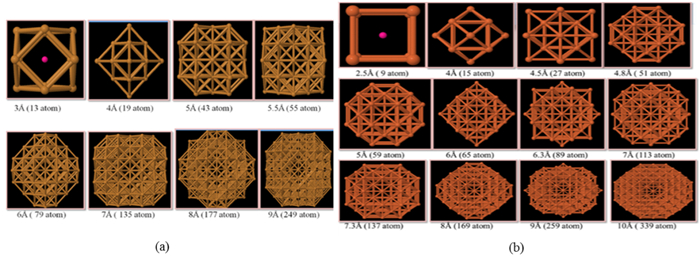
As a classic problem of distributed scheduling, the distributed flow-shop scheduling problem (DFSP) involves both the job allocation and the operation sequence inside the factory, and it has been proved to be an NP-hard problem. Many intelligent algorithms have been proposed to solve the DFSP. However, the efficiency and quality of the solution cannot meet the production requirements. Therefore, this paper proposes a bi-objective particle swarm optimization with direction search and differential evolution to solve DFSP with the criteria of minimizing makespan and total processing time. The direction search strategy explores the particle swarm in multiple directions of the Pareto front, which enhances the strong convergence ability of the algorithm in different areas of Pareto front and improves the solution speed of the algorithm. The search strategy based on differential evolution is the local search strategy of the algorithm, which can prevent the multiobjective particle swarm optimization from converging prematurely and avoid falling into local optimum, so that a better solution can be found. The combination of these two strategies not only increases the probability of particles moving in a good direction, but also increases the diversity of the particle swarm. Finally, experimental results on benchmark problems show that, compared with traditional multiobjective evolutionary algorithms, the proposed algorithm can accelerate the convergence speed of the algorithm while guaranteeing that the obtained solutions have good distribution performance and diversity.
Citation: Wenqiang Zhang, Chen Li, Mitsuo Gen, Weidong Yang, Zhongwei Zhang, Guohui Zhang. Multiobjective particle swarm optimization with direction search and differential evolution for distributed flow-shop scheduling problem[J]. Mathematical Biosciences and Engineering, 2022, 19(9): 8833-8865. doi: 10.3934/mbe.2022410
[1] | Luca Azzolin, Luca Dedè, Antonello Gerbi, Alfio Quarteroni . Effect of fibre orientation and bulk modulus on the electromechanical modelling of human ventricles. Mathematics in Engineering, 2020, 2(4): 614-638. doi: 10.3934/mine.2020028 |
[2] | Yu Leng, Lampros Svolos, Dibyendu Adak, Ismael Boureima, Gianmarco Manzini, Hashem Mourad, Jeeyeon Plohr . A guide to the design of the virtual element methods for second- and fourth-order partial differential equations. Mathematics in Engineering, 2023, 5(6): 1-22. doi: 10.3934/mine.2023100 |
[3] | Claudio Canuto, Davide Fassino . Higher-order adaptive virtual element methods with contraction properties. Mathematics in Engineering, 2023, 5(6): 1-33. doi: 10.3934/mine.2023101 |
[4] | Andrea Borio, Martina Busetto, Francesca Marcon . SUPG-stabilized stabilization-free VEM: a numerical investigation. Mathematics in Engineering, 2024, 6(1): 173-191. doi: 10.3934/mine.2024008 |
[5] | Thomas J. Radley, Paul Houston, Matthew E. Hubbard . Quadrature-free polytopic discontinuous Galerkin methods for transport problems. Mathematics in Engineering, 2024, 6(1): 192-220. doi: 10.3934/mine.2024009 |
[6] | Zaffar Mehdi Dar, M. Arrutselvi, Chandru Muthusamy, Sundararajan Natarajan, Gianmarco Manzini . Virtual element approximations of the time-fractional nonlinear convection-diffusion equation on polygonal meshes. Mathematics in Engineering, 2025, 7(2): 96-129. doi: 10.3934/mine.2025005 |
[7] | Zhiwen Zhao . Exact solutions for the insulated and perfect conductivity problems with concentric balls. Mathematics in Engineering, 2023, 5(3): 1-11. doi: 10.3934/mine.2023060 |
[8] | Stefano Berrone, Stefano Scialò, Gioana Teora . The mixed virtual element discretization for highly-anisotropic problems: the role of the boundary degrees of freedom. Mathematics in Engineering, 2023, 5(6): 1-32. doi: 10.3934/mine.2023099 |
[9] | Daniele Cerroni, Florin Adrian Radu, Paolo Zunino . Numerical solvers for a poromechanic problem with a moving boundary. Mathematics in Engineering, 2019, 1(4): 824-848. doi: 10.3934/mine.2019.4.824 |
[10] | Luca Placidi, Emilio Barchiesi, Francesco dell'Isola, Valerii Maksimov, Anil Misra, Nasrin Rezaei, Angelo Scrofani, Dmitry Timofeev . On a hemi-variational formulation for a 2D elasto-plastic-damage strain gradient solid with granular microstructure. Mathematics in Engineering, 2023, 5(1): 1-24. doi: 10.3934/mine.2023021 |
As a classic problem of distributed scheduling, the distributed flow-shop scheduling problem (DFSP) involves both the job allocation and the operation sequence inside the factory, and it has been proved to be an NP-hard problem. Many intelligent algorithms have been proposed to solve the DFSP. However, the efficiency and quality of the solution cannot meet the production requirements. Therefore, this paper proposes a bi-objective particle swarm optimization with direction search and differential evolution to solve DFSP with the criteria of minimizing makespan and total processing time. The direction search strategy explores the particle swarm in multiple directions of the Pareto front, which enhances the strong convergence ability of the algorithm in different areas of Pareto front and improves the solution speed of the algorithm. The search strategy based on differential evolution is the local search strategy of the algorithm, which can prevent the multiobjective particle swarm optimization from converging prematurely and avoid falling into local optimum, so that a better solution can be found. The combination of these two strategies not only increases the probability of particles moving in a good direction, but also increases the diversity of the particle swarm. Finally, experimental results on benchmark problems show that, compared with traditional multiobjective evolutionary algorithms, the proposed algorithm can accelerate the convergence speed of the algorithm while guaranteeing that the obtained solutions have good distribution performance and diversity.
