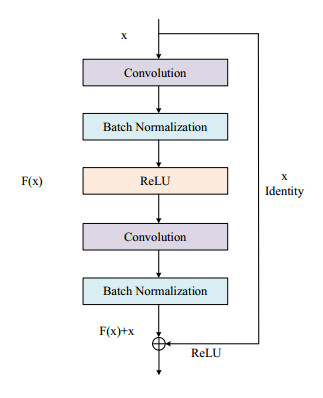
Citation: Azmy S. Ackleh, Nicolas Saintier, Jakub Skrzeczkowski. Sensitivity equations for measure-valued solutions to transport equations[J]. Mathematical Biosciences and Engineering, 2020, 17(1): 514-537. doi: 10.3934/mbe.2020028
[1] | Xiaoju Zhang, Kai Zheng, Yao Lu, Huanhuan Ma . Global existence and long-time behavior of solutions for fully nonlocal Boussinesq equations. Electronic Research Archive, 2023, 31(9): 5406-5424. doi: 10.3934/era.2023274 |
[2] | Bidi Younes, Abderrahmane Beniani, Khaled Zennir, Zayd Hajjej, Hongwei Zhang . Global solution for wave equation involving the fractional Laplacian with logarithmic nonlinearity. Electronic Research Archive, 2024, 32(9): 5268-5286. doi: 10.3934/era.2024243 |
[3] | Ahmed Alsaedi, Madeaha Alghanmi, Bashir Ahmad, Boshra Alharbi . Uniqueness results for a mixed p-Laplacian boundary value problem involving fractional derivatives and integrals with respect to a power function. Electronic Research Archive, 2023, 31(1): 367-385. doi: 10.3934/era.2023018 |
[4] | Ping Yang, Xingyong Zhang . Existence of nontrivial solutions for a poly-Laplacian system involving concave-convex nonlinearities on locally finite graphs. Electronic Research Archive, 2023, 31(12): 7473-7495. doi: 10.3934/era.2023377 |
[5] | Mingfa Fei, Wenhao Li, Yulian Yi . Numerical analysis of a fourth-order linearized difference method for nonlinear time-space fractional Ginzburg-Landau equation. Electronic Research Archive, 2022, 30(10): 3635-3659. doi: 10.3934/era.2022186 |
[6] | Hongyu Li, Liangyu Wang, Yujun Cui . Positive solutions for a system of fractional q-difference equations with generalized p-Laplacian operators. Electronic Research Archive, 2024, 32(2): 1044-1066. doi: 10.3934/era.2024051 |
[7] | Yuchen Zhu . Blow-up of solutions for a time fractional biharmonic equation with exponentional nonlinear memory. Electronic Research Archive, 2024, 32(11): 5988-6007. doi: 10.3934/era.2024278 |
[8] | Cheng He, Changzheng Qu . Global weak solutions for the two-component Novikov equation. Electronic Research Archive, 2020, 28(4): 1545-1562. doi: 10.3934/era.2020081 |
[9] | N. Bazarra, J. R. Fernández, R. Quintanilla . A dual-phase-lag porous-thermoelastic problem with microtemperatures. Electronic Research Archive, 2022, 30(4): 1236-1262. doi: 10.3934/era.2022065 |
[10] | Liangying Miao, Man Xu, Zhiqian He . Existence and multiplicity of positive solutions for one-dimensional p-Laplacian problem with sign-changing weight. Electronic Research Archive, 2023, 31(6): 3086-3096. doi: 10.3934/era.2023156 |
In recent years, the printing press has developed in the direction of green, high speed, automation and intelligence in modern industry. Rolling bearings are one of the most important components in rotating machinery, and play a role in reducing the friction coefficient during motion, and ensuring rotary accuracy in the printing press [1,2,3,4]. Therefore, it's health status has an important influence on the printing press's overall performance, stability and life [5]. Due to the corrosion of the rolling bears by chemical substances, such as fountain solution, ink, or bearing failure, may be due to insufficient lubrication, water or foreign matter intrusion, improper bearing assembly, long time operation of the machine, etc. It is easy to cause failure and abnormal vibration of the printing press bearings, then cause faults in the printing press, such as paper gripper being gripped, inaccurate overprinting, ink bar and poor overprinting [1]. Also, the printing presses usually work in the poor environment, so fault is not easy to be found. This strong background noise environment can lead to low fault diagnosis accuracy, which can lead to safety problems. Therefore, monitoring the operating status of the printing press bearings plays an increasingly important role in actual production, and by introducing fault diagnosis technology in machinery, it can find the potential problems of machinery in time, and also can be solved in a timely manner, greatly reducing the failure rate of equipment.
Traditional fault diagnosis is achieved by extracting bearing fault features in the original signal through signal processing techniques and mathematical transformation, and then identifying the information that can reflect the fault features [7]. However, with the increased productivity and the expanding scale of equipment, the bearing vibration signals collected by the equipment have the features of massive, multi-source and high-dimensional, which leads to inefficiency and low accuracy of the traditional fault diagnosis methods [8,9]. While intelligent fault diagnosis is based on data-driven methods, they can quickly and accurately handle a large amount of complex data, so it has become one of the current research hotspots for condition monitoring and fault diagnosis of mechanical equipment.
