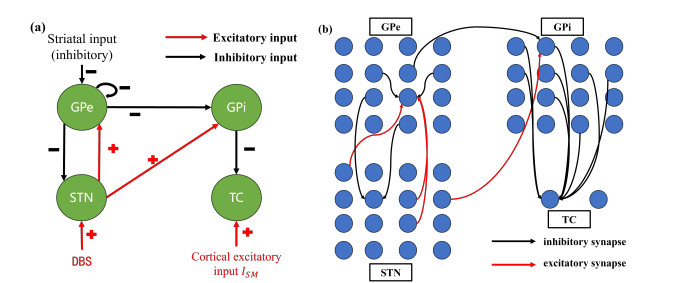
This research presents a novel optimization modeling framework for the existing Soil and Water Assessment Tool (SWAT), which can be used to optimize perennial feedstock production. This novel multi-objective evolutionary algorithm (MOEA) uses SWAT outputs to determine optimal spatial placement of variant cropping systems, considering environmental impacts from land-cover change and management practices. The final solution to the multi-objective problem is presented as a set of Pareto optimal solutions, where one is suggested considering the proximity to the ideal vector [1,0,0,0]. This unique approach provides a well-suited method to assist researchers and stakeholders in understanding the environmental impacts when cultivating biofuel feedstocks. The application of the proposed MOEA is illustrated by analyzing SWAT's example data set for Lake Fork Watershed. Nine land-cover scenarios were evaluated in SWAT to determine their optimal spatial placement considering maximizing biomass production while minimizing sediment yield, organic nitrogen yield, and organic phosphorous yield.
Citation: Ana Cram, Jose Espiritu, Heidi Taboada, Delia J. Valles-Rosales, Young Ho Park, Efren Delgado, Jianzhong Su. Multi-objective biofuel feedstock optimization considering different land-cover scenarios and watershed impacts[J]. Clean Technologies and Recycling, 2022, 2(2): 103-118. doi: 10.3934/ctr.2022006
[1] | Zilu Cao, Lin Du, Honghui Zhang, Lianghui Qu, Luyao Yan, Zichen Deng . Firing activities and magnetic stimulation effects in a Cortico-basal ganglia-thalamus neural network. Electronic Research Archive, 2022, 30(6): 2054-2074. doi: 10.3934/era.2022104 |
[2] | Honghui Zhang, Yuzhi Zhao, Zhuan Shen, Fangyue Chen, Zilu Cao, Wenxuan Shan . Control analysis of optogenetics and deep brain stimulation targeting basal ganglia for Parkinson's disease. Electronic Research Archive, 2022, 30(6): 2263-2282. doi: 10.3934/era.2022115 |
[3] | Xiaofang Jiang, Hui Zhou, Feifei Wang, Bingxin Zheng, Bo Lu . Bifurcation analysis on the reduced dopamine neuronal model. Electronic Research Archive, 2024, 32(7): 4237-4254. doi: 10.3934/era.2024191 |
[4] | Bo Lu, Xiaofang Jiang . Reduced and bifurcation analysis of intrinsically bursting neuron model. Electronic Research Archive, 2023, 31(10): 5928-5945. doi: 10.3934/era.2023301 |
[5] | Xia Shi, Ziheng Zhang . Multiple-site deep brain stimulation with delayed rectangular waveforms for Parkinson's disease. Electronic Research Archive, 2021, 29(5): 3471-3487. doi: 10.3934/era.2021048 |
[6] | Xiaolong Tan, Hudong Zhang, Yan Xie, Yuan Chai . Electromagnetic radiation and electrical stimulation controls of absence seizures in a coupled reduced corticothalamic model. Electronic Research Archive, 2023, 31(1): 58-74. doi: 10.3934/era.2023004 |
[7] | Qixiang Wen, Shenquan Liu, Bo Lu . Firing patterns and bifurcation analysis of neurons under electromagnetic induction. Electronic Research Archive, 2021, 29(5): 3205-3226. doi: 10.3934/era.2021034 |
[8] | Danqi Feng, Yu Chen, Quanbao Ji . Contribution of a Ca2+-activated K+ channel to neuronal bursting activities in the Chay model. Electronic Research Archive, 2023, 31(12): 7544-7555. doi: 10.3934/era.2023380 |
[9] | Xueyong Zhou, Xiangyun Shi . Stability analysis and backward bifurcation on an SEIQR epidemic model with nonlinear innate immunity. Electronic Research Archive, 2022, 30(9): 3481-3508. doi: 10.3934/era.2022178 |
[10] | Yuzhi Zhao, Honghui Zhang, Zilu Cao . The important role of astrocytes in activity pattern transition of the subthalamopallidal network related to Parkinson's disease. Electronic Research Archive, 2024, 32(6): 4108-4128. doi: 10.3934/era.2024185 |
This research presents a novel optimization modeling framework for the existing Soil and Water Assessment Tool (SWAT), which can be used to optimize perennial feedstock production. This novel multi-objective evolutionary algorithm (MOEA) uses SWAT outputs to determine optimal spatial placement of variant cropping systems, considering environmental impacts from land-cover change and management practices. The final solution to the multi-objective problem is presented as a set of Pareto optimal solutions, where one is suggested considering the proximity to the ideal vector [1,0,0,0]. This unique approach provides a well-suited method to assist researchers and stakeholders in understanding the environmental impacts when cultivating biofuel feedstocks. The application of the proposed MOEA is illustrated by analyzing SWAT's example data set for Lake Fork Watershed. Nine land-cover scenarios were evaluated in SWAT to determine their optimal spatial placement considering maximizing biomass production while minimizing sediment yield, organic nitrogen yield, and organic phosphorous yield.
Parkinson's disease (PD) is a common neurodegenerative disease caused by a dopamine deficiency in the substantia nigra of the midbrain[1,2]. It has been found that dopamine deficiency affects the electrical activity of the striatum, which, in turn, affects the electrical activity of the network composed of the basal ganglia (BG) and the thalamic (TC) neuron. In addition to pharmacological and surgical treatments[3], deep brain stimulation (DBS), which modulates the electrical activity of the basal ganglia through high-frequency electrical stimulation[4], is also an important therapeutic tool [5,6]. Identifying the complex dynamics of electrical activities of the thalamic and basal ganglia networks are important for understanding PD and DBS[7].
A network model consisting of the basal ganglia (including four nuclei: subthalamic nucleus (STN), the globus pallidus (GPe), the internal segment of the globus pallidus (GPi), and the striatum), TC and cortex has been widely used to study PD [8,9], as shown in Figure 1. The "+" represents the excitatory effect and the "-" represents the inhibitory effect. The effect of the striatum is treated as a constant negative current acting on the GPe, and the cortical effect on the thalamus is modeled as a periodic positive pulse current. In the normal state, the thalamus faithfully responds to incoming sensorimotor signals (pulse stimulations) from the cortex, that is, each sensorimotor signal induces one action potential of the thalamus, which turns into a PD state when the thalamus is no longer able to faithfully relay the sensorimotor input [10]. For example, some sensorimotor signals induce bursting of the thalamus. When DBS is applied to the STN, its subsequent firing will be enhanced. The increased firing rate of the STN will lead to an increase in GPe firing and a decrease in GPi firing. Consequently, this reduction in the inhibitory effect from the GPi to the TC will result in the cessation of thalamic bursting and the restoration of firing corresponding to pulse stimulation. This signifies the therapeutic effect in the context of PD. Although recent studies have begun to model the striatum and cortex to study β-oscillations related to PD[11], thalamic firing, especially bursting, remains an important indicator of PD. It is well known that bursting in the nervous system is associated with numerous brain functions and brain disorders[12,13,14,15]. In general, the generation of bursting involves complex fast-slow variables and numerous bifurcations, which are regulated by multiple factors [16,17,18,19,20]. Therefore, revealing the mechanism of bursting generation in the thalamus is important for understanding and treating of PD.
