℏ(τℓ),Iℏ(τℓ),Qℏ(τℓ),Rℏ(τℓ),Sr(τℓ),Er(τℓ),Ir(τℓ))Γ(ρ+2)×hρ1{(m+1−ℓ)ρ(m−ℓ+2+ρ)−(m−ℓ)ρ(m−ℓ+2+2ρ)}−Θ1(τℓ−1,Sℏ(τℓ−1),Eℏ(τℓ−1),Iℏ(τℓ−1),Qℏ(τℓ−1),Rℏ(τℓ−1),Sr(τℓ−1),Er(τℓ−1),Ir(τℓ−1))h1×hρ1{(m+1−ℓ)ρ(m−ℓ+2+ρ)−(m−ℓ)ρ(m−ℓ+2+2ρ)}). |
Thus, the following are the descriptions for the rest of the model cohorts:
Eℏ(τm+1)=Eℏ(τ0)+1−ρABC(ρ)Θ2(τm,Sℏ(τm),Eℏ(τm),Iℏ(τm),Qℏ(τm),Rℏ(τm),Sr(τm),Er(τm),Ir(τm))+ρABC(ρ)Γ(ρ)m∑ℓ=1({Θ2(τℓ,Sℏ(τℓ),Eℏ(τℓ),Iℏ(τℓ),Qℏ(τℓ),Rℏ(τℓ),Sr(τℓ),Er(τℓ),Ir(τℓ))Γ(ρ+2)×hρ1{(m+1−ℓ)ρ(m−ℓ+2+ρ)−(m−ℓ)ρ(m−ℓ+2+2ρ)}−Θ2(τℓ−1,Sℏ(τℓ−1),Eℏ(τℓ−1),Iℏ(τℓ−1),Qℏ(τℓ−1),Rℏ(τℓ−1),Sr(τℓ−1),Er(τℓ−1),Ir(τℓ−1))h1×hρ1{(m+1−ℓ)ρ(m−ℓ+2+ρ)−(m−ℓ)ρ(m−ℓ+2+2ρ)}), |
Iℏ(τm+1)=Iℏ(τ0)+1−ρABC(ρ)Θ3(τm,Sℏ(τm),Eℏ(τm),Iℏ(τm),Qℏ(τm),Rℏ(τm),Sr(τm),Er(τm),Ir(τm))+ρABC(ρ)Γ(ρ)m∑ℓ=1({Θ3(τℓ,Sℏ(τℓ),Eℏ(τℓ),Iℏ(τℓ),Qℏ(τℓ),Rℏ(τℓ),Sr(τℓ),Er(τℓ),Ir(τℓ))Γ(ρ+2)×hρ1{(m+1−ℓ)ρ(m−ℓ+2+ρ)−(m−ℓ)ρ(m−ℓ+2+2ρ)}−Θ3(τℓ−1,Sℏ(τℓ−1),Eℏ(τℓ−1),Iℏ(τℓ−1),Qℏ(τℓ−1),Rℏ(τℓ−1),Sr(τℓ−1),Er(τℓ−1),Ir(τℓ−1))h1×hρ1{(m+1−ℓ)ρ(m−ℓ+2+ρ)−(m−ℓ)ρ(m−ℓ+2+2ρ)}), |
Qℏ(τm+1)=Qℏ(τ0)+1−ρABC(ρ)Θ4(τm,Sℏ(τm),Eℏ(τm),Iℏ(τm),Qℏ(τm),Rℏ(τm),Sr(τm),Er(τm),Ir(τm))+ρABC(ρ)Γ(ρ)m∑ℓ=1({Θ4(τℓ,Sℏ(τℓ),Eℏ(τℓ),Iℏ(τℓ),Qℏ(τℓ),Rℏ(τℓ),Sr(τℓ),Er(τℓ),Ir(τℓ))Γ(ρ+2)×hρ1{(m+1−ℓ)ρ(m−ℓ+2+ρ)−(m−ℓ)ρ(m−ℓ+2+2ρ)}−Θ4(τℓ−1,Sℏ(τℓ−1),Eℏ(τℓ−1),Iℏ(τℓ−1),Qℏ(τℓ−1),Rℏ(τℓ−1),Sr(τℓ−1),Er(τℓ−1),Ir(τℓ−1))h1×hρ1{(m+1−ℓ)ρ(m−ℓ+2+ρ)−(m−ℓ)ρ(m−ℓ+2+2ρ)}), |
Rℏ(τm+1)=Rℏ(τ0)+1−ρABC(ρ)Θ5(τm,Sℏ(τm),Eℏ(τm),Iℏ(τm),Qℏ(τm),Rℏ(τm),Sr(τm),Er(τm),Ir(τm))+ρABC(ρ)Γ(ρ)m∑ℓ=1({Θ5(τℓ,Sℏ(τℓ),Eℏ(τℓ),Iℏ(τℓ),Qℏ(τℓ),Rℏ(τℓ),Sr(τℓ),Er(τℓ),Ir(τℓ))Γ(ρ+2)×hρ1{(m+1−ℓ)ρ(m−ℓ+2+ρ)−(m−ℓ)ρ(m−ℓ+2+2ρ)}−Θ5(τℓ−1,Sℏ(τℓ−1),Eℏ(τℓ−1),Iℏ(τℓ−1),Qℏ(τℓ−1),Rℏ(τℓ−1),Sr(τℓ−1),Er(τℓ−1),Ir(τℓ−1))h1×hρ1{(m+1−ℓ)ρ(m−ℓ+2+ρ)−(m−ℓ)ρ(m−ℓ+2+2ρ)}), |