The mechanical structure of the printing press is complex, and the printing press generates strong noises. So, the vibration signals of the printing press bearings collect the features with strong noise. It is hard to extract the fault features accurately in the vibration signal with strong noise, and it's easy to leads to the gradient disappearance and overfitting problem of the network model during training of traditional machine learning, such as Support Vector Machine (SVM) and Logistic Regression. As a new network architecture, the ResNet can effectively solve the gradient disappearance or gradient explosion caused by the data or network depth. In addition, the ResNet has a great ability to extract features, which can solve the problem of overfitting when training other network models, and reduce the reliance on fault diagnosis experience of expert and signal processing techniques [10,11,12]. Therefore, the ResNet is selected as the base network in this proposed method. Lin [13] proposed an improved one-dimensional convolution ResNet fault diagnosis. The extraction and compression of data fault features are first completed by convolution pooling. Then, an improved ResNet is added to avoid network degradation and uneven data distribution in the training model. Zagoruyko and Komodakis [14] studied the use of a wide residual block, and demonstrated it, experimentally, at a reasonable depth. Konovalenko [15] studied a deep ResNet-based model for rolling steel surface defect recognition and classification, and constructed a classifier to detect three types of defects on flat metal surfaces. Yan [16] proposed the deep order-wavelet convolutional variational autoencoder (DOWCVAE), which improves the feature learning capability of convolutional variational autoencoder ordinary convolutional variational encoder (CVAE). Peng [17] combined ResNet and data fusion to achieve higher recognition accuracy. Yan [18] proposed a deep regularized variational autoencoder (DRVAE) intelligent fault diagnosis model for rotor-bearing systems, which solves the overfitting problem of the original variational autoencoder (VAE) and enhances the feature learning capability of the network model. Wang [19] proposed a deep separable ResNet model, which uses a reduced number of parameters through deep separable convolution, and uses residual connections to transfer features, which can effectively predict the remaining life of rolling bearings. Liang [20] proposed a frequency domain analysis method based on wavelet transform, and improved ResNet with global singular value decomposition (SVD) adaptive. Zhang [1] proposed a blockchain-based distributed joint transfer learning method to improve the accuracy of mechanical fault diagnosis, and applied it to collaborative mechanical fault diagnosis. Yan [22] proposed multiscale cascading deep belief network (MCDBN) for rotating machinery fault diagnosis, which improves fault identification accuracy by learning a wider range of feature representations. Lin [23] proposed a photovoltaic array fault diagnosis scheme using a multi-scale SE-ResNet, and designed a multi-scale perceptual field fusion module to improve the diagnosis performance of the model. Wan [24] used an improved deep ResNet as a feature extractor to extract metastable features from the original vibration signal. The classifier used the extracted domain-invariant features to complete cross-domain fault identification. Yan [25] proposed a wind speed prediction model based on long short-term memory, deep belief network and grasshopper optimization algorithm (GOA) to improve the accuracy and efficiency of wind speed prediction. Yang [26] classified real-time data collected from dissolved oxygen sensors to diagnosis online faults and experimentally proved that ResNet has great performance. Hao [27] proposed a new network structure by replacing the fully connected layer part of traditional ResNet with the global average pooling technique. The problem of too many parameters of the traditional ResNet model was effectively solved. Zhang [28] proposed a fault diagnosis method based on adaptive loss-weighted meta-ResNet (ALWM-ResNet), which uses a weighted network and a meta-network cloned from the original ResNet to establish a mapping of weighting functions, and adaptively learn weights from data containing clean labels. However, the convolution kernel in the ResNet structure has a fixed shape, poor adaptability to changes in unknown images, and poor generalization ability [29,30]. Thus, this proposed method introduces a deformable convolution layer based on the ResNet model, so that the convolution layer can adaptively change the shape of the convolution kernel according to the different input samples, so the convolution kernel can precisely locate and track the target for small fault features, and use the deformable convolution to adaptively identify the fault feature points, thus learning more detailed features and improving the accuracy of feature extraction.
However, the signals collected under actual working conditions often contain complex noises, leading to a decline in accuracy if they are inputting direct into the model. Therefore, this proposed method introduces the signal preprocessing method to extract fault features before input to improve the accuracy of the model. The Short Time Fourier Transform (STFT), Wigner-Ville Distribution (WVD), Empirical Mode Decomposition (EMD), and Wavelet Analysis are widely used preprocessing methods in the field of fault diagnosis. Hartono [31] proposed a joint time-frequency analysis method for gear fault diagnosis using the combined autoregressive model-based filtering and redistribution with the smoothing pseudo-Wigner Ville distribution (RSPWVD) method. Nezamivand [32] performed empirical modal decomposition and wavelet packet decomposition of vibration signals, and achieved good results for fault state identification of rolling bearings by SVM. Surti [33] proposed a new technique for early bearing fault detection and diagnosis based on discrete wavelet transform (DWT) and K-nearest neighbor (KNN), which showed good accuracy and the ability to distinguish between healthy and unhealthy bearing conditions. Li [34] proposed a deep learning-based remaining useful life (RUL) prediction method to solve the sensor failure problem, and introduced adversarial learning to extract invariant features in generalized sensors. Dubey [1] proposed a Hilbert transform footprint analysis and neural network for ball bearing fault analysis, and the method achieved high fault classification accuracy. Tian [36] proposed a method to detect bearing faults and monitor bearing degradation in electric motors using Programmable Counter Array and semi-supervised KNN distance to combine these features to form health indicators for detection. Amar [37] proposed a novel vibration spectrum imaging (VSI) feature enhancement system for low signal-to-noise ratio (SNR) conditions that enhances and provides a visual representation of feature vibrational spectral features in the form of images. Zhang [38] preliminarily constructed the wavelet-overlapping group sparse (WOGS) optimization model based on the overlapping features of Morlet wavelet transform coefficients, and constructed the weight coefficients in the model by analyzing the pulse features of the signal. Mao [39] used a classification method combining multiscale alignment entropy and support vector machine to achieve the fault-type classification of bearings. Zhao [40] proposed a new convolution neural network scheme based on attention-enhanced convolution blocks (AECB) to achieve higher training accuracy for control moment gyroscope (CMG) fault diagnosis data sets with different sliding window parameters.
The above method used the data collected under the standard data set, and used simple preprocessing into the neural network to achieve bearing fault diagnosis. However, the above-preprocessing methods need to select the appropriate window function or wavelet basis function, as traditional preprocessing methods lead to modal blending and endpoint effects, resulting in low fault diagnosis accuracy, such as STFT, Wavelet Transform and WVD. This isdue to the faulty bearing signals being collected within strong noise vibrations in the actual working conditions. The FSWT combines the advantages of STFT and Wavelet Transform, which not only reduces the dependence of wavelets and wavelet packets on wavelet basis functions in reconstructing signals, but also realizes the reconstruction of signals in arbitrary frequency bands and the accurate description of local features, which can flexibly realize the filtering and segmentation of signals [41]. Furthermore, the TFD after FSWT processing contains feature information of the vibration signal in both time domain and frequency domain, which is beneficial to neural networks for feature extraction and improves the efficiency of model identification effectively.
In summary, this method proposes a diagnosis method that integrate FSWT, deformable convolution layer, and ResNet to improve diagnosis efficiency under strong background noise conditions while ensuring diagnosis accuracy. The experimental results show that the proposed method has higher recognition accuracy than other diagnoses. In addition, the diagnosis accuracy is improved while the model training efficiency is enhanced. The main contributions of this proposed method are summarized as follows.