On one hand, thalamic bursting can be induced by positive impulse stimulation, which is in line with conventional knowledge that positive impulse stimulation enhances firing [21]. On the other hand, thalamic bursting could also be induced by inhibitory synaptic currents from GPi via the post inhibitory rebound (PIR) mechanism. PIR is a paradoxical phenomenon in which inhibitory pulse stimulation induces firing associated with a variety of brain functions or disorders[22,23,24,25,26,27]. Theoretically, PIR is related to the Hopf bifurcation and the saddle-node bifurcation on an invariant cycle. In neurophysiology, PIR is related to the either T-type calcium current or hyperpolarization-activated cation current. Experimentally, TC neurons have been found to produce bursting after receiving inhibitory currents[28] and is mediated by T-type calcium currents[29]. In addition to the PIR, recent experimental and theoretical studies have found many other paradoxical phenomena such as the enhancement of bursting induced by inhibitory effects and the reduction of bursting induced by excitatory effects [18,30,31,32,33,34]. Furthermore, the intricate bifurcations of these paradoxical bursts are uncovered by dissecting the fast and slow variables[16,35,36,37]. This provides novel theoretical insights into the bursting phenomenon and its modulation mechanisms. To date, there are relatively few studies on the fast-slow variable dissection for multiple types of bursting in mathematical models of the thalamic neurons, and there are few analyses of the fast-slow variable dissection variables for PIR bursting. Consequently, it becomes crucial to investigate whether the bursting of thalamic neurons is triggered by positive impulses, is induced by the PIR mechanism, or is associated with complex bifurcations. Such investigations hold great significance in deepening our understanding of PD and exploring effective therapeutic methodologies.
In this paper, for the model of the coupled system shown in Figure 1(a), we simulated the firing activities within the network level corresponding to PD and DBS treatments. By combining the bifurcations of isolated thalamic neurons, we found that the occurrence of thalamic bursting in relation to PD is not triggered by PIR but is induced by inhibitory synaptic currents. This finding challenges the conventional belief that negative currents suppress neuronal firing. Furthermore, our study also examined the responses of isolated neurons and those receiving different types of inhibitory and excitatory synaptic currents within the neural network, as well as the dissection of fast-slow variables. The inhibitory synaptic current of the GPi in the network to the thalamic neurons was the reason for the paradoxical bursting of TC neurons in the network. After the stimulation of the STN by DBS, the inhibitory synaptic current disappeared through the network dynamics, which led to the disappearance of the abnormal bursting of TC neurons. Moreover, the fast-slow dynamics and the bifurcation mechanism of the paradoxical bursting in the TC neurons were further revealed. The results of this study revealed the dynamics of the paradoxical bursting of thalamic neurons and provided a new viewpoint on the thalamic bursting associated with PD, which can help to understand the treatment of PD and DBS.
The neuron and network models used in this paper are from the literature[9], which have been widely used to study electrical activity associated with PD.
The thalamic neuron is modeled as follows:
CdVdt=∑TCI=−IL−INa−IK−IT+ISM+Iapp | (2.1a) |
dhdt=h∞(V)−h(V)τh(V) | (2.1b) |
drdt=r∞(V)−r(V)τr(V) | (2.1c) |
The three variables are membrane potential V, the inactivation variable for sodium channel h, and the inactivation gating variable related to the low threshold calcium current r. IL=gL(V−EL), INa=gNam3∞h(V−ENa), IK=gK(0.75(1−h)4)(V−EK) and IT=gTp2∞r(V−ET) represent the leakage current, the sodium current, the potassium current, and the low-threshold T-calcium current, respectively. ISM represents sensorimotor input current from the cortex, and Iapp is an external constant current used to regulate the firing of neurons. C represents the membrane capacitance of the neuron. τh and τr represent the time constants of INa and IT, respectively. gL, gNa, gK and gT are the corresponding maximum conductances, and EL, ENa, EK and ET are the corresponding reversal potentials. The expressions for the functions m∞, h∞, p∞ and r∞ are m∞=11+exp(−V+377), h∞=11+exp(V+414), p∞=11+exp(−V+606.2) and r∞=11+exp(V+844), respectively. The time constants τr and τh are expressed as follows: τr=28+exp(−V+2510.5) and τh=10.128exp(−V+4618)+41+exp(−V+235).
The parameter values of the TC model are as follows: C=1μF/cm2, gL=0.05mS/cm2, EL=−70mV, gNa=3mS/cm2, ENa=50mV, gK=5mS/cm2, EK=−90mV, gT=5mS/cm2, ET=0andmV.
The subthalamic nucleus (STN) is modeled as follows:
dVdt=∑STNI=−IL−INa−IK−IT−ICa−IAHP+IDBS+Iapp | (2.2a) |
dndt=ϕnn∞(V)−n(V)τn(V) | (2.2b) |
dhdt=ϕhh∞(V)−h(V)τh(V) | (2.2c) |
drdt=ϕrr∞(V)−r(V)τr(V) | (2.2d) |
dCadt=ε(−ICa−IT−kCa⋅Ca) | (2.2e) |
There are five variables in this model: the voltage variable V, the activation variable of the potassium channel n, the activation variable of sodium channel h, the inactivation gating variable related to low threshold calcium currents r, and the calcium-activated potassium gating variable for the calcium-activated potassium current Ca. IL=gL(V−EL), INa=gNam3∞h(V−ENa), IK=gKn4(V−EK), IT=gTa3∞b2∞(V−ECa), ICa=gCas2∞(V−ECa), and IAHP=gAHP(V−EK)(Ca/(Ca+k1)) represent the leakage current, the sodium current, the potassium current, the low-threshold T-calcium current, the calcium current, and the calcium-activated potassium ion current, respectively. Iapp represents the external excitation current, usually expressed as a constant current, which is used to regulate the neuron's firing behavior; IDBS is the periodic current acting on the STN, which is used to change the neuron's firing frequency. C represents the capacitance of the neuron, τh, τn and τr represent the time constant of INa, IK and IT current, respectively. gL, gNa, gK, gT, gCa and gAHP are the corresponding maximum conductances, and EL, ENa, EK, and ECa are the corresponding reversal potentials. The functions m∞, n∞, a∞, s∞, h∞, r∞ and b∞ are expressed as m∞=11+exp(−V+3015), n∞=11+exp(−V+328), a∞=11+exp(−V+637.8), s∞=11+exp(−V+398), h∞=11+exp(V+393.1), r∞=11+exp(V+672) and b∞=11+exp(r−0.4−0.1)−11+exp(4), respectively. The time constant functions τh, τn, and τr are τh=1+5001+exp(V+573), τn=1+1001+exp(V+8026), and τr=40+17.51+exp(V−682.2), respectively.
