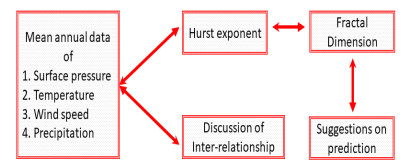
Climate change is an inevitable and important problem faced worldwide. Around the world, many researchers are doing research work on climate change with different factors. The authors of this study have used the fractal dimension to analyze the 41 years of climate change in India, from 1981 to 2021. The meteorological parameters, surface pressure, temperature, wind speed, and precipitation of 45 places in India were investigated for this research study. Each parameter was individually examined. As a result of this research, the mean average fractal dimension value of all parameters was acquired from 1.184 to 1.198. It was found that all the parameters within the data set, had a long-term persistence behavior. With these values, a suggestion for the prediction of the parameters was proposed. The results of the integrated analysis of these parameters showed that, with the exception of a location, all landscapes had a feature that precipitation increased with the temperature. Moreover, with the exception of two landscapes (the island and the frosty mountains), all areas received heavy rainfall during periods of low wind. Thus, this study contributes to a better understanding of the fractal aspects of climate change and the complexity of irregularity and the classification of weather characteristics.
Citation: M. Meenakshi, A. Gowrisankar, Jinde Cao, Pankajam Natarajan. Fractal dimension approach on climate analysis of India[J]. Mathematical Modelling and Control, 2025, 5(1): 15-30. doi: 10.3934/mmc.2025002
[1] | C. Kavitha, A. Gowrisankar . Fractional integral approach on nonlinear fractal function and its application. Mathematical Modelling and Control, 2024, 4(3): 230-245. doi: 10.3934/mmc.2024019 |
[2] | Yi Tian . Approximate solution of initial boundary value problems for ordinary differential equations with fractal derivative. Mathematical Modelling and Control, 2022, 2(2): 75-80. doi: 10.3934/mmc.2022009 |
[3] | Rashid Jan, Normy Norfiza Abdul Razak, Sania Qureshi, Imtiaz Ahmad, Salma Bahramand . Modeling Rift Valley fever transmission: insights from fractal-fractional dynamics with the Caputo derivative. Mathematical Modelling and Control, 2024, 4(2): 163-177. doi: 10.3934/mmc.2024015 |
[4] | Weiguang Zhou, Guangxin Wang, Jixiao Lu, Hongxin Ruan, Jun Wang, Rui Zhang . On a data model associated with antitrust behaviors. Mathematical Modelling and Control, 2024, 4(4): 459-469. doi: 10.3934/mmc.2024036 |
[5] | Ihtisham Ul Haq, Nigar Ali, Hijaz Ahmad . Analysis of a chaotic system using fractal-fractional derivatives with exponential decay type kernels. Mathematical Modelling and Control, 2022, 2(4): 185-199. doi: 10.3934/mmc.2022019 |
[6] | Daizhan Cheng, Zhengping Ji, Jun-e Feng, Shihua Fu, Jianli Zhao . Perfect hypercomplex algebras: Semi-tensor product approach. Mathematical Modelling and Control, 2021, 1(4): 177-187. doi: 10.3934/mmc.2021017 |
[7] | J. O. Akanni, S. Ajao, S. F. Abimbade, Fatmawati . Dynamical analysis of COVID-19 and tuberculosis co-infection using mathematical modelling approach. Mathematical Modelling and Control, 2024, 4(2): 208-229. doi: 10.3934/mmc.2024018 |
[8] | Anusmita Das, Kaushik Dehingia, Nabajit Ray, Hemanta Kumar Sarmah . Stability analysis of a targeted chemotherapy-cancer model. Mathematical Modelling and Control, 2023, 3(2): 116-126. doi: 10.3934/mmc.2023011 |
[9] | Xingwen Liu, Shouming Zhong . Stability analysis of delayed switched cascade nonlinear systems with uniform switching signals. Mathematical Modelling and Control, 2021, 1(2): 90-101. doi: 10.3934/mmc.2021007 |
[10] | Kutorzi Edwin Yao, Mahvish Samar, Yufeng Shi . Approximation approach for backward stochastic Volterra integral equations. Mathematical Modelling and Control, 2024, 4(4): 390-399. doi: 10.3934/mmc.2024031 |
Climate change is an inevitable and important problem faced worldwide. Around the world, many researchers are doing research work on climate change with different factors. The authors of this study have used the fractal dimension to analyze the 41 years of climate change in India, from 1981 to 2021. The meteorological parameters, surface pressure, temperature, wind speed, and precipitation of 45 places in India were investigated for this research study. Each parameter was individually examined. As a result of this research, the mean average fractal dimension value of all parameters was acquired from 1.184 to 1.198. It was found that all the parameters within the data set, had a long-term persistence behavior. With these values, a suggestion for the prediction of the parameters was proposed. The results of the integrated analysis of these parameters showed that, with the exception of a location, all landscapes had a feature that precipitation increased with the temperature. Moreover, with the exception of two landscapes (the island and the frosty mountains), all areas received heavy rainfall during periods of low wind. Thus, this study contributes to a better understanding of the fractal aspects of climate change and the complexity of irregularity and the classification of weather characteristics.