Studies of the structure and properties of small copper and iron clusters became important because of the recent development of nanotechnologies. Moreover, the observation of changes in the atomic structure and electronic properties of clusters in relation to their size affords information on the mechanism of the transition from macroscopic to nanosized state. These nanoparticles are attractive as sensors [1], catalysts [2], nanoelectronics, and optoelectronic devices [3]. Their electronic, structural, magnetic, and optical properties are dramatically different from their bulk counterparts and are subject to intense investigation. With the intention of control the properties of nanoparticles through their size, their mechanism of nucleation and growth needs to be clarified, while physical properties associated with downsizing the metal nanoparticles into small nanoclusters attract attention in experimental and theoretical aspects [4]. A fundamental challenge for experimentalists and theorists alike is to obtain qualitative and quantitative information about the structural properties of nanoparticles that are not amenable to direct imaging.
In this regard, X-ray absorption fine structure (XAFS) analysis has proven to be a powerful qualitative and quantitative tool for structural investigations of nanoclusters. XAFS is divided into two parts, depending whether we are referring to the region near the absorption edge, which is X-ray absorption near-edge structure (XANES), or at higher energy with respect to the edge, it is extended X-ray absorption fine structure (EXAFS). XANES is now the recognized method allowing to define in principle the full 3D local atomic geometry, including symmetry and fine details of the electronic structure of the materials under study. EXAFS is a very powerful structural technique to measure the mean values of interatomic distances, coordination numbers, and static disorder. FEFF9 [5] is a software package which does both XANES as well as EXAFS calculations in an advanced manner. This computer code uses the real-space Green's function approach and includes improved treatments of many-body effects such as inelastic losses, core-hole effects, vibrational amplitudes, and the extension to full spectrum calculations of optical constants including solid-state effects. Each FEFF calculation involves the following 3 steps:
● Selection of the absorbing site and the cluster of atoms to be included in the scattering calculations.
● Generation of the FEFF input files for each site and its surrounding atomic cluster.
● Execution of the FEFF binary on the generated input files [6].
In this work, we describe the XANES and EXAFS simulation focused on the early stage of nucleation and the growth of Cu and Fe nanoparticles. Structural evolution for both Cu and Fe clusters were studied at different cluster sizes starting close to the unit cell size for each of them.
Theoretical analysis of the Cu and Fe K-edges of absorption in the different cluster sizes was carried out based on a self-consistent real space full multiple-scattering method using FEFF9 code [5]. The code builds in inelastic losses in terms of a self-energy and includes vibrational effects in terms of Debye–Waller factors. For periodic structures, reciprocal space calculations based on periodic boundary conditions are also available. FEFF can use both full multiple scattering based on Lanczos algorithms and a high-order path expansion based on the Rehr–Albers multiple scattering formalism. The spectra have been simulated using several types of exchange potential: partially nonlocal potential, Hedin–Lundqvist potential and Dirac–Hara potential. Spectral dependence on the relaxation of electrons in the presence of a core hole has been studied as well. The best agreement with the experimental data has been achieved for the spectra calculated with Hedin–Lundqvist potential in the presence of core-hole. For the Cu K-edge calculation of an fcc phase, a lattice with the parameter equal to 3.61 Å [7] with space group Fm-3m was used. For the calculation of the Fe K-edge in the bcc phase, a lattice with the parameter equal to 2.87 Å [7] with space group Im-3m was used. ATOMS on the web connected to FEFF9 site was used in order to create an input file for FEFF9 program. The parameters required for ATOMS are the space group, the lattice constants, the edge of interest (Cu or Fe k-edge in the present case) and finally the central atom and cluster size. At that place there are many other parameters defined in control cards. These cards have utilized in self-consistent field that required in XANES calculation for the Fermi level estimation and full multiple scattering card that used to adjust the number of atoms used in the computation. In addition, the exchange and core hole cards which specify the exchange correlation potential model and the core treatment during calculation respectively, have applied [6].