Sr(τm+1)=Sr(τ0)+1−ρABC(ρ)Θ6(τm,Sℏ(τm),Eℏ(τm),Iℏ(τm),Qℏ(τm),Rℏ(τm),Sr(τm),Er(τm),Ir(τm))+ρABC(ρ)Γ(ρ)m∑ℓ=1({Θ6(τℓ,Sℏ(τℓ),Eℏ(τℓ),Iℏ(τℓ),Qℏ(τℓ),Rℏ(τℓ),Sr(τℓ),Er(τℓ),Ir(τℓ))Γ(ρ+2)×hρ1{(m+1−ℓ)ρ(m−ℓ+2+ρ)−(m−ℓ)ρ(m−ℓ+2+2ρ)}−Θ6(τℓ−1,Sℏ(τℓ−1),Eℏ(τℓ−1),Iℏ(τℓ−1),Qℏ(τℓ−1),Rℏ(τℓ−1),Sr(τℓ−1),Er(τℓ−1),Ir(τℓ−1))h1×hρ1{(m+1−ℓ)ρ(m−ℓ+2+ρ)−(m−ℓ)ρ(m−ℓ+2+2ρ)}), |
Er(τm+1)=Er(τ0)+1−ρABC(ρ)Θ7(τm,Sℏ(τm),Eℏ(τm),Iℏ(τm),Qℏ(τm),Rℏ(τm),Sr(τm),Er(τm),Ir(τm))+ρABC(ρ)Γ(ρ)m∑ℓ=1({Θ7(τℓ,Sℏ(τℓ),Eℏ(τℓ),Iℏ(τℓ),Qℏ(τℓ),Rℏ(τℓ),Sr(τℓ),Er(τℓ),Ir(τℓ))Γ(ρ+2)×hρ1{(m+1−ℓ)ρ(m−ℓ+2+ρ)−(m−ℓ)ρ(m−ℓ+2+2ρ)}−Θ7(τℓ−1,Sℏ(τℓ−1),Eℏ(τℓ−1),Iℏ(τℓ−1),Qℏ(τℓ−1),Rℏ(τℓ−1),Sr(τℓ−1),Er(τℓ−1),Ir(τℓ−1))h1×hρ1{(m+1−ℓ)ρ(m−ℓ+2+ρ)−(m−ℓ)ρ(m−ℓ+2+2ρ)}). |
Ir(τm+1)=Ir(τ0)+1−ρABC(ρ)Θ8(τm,Sℏ(τm),Eℏ(τm),Iℏ(τm),Qℏ(τm),Rℏ(τm),Sr(τm),Er(τm),Ir(τm))+ρABC(ρ)Γ(ρ)m∑ℓ=1({Θ8(τℓ,Sℏ(τℓ),Eℏ(τℓ),Iℏ(τℓ),Qℏ(τℓ),Rℏ(τℓ),Sr(τℓ),Er(τℓ),Ir(τℓ))Γ(ρ+2)×hρ1{(m+1−ℓ)ρ(m−ℓ+2+ρ)−(m−ℓ)ρ(m−ℓ+2+2ρ)}−Θ8(τℓ−1,Sℏ(τℓ−1),Eℏ(τℓ−1),Iℏ(τℓ−1),Qℏ(τℓ−1),Rℏ(τℓ−1),Sr(τℓ−1),Er(τℓ−1),Ir(τℓ−1))h1×hρ1{(m+1−ℓ)ρ(m−ℓ+2+ρ)−(m−ℓ)ρ(m−ℓ+2+2ρ)}). |
Nonlinearities exist in both the integer-order and projected fractional-order systems, necessitating the application of the development of computational approaches to produce the requisite simulation model. For dynamic simulations of the mathematical formalism, a standard numerical approach from conventional calculus characterized as the Lagrange polynomial [44], has been employed; but, for fractional-order structures, recently created numerical techniques in [32, 35, 54] were implemented by utilizing the Atangana-Baleanu fractional derivative operator. Furthermore, the basic reproduction number is an important metric in patterns since it provides us with a plethora of data about the condition. We presented stability projections demonstrating the fluctuations of R0 by using influential factors to analyze the influence of numerous disease spreading characteristics on the basic reproductive number.
