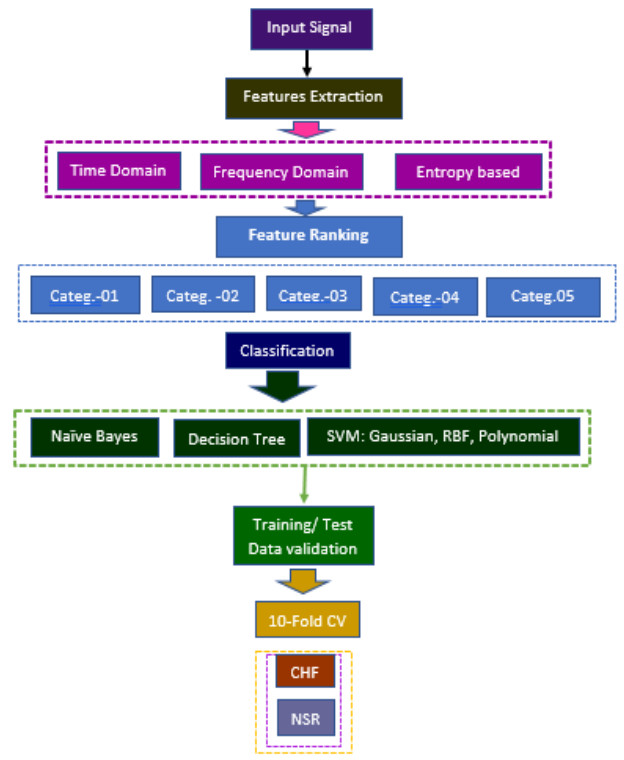
In this paper, we will prove that a closed Möbius minimal and Möbius isotropic hypersurface without umbilic points in the unit sphere S5 is Möbius equivalent to either the torus S2(1√2)×S2(1√2)→S5 or the Cartan minimal hypersurface in S5 with four distinct principal curvatures.
Citation: Bangchao Yin, Shujie Zhai. Classification of Möbius minimal and Möbius isotropic hypersurfaces in S5[J]. AIMS Mathematics, 2021, 6(8): 8426-8452. doi: 10.3934/math.2021489
[1] | Faisal Mehmood Butt, Lal Hussain, Anzar Mahmood, Kashif Javed Lone . Artificial Intelligence based accurately load forecasting system to forecast short and medium-term load demands. Mathematical Biosciences and Engineering, 2021, 18(1): 400-425. doi: 10.3934/mbe.2021022 |
[2] | Xin Jing, Jungang Luo, Shangyao Zhang, Na Wei . Runoff forecasting model based on variational mode decomposition and artificial neural networks. Mathematical Biosciences and Engineering, 2022, 19(2): 1633-1648. doi: 10.3934/mbe.2022076 |
[3] | Jing Cao, Dong Zhao, Chenlei Tian, Ting Jin, Fei Song . Adopting improved Adam optimizer to train dendritic neuron model for water quality prediction. Mathematical Biosciences and Engineering, 2023, 20(5): 9489-9510. doi: 10.3934/mbe.2023417 |
[4] | Fengyong Li, Meng Sun . EMLP: short-term gas load forecasting based on ensemble multilayer perceptron with adaptive weight correction. Mathematical Biosciences and Engineering, 2021, 18(2): 1590-1608. doi: 10.3934/mbe.2021082 |
[5] | Keruo Jiang, Zhen Huang, Xinyan Zhou, Chudong Tong, Minjie Zhu, Heshan Wang . Deep belief improved bidirectional LSTM for multivariate time series forecasting. Mathematical Biosciences and Engineering, 2023, 20(9): 16596-16627. doi: 10.3934/mbe.2023739 |
[6] | Rashad A. R. Bantan, Zubair Ahmad, Faridoon Khan, Mohammed Elgarhy, Zahra Almaspoor, G. G. Hamedani, Mahmoud El-Morshedy, Ahmed M. Gemeay . Predictive modeling of the COVID-19 data using a new version of the flexible Weibull model and machine learning techniques. Mathematical Biosciences and Engineering, 2023, 20(2): 2847-2873. doi: 10.3934/mbe.2023134 |
[7] | Favour Adenugba, Sanjay Misra, Rytis Maskeliūnas, Robertas Damaševičius, Egidijus Kazanavičius . Smart irrigation system for environmental sustainability in Africa: An Internet of Everything (IoE) approach. Mathematical Biosciences and Engineering, 2019, 16(5): 5490-5503. doi: 10.3934/mbe.2019273 |
[8] | Rami AL-HAJJ, Mohamad M. Fouad, Mustafa Zeki . Evolutionary optimization framework to train multilayer perceptrons for engineering applications. Mathematical Biosciences and Engineering, 2024, 21(2): 2970-2990. doi: 10.3934/mbe.2024132 |
[9] | Yueming Zhou, Junchao Yang, Amr Tolba, Fayez Alqahtani, Xin Qi, Yu Shen . A Data-Driven Intelligent Management Scheme for Digital Industrial Aquaculture based on Multi-object Deep Neural Network. Mathematical Biosciences and Engineering, 2023, 20(6): 10428-10443. doi: 10.3934/mbe.2023458 |
[10] | Weibin Jiang, Xuelin Ye, Ruiqi Chen, Feng Su, Mengru Lin, Yuhanxiao Ma, Yanxiang Zhu, Shizhen Huang . Wearable on-device deep learning system for hand gesture recognition based on FPGA accelerator. Mathematical Biosciences and Engineering, 2021, 18(1): 132-153. doi: 10.3934/mbe.2021007 |
In this paper, we will prove that a closed Möbius minimal and Möbius isotropic hypersurface without umbilic points in the unit sphere S5 is Möbius equivalent to either the torus S2(1√2)×S2(1√2)→S5 or the Cartan minimal hypersurface in S5 with four distinct principal curvatures.
Heart Rate Variability (HRV) is a convenient non-invasive tool for the measurement of autonomous cardiac function by sympathetic and parasympathetic branches of the nervous system i.e. to introduce electrocardiography (ECG) time series analysis [1] and complex systems and the technology of variability analysis [2]. Conventional techniques used for the quantification of various HRV signals by employing linear strategies have represented that decrease in the variability have direct association with increase in the heart failure mortality. However, in some situations the HRV data cannot be evaluated by using linear methods [3].
In the recent studies, the researchers developed and employed different techniques for the detection of congestive heart failure (CHF) subjects using Inter-beat Interval (IBI) time series extracted from ECG signals including symbolic time series was used by [4] to study the dynamics of interbeat heart interval [5], threshold dependent symbolic entropy to classify healthy and pathological subjects, wavelet based soft decision technique to detect congestive heart failure [6] and combined classical HRV indices with wavelet entropy to detect congestive heart failure [3]. Isler and Kuntalp [3] considered wavelet entropy features and HRV features along with KNN classifier to distinguish regular subjects and CHF subjects. Hossen and Al-Ghunaimi [6], used wavelet-based soft determination methodology for estimating the spectral density of average power of IBI time series for screening of CHF subjects. Thuraisingham [7] proposed a novel technique using features from KNN classification method and second order difference plot of IBI time series to distinguish CHF and normal subjects. Yu and Lee [8] proposed a mutual information based featured to detect congestive heart failure. Pecchia et al. [9] proposed short term power features along with very simple threshold based classifier for the detection of CHF. Aziz et al. [5] used symbolic time series analysis for distinguishing healthy subjects from CHF patients. Altan et al. [10] extracted features from IBI time series using Hilbert-Huang transform and multilayer perceptron neural network used to classify normal subjects, CHF and coronary artery disease subjects. Awan et al. [4] introduced multiscale simplified improved Shannon entropy for extracting features from IBI time and used different classifiers for discriminating NSR and CHF subjects. Choudhary et al. [11] proposed grouped horizontal visibility graph entropy for discriminating normal, CHF and atrial fibrillation subjects. Recently, Isler et al. [12] applied Multi-stage classification of congestive heart failure based on short-term heart rate variability. Moreover, Narin et al. [13] predicted paroxysmal atrial fibrillation based on short-term heart rate variability. The researchers [14] tested the irregularity or very short electrocardiogram (ECG) signals as a method for predicting a successful defibrillation in patients with ventricular fibrillation.