The theoretical models have constructed for metallic nanoparticles such as copper and iron at n-atoms of clusters (n = 13 to 249 atoms) for copper, and (n = 9 to 339 atoms) for iron. Then, we have carried some adjustments to the experimental reference data [8,9], which used in the simulation comparison. Proposed models of the copper and iron nanoclusters can be achieved when the size of the cluster changes in the diameter from 3 to 9 Å and from 2.5 to 10 Å for copper and iron respectively, as in Figure 1. Finally, extract the structural parameters and the electronic properties of metallic nanoparticles in different sizes by comparing theoretical models with reference spectra of experimental data. To extract the structural parameters such as coordination number (N), interatomic distance (R) and Debye–Waller factor (σ2) by EXAFS technique we carried out the fit procedure with experimental data as a reference by using IFEFFIT software package [10]. In the FEFF software, the oscillations are taken as a sum of different multiple scattering contributions. Each of them can be expressed as:
χn(k)=χnoexp(−Lnλn−2k2σ2n) | (1) |
χno(k)=Fn(k)sin(kLn+θn(k)) | (2) |
where, n represents different single or multiple scattering paths and Ln is the total path length. F and θn are the amplitude and phase, which depend on k, on the specifics of the scattering path involved, and on the atomic potential parameters [11].
Figure 2 summarizes the calculated XANES spectra for the model n-atom clusters (n = 13–249) compared to the experimental data. The results for an fcc metal reproduce the characteristic near-edge features around 9000 eV which appear when n exceeds 43. As smaller clusters (n ≤ 19) have a single peak at this energy, the presence/absence of another peak around 9000 eV can serve as a signature of a small cluster (n ≤ 19). Focusing on small n-atom clusters (n ≤ 19), the existence of divergence observed in that curves may be because the cluster sizes (3–4 Å) are close to the lattice parameter of Cu (3.61 Å). Bazin and Rehe [11] have shown that the 13-atom cluster environment is not enough to produce the resonance of the second peak, and the same approach was made for a55-copper-atom cluster, where the authors considered that the features of copper K-edge are already present but with lower intensities because the surface atoms have a nonisotropic environment. It is visible that all main details of the experimental spectrum can be seen already in a cluster of 79 atoms. The further addition of atoms results only on a minor variation of relative intensities of some peaks.
In Figure 3 the results of calculations of the Fe K-edge XANES in bcc iron for atomic clusters having different sizes are presented. From Figure 3 it can be observed that all main features of the XANES spectrum were reproduced already for a cluster containing 89 atoms. The addition of the next spheres of atoms does not result in significant changes in the spectrum. Like in Cu for small n-atoms clusters (n ≤ 27), the divergence observed in that curves may be because the cluster sizes (2.5–4.5 Å) are close to the lattice parameter of Fe (2.87 Å) or the previous interpretation of Bazin and Rehr [11] for copper could be applied here for iron.
The agreement obtained between theoretical and experimental data (Figures 1 and 2) shows that theoretical approach used in the present study works well and it is possible to apply this technique to obtain the information on local atomic structure Cu and Fe nanoparticles.
By using the EXAFS technique and fitting method for the first peak Fourier transform, it is possible to calculate the structural parameters N, R, and σ2 of the first coordination shell in all proposed models. The coordination number of atoms around the absorbing atom in cluster size from 3 to 9 Å has been calculated when fitting the models with experimental data of Cu bulk, as well as interatomic distance and Debye–Waller factor. When representing the relationship between the number of atoms and the three structural parameters, it find that, increasing the number of atoms leads to rapidly increase the structural parameters until we reach the size of 5 Å (43 atoms) for Cu, after that the values of these parameters are approximately stable, as shown in Figure 4.
This steadiness indicates that the form of clusters began approaching the form of face-centered cubic copper crystals and arrived at stability in this form. This finding proves what has previously been achieved in the XANES spectra that have started to stability after size 5 Å (43 atoms). Figure 5 represents the relationship between the size of clusters or number of atoms and the three structural parameters for Fe. Here also the increasing of the size cluster rapidly leads to increase the structural parameters until we reach the size of 6.3 Å (89 atoms); after that, the values of these parameters are approximately stable. This stability indicates that the form of clusters began approaching the form of the body-centered cubic structure of iron crystals and arrived at stability in this form. This finding proves what has previously been achieved in the XANES spectra that have started to stability after size 6.3 Å (89 atoms).