Figures 4–7 shows that MPX and variola infections are phylogenically connected and the smallpox vaccine is 65 % efficacious in eliminating MPX within the Atangana-Baleanu fractional derivative operator. Cross-reactive immune responses exist against the Orthopoxvirus genera, which means that immunized people have a considerably reduced chance of illness and fatality than uninfected people. For the purpose of clarification, the vaccine's unsatisfactory protection probability was deleted from the numerical model's formulation. Inadequate fortification, as highlighted in [55], exacerbates the challenge of proactive preventive acts, as poorer vaccine efficiency can contribute to higher vaccine penetration and indicative impacts; meanwhile, the epidemic's influence can be more difficult to ameliorate. We can deduce that diminishing the fractional-order ρ significantly decreases the occurrence of vulnerable, exposed, infectious, isolated and restored human populations as well as the rodent community.
As shown in Figures 8–11, determining the value of φℏ reduces virus transmission. In addition to immunization, it would be interesting to identify and evaluate potential preventive interventions that could have a discernible impact on MPX propagation. For example, reducing animal-to-human interaction and starting an awareness program about the consequences of consuming unprocessed meat, which appears to be the primary cause of squirrel-to-human [56] dissemination, would drastically reduce the animal-to-human propagation incidence. It may result in a high operating cost (for example, a reduction in meat availability), but it does not necessitate the costly or poorly functioning healthcare systems required for vaccination, providing the public with a commonly approachable prophylactic strategy. Such an amendment's mathematical analysis would grow more complicated. The basic idea may be similar to that of [57], the authors of which investigated cholera preventative conditions in which people could be vaccinated or consume dangerously toxic water. Figures 8–11 show how dropping the ρ from 1 deflects the susceptible human incident Sℏ curves, resulting in a considerable mitigation in the number of occurrences in the cohorts.
The level of interaction involving the rodent community has a significant effect on the MPX spread, as shown in Figures 12–15. We included a cohort Qℏ in the model, which contains the separated portion of infectious individuals. We demonstrated how the contaminated community would respond in the context of specific treatments by using numerical modeling. Furthermore, the real data [43] included with the fractional-order, which predicts that real data is in strong harmony the fractional-order one.
In a nutshell, Figures 16–19 depicts a cumulative comparative evaluation of both classical and suggested fractional-order modelling techniques with preventive measures and non-preventive measures, showing that several of the observations from the Atangana–Baleanu operator are pretty close to the factual arguments for about 8 weeks, indicating that the suggested fractional-order derivative operator has the best effectiveness proportion.
Hence, we conclude that Atangana-Baleanu fractional-order modeling in the Caputo context is still a useful approach for gaining a better grasp of how MPX works in different situations. Epidemiologic modelling and fractional analysis have a broad array of applications. We expect that the simulations can be used as a prognostic approach to precisely comprehend the transmission of MPX as more occurrences of the virus are documented in the human population.
To better comprehend the spread of MPX infection, a non linear deterministic mathematical model has been devised by applying the Atangana-Baleanu fractional derivative in the Caputo viewpoint. The suggested paradigm consists of eight compartments that are collectively exhaustive. The human species has been separated into five cohorts, each with its own variety of challenges. Likewise, the rodent community was classified into three categories. In addition, the essential features of the suggested framework have been demonstrated. The next-generation matrix approach was used to determine the basic reproduction value. There are two equilibria in the developed framework: a DFE point and an EEP. The stability requirements for both equilibrium conditions have been determined. Furthermore, the presence of an endemic equilibrium means that a backward bifurcation is conceivable. We have also demonstrated the interactive effects of several settings on the fractional-order by using numerical computations. According to our findings, the numerical results show that decreasing the order of the fractional derivative from 1 straightens the graphs and reduces the probability of susceptible individuals. The ABC fractional operator expressing the hereditary property is credited with this crucial breakthrough. The generalized ML function outperformed the exponential decay and index law kernels due to resilient reminiscence linked to the Atangana–Baleanu fractional derivative. Moreover, the Atangana–Baleanu fractional order derivative is also Liouville–Caputo and Caputo–Fabrizio, indicating that it has both Markovian and non-Markovian features. We, the investigators of this extensive review on the impact of various fractional formulations, including fractal–fractional derivatives, who have also analyzed the efficiency of the ABC fractional operator results on systems characterized by numerous and additional prevalent pathogen systems, may validate this notion.
The authors declare that there is no conflict of interest.