The parameter values for the STN model are as follows: C=lpF/μm2, gL=2.25mS/cm2, gNa=37.5mS/cm2, gK=45mS/cm2, gT=0.5mS/cm2, gCa=0.5mS/cm2, gAHP=9mS/cm2, EL=−60mV, ENa=55mV, EK=−80mV, ECa=140mV, ϕn=0.75, ϕh=0.75, ϕr=0.2, ε=3.75×10−5, and k1=15, kCa=22.5.
The GPe and GPi models are identical, except for the external stimulus current Iapp. The model is as follows:
CdVdt=∑GPe/GPiI=−IL−INa−IK−IT−ICa−IAHP+Iapp | (2.3a) |
dndt=ϕnn∞(V)−n(V)τn(V) | (2.3b) |
dhdt=ϕhh∞(V)−h(V)τh(V) | (2.3c) |
drdt=ϕrr∞(V)−r(V)τr(V) | (2.3d) |
dCadt=ε(−ICa−IT−kCaCa) | (2.3e) |
The model has five variables: the membrane potential V, the activation variable of potassium channel n, the inactivation variable of sodium channel h, the inactivation gating variable related to low-threshold calcium current r, and the gating variable related to calcium-activated potassium current Ca. IL=gL(V−EL), INa=gNam3∞h(V−ENa), IK=gKn4(V−EK), IT=gTa3∞r(V−ECa), ICa=gCas2∞(V−ECa), and IAHP=gAHP(V−EK)(Ca/(Ca+k1)) represent the leakage current, the sodium current, the potassium current, the low-threshold T-calcium current, the calcium current, and the calcium-activated potassium current, respectively. Iapp represents the external excitation current used to modulate the neuronal firing behavior. To more closely match the experiment, the Iapp for the GPi is higher than the Iapp for the GPe. C represents the capacitance of the neuron, τh, τn, and τr represent the time constant of the INa, IK, and IT currents, respectively. gL, gNa, gK, gT, gCa, and gAHP are the corresponding maximum conductances, and EL, ENa, EK and ECa are the corresponding reversal potentials. The functions m∞, s∞, a∞, n∞, h∞ and r∞ have the following expressions: m∞=11+exp(−V+3710), n∞=11+exp(−V+5014), a∞=11+exp(−V+572), s∞=11+exp(−V+352), h∞=11+exp(V+5812), and r∞=11+exp(V+702). The time constants τh, τn, and τr are as follows: τh=0.05+0.271+exp(−V+4012), τn=0.05+0.271+exp(−V+4012), and τr=30.
The parameter values used for the GPe and GPi neuron models are as follows: C=lpF/μm2, gL=0.1mS/cm2, gNa=120mS/cm2, gK=30mS/cm2, gT=0.5mS/cm2, gCa=0.15mS/cm2, gAHP=30mS/cm2, EL=−55mV, ENa=55mV, EK=−80mV, ECa=120mV, ϕn=0.1, ϕh=0.05, ϕr=1, ε=1×10−4, k1=30, kCa=15, Iapp=2.2μA/cm2 in GPe neurons and Iapp=3μA/cm2 in GPi neurons. Because GPe receives inhibitory action from the striatum, the GPe is chosen to be Iapp=2.2μA/cm2, which is lower than the GPi.
The network model shown in Figure 1(a) contains 16 STN neurons, 16 GPe neurons, 16 GPi neurons, and two TC neurons. Each STN neuron received inhibitory inputs from 2 GPe neurons. Each GPe neuron received excitatory inputs from three STN neurons. Each GPi received excitatory inputs from one STN neuron and inhibitory inputs from two GPe neurons. Each TC neuron received inhibitory inputs from eight GPi neurons. Each GPe neuron received inhibitory inputs from two other GPe neurons, as shown in Figure 1(b).
CdVTCdt=∑TCI+IGPi→TC+ISM | (2.4a) |
CdVSTNdt=∑STNI+IGPe→STN+IDBS | (2.4b) |
CdVGPedt=∑GPeI+IGPe→GPe+ISTN→GPe | (2.4c) |
CdVGPidt=∑GPiI+IGPe→GPi+ISTN→GPi | (2.4d) |
VTC, VSTN, VGPe, and VGPi represent the membrane potentials of TC, STN, GPi, and GPe neurons, respectively. IGPi→TC represents the inhibitory synaptic currents received by the thalamus from GPi, and ISM represents the excitatory input from the cortex to thalamus. IGPe→STN represents the inhibitory synaptic current from the GPe to the STN, and IDBS represents the positive and high-frequency stimulation applied to the STN. IGPe→GPe represents the inhibitory synaptic current from the GPe neuron to the other GPe neurons, and ISTN→GPe represents the excitatory synaptic current from the STN to the GPe. IGPe→GPi represents the inhibitory synaptic current from the GPe to the GPi, and ISTN→GPi is excitatory synaptic current from the STN to the GPi. It should be noted that each model of the network should be given a subscript to show the number of neurons; however, the models with subscripts look more complex, the model descriptions in this paper are relatively simple to be easily understood.
The excitatory input from the cortex to the thalamus ISM is a periodic pulse current (see the second half of the red solid line in Figure 6), and the function is ISM=iSMH(sin(2πt/ρSM))×[1−H(sin(2π(t+δSM)/ρSM))], where H is the Heaviside function:
H(x)={0,x<00.5,x=01,x>1 |
ρSM, δSM, and iSM are the period, the duration, and the amplitude of the pulse, respectively.
IDBS is the high-frequency pulse current applied to the STN and the deep brain stimulation (see the second half of the red solid line in Figure 7(a)), and the function is IDBS=iDBSH(sin(2πt/ρDBS))×[1−H(sin(2π(t+δDBS)/ρDBS))], where H is the Heaviside function, ρDBS is the period of the pulse, δDBS is the pulse duration, and iDBS is the amplitude.
The synaptic current function is expressed as Iα→β=gα→β(Vβ−Eα→β)∑jsjα, where α represents a presynaptic neuron, β denotes a postsynaptic neuron, Iα→β represents the synaptic current from the presynaptic neuron α to the postsynaptic neuron β, gα→β is the maximum synaptic conductance, Vα is the membrane potential of the presynaptic neuron, Vβ is the membrane potential of the postsynaptic neuron, Eα→β is the inverse synaptic potential, j represents the number of presynaptic neurons and ∑jsjα corresponds to the sum of the gating variables of the j presynaptic neurons. The expression of the s function is as follows:
dsαdt=Aα(1−sα)H(Vα−θα)−Bαsα | (2.5a) |
H is the Heaviside function, Aα and Bα denote the rate of activation and deactivation of the synaptic currents, respectively, and θα is the synaptic threshold of the presynaptic neuron (a presynaptic neuron with a membrane potential higher than θα induce the synapse activated). The parameters of the synaptic variable sα and the synaptic current Iα→β in the network are shown in Tables 1 and 2.
