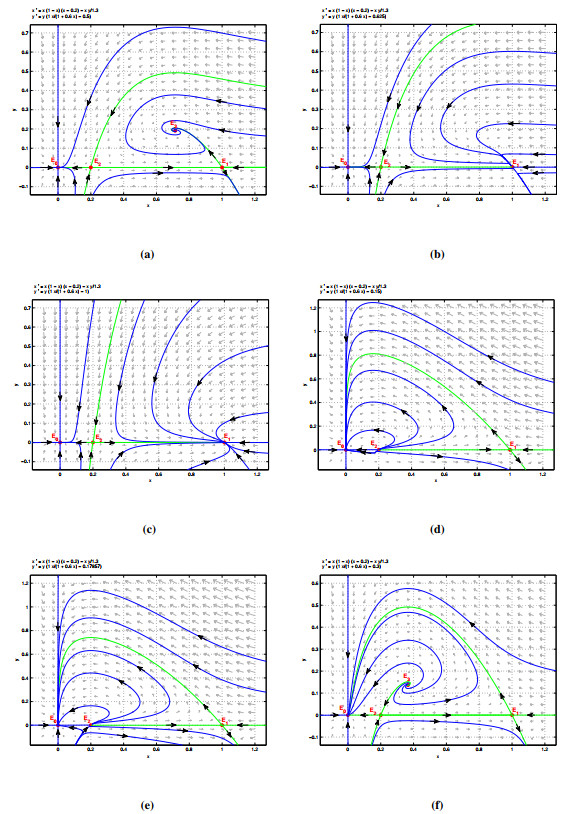
To calculate fractional flow reserve (FFR) based on computed tomography angiography (i.e., FFRCT) by considering the branch flow distribution in the coronary arteries.
FFR is the gold standard to diagnose myocardial ischemia caused by coronary stenosis. An accurate and noninvasive method for obtaining total coronary blood flow is needed for the calculation of FFRCT.
A mathematical model for estimating the coronary blood flow rate and two approaches for setting the patient-specific flow boundary condition were proposed. Coronary branch flow distribution methods based on a volume-flow approach and a diameter-flow approach were employed for the numerical simulation of FFRCT. The values of simulated FFRCT for 16 patients were compared with their clinically measured FFR.
The ratio of total coronary blood flow to cardiac output and the myocardial blood flow under the condition of hyperemia were 16.97% and 4.07 mL/min/g, respectively. The errors of FFRCT compared with clinical data under the volume-flow approach and diameter-flow approach were 10.47% and 11.76%, respectively, the diagnostic accuracies of FFRCT were 65% and 85%, and the consistencies were 95% and 90%.
The mathematical model for estimating the coronary blood flow rate and the coronary branch flow distribution method can be applied to calculate the value of clinical noninvasive FFRCT.
Citation: Honghui Zhang, Jun Xia, Yinlong Yang, Qingqing Yang, Hongfang Song, Jinjie Xie, Yue Ma, Yang Hou, Aike Qiao. Branch flow distribution approach and its application in the calculation of fractional flow reserve in stenotic coronary artery[J]. Mathematical Biosciences and Engineering, 2021, 18(5): 5978-5994. doi: 10.3934/mbe.2021299
[1] | Li Cai, Qian Zhong, Juan Xu, Yuan Huang, Hao Gao . A lumped parameter model for evaluating coronary artery blood supply capacity. Mathematical Biosciences and Engineering, 2024, 21(4): 5838-5862. doi: 10.3934/mbe.2024258 |
[2] | Benchawan Wiwatanapataphee, Yong Hong Wu, Thanongchai Siriapisith, Buraskorn Nuntadilok . Effect of branchings on blood flow in the system of human coronary arteries. Mathematical Biosciences and Engineering, 2012, 9(1): 199-214. doi: 10.3934/mbe.2012.9.199 |
[3] | Xintong Wu, Yingyi Geng, Xinhong Wang, Jucheng Zhang, Ling Xia . Continuous extraction of coronary artery centerline from cardiac CTA images using a regression-based method. Mathematical Biosciences and Engineering, 2023, 20(3): 4988-5003. doi: 10.3934/mbe.2023231 |
[4] | Ziyu Jin, Ning Li . Diagnosis of each main coronary artery stenosis based on whale optimization algorithm and stacking model. Mathematical Biosciences and Engineering, 2022, 19(5): 4568-4591. doi: 10.3934/mbe.2022211 |
[5] | Nattawan Chuchalerm, Wannika Sawangtong, Benchawan Wiwatanapataphee, Thanongchai Siriapisith . Study of Non-Newtonian blood flow - heat transfer characteristics in the human coronary system with an external magnetic field. Mathematical Biosciences and Engineering, 2022, 19(9): 9550-9570. doi: 10.3934/mbe.2022444 |
[6] | B. Wiwatanapataphee, D. Poltem, Yong Hong Wu, Y. Lenbury . Simulation of Pulsatile Flow of Blood in Stenosed Coronary Artery Bypass with Graft. Mathematical Biosciences and Engineering, 2006, 3(2): 371-383. doi: 10.3934/mbe.2006.3.371 |
[7] | Martina Bukač, Sunčica Čanić . Longitudinal displacement in viscoelastic arteries:A novel fluid-structure interaction computational model, and experimental validation. Mathematical Biosciences and Engineering, 2013, 10(2): 295-318. doi: 10.3934/mbe.2013.10.295 |
[8] | Bin Zhang, Kuan Zeng, Rongzhen Li, Huiqi Jiang, Minnan Gao, Lu Zhang, Jianfen Li, Ruicong Guan, Yuqiang Liu, Yongjia Qiang, Yanqi Yang . Construction of the gene expression subgroups of patients with coronary artery disease through bioinformatics approach. Mathematical Biosciences and Engineering, 2021, 18(6): 8622-8640. doi: 10.3934/mbe.2021427 |
[9] | Fan He, Minru Li, Xinyu Wang, Lu Hua, Tingting Guo . Numerical investigation of quantitative pulmonary pressure ratio in different degrees of stenosis. Mathematical Biosciences and Engineering, 2024, 21(2): 1806-1818. doi: 10.3934/mbe.2024078 |
[10] | Yuqian Mei, Debao Guan, Xinyu Tong, Qian Liu, Mingcheng Hu, Guangxin Chen, Caijuan Li . Association of cerebral infarction with vertebral arterial fenestration using non-Newtonian hemodynamic evaluation. Mathematical Biosciences and Engineering, 2022, 19(7): 7076-7090. doi: 10.3934/mbe.2022334 |
To calculate fractional flow reserve (FFR) based on computed tomography angiography (i.e., FFRCT) by considering the branch flow distribution in the coronary arteries.
FFR is the gold standard to diagnose myocardial ischemia caused by coronary stenosis. An accurate and noninvasive method for obtaining total coronary blood flow is needed for the calculation of FFRCT.