Climate change refers to the long-term shifts of temperature and weather patterns in environmental conditions of the earth. Climate change occurs due to many internal and external factors such as greenhouse effect, burning fossil fuels, deforestation, urbanization, pollution from industry revolution, etc. Climate change has become a global concern over the last few decades since it affects the livelihood of creatures on the earth in various ways. The climatic conditions are highly dependent on the surface pressure, temperature, wind speed, and precipitation that eventually influences seasons and discerns the dynamics of wetness and dryness.
Climate change is an inevitable and important issue of the world. Globally all livelihoods are realizing the changes in climate by feeling high temperatures, heavy rainfalls, landslides, earthquakes, snowfall, toxic foam in rivers, heat waves, droughts, wildfires, tsunamis, the submergence of land under sea water, storms, hurricanes, and so on. Nowadays, scientists are under pressure, and struggle more to control and reduce the pollution. Many scientists have devoted their life to protect the earth and livelihoods. For the reason, the United Nations (UN) has taken many measures on climate change. The report of climate change, submitted by the UN on 4th, April 2022, declared that "to reduce the global warming limit to below 1.5 degree Celsius, if not so then the world is on a 'fast track' to disaster".
According to the most recent flagship report from the World Meteorological Organization, released on 18th May 2022, greenhouse gas concentrations, sea level, ocean heat levels, and acidity all set new records for 2021, while some glaciers surpassed the point of no return. The sustainable development goals, also known as the global goals, have been adopted by the UN in 2015 as a universal call to action to end poverty, protect the planet, and ensure that all people enjoy peace and prosperity by 2030. The global sustainable development Report of 2023 expected to achieve the aforementioned points [1].
The authors of the article [2] mentioned that; the greenhouse gases emission rate would be more than 6 tons at the end of 2047 and is causing more changes in the atmosphere, on land, and in the seas. India ranks third in the world for emitting greenhouse gases. For this, in June 2008, a "National Action Plan on Climate Change" was unveiled in order to realize India's objective of sustainable development in the face of climate change. Eight particular goals were listed in this "National Action Plan", including "National Mission for Sustaining the Himalayan Eco-Systems" and "National Mission on Strategic Knowledge for Climate Change". The Department of Science & Technology of the Ministry of Science Technology, is in charge of these two missions [3,4].
According to the meteorological department, six decisive factors influence the climate condition. These factors are mountains, latitude, surface distance from the sea and land, water distribution, relief, and altitude. The geographical feature of India, is surrounded by the bay of Bengal in the east, the Arabian Sea in the west, and the Indian Ocean in the south. The Himalayas are snow mountains, which act as a barrier from the north. The Thar desert is located to the northwest. The Indo-Gangetic plain with three major rivers (the Ganges, the Indus, and the Brahmaputra), Plateaus, and Valleys are also there. Additionally, India has two Islands, Andaman and Nicobar Islands in the Bay of Bengal, and the Lakshadweep Islands in the Arabian Sea. From all these types of terrains, the authors have randomly chosen locations for this research.