Aα | Bα | θα |
AGPi=2 | BGPi=0.08 | θGPi=−20 |
AGPe=1 | BGPe=0.1 | θGPe=−20 |
ASTN=1 | BSTN=0.05 | θSTN=−30 |
Synaptic current (μA/cm2) | Conductance (mS/cm2) | Reversal potential (mV) |
IGPe→STN | gGPe→STN=0.9 | EGPe→STN=0.9 |
ISTN→GPe | gSTN→GPe=0.3 | ESTN→GPe=0.3 |
IGPe→GPe | gGPe→GPe=1 | EGPe→GPe=1 |
ISTN→GPi | gSTN→GPi=0.3 | ESTN→GPi=0.3 |
IGPe→GPi | gGPe→GPi=0.75 | EGPe→GPi=0.7 |
IGPi→TC | gGPi→TC=0.1 | EGPi→TC=0.1 |
In the thalamic neuron model, τr, which represents the time constant of variable r, is much larger than τh, and the time constant of the variable h. Then, r is the slow variable and Eqs (2.1a) and (2.1b) are the fast subsystems. First, bifurcations of the fast subsystem are obtained with respect to r. Second, the phase trajectory of the bursting of the whole system (Eqs (2.1a)–(2.1c)) with different Iapp values are superimposed on the bifurcations of the fast system. Finally, bifurcations related to the beginning and the ending phases of the bursting are identified.
In this paper, the fourth-order Runge-Kutta method is used to solve the equations with an integration step of 0.01 ms, and the bifurcation calculations are obtained through MATLAB and its MATCONT subroutine package software [38].
Using Iapp as the bifurcation parameter, the bifurcation of the TC neuron is obtained, as shown in Figure 2(a). The equilibrium curve is "S" shaped and divided into upper, middle, and lower branches. The lower branch consists of stable nodes (red solid line) and unstable foci (blue dashed line). With the increase of Iapp, the stable node of the lower branch loses its stability through a subcritical Hopf bifurcation (SubH1, Iapp≈−0.59969μA/cm2). The system undergoes an unstable focus, which then turns into a stable node again through a second subcritical Hopf bifurcation (SubH2, Iapp≈−0.10138μA/cm2). The middle branch consists of saddle points (magenta dashed line). The upper branch consists of the focus. As Iapp increases, the unstable focus (blue dashed line) is transformed into a stable focus (red solid line) by the supercritical Hopf bifurcation SupH (Iapp≈39.19564μA/cm2). The stable node of the lower branch meets the saddle point of the middle branch and disappears to form the saddle-node bifurcation SN1 (Iapp≈0.56239μA/cm2), which is located in the lower right of Figure 2(a). Additionally, the boundary between the upper and middle branches is the saddle-node bifurcation SN2 (Iapp≈−1.755587μA/cm2), which is located at the upper left of Figure 2(a). The stable limit cycle emerges via the SupH bifurcation on the upper branch, and between the SupH (Iapp≈39.19564μA/cm2) and Iapp≈0.32504μA/cm2; its maximum amplitudes and minimum amplitudes are indicated by the upper and lower green lines, denoted by Vmax and Vmin, respectively. The stable limit cycle corresponds to the firing. The two subcritical Hopf bifurcations in the lower branch, SubH1 and SubH2, give birth to two unstable limit cycles (green dashed lines).
Figure 2(b) is a magnification of Figure 2(a) near Iapp=0μA/cm2. At Iapp=0μA/cm2, there is a coexistence of the resting state and stable firing. The upper and lower purple curves indicate the maximum and minimum of the membrane potential corresponding to the firing, which is acquired through a numerical simulation. The parameter region for the coexisting firing is very narrow. Unfortunately, the bifurcation related to the firing and resting states has not been acquired. The electrical activity of the pre-coupled TC neurons studied in this paper at a resting state at Iapp=0μA/cm2.
Unstable limit cycles emerge from both SubH1 and SubH2, as shown by the green dashed curves in Figure 2(b). The unstable limit cycles encounter the saddles on the middle branch to disappear via a saddle-homoclinic orbit bifurcation. Then, an unstable equilibrium point appears between SubH1 and SubH2, as shown by the blue dashed curve on the lower branch. With a numerical simulation, a stable firing between Iapp≈−0.61674μA/cm2 and Iapp≈−0.102μA/cm2 (the upper and lower purple curves correspond to the maximum and minimum values respectively) is acquired. The firing is stable bursting, as shown in Figure 3. Unfortunately, the bifurcations related to the firing and resting states remain unclear. The firing corresponds to a type of paradoxical bursting. There are two reasons for calling it paradoxical bursting. The first reason is that the Iapp value of the bursting is lower than that of the resting state, thereby suggesting that the bursting is paradoxical, contrary to the traditional notion that an increase in Iapp induces a firing from the resting state. The second reason is that the number of spikes within a burst decreases as Iapp decreases, as shown in Figure 3(a)–(d). As Iapp decreases, the bursting exhibits a period-adding bifurcation, as shown by the interspike intervals (ISIs) in Figure 3(e). The correlation between this paradoxical bursting and the bursting of TC neurons in the network is explained in the following sections.
Figure 4(a) illustrates the bifurcation of the isolated STN neuron influenced by Iapp. The equilibrium curve presents an "S" shape comprised of the lower branch as stable nodes (solid red line), the middle branch as saddles (illustrated by a magenta dashed line), and the focus on the upper branch. As Iapp increases, the unstable focus is transformed into a stable focus (solid red line) by a subcritical Hopf bifurcation (SubH, Iapp≈151.51554μA/cm2). A saddle-node bifurcation on an invariant cycle (SNIC), occurs at Iapp≈−5.45555154μA/cm2(SNIC), via which the stable node from the lower branch meets the saddle from the middle branch. The boundary that separates the middle (saddle) from the upper branch corresponds to a saddle-node bifurcation (SN). This bifurcation occurs at approximately −33.83116μA/cm2 of Iapp.
As Iapp further increases, an unstable limit cycle emerges around the upper branch (maximum amplitude Vmax and minimum amplitude Vmin delineated by upper and lower dashed green lines, respectively) via the SubH bifurcation. Concurrently, a stable limit cycle (solid green lines) also emerges from the SNIC and intersects with the unstable limit cycle. This intersection point forms either a saddle-node or a fold bifurcation of the limit cycle(LPC, Iapp≈−205.01926μA/cm2).
Figure 4(b) shows the bifurcations of the GPe and GPi neurons with respect to Iapp, and the insert panel represents the global view of the bifurcation. The changes of the equilibrium curves are monotonical, which are divided into three segments by the subcritical Hopf bifurcation (SubH, Iapp≈−0.65538μA/cm2) and the supercritical Hopf bifurcation (SupH, Iapp≈603.4613μA/cm2, shown in the insert panel). The red solid curve represents the stable node and the blue dashed curve represents the unstable focus. As Iapp changes, the stable limit cycle (green solid line) induced by the SupH bifurcation intersects with the unstable limit cycle (green dashed line) caused by SubH to form the fold bifurcation of limit cycle (LPC, Iapp≈−0.44753μA/cm2).