A mathematical model for estimating the coronary blood flow rate and two approaches for setting the patient-specific flow boundary condition were proposed. Coronary branch flow distribution methods based on a volume-flow approach and a diameter-flow approach were employed for the numerical simulation of FFRCT. The values of simulated FFRCT for 16 patients were compared with their clinically measured FFR.
The ratio of total coronary blood flow to cardiac output and the myocardial blood flow under the condition of hyperemia were 16.97% and 4.07 mL/min/g, respectively. The errors of FFRCT compared with clinical data under the volume-flow approach and diameter-flow approach were 10.47% and 11.76%, respectively, the diagnostic accuracies of FFRCT were 65% and 85%, and the consistencies were 95% and 90%.
The mathematical model for estimating the coronary blood flow rate and the coronary branch flow distribution method can be applied to calculate the value of clinical noninvasive FFRCT.
In the field of mathematical ecology, mathematical models are extensively used to explore and describe the complex interactions among individuals and between individuals and their surroundings by many scholars [1,2,3,4,5]. Especially, the predator-prey models have received great attention over the last one hundred years. Since the classical two species Lotka-Volterra model was put forward [1], there are a great many of experiments and papers investigating the interactions between predators and prey from each aspect [6,7,8,9,10]. Such as, in [7] the authors studied the complex dynamics of a predator-prey model with the nonlinear Michaelis-Menten type predator harvesting. Li et al. [8] proposed a Leslie-Gower predator-prey model with simplified Holling-type Ⅳ functional response. In [10] S. Djilali and S. Bentout proposed a delayed diffusive predator-prey model with prey social behavior and predator harvesting, and analyzed the dynamics of the deterministic model and diffusive model.
In recent years, it is widely recognized the Allee effect has important significance on the population dynamical relation and may make the dynamics more richer. Specifically, Allee effect refers to a positive correlation between population density and individual average fitness when the population is small [11,12]. From the view point of ecology, Allee effect on species can occur under some mechanisms, such as the change of habitat, inbreeding depression, difficulties in finding a spouse, avoiding predators, and the predation risk caused by environment conditions [13,14,15]. Moreover, the Allee effect is mainly divided into two forms: Strong and weak [16]. The strong Allee effect denotes the negative per capita growth rate when population density is below the so-called Allee threshold and the growth rate becomes positive when the population density is above this threshold [17]. This means, the population must surpass the threshold to grow. Contrarily, a population with a weak Allee effect has no such above threshold and the weak Allee effect means that when the species density is low, the per capita growth rate becomes small, but remains positive [18,19]. This implies the Allee effect may lead to much more complex dynamics and thereby affect the survival of the population. In fact in [20], Hilker et al. have introduced the strong Allee effect in a simple epidemic model [21,22] and shown that Allee effect can induce surprisingly rich dynamical behaviors, like periodic oscillations, multiple alternative steady states and homoclinic loops with the extinction of host populations. The complexity reveals, the introduction of the Allee effect in mathematical systems has important practical significance to the study of long-term species morphology and the development of species. This could have far-reaching implications in areas of biological protection.
On the other hand, there is another key objective that can also affect the dynamical behaviors of the prey-predator models: Functional response [23]. It refers to the average number of prey killed by each individual predator per unit of time and has two main types: Predator-dependent (as a function of prey and predators densities) and prey-dependent (as a function of the prey density) [24]. Exactly, functional response denotes the latter, like the famous Holling family [25], plays a predominant role in species dynamics models. For instance, since 1965, Holling's type Ⅱ functional response has acted as the basis for many literatures [26,27,28,29,30] on predator-prey theory.
Specially, for insect pest management, in [28] Sun et al. considered a prey-predator system with Holling type Ⅱ functional response in the predator:
{dxdt=x(r−rxK−by),dydt=y(λbx1+bhx−d1), | (1.1) |
where x(t) and y(t) denote the population densities of prey and predator at time t, respectively. r is the growth rate of x(t), K is the environment capacity in the absence of predator. bx1+bhx, which is assumed to be the typical Holling type Ⅱ functional response form, gives a description of the per capita conversion rate from prey to predator. Concretely, b and h represent the searching rate and handling time of predator, λ denotes the conversion efficiency. All the parameters are positive. By dynamical analysis, Sun et al. [28] concluded if 0≤h≤λd1, system (1.1) has two saddles P0(0,0), P1(K,0), and a globally asymptotically stable focus P3(d1b(λ−hd1),r(Kb(λ−hd1)−d1)Kb2(λ−hd1)).
Thus, motivated by the above discussion, we naturally want to know: When an Allee effect is subject to the first species of system (1.1), what will happen to the dynamical properties? Hence, we construct the following ecological model with a strong Allee effect in prey
{dxdt=x(r−rxK)(x−A)−bxy,dydt=y(λbx1+bhx−d1), | (1.2) |
where A is the strong Allee effect parameter and it satisfies 0<A<K and other parameters are the same as system (1.1). Let ¯t=Krt, ¯x=1Kx and ¯y=bry, and drop the bars, we get
{dxdt=x(1−x)(x−α)−1Kxy,dydt=y(ωx1+ηx−σ), | (1.3) |
where α=AK, ω=λbr, σ=d1Kr, η=bhK, and the strong Allee effect parameter α taken as 0<α<1.
Our main purpose aims to see how the strong Allee effect affect the dynamics of the prey-predator model with Holling type Ⅱ functional response. The layout of this paper is as follows. First, the positivity and boundedness of solution of system (1.3) are studied in Section 2. Then, in Section 3, we give the analysis of equilibria of system (1.3), including the distribution of all the possible equilibria and their stability. In Section 4, we verify the existence of transcritical bifurcation and Hopf-bifurcation. Additionally, numerical simulations and computations are carried out to explore and visualize the impact of the strong Allee effect in Section 5. Finally, this paper is concluded with a brief summary in Section 6.
Next, the positivity and boundedness of the solutions of system (1.3) will be shown in the region R2+={(x,y):x≥0,y≥0}.
Lemma 2.1. For all t≥0, every solution of system (1.3) with initial value is positive.
Proof. Solving system (1.3) with positive initial condition (x(0),y(0)), we get the result:
x(t)=x(0)[exp∫t0((1−x(s))(x(s)−α)−1Ky(s))ds]>0,y(t)=y(0)[exp∫t0(ωx(s)(1+ηx(s))−σ)ds]>0. |
Hence, any solution of system (1.3) starting from the interior of the first quadrant xy-plane still remains in it for all future times.
Lemma 2.2. Every solution of system (1.3) with positive initial value is uniformly bounded.