[1] |
A. W. Rimoin, P. M. Mulembakani, S. C. Johnstonm, J. O. Lloyd Smith, N. K. Kisalu, T. L. Kinkela, et al., Major increase in human monkeypox incidence 30 years after smallpox vaccination campaigns cease in the Democratic Republic of Congo, Proc. Natl. Acad. Sci. USA, 107 (2010), 16262–16267. http://dx.doi.org/10.1073/pnas.1005769107 doi: 10.1073/pnas.1005769107
![]() |
[2] | N. Sklenovská, M. Van Ranst, Emergence of monkeypox as the most important orthopoxvirus infection in humans, Front. Public Health, 241 (2018). https://doi.org/10.3389/fpubh.2018.00241 |
[3] | Singapore Ministry of Health, Europe, US on alert over detection of Monkeypox cases: What is the virus, symptoms and its transmission across the globe. Available from: https://news.knowledia.com/IN/en/articles/europe-us-on-alert-over-detection-of-monkeypox-cases-what-is-the-virus-5. |
[4] | F. Fenner, D. A. Henderson, I. Arita, Z. Jezek, I. D. Ladnyi, Smallpox and its eradication, W. H. O., 1988. |
[5] | R. B. Kennedy, J. M. Lane, D. A. Henderson, G. A. Poland, Smallpox and vaccinia, Vaccines (chapter 32), Amsterdam: Elsevier, (2012), 718–727. |
[6] |
P. E. M. Fine, Z. Jezek, B. Grab, H. Dixon, The transmission potential of monkeypox virus in human populations, Int. J. Epidemiol. , 17 (1988), 643–650. http://dx.doi.org/10.1093/ije/17.3.643 doi: 10.1093/ije/17.3.643
![]() |
[7] |
K. D. Reed, J. W. Melski, M. B. Graham, R. L. Regnery, M. J. Sotir, M. V. Wegner, et al., The detection of monkeypox in humans in the Western Hemisphere, Engl. J. Med. , 350 (2004), 342–350. http://dx.doi.org/10.1056/ NEJMoa032299 doi: 10.1056/NEJMoa032299
![]() |
[8] | M. Roberts, Monkeypox to get a new name, says WHO. Available from: https://en.kataeb.org/articles/monkeypox-to-get-a-new-name-says-who. |
[9] |
L. A. Learned, M. G. Reynolds, D. W. Wassa, Y. Li, V. A. Olson, K. Karem, et al., Extended interhuman transmission of monkeypox in a hospital community in the Republic of the Congo, Am. J. Trop. Med. Hygiene, 73 (2005), 428–434. https://doi.org/10.4269/ajtmh.2005.73.428 doi: 10.4269/ajtmh.2005.73.428
![]() |
[10] |
R. A. Elderfield, S. J. Watson, A. Godlee, W. E. Adamson, C. I. Thompson, J. Dunning, M. Fernandez-Alonso, D. Blumenkrantz, T. Hussell, M. Zambon, Accumulation of human-adapting mutations during circulation of A (H1N1) pdm09 influenza virus in humans in the United Kingdom, J. Virol. , 88 (2014), 13269–13283. https://doi.org/10.1128/JVI.01636-14 doi: 10.1128/JVI.01636-14
![]() |
[11] |
N. C. Elde, S. J. Child, M. T. Eickbush, J. O. Kitzman, K. S. Rogers, J. Shendure, et al., Poxviruses deploy genomic accordions to adapt rapidly against host antiviral defenses, Cell, 150 (2012), 831–841. https://doi.org/10.1016/j.cell.2012.05.049 doi: 10.1016/j.cell.2012.05.049
![]() |
[12] |
R. J. Jackson, A. J. Ramsay, C. D. Christensen, S. Beaton, D. F. Hall, I. A. Ramshaw, Expression of mouse interleukin-4 by a recombinant ectromelia virus suppresses cytolytic lymphocyte responses and overcomes genetic resistance to mousepox. J. Virol. , 75 (2001), 1205–1210. https://doi.org/10.1128/JVI.75.3.1205-1210.2001 doi: 10.1128/JVI.75.3.1205-1210.2001
![]() |
[13] |
S. Bidari, E. E. Goldwyn, Stochastic models of influenza outbreaks on a college campus, Lett. Biomath. , 6 (2019), 1–14. https://doi.org/10.1080/23737867.2019.1618744 doi: 10.1080/23737867.2019.1618744
![]() |
[14] |
J. C. Blackwood, L. M. Childs, An introduction to compartmental modeling for the budding infectious disease modeler. Lett. Biomath. , 5 (2018), 195–221. https://doi.org/10.30707/LiB5.1Blackwood doi: 10.30707/LiB5.1Blackwood
![]() |
[15] | C. Bhunu, S. Mushayabasa, Modelling the transmission dynamics of Pox-like infections, IAENG Int. J. Appl. Math. , 41 (2011), 141–149. |
[16] |