The angular momentum projected density of states (lDOS) and simulated XANES spectra of Cu cluster (135-atoms) and Fe cluster (51-atoms) are presented in Figures 5 and 6 respectively. The Figures display the density of states (s, p, and d) together with the absorption coefficient (xmu) of the normalized XANES spectra. In a metal, the Fermi level is inside one of more allowed bands. The band is partially occupied. In the semiconductor and insulating materials, the Fermi level is halfway between the conduction and valence bands [12]. The green line indicates the calculated local density of p states of the central copper and iron atoms in their clusters. It can be seen from Figure 6 that p-orbital contributions dominate the K absorption edge, and this is not the case for s-orbital where its maximum is observed before the edge. The absorption edge of Cu clusters almost reproduces the unoccupied band of p electrons. It is clear that the theoretically evaluated p-orbital in Figure 7 reproduces most details of the XANES particularly at the pre-edge feature of iron. The density of states for Fe appears to be due to excitation into the unoccupied p and d orbitals. Despite the 1 s→3 d transition is forbidden by dipole selection rules, the contribution of d-orbital is seen. May be the both of the 3 d + 4 p combining and the direct quadrupolar coupling is the cause for the appearing of the d-orbit [13].
Comparing to the size-dependent XANES calculation based on a multiple scattering (MS) calculation for n-atom model clusters, it was found that the experimental XANES data are well-reproduced by a model structure consisting of 79 copper atoms and 89 iron atoms for Cu and Fe respectively. Increase the size of the clusters affects the characteristics of the structure for both Cu and Fe clusters, which was observed in the different XANES spectra at different cluster sizes. The Cu K absorption edge is dominated by p-orbital contributions. The variation of the cluster sizes influences the structural parameters such as N, R, and σ2 as revealed from EXAFS results.
All authors declare no conflicts of interest in this paper.
[1] |
F. Pezzella, G. Morganti, G. Ciaschetti, A genetic algorithm for the flexible job-shop scheduling problem, Comput. Oper. Res., 35 (2008), 3202–3212. https://doi.org/10.1016/j.cor.2007.02.014 doi: 10.1016/j.cor.2007.02.014
![]() |
[2] |
Z. Shao, D. Pi, W. Shao, Hybrid enhanced discrete fruit fly optimization algorithm for scheduling blocking flow-shop in distributed environment, Expert Syst. Appl., 145 (2020), 113147. https://doi.org/10.1016/j.eswa.2019.113147 doi: 10.1016/j.eswa.2019.113147
![]() |
[3] |
J. Behnamian, S. Fatemi Ghomi, A survey of multi-factory scheduling, J. Intell. Manuf., 27 (2016), 231–249. https://doi.org/10.1007/s10845-014-0890-y doi: 10.1007/s10845-014-0890-y
![]() |
[4] |
M. Yazdani, S. Gohari, B. Naderi, Multi-factory parallel machine problems: Improved mathematical models and artificial bee colony algorithm, Comput. Ind. Eng., 81 (2015), 36–45. https://doi.org/10.1016/j.cie.2014.12.023 doi: 10.1016/j.cie.2014.12.023
![]() |
[5] |
C. Lu, Y. Huang, L. Meng, L. Gao, B. Zhang, J. Zhou, A pareto-based collaborative multi-objective optimization algorithm for energy-efficient scheduling of distributed permutation flow-shop with limited buffers, Rob. Comput. Integr. Manuf., 74 (2022), 102277. https://doi.org/10.1016/j.rcim.2021.102277 doi: 10.1016/j.rcim.2021.102277
![]() |
[6] |
T. Meng, Q. K. Pan, L. Wang, A distributed permutation flowshop scheduling problem with the customer order constraint, Knowl.-Based Syst., 184 (2019), 104894. https://doi.org/10.1016/j.knosys.2019.104894 doi: 10.1016/j.knosys.2019.104894
![]() |
[7] |
S. Hatami, R. Ruiz, C. Andres-Romano, The distributed assembly permutation flowshop scheduling problem, Int. J. Prod. Res., 51 (2013), 5292–5308. https://doi.org/10.1080/00207543.2013.807955 doi: 10.1080/00207543.2013.807955
![]() |
[8] |