Proof. Let Φ=Kωx+y and δ=min{α,σ}, we have
dΦdt+δΦ=Kω[x(1−x)(x−α)−1Kxy]+ωxy1+ηx−σy+δKωx+δy≤−Kωx3+Kω(1+α)x2−Kω(α−δ)x−(σ−δ)y≤−Kωx3+Kω(1+α)x2≤4Kω(1+α)327≜ϱ. |
Integrating the above inequality, we have Φ(t)≤e−δtΦ(0)+ϱδ(1−e−δt) and limsupΦ(t)≤ϱδ−1, as t→∞, independently of the initial conditions. Therefore, the solutions of system (1.3) are uniformly bounded.
Remark 2.1. From the perspective of ecology, uniform boundedness of system (1.3) means that the interacting two species are ecologically well behaved and none of them can grow exponentially for a long period of time owing to limited food sources.
Obviously, for all permissible parameters, system (1.3) always has three boundary equilibria E0(0,0), E1(1,0) and E2(α,0). If system (1.3) admits the positive equilibrium, then x and y must satisfy the equation as follows:
{(1−x)(x−α)−yK=0,ωx1+ηx−σ=0. | (3.1) |
By some simple algebraic calculations, we obtain the system has a unique positive equilibrium E3(σω−ση,K(ω−(η+1)σ)(σ−α(ω−ση))(ω−ση)2) for ωα1+ηα<σ<ω1+η.
Theorem 3.1. The trivial equilibrium E0 is a hyperbolic stable node.
Proof. The Jacobian matrix at E0(0,0) is given by
JE0=(−α00−σ), | (3.2) |
which, clearly, has two negative eigenvalues λ1(E0)=−α<0 and λ2(E0)=−σ<0. Hence, the extinction equilibrium E0 in the first quadrant is always a stable node.
Theorem 3.2. For the boundary equilibrium E1:
(i) if σ<ω1+η, then E1 is a hyperbolic saddle;
(ii) if σ=ω1+η, then E1 is a non-hyperbolic saddle node. It means Sϵ(E1) is divided into two parts by two separatrices that tend to E1 along the left and the right of E1, where Sϵ(E1) is a neighborhood of E1 with sufficient small radius ϵ. One part consists of two hyperbolic sectors and another is a parabolic sector which is on the upper half plane.
(iii) if σ>ω1+η, then E1 is a stable node.
Proof. The corresponding Jacobian matrix at E1(1,0) is calculated as follows:
JE1=(α−1−1K0ω1+η−σ), | (3.3) |
with two eigenvalues λ1(E1)=α−1<0 and λ2(E1)=ω1+η−σ. Thus E1 is a hyperbolic saddle if σ<ω1+η and a stable node if σ>ω1+η (see Fig. 1(a) and (c)). For σ=ω1+η, namely, the eigenvalue λ2(E1)=0 and E1 is non-hyperbolic, so we can not judge its type from the eigenvalues directly. Below we will use Theorem 7.1 in Chapter 2 in [31] to discuss the stability properties of E1.
By letting (ˉx,ˉy)=(x−1,y), we change system (1.3) into a standard form and then expand it to
{dˉxdt=(α−1)ˉx−1Kˉy−(2−α)ˉx2−1Kˉxˉy−ˉx3,dˉydt=c1ˉxˉy+c2ˉx2ˉy+c3ˉx3ˉy+Q0(ˉx,ˉy), | (3.4) |
where c1=ω(1+η)2, c2=−ωη(1+η)3, c3=ωη2(1+η)4, and Q0(ˉx,ˉy) represents a power series with terms ˉxiˉyj (i+j≥5).
By the linear transformation
(ˉxˉy)=(1−10(1−α)K)(uv), | (3.5) |
then system (3.4) becomes
{dudt=d1u+d2u2+d3uv+d4v2+d5u3+d6u2v+d7uv2+d8v3+Q1(u,v),dvdt=c1uv−c1v2+c2u2v−2c2uv2+c2v3+Q2(u,v), | (3.6) |
where
d1=α−1, d2=−2+α, d3=−2α+3−c1, d4=−1−c1,d5=−1, d6=c2−3, d7=−3−2c2, d8=c2−1, |
and Q1(u,v) and Q2(u,v) represent power series with terms uivj(i+j≥4).
Let τ=d1t, then system (3.6) becomes
{dudτ=u+d2d1u2+d3d1uv+d4d1v2+d5d1u3+d6d1u2v+d7d1uv2+d8d1v3+Q1(u,v)d1≜u+P(u,v),dvdτ=c1d1uv−c1d1v2+c2d1u2v−2c2d1uv2+c2d1v3+Q2(u,v)d1≜Q(u,v). | (3.7) |
From the implicit function theorem and dudτ=0, we can obtain a unique function
u=φ1(v)=−d4d1v2+d3d4−d1d8d21v3+⋯, |
which satisfies φ1(0)=φ′1(0)=0 and φ1(v)+P(φ1(v),v)=0. Then substituting it into the second equation of (3.7), we have that
dvdτ=−c1d1v2−c1d4−c2d1d21v3−c1(d3d4−d1d8)−2c2d1d4d31v4+O(|v|5). | (3.8) |
The coefficient of v2 is −c1d1<0. Thus, by using Theorem 7.1 in Chapter 2 in [31], we get E1 is a saddle node. This means that a neighborhood of Sε(E1) is divided into two parts by two separatrices that tend to E1 along the left and the right of E1 (see Figure 1(b)). One part consists of two hyperbolic sectors and another is a parabolic sector which is on the upper half plane.
Theorem 3.3. For the boundary equilibrium E2:
(i) if σ<ωα1+ηα, then E2 is an unstable node;
(ii) if σ=ωα1+ηα, then E2 is a non-hyperbolic saddle node. This means Sϵ(E2) is divided into two parts by two separatrices that move away along the left and the right of E2. One part consists of two hyperbolic sectors, and another is a parabolic sector which is on the upper half-plane;
(iii) if σ>ωα1+ηα, then E2 is a hyperbolic saddle.
Proof. The Jacobian matrix JE2 of E2(α,0) is
JE2=(α(1−α)−1Kα0ωα1+ηα−σ). | (3.9) |
Then the eigenvalues λ1(E2)=α(1−α)>0 and λ2(E2)=ωα1+ηα−σ. Clearly, E2 is an unstable node if σ<ωα1+ηα and a saddle if σ>ωα1+ηα (see Figure 1(d) and (f)). However, when σ=ωα1+ηα, the eigenvalue λ2(E2)=0 which indicates E2 is non-hyperbolic. In order to deduce its stability, we translate E2 to the origin via the translation (ˉx,ˉy)=(x−α,y) and then expand system (1.3) to
{dˉxdt=(1−α)αˉx−αKˉy+(1−2α)ˉx2−1Kˉxˉy−ˉx3,dˉydt=f1ˉxˉy+f2ˉx2ˉy+M0(ˉx,ˉy), | (3.10) |
where f1=ω(1+ηα)2, f2=−ωη(1+ηα)3, and M0(ˉx,ˉy) represents a power series in (ˉx,ˉy) with terms ˉxiˉyj(i+j≥4).