S. Usman, I. I. Adamu, Modeling the transmission dynamics of the monkeypox virus infection with treatment and vaccination interventions, J. Appl. Math. Phy. , 5 (2017), 2335–2353. https://doi.org/10.4236/jamp.2017.512191 doi: 10.4236/jamp.2017.512191
![]() |
[17] |
A. Atangana, D. Baleanu, New fractional derivatives with nonlocal and non-singular kernel: Theory and application to heat transfer model, Therm. Sci. , 20 (2016), 763–769. https://doi.org/10.2298/TSCI160111018A doi: 10.2298/TSCI160111018A
![]() |
[18] |
S. A. Iqbal, M. G. Hafez, Y. M. Chu, C. Park, Dynamical Analysis of nonautonomous RLC circuit with the absence and presence of Atangana-Baleanu fractional derivative, J. Appl. Anal. Comput. 12 (2022), 770–789. https://doi.org/10.11948/20210324 doi: 10.11948/20210324
![]() |
[19] | Y. M. Chu, S. Bashir, M. Ramzan, M. Y. Malik, Model-based comparative study of magnetohydrodynamics unsteady hybrid nanofluid flow between two infinite parallel plates with particle shape effects, Math. Meth. Appl. Sci., 2022. https://doi.org/10.1002/mma.8234 |
[20] |
W. M. Qian, H. H. Chu, M. K. Wang, Y. M. Chu, Sharp inequalities for the Toader mean of order −1 in terms of other bivariate means, J. Math. Inequal. , 16 (2022), 127–141. https://doi.org/10.7153/jmi-2022-16-10 doi: 10.7153/jmi-2022-16-10
![]() |
[21] |
T. H. Zhao, H. H. Chu, Y. M. Chu, Optimal Lehmer mean bounds for the nth power-type Toader mean of n=−1,1,3, J. Math. Inequal. , 16 (2022), 157–168. https://doi.org/10.7153/jmi-2022-16-12 doi: 10.7153/jmi-2022-16-12
![]() |
[22] | M. Caputo, M. Fabrizio, A new definition of fractional derivative without singular kernel, Progr. Fract. Differ. Appl. , 1 (2015), 1–13. |
[23] |
J. F. Gómez-Aguilar, H. Yéppez-Marténez, C. Calderón-Ramón, I. Cruz-Orduña, R. F. Escobar-Jiménez, V. H. Olivares-Peregrino, Modeling of a mass-spring-damper system by fractional derivatives with and without a singular kernel, Entropy, 17 (2015), 6289–6303. https://doi.org/10.3390/e17096289 doi: 10.3390/e17096289
![]() |
[24] | F. Z. Wang, M. N. Khan, I. Ahmad, H. Ahmad, H. Abu-Zinadah, Y. M. Chu, Numerical solution of traveling waves in chemical kinetics: time-fractional fishers equations, Fractals, 30 (2022). https://doi.org/10.1142/S0218348X22400515 |
[25] | S. Rashid, E. I. Abouelmagd, S. Sultana, Y. M. Chu, New developments in weighted n-fold type inequalities via discrete generalized ˆh-proportional fractional operators, Fractals, 30 (2022). https://doi.org/10.1142/S0218348X22400564 |
[26] |
S. Rashid, B. Kanwal, A. G. Ahmad, E. Bonyah, S. K. Elagan, Novel numerical estimates of the pneumonia and meningitis epidemic model via the nonsingular kernel with optimal analysis, Complexity, 2022 (2022), 1–25. https://doi.org/10.1155/2022/4717663 doi: 10.1155/2022/4717663
![]() |
[27] | S. W. Yao, S. Rashid, M. Inc, E. Elattar, On fuzzy numerical model dealing with the control of glucose in insulin therapies for diabetes via nonsingular kernel in the fuzzy sense, AIMS Math., 7 (2022). https://doi.org/10.3934/math.2022987 |
[28] |
S. Rashid, F. Jarad, A. K. Alsharidi, Numerical investigation of fractional-order cholera epidemic model with transmission dynamics via fractal-fractional operator technique, Chaos Solitons Fractals, 162 (2022), 112477. https://doi.org/10.1016/j.chaos.2022.112477 doi: 10.1016/j.chaos.2022.112477
![]() |
[29] |
F. Jin, Z. S. Qian, Y. M. Chu, M. ur Rahman, On nonlinear evolution model for drinking behavior under Caputo-Fabrizio derivative, J. Appl. Anal. Comput. 12 (2022), 790–806. https://doi.org/10.11948/20210357 doi: 10.11948/20210357
![]() |
[30] |
S. Rashid, M. K. Iqbal, A. M. Alshehri, F. Jarad, R. Ashraf, A comprehensive analysis of the stochastic fractal-fractional tuberculosis model via Mittag-Leffler kernel and white noise, Results Phy. . 39 (2022), 105764. https://doi.org/10.1016/j.rinp.2022.105764 doi: 10.1016/j.rinp.2022.105764