F. Xiong, M. Chu, Z. Li, Y. Du, L. Wang, Just-in-time scheduling for a distributed concrete precast flow shop system, Comput. Oper. Res., 129 (2021), 105204. https://doi.org/10.1016/j.cor.2020.105204 doi: 10.1016/j.cor.2020.105204
![]() |
[9] | M. Gen, R. Cheng, L. Lin, Network models and optimization: Multiobjective genetic algorithm approach, Springer Science & Business Media, 2008. |
[10] | B. Jiao, S. Yan, A niche sharing scheme-based co-evolutionary particle swarm optimization algorithm for flow shop scheduling problem, Syst. Cybernet. Inf., 15 (2017), 46–54. |
[11] | X. Yu, M. Gen, Introduction to Evolutionary Algorithms, Springer Science & Business Media, 2010. |
[12] |
J. Gao, R. Chen, W. Deng, An efficient tabu search algorithm for the distributed permutation flowshop scheduling problem, Int. J. Prod. Res., 51 (2013), 641–651. https://doi.org/10.1080/00207543.2011.644819 doi: 10.1080/00207543.2011.644819
![]() |
[13] |
S. y. Wang, L. Wang, M. Liu, Y. Xu, An effective estimation of distribution algorithm for solving the distributed permutation flow-shop scheduling problem, Int. J. Prod. Econ., 145 (2013), 387–396. https://doi.org/10.1016/j.ijpe.2013.05.004 doi: 10.1016/j.ijpe.2013.05.004
![]() |
[14] |
Y. Xu, L. Wang, S. Wang, M. Liu, An effective hybrid immune algorithm for solving the distributed permutation flow-shop scheduling problem, Eng. Optim., 46 (2014), 1269–1283. https://doi.org/10.1080/0305215X.2013.827673 doi: 10.1080/0305215X.2013.827673
![]() |
[15] |
B. Naderi, R. Ruiz, A scatter search algorithm for the distributed permutation flowshop scheduling problem, Eur. J. Oper. Res., 239 (2014), 323–334. https://doi.org/10.1016/j.ejor.2014.05.024 doi: 10.1016/j.ejor.2014.05.024
![]() |
[16] |
H. Bargaoui, O. B. Driss, K. Ghédira, A novel chemical reaction optimization for the distributed permutation flowshop scheduling problem with makespan criterion, Comput. Ind. Eng., 111 (2017), 239–250. https://doi.org/10.1016/j.cie.2017.07.020 doi: 10.1016/j.cie.2017.07.020
![]() |
[17] |
R. Ruiz, Q. K. Pan, B. Naderi, Iterated greedy methods for the distributed permutation flowshop scheduling problem, Omega, 83 (2019), 213–222. https://doi.org/10.1016/j.omega.2018.03.004 doi: 10.1016/j.omega.2018.03.004
![]() |
[18] | A. Kaveh, T. Bakhshpoori, Artificial bee colony algorithm, in Metaheuristics: Outlines, MATLAB Codes and Examples, Springer, 2019, 19–30. https://doi.org/10.1007/978-3-030-04067-3_3 |
[19] |
F. Zhao, H. Liu, Y. Zhang, W. Ma, C. Zhang, A discrete water wave optimization algorithm for no-wait flow shop scheduling problem, Expert Syst. Appl., 91 (2018), 347–363. https://doi.org/10.1016/j.eswa.2017.09.028 doi: 10.1016/j.eswa.2017.09.028
![]() |
[20] |
J. f. Chen, L. Wang, Z. p. Peng, A collaborative optimization algorithm for energy-efficient multi-objective distributed no-idle flow-shop scheduling, Swarm Evol. Comput., 50 (2019), 100557. https://doi.org/10.1016/j.swevo.2019.100557 doi: 10.1016/j.swevo.2019.100557
![]() |
[21] |
V. Fernandez-Viagas, P. Perez-Gonzalez, J. M. Framinan, The distributed permutation flow shop to minimise the total flowtime, Comput. Ind. Eng., 118 (2018), 464–477. https://doi.org/10.1016/j.cie.2018.03.014 doi: 10.1016/j.cie.2018.03.014
![]() |
[22] |
W. Shao, Z. Shao, D. Pi, Multi-objective evolutionary algorithm based on multiple neighborhoods local search for multi-objective distributed hybrid flow shop scheduling problem, Expert Syst. Appl., 183 (2021), 115453. https://doi.org/10.1016/j.eswa.2021.115453 doi: 10.1016/j.eswa.2021.115453
![]() |
[23] | Y. Del Valle, G. K. Venayagamoorthy, S. Mohagheghi, J. C. Hernandez, R. G. Harley, Particle swarm optimization: basic concepts, variants and applications in power systems, IEEE Trans. Evol. Comput., 12 (2008), 171–195. |
[24] | J. Kennedy, R. C. Eberhart, A discrete binary version of the particle swarm algorithm, in 1997 IEEE International Conference on Systems, Man, and Cybernetics. Computational Cybernetics and Simulation, 5 (1997), 4104–4108. https://doi.org/10.1109/ICSMC.1997.637339 |