In addition to the above, the climatic conditions of India are highly dependent upon wind flow, rainfall, temperature, and surface pressure, which eventually influence the seasons, and discern the dynamics of wetness and dryness. Due to this, India has four major and broad climate conditions: tropical wet, tropical dry, subtropical humid, and montane. Moreover, India has two monsoon rainfalls: one is the North-East monsoon (October-November) formed from the Bay of Bengal; and the other is the South-West monsoon (June-September) formed from the Arabian Sea. India may be classified into several areas based on climate. The majority of the country has a tropical climate, with a mix of rainy and dry tropical weather in the interior. The northern regions have a humid tropical climate, and the wet tropical regions are near the western coast. A semi-arid climate that also covers the northwest of the nation extends in a band up the center of the continent. India can endure extremely chilly temperatures, even though stereotypes often depict the nation as being hot. This occurs primarily in the cold, arid, and windswept Himalayas and other northern mountainous areas [5].
Many researchers study how climate change affects various countries' meteorological parameters, including the surface pressure, temperature, wind speed, and precipitation. We observed that no one has ever conducted a comprehensive and integrated analysis of these parameters in relation to the Indian climate. Thus, in this article, the authors examined the Indian climate using both individual and integrated analyses of those parameters that were examined in connection to one another. Thus the study contributes to a better understanding of the fractal aspects of climate change and the complexity of irregularity and the classification of weather characteristics. This study aims to determine how parameters such as surface pressure, temperature, wind speed, and precipitation affect the Indian climate. These four parameters are interrelated. Changes in one of these parameters can often influence or be influenced by others. These four natural parameters are briefly described below.
The weather is determined by one of the parameters, namely atmospheric pressure [6]. The air around us has weight, and it presses against everything it touches. That pressure is called either atmospheric pressure, air pressure, or surface pressure. Cloudiness, wind, storms, and precipitation are frequently experienced when a low-pressure system enters a region, while fair, stable, and tranquil weather conditions are formed by a high-pressure system. The unit of surface pressure is kilo Pascal (kPa). The next parameter is temperature, which is a physical quantity that quantitatively expresses the perceptions of hotness and coldness [7]. In this article, the unit of temperature is mentioned as the degree of celsius (∘C). An increase in temperature generates a hot climate, which results in precipitation. It can affect the distribution of pressure and influences wind patterns. Another important parameter is the wind speed which is the movement of air from areas of high pressure to low pressure. In addition to bringing hot or cold air into a climate, wind also introduces moisture into the atmosphere, changing weather patterns. Therefore, as the wind changes, the weather also changes. Air pressure in places of low pressure is a significant determinant of wind direction. Low-pressure systems are often connected with thunderstorms and winter storms [8]. The unit of measurement is m/s. Wind speed is affected by factors such as pressure gradients, surface roughness, and the rotation of the Earth (Coriolis effect). Another important parameter is precipitation. Any sort of liquid or frozen water that condenses in the sky and eventually makes its way back down to Earth's surface is referred to as precipitation. After becoming too heavy to remain suspended in the air, precipitation falls to the earth. It can fall as rain, sleet, hail, graupel, or snow, among other types of precipitation. One of the three key components that makes up the water cycle on a global scale is precipitation. It is primarily influenced by temperature, humidity, and atmospheric instability. Raising air, cools and condenses, thus forming clouds and eventually leading to precipitation. The unit of precipitation is mm/day [9].
Mandelbrot and Wallis were the first persons to apply a fractal analysis to some geophysical records for a long-run time series study. They utilized the Hurst general idea for long range statistical dependence in geophysical records. This was followed by many researchers for weather conditions, climate change, and so on. [10]. Bodri said that mean annual surface air temperature is a fractal, he concluded that it gives a more accurate value even with a small time span compared to other statistical approaches of a large time span [11]. Therefore, the authors of this article have taken parameter data for the period of 41 years. In [12], Govindhan and Sant used a fractal dimensional analysis to investigate the Indian climate dynamics with three parameters-temperature, pressure, and precipitation. For this research work, another weather parameter, namely wind speed is included.