![]() |
[31] |
M. Al-Qurashi, S. Rashid, F. Jarad, A computational study of a stochastic fractal-fractional hepatitis B virus infection incorporating delayed immune reactions via the exponential decay, Math. Biosci. Eng, 19 (2022), 12950–12980. https://doi.org/10.3934/mbe.2022605 doi: 10.3934/mbe.2022605
![]() |
[32] |
S. Rashid, A. Khalid, S. Sultana, F. Jarad, K. M. Abulanja, Y. S. Hamed, Novel numerical investigation of the fractional oncolytic effectiveness model with M1 virus via generalized fractional derivative with optimal criterion, Results Phy. , 37 (2022), 105553. https://doi.org/10.1016/j.rinp.2022.105553 doi: 10.1016/j.rinp.2022.105553
![]() |
[33] |
S. Rashid, B. Kanwal, F. Jarad, S. K. Elagan, A peculiar application of the fractal-fractional derivative in the dynamics of a nonlinear scabies model, Results Phys. , 38 (2022), 105634. https://doi.org/10.1016/j.rinp.2022.105634 doi: 10.1016/j.rinp.2022.105634
![]() |
[34] |
S. Rashid, Y. G. Sánchez, J. Singh, Kh. M. Abualnaja, Novel analysis of nonlinear dynamics of a fractional model for tuberculosis disease via the generalized Caputo fractional derivative operator (case study of Nigeria), AIMS Math. , 7 (2022), 10096–10121. https://doi.org/10.3934/math.2022562 doi: 10.3934/math.2022562
![]() |
[35] |
S. Rashid, F. Jarad, A. G. Ahmad, Kh. M. Abualnaja, New numerical dynamics of the heroin epidemic model using a fractional derivative with Mittag-Leffler kernel and consequences for control mechanisms, Results Phys. , 35 (2022), 105304. https://doi.org/10.1016/j.rinp.2022.105304 doi: 10.1016/j.rinp.2022.105304
![]() |
[36] |
S. N. Hajiseyedazizi, M. E. Samei, J. Alzabut, Y. M. Chu, On multi-step methods for singular fractional q-integro-differential equations, Open Math. 19 (2021), 1378–1405. https://doi.org/10.1515/math-2021-0093 doi: 10.1515/math-2021-0093
![]() |
[37] | S. Rashid, E. I. Abouelmagd, A. Khalid, F. B. Farooq, Y. M. Chu, Some recent developments on dynamical ℏ-discrete fractional type inequalities in the frame of nonsingular and nonlocal kernels, Fractals, 30 (2022). https://doi.org/10.1142/S0218348X22401107 |
[38] | Y. M. Chu, U. Nazir, M. Sohail, M. M. Selim, J. R. Lee, Enhancement in thermal energy and solute particles using hybrid nanoparticles by engaging activation energy and chemical reaction over a parabolic surface via finite element approach, Fractal Fract. 5 (2021), 119. https://doi.org/10.3390/fractalfract5030119 |
[39] |
T. Abdeljawad, D. Baleanu, Integration by parts and its applications of a new nonlocal fractional derivative with Mittag-Leffler nonsingular kernel, J. Nonlinear Sci. Appl. , 10 (2017), 1098–1107. http://dx.doi.org/10.22436/jnsa.010.03.20 doi: 10.22436/jnsa.010.03.20
![]() |
[40] |
J. Singh, D. Kumar, D. Baleanu, On the analysis of fractional diabetes model with exponential law, Adv. Differ. Equ. , 2018 (2018), 231. https://doi.org/10.1186/s13662-018-1680-1 doi: 10.1186/s13662-018-1680-1
![]() |
[41] |
Z. M. Odibat, N. T. Shawagfeh, Generalized Taylor's formula, Appl. Math. Comput. 186 (2007), 286–293. https://doi.org/10.1016/j.amc.2006.07.102 doi: 10.1016/j.amc.2006.07.102
![]() |
[42] |
S. V. Bankuru, S. Kossol, W. Hou, P. Mahmoudi, J. Rychtáˆr, D. Taylor, A game-theoretic model of Monkeypox to assess vaccination strategies, PeerJ, 8 (2020), 9272. http://doi.org/10.7717/peerj.927 doi: 10.7717/peerj.927
![]() |
[43] | O. J. Peter, S. Kumar, N. Kumari, F. A. Oguntolu, K. Oshinubi, R. Musa, Transmission dynamics of Monkeypox virus: A mathematical modelling approach, Model. Earth Sys. Envir., 2021. https://doi.org/10.1007/s40808-021-01313-2 |
[44] |