The machine learning algorithms rely on the type and relevancy of feature extraction approach. The classification efficiency can be enhanced by extracting the most relevant features which is a hot topic in machine learning and signal & image processing problems. In the past, researchers have obtained numerous characteristics from different physiological signals and systems. Wang et al. [15] proposed multi-domain feature extracting approach to identify the accurate epileptic seizure detection. Hussain [31] proposed multi-modal (multi-domain, and nonlinear) feature extracting approach for epileptic seizure detection, arrhythmia detection [16] and Rathore et al. proposed hybrid feature to detect colon cancer [17]. After extracting the features, all features are not contributing equally, their importance can be determined by ranking the features based on different ranking algorithms. The management for feature selection and follow-up for the relevant features information processing, the Feature Importance Ranking (FIR) plays an important role in the area feature selection methods based on mutual information criteria of max dependency [18], feature ranking algorithm to detect cardiac arrythmia [19], feature ranking to reconstruct dynamical network [20], feature selection to assess thyroid cancer pronosis [22], and Multi-objective-based radiomic feature selection for lesion malignancy classification [21]. The main objective of the FIR is to arrange the features in accordance with their relative significant value. Depending to which the labels on training samples are used, all methods are divided into supervised and unsupervised approaches, whereas the supervised process labels are used [21]. From a technical point of view, some approaches, such as the Wilcoxon rank-sum test and t-test, use statistical analysis and class separability parameters to measure inter-feature relationships, and some other approaches investigate reciprocal knowledge [18], sparse regression, spectral analysis, and include some classification efficiency into accounts and the selection of classifiers for machine learning [21]. Leguia et al. [20] used the Random Forest and Relief-F to ranked the feature importance of each node to predict the value of each other node. Karnan et al. [19] proposed feature ranking score (FRS) algorithms on different statistical parameters to select the optimal parameters for classification of signals from public domain MIT-BIH arrhythmia data. These optimal features are provided to the least square support vector. Mourad et al. [22] combined the features selection algorithms and machine learning algorithms (Kruskal-Wallis’ analysis, Relief-F and Fisher’s discriminant ratio) to analyse the specific attributes of de-identified thyroid cancer patients in the SEER sample.
In this study, we employed FIR for extracting most contributing factors based on the feature ranking categorized (1 to 5) for detection of healthy and CHF subjects for clinical decision making. The category value 1 depicts that feature is most important and the value 5 reveals that feature least important. Moreover, the greatest ROC value indicate that feature is more important and as the ROC value decreases, the importance of the feature deceases accordingly. We first extracted multimodal features from CHF and NSR subjects and then ranked them based on EROC and random classifier slop [23], which ranks features dependent on the criterion for class separability of the region between EROC and Classifier at periodic intervals slope.
The RR time series interval data were taken from the Physionet databases [24]. Data from the Normal Sinus Rhythm (NSR) subject, Congestive Heart Failure (CHF) subjects and Atrial Fibrillation (AF) subjects in the cardiac interval (RR interval) time series were analysed [24]. The heart activity data from NSR subjects had been taken from 24-Hour recordings of 72 subjects by using Holter monitor system. The dataset consist of 35 Males and 37 Females (54 from the NSR subjects of the RR-interval and 18 from the Normal sinus rhythm RR internal Database used in the study [25]. The age range of 20–78 years for the measured population was 54.6 ± 16.2 (mean ± SD). At 128 Hz, ECG data was sampled. The CHF group consisted of 44 participants aged from 22–78 years, 29 Males and 15 Females aged 55.5 ± 11.4 and the data from the RR CHF interval and 15 years from the Congestive Heart Failure RR interval Database used in the study [26] were collected for 29 CHF subjects [24]. According to the practical classification system of the New York Heart Association (NYHA), CHF subjects can be divided into four categories. This method categorizes patients by the signs of the patient's regular behaviour and quality of life. In this study we used 20,000 samples of each subject to distinguish CHF from NSR patients.
After extracting features, another important criterion is also to get the most appropriate features with high ranks. This can be done by ranking the features based on various criteria. Firstly, from CHF and NSR subjects, we extracted multimodal features. We then ranked the features to differentiate the CHF from NSR subjects based on receiver operating curve (ROC) value. We then applied the machine learning classification techniques based on the different inputs of the ranked features to evaluate the detection performance.
The Figure 1 shows the schematic diagram for CHF detection. In the first step, we extracted the general multimodal features from NSR subject and the CHF subjects. In the second step, we ranked the extracted features based on ROC values. In the third step, we employed different machine learning algorithms such as Decision Tree, SVM along with its kernels tricks, and Naïve Bayes approach on five different categories of ranked features i.e. Category 01 with all extracted features, Category 02 with top 05 ranked features with higher ROC values obtained, Category 03 top nine ranked features, Category 04 last thirteen ranked features and Category 05 the last two ranked features with very low ROC values. Finally, for testing and training of data validation, we employed standard 10-fold cross validation.
The time and frequency domain approaches are commonly used to collect the time series and heart spectral dynamics control of these signals vibrations to quantify the heterogeneity of physiological signals (i.e. EEG or ECG) caused by various pathologies. The techniques of the time domain are used for the tracking of the short-term, medium-term and long-term fluctuations of physiological signals and processes, while preserving the effects of various spectrums. The definition for patients suffering from various heterogeneity dysfunctions is detailed in (Task Force of the European Society of Cardiology and the North American Society of Pacing and Electrophysiology and Electrophysiology 1996; Seely and Macklem 2004) including heart rate variability in insomnia patients [27], Ultra-shortened time-domain HRV parameters [28], evaluating the homeostasis assessment model insulin resistance and the Cardiac autonomic System in bariatric surgery patients [29] and short-term measurement of heart rate variability during spontaneous breathing in people with chronic obstructive pulmonary disease [30]. etc. We have used same time domain, frequency domain, nonlinear entropy based and wavelet based features in previous studies to detect epileptic seizure [31], congestive heart failure [32] and arrhytymia detection [16].
Biological signals are production of beating heart and several muscle interacting components that display complex patterns variation and rhythms on monitoring devices. To analyse the fundamental mechanisms of these processes, these rhythmical shifts and patterns provide very valuable secret details. Extracting useful knowledge using conventional methods of data mining is impractical. The complexity of the physiological processes that are degraded by ageing and disease consists of systemic components and coupling between them. The researchers in the past applied various complexity based methods such as epileptic seizure detection using multi-modal features [31], seizure detection using symbolic entropy [33], lung cancer detection based on refined fuzzy entropy [34], arrythmia detection using refined fuzzy entropy [16], electroencephalographic (EEG) signals with motor movement using multi scale sample entropy [35], EEG alcoholic and control subjects using multiscale entropy with KD tree algorithmic approach [36], regression analysis to detect seizure [37]. The healthy subjects are more complex than pathological subjects. In the healthy subjects, all the structural elements and integrated functions within the structural elements are properly functional and linked for inter-communication, thus increasing their complexity computation value and entropy values. But, due to the weakening of the coupling between the structural elements, the computed complexity value and entropy value of the diseased subjects is decreased.