Let
T=(110K(1−α)) |
be an invertible matrix. We use the following linear transformation
(uv)=T(ˉxˉy), | (3.11) |
then system (3.10) turns into
{dudt=e1u+e2u2+e3uv+e4v2+e5u3+e6v3+e7u2v+e8uv2+M1(u,v),dvdt=f1uv+f1v2+f2u2v+2f2uv2+f2v3+M2(u,v), | (3.12) |
where e1=(1−α)α, e2=1−2α, e3=1−3α−f1, e4=−α−f1, e5=−1, e6=f2−1, e7=f2−3, and e8=2f2−3. M1(u,v) and M2(u,v) represent power series with terms uivj(i+j≥4).
Set τ=e1t, we attain
{dudτ=u+e2e1u2+e3e1uv+e4e1v2+e5e1u3+e6e1v3+e7e1u2v+e8e1uv2+M1(u,v)e1≜u+U(u,v),dvdτ=f1e1uv+f1e1v2+f2e1u2v+2f2e1uv2+f2e1v3+M2(u,v)e1≜N(u,v). | (3.13) |
From dudτ=0, we obtain the implicit function
u=φ1(v)=−e4e1v2−e1e6−e3e4e21v3+⋯, |
which satisfies φ1(0)=φ′1(0)=0 and φ1(v)+U(φ1(v),v)=0. Then substituting it into the second equation of (3.13), we have
dvdτ=f1e1v2−f1e4−f2e1e21v3+O(|v|4). | (3.14) |
Hence, according to the Theorem 7.1 in Chapter 2 in [31], we have m=2 and am=ω(1−α)α(1+ηα)2>0, so E2 is a saddle node. That indicates a neighborhood of Sε(E2) is divided into two parts by two separatrices that move away along the left and the right of E2. One part consists of two hyperbolic sectors and another is a parabolic sector which is on the upper half plane (see Figure 1(e)).
Theorem 3.4. For the positive equilibrium E3:
(i) if ω(1+α)2+η(1+α)<σ<ω1+η, then E3 is locally asymptotically stable;
(ii) if σ=ω(1+α)2+η(1+α), then E3 is a center or weak focus;
(iii) if ωα1+ηα<σ<ω(1+α)2+η(1+α), then E3 is unstable.
Proof. The Jacobian matrix JE3 of E3(x∗,y∗) is
JE3=(x∗(1−2x∗+α)−1Kx∗ωy∗(1+ηx∗)20). | (3.15) |
From x∗(1−x∗)(x∗−α)−1Kx∗y∗=0, we can derive
JE3=(x∗(1+α)(ω−ση)−2σω−ση−1Kx∗ωy∗(1+ηx∗)20). | (3.16) |
So the determinant and the trace of Jacobian matrix JE3 are calculated by
Det[JE3]=ωx∗y∗K(1+ηx∗)2 and Tr[JE3]=x∗(1+α)(ω−ση)−2σω−ση. |
When ωα1+ηα<σ<ω1+η, Det[JE3] is positive, but the sign of Tr[JE3] cannot be obtained directly. If ω(1+α)2+η(1+α)<σ<ω1+η, then Tr[JE3]<0, that is E3 is locally asymptotically stable (see Figure 2(a)). If σ=ω(1+α)2+η(1+α), then Tr[JE3]=0, that implies JE3 has a pair of purely imaginary eigenvalues. It confirms that the equilibrium E3 may be a center, a weak focus or a center focus. We also can easily show that F(x,y)=−x3+(1+α)x2−1Kxy and G(x,y)=ωxy1+ηx, which are the nonlinear parts of system (1.3), are analytic functions. Thus, from the corollary of Theorem 2.1 in Chapter 4 in [31], we obtain that E3 is a center (see Figure 2(b), (c)) or weak focus. If ωα1+ηα<σ<ω(1+α)2+η(1+α), then Tr[JE3]>0, it means E3 is unstable (see Figure 2(d), (e)). Thus, this completes the proof.
In this part, we mainly put the focus on the various bifurcation behaviors of system (1.3).
Theorem 4.1. For transcritical bifurcation, the following statements are true.
(1) When the parameters satisfy σ=σTC1=ω1+η, system (1.3) undergoes a transcritical bifurcation referred as TC1 at E1;
(2) When the parameters satisfy σ=σTC2=ωα1+ηα, system (1.3) undergoes another transcritical bifurcation referred as TC2 at E2.
Proof. (1) Now we verify the transversality condition for transcritical bifurcation by using Sotomayor's theorem [32,33]. If σ=σTC1=ω1+η, then Det[JE1]=0, which means one eigenvalue of the Jacobian matrix JE1 is zero. Let V and W represent the two eigenvectors corresponding to the zero eigenvalue of JE1 and it's transpose JTE1, respectively, and they are given by
V=(v1v2)=(1K(α−1)) and W=(w1w2)=(01). |
Furthermore, we have
Fσ(E1;σTC1)=(0−y)(E1;σTC1)=(00), | (4.1) |
DFσ(E1;σTC1)V=(000−1)(1K(α−1))(E1;σTC1)=(0K(α−1)), | (4.2) |
D2F(E1;σTC1)(V,V)=(∂2F1∂x2v21+2∂2F1∂x∂yv1v2+∂2F1∂y2v22∂2F2∂x2v21+2∂2F2∂x∂yv1v2+∂2F2∂y2v22)(E1;σTC1)=(−22Kω(α−1)(1+η)2). |
Also
WTFσ(E1;σTC1)=0, |
WT[DFσ(E1;σTC1)V]=K(α−1)≠0, |
WT[D2F(E1;σTC1)(V,V)]=2Kω(α−1)(1+η)2≠0. |
Consequently, system (1.3) undergoes a transcritical bifurcation at σ=σTC1.
(2) Now we also apply Sotomayor's theorem to prove the transversality condition for another transcritical bifurcation at σ=σTC2=ωα1+ηα. Similar to the process of the former, the transversality condition is derived.
WTFσ(E2;σTC2)=0, |
WT[DFσ(E2;σTC2)V]=−Kα(1−α)≠0, |
and
WT[D2F(E2;σTC2)(V,V)]=−2Kωα(1−α)(1+η)2≠0, |
where
V=(v1v2)=(1Kα(1−α)) and W=(w1w2)=(01) |
are the two eigenvectors corresponding to the zero eigenvalue of the Jacobian matrix JE2 and it's transpose JTE2, respectively. Hence, we complete the proof.