(1) In order to reduce the influence of noise on diagnosis accuracy, this method proposes a method preprocessing bearing vibration signals using FSWT. Compared with other methods, this method can improve the efficiency and accuracy of subsequent intelligent fault diagnosis significantly.
(2) In this proposed method, the ResNet is selected to solve the problem of gradient disappearance and overfitting during the training of traditional network models. At the same time, in order to enhance the extraction ability of the model for subtle features in the TFD, the deformable convolution layer is introduced into the ResNet, which improves the adaptiveness of the convolution layer shape so that the model can effectively capture the subtle features drowned in noise.
(3) This proposed method is tested with the Case Western Reserve University (CWRU) data set with an accuracy of 99.77%. In the application of actual working conditions, the model is also superior to other methods, and the diagnostic accuracy is 93.90%.
The rest of the paper is organized as follows: Section 2 presents the frequency slice wavelet transform. Section 3 introduces the ResNet model used in this proposed method. Section 4 introduces the structure of the deformable convolution layer and the structure of the DC-ResNet. Section 5 introduces the fault diagnosis process, parameters of the DC-ResNet and model hyperparameters proposed. The experimental validation of the method and the analysis of the experimental results are presented in Section 6. Finally, the conclusions are shown in Section 7.
The common method of rolling bearing fault diagnosis is based on vibration signals. In traditional bearing fault diagnosis, the one-dimensional time-domain vibration signal is usually spliced into the two-dimensional signal, and the splicing will lead the vibration signal addition of two-dimensional information, which is not available in the original time-domain vibration signal. As the input of the ResNet, the two-dimensional information created artificially will affect the feature extraction of the signal by the ResNet, thus affecting the accuracy of the fault diagnosis. The FSWT have advantages over STFT and wavelet transform, can realize signal filtering and segmentation flexibly [42], and the original time-domain signal can be converted to a time-frequency signal. Due to the rolling bearing fault signals of the printing press, the collection is a signal with strong noise, and the time-frequency domain features of the signal can be better observed by FSWT. Therefore, in this proposed method, FSWT is used to preprocess the bearing vibration signals of the printing press.
Let the signal f(t)∈L2(R), if ˆp(ω) is the Fourier transform of p(t), then its FSWT is defined in the frequency domain as [43].
Wf(t,ω,λ,σ)=12πλ∫+∞−∞f(u)ˆp∗(u−ωσ)eiutdu, | (2.1) |
where λ is the energy factor (λ≠0), scale σ is either an invariant or a function of ω, t and u, "*" is the conjugate of the function, ω and t are observation frequency and observation time, u is the frequency of assessment and ˆp(ω) is the FSF. By the Parseval equation, the above equation can be transformed to the time domain as follows:
Wf(t,ω,λ,σ)=σλϵiωt∫∞−∞f(τ)e−iwτp∗(σ(τ−t))dτ | (2.2) |
In general, taking λ=1, let σ=ω/κ, κ>0, then the Eq (2.1) can be rewritten as:
W(t,ω,κ)=12π∫+∞−∞ˆf(u)ˆp(κu−ωω)eiutdu | (2.3) |
Among them, κ is unrelated to ω, and u is mainly used to adjust the sensitivity of the FSWT to frequency or time, and it is called the time-frequency resolution factor. From the Heisenberg uncertainty principle, it is not possible to obtain a high resolution in both the frequency and time domains, so we then used σ and ω to estimate the time-frequency resolution factor, while introducing two evaluation coefficients: the frequency resolution ratio η and the amplitude expectation response ratio v(0<v≤1), where v is usually taken as √2/2,0.5,0.25, etc.
The inversion of frequency slice wavelets can reconstruct the original signal in many different ways, and the commonly used forms of inverse transform are as follows:
f(t)=12πλ∫+∞−∞∫+∞−∞W(τ,ω,λ,σ)eiω(t−τ)dτdσ | (2.4) |
The Eq (2.4) shows that the inverse transform is independent on p(t), p(ω) and σ, and the fast Fourier transform algorithm can directly obtain the reconstructed signal. The FSWT realizes time-frequency analysis of the signal, which can filter and segment the signal components in any frequency.
ResNet was proposed by He of Microsoft Research in 2015 [44]. The ResNet solves the training difficulties caused by increasing the depth of the network, and its network performance is far better than the traditional network models.
Suppose a regular convolution neural network has L layers, where the input of layer i (i∈1,2,...,L) is xi and its corresponding parameter is wi, and the output of this layer is yi=xi+1. For the sake of simplicity of presentation, ignoring the number of layers and bias, the relationship between them can be expressed as Eq (2.5):
y=F(x,wf), | (2.5) |
where F is the nonlinear activation function and wf is the convolution operation. The depth of ResNet can be expressed by Eq (2.6):
y=F(x,wf)+x | (2.6) |
A simple deformation of Eq (2.6) yields Eq (2.7):
F(x,wf)=y−x | (2.7) |
The function F that the network needs to learn is actually the residual term y−x at the right end of the formula, called the residual function.
As is shown in Figure 1, there are two branches in the residual learning module: The residual function F(x) and the input constant mapping x. These two branches are integrated by adding the corresponding elements through the nonlinear transformation activation function ReLU, then forms the whole residual learning module, and the structure formed by stacking multiple residual modules is called "ResNet". This deep learning framework adds residual connectivity, which is more convenient than the original mapping without residual connectivity. It uses a stacked nonlinear combination to fit a constant mapping, making its residuals more likely to converge to zero. Optimizing the residual function y=F(x,wf)+x is easier than optimizing a complex nonlinear mapping F(x,wf)=y−x, when the network layers are deep enough.
In deformable convolution [45], an offset parameter {ΔPn|n=1,2,...,N} is added to the rectangular region 𝑅 where the regular convolution neural network acts, as is shown in Figure 2.
Where N=|R|, the output p0 in the feature diagram position is calculated as Eq (2.8):
y(p0)=∑r∈Rw(r)∗x(p0+r+Δr) | (2.8) |
Since Δr is a floating-point number, bilinear interpolation is used to sample x. Make p=p0+r+Δr and use q to represent all possible integer space locations within the feature diagram x, the bilinear interpolation for x are as follows:
x(p)=∑qG(q,p)x(q), | (2.9) |
where G denotes a two-dimensional bilinear interpolation kernel that can be decomposed into two one-dimensional kernels.