Pincus in 1991 proposed approximate entropy (ApEn) [38] to compute the regularity presence in the bio-signal time series recording data. The measurement of the entropy indicates that the probability of related or similar patterns does not repeat in observation. Mathematically,
ApEn(m,r,N)=Cm(r)−Cm+1(r) | (2.1) |
The Cm(r)and Cm+1(r) are being computed as detailed in [36]. Two parameters are set to measure the average entropy, i.e. m, which is the length of the window, and r, the criterion of similarity. We selected m = 3 and r = 0.15 times the standard deviation of data in this analysis as given in [38].
Sample entropy (SampEn) as proposed by [39], which is a revamped form the approximate entropy. In contrast to the average entropy, sample entropy is more stable then approximate entropy since it is independent to estimate the randomness of data duration and trouble-free execution. Recently, researchers used a sample entropy version based on the KD tree algorithmic approach, which is more stable in terms of time and space complexity as detailed in [36].
In 1975, Bentley design a binary tree algorithm known as the K-Dimensional (KD) space partition tree. A rectangle “Bv” is connected with each of its 'v' nodes. The 'v' would be the leaf node if “Bv” does not have any point in its interior. In other examples, by creating a vertical and horizontal line such that each rectangle comprises at no more than half of the lines, “Bv” can be separated into two rectangles. Details of the KD tree algorithm are computed by Hussain et al [36]. Using the following steps, the complexity of time and space is minimized.
Step 1. Original time series of RR-Interval was transformed to spaced points set from {X}={x1,x2,x3,….xN} be a time series of length N. The time and memory cost are O (N).
Step 2. Using N-m points of the series for which total cost is O (N log N) and memory is O(N)), the K-dimensional tree is constructed. Here construct time is O (N log N) for k-d tree.
Step 3. Range query: The time cost is NO(N11d) for N queries for d-dimensional k-d search and the memory cost are O(N). Where O(N11d) is search time for k-d tree.
Wavelet-based entropic measurements were also computed by researchers in the past to identify the nonlinearity presence in the results. The most widespread wavelet entropy techniques [40] include Shannon, Threshold, Log Energy, Sure and Norm etc. Shannon entropy [40] was used by calculating wavelet coefficients which is created from the wavelet packets (WPT) to calculate the signal intensity, where maximum values indicate a high uncertainty in the CHF or NSR subjects and hence greater complexity. In addition, wavelet entropy was used [41] to capture the underlying dynamical mechanism connected with the bio-signal. The entropy 'E' must be a cost function of additive information such that E (0) = 0.
E(S)=∑iE(Si) | (2.2) |
Where S is a signal and (Si) are signal coefficients on an orthonormal basis. The function E (S) is defined as wavelet entropy as expressed inequation (5).
The Claude Shannon first suggested the entropy of Shannon in 1948 [42] and is most commonly used in information science. In addition, it is the measurement of the vulnerability associated with a randomness of the data space. Shannon entropy precisely estimate the predicted value of the results found in a packet. We can describe the Shannon entropy of a random variable S as follows:
E(S)=−n∑i=1S2ilog2(S2i) | (2.3) |
Where Si represents coefficients of signal S in an orthonormal basis. If the entropy value is greater than one, the component has a potential to reveal more information about the signal and it needs to be decomposed further in order to obtain simple frequency component of the signal [43]. By using the entropy, it gave a useful criterion for comparing and selection the best basis.
This entropy measure was proposed by [44] can mathematically defined such as:
E(S)=∑i|Si|pN | (2.4) |
Where p is the power, the terminal node signal must be 1≪P<2and (Si) I is the terminal waveform signal.
E(Si) = 1 if |Si| > p and 0 elsewhere so E(s) = # {i such that |Si | > p} is the number of time instants when the signal is greater than a threshold p.
The threshold entropy value was determined using a value of 0.2.
The threshold of the parameter P and the values of P ≥ 0 are used.
E(s)=n−#{isuchthat|si|≤p}+∑imin(si2,p2) | (2.5) |
Where, the discrete wavelet entropy E is a real number, s is the terminal node signal and (si) i the waveform of terminal node signals. In Sure entropy, p is a positive threshold value and must be p ≥ 2 [45].
The entropy of Sure was measured at threshold 3.
The P is used in Normal Entropy as the power and value of P ≥ 1. The intensity in lp norm entropy is:
E(si)=|si|p | (2.6) |
soE(s)=∑i|si|pi=||S||pp |
The entropy of the norm was estimated at 1.1 with power.
The wavelet norm entropy represents the ordering of nonstationarity of time series fluctuation.
HlogEn(B)=−∑N−1i=0(log2(Pi(B)))2 | (2.7) |
Where Pi(B) denotes the function of probability distribution and is a logarithmic amount of the distribution square of these probabilities.
Feature ranking algorithms are mostly used for ranking features independently without using any supervised or unsupervised learning algorithm. A specific method is used for feature ranking in which each feature is assigned a scoring value, then selection of features will be made purely on the basis of these scoring values [46]. The finally selected distinct and stable features can be ranked according to these scores and redundant features can be eliminated for further classification. To perform this step, feature selection algorithms such as wrapper method and filter method can be used. As filter method is an unsupervised technique that analyse the inherent distribution properties of the features, on the other hand wrapper method correlates the features properties with the class labels [47]. In the past, multiple experiments studies have shown that every well-known function discovery algorithm that exposes the rating to errors has been used to pick features [48]. Feature ranking can be affected by the selection of algorithm for feature selection for classification purpose.
Radiomic feature ranking is a type of feature ranking method that is used to select features based on their high scoring values. The algorithm described in [49] selects the features that are showing minimum correlation with each other. However, Laplacian score [50] calculates a scoring value for each individual feature which shows the locality preserving power of a feature. In greedy feature selection algorithm [51], for all the chosen features a nearest neighbour graph is used and reconstruction error is repeatedly calculated for assigning ranks to the selected features subset. Mitra et al. [52] proposed a minimum information index for feature ranking. Multi-cluster feature selection (MCFS) [53] algorithm is proposed to measure the correlations between various features and then select and rank the features accordingly. Zeng and Cheung [54] proposed another clustering algorithm that takes into account the correlation between each feature by employing Local Learning Based Clustering (LLC) method. Zhao et al. [55] proposed a normalized Laplacian matrix method obtained from the similarity graph of pair-wise features.
Feature selection phase can be repeated by using wrapper method [56]. Relief-F algorithm [57] sort the features into one group that have similar values for the closest neighbours with the same binary class and higher characteristics based on the associated values that shows various values for the closest neighbours in various groups. Fisher Score [47] is another algorithm which assigns a scoring value to each feature by calculating the intra-class variance and inter-class separation ratio. Feature based Neighbourhood Component Analysis (FNCA) [56] learns weights of features to minimize the objective function which is used to calculate the cumulative lack of regression over training data leaves one out.. The Infinite Latent Feature Selection (ILFS) [54] technique is also an impressive algorithm that is used in rank assigning to the features by estimating the relevancy using conditional probability of all subsets of the features. Features Selection via Eigenvector Centrality [58] is another technique used for ranking the features by connecting them to a graph of clustering, then discovering the correlation between separate pairs of features. Concave Optimization [59] is another novel method that is used in feature selection and ranking. Two function classes are defined in this approach by using a separate plane that is generated by using a series of features that can discern between a pair of classes.
The above-mentioned algorithms can be used for ranking the radiomic and any other features individually by usage of filter methods and wrapping techniques. The scoring values that is assigned to each feature by ranking group methods to get the final ranking scoring values of all the functions, they were summed. To get more precise scoring values, the key objective is averaging the features scoring value and to give equal weight to all rating algorithms. Then top 25 selected features having average scoring values can be calculated from the filter methods and wrapper methods [47].