[25] |
T. Jamrus, C. F. Chien, M. Gen, K. Sethanan, Hybrid particle swarm optimization combined with genetic operators for flexible job-shop scheduling under uncertain processing time for semiconductor manufacturing, IEEE Trans. Semicond. Manuf., 31 (2017), 32–41. https://doi.org/10.1109/TSM.2017.2758380 doi: 10.1109/TSM.2017.2758380
![]() |
[26] |
T. Jamrus, C. F. Chien, M. Gen, K. Sethanan, Multistage production distribution under uncertain demands with integrated discrete particle swarm optimization and extended priority-based hybrid genetic algorithm, Fuzzy Optim. Decis. Making, 14 (2015), 265–287. https://doi.org/10.1007/s10700-014-9200-6 doi: 10.1007/s10700-014-9200-6
![]() |
[27] |
C. Moon*, J. Kim, M. Gen, Advanced planning and scheduling based on precedence and resource constraints for e-plant chains, Int. J. Prod. Res., 42 (2004), 2941–2955. https://doi.org/10.1080/00207540410001691956 doi: 10.1080/00207540410001691956
![]() |
[28] |
A. Okamoto, M. Gen, M. Sugawara, Integrated data structure and scheduling approach for manufacturing and transportation using hybrid genetic algorithm, J. Intell. Manuf., 17 (2006), 411–421. https://doi.org/10.1007/s10845-005-0014-9 doi: 10.1007/s10845-005-0014-9
![]() |
[29] | R. Storn, K. Price, Differential evolution–a simple and efficient heuristic for global optimization over continuous spaces, J. Global Optim., 11 (1997), 341–359. |
[30] |
E. Jiang, L. Wang, J. Wang, Decomposition-based multi-objective optimization for energy-aware distributed hybrid flow shop scheduling with multiprocessor tasks, Tsinghua Sci. Technol., 26 (2021), 646–663. https://doi.org/10.26599/TST.2021.9010007 doi: 10.26599/TST.2021.9010007
![]() |
[31] |
C. A. C. Coello, G. T. Pulido, M. S. Lechuga, Handling multiple objectives with particle swarm optimization, IEEE Trans. Evol. Comput., 8 (2004), 256–279. https://doi.org/10.1109/TEVC.2004.826067 doi: 10.1109/TEVC.2004.826067
![]() |
[32] |
B. Naderi, R. Ruiz, The distributed permutation flowshop scheduling problem, Comput. Oper. Res., 37 (2010), 754–768. https://doi.org/10.1016/j.cor.2009.06.019 doi: 10.1016/j.cor.2009.06.019
![]() |
[33] |
J. J. Wang, L. Wang, A knowledge-based cooperative algorithm for energy-efficient scheduling of distributed flow-shop, IEEE Trans. Syst. Man Cybern.: Syst., 50 (2018), 1805–1819. https://doi.org/10.1109/TSMC.2017.2788879 doi: 10.1109/TSMC.2017.2788879
![]() |
[34] |
A. P. Rifai, H. T. Nguyen, S. Z. M. Dawal, Multi-objective adaptive large neighborhood search for distributed reentrant permutation flow shop scheduling, Appl. Soft Comput., 40 (2016), 42–57. https://doi.org/10.1016/j.asoc.2015.11.034 doi: 10.1016/j.asoc.2015.11.034
![]() |
[35] |
J. Deng, L. Wang, A competitive memetic algorithm for multi-objective distributed permutation flow shop scheduling problem, Swarm Evol. Comput., 32 (2017), 121–131. https://doi.org/10.1016/j.swevo.2016.06.002 doi: 10.1016/j.swevo.2016.06.002
![]() |
[36] |
C. Lu, L. Gao, J. Yi, X. Li, Energy-efficient scheduling of distributed flow shop with heterogeneous factories: A real-world case from automobile industry in china, IEEE Trans. Ind. Inf., 17 (2020), 6687–6696. https://doi.org/10.1109/TII.2020.3043734 doi: 10.1109/TII.2020.3043734
![]() |
[37] |
S. W. Lin, K. C. Ying, Minimizing makespan for solving the distributed no-wait flowshop scheduling problem, Comput. Ind. Eng., 99 (2016), 202–209. https://doi.org/10.1016/j.cie.2016.07.027 doi: 10.1016/j.cie.2016.07.027
![]() |
[38] |
K. C. Ying, S. W. Lin, C. Y. Cheng, C. D. He, Iterated reference greedy algorithm for solving distributed no-idle permutation flowshop scheduling problems, Comput. Ind. Eng., 110 (2017), 413–423. https://doi.org/10.1016/j.cie.2017.06.025 doi: 10.1016/j.cie.2017.06.025
![]() |
[39] |
C. Y. Cheng, K. C. Ying, H. H. Chen, H. S. Lu, Minimising makespan in distributed mixed no-idle flowshops, Int. J. Prod. Res., 57 (2019), 48–60. https://doi.org/10.1080/00207543.2018.1457812 doi: 10.1080/00207543.2018.1457812