In particular, from Figure 1(a), we can see that for σ<σTC1, there are two equilibria E1 and E3. And E1 is unstable and E3 is stable. For σ=σTC1 (see Figure 1(b)), these two equilibria coalesce. For σ>σTC1(see Figure 1(c)), E1 is stable and E3 is unstable. In this case, E3 is a negative equilibrium. Biologically, we consider the positive equilibria, so we ignore it in the corresponding phase portrait. Thus, an exchange of stability has occurred at σ=σTC1, that is to say, the transcritical bifurcation at σ=σTC1 occurs by the Sotomayor's theorem. Moreover, the occurrence of another transcritical bifurcation at σ=σTC2 is similarly illustrated in Figure 1(d), (e), (f).
In this subsection, the possible occurrence of Hopf-bifurcation around the interior equilibrium E3 about the bifurcation parameter σ is explored. In a two dimensional system, it is concluded Hopf-bifurcation occurs as the stability of the interior equilibrium changes (from stable to unstable or vice-versa) and a periodic solution appears (or disappears). Next, we will give the proof of the existence for Hopf-bifurcation of system (1.3).
Theorem 4.2. The conditions for occurrence of Hopf-bifurcation at the critical value σ=σH=ω(1+α)2+η(1+α) around the positive equilibrium E3(x∗,y∗) are ωα1+ηα<σ<ω1+η and ddσTr[JE3]≠0 at σ=σH.
Proof. From the Jacobian matrix JE3 calculated at E3(x∗,y∗), we consider σ as a bifurcation parameter. For occurrence of Hopf-bifurcation, the characteristic equation of JE3 must have a pair of purely imaginary roots. At σ=σH, Tr[JE3]=0, then the characteristic equation of JE3 becomes
λ2+Det[JE3]=0. | (4.3) |
When ωα1+ηα<σ<ω1+η, Det[JE3]>0, namely the above characteristic equation has a pair of conjugated imaginary eigenvalues λ1,2=±iθ, where θ=√Det[JE3].
Now we check the transversality condition ddσ{Re(λ)}|σ=σH≠0 which confirms the eigenvalues cross the imaginary axis transversely with non-zero speed. Let σ be any point in the neighbourhood of σH, the eigenvalues of the Jacobian matrix JE3 at σ are λ1,2=χ(σ)±iθ(σ), where χ(σ)=Tr[JE3]2 and θ(σ)=√Det[JE3]−Tr2[JE3]4. Then
ddσ{Re(λ)}|σ=σH=ddσχ(σ)|σ=σH=d2dσTr[JE3]|σ=σH=348ω(1+α)(2+(1+α)η)2≠0. | (4.4) |
Thus the transversality condition is verified and a Hopf-bifurcation of system (1.3) occurs at σ=σH.
In order to determine the direction of Hopf-bifurcation and the stability of the periodic solution which originates from the positive equilibrium via Hopf bifurcation, the first Lyapunov coefficient [33] needs to be computed.
Firstly, set z1=x−x∗ and z2=y−y∗, then system (1.3) can be written as
{˙z1=m10z1+m01z2+m20z21+m11z1z2+m02z22+m30z31+m21z21z2+m12z1z22+m03z32,˙z2=n10z1+n01z2+n20z21+n11z1z2+n02z22+n30z31+n21z21z2+n12z1z22+n03z32+O1(z1,z2), | (4.5) |
where
m10=(1−2x∗+α)x∗, m01=−1Kx∗, m20=1−3x∗+α, m11=−1K, m02=0,m30=−1, m21=m12=m03=0, n10=ωy∗(1+ηx∗)2, n01=0, n20=−ωηy∗(1+ηx∗)3,n11=ω(1+ηx∗)2, n02=0, n30=−ωη2y∗(1+ηx∗)4, n21=−ωη(1+ηx∗)3, n12=n03=0. |
By ignoring the higher order terms of degree 4 and above, then system (4.5) becomes
˙Z=JE3Z+A(Z), | (4.6) |
where
Z=(Z1Z2)and A=(A1A2)=(m20z21+m11z1z2+m02z22+m30z31+m21z21z2+m12z1z22+m03z32n20z21+n11z1z2+n02z22+n30z31+n21z21z2+n12z1z22+n03z32). |
The eigenvector ˉv of JE3 for the eigenvalue iθ at σ=σH is ˉv=(m01iθ−m10).
Now we define
Q=(Re(ˉv),−Im(ˉv))=(m010−m10−θ). |
Let Z=QU, where U=(uv). Under this transformation, system (4.6) becomes
˙U=(Q−1JE3Q)U+A(QU). |
Thus
(˙u˙v)=(0−θθ0)=(uv)+(S1(u,v;σ=σH)S2(u,v;σ=σH)), | (4.7) |
where S1 and S2 are non-linear in u and v given by
S1(u,v;σ=σH)=1m01A1, S2(u,v;σ=σH)=−1θm01(m10A1+m01A2) |
with
A1=(m20m201−m11m01m10+m02m210)u2+θ(2m02m10−m11m01)uv+θ2m02v2+(m12m01m210−m03m310+m30m301−m21m201m10)u3+θ(2m12m10m01−m21m201−3m03m210)u2v+θ2(m12m01−3m03m10)uv2−θ3m03v3,A2=(n20m201−n11m01m10+n02m210)u2+θ(2n02m10−n11m01)uv+θ2n02v2+(n12m01m210−n03m310+n30m301−n21m201m10)u3+θ(2n12m10m01−n21m201−3n03m210)u2v+θ2(n12m01−3n03m10)uv2−θ3n03v3. |
Regarding the normal form (4.7), we calculate the first Lyapunov coefficient and obtain
l1=116(S1uuu+S1uvv+S2vvv) +116θ(S1uv(S1uu+S1vv)−S2uv(S2uv+S2vv)−S1uuS2uu+S1vvS2vv)=116(6m30m201+2n21m201)+116θ(−2ωm20m01+2θn11n20m301+4θn20m20m301)=−14x∗2(1K2+x∗2K3(1+ηx∗)). |
Evidently, l1<0, so that a stable Hopf-bifurcating limit cycle appears and the Hopf-bifurcation is supercritical, which indicates the stable periodic solution overlaps an unstable interior equilibrium. From view of ecology, this phenomenon means that both predators and prey reach an oscillatory coexistence state. And, in the next section, we are going to explore this phenomenon a step further with the help of numerical simulations.
To verify the validity of the obtained analytical results, in this part, some numerical simulations and computations are carried out. Firstly, the phase portraits corresponding to the scenario of no positive equilibrium and one positive equilibrium are plotted in Figures 1 and 2 by varying the key parameter value σ and keeping other fixed parameters values α=0.2, K=1.3, ω=1 and η=0.6. Further, using this set of parameter values and different values of σ, we also give some time series diagrams of system (1.3) for a better visualization of how the strong Allee effect influence the dynamics of the model, with special attention to the stability and cyclical behavior.
Case 5.1. For σ = 0.5, the high mortality of natural enemy predator, prey and the natural enemy populations reach a stable coexistence state because of the reduced predation pressure on prey and Allee effect (Figures 2(a) and 3).