Let us consider an example that illustrates the feature ranking methodology [20]; we have an equation
y=f(x1,x2,…)=x21+x2+2 | (2.8) |
Assume that f is an unknown function, but y depends on several variables of xi is known. Simply we can say that, xi represents the features and y represents the target variable. This task can be solved by employing machine learning algorithm M,i.e.
f≈ˆf=M(D) | (2.9) |
Where, D represents the data set and f shows the prediction model for any observation (x1,x2,x3) can be used for predicting the value of y,ˆy=ˆf(x1,x2,x3). Now, consider a data set D comprised of L attributes tuples (x1,x2,x3;y) and we want to reconstruct f. Remember that, in data we have x3 feature, which does not influence y, because only available data is collected via features selection and ranking that “may or may not” influence the target variable.
To check the feature importance, there are more than 30 algorithms developed. In this study, we computed ROC for feature importance ranking (FIR) as detailed in [60]. This method ranks the features based on the class separability criteria of the area between the empirical receiver operating characteristic curve (EROC) and the random classifier slop [23]. In this study, we extracted 22 multimodal features from CHF and NSR subjects. We then ranked these features based on above criteria, and Figure 2 below sorted the multimodal features based on their importance obtained. We then categorized these features based on the ROC values to further classify the CHF and NSR subjects to see the overall detection performance based on ranked features instead of using all the features. The top 5 features even show the higher detection than 82%. This will further help the clinicians to make the decision for future diagnosis and treatment of the patients. The highest ROC value indicates the highest ranked and highly important feature and as the ROC value decreased the feature importance decreased accordingly. In this study, the feature importance is depicted in descending order based on the ROC values obtained. Figure 2 shows the importance of ranked Multimodal features based on the class separability criteria of the area between EROC and random classifier slope.
SVM is one of the most versatile approaches used for classification purposes of supervised learning techniques. SVM has recently been used excellently for concerns of graphical pattern recognition [61], Artificial Intelligence (Machine Learning) [62] and Computer aided medical diagnosis Health problems [63]. In addition, SVM is used in numerous applications in several field, such as identification and detection, text recognition, retrial of content-based images, bioinformatics, voice recognition, etc. In infinite or high dimensional space, SVM creates a hyperplane or series of hyper-plan that could be used to classify a successful separation while using this hyper-plane that has the greatest distance to the closest training instances in each class (also called as the functional margin), typically the greater the margin implies the classifier's relatively generalization classification error. SVM attempts to determine the hyper-plane that provides the training example with the greatest minimum width. This concept is also known as margin in SVM theory. The optimum margin is obtained for the maximised hyperplane. SVM has another significant function that offers the higher efficiency of generalization. Basically, SVM is a two-class classifier that relies on nonlinear training instances or a maximum dimension to transform data into a hyperplane. Let us define x.w+b=0 a hyperplane, where w is its normal. The linearly separable instances is labelled as:
{xi,yi},xi∈RNd,yi∈{−1,1},i=1,2,....,N |
Here yi is the class label of two (Positive, Negative) class of SVM. The optimal limit with full margin is achieved by decreasing the objective function. i.e. E=w2subject to:
xi.w+b≥1foryi=+1 |
xi.w+b≤1foryi=−1 | (2.10) |
This can be incorporated into a series of disparities as follows:
(xi.b+b)yi≥1foralli |
Decision Tree determined the series regularity and similarities of the dataset which can be verified by the classifier and grouped into different classes. Liu et al. [64] used DT to assign data based on the option of an attribute that maximizes and improves the division of data. The characteristics are divided into multiple divisions before the termination conditions are fulfilled. The DT algorithm is mathematically developed using the following equations:
¯X={X1,X2,X3,……..Xm}T |
Xi={x1,x2,x3,……,xij,……xin} |
S={S1,S2,S3,……,Si,……Sm} | (2.11) |
Where m corresponds to the number of observations available, n represents the several independent variable, S use the m-dimension vector of the variable projected from ¯X.Xi is the ith component of autonomous n-dimension variables, x1,x2,x3,……,xij,……xin of the Xi vector pattern and T is the transpose notation.
The aim of DTs is to predict the ¯X observations. It is possible to construct multiple DTs from ¯Xto various precision levels; although, the desirable DT is difficult since search space has a broad parameter dimension. Reasonable algorithms should be built for DT to represent the negotiate-off between precision and complexity. In this situation, the partitioning of the dataset ¯Xusing DT algorithms uses a collection of local ideal decisions on the function instances. Which according to corresponding optimization method, Optimal DT, Tk0 is built.
ˆR(Tk0)=min{ˆR(Tk0)},k=1,2,3,……K |
ˆR(T)=∑kt∈T{r(t)p(t)} | (2.12) |
Where ˆR(T) denotes the uncertainty level during most of the Tk tree misclassification, Tk0 represent the desirable DT that decrease the classification error in the binary tree misclassification, T represent the binary tree ∈, {T1,T2,T3,……Tk,t1}. The tree index is represented by k, tree node by t, root node by t1, error resubstituting by r(t) misclassifying node t, likelihood that p(t) denotes some decrease in node t. The sub-trees of the right and left partitions are denoted by TL and TR. The tree T is created by portioning the feature plan. For larger datasets, there is classification problems as these data sets are these circumstances, the decision tree is a suitable strategy and contains errors. The objects are taken as input and the output in form of yes/ no decision is provided by the algorithm. The decision tree algorithms use Boolean function [65] and sample selection [66]. The decision tree algorithms are used in many applications such as bioinformatics economics, medical diagnoses problems and other scientific situations etc. [67].
Naive Bayes is among the simplest probabilistic classifiers. In many real-world implementations, it also performs remarkably well, considering the firm presumption that, provided the class, all functions are conditionally independent. Pearl's (1988) proposed Bayesian Networks (BNs) are high-level description of distributed probabilities over a set of parameters X={X1,X2,,,,,,,,Xn}used by a learning method. The learning method of the NBs is split into two steps: learning constructs and learning parameters. A directed acyclic graph from the set X is being built by the former. Every node refers to the parameter in the graph, and each Arc represents a probabilistic interaction between two parameters, whereas the Arc path implies the causality direction. The probabilistic node is called the parent of the other node when two nodes are connected by an arc, and another is called the child. To denote both the vector (feature) and its respective node, we use Xi, and Pa(Xi) to denote the parent set of the X-i node. The discovery of probability distributions, class probabilities and conditional probabilities associated with each component is called parameter learning, provided a framework [68].
The KNN classification was built from the need for discriminant analysis where it is unclear or difficult to establish accurate parametric estimates of probability densities. In the world of machine learning, KNN is the most commonly used algorithm for pattern recognition and many other fields used for classification problems. This algorithm is also known as an example-based algorithm (lazy learning). A model or classifier is not created automatically, but all samples of training data are preserved and kept until it is appropriate to identify new observations. This lazy learning algorithm feature makes it easier than eager learning to create a classifier until it is required to classify new observations.
In the world of machine learning, KNN is the most commonly used algorithm for pattern recognition and many other fields used for classification problems [69]. This algorithm is also recognizing as an example-based algorithm. A predictive algorithm is not created automatically, however all samples of training instances are preserved and kept until it is appropriate to identify new observations. This KNN algorithm feature makes it easier and simpler to construct a classifier than eager learning before new insights need to be listed. Where complex data must be modified and revised more easily, this algorithm is even more significant. KNN with various distance metrics was used [70]. The KNN algorithm operates using the Euclidean distance theorem in conjunction with the following steps.
Step I: To train and validate the model, provide the extracted feature set to KNN.