![]() |
[40] |
G. Zhang, K. Xing, Differential evolution metaheuristics for distributed limited-buffer flowshop scheduling with makespan criterion, Comput. Oper. Res., 108 (2019), 33–43. https://doi.org/10.1016/j.cor.2019.04.002 doi: 10.1016/j.cor.2019.04.002
![]() |
[41] |
F. Zhao, X. He, L. Wang, A two-stage cooperative evolutionary algorithm with problem-specific knowledge for energy-efficient scheduling of no-wait flow-shop problem, IEEE Trans. Cybern., 51 (2020), 5291–5303. https://doi.org/10.1109/TCYB.2020.3025662 doi: 10.1109/TCYB.2020.3025662
![]() |
[42] |
F. Zhao, L. Zhang, J. Cao, J. Tang, A cooperative water wave optimization algorithm with reinforcement learning for the distributed assembly no-idle flowshop scheduling problem, Comput. Ind. Eng., 153 (2021), 107082. https://doi.org/10.1016/j.cie.2020.107082 doi: 10.1016/j.cie.2020.107082
![]() |
[43] |
F. Zhao, R. Ma, L. Wang, A self-learning discrete jaya algorithm for multiobjective energy-efficient distributed no-idle flow-shop scheduling problem in heterogeneous factory system, IEEE Trans. Cybern., 2021. https://doi.org/10.1109/TCYB.2021.3086181 doi: 10.1109/TCYB.2021.3086181
![]() |
[44] |
H. Jia, J. Y. Fuh, A. Y. Nee, Y. Zhang, Web-based multi-functional scheduling system for a distributed manufacturing environment, Concurrent Eng., 10 (2002), 27–39. https://doi.org/10.1177/1063293X02010001054 doi: 10.1177/1063293X02010001054
![]() |
[45] | X. Zhang, X. T. Li, M. H. Yin, An enhanced genetic algorithm for the distributed assembly permutation flowshop scheduling problem, Int. J. Bio-Inspired Comput., 15 (2020), 113–124. |
[46] | J. J. Wang, L. Wang, An iterated greedy algorithm for distributed hybrid flowshop scheduling problem with total tardiness minimization, in 2019 IEEE 15th International Conference on Automation Science and Engineering (CASE), IEEE, (2019), 350–355. https://doi.org/10.1109/COASE.2019.8842885 |
[47] |
M. F. Tasgetiren, Y. C. Liang, M. Sevkli, G. Gencyilmaz, A particle swarm optimization algorithm for makespan and total flowtime minimization in the permutation flowshop sequencing problem, Eur. J. Oper. Res., 177 (2007), 1930–1947. https://doi.org/10.1016/j.ejor.2005.12.024 doi: 10.1016/j.ejor.2005.12.024
![]() |
[48] |
Q. K. Pan, M. F. Tasgetiren, Y. C. Liang, A discrete particle swarm optimization algorithm for the no-wait flowshop scheduling problem, Comput. Oper. Res., 35 (2008), 2807–2839. https://doi.org/10.1016/j.cor.2006.12.030 doi: 10.1016/j.cor.2006.12.030
![]() |
[49] |
A. Rahimi-Vahed, S. Mirghorbani, A multi-objective particle swarm for a flow shop scheduling problem, J. Comb. Optim., 13 (2007), 79–102. https://doi.org/10.1007/s10878-006-9015-7 doi: 10.1007/s10878-006-9015-7
![]() |
[50] |
Y. X. Su, R. Chi, Multi-objective particle swarm-differential evolution algorithm, Neural Comput. Appl., 28 (2017), 407–418. https://doi.org/10.1007/s00521-015-2073-y doi: 10.1007/s00521-015-2073-y
![]() |
[51] |
Z. Cui, J. Zhang, D. Wu, X. Cai, H. Wang, W. Zhang, et al., Hybrid many-objective particle swarm optimization algorithm for green coal production problem, Inf. Sci., 518 (2020), 256–271. https://doi.org/10.1016/j.ins.2020.01.018 doi: 10.1016/j.ins.2020.01.018
![]() |
[52] |
W. Zhang, W. Hou, C. Li, W. Yang, M. Gen, Multidirection update-based multiobjective particle swarm optimization for mixed no-idle flow-shop scheduling problem, Complex Syst. Modell. Simul., 1 (2021), 176–197. https://doi.org/10.23919/CSMS.2021.0017 doi: 10.23919/CSMS.2021.0017
![]() |
[53] |
J. Q. Li, M. X. Song, L. Wang, P. Y. Duan, Y. Y. Han, H. Y. Sang, et al., Hybrid artificial bee colony algorithm for a parallel batching distributed flow-shop problem with deteriorating jobs, IEEE Trans. Cybern., 50 (2019), 2425–2439. https://doi.org/10.1109/TCYB.2019.2943606 doi: 10.1109/TCYB.2019.2943606
![]() |
[54] |