M. Toufik, A. Atangana, New numerical approximation of fractional derivative with non-local and non-singular kernel: Application to chaotic models, Eur. Phys. J. Plus, 132 (2017), 444. https://doi.org/10.1140/epjp/i2017-11717-0 doi: 10.1140/epjp/i2017-11717-0
![]() |
[45] |
C. Bhunu, W. Garira, G. Magombedze, Mathematical analysis of a two strain hiv/aids model with antiretroviral treatment. Acta Biotheor. , 57 (2009), 361–381. https://doi.org/10.1007/s10441-009-9080-2 doi: 10.1007/s10441-009-9080-2
![]() |
[46] | C. Bhunu, S. Mushayabasa, Modelling the transmission dynamics of pox-like infections, IAENG Int. J. Appl. Math. , 41 (2011), 141–149. |
[47] |
M. R. Odom, R. Curtis Hendrickson, E. J. Lefkowitz, Poxvirus protein evolution: Family wide assessment of possible horizontal gene transfer events, Virus Res. , 144 (2009), 233–249. https://doi.org/10.1016/j.virusres.2009.05.006 doi: 10.1016/j.virusres.2009.05.006
![]() |
[48] |
Y. Lia, Y. Chen, I. Podlubny, Mittag–Leffler stability of fractional order nonlinear dynamic systems, Automatica, 45 (2009), 1965–1969. https://doi.org/10.1016/j.automatica.2009.04.003 doi: 10.1016/j.automatica.2009.04.003
![]() |
[49] |
S. Somma, N. Akinwande, U. Chado, A mathematical model of monkey pox virus transmission dynamics, IFE J. Sci. , 21 (2019), 195–204. https://doi.org/10.4314/ijs.v21i1.17 doi: 10.4314/ijs.v21i1.17
![]() |
[50] |
O. Diekmann, J. Heesterbeek, M. G. Roberts, The construction of next-generation matrices for compartmental epidemic models, J. R. Soc. Interface, 7 (2010), 873–885. https://doi.org/10.1098/rsif.2009.0386 doi: 10.1098/rsif.2009.0386
![]() |
[51] | P. van den Driessche, J. Watmough, Further notes on the basic reproduction number, Springer, Berlin, Heidelberg, (2008), 159–178. |
[52] |
C. Castillo-Chavez, B. Song, Dynamical models of tuberculosis and their applications, Math. Biosci. Eng. , 1 (2004), 361. https://doi.org/10.3934/mbe.2004.1.361 doi: 10.3934/mbe.2004.1.361
![]() |
[53] |
E. Ahmed, A. M. A. El-Sayed, H. A. A. El-Saka, On some routh–hurwitz conditions for fractional order differential equations and their applications in lorenz, rössler, chua and chen systems, Phys. Lett. A, 358 (2006), 1–4. https://doi.org/10.1016/j.physleta.2006.04.087 doi: 10.1016/j.physleta.2006.04.087
![]() |
[54] |
C. T. Deressa, G. F. Duressa, Analysis of Atangana–Baleanu fractional-order SEAIR epidemic model with optimal control, Adv. Diff. Equ. , 2021 (2021), 174. https://doi.org/10.1186/s13662-021-03334-8 doi: 10.1186/s13662-021-03334-8
![]() |
[55] |
B. Wu, F. Fu, L. Wang, Imperfect vaccine aggravates the long-standing dilemma of voluntary vaccination, PLOS ONE, 6 (2011), 20577. https://doi.org/10.1371/journal.pone.0020577 doi: 10.1371/journal.pone.0020577
![]() |
[56] | L. Khodakevich, Z. Ježek, D. Messinger, Monkeypox virus: Ecology and public health significance, Bull. W. H. O. , 66 (1988), 747–752. |
[57] |
J. Kobe, N. Pritchard, Z. Short, I. V. Erovenko, J. Rychtář, J. T. Rowel, A game theoretic model of cholera with optimal personal protection strategies. Bull. Math. Bio., 80 (2018), 2580–2599. https://doi.org/10.1007/s11538-018-0476-5 doi: 10.1007/s11538-018-0476-5
![]() |
1. | Sehrish Ramzan, Syeda Alishwa Zanib, Muzamil Abbas Shah, Nadeem Abbas, Wasfi Shatanawi, Analytical study of a modified monkeypox virus model using Caputo–Fabrizio fractional derivatives, 2024, 10, 2363-6203, 6475, 10.1007/s40808-024-02115-y | |
2. | Guyue Liu, Huilai Li, Dynamical analysis of a class of Monkeypox epidemic model, 2024, 28, 0354-9836, 3367, 10.2298/TSCI2404367L | |
3. | Obiora Cornelius Collins, Kevin Jan Duffy, Dynamics and control of mpox disease using two modelling approaches, 2024, 10, 2363-6203, 1657, 10.1007/s40808-023-01862-8 | |