Step II: Measure distance using Euclidean distance formula.
d(xi,yi)=∑ni=1√(xi−yi)2 | (2.13) |
Step III: Sort the values calculated using Euclidean distance using di≤di+1 where i = 1, 2, 3, ...k
Step IV: Depending on the quality of the results, apply the means or vote.
Step V: The K value (i.e. the number of nearest neighbors) depends on the sum and type of the KNN data supplied. The value of k is retained as large for large data, while the value of k is still kept tiny for small data.
For data training and testing formulation of the parameter, the Jack-knife k-fold cross validation methodology was used. 10-fold CVs is used in this research to test the efficiency of classifiers for various methods of extracting features. The most widely used and well-known methodology to test the output of classifiers is the 10-fold CV. The data is divided into 10 folds using 10-fold CV, 9 folds are involved in preparation, and sections of samples of remaining folds are expected based on the 9-fold testing. The research samples in the research fold are purely inaccessible to the qualified models. The entire process is replicated 10 times and is estimated appropriately by each class study. For other CVs, a corresponding approach is used. Finally, the projected labels for unseen samples are used to determine the precision of the designation. For any combination of system parameters, this procedure is repeated, and classification output for the sample has been recorded.
The ROC is graphed against the true positive rate (TPR), i.e. sensitivity and false positive rate (FPR), i.e. the CHF and NSR subjects' specificity values. The mean values of features for NSR subjects are graded as 1 and 0 for CHF subjects. The ROC function is then transferred to this vector, which plots each sample value against the values of specificity and sensitivity. ROC is one of the popular methods of calculating success in order to diagnose and interpret the efficacy of a classifier [71]. The TPR is graphed against the y-axis, and the x-axis is graphed against the FPR. The portion of a square unit is represented by the area under the curve (AUC). Its value varies from 0 to 1. The distinction is shown by AUC > 0.5. The superior diagnostic tool is shown by the greater AUC. TPR represents right positive cases calculated by dividing the total positive cases, while FPR represents negative cases expected as positive, calculated by dividing the total number of negative cases.
In this study we extracted 22 multimodal features i.e. frequency domain features [very low frequency (VLF), ultra-low frequency (ULF), low frequency (LF), high frequency (HF), ratio of LF and HF (LFHF), total power (TP)], , time domain features [standard deviation of the consecutive intervals in each segment (SDNN), Standard deviation of the averages of EEG intervals (SDANN), standard deviation of differences between adjacent time series intervals in each segment (SDSD), square root of the mean squared differences of N successive EEG time series intervals (RMSSD)]; statistical features (Skewness, smoothness, kurtosis, variance, root mean square), Nonlinear entropy features (approximate entropy, sample entropy based on KD tree) and wavelet entropy features (Threshold, Shannon, sure, norm, log energy) from Congestive Heart Failure (CHF) and Normal Sinus Rhythm (NSR) signals. We ranked the features and categorized the ranking based on ROC values obtained Category 01: all ranked features, Category 02: top five ranking features (Wavelet threshold, VLF, Kurtosis, ULF, TP), Category 03: top 09 ranking features (Wavelet threshold, VLF, Kurtosis, ULF, TP, LF, Skewness, SDNN, Variance), Category 04: last 13 ranked features (SDSD, HF, Wavelet sure, RMS, Approx. Entropy, Wavelet Shannon, Wavelet norm, smoothness, wavelet log energy, RMSSD, MSEKD, SDANN, LFHF) and Category 05: last two ranked features (SDANN, LFHF). The feature ranks are categorized on the value of ROC obtained using ranking method. We then employed machine learning techniques such as Decision Tree, Naïve Bayes, SVM Gaussian, RBF and Polynomial. Based on the all multimodal features, the highest performance was obtained using SVM Gaussian with sensitivity (93.06%), specificity (81.82%), PPV (89.33%), NPV (87.80%), accuracy (88.79%), FPR (0.1818) and AUC (0.9441) followed by Naïve Bayes with accuracy (88.79%), AUC (0.9296); SVM RBF with accuracy (87.93%) and AUC (0.9347); SVM polynomial with accuracy (87.93%), AUC (0.9343); decision tree with accuracy (78.45%), AUC (0.9296) as reflected in Table 1.
Method | Sensitivity | Specificity | PPV | NPV | Accuracy | FPR | AUC |
Category 01: All Ranked features | |||||||
Naïve Bayes | 0.8889 | 0.8864 | 0.9275 | 0.8298 | 0.8879 | 0.1136 | 0.9296 |
Decision Tree | 0.8194 | 0.7273 | 0.831 | 0.7111 | 0.7845 | 0.2727 | 0.9296 |
SVM Gaussian | 0.9306 | 0.8182 | 0.8933 | 0.878 | 0.8879 | 0.1818 | 0.9441 |
SVM RBF | 0.9028 | 0.8409 | 0.9028 | 0.8409 | 0.8793 | 0.1591 | 0.9347 |
SVM poly. | 0.9722 | 0.7273 | 0.8537 | 0.9412 | 0.8793 | 0.2727 | 0.9343 |
Category 02: Top five Ranked Features | |||||||
Naïve Bayes | 0.8611 | 0.7727 | 0.8611 | 0.7727 | 0.8276 | 0.2273 | 0.8722 |
Decision Tree | 0.8889 | 0.5 | 0.7442 | 0.7333 | 0.7414 | 0.5 | 0.8722 |
SVM Gaussian | 0.9722 | 0.5909 | 0.7955 | 0.9286 | 0.8276 | 0.4091 | 0.8633 |
SVM RBF | 0.9306 | 0.5909 | 0.7882 | 0.8387 | 0.8017 | 0.4091 | 0.8204 |
SVM poly. | 0.8611 | 0.5455 | 0.7561 | 0.7059 | 0.7414 | 0.4545 | 0.7869 |
Category 03: Top Nine Ranked Features | |||||||
Naïve Bayes | 0.8889 | 0.7727 | 0.8649 | 0.8095 | 0.8448 | 0.2273 | 0.8767 |
Decision Tree | 0.8472 | 0.6364 | 0.7922 | 0.7179 | 0.7672 | 0.3636 | 0.8767 |
SVM Gaussian | 0.8611 | 0.6818 | 0.8158 | 0.75 | 0.7931 | 0.3182 | 0.8523 |
SVM RBF | 0.8472 | 0.6591 | 0.8026 | 0.725 | 0.7759 | 0.3409 | 0.8479 |
SVM poly. | 0.875 | 0.5455 | 0.759 | 0.7273 | 0.75 | 0.4545 | 0.6979 |
Category 04: Last thirteen Ranked Features | |||||||
Naïve Bayes | 0.8056 | 0.7045 | 0.8169 | 0.6889 | 0.7672 | 0.2955 | 0.828 |
Decision Tree | 0.8333 | 0.7955 | 0.8696 | 0.7447 | 0.819 | 0.2045 | 0.828 |
SVM Gaussian | 0.875 | 0.5909 | 0.7778 | 0.7429 | 0.7672 | 0.4091 | 0.8422 |
SVM RBF | 0.875 | 0.6136 | 0.7875 | 0.75 | 0.7759 | 0.3864 | 0.8469 |
SVM poly. | 0.9167 | 0.6136 | 0.7952 | 0.8182 | 0.8017 | 0.3864 | 0.8374 |
Category 05: Last Two Ranked Features | |||||||
Naïve Bayes | 0.9722 | 0.4318 | 0.7368 | 0.9048 | 0.7672 | 0.5682 | 0.761 |
Decision Tree | 0.7361 | 0.5455 | 0.726 | 0.5581 | 0.6638 | 0.4545 | 0.761 |
SVM Gaussian | 0.8889 | 0.4545 | 0.7273 | 0.7143 | 0.7241 | 0.5455 | 0.7301 |
SVM RBF | 0.8889 | 0.4545 | 0.7273 | 0.7143 | 0.7241 | 0.5455 | 0.72 |
SVM poly. | 0.8889 | 0.4545 | 0.7273 | 0.7143 | 0.7241 | 0.5455 | 0.7336 |
Based on the category 02 ranking features, the overall highest detection performance was obtained using Naïve followed by SVM Gaussian, decision tree and SVM polynomial. Using the category 03 features, we obtained highest performance using Naïve Bayes followed by SVM Gaussian, decision tree and SVM polynomial. Using the category 04 features, the highest performance was obtained using SVM polynomial followed by decision tree, SVM RBF Gaussian and Naïve Bayes. Based on the category 05 features, the highest detection performance was obtained using Naïve Bayes followed by SVM polynomial, SVM RBF, SVM Gaussian and decision tree.