The article [13] of Sahu and Mishra explained how the farmers were affected by climate change and the reasons for no youngster's interest in agriculture in Odisha. Farmers are struggling due to climate change, not only in Odisha, but all over India. The article [4] by Tewari et al. discussed India's western Himalayan and reviewed evidence of warming and changes in precipitation and its extreme events. Many scientists have stated that if global warming is not controlled then all the glaciers will melt and the water level will increase in the world. Due to this, there is a danger that many lands will submerge under the seawater.
The daily average time series of air temperature, surface pressure, relative humidity, and wind speed for nine meteorological stations in Saudi Arabia were examined by authors Rehman and Siddiqi for their study [14], which examined the measure of climate predictability. They utilized the 16 years of data from 1990 to 2005. Once they had the Hurst exponent, they discovered the fractal dimension by applying the wavelets technique. Temperature and pressure were shown to have no effect on the precipitation and wind speed time series climate prediction indices. Additionally, individual parameter predictability indices were shown to exhibit persistent behavior across all seasons for the entire data set, but anti-persistence in the majority of cases for the winter and summer data sets. Using a fractal dimension, Cui et al. explored wind speeds during typhoons [15]. They discovered that the wind speed time series was anti-persistent and that the fractal dimension fluctuated between 1.55 and 1.75 across the typhoon. Their research made it easier to figure out the fractal aspects in wind speeds during typhoons, and the results imply that typhoon simulation systems could benefit from including the fractal dimension's properties.
From the above literature survey, it is observed that no one had ever worked under climate change with these four parameters together for Indian climate utilizing a fractal dimension. We ensure that this paper attempts to fill the gap in the literature review by combining research work with surface pressure, temperature, wind speed, and precipitation for the Indian climate. A fractal dimensional analysis of geophysical time series is a well-established investigative tool to explore the dynamics by a scale invariant. Geophysical and geological phenomena appear scale invariant and may be studied within the framework of fractal geometry.
A schematic diagram illustrating the workflow of this study is presented in Figure 1.
It visually represents the key components and their relationships within the research framework. Four meteorological parameters (surface pressure, temperature, wind speed, and precipitation) are analysed in this paper using the Hurst exponent and fractal dimension. Thee fractal dimension value and the correlation between each mean annual parameter is examined. The procedure and supplies used in this work are outlined in Section 2. Data sources and related information are provided in Section 3. The Hurst exponent and fractal dimension approach are explained in Section 4. The findings of this study are displayed in Section 5. Section 6 delineates the interdependencies among the four parameters. In Section 7, the authors provide their conclusions and observations based on their findings.
A time series is a group of observations of well-defined data points produced over time via successive measurements. The statistical characteristics of a fractal time series are drastically different from those of a conventional one. For instance, it might be of the power spectrum function, a slowly decaying auto-correlation function, or a heavy-tailed probability distribution function type. It may be statistically dependent, short or long-range dependent, and exhibit global or local self-similarity. In contrast to ordinary time series, fractal ones have unique properties of their own. The idea of memory is generally strongly tied to its power law. One thing to keep in mind is that a series like this typically lacks a mean and/or variance. This might be a major factor in the use of the Hurst parameter and measurements of a fractal dimension in the study of fractal time series[16].
A shift over time is referred to as a trend. A trend line or line of best fit is a line drawn on a graph to indicate a pattern or trend. A line graph and a trend line are not the same thing. It is not simply put together by joining points; rather, it is put together to see whether a pattern emerges. A linear trend line often depicts a consistent increase or decrease in a variable. Predictions may also be made using trend lines. Increased or decreased patterns in the weather, such as temperature or precipitation, are one application where trend lines are employed in the real world. Scatter plots are typically used to create trend lines. In the article [17], the positive slope, negative slope, and slope of zero on the trend line showed how the variables related to one another.