4. | James Andrawus, Yau Umar Ahmad, Agada Apeh Andrew, Abdullahi Yusuf, Sania Qureshi, Ballah Akawu Denue, Habu Abdul, Soheil Salahshour, Impact of surveillance in human-to-human transmission of monkeypox virus, 2024, 1951-6355, 10.1140/epjs/s11734-024-01346-5 | |
5. | Weerawat Sudsutad, Chatthai Thaiprayoon, Jutarat Kongson, Weerapan Sae-dan, A mathematical model for fractal-fractional monkeypox disease and its application to real data, 2024, 9, 2473-6988, 8516, 10.3934/math.2024414 | |
6. | Yau Umar Ahmad, James Andrawus, Abdurrahman Ado, Yahaya Adamu Maigoro, Abdullahi Yusuf, Saad Althobaiti, Umar Tasiu Mustapha, Mathematical modeling and analysis of human-to-human monkeypox virus transmission with post-exposure vaccination, 2024, 10, 2363-6203, 2711, 10.1007/s40808-023-01920-1 | |
7. | Mohammad Sharif Ullah, K.M. Ariful Kabir, Behavioral game of quarantine during the monkeypox epidemic: Analysis of deterministic and fractional order approach, 2024, 10, 24058440, e26998, 10.1016/j.heliyon.2024.e26998 | |
8. | Dilara Yapışkan, Mine Yurtoğlu, Derya Avcı, Beyza Billur İskender Eroğlu, Ebenezer Bonyah, A Novel Model for Monkeypox Disease: System Analysis and Optimal Preventive Strategies, 2023, 47, 2731-8095, 1665, 10.1007/s40995-023-01525-4 | |
9. | Wilfredo Meza Cuba, Juan Carlos Huaman Alfaro, Hasnain Iftikhar, Javier Linkolk López-Gonzales, Modeling and Analysis of Monkeypox Outbreak Using a New Time Series Ensemble Technique, 2024, 13, 2075-1680, 554, 10.3390/axioms13080554 | |
10. | Kaushal Soni, Arvind Kumar Sinha, Modeling marburg virus control with limited hospital beds: a fractional approach, 2025, 100, 0031-8949, 015251, 10.1088/1402-4896/ad9ae6 | |
11. | Jutarat Kongson, Chatthai Thaiprayoon, Weerawat Sudsutad, Analysis of a mathematical model for the spreading of the monkeypox virus with constant proportional-Caputo derivative operator, 2025, 10, 2473-6988, 4000, 10.3934/math.2025187 |
Symbols | Explanations | Values | References | |
χℏ | Proportion of humans acquisition | 0.029 | [45] | |
χr | Proportion of rodents acquisition | 0.2 | [45] | |
η1 | Rodent-human interaction rate | 0.00025 | [46] | |
η2 | Rate of human-to-human interaction | 0.00006 | [46] | |
η3 | Rate of rodent-to-rodent interaction | 0.027 | [46] | |
δ1 | Rate of exposed people to infectious people | 0.2 | Supposed | |
δ2 | Rate of confirmed reported incidents | 2.0 | Estimated | |
σ | Rate of untreated after screening | 2.0 | Estimated | |
ψ | Transition from the separated to the restored group | 0.52 | Supposed | |
γ | Humans' rate of recuperation | 0.83 | [45] | |
υℏ | Natural mortality rate for people | 1.5 | [46] | |
υr | Natural mortality rate for rodents | 0.002 | [46] | |
φℏ | Proportion of rodents dying as a result of disease | 0.5 | Supposed | |
φr | Proportion of humans dying as a result of disease | 0.2 | [47] |
Symbols | Explanations | Values | References | |
χℏ | Proportion of humans acquisition | 0.029 | [45] | |
χr | Proportion of rodents acquisition | 0.2 | [45] | |
η1 | Rodent-human interaction rate | 0.00025 | [46] | |
η2 | Rate of human-to-human interaction | 0.00006 | [46] | |
η3 | Rate of rodent-to-rodent interaction | 0.027 | [46] | |
δ1 | Rate of exposed people to infectious people | 0.2 | Supposed | |
δ2 | Rate of confirmed reported incidents | 2.0 | Estimated | |
σ | Rate of untreated after screening | 2.0 | Estimated | |
ψ | Transition from the separated to the restored group | 0.52 | Supposed | |
γ | Humans' rate of recuperation | 0.83 | [45] | |
υℏ | Natural mortality rate for people | 1.5 | [46] | |
υr | Natural mortality rate for rodents | 0.002 | [46] | |
φℏ | Proportion of rodents dying as a result of disease | 0.5 | Supposed | |
φr | Proportion of humans dying as a result of disease | 0.2 | [47] |