According to the bifurcation diagrams, the neuronal firings before coupling are shown in Figure 5. Figure 5(a) shows the fast-spiking of the STN neuron (Iapp=25μA/cm2), Figure 5(b) shows the spiking of the GPe neuron (Iapp=2.2μA/cm2), Figure 5(c) shows the firing activity of the GPi neuron (Iapp=3μA/cm2), and Figure 5(d) shows the resting state of the TC neuron (Iapp=0μA/cm2). These firing activities are not far from the bifurcation point; therefore, it can be surmised that the complex firing can be evoked when subjected to oscillating synaptic current within the network. When the synaptic current is positive (excitatory), the firing is enhanced; when the synaptic current is negative (inhibitory), the firing is weakened or even stopped, and then bursting may be formed.
For the resting state (Iapp=0μA/cm2) of a TC neuron, after being stimulated with a periodic pulse current ISM (iSM=5μA/cm2, ρSM=50ms, δSM=5ms) from the cortex (t> 1500 ms), spiking induced by the stimulus pulses is evoked that corresponds to the normal state, as shown in Figure 6. The solid red represents the pulse stimulation. The spiking associated with the limit cycle is shown by the green solid lines in Figure 2(a), (b).
As shown in Figure 7(a), the STN neuron exhibits a fast-spiking state at Iapp=25μA/cm2 (t< 4400 ms). When a high-frequency periodic pulse current IDBS(red, iDBS=200μA/cm2, ρDBS=6ms, δDBS=0.6ms) is applied to stimulate the STN neuron at t = 4400 ms, the firing frequency becomes faster. Figure 7(b) is a magnification of Figure 7(a), after which corresponds high-frequency DBS stimulation, the firing frequency of the STN neuron becomes higher, which corresponds to the frequency of IDBS. Within the network, IDBS induced high-frequency spiking of the STN neuron to eliminate bursting of the TC neuron corresponding to PD, which will be investigated in Section 3.3.4.
A TC neuron at a resting state, receives a negative pulse of 100 ms for a duration of 1000 ms (red), and exhibits a PIR spike (black), as shown in Figure 8. A relatively weak (−0.195μA/cm2) negative pulse induces a PIR spike, as shown in Figure 8(a), and a relatively strong (−0.21μA/cm2) negative pulse induces a PIR burst, as shown in Figure 8(b). Obviously, the negative pulse first causes a decrease in the membrane potential; after the end of the stimulation, the membrane potential increases, thereby resulting in a PIR spike. An increase in the membrane potential after the end of the stimulation is caused by the activation of a positive T-calcium current (first decrease and then increase), which is induced by the decrease in membrane potential during the stimulation period, as shown by the green solid line in Figure 8. After the end of the stimulation, the positive T-calcium current continues to exist and increases, thus causing the membrane potential to rise before the spike or burst.
Therefore, the decrease in membrane potential induced by negative stimulation, which occurs before the PIR spike, is an important feature of the PIR spike. In this paper, we will use this to determine whether the bursting of TC neurons in the network is a PIR spike or not (see Section 3.3.2). The stronger the negative current stimulation is, the larger the positive T-calcium current is. The long decay time caused the burst with more spikes in it, as shown in Figure 8(b). Therefore, the slow-varying factor that determines the generation of bursting is the slow decay of the T-calcium current. Although the TC neurons have the capacity to produce a PIR spike, and TC neurons in the network are also influenced by an inhibitory synaptic current, this paper will reveal that the bursting of the TC neuron in the network corresponding to PD is not a PIR spike (see Figure 9(d), (e)), because the spike is not preceded by a negative pulse.
Before coupling, STN, GPe and GPi neurons are in spiking (Figure 5), and the TC neuron receiving the corresponding cortical stimulation ISM also exhibits spiking corresponding to the stimulation ISM (Figure 6). The firings of the coupled neurons are shown in Figure 9. The black solid curve represents the neuronal membrane potential, the red solid curve indicates the ISM stimulation received by the thalamic neuron, and the blue solid curve is the inhibitory synaptic current IGPi→TC from the GPi neuron to thalamic neuron.
Since the STN neuron mainly receives an inhibitory synaptic current from the GPe neuron, STN firing is suppressed to sparse firing, as shown in Figure 9(a). The inhibitory synaptic current is strong in the duration of no spiking (quiescent state). The GPe neuron receives two synaptic currents, which are the inhibitory current of other GPe neurons and the excitatory current of STN neurons. When the inhibitory current is stronger than the excitatory current, the spiking is suppressed to form a quiescent state; when the excitatory current is stronger than the inhibitory current, the firing is enhanced. Then, bursting with alternations between the quiescent state and firing appears, as shown in Figure 9(b). GPi neuron is subjected to an inhibitory synaptic current from the GPe neuron and the excitatory current from the STN neuron; similarly, bursting is generated, as shown in Figure 9(c).
In particular, the TC neuron receives an inhibitory synaptic current from the GPi neuron. Under this inhibitory synaptic current, the TC neuron no longer produces spiking in response to each spike of the input current ISM (red), as shown in Figure 9(d), (e) (Figure 9(e) is longer), with the inhibitory synaptic current in blue. At this time, TC neurons respond to the ISM pulse stimulation in three ways:
1) No action potential is generated, as shown in the first stimulation in Figure 9(e), which should be caused by the strong suppression of the inhibitory synaptic current at this time;
2) A Spike is still generated, as shown in the fifth stimulation in Figure 9(e), which is caused by the insufficiently strong inhibitory synaptic current at this time; and
3) Either a double-spike or multiple-spikes bursts are evoked, as depicted in the third stimulation in Figure 9(d), (e). The mechanism for the generation of either a double-spike or multiple-spike bursts is very complex, which has not yet been understood. This is the core of this paper, which is obtained by analyzing the joint action of the inhibitory synaptic current IGPi→TC and the cortical ISM.
The amplification of the bursting and inhibitory synaptic current in Figure 9(e) is shown in Figure 10(a). Corresponding to the action potential, the inhibitory synaptic current generates a large negative pulse, which is due to the expression of the synaptic current as IGPi→TC=gGPi→TC(VTC−EGPi→TC)∑jsjGPi. Therefore, when the postsynaptic membrane potential VTC (action potential of TC neuron) is higher, then a stronger synaptic current is received by the postsynaptic neuron, because the size of synaptic current is related to VTC. The fact is that the large negative synaptic current pulse does not precede the action potential; moreover, it indicates that the spikes are not caused by the negative synaptic current. On the contrary, the negative synaptic current pulse is caused by the action potential. Therefore, the bursting is not PIR bursting.
The blue and red colors in Figure 10(b) are the inhibitory synaptic current and the ISM stimulation, respectively. It can be found that, except for the large negative synaptic current pulse corresponding to the action potential, the blue pulse corresponding to a ISM below the black dashed line (−2μA/cm2), the inhibitory synaptic current between the neighboring red pulse is weaker than that the black dashed line, −2μA/cm2, for most of the time, as shown in Figure 10(b). Therefore, two speculations can be made. One is that the bursting of TC neurons should be generated by the combined effect of a relatively weak inhibitory synaptic current and a positive pulse ISM, which will be illustrated by the response of isolated TC neurons to an external negative stimulation and a positive pulse (see Section 3.3.2); and the other is the elimination of the inhibitory synaptic current can induce spiking of TC neurons in response to the ISM pulses recovered, which will be illustrated by the DBS stimulation to eliminate an inhibitory synaptic current to recover the spiking of TC neurons by removing the inhibitory synaptic current (see Section 3.3.4).