Case 5.2. For gradually decreasing the value of σ, the positive equilibrium E3 of model (1.3) loses stability via a Hopf bifurcation at σH = 0.441176 (Figure 2(b), (c)) and the Hopf bifurcation is supercritical as the first Lyapunov coefficient l1=−0.072449680827<0. Meanwhile, as shown in Figure 4, both the total populations begin to oscillate. From Figure 2(d), (e), it is found when σ<σH slightly, a stable limit cycle from E3 is bifurcated. Prey and predator populations reach an oscillation coexistence state (Figure 5). The amplitude of the oscillation is initially small, but then increases rapidly with decreasing σ, which is illustrated with a three-dimensional bifurcation diagram in Figure 6. Biologically, the populations fluctuate a lot, which corresponds to the sudden increase of prey.
Case 5.3. For small value σ = 0.3, the low mortality of natural enemy predator, we observe that the limit cycle disappears and both prey and predator approach the extinction state for the higher predation pressure on prey and the Allee effect (Figures 1(f) and 7).
Remark 5.1. One can see that the model is sensitive to the initial condition. The strong Allee effect causes more complex dynamics, with total population density tending to either the extinction state E0(0,0) or coexistence state E3(x∗,y∗) depending on the selection of the initial condition. In the real world, for biological protection, our purpose is generally to maintain the sustainable survival of species rather than eradicating the species completely. Accordingly, in the above cases, we choose the initial condition [x(0),y(0)] = [1, 0.1].
In this article, a predator-prey model with strong Allee effect in prey was proposed. We investigated the classification and stability of each equilibrium of system (1.3) and fulfilled a comprehensive bifurcation analysis. Qualitative analysis shows that the model's dynamics grow more complicated when Allee effect is incorporated into the first species. More specifically, with the comparison to system (1.1) [28] in absence of Allee effect, we find some new dynamical properties of model (1.3).
(1) System (1.3) possesses more types of equilibria due to the strong Allee effect. The extinction equilibrium is always an attractor, whereas the stability of other two boundary equilibria and the unique interior equilibrium changes with different parameter conditions. For the system with no Allee effect, both boundary equilibria are saddles and the interior equilibrium is always globally asymptotically stable.
(2) System (1.3) exhibits more bifurcation behaviors for the existence of multiple types of equilibria. Only one transcritical bifurcation occurs for system (1.1), whereas system (1.3) undergoes two transcritical bifurcations and one Hopf-bifurcation. Also a stable limit cycle around unstable positive equilibrium E3 corresponding to system (1.3) appears.
It can be clearly seen that the strong Allee effect can lead to potential changes in system dynamics. It also has a significant role in ecology. Allee effect, such as, can destabilize the coexistence steady state. From stable coexistence to oscillating coexistence, and then from oscillating coexistence to overall populations extinction state, the prey and predator populations go through multiple stability switches. Moreover, in all cases, the possibility of total species extinction in the model is caused by Allee effect. This suggests that if prey or predators are subject to Allee effect, the measures we adopt for nature preservation should take this into consideration, which is important for analyzing the long-term survival of all populations.
We just consider the impact of Allee effect in prey on the dynamical behaviors of the predator-prey system in this paper. It will be an interesting theme to study the dynamics of the model with Allee effects in both populations. We will leave this as future work.
The second author was supported by the National Natural Science Foundation of China (No.12001503) and the third author was supported by the Project of Beijing Municipal Commission of Education (KM 202110015001).
The authors declare no conflict of interest.
[1] | E. Conte, J. Sonck, S. Mushtaq, C. Collet, T. Mizukami, E. Barbato, et al., FFRCT and CT perfusion: A review on the evaluation of functional impact of coronary artery stenosis by cardiac CT, Int. J. Cardiol., 9 (2020), 289-296. |
[2] |
A. Cesaro, F. Gragnano, D. D. Girolamo, E. Moscarella, V. Diana, I. Pariggiano, et al., Functional assessment of coronary stenosis: an overview of available techniques. Is quantitative flow ratio a step to the future?, Expert. Rev. Cardiovasc. Ther., 16 (2018), 951-962. doi: 10.1080/14779072.2018.1540303
![]() |
[3] | G. Y. Li, H. R. Wang, M. Z. Zhang, S. Tupin, A. K. Qiao, Y. J. Liu, et al., Prediction of 3D cardiovascular hemodynamics before and after coronary artery bypass surgery via deep learning, Commun. Biol., 99 (2021), 1-12. |
[4] |
N. S. Kleiman, Bringing it all together: integration of physiology with anatomy during cardiac catheterization, J. Am. Coll. Cardiol., 58 (2011), 1219-1221. doi: 10.1016/j.jacc.2011.06.019
![]() |
[5] |
C. A. Taylor, T. A. Fonte, J. K. Min, Computational fluid dynamics applied to cardiac computed tomography for noninvasive quantification of fractional flow reserve: scientific basis, J. Am. Coll. Cardiol., 61 (2013), 2233-2241. doi: 10.1016/j.jacc.2012.11.083
![]() |
[6] |
J. K. Min, C. A. Taylor, S. Achenbach, B. K. Koo, J. Leipsic, B. L. Nørgaard, et al., Noninvasive fractional flow reserve derived from coronary CT angiography: clinical data and scientific principles, JACC Cardiovasc. Imaging, 8 (2015), 1209-1222. doi: 10.1016/j.jcmg.2015.08.006
![]() |
[7] |
C. Tesche, C. N. D. Cecco, M. H. Albrecht, T. M. Duguay, R. R. Bayer, S. E. Litwin, et al., Coronary CT angiography-derived fractional flow reserve, Radiology, 285 (2017), 17-33. doi: 10.1148/radiol.2017162641
![]() |
[8] |