Figure 3 (a–e) shows the area under the receiver operating curve to distinguish the CHF subjects from NSR subjects by extracting multimodal features with feature ranking using robust machine learning classifiers. We categorized the AUC performance based on category 01 ranked a) all 22 multimodal features, category 02 features b) Top five Ranked features, category 03 features c) Top nine ranked features, category 04 features d) last ranked 13 features and category 05 features e) last ranked two features.
Using the category 01 ranked features, the highest separation was obtained using SVM Gaussian with AUC (0.9441) followed by SVM RBF with AUC (0.9347), SVM polynomial with AUC (0.9343); Naïve Bayes and decision tree with AUC (0.9296). By using category 02 ranked features, the highest separation was obtained using decision tree & Naïve Bayes with AUC (0.8722) followed by SVM Gaussian with AUC (0.8633), SVM RBF with AUC (0.8204), SVM polynomial with AUC (0.7869). Based on the category 03 ranked features, the highest AUC was obtained using NB & decision tree. Likewise, based on the category 04 ranked features, the highest separation was obtained using SVM. Moreover, based on the category 05 ranked features, the highest separation was obtained using Naïve Bayes and decision tree. The AUC values of Naïve Bayes and Decision tree are same so are merged with one color.
The Table 2 reflect the summary of results obtained for different feature extracting strategies and classification algorithms. We aimed to check the features importance in detecting the congestive heart failure by ranking the features. This ranking will help the clinicians that which features are more import for them to make further decision. From the results depicted in Table 2, it is important to note that using all 22 multimodal features together, the highest accuracy (88.79%), AUC (0.9441) was obtained, while using only top five ranked features there was a very low decrease in performance with accuracy (82.76%), AUC (0.8722). This indicates that these five ranked features are more important than the other all extracted features in decision making. Similarly, the low ranked features further yielded the low detection performance.
Author | Method | Performance |
Li et al. [72] | Convolutional Neural network | Accuracy = 81.9%; Accuracy = 81.92% |
Isler Kuntalp [3] | K-nearest Neighbor | Sensitivity = 82.74%; Specificity = 96.27% |
Narin et al. [73] | Support Vector Machine | Sensitivity = 79.33%; Specificity = 94.47% |
Isler Kuntalp., [74] | K-nearest Neighbor | Sensitivity = 82.72%; Specificity = 100.0% |
Pecchia et al. [9] | Classification and regression tree | Sensitivity = 89.75%; Specificity = 100.0% |
Elfadil Ibrahim. [75] | Spectral Neural network | Accuracy = 83.65% |
Yang et al. [76] | Support vector machine Naïve Bayes | Accuracy = 74.42% |
Son et al. [77] | Decision tree | Sensitivity = 97.53% |
This work | SVM Gaussian | Sensitivity (93.06%); Specificity (81.82%)Accuracy (88.79%); AUC (0.9441) |
Naïve Bayes | Accuracy (82.76%); AUC (0.8722) | |
SVM Gaussian | Accuracy (76.72%); AUC (0.828) | |
Naïve Bayes | Accuracy (76.72%); AUC (0.761) |
The heart rate dynamics are highly complex and nonlinear. The patients admitted in the emergency department with complain of shortness of breathing, increase of lower extremity edema, dyspnea on exertion, lower extremity edema, and or worsening fatigue should have heart failure, which require differential diagnosis. The temporal and spectral dynamics can be analyzed the time domain and frequency domain methods. The dynamics of complex systems also degraded due to aging and disease. To capture these dynamics, we extracted the nonlinear entropy and wavelet-based entropy measures. The researchers in the past extracted multidomain and modal features to detect epileptic seizure [31,33], arrhythmia detection [16], seizure detection using time frequency representation methods [78] and cancer detection such as lung cancer dynamics using refined fuzzy entropy methods [34], lung cancer detection based on multimodal features [79] and colon cancer based on hybrid feature extracting strategy [17]. Recently Singh et al. [80] analysed that coronary heart disease with diabetes mellitus patients get significant results in clinical symptoms with improvement in the quality of life. To detect the heart rate variability, they employed SVM with RBF and decision tree [81]. The results obtained their studies revealed very good detection performance [82].
This study is aimed to compute the congestive heart failure detection performance by ranking the multimodal features. The feature ranking may help the clinicians that which features are most suitable for further decision making. The feature ranking method also ranked the feature importance. There are different feature ranking methods, we ranked the importance of Multimodal features based on the class separability criteria of the area between EROC and random classifier slope. The ranked features are than categorized based on ROC values achieved i.e. high ROC values, medium, low and very small ROC values. These categories can also help us to determine the detection performance by using all extracted features and categorized ROC values. We have observed that among the 22 multimodal features, the top ranked features gained a reasonable high detection performance. The top five selected features based on ranking methods were from wavelet, frequency domain and few statistical such as wavelet threshold, very low frequency, kurtosis, ultra-low frequency, total power. This indicates that these features are very helpful in detection the congestive heart failure. Moreover, with the lowest ROC ranked features, the detection performance was decreased. With the very low ranked features such as SDANN and LFHF, the performance was decreased further. These different categories also helped to determine the detection performance in a better way.
Heart rate variability analysis is a non-invasive tool used for assessing the cardiac autonomic control of nervous system. The congestive heart failure is the major problem worldwide. The researchers are developing efficient tools to improve the detection performance. In the past, researchers used different features extraction approaches. However, feature ranking also plays a vital role to judge the importance of features based on various factors. The important features can be very helpful for clinicians and radiologists to make the early decision. In this study, we extracted the multimodal features from both CHF and NSR subjects. We then ranked the features based on ROC values. The performance was measured based on categorized ranked features from one to five different ranking categories in order to see the performance results with top, medium, and low ranked features. Based on all features used, the highest performance with accuracy (88.79%), AUC (0.9441) was obtained using SVM Gaussian. Based on the top five ranked features (i.e. wavelet entropy threshold, VLF, kurtosis, ULF, TP) with ROC value > 3 yielded highest detection performance with accuracy (82.76%), AUC (0.822) using, whereas top 9 features with ROC value between 2–3 yielded an accuracy (84.48%), AUC (0.8767) using Naïve Bayes. The ranked features based on their importance will greatly be helpful for clinicians for further decision making and can greatly impact in reducing the mortality rate. The results with top ranked features contributed a lot, while the performance results with low ranked features dramatically decreased.
Currently, we have used the dataset with small sample size and lack of clinical information. In future, we will acquire big data and clinical profile of the patients. Moreover, we will explore more relationship to determine the feature importance base on different ranking methods and associations among the features. We will also extract and ranked these features for New York Heart Association (NYHA) functional classes and compute associations and ranks accordingly. We will also explore the association between different multimodal extracted features by computing the strengths and coupling relation which will further assist the clinicians to find the association and strength between and among the extracted features. The ranked features will further help the clinicians for further diagnosis and treatment of the patient.