The results in Figure 11 corroborate that the bursting of the thalamic neuron within the network is induced by the combined action of an inhibitory synaptic current and an excitatory pulse current (iSM=5μA/cm2, ρSM=50ms, δSM=5ms). A thalamic neuron stimulated with Iapp=0μA/cm2 exhibits spiking, as shown in Figure 11(a). Currents of −1μA/cm2 and −1.3μA/cm2 are selected to simulate an inhibitory synaptic current weaker than −2μA/cm2. The introduction of a negative current of −1μA/cm2 (as shown by the blue solid line in Figure 11(b)) to Figure 11(a) induces bursting, as shown in Figure 11(b), and the introduction of a negative current of −1.3μA/cm2 (as shown by the blue solid line in Figure 11(c)) to Figure 11(a) also causes a bursting, as shown in Figure 11(c). The negative currents in Figure 11(b), (c) correspond to the inhibitory synaptic current in Figure 10(b). Therefore, the bursting of TC neurons that correspond to PD is induced by an inhibitory current. Considering that the paradoxical bursting in Figure 3 is also induced by a negative current, the bursting of TC neurons in the network that correspond to PD also correspond to the paradoxical bursting of TC neurons.
The results shown in Figure 12 confirm another speculation proposed in Section 3.3.1, that removing inhibitory synaptic currents can eliminate bursting of the TC neuron and restore the spiking corresponding to the pulses ISM (blue solid lines). For the state corresponding to PD shown in Figure 9, a DBS current is applied to each STN neuron in the network, as shown in Figure 12(a), with the black solid curve representing the neuron membrane potential and the red solid curve denoeting IDBS. Under the high-frequency stimulation IDBS, the STN neurons become high-frequency spiking, as shown in Figure 12(a), (e); Figure 12(e) is a magnification of Figure 12(a). The high spiking frequency of the STN neuron causes the excitatory effect from the STN neuron to the GPe neuron enhanced. Then, the GPe neuron's spiking enhances and the spiking frequency increases, as shown in Figure 12(b), (f); the magnification of Figure 12(b) is shown in Figure 12(f). Each GPi neuron receives an inhibitory effect from two GPe neurons and an excitatory effect from one STN neuron. Then, the inhibitory effect of the GPe neuron becomes strong, which makes the activities of the GPi neurons either weak or nonexistent to become resting state, as shown in Figure 12(c). Therefore, the inhibitory synaptic current from the GPi neuron to the TC neuron is either weak or nonexistent, and Figure 12(d) corresponds to the disappearance of the inhibitory synaptic current. At this time, the TC neuron only receives ISM stimulation and recovers spiking in response to the ISM current, which corresponds to the normal state. This suggests that DBS stimulation is a treatment measure for PD.
The paradoxical bursting of a thalamic neuron in the network related to PD is matched by a similar bursting (i.e., the paradoxical bursting) in an isolated thalamic neuron. In this section, the fast-slow dynamic of the four bursting patterns depicted in Figure 3 is analyzed. In the equilibrium bifurcation of the first kind of bursting (as shown in Figure 3(a) featured in Figure 13(a)), an exemplified "Z"-shaped equilibrium curve is divided into three parts: the upper, middle, and lower branches. The upper branch is made up of the focus. With the steady rise of the bifurcation parameter, the unstable focus (black dashed line) morphs into a stable focus (red solid line) via a supercritical Hopf bifurcation (SupH, r≈−0.15645). The saddle point (blue dashed line) characterizes the middle branch while the lower branch is formed by the stable node (red solid line). Both the lower and middle branches intersect to create a saddle-node bifurcation (SN2 at r≈0.19231)(i.e., a fold bifurcation), with another saddle-node bifurcation (SN1, r≈−0.00605) formed by the intersection of the upper and middle branches. An upper branch-emerging stable limit cycle is introduced (The maximum amplitude Vmax and minimum amplitude Vmin are indicated by the upper and lower green lines, respectively), via the SupH bifurcation. This stable limit cycle coincides with the saddle point on the middle branch to produce a saddle-homoclinic orbit (SH) bifurcation (r≈0.06868) as the bifurcation parameter decreases. An overlying phase trajectory of the bursting (purple solid line) on Figure 13(b) leads to Figure 13(c). Figure 13(d)–(f) similarly detail the dissection results of the bursting from Figure 3(b)–(d), each in regards to fast-slow variable, respectively.
The first spikes in the bursting in Figure 13(c)–(f) start at the SN bifurcation(i.e., a fold bifurcation), and the phase trajectories jump from the lower branch to the stable limit cycle, and then become spiking around the stable limit cycle. After two or more spikes, the phase trajectories reach the homoclinic (SH) bifurcation to jump down to the stable node in the lower branch, and a period of burst is completed. Therefore, the burst begins at the fold (SN) bifurcation and ends at the homoclinic (SH) bifurcation, resulting in the "Fold/Homoclinic" bursting. This result is different from the results of other models of thalamic neurons in the literature [39], wherein the fast subsystem produces a saddle-node bifurcation on an invariant cycle. This suggests a future comprehensive study of different thalamic models of neurons.
The pathogenesis and treatment of PD have been extensively studied in experimental and theoretical models[5,6,7,8,9,10,11,12]. The basal ganglia regulates the electrical activity of the thalamus, and bursting of TC neuron is one of its manifestations. Through DBS of different nuclei of the basal ganglia, such as the subthalamic nucleus, bursting can be eliminated to restore normal firing, which can effectively used to treat PD[4,40,41]. Although there have been numerous studies conducted, the exact mechanism behind the generation and elimination of thalamic bursting remains unclear, due to various responses under excitatory and inhibitory modulation[21,28,29,42,43]. In this paper, we investigated the nonlinear dynamics of generation and elimination of PD-related thalamic bursting [8]. By identifying different responses under excitatory and inhibitory modulation, and combining them with a number of existing electrophysiological experiments [5,14,44,45,46,47], the following conclusions were obtained.
First, the single thalamic neuron exhibits paradoxical bursting, which refers to the phenomenon that inhibitory effects induce bursting from a resting state. This contradicts the traditional belief that inhibitory effects suppress the firing activity, hence being named "paradoxical bursting". In this paper, we utilized a thalamic neuron model that was extensively documented in the existing literature[8]; through a bifurcation analysis and a numerical simulation, we found that the bursting phenomenon can be triggered from a resting state by reducing the transmembrane current from 0. The occurrence of paradoxical bursting, as well as the occurrence of recent paradoxical bursting triggered by enhanced excitatory/inhibitory effects[18,48], offer valuable insights into bursting dynamics and excitatory/inhibitory modulations.
Second, an isolated thalamic neuron possesses the capability to generate post-inhibitory rebound bursting when subjected to an inhibitory pulse stimulus. However, the bursting behavior of thalamic neurons within a network is not characterized by rebound bursting. At a resting state of the thalamic neuron, the application of an inhibitory pulse stimulation activates the positive T-type calcium current to trigger a PIR, which corresponds to the rebound firing found in experimental studies[18]. Rebound firing represents a significant paradoxical electrical activity of the nervous system [48,49], and its relationship with epilepsy and PD has been well-established [5,15,25,50,51]. This contradicts the conventional understanding that inhibitory effects suppress firing. One necessary characteristic of the rebound spike is that the incoming inhibitory pulse stimulation should precede the rebound itself. However, in the network examined in this study, the inhibitory synaptic current pulse appears after the spike or burst, thereby leading to the conclusion that the bursting of thalamic neurons in this context is not rebound bursting.