M. van Assen, C. N. D. Cecco, M. Eid, P. von Knebel Doeberitz, M. Scarabello, F. Lavra, et al., Prognostic value of CT myocardial perfusion imaging and CT-derived fractional flow reserve for major adverse cardiac events in patients with coronary artery disease, J. Cardiovasc. Comput. Tomogr., 13 (2019), 26-33. doi: 10.1016/j.jcct.2019.02.005
![]() |
[9] | K. Nieman. The feasibility, findings and future of CT-FFR in the emergency ward, J. Cardiovasc. Comput. Tomogr., 19 (2019), 287-288. |
[10] |
B. K. Koo, A. Erglis, J. H. Doh, D. V. Daniels, S. Jegere, H. S. Kim, et al., Diagnosis of ischemia-causing coronary stenoses by noninvasive fractional flow reserve computed from coronary computed tomographic angiograms. Results from the prospective multicenter DISCOVER-FLOW (diagnosis of ischemia-causing stenoses obtained via noninvasive fractional flow reserve) study, J. Am. Coll. Cardiol., 58 (2011), 1989-1997. doi: 10.1016/j.jacc.2011.06.066
![]() |
[11] |
J. K. Min, B. K. Koo, A. Erglis, J. H. Doh, D. V. Daniels, S. Jegere, et al., Effect of image quality on diagnostic accuracy of noninvasive fractional flow reserve: results from the prospective multicenter international DISCOVER-FLOW study, J. Cardiovasc. Comput. Tomogr., 6 (2012), 191-199. doi: 10.1016/j.jcct.2012.04.010
![]() |
[12] |
J. K. Min, D. S. Berman, M. J. Budoff, F. A. Jaffer, J. Leipsic, M. B. Leon MB, et al., Rationale and design of the DeFACTO (determination of fractional flow reserve by anatomic computed tomographic angiography) study, J. Cardiovasc. Comput. Tomogr., 5 (2011), 301-309. doi: 10.1016/j.jcct.2011.08.003
![]() |
[13] |
J. K. Min, J. Leipsic, M. J. Pencina, D. S. Berman, B. K. Koo, C. van Mieghem, et al., Diagnostic accuracy of fractional flow reserve from anatomic CT angiography, JAMA, 308 (2012), 1237-1245. doi: 10.1001/2012.jama.11274
![]() |
[14] |
R Nakazato, H. B. Park, D. S. Berman, H. Gransar, B. K. Koo, A. Erglis, et al., Noninvasive fractional flow reserve derived from computed tomography angiography for coronary lesions of intermediate stenosis severity results from the DeFACTO study, Circ. Cardiovasc. Imaging, 6 (2013), 881-889. doi: 10.1161/CIRCIMAGING.113.000297
![]() |
[15] |
J. Leipsic, T. H. Yang, A. Thompson, B. K. Koo, G. B. J. Mancini, C. Taylor, et al., CT angiography (CTA) and diagnostic performance of noninvasive fractional flow reserve: results from the determination of fractional flow reserve by anatomic CTA (DEFACTO) study, AJR. Am. J. Roentgenol., 202 (2014), 989-994. doi: 10.2214/AJR.13.11441
![]() |
[16] |
S. Gaur, S. Achenbach, L. Leipsic, L. Mauri, H. G. Bezerra, Jensen JM, et al., Rationale and design of the HeartFlowNXT (heartflow analysis of coronary blood flow using CT angiography: next steps) study, J. Cardiovasc. Comput. Tomogr., 7(2013), 279-288. doi: 10.1016/j.jcct.2013.09.003
![]() |
[17] |
B. L. Nørgaard, J. Leipsic, S. Gaur, S. Seneviratne, B. S. Ko, H. Ito, et al., Diagnostic performance of noninvasive fractional flow reserve derived from coronary computed tomography angiography in suspected coronary artery disease: The NXT trial (analysis of coronary blood flow using CT angiography: next steps), J. Am. Coll. Cardiol., 63 (2014), 1145-1155. doi: 10.1016/j.jacc.2013.11.043
![]() |
[18] |
T. Miyoshi, K. Osawa, H. Ito, S. Kanazawa, T. Kimura, H, Shiomi, et al., Non-invasive computed fractional flow reserve from computed tomography (CT) for diagnosing coronary artery disease, Circ. J., 79 (2015), 406-412. doi: 10.1253/circj.CJ-14-1051
![]() |
[19] |
K. Tanaka, H. G. Bezerra, S. Gaur, G. F. Attizzani, H. E. Bøtker, M. A. Costa, et al., Comparison between nNon-invasive (coronary computed tomography angiography derived) and invasive-fractional flow reserve in patients with serial stenoses within one coronary artery-A NXT trial substudy, Ann. Biomed. Eng., 44 (2016), 580-589. doi: 10.1007/s10439-015-1436-y
![]() |
[20] |
C. X. Tang, C. Y. Liu, M. J. Lu, U. J. Schoepf, C. Tesche, R. R. Bayer, et al., CT FFR for ischemia-specific CAD with a new computational fluid dynamics algorithm: a Chinese multicenter study, JACC Cardiovasc. Imaging, 13 (2020), 980-990. doi: 10.1016/j.jcmg.2019.06.018
![]() |
[21] |
L. D. Rasmussen, S. Winther, J. Westra, C. Isaksen, J. A. Ejlersen, L. Brix, et al., Danish study of non-Invasive testing in coronary artery disease 2 (Dan-NICAD 2): Study design for a controlled study of diagnostic accuracy, Am. Heart. J, 215 (2019), 114-128. doi: 10.1016/j.ahj.2019.03.016
![]() |
[22] | J. M. Carson, S. Pant, C. Roobottom, R. Alcock, Non-invasive coronary CT angiography-derived fractional flow reserve: A benchmark study comparing the diagnostic performance of four different computational methodologies, Int. J. Numer. Method. Biomed. Eng., 17 (2019), e3235. |
[23] |
J. S. Choy, G. S. Kassab. Scaling of myocardial mass to flow and morphometry of coronary arteries, J. Appl. Physiol., 104 (2008), 1281-1286. doi: 10.1152/japplphysiol.01261.2007
![]() |
[24] |
C. J. Arthurs, K. D. Lau, K. N. Asrress, S. R. Redwood, C. A. Figueroa, A mathematical model of coronary blood flow control: simulation of patient-specific three-dimensional hemodynamics during exercise, Am. J. Physiol. Heart Circ. Physiol., 310 (2016), 1242-1258. doi: 10.1152/ajpheart.00517.2015
![]() |
[25] | Z. Duanmu, W. W. Chen, H. Gao, X. L. Yang, X. Luo, N. A. Hill, A one-dimensional hemodynamic model of the coronary arterial tree, Front. Physiol., 10 (2019), 853. |
[26] | Z. Duanmu, M. Yin, X. Fan, X. Yang, X. Luo, A patient-specific lumped-parameter model of coronary circulation, Sci. Rep., 8 (2018), 1-10. |
[27] |
Y. Huo, G. S. Kassab, The scaling of blood flow resistance: from a single vessel to the entire distal tree, Biophys. J., 96 (2009), 339-346. doi: 10.1016/j.bpj.2008.09.038
![]() |
[28] |
J. M. Zhang, L. Zhong, T. Luo, A. M. Lomarda, Y. Huo, J. Yap, et al., Simplified models of non-invasive fractional flow reserve based on CT images, PLoS One, 11 (2016), e0153070. doi: 10.1371/journal.pone.0153070