The authors extend their appreciation to the research is funded by the Deanship of Scientific Research, University of Jeddah, Saudi Arabia vide grant number UJ-02-013-ICGR.
The authors declare no conflict of interest in this paper.
[1] |
E. Cartan, Sur des familles remarquables d'hypersurfaces isoparamˊetriques dans les espaces spheriques, Math. Z., 45 (1939), 335-367. doi: 10.1007/BF01580289
![]() |
[2] |
S. P. Chang, A closed hypersurface with constant scalar curvature and constant mean curvature in S4 is isoparametric, Comm. Anal. Geom., 1 (1993), 71–100. doi: 10.4310/CAG.1993.v1.n1.a4
![]() |
[3] | S. P. Chang, On minimal hypersurfaces with constant scalar curvatures in S4, J. Differ. Geom., 37 (1993), 523–534. |
[4] |
Q. T. Deng, H. L. Gu, Q. Y. Wei, Closed Willmore minimal hypersurfaces with constant scalar curvature in S5(1) are isoparametric, Adv. Math., 314 (2017), 278–305. doi: 10.1016/j.aim.2017.05.002
![]() |
[5] |
Z. Guo, H. Li, C. P. Wang, The second variational formula for Willmore submanifolds in Sn, Results Math., 40 (2001), 205–225. doi: 10.1007/BF03322706
![]() |
[6] | Z. Guo, J. B. Fang, L. M. Lin, Hypersurfaces with isotropic Blaschke tensor, J. Math. Soc. Japan, 63 (2011), 1155–1186. |
[7] |
Z. J. Hu, H. Li, Classification of hypersurfaces with parallel Möbius second fundamental form in Sn+1, Sci. China Ser. A, 47 (2004), 417–430. doi: 10.1360/03ys0134
![]() |
[8] |
Z. J. Hu, H. Z. Li, C. P. Wang, Classification of Möbius isoparametric hypersurfaces in S5, Monatsh. Math., 151 (2007), 201–222. doi: 10.1007/s00605-006-0420-x
![]() |
[9] |
Z. J. Hu, S. J. Zhai, Möbius isoparametric hypersurfaces with three distinct principal curvatures, Ⅱ, Pac. J. Math., 249 (2011), 343–370. doi: 10.2140/pjm.2011.249.343
![]() |
[10] |
Z. J. Hu, S. J. Zhai, Submanifolds with parallel Möbius second fundamental form in the unit sphere, Results Math., 73 (2018), 1–46. doi: 10.1007/s00025-018-0773-1
![]() |
[11] |
H. Li, Willmore hypersurfaces in a sphere, Asian J. Math., 5 (2001), 365–377. doi: 10.4310/AJM.2001.v5.n2.a4
![]() |
[12] | H. Li, H. L. Liu, C. P. Wang, G. S. Zhao, Möbius isoparametric hypersurfaces in Sn+1 with two distinct principal curvatures, Acta Math. Sin. 18 (2002), 437–446. |
[13] |
T. Z. Li, J. Qing, C. P. Wang, Möbius curvature, Laguerre curvature and Dupin hypersurface, Adv. Math., 311 (2017), 249–294. doi: 10.1016/j.aim.2017.02.024
![]() |
[14] | H. L. Liu, C. P. Wang, G. S. Zhao, Möbius isotropic submanifolds in Sn, Tohoku Math. J., 53 (2001), 553–569. |
[15] |
T. Lusala, M. Scherfner, L. A. M. Sousa Jr, Closed minimal Willmore hypersurfaces of S5(1) with constant scalar curvature, Asian J. Math., 9 (2005), 65–78. doi: 10.4310/AJM.2005.v9.n1.a6
![]() |
[16] |
C. K. Peng, C. L. Terng, The scalar curvature of minimal hypersurfaces in spheres, Math. Ann., 266 (1983), 105–113. doi: 10.1007/BF01458707
![]() |
[17] | Z. Z. Tang, W. J. Yan, On the Chern conjecture for isoparametric hypersurfaces, arXiv preprint arXiv: 2001.10134, 2020. |
[18] |
C. P. Wang, Möbius geometry of submanifolds in Sn, Manuscripta Math., 96 (1998), 517–534. doi: 10.1007/s002290050080
![]() |
1. | Wenya Zhao, Peili Zhang, Da Chen, Hao Wang, Binghua Gu, Jue Zhang, Data mining from process monitoring of typical polluting enterprise, 2023, 195, 0167-6369, 10.1007/s10661-023-11733-5 | |
2. | Rami AL-HAJJ, Mohamad M. Fouad, Mustafa Zeki, Evolutionary optimization framework to train multilayer perceptrons for engineering applications, 2024, 21, 1551-0018, 2970, 10.3934/mbe.2024132 | |
3. | Swamiraj Nithiyanantha Vasagam, Bhoopalan Ravikumar, Rajkumar Kavibharathi, Jeyasekaran Keerthana, Ramaseshan Sathya Narayanan, Kharbanda Geetika, Prediction of leather footwear export using learning algorithms based on ANN model, 2024, 238, 09574174, 121809, 10.1016/j.eswa.2023.121809 |
Method | Sensitivity | Specificity | PPV | NPV | Accuracy | FPR | AUC |
Category 01: All Ranked features | |||||||
Naïve Bayes | 0.8889 | 0.8864 | 0.9275 | 0.8298 | 0.8879 | 0.1136 | 0.9296 |
Decision Tree | 0.8194 | 0.7273 | 0.831 | 0.7111 | 0.7845 | 0.2727 | 0.9296 |
SVM Gaussian | 0.9306 | 0.8182 | 0.8933 | 0.878 | 0.8879 | 0.1818 | 0.9441 |
SVM RBF | 0.9028 | 0.8409 | 0.9028 | 0.8409 | 0.8793 | 0.1591 | 0.9347 |
SVM poly. | 0.9722 | 0.7273 | 0.8537 | 0.9412 | 0.8793 | 0.2727 | 0.9343 |
Category 02: Top five Ranked Features | |||||||
Naïve Bayes | 0.8611 | 0.7727 | 0.8611 | 0.7727 | 0.8276 | 0.2273 | 0.8722 |
Decision Tree | 0.8889 | 0.5 | 0.7442 | 0.7333 | 0.7414 | 0.5 | 0.8722 |
SVM Gaussian | 0.9722 | 0.5909 | 0.7955 | 0.9286 | 0.8276 | 0.4091 | 0.8633 |
SVM RBF | 0.9306 | 0.5909 | 0.7882 | 0.8387 | 0.8017 | 0.4091 | 0.8204 |
SVM poly. | 0.8611 | 0.5455 | 0.7561 | 0.7059 | 0.7414 | 0.4545 | 0.7869 |
Category 03: Top Nine Ranked Features | |||||||
Naïve Bayes | 0.8889 | 0.7727 | 0.8649 | 0.8095 | 0.8448 | 0.2273 | 0.8767 |
Decision Tree | 0.8472 | 0.6364 | 0.7922 | 0.7179 | 0.7672 | 0.3636 | 0.8767 |
SVM Gaussian | 0.8611 | 0.6818 | 0.8158 | 0.75 | 0.7931 | 0.3182 | 0.8523 |