G(q,p)=g(qx,px)g(qy,py), | (2.10) |
where g(a,b)=max(0,1−|a−b|). For the vast majority of position q, G = 0 and G is the transformation kernel to be learned. The gradient with respect to the offset parameter Δr is calculated as follows:
∂y(p0)∂Δr=∑r∈Rw(r)∂x(p0+r+Δr)∂Δr=∑r∈R[w(r)∑q∂G(q,p0+r+Δr)∂Δrx(q)] | (2.11) |
From the feature diagram of input, the deformable convolution learns the offset parameter through the convolution network with two directions, indicating both x and y directions. With the feature diagram input and the offsets carried out, the computation of the deformable convolution can be performed.
For bearing fault diagnosis, the TFD obtained by FSWT embodies more complex fault features. Since the standard convolution kernel lacks the geometric transformation mechanism inside the diagram, its fix geometry can only be sampled at the fixed position, but the fixed position may lose fault features. Therefore, the network needs deformable convolution kernels to adapt to the feature positions of the diagram. These deformable convolution kernels can perform more detailed feature mining on the TFD to improve the accuracy of bearing fault diagnosis. In this proposed method, the convolution layer in the residual block structure is replaced by a deformable convolution layer, as is shown in Figure 3.
The basic ResNet structure is ResNet18. Deformable convolution layers are introduced to the basic network structure, replacing all the traditional 3 × 3 convolution layers with deformable convolution layers in the original ResNet18. Then, more detailed features are learned by using the adaptive nature of the shape of the convolution kernel and high-precision fault diagnosis of printing press bearings is achieved. The network structure is shown in Figure 4 and Table 1.
DC-ResNet Structure | Output Dimension |
Input Layer | (190,150, 3) |
Convolution Layer 1 | (184,144, 64) |
Max Pooling | (92, 72, 64) |
Residual Block 1 | (92, 72, 64) |
Residual Block 2 | (92, 72, 64) |
Residual Block 3 | (92, 72,128) |
Residual Block 4 | (92, 72,128) |
Residual Block 5 | (92, 72,256) |
Residual Block 6 | (92, 72,256) |
Residual Block 7 | (92, 72,512) |
Residual Block 8 | (92, 72,512) |
Global Average Pooling | 512 |
Output Layer | 10 |
The flowchart of the bearing fault diagnosis method of the printing press combines the time-frequency processing of FSWT with DC-ResNet, as is shown in Figure 5, and its detailed steps are as follows:
(1) Use sensor acquisition vibration signals of bearing faults during the operation of the printing press.
(2) Preprocess the bearing vibration signal collected above using FSWT, and obtain the corresponding TFD of the bearing vibration signal.
(3) Initialize the ResNet18 network model parameters, set its learning rate to 0.001 and the number of iterations to 50, and migrate the model parameters and data to the GPU to accelerate the computational process.
(4) Input the TFD of the vibration signal obtained in step (2) into the network model of ResNet18 and normalize it. Introduce deformable convolution layers to replace all the 3 × 3 convolution layers in the original network, optimize the gradient descent using the Adagrad optimizer to obtain the optimal model parameters, output the DC-ResNet model parameters, and test them with an unknown category data set.
(5) Get the test results of bearing fault diagnosis, and then get the printing press bearing health status.
To verify the effectiveness of the proposed method, the vibration acceleration data of rolling bearings from CWRU are used for experimental analysis [46]. This fault diagnosis experimental setup consists of a torque meter, a power meter and a three-phase asynchronous motor. The sample data contains signals of three different fault types at a sampling frequency of 12 kHz, as well as one normal vibration signal: normal condition, inner race fault, outer race fault and ball fault. Within each fault type, there are three different types of fault depths, 0.18 mm, 0.36 mm and 0.54 mm respectively. The data set contains a total of 9 types of faults and 1 type of normal data.
Figure 6 shows the TFD of the bearing vibration signals of time domain waveforms after Morlet wavelet transform processing and the TFD after FSWT processing, which collected under three typical fault types: inner race fault, outer race fault and ball fault. Figure 6 shows comparison of the TFD obtained after FSWT with the TFD obtained after the Morlet wavelet transform, where the former has better time-frequency focus, more concentrated energy distribution and more obvious fault features, which are more conducive to the identification and classification of bearing faults.
Figure 7 shows the control experimental data set. It uses grayscale diagrams formed by simply splicing the one-dimensional time-domain vibration signals into two-dimensional signals.
In order to verify the effectiveness of applying the FSWT to the ResNet in this proposed method, a comparison experiments was conducted. The TFD of FSWT are used as the input of the ResNet18, and the grayscale diagram and Morlet wavelet TFD are used for comparison experiments. Each set of experiments is repeated five times, and the final average diagnosis results obtain by these three preprocessing methods are shown in Table 2. As can be seen from Table 2, compared with the grayscale diagram and Morlet wavelet TFD, the data set obtain by FSWT has a significant improvement in terms of accuracy, and the average accuracy can reach 99.77%, which is higher than the diagnosis results of Morlet wavelet TFD (88.72%) and grayscale diagram (74.92%), proving that the FSWT to the ResNet has more advantages and effectiveness of this proposed method.
Preprocessing Methods | Number of Training Samples/Test Samples | Average Accuracy (%) |
FSWT | 3000/1000 | 99.77 |
Morlet Wavelet Transform | 88.72 | |
Grayscale | 74.92 |
In order to compare the effects of three different data preprocessing methods on the model diagnosis performance, confusion matrix is introduced [47]. As is shown in Figure 8, the predicted labels of 1000 test samples are compared with the true labels and obtain the confusion matrix of the three data sets based on the ResNet18 model. The color band on the right side of the figure indicates the degree of being accurately classified. The color block at the diagonal position is the number of samples correctly classified in each category, with darker colors representing more correctly classified samples. The color block at the remaining positions is the number of samples misclassified in each category, with lighter colors representing fewer samples misclassified. Among them, Figure 8a shows the confusion matrix heatmap of the grayscale diagram data set, the distribution of each color block is scattered. Each fault is misclassified, and the diagnosis accuracy is low. Figure 8b shows the confusion matrix heatmap of the Morlet wavelet transform TFD data set, from which it is obvious that the color block distribution is significantly better, indicating that, after the Morlet wavelet processing, the ResNet18 model has a certain improvement in the classification effect of bearing faults. Figure 8c shows the confusion matrix heatmap of the TFD data set when FSWT is applied, from which it can be seen that the color block distribution is obviously concentrated, and only individual diagrams are classified wrongly. The above conclusions indicating that the classification accuracy of the ResNet18 model is further improved after the FSWT processing, and has a good classification effect.