![]() |
[29] |
M. Abe, H. Tomiyama, H. Yoshida, D. Doba, Diastolic fractional flow reserve to assess the functional severity of moderate coronary artery stenoses: comparison with fractional flow reserve and coronary flow velocity reserve, Circulation, 102 (2000), 2365-2370. doi: 10.1161/01.CIR.102.19.2365
![]() |
[30] | M. Yang, X. Shen, S. Chen, Assessment of the effect of pulmonary hypertension on right ventricular volume and free wall mass by dynamic three-dimensional voxel imaging of echocardiography, Chin. J. Uitrasonography, 7 (2000), 401-404. |
[31] |
C. H. Lorenz, E. S. Walker, V. L. Morgan, S. S. Klein, T. P. Graham, Normal human right and left ventricular mass, systolic function, and gender differences by cine magnetic resonance imaging, J. Cardiovasc. Magn. Res., 1 (1999), 7-21. doi: 10.3109/10976649909080829
![]() |
[32] | P. Sharma, L. Itu, X. Zheng, A. Kamen, D. Bernhardt, C. Suciu, et al., A framework for personalization of coronary flow computations during rest and hyperemia, Ann. Int. Conf. IEEE Eng. Med. Biol. Soc., 2012, 6665-6668. |
[33] | L. Itu, S. Rapaka, T. Passerini, B. Georgescu, C. Schwemmer, M. Schoebinger, et al., A machine-learning approach for computation of fractional flow reserve from coronary computed tomography, J. Appl. Physiol., 121 (2018), 42-52. |
[34] |
H. Anderson, M. Stokes, M. Leon, S. Abu-Halawa, Y. Stuart, R. Kirkeeide, Coronary artery flow velocity is related to lumen area and regional left ventricular mass, Circulation, 102 (2000), 48-54. doi: 10.1161/01.CIR.102.1.48
![]() |
[35] | Y. X. Zhao, J. M. Liu, D. G. Xu, X. B. Yan, L. C. Lu, S. Z. Xiao, et al., Population based study of change trend of the ratio of diastolic to systolic duration during exercise, Chin. J. Appl. Physiol., 29 (2013), 134-136. |
[36] |
N. G. Uren, J. A. Melin, B. De Brunye, W. Wijns, T. Baudhuin, P. G. Camici, Relation between myocardial blood flow and the severity of coronary-artery stenosis, N. Engl. J. Med., 330 (1994), 1782-1788. doi: 10.1056/NEJM199406233302503
![]() |
[37] |
C. D. Murray, The physiological principle of minimum work I. the vascular system and the cost of blood volume, Proc. Natl. Acad. Sci., 12 (1926), 207-214. doi: 10.1073/pnas.12.3.207
![]() |
[38] |
C. Seiler, K. L. Gould, R. L. Kirkeeide, Basic structure-function relations of the epicardial coronary vascular tree. Basis of quantitative coronary arteriography for diffuse coronary artery disease, Circulation, 85 (1992), 1987-2003. doi: 10.1161/01.CIR.85.6.1987
![]() |
[39] |
C. Seiler, R. L. Kirkeeide, K. L. Gould, Measurement from arteriograms of regional myocardial bed size distal to any point in the coronary vascular tree for assessing anatomic area at risk, J. Am. Coll. Cardiol., 21 (1993), 783-797. doi: 10.1016/0735-1097(93)90113-F
![]() |
[40] |
K. E. Lee, S. S. Kwon, Y. C. Ji, E. S. Shin, J. H. Choi, S. J. Kim, et al., Estimation of the flow resistances exerted in coronary arteries using a vessel length-based method, Pflugers. Arch., 468 (2016), 1-10. doi: 10.1007/s00424-015-1762-9
![]() |
[41] |
S. Sakamoto, S. Takahashi, A. U. Coskun, M. I. Papafaklis, A. Takahashi, S. Saito, et al., Relation of distribution of coronary blood flow volume to coronary artery dominance, Am. J. Cardiol., 111 (2013), 1420-1424. doi: 10.1016/j.amjcard.2013.01.290
![]() |
[42] |
C. A. Bulant, P. J. Blanco, G. D. Maso Talou, C. G. Bezerra, P. A. Lemos, R. A. Feij, A head-to-head comparison between CT- and IVUS-derived coronary blood flow models, J. Biomech., 51 (2017), 65-76. doi: 10.1016/j.jbiomech.2016.11.070
![]() |
[43] |
E. Kato, S. Fujimoto, K. K. Kumamaru, Y. O. Kawaguchi, T. Dohi, C. Aoshima, et al., Adjustment of CT-fractional flow reserve based on fluid-structure interaction underestimation to minimize 1-year cardiac events, Heart Vessels, 35 (2020), 162-169. doi: 10.1007/s00380-019-01480-4
![]() |
[44] | H. Mejía-Rentería, J. M. Lee, F. Lauri, N. W. van der Hoeven, G. A de Waard, F. Macaya, et al., Influence of microcirculatory dysfunction on angiography-based functional assessment of coronary stenoses, JACC Cardiovasc. Interv., 23 (2018), 741-753. |
[45] | J. J. Layland, R. J. Whitbourn, A. T. Burns, J. Somaratne, G. Leitl, A. I. Macisaac et al., The index of microvascular resistance identifies patients with periprocedural myocardial infarction in elective percutaneous coronary intervention, J. Interv. Cardiol., 98 (2012), 1492-1497. |
[46] |
A. Erkol, S. Pala, C. Kırma, V. Oduncu, C. Dündar, A. Izgi, et al., Relation of circulating osteoprotegerin levels on admission to microvascular obstruction after primary percutaneous coronary intervention, Am. J. Cardiol., 107 (2011), 857-862. doi: 10.1016/j.amjcard.2010.10.071
![]() |
1. | Wei He, Long Yu, Wang Qin, Yuan Wang, Keqiang Wang, Weifeng Guo, Shengzhang Wang, A modified method of noninvasive computed tomography derived fractional flow reserve based on the microvascular growth space, 2022, 221, 01692607, 106926, 10.1016/j.cmpb.2022.106926 | |
2. | Xue Wang, Jincheng Liu, Na Li, Junling Ma, Mingyan Chen, Yili Feng, Bao Li, Jian Liu, Youjun Liu, Liyuan Zhang, Left and right coronary artery blood flow distribution method based on dominant type, 2023, 2040-7939, 10.1002/cnm.3681 | |
3. | Long Yu, Weifeng Guo, Wei He, Wang Qin, Mengsu Zeng, Shengzhang Wang, A novel method for calculating CTFFR based on the flow ratio between stenotic coronary and healthy coronary, 2023, 01692607, 107469, 10.1016/j.cmpb.2023.107469 | |
4. | Honghui Zhang, Xiaorui Song, Rile Wu, Na Li, Qianwen Hou, Jinjie Xie, Yang Hou, Aike Qiao, A novel method for noninvasive quantification of fractional flow reserve based on the custom function, 2023, 11, 2296-4185, 10.3389/fbioe.2023.1207300 | |
5. | Honghui Zhang, Rile Wu, Ning Yang, Jinjie Xie, Yang Hou, Retracted: Research on individualized distribution approach of coronary resting blood flow for noninvasive calculation of fractional flow reserve, 2023, 240, 01692607, 107704, 10.1016/j.cmpb.2023.107704 |