Finally, the bursting of thalamic neurons in the network associated with PD is a paradoxical form of bursting initiated by the inhibitory synaptic current. However, a DBS applied to the STN eliminates the inhibitory synaptic current received by the thalamic neuron, thus resulting in the restoration of a regular spiking pattern. In the normal state, the thalamic neuron faithfully responds to the excitatory input from the cortex ISM. Within the network, the frequency of STN spiking is reduced by the inhibitory effect of GPe. Therefore, the firing with high frequency changes to bursting alternating between a quiescent state (inhibitory effect is strong) and burst (exciatory effect is strong). GPi inhibitory synapses to the thalamic neurons also exhibit time-varying properties. As a result, there are three types of firing response to a pulse: no spiking, spiking, and bursting. The former two scenarios are easily understood because the inhibitory synaptic current is either strong enough to suppress the spiking or is not strong enough to affect the spiking. However, the last scenario, in which the inhibitory effect induces a bursting, has been difficult to be understand up to now. The present study reveals that the bursting is a paradoxical bursting induced by the inhibitory synaptic current from the basal ganglia, which presents a plausible theoretical explanation for the thalamic bursting. When a DBS stimulation is applied, the STN firing rate is increased, the activity of GPe is enhanced, and GPi is inhibited by the enhanced GPe; thus, the electrical activity of GPi is weakened or even becomes the resting state. Then, the inhibitory synaptic current to the thalamus either reduces or disappears, and the thalamus resumes simple spiking corresponding to normal state. This result reveals the principle of DBS stimulation in the treatment of PD at the level of network, which is the interrelationship between the excitatory and inhibitory modulations to electrical activities of the nuclei in the basal ganglia. In the future, we can continue to study the mechanism of DBS stimulation of other nuclei, such as GPe, in the treatment of PD.
By elucidating the intricate and paradoxical nonlinear dynamics of individual neurons and the complex dynamics of the network influenced by both excitatory and inhibitory effects, this research offers a new theoretical comprehension of the mechanisms underlying the emergence and disappearance of thalamic bursting in PD. The bursting activity observed is a result of paradoxical behavior induced by inhibitory modulation rather than by rebound bursting. The amplification of spiking activity in the thalamic nucleus can decrease the inhibitory synaptic current originating from the GPi to the thalamus and eliminate the paradoxical bursting, thereby yielding therapeutic effects. Our findings contribute to the expansion of examples of paradoxical nonlinear phenomena, improve our understanding of inhibitory regulation, and propose a new mechanism underlying thalamic bursting in PD. In the future, bursting related to PD should be studied in multiple thalamic models and network models containing more parts related to PD, with more modulations being considered.
The authors declare they have not used Artificial Intelligence (AI) tools in the creation of this article.
This work is supported by National Natural Science Foundation of China (No. 12072236), Postgraduate Education Reform and Quality Improvement Project of Henan Province (No. YJS2023SZ15), and The key program of Henan province higher education (No. 24A110005).
The authors declare there is no conflicts of interest.
[1] | Congress US, Energy Independence and Security Act of 2007. Public Law 110-140. Congress Washington DC, 2007. Available from: https://uscode.house.gov/statutes/pl/110/140.pdf. |
[2] | Neitsch SL, Arnold JG, Kiniry JR, et al. (2011) Soil and Water Assessment Tool theoretical documentation version 2009. Texas Water Resources Institute Technical Report no. 406. Available from: https://oaktrust.library.tamu.edu/handle/1969.1/128050. |
[3] | Economic Research Service (ERS), U.S. Department of Agriculture (USDA). Food Environment Atlas, 2022. Available from: https://www.ers.usda.gov/data-products/us-bioenergy-statistics/. |
[4] | National Research Council (2008) Water Implications of Biofuels Production in the United States, Washington DC: National Academies Press. |
[5] | Eghball B, Gilley JE, Kramer LA, et al. (2000) Narrow grass hedge effects on phosphorus and nitrogen in runoff following manure and fertilizer application. J Soil Water Conserv 55: 172-176. |
[6] | Turner RE, Rabalais NN, Dortch Q, et al. (1995) Evidence for nutrient limitation on sources causing hypoxia on the Louisiana shelf, Proceedings of the 1st Gulf of Mexico Hypoxia Management Conference, 106-112. |
[7] |
Blanco‐Canqui H (2010) Energy crops and their implications on soil and environment. Agron J 102: 403-419. https://doi.org/10.2134/agronj2009.0333 doi: 10.2134/agronj2009.0333
![]() |
[8] |
McGregor KC, Dabney S, Johnson JR (1999) Runoff and soil loss from cotton plotswith and without stiff-grass hedges. Trans ASAE 42: 361-368. https://doi.org/10.13031/2013.13367 doi: 10.13031/2013.13367
![]() |
[9] |
Blanco-Canqui H, Gantzer CJ, Anderson SH, et al. (2004) Grass barriers for reduced concentrated flow induced soil and nutrient loss. Soil Sci Soc Am J 68: 1963-1972. https://doi.org/10.2136/sssaj2004.1963 doi: 10.2136/sssaj2004.1963
![]() |
[10] |
Blanco-Canqui H, Gantzer CJ, Anderson SH, et al. (2004) Grass barrier and vegetative filter strip effectiveness in reducing runoff, sediment, nitrogen, and phosphorus loss. Soil Sci Soc Am J 68: 1670-1678. https://doi.org/10.2136/sssaj2004.1670 doi: 10.2136/sssaj2004.1670
![]() |
[11] |
Blanco‐Canqui H (2010) Energy crops and their implications on soil and environment. Agron J 102: 403-419. https://doi.org/10.2134/agronj2009.0333 doi: 10.2134/agronj2009.0333
![]() |
[12] | Hannah L, Lovejoy TE, Schneider SH (2019) Biodiversity and climate change in context, Climate Change and Biodiversity, New Haven: Yale University Press. https://doi.org/10.2307/j.ctv8jnzw1 |
[13] |