Q. K. Pan, L. Gao, L. Wang, J. Liang, X. Y. Li, Effective heuristics and metaheuristics to minimize total flowtime for the distributed permutation flowshop problem, Expert Syst. Appl., 124 (2019), 309–324. https://doi.org/10.1016/j.eswa.2019.01.062 doi: 10.1016/j.eswa.2019.01.062
![]() |
[55] |
J. P. Huang, Q. K. Pan, Z. H. Miao, L. Gao, Effective constructive heuristics and discrete bee colony optimization for distributed flowshop with setup times, Eng. Appl. Artif. Intell., 97 (2021), 104016. https://doi.org/10.1016/j.engappai.2020.104016 doi: 10.1016/j.engappai.2020.104016
![]() |
[56] |
M. E. Baysal, A. Sarucan, K. Büyüközkan, O. Engin, Artificial bee colony algorithm for solving multi-objective distributed fuzzy permutation flow shop problem, J. Intell. Fuzzy Syst., (2022), 1–11. https://doi.org/10.3233/JIFS-219202 doi: 10.3233/JIFS-219202
![]() |
[57] |
W. L. Liu, Y. J. Gong, W. N. Chen, Z. Liu, H. Wang, J. Zhang, Coordinated charging scheduling of electric vehicles: A mixed-variable differential evolution approach, IEEE Trans. Intell. Transp. Syst., 21 (2019), 5094–5109. https://doi.org/10.1109/TITS.2019.2948596 doi: 10.1109/TITS.2019.2948596
![]() |
[58] |
S. Zhou, L. Xing, X. Zheng, N. Du, L. Wang, Q. Zhang, A self-adaptive differential evolution algorithm for scheduling a single batch-processing machine with arbitrary job sizes and release times, IEEE Trans. Cybern., 51 (2019), 1430–1442. https://doi.org/10.1109/TCYB.2019.2939219 doi: 10.1109/TCYB.2019.2939219
![]() |
[59] |
W. Zhang, Y. Wang, Y. Yang, M. Gen, Hybrid multiobjective evolutionary algorithm based on differential evolution for flow shop scheduling problems, Comput. Ind. Eng., 130 (2019), 661–670. https://doi.org/10.1016/j.cie.2019.03.019 doi: 10.1016/j.cie.2019.03.019
![]() |
[60] |
Y. Y. Han, D. Gong, X. Sun, A discrete artificial bee colony algorithm incorporating differential evolution for the flow-shop scheduling problem with blocking, Eng. Optim., 47 (2015), 927–946. https://doi.org/10.1080/0305215X.2014.928817 doi: 10.1080/0305215X.2014.928817
![]() |
[61] |
W. Zhang, M. Gen, J. Jo, Hybrid sampling strategy-based multiobjective evolutionary algorithm for process planning and scheduling problem, J. Intell. Manuf., 25 (2014), 881–897. https://doi.org/10.1007/s10845-013-0814-2 doi: 10.1007/s10845-013-0814-2
![]() |
[62] |
Y. Li, C. Wang, L. Gao, Y. Song, X. Li, An improved simulated annealing algorithm based on residual network for permutation flow shop scheduling, Complex Intell. Syst., 7 (2021), 1173–1183. https://doi.org/10.1007/s40747-020-00205-9 doi: 10.1007/s40747-020-00205-9
![]() |
[63] | M. Baioletti, A. Milani, V. Santucci, Algebraic particle swarm optimization for the permutations search space, in 2017 IEEE Congress on Evolutionary Computation (CEC), IEEE, (2017), 1587–1594. https://doi.org/10.1109/CEC.2017.7969492 |
[64] |
E. Taillard, Benchmarks for basic scheduling problems, Eur. J. Oper. Res., 64 (1993), 278–285. https://doi.org/10.1016/0377-2217(93)90182-M doi: 10.1016/0377-2217(93)90182-M
![]() |
[65] |
A. P. Rifai, S. T. W. Mara, A. Sudiarso, Multi-objective distributed reentrant permutation flow shop scheduling with sequence-dependent setup time, Expert Syst. Appl., 183 (2021), 115339. https://doi.org/10.1016/j.eswa.2021.115339 doi: 10.1016/j.eswa.2021.115339
![]() |
[66] |
G. Wang, X. Li, L. Gao, P. Li, An effective multi-objective whale swarm algorithm for energy-efficient scheduling of distributed welding flow shop, Ann. Oper. Res., 310 (2022), 223–255. https://doi.org/10.1007/s10479-021-03952-1 doi: 10.1007/s10479-021-03952-1
![]() |
[67] |
S. Yang, Z. Xu, The distributed assembly permutation flowshop scheduling problem with flexible assembly and batch delivery, Int. J. Prod. Res., 59 (2021), 4053–4071. https://doi.org/10.1080/00207543.2020.1757174 doi: 10.1080/00207543.2020.1757174
![]() |
[68] | J. Derrac, S. García, D. Molina, F. Herrera, A practical tutorial on the use of nonparametric statistical tests as a methodology for comparing evolutionary and swarm intelligence algorithms, Swarm Evol. Comput., 1 (2011), 3–18. |