In order to ensure the well training effect of the fault diagnosis algorithm proposed in this paper, three types of hyperparameters in DC-ResNet are tested, which are learning rate, batch size and epoch. A total of 12 different groups of learning rate, batch size and epoch were selected for the experiments, and each group of experiments was repeated five times, and the final accuracy was the average of the five experiments, as shown in Table 3.
Learning Rate | Batch Size | Epoch | Average Accuracy (%) |
0.1 | 16 | 30 | 25.26 |
0.1 | 32 | 50 | 28.25 |
0.1 | 64 | 70 | 24.59 |
0.01 | 16 | 30 | 94.99 |
0.01 | 32 | 50 | 96.25 |
0.01 | 64 | 70 | 92.68 |
0.001 | 16 | 30 | 93.56 |
0.001 | 32 | 50 | 99.83 |
0.001 | 64 | 70 | 99.72 |
0.0001 | 16 | 30 | 85.33 |
0.0001 | 32 | 50 | 91.69 |
0.0001 | 64 | 70 | 87.16 |
From Table 3, when the learning rate = 0.1, the network cannot converge due to the large value of the learning rate, resulting in the phenomenon of gradient explosion; when the learning rate = 0.01, the learning rate is still large, and the network cannot converge precisely to the specified accuracy range; when the accuracy rate = 0.001, the accuracy rates are all at higher values, and when the batch size = 32, epoch = 50, the model accuracy reaches the peak; when the accuracy rate = 0.0001, the learning rate is so small, which leads to the slow convergence of the network and reduces the efficiency. In addition, it can be seen that, when the learning rate is kept constant and the batch size = 32 and epoch = 50, the model accuracy is higher than that of the model with other parameters in the same group. Therefore, the hyperparameters chosen in this paper are learning rate = 0.001, batch size = 32 and epoch = 50. In order to demonstrate the effectiveness of the DC-ResNet, comparative experiments are conducted on two different network models: ResNet50 and ResNet101. Then, using the same data set, deformable convolution layers are introduced in both ResNet50 and ResNet101, named DC-ResNet50 and DC-ResNet101. Each set of experiments are repeated five times, and the accuracy and the computing time of the program are recorded for each experiment. The final average accuracy and the average computing time of the program are obtained, as is shown in Table 4. From Table 4, compared with the ResNet50 and ResNet101, the average accuracy of the ResNet18 can reach 99.77%, and the accuracy is close to that of ResNet50 (99.82%) and ResNet101 (99.70%). However, the ResNet50 and ResNet101 are much larger than the proposed method in terms of program computing time. So, this proposed method has more advantages in combining both program computing time and accuracy, which proves that the DC-ResNet has certain advantages when performing bearing fault diagnosis and proves the effectiveness in this proposed method.
Experimental Methods | Input Samples | Average Accuracy (%) | Computing time(s) |
DC-ResNet | TFD of FSWT | 99.83 | 961.2 |
ResNet18 | 99.77 | 837.6 | |
DC-ResNet50 | 99.83 | 9279.6 | |
ResNet50 | 99.82 | 1300.2 | |
DC-ResNet101 | 99.76 | 18199.2 | |
ResNet101 | 99.70 | 1832.0 |
T-Distributed Stochastic Neighbor Embedding (T-SNE) is a technique that can reduce high-dimensional data to two-dimensional or three-dimensional, and visualize the spatial distribution of the data [48]. Figure 9 shows a schematic diagram of feature visualization after partial training samples are dimensionally reduced by T-SNE. Figure 9a shows the result of partial original training samples after being down scaled, from which it can be seen that the overlap between samples of different categories is very serious. Figure 9b shows the results of the dimensional reduction of the samples in the fully connected layer after DC-ResNet processing. Although there are still a small number of misclassifications, most of the categories can be separated. It is obvious that after DC-ResNet layer-by-layer feature extraction, all kinds of faults tend to separate from each other.
In addition, it can be seen from Table 4 that the diagnosis accuracy of the ResNet18 network model has reached 99.77%, and DC-ResNet has reached 99.83%. The experimental results obtained from DC-ResNet50 and DC-ResNet101 are much greater than the method used in terms of program computing time, with a small increase in both accuracies. It proves that this proposed method has higher diagnosis accuracy and higher efficiency with less computing time, and it is more suitable for bearing fault diagnosis.
In order to demonstrate, intuitively, the effectiveness of the DC-ResNet model proposed for classification detection on TFD of FSWT data set, the relationship between feature diagram and weight distribution diagram are analyzed by using the Gradient Weighted Class Activation Mapping (Grad-CAM) visual analysis technique [49], it can be judged whether the weight distribution of the model pays more attention to the feature area.
Figure 10, shows the visualization results of Grad CAM by using ResNet18 and DC-ResNet. It is shown that, not only the convolution kernel weights focus more on the region where the fault features are located, but also it has good recognition effect on the tiny fault features. It illustrates that the features extracted by the DC-ResNet are the regions where the fault features are located, proving that the model does not produce overfitting and has certain generalization ability.
The experimental platform used in this proposed method is the working conditions of the printing press-bearing fault diagnosis experimental platform, as is shown in Figure 11. The sensor used to measure the acceleration signal in the process of signal acquisition is a piezoelectric sensor, which is mounted on the rolling bearing seat for the acquisition of acceleration signal of the bearing, and the acquired signal is saved in the computer for bearing fault diagnosis, subsequently. The bearing model is JYB6004, and its main parameters are shown in Table 5. The bearing fault processing method adopts electron discharge machining, and the fault depths are 0.2 mm, 0.4 mm and 0.6 mm, respectively. Meanwhile, three kinds of faults are introduced into the bearing in three positions: inner race fault, outer race fault and cage fault. After completing the construction of the experimental platform of the rolling bearing of the printing press, the vibration acceleration data of the bearing fault under the actual working condition were collected. In this paper, the speed of the paper delivery shaft of the printing press is set to 800 RPM and the sampling frequency is 12 kHz, and the vibration data of each type of printing press bearing under normal condition is collected in this state, where the bearings with these fault types and the bearings in normal condition constitute the rolling bearing experimental data set used in this experiment. The data set contains a total of nine types of faults and one normal data.