SVM RBF | 0.8472 | 0.6591 | 0.8026 | 0.725 | 0.7759 | 0.3409 | 0.8479 |
SVM poly. | 0.875 | 0.5455 | 0.759 | 0.7273 | 0.75 | 0.4545 | 0.6979 |
Category 04: Last thirteen Ranked Features | |||||||
Naïve Bayes | 0.8056 | 0.7045 | 0.8169 | 0.6889 | 0.7672 | 0.2955 | 0.828 |
Decision Tree | 0.8333 | 0.7955 | 0.8696 | 0.7447 | 0.819 | 0.2045 | 0.828 |
SVM Gaussian | 0.875 | 0.5909 | 0.7778 | 0.7429 | 0.7672 | 0.4091 | 0.8422 |
SVM RBF | 0.875 | 0.6136 | 0.7875 | 0.75 | 0.7759 | 0.3864 | 0.8469 |
SVM poly. | 0.9167 | 0.6136 | 0.7952 | 0.8182 | 0.8017 | 0.3864 | 0.8374 |
Category 05: Last Two Ranked Features | |||||||
Naïve Bayes | 0.9722 | 0.4318 | 0.7368 | 0.9048 | 0.7672 | 0.5682 | 0.761 |
Decision Tree | 0.7361 | 0.5455 | 0.726 | 0.5581 | 0.6638 | 0.4545 | 0.761 |
SVM Gaussian | 0.8889 | 0.4545 | 0.7273 | 0.7143 | 0.7241 | 0.5455 | 0.7301 |
SVM RBF | 0.8889 | 0.4545 | 0.7273 | 0.7143 | 0.7241 | 0.5455 | 0.72 |
SVM poly. | 0.8889 | 0.4545 | 0.7273 | 0.7143 | 0.7241 | 0.5455 | 0.7336 |
Author | Method | Performance |
Li et al. [72] | Convolutional Neural network | Accuracy = 81.9%; Accuracy = 81.92% |
Isler Kuntalp [3] | K-nearest Neighbor | Sensitivity = 82.74%; Specificity = 96.27% |
Narin et al. [73] | Support Vector Machine | Sensitivity = 79.33%; Specificity = 94.47% |
Isler Kuntalp., [74] | K-nearest Neighbor | Sensitivity = 82.72%; Specificity = 100.0% |
Pecchia et al. [9] | Classification and regression tree | Sensitivity = 89.75%; Specificity = 100.0% |
Elfadil Ibrahim. [75] | Spectral Neural network | Accuracy = 83.65% |
Yang et al. [76] | Support vector machine Naïve Bayes | Accuracy = 74.42% |
Son et al. [77] | Decision tree | Sensitivity = 97.53% |
This work | SVM Gaussian | Sensitivity (93.06%); Specificity (81.82%)Accuracy (88.79%); AUC (0.9441) |
Naïve Bayes | Accuracy (82.76%); AUC (0.8722) | |
SVM Gaussian | Accuracy (76.72%); AUC (0.828) | |
Naïve Bayes | Accuracy (76.72%); AUC (0.761) |
Method | Sensitivity | Specificity | PPV | NPV | Accuracy | FPR | AUC |
Category 01: All Ranked features | |||||||
Naïve Bayes | 0.8889 | 0.8864 | 0.9275 | 0.8298 | 0.8879 | 0.1136 | 0.9296 |
Decision Tree | 0.8194 | 0.7273 | 0.831 | 0.7111 | 0.7845 | 0.2727 | 0.9296 |
SVM Gaussian | 0.9306 | 0.8182 | 0.8933 | 0.878 | 0.8879 | 0.1818 | 0.9441 |
SVM RBF | 0.9028 | 0.8409 | 0.9028 | 0.8409 | 0.8793 | 0.1591 | 0.9347 |
SVM poly. | 0.9722 | 0.7273 | 0.8537 | 0.9412 | 0.8793 | 0.2727 | 0.9343 |
Category 02: Top five Ranked Features | |||||||
Naïve Bayes | 0.8611 | 0.7727 | 0.8611 | 0.7727 | 0.8276 | 0.2273 | 0.8722 |
Decision Tree | 0.8889 | 0.5 | 0.7442 | 0.7333 | 0.7414 | 0.5 | 0.8722 |
SVM Gaussian | 0.9722 | 0.5909 | 0.7955 | 0.9286 | 0.8276 | 0.4091 | 0.8633 |
SVM RBF | 0.9306 | 0.5909 | 0.7882 | 0.8387 | 0.8017 | 0.4091 | 0.8204 |
SVM poly. | 0.8611 | 0.5455 | 0.7561 | 0.7059 | 0.7414 | 0.4545 | 0.7869 |
Category 03: Top Nine Ranked Features | |||||||
Naïve Bayes | 0.8889 | 0.7727 | 0.8649 | 0.8095 | 0.8448 | 0.2273 | 0.8767 |
Decision Tree | 0.8472 | 0.6364 | 0.7922 | 0.7179 | 0.7672 | 0.3636 | 0.8767 |
SVM Gaussian | 0.8611 | 0.6818 | 0.8158 | 0.75 | 0.7931 | 0.3182 | 0.8523 |
SVM RBF | 0.8472 | 0.6591 | 0.8026 | 0.725 | 0.7759 | 0.3409 | 0.8479 |
SVM poly. | 0.875 | 0.5455 | 0.759 | 0.7273 | 0.75 | 0.4545 | 0.6979 |
Category 04: Last thirteen Ranked Features | |||||||
Naïve Bayes | 0.8056 | 0.7045 | 0.8169 | 0.6889 | 0.7672 | 0.2955 | 0.828 |
Decision Tree | 0.8333 | 0.7955 | 0.8696 | 0.7447 | 0.819 | 0.2045 | 0.828 |
SVM Gaussian | 0.875 | 0.5909 | 0.7778 | 0.7429 | 0.7672 | 0.4091 | 0.8422 |
SVM RBF | 0.875 | 0.6136 | 0.7875 | 0.75 | 0.7759 | 0.3864 | 0.8469 |
SVM poly. | 0.9167 | 0.6136 | 0.7952 | 0.8182 | 0.8017 | 0.3864 | 0.8374 |
Category 05: Last Two Ranked Features | |||||||
Naïve Bayes | 0.9722 | 0.4318 | 0.7368 | 0.9048 | 0.7672 | 0.5682 | 0.761 |
Decision Tree | 0.7361 | 0.5455 | 0.726 | 0.5581 | 0.6638 | 0.4545 | 0.761 |
SVM Gaussian | 0.8889 | 0.4545 | 0.7273 | 0.7143 | 0.7241 | 0.5455 | 0.7301 |
SVM RBF | 0.8889 | 0.4545 | 0.7273 | 0.7143 | 0.7241 | 0.5455 | 0.72 |
SVM poly. | 0.8889 | 0.4545 | 0.7273 | 0.7143 | 0.7241 | 0.5455 | 0.7336 |
Author | Method | Performance |
Li et al. [72] | Convolutional Neural network | Accuracy = 81.9%; Accuracy = 81.92% |
Isler Kuntalp [3] | K-nearest Neighbor | Sensitivity = 82.74%; Specificity = 96.27% |
Narin et al. [73] | Support Vector Machine | Sensitivity = 79.33%; Specificity = 94.47% |
Isler Kuntalp., [74] | K-nearest Neighbor | Sensitivity = 82.72%; Specificity = 100.0% |
Pecchia et al. [9] | Classification and regression tree | Sensitivity = 89.75%; Specificity = 100.0% |
Elfadil Ibrahim. [75] | Spectral Neural network | Accuracy = 83.65% |
Yang et al. [76] | Support vector machine Naïve Bayes | Accuracy = 74.42% |
Son et al. [77] | Decision tree | Sensitivity = 97.53% |
This work | SVM Gaussian | Sensitivity (93.06%); Specificity (81.82%)Accuracy (88.79%); AUC (0.9441) |
Naïve Bayes | Accuracy (82.76%); AUC (0.8722) | |
SVM Gaussian | Accuracy (76.72%); AUC (0.828) | |
Naïve Bayes | Accuracy (76.72%); AUC (0.761) |