Tallis H, Polasky S (2009) Mapping and valuing ecosystem services as an approach for conservation and natural‐resource management. Ann NY Acad Sci 1162: 265-283. https://doi.org/10.1111/j.1749-6632.2009.04152.x doi: 10.1111/j.1749-6632.2009.04152.x
![]() |
[14] |
Engel B, Chaubey I, Thomas M, et al. (2010) Biofuels and water quality: challenges and opportunities for simulation modeling. Biofuels 1: 463-477. https://doi.org/10.4155/bfs.10.17 doi: 10.4155/bfs.10.17
![]() |
[15] |
Gallardo-Vázquez D, Valdez-Juárez LE, Lizcano-Á lvarez JL (2019) Corporate social responsibility and intellectual capital: Sources of competitiveness and legitimacy in organizations' management practices. Sustainability 11: 5843. https://doi.org/10.3390/su11205843 doi: 10.3390/su11205843
![]() |
[16] |
Nä schen K, Diekkrüger B, Evers M, et al. (2019) The impact of land use/land cover change (LULCC) on water resources in a tropical catchment in Tanzania under different climate change scenarios. Sustainability 11: 7083. https://doi.org/10.3390/su11247083 doi: 10.3390/su11247083
![]() |
[17] |
Tang C, Li J, Zhou Z, et al. (2019) How to optimize ecosystem services based on a Bayesian model: A case study of Jinghe River Basin. Sustainability 11: 4149. https://doi.org/10.3390/su11154149 doi: 10.3390/su11154149
![]() |
[18] | Kaini P, Artita K, Nicklow JW (2007) Evaluating optimal detention pond locations at a watershed scale, World Environmental and Water Resources Congress 2007: Restoring Our Natural Habitat, 1-8. https://doi.org/10.1061/40927(243)170 |
[19] |
Kaini P, Artita K, Nicklow JW (2012) Optimizing structural best management practices using SWAT and genetic algorithm to improve water quality goals. Water Resour Manag 26: 1827-1845. https://doi.org/10.1007/s11269-012-9989-0 doi: 10.1007/s11269-012-9989-0
![]() |
[20] | Kaini P, Artita K, Nicklow JW (2009) Generating different scenarios of BMP designs in a watershed scale by combining NSGA-II with SWAT, World Environmental and Water Resources Congress 2009: Great Rivers, 1-9. https://doi.org/10.1061/41036(342)493 |
[21] | Artita KS, Kaini P, Nicklow JW (2008) Generating alternative watershed-scale BMP designs with evolutionary algorithms, World Environmental and Water Resources Congress 2008: Ahupua'A, 1-9. https://doi.org/10.1061/40976(316)127 |
[22] |
Maringanti C, Chaubey I, Arabi M, et al. (2008) A multi-objective optimization tool for the selection and placement of BMPs for pesticide control. Hydrol Earth Syst Sci Discuss 5: 28-29. https://doi.org/10.5194/hessd-5-1821-2008 doi: 10.5194/hessd-5-1821-2008
![]() |
[23] |
Maringanti C, Chaubey I, Arabi M, et al. (2011) Application of a multi-objective optimization method to provide least cost alternatives for NPS pollution control. Environ Manage 48: 448-461. https://doi.org/10.1007/s00267-011-9696-2 doi: 10.1007/s00267-011-9696-2
![]() |
[24] |
Herman MR, Nejadhashemi AP, Daneshvar F, et al. (2016) Optimization of bioenergy crop selection and placement based on a stream health indicator using an evolutionary algorithm. J Environ Manage 181: 413-424. https://doi.org/10.1016/j.jenvman.2016.07.005 doi: 10.1016/j.jenvman.2016.07.005
![]() |
[25] |
Gitau MW, Veith TL, Gburek WJ (2004) Farm-level optimization of BMP placement for cost-effective pollution reduction. Trans ASAE 47: 1923-1931. https://doi.org/10.13031/2013.17805 doi: 10.13031/2013.17805
![]() |
[26] |
Gitau MW, Veith TL, Gburek WJ, et al. (2006) Watershed level best management practice selection and placement in the Town Brook Watershed, New York. J Am Water Resour As 42: 1565-1581. https://doi.org/10.1111/j.1752-1688.2006.tb06021.x doi: 10.1111/j.1752-1688.2006.tb06021.x
![]() |
[27] |
Muleta MK, Nicklow JW (2002) Evolutionary algorithms for multiobjective evaluation of watershed management decisions. J Hydroinf 4: 83-97. https://doi.org/10.2166/hydro.2002.0010 doi: 10.2166/hydro.2002.0010
![]() |
[28] |
Ng TL, Eheart JW, Cai X, et al. (2010) Modeling Miscanthus in the Soil and Water Assessment Tool (SWAT) to simulate its water quality effects as a bioenergy crop. Environ Sci Technol 44: 7138-7144. https://doi.org/10.1021/es9039677 doi: 10.1021/es9039677
![]() |
[29] | USDA Plants Database, Natural Resources Conservation Service. United States Department of Agriculture, 2022. Available from: https://plants.usda.gov/home. |
[30] |
Taboada HA, Coit DW (2012) A new multiple objective evolutionary algorithm for reliability optimization of series-parallel systems. Int J Appl Evol Comput 3: 1-18. https://doi.org/10.4018/jaec.2012040101 doi: 10.4018/jaec.2012040101
![]() |
[31] |
Gassman PW, Reyes MR, Green CH, et al. (2007) The Soil and Water Assessment Tool: historical development, applications, and future research directions. Trans ASABE 50: 1211-1250. https://doi.org/10.13031/2013.23637 doi: 10.13031/2013.23637
![]() |
[32] | Arnold JG, Kiniry JR, Srinivasan R, et al. (2013) SWAT 2012 Input/Output Documentation. Texas Water Resources Institute. Available from: https://oaktrust.library.tamu.edu/handle/1969.1/149194. |
[33] | Winchell M, Srinivasan R, Di Luzio M, et al. (2010) ArcSWAT interface for SWAT 2009, User's Guide, Blackland Research Center, Texas Agricultural Experiment Station, Temple. |
1. | Feibiao Zhan, Jian Song, Complex rhythm and synchronization of half-center oscillators under electromagnetic induction, 2024, 32, 2688-1594, 4454, 10.3934/era.2024201 |
Aα | Bα | θα |
AGPi=2 | BGPi=0.08 | θGPi=−20 |
AGPe=1 | BGPe=0.1 | θGPe=−20 |
ASTN=1 | BSTN=0.05 | θSTN=−30 |
Synaptic current (μA/cm2) | Conductance (mS/cm2) | Reversal potential (mV) |
IGPe→STN | gGPe→STN=0.9 | EGPe→STN=0.9 |
ISTN→GPe | gSTN→GPe=0.3 | ESTN→GPe=0.3 |
IGPe→GPe | gGPe→GPe=1 | EGPe→GPe=1 |
ISTN→GPi | gSTN→GPi=0.3 | ESTN→GPi=0.3 |
IGPe→GPi | gGPe→GPi=0.75 | EGPe→GPi=0.7 |
IGPi→TC | gGPi→TC=0.1 | EGPi→TC=0.1 |
Aα | Bα | θα |
AGPi=2 | BGPi=0.08 | θGPi=−20 |
AGPe=1 | BGPe=0.1 | θGPe=−20 |
ASTN=1 | BSTN=0.05 | θSTN=−30 |
Synaptic current (μA/cm2) | Conductance (mS/cm2) | Reversal potential (mV) |
IGPe→STN | gGPe→STN=0.9 | EGPe→STN=0.9 |
ISTN→GPe | gSTN→GPe=0.3 | ESTN→GPe=0.3 |
IGPe→GPe | gGPe→GPe=1 | EGPe→GPe=1 |
ISTN→GPi | gSTN→GPi=0.3 | ESTN→GPi=0.3 |
IGPe→GPi | gGPe→GPi=0.75 | EGPe→GPi=0.7 |
IGPi→TC | gGPi→TC=0.1 | EGPi→TC=0.1 |