Bearing type | Inner diameter /mm | Outer diameter /mm | Width /mm | Weight /g |
JYB6004 | 20 | 42 | 12 | 69 |
Figure 12 shows the time domain waveforms, the TFD after Morlet wavelet transform processing, and the TFD after FSWT processing of the bearing vibration signals collected under three types of faults: inner race fault, outer race fault and cage fault. From Figure 12, for the bearing vibration signals collected under the actual working conditions with strong noise, they are easily disturbed by noise in the process of time-frequency transform, which leads to the lack of obvious fault features extracted from the TFD after Morlet wavelet transform processing. At the same time, the TFD of FSWT has a good time-frequency focus, and the energy distribution is more concentrated. The TFD of FSWT is divided into a training set and a test set in the ratio of 7:3, which is the training set and the test set in the training process of the network model of the proposed method.
Figure 13 shows the control experimental data set. It uses grayscale diagrams formed by simply stitching the one-dimensional time-domain vibration signals into two-dimensional signals.
The accuracy and loss curve in one experimental iteration obtained by inputting the preprocessing TFD of the printing press bearings vibration signal into the DC-ResNet model is shown in Figure 14. It can be seen that the loss value gradually decreases with the increase in the number of iterations. At the same time, the accuracy rate shows a trend of gradual increase, and finally stabilizes at more than 90%. The analysis results show that the fault diagnosis method based on the DC-ResNet can extract the fault features from the TFD, effectively.
The DC-ResNet is compared with the AlexNet network and LSTM, and the TFD is used as the input of the neural network. The number of train samples is 10000, and the number of test samples is 3000, and each group of experiments is repeated five times, and the final results obtained by the three models are shown in Figure 15. Compared with the traditional AlexNet and LSTM network models, the DC-ResNet network structure improves fault diagnosis accuracy significantly. The average diagnosis accuracy can reach 93.90%, which proves that the network structure has certain advantages when performing bearing fault diagnosis in actual printing conditions with strong noise. From Figure 15, it can be seen that the accuracy and stability of the experimental results obtained by the three neural networks are significantly improved after using the FSWT preprocessing TFD as the input. When the TFD of FSWT is used as the input of shallow model with weak feature extraction ability, the shallow model diagnosis accuracy is improved. Therefore, the TFD of FSWT has superior performance in fault feature extraction.
In addition, it can also be seen from Figure 15 that the proposed method has high accuracy and well stability when FSWT is used for signal preprocessing. After five averages, the experimental results obtained by the three methods are shown in Table 6. It shows that the average diagnosis accuracy can reach 93.90%, which is significantly better than that of the compared methods, AlexNet (83.27%) and LSTM (83.36%).
Method | Preprocessing Method | Average Accuracy (%) |
DC-ResNet | FSWT | 93.90% |
Grayscale | 77.52% | |
ResNet18 | FSWT | 89.98% |
Grayscale | 67.14% | |
AlexNet | FSWT | 83.27% |
Grayscale | 68.20% | |
LSTM | FSWT | 83.36% |
Grayscale | 67.15% |
Table 7 shows the average diagnosis results with the introduction of deformable convolution layers. It shows that the DC-ResNet structure has better feature extraction ability than the ordinary ResNet18, and the average diagnosis accuracy can reach 93.90%, which is higher than ResNet18 (89.98%). When the diagnosis accuracy is guaranteed, it can be seen from the mean square error that the method proposed is more stable than other two methods. In summary, the DC-ResNet proposed has higher accuracy and stability in bearing fault diagnosis, and improves the performance of printing press bearing fault diagnosis.
Method | Preprocessing Method | Average Accuracy (%) | MSE |
DC-ResNet | FSWT | 93.90% | 0.0038 |
ResNet18 | 89.98% | 0.0106 |
The confusion matrix of the DC-ResNet on the printing press-bearing data set is shown in Figure 16. From the figure, it can be seen that the color block distribution is concentrated on the diagonal, where only individual images are misclassified, and the overall classification is good, which indicates the DC-ResNet achieves good classification results on the printing press-bearing data set.
Figure 17 shows the feature visualization diagram of some training samples of the printing press-bearing data set after T-SNE dimensional reduction. Figure 17a shows the results of the original training samples after T-SNE dimensional reduction, from which it can be seen that the overlap between samples of different categories is very serious. Figure 17b shows the results of the dimensional reduction of samples in the fully connected layer after DC-ResNet processing. There is still a small number of misclassifications, but most of the categories can be separated. It is obvious that, after the layer-by-layer feature extraction of the network model, the various types of faults tend to separate from each other.
Figure 18 shows the visualization results of Grad-CAM in ResNet18 and DC-ResNet. It is obvious from the figure that, for the printing press bearing data set, the ResNet18 is not effective in recognizing the fault features. However, the DC-ResNet pays more attention to the tiny fault features, and the recognition effect is good. It illustrates that the classification features extracted by the network model are the regions where the fault features are located, proving that the model does not produce overfitting and has certain generalization ability.
An intelligent bearing fault diagnosis method combining FSWT and DC-ResNet is proposed. Firstly, the vibration signal is preprocessed by FSWT to obtain the TFD. Secondly, the obtained TFD is input to ResNet to extract the fault features for fault identification. In this process, the deformable convolution layer is introduced for the strong noise vibration signal under the actual working condition of the printing press to improve the self-adaptability of the DC-ResNet. In the experimental part, the effectiveness of the proposed method is verified using the data set collected under experimental conditions and the data set of printing press bearings collected under actual working conditions. Thus, the analysis results can be concluded as follows:
(1) The FSWT is used to preprocess the original vibration signal, reducing the dependence on wavelet basis functions, while reconstructing the signal by wavelets and wavelet packets, and realizing the reconstruction of the signal in arbitrary frequency bands and the accurate description of local features. This preprocessing method can extract the information of bearing fault features effectively, and it can also improve the accuracy of the subsequent intelligent fault diagnosis model.