The Hurst exponent is used as a measure of long-term memory for time series. It relates the auto-correlations of the time series, and the rate at which these decrease as the lag between pairs of values increases. The Hurst exponent was proposed by British hydrologist Hurst for the practical matter of determining optimum dam sizing for the Nile River's volatile rain and drought conditions that had been observed over a long period of time. He observed that both the axis of time and a statistical quantity were not equivalent for an observation or time series [18]. Therefore, a rescaling of time is necessary to adjust the time series. The authors Santo et al.[19] rescaled the time scale k ∈ N by a factor ′a′ and the corresponding time series x(k)Nk=1 by
aHx(ak) |
(i.e.,aHx(ak)→x(k)), which actually reflected the statistical self-similarity (self-affinity) of the time series. Then, they computed the exponent H based on the statistical quantity applied to the rescaled range analysis, which is the Hurst exponent (H). The H is referred to as the "index of dependence" or "index of long-range dependence". It quantifies the relative tendency of a time series to either regress to the mean or to cluster in one direction. Hurst's claim led to the following estimation procedure: In the cases where R(t,s)/S(t,s) is known for a single sample from t+1 to t+s, he estimates H as equal to the following
K(t,s)=log(R/S)/(logs−log2). |
In cases where R(t,s)/S(t,s) is known for more than one sample, he averages the values of the function K(t,s) that corresponds to all the points of coordinates s and R/S. The H value in the range of 0.5 to 1 indicates a time series with a long-term positive auto correlation, meaning that both the high value and the values of long time into the future will also tend to be high. The H value in the range of 0 to 0.5 indicates a time series with a long-term switching between the high and low values in adjacent pairs, meaning that a single high value will probably be followed by a low value, and that the value after that will tend to be high, with this tendency to switch between high and low values lasting a long time into the future. The value of H=0.5 can indicate a completely uncorrelated series; however, in fact, it is the value applicable to the series for which the auto correlations at small time lags can be positive or negative, but where the absolute values of the auto correlations decay exponentially quickly to zero. This is in contrast, to the typically power-law decay for the
0.5<H<1 |
and
0<H<0.5 |
Mandelbrot coined the word fractal from the Latin word "fractus" in 1975, which means irregular or fragmented. (i.e., broken or fractured). Therefore, he is called the father of fractal geometry. Fractals are endless and complex geometric shapes with fine structures at arbitrarily small scales. In other words, the magnification of the tiny features of a fractal is reminiscent of the whole. Similarity can be exact (invariant), but more often it is approximate or statistical. Based on the self-similar property, fractals are classified into two types: random fractals (statistically self-similar) and deterministic fractals (exactly self-similar). Some examples of a random fractal are cauliflower, neem tree, coastal lines, ightning, and so on, and some examples of deterministic fractals are Cantor set, Von Coach, Sierpinski triangle, Mengers sponge, and so on [23,24,25]. Quantifying the degree of variation around the main trend of the data as it changes over time is the foundation of a fractal analysis of time series. This is done to assess the scale invariance, which is represented by the value of H.
Fractals are subsets of Euclidean space that can have fractional (non-integer) dimensions. According to Mandelbrot, the definition of a fractal is "a set for which the Hausdorff-Besicovitch dimension strictly exceeds the topological dimension" [26,27]. There are various ways to determine the fractal dimension of a time series. Some examples include the box-counting method, the similarity dimension method, and so on. Complexity and irregularities can be naturally found in many physical and biological nonlinear systems naturally and have been analyzed by the tools of fractal theory and computed by the non-integer or fractional measure called fractal dimension. Fractals themselves have their dimension, known as the fractal dimension. It is a measure of the prominence of irregularities in a set when viewed at very small scales. It contains much information about the geometrical properties of a set [28]. It is usually (but not always) a non-integer dimension that is greater than their topological dimension DT, and less than their Euclidean dimension DE.
The similarity dimension, denoted DS, characterizes the construction of regular fractal objects. The measure of the (similarity) dimension of the set made up of N copies of itself, scaled by a factor 1/ϵ might be thought of as having the following dimension:
DS=logNlog(1/ϵ) |
(or) the number of objects N with a characteristic size greater than r scales with the relation
N=Cr−D, |
where D is the similarity dimension and C is a constant of proportionality [29].