(2) In this proposed method, the traditional ResNet18 is improved for the complex and tiny fault features of strong noise vibration signals under the actual working conditions of printing presses. The ResNet convolution layer is reconstructed by using the deformable convolution structure. The DC-ResNet can learn more detailed features by using the adaptive nature of the shape of the deformable convolution layer, which improves the accuracy of intelligent fault diagnosis of printing press bearings.
(3) The effectiveness of the method was verified using experiments. Among them, the accuracy reaches 99.77% under experimental conditions and 93.90% for the data set of printing press bearings under actual working conditions. The experimental results show that the DC-ResNet can classify different rolling bearings faults under strong background noise, and the accuracy and stability of fault diagnosis are greatly improved.
This research is supported by the Natural Science Foundation of Shanxi Province (Grant No.2022JZ-30), and Scientific Research Program of Shanxi Provincial Department of Education (Grant No.20JY054).
We declare that there are no conflicts of interest.
[1] | J. Smoller, Shock waves and reaction diffusion equations, volume 258. Springer Science & Business Media, 2012. |
[2] | B. Perthame, Transport equations in biology, Frontiers in Mathematics. Birkhäuser Verlag, Basel, 2007. |
[3] | L. Pareschi and G. Toscani, Interacting multiagent systems: kinetic equations and Monte Carlo methods, OUP Oxford, 2013. |
[4] | M. Pérez-Llanos, J. P. Pinasco, N. Saintier, et al., Opinion formation models with heterogeneous persuasion and zealotry, SIAM J. Math. Anal., 50 (2018), 4812-4837. |
[5] | L. Pedraza, J. P. Pinasco and Saintier, Measure-valued opinion dynamics, submitted, 2019. |
[6] | F. Camilli, R. De Maio and A. Tosin, Transport of measures on networks, Netw. Heterog. Media, 12 (2017), 191-215. |
[7] | F. Camilli, R. De Maio and A. Tosin, Measure-valued solutions to nonlocal transport equations on networks, J. Differ. Equations, 264 (12), 7213-7241. |
[8] | S. Cacace, F. Camilli, R. De Maio, et al., A measure theoretic approach to traffic flow optimisation on networks, Eur. J. Appl. Math., (2018), 1-23. |
[9] | J. A. Cañizo, J. A. Carrillo and S. Cuadrado, Measure solutions for some models in population dynamics, Acta Appl. Math., 123 (2013), 141-156. |
[10] | M. Di Francesco and S. Fagioli, Measure solutions for non-local interaction pdes with two species, Nonlinearity, 26 (2013), 2777. |
[11] | J. A. Carrillo, R. M. Colombo, P. Gwiazda, et al., Structured populations, cell growth and measure valued balance laws, J. Differ. Equations, 252 (2012), 3245-3277. |
[12] | J. H. M. Evers, S. C. Hille and A. Muntean, Mild solutions to a measure-valued mass evolution problem with flux boundary conditions, J. Differ. Equations, 259 (2015), 1068-1097. |
[13] | K. Adoteye, H. T. Banks and K. B. Flores, Optimal design of non-equilibrium experiments for genetic network interrogation, Appl. Math. Lett., 40 (2015), 84-89. |
[14] | M. Burger, Infinite-dimensional optimization and optimal design, 2003. |
[15] | H. T. Banks and K. Kunisch, Estimation techniques for distributed parameter systems, Birkhäuser Verlag, Basel, 1989. |
[16] | A. S. Ackleh, J. Carter, K. Deng, et al., Fitting a structured juvenile-adult model for green tree frogs to population estimates from capture-mark-recapture field data, Bull. Math. Biol., 74 (2012), 641-665. |
[17] | M. T. Wentworth, R. C. Smith and H. T. Banks, Parameter selection and verification techniques based on global sensitivity analysis illustrated for an hiv model, SIAM-ASA J. Uncertain., 4 (2016), 266-297. |
[18] | A. S. Ackleh, X. Li and B. Ma, Parameter estimation in a size-structured population model with distributed states-at-birth, In IFIP Conference on System Modeling and Optimization, pages 43-57. Springer, 2015. |
[19] | A. S. Ackleh and R. L. Miller, A model for the interaction of phytoplankton aggregates and the environment: approximation and parameter estimation, Inverse Probl. Sci. En., 26 (2018), 152-182. |
[20] | J. A. Canizo, J. A. Carrillo and J. Rosado, A well-posedness theory in measures for some kinetic models of collective motion, Math. Mod. Meth. Appl. S., 21 (2011), 515-539. |
[21] | S. Maniglia, Probabilistic representation and uniqueness results for measure-valued solutions of transport equations, J. Math. Pures Appl., 87 (2007), 601-626. |
[22] | P. Gwiazda, T. Lorenz and A. Marciniak-Czochra, A nonlinear structured population model: Lipschitz continuity of measure-valued solutions with respect to model ingredients, J. Differ. Equations, 248 (2010), 2703-2735. |
[23] | P. Gwiazda, S. C. Hille, K. Łyczek, et al., Differentiability in perturbation parameter of measure solutions to perturbed transport equation, arXiv preprint arXiv:1806.00357, 2018. |
[24] | J. Skrzeczkowski, Measure solutions to perturbed structured population models-differentiability with respect to perturbation parameter, arXiv preprint arXiv:1812.01747, 2018. |
[25] | C. Villani, Topics in optimal transportation, Springer Texts in Statistics. Springer, New York, 2006. |
[26] | K. B. Athreya and S. N. Lahiri, Measure theory and probability theory, Springer Texts in Statistics. Springer, New York, 2006. |
[27] | L. Ambrosio, N. Gigli and G. Savaré, Gradient flows in metric spaces and in the space of probability measures, Lectures in Mathematics ETH Zürich. Birkhäuser Verlag, Basel, second edition, 2008. |
[28] | H. Brezis, Functional analysis, Sobolev spaces and partial differential equations, Universitext. Springer, New York, 2011. |
[29] | L. Székelyhidi, Jr. From isometric embeddings to turbulence, In HCDTE lecture notes. Part Ⅱ. Nonlinear hyperbolic PDEs, dispersive and transport equations, volume 7 of AIMS Ser. Appl. Math., page 63. Am. Inst. Math. Sci. (AIMS), Springfield, MO, 2013. |
[30] | L. C. Evans, Partial differential equations, volume 19 of Graduate Studies in Mathematics, American Mathematical Society, Providence, RI, second edition, 2010. |
[31] |
P. Gwiazda, J. Jabłoński, A. Marciniak-Czochra, et al., Analysis of particle methods for structured population models with nonlocal boundary term in the framework of bounded lipschitz distance, Numer. Meth. Part. D. E., 30 (2014), 1797-1820. doi: 10.1002/num.21879
![]() |
[32] | J. A. Carrillo, P. Gwiazda and A. Ulikowska, Splitting-particle methods for structured population models: convergence and applications, Math. Mod. Meth. Appl. S., 24 (2014), 2171-2197. |
[33] | R. M. Dudley, Convergence of Baire measures, Studia Math., 27 (1966), 251-268. |
DC-ResNet Structure | Output Dimension |
Input Layer | (190,150, 3) |
Convolution Layer 1 | (184,144, 64) |
Max Pooling | (92, 72, 64) |
Residual Block 1 | (92, 72, 64) |
Residual Block 2 | (92, 72, 64) |
Residual Block 3 | (92, 72,128) |
Residual Block 4 | (92, 72,128) |
Residual Block 5 | (92, 72,256) |
Residual Block 6 | (92, 72,256) |
Residual Block 7 | (92, 72,512) |
Residual Block 8 | (92, 72,512) |
Global Average Pooling | 512 |
Output Layer | 10 |
Preprocessing Methods | Number of Training Samples/Test Samples | Average Accuracy (%) |
FSWT | 3000/1000 | 99.77 |
Morlet Wavelet Transform | 88.72 | |
Grayscale | 74.92 |
Learning Rate | Batch Size | Epoch | Average Accuracy (%) |
0.1 | 16 | 30 | 25.26 |
0.1 | 32 | 50 | 28.25 |
0.1 | 64 | 70 | 24.59 |
0.01 | 16 | 30 | 94.99 |
0.01 | 32 | 50 | 96.25 |
0.01 | 64 | 70 | 92.68 |
0.001 | 16 | 30 | 93.56 |
0.001 | 32 | 50 | 99.83 |
0.001 | 64 | 70 | 99.72 |
0.0001 | 16 | 30 | 85.33 |
0.0001 | 32 | 50 | 91.69 |
0.0001 | 64 | 70 | 87.16 |
Experimental Methods | Input Samples | Average Accuracy (%) | Computing time(s) |
DC-ResNet | TFD of FSWT | 99.83 | 961.2 |
ResNet18 | 99.77 | 837.6 | |
DC-ResNet50 | 99.83 | 9279.6 | |
ResNet50 | 99.82 | 1300.2 | |
DC-ResNet101 | 99.76 | 18199.2 | |
ResNet101 | 99.70 | 1832.0 |
Bearing type | Inner diameter /mm | Outer diameter /mm | Width /mm | Weight /g |
JYB6004 | 20 | 42 | 12 | 69 |
Method | Preprocessing Method | Average Accuracy (%) |
DC-ResNet | FSWT | 93.90% |
Grayscale | 77.52% | |
ResNet18 | FSWT | 89.98% |
Grayscale | 67.14% | |
AlexNet | FSWT | 83.27% |
Grayscale | 68.20% | |
LSTM | FSWT | 83.36% |
Grayscale | 67.15% |
Method | Preprocessing Method | Average Accuracy (%) | MSE |
DC-ResNet | FSWT | 93.90% | 0.0038 |
ResNet18 | 89.98% | 0.0106 |
DC-ResNet Structure | Output Dimension |
Input Layer | (190,150, 3) |
Convolution Layer 1 | (184,144, 64) |
Max Pooling | (92, 72, 64) |
Residual Block 1 | (92, 72, 64) |
Residual Block 2 | (92, 72, 64) |
Residual Block 3 | (92, 72,128) |
Residual Block 4 | (92, 72,128) |
Residual Block 5 | (92, 72,256) |
Residual Block 6 | (92, 72,256) |
Residual Block 7 | (92, 72,512) |
Residual Block 8 | (92, 72,512) |
Global Average Pooling | 512 |
Output Layer | 10 |
Preprocessing Methods | Number of Training Samples/Test Samples | Average Accuracy (%) |
FSWT | 3000/1000 | 99.77 |
Morlet Wavelet Transform | 88.72 | |
Grayscale | 74.92 |
Learning Rate | Batch Size | Epoch | Average Accuracy (%) |
0.1 | 16 | 30 | 25.26 |
0.1 | 32 | 50 | 28.25 |
0.1 | 64 | 70 | 24.59 |
0.01 | 16 | 30 | 94.99 |
0.01 | 32 | 50 | 96.25 |
0.01 | 64 | 70 | 92.68 |
0.001 | 16 | 30 | 93.56 |
0.001 | 32 | 50 | 99.83 |
0.001 | 64 | 70 | 99.72 |
0.0001 | 16 | 30 | 85.33 |
0.0001 | 32 | 50 | 91.69 |
0.0001 | 64 | 70 | 87.16 |
Experimental Methods | Input Samples | Average Accuracy (%) | Computing time(s) |
DC-ResNet | TFD of FSWT | 99.83 | 961.2 |
ResNet18 | 99.77 | 837.6 | |
DC-ResNet50 | 99.83 | 9279.6 | |
ResNet50 | 99.82 | 1300.2 | |
DC-ResNet101 | 99.76 | 18199.2 | |
ResNet101 | 99.70 | 1832.0 |
Bearing type | Inner diameter /mm | Outer diameter /mm | Width /mm | Weight /g |
JYB6004 | 20 | 42 | 12 | 69 |
Method | Preprocessing Method | Average Accuracy (%) |
DC-ResNet | FSWT | 93.90% |
Grayscale | 77.52% | |
ResNet18 | FSWT | 89.98% |
Grayscale | 67.14% | |
AlexNet | FSWT | 83.27% |
Grayscale | 68.20% | |
LSTM | FSWT | 83.36% |
Grayscale | 67.15% |
Method | Preprocessing Method | Average Accuracy (%) | MSE |
DC-ResNet | FSWT | 93.90% | 0.0038 |
ResNet18 | 89.98% | 0.0106 |