In fractal geometry, the generalized Hurst exponent has been denoted by H and HQ in honor of both Harold Edwin Hurst and Ludwig Otto Holder by Benoit Mandelbrot. In this study, the Hurst exponent and the fractal dimension have been denoted as "H" and "D". "H" is directly related to the fractal dimension "D" and is a measure of a data series "mild" or "wild" randomness. The Hurst coefficient H defines a fractal dimension D as the following:
H=E+1−D, | (2.1) |
where E is the Euclidean dimension and the measure of E is 0 for a point, 1 for a line, and 2 for a surface. Whenever H is less than 1, then the object intrudes into the next dimension, just as a curved or rough surface requires embedding in three-dimensional (3-D) space to be visualized [30]. Substitute E=1 in Eq (2.1), then,
H=2−DorD=2−H. | (2.2) |
That is, for a one-dimensional (1-D) object such as a time-varying function, it is represented as
H=2−D |
as in the article [31].
The data used in this article are taken from the website Power Data Access Viewer, which provides solar and meteorological data sets from NASA research for support of renewable energy, building energy efficiency, and agricultural needs. The time series of mean annual surface pressure (MASP), mean annual temperature (MAT), mean annual wind speed (MAWS), and mean annual precipitation (MAP) for 41 years from 1981 to 2021 was selected. These data on MASP, MAT, MAWS, and MAP are nothing more than averages of the following variables: surface pressure at the earth's surface; air (dry bulb) temperature at a height of 10 metres; wind speed at a height of 2 metres; and a bias-corrected average of total precipitation at the earth's surface in water mass (including water content in snow). Since India has different terrains, locations for this research work were selected from 45 places such as coastal regions, mountain areas, plateaus, deserts, Islands, and plains. The locations selected for this research work are given in the following Table 1.
SI. No. | Location name | Latitude | Longitude |
1 | Agartala, West Tripura, Tripura | 23.8296 | 91.2901 |
2 | Agumbe, Kundapura, Udupi, Karnataka | 13.508 | 75.0946 |
3 | Amaravathi, Guntur, Andhra Pradesh | 16.5703 | 80.3606 |
4 | Balasore, Odisha | 21.5053 | 86.9159 |
5 | Bānda, Uttar Pradesh | 25.4754 | 80.3356 |
6 | Bengaluru, Karnataka | 12.9703 | 77.5855 |
7 | Bhopal, Madhya Pradesh | 23.2644 | 77.4018 |
8 | Bhubaneswar, Odhisa | 20.2698 | 85.841 |
9 | Bilaspur, Chhattisgarh | 22.0787 | 82.1403 |
10 | Bramhapuri, Mahārāshtra | 20.6 | 79.8666 |
11 | Chandigarh, Harayana | 30.7306 | 76.8478 |
12 | Chennai, Tamil Nadu | 13.0549 | 80.2311 |
13 | Cherrapunji, East Khasi Hills, Meghalaya | 25.2851 | 91.7184 |
14 | Chittoor, Andhra Pradesh | 13.2067 | 79.0883 |
15 | Cuddalore, Tamil Nadu | 11.7462 | 79.7586 |
16 | Delhi, New Delhi, Delhi | 28.6342 | 77.2168 |
17 | Dispur, Kamrup Metropolitan, Assam | 26.136 | 91.8081 |
18 | Gairsain, Chamoli, Uttarakhand | 30.0554 | 79.2904 |
19 | Gandhinagar, Gujarat | 23.2413 | 72.638 |
20 | Imphal, Imphal West, Manipur | 24.8077 | 93.9273 |
21 | Jaisalmer, Rajasthan | 26.9194 | 70.9146 |
22 | Kavaratti Sub-Division, Lakshadweep | 10.5644 | 72.639 |
23 | Kodaikanal, Dindigul, Tamil Nadu | 10.2312 | 77.4882 |
24 | Kohima, Nagaland | 25.6631 | 94.0933 |
25 | Kolkata, West Bengal | 22.5708 | 88.372 |
26 | Kottayam, Kerala | 9.5947 | 76.5328 |
27 | Lachen, Northen sikkim | 27.7456 | 88.5014 |
28 | Ladākh, Jammu and Kashmir | 34.337 | 77.4057 |
29 | Ludiana, Punjab | 30.9063 | 75.8489 |
30 | Mahabaleshwar, Satara, Maharashtra | 17.9262 | 73.6593 |
31 | Mawsynram, East Khasi Hills, Meghalaya | 25.2996 | 91.5828 |
32 | Mumbai, Maharashtra | 18.9373 | 72.8349 |
33 | Munnar, Devikulam, Idukki, Kerala | 10.0742 | 77.0625 |
34 | Nagpur, Maharashtra | 21.1563 | 79.0821 |
35 | Ooty, The Nilgiris, Tamil Nadu | 11.4059 | 76.6991 |
36 | Patna, Bihar | 25.6015 | 85.1372 |
37 | Port Blair, Andaman & Nicobar Islands | 11.6629 | 92.7391 |
38 | Ramagundam, Peddapalle, Telangana | 18.7565 | 79.4843 |
39 | Ranchi, Jharkhand | 23.3515 | 85.3127 |
40 | Shimla, Himachal Pradesh | 31.1033 | 77.1581 |
41 | Sriganganagar, Rajasthan | 29.912 | 73.8756 |
42 | Srinagar, Jammu & Kashmir | 34.0862 | 74.7984 |
43 | Surat, Gujarat | 21.1857 | 72.837 |
44 | Tiruvananantapuram, Kerala | 8.5069 | 76.9569 |
45 | Udaynarayanpur, Haora, West Bengal | 22.7144 | 87.9761 |
The authors intend to investigate these data using H and D since MASP, MAT, MAWS, and MAP are one-dimensional components.
The H is the slope of trend lines obtained by the plot of log(R/S) and log(n), where log(R/S) is a logarithmic value of a rescaled range analysis and log(n) is the logarithmic of the multiple lengths of time. To find the rescaled range analysis, rescale the time series t, in two powers [32]. Hurst stated that the distance that a random particle covers increases with the square root of time within those shorter time series as follows:
R=√T, |
where R is the distance covered (range) and T is the time index. Next, calculate the mean of the range:
Mean of Sample=ms=1nn∑t=1Xt, |
where s is the series of the sample, n is the size of the range of the sample, and X is the value of an element in the range. Next, find the deviation for each range. The new time series is as follows:
Y=Xt−ms, |
for t=1,2,…,n. Continuing with it, find the series that is the running total of the deviations from the mean:
yt=t∑i=1Yi. |
Then, calculate the widest difference in the series of deviation by the following:
Rt=max(Y1,Y2,…,Yt)−min(Y1,Y2,…,Yt), |
for t=1,2,…,n. Later, find the standard deviation for each range:
σ=√[1tt∑i=1(Xi−ms)2]. |
Calculate the rescaled range for each range in the time series:
R/S=Rtσt, |
for t=1,2,…,n., where R/S is the rescaled range for each range in the time series, R is the range created, and σ is the standard deviation for the range under consideration. Finally, average of the rescaled range values for each region to summarize each range. The rescaled range of the time series is obtained. Then, calculate H,
(R/S)=(c.n)H, |
where (R/S) denotes the rescaled range, n denotes the duration of individual short time series, c is the constant, and H denotes the Hurst exponent. Take logarithms on both sides of the above equation, which becomes the following:
log(R/S)n=logc+H.logn. |
Replace the log values as follows:
Y=log(R/S)n, X=log(n), C=logc. |
Then it gives the equation of a straight line with slope and y-intercept as follows:
Y=HX+C. |
From this equation, it is clear that H is a slope of the trend line equation.
The fractal dimension D value is obtained by substituting H value in Eq (2.2). The H values lie between 0<H<1, and the corresponding fractal dimension values lie between 1<D<2. A time series with a long-term positive autocorrelation is indicated if the fractal dimension D values lie between 1<D<1.5, which means that a high value follows another high value and a low value follows another low value. The fractal dimension D value suggests a time series with long-term flipping between high and low values in adjacent pairs if it falls between 1.5<D<2. This means that a single high value will likely be followed by a low value, and the value that follows will likely also tend to be high, with this tendency to switch between high and low values continuing for a very long time. Moreover, it is a random walk and cannot be accurately predicted if the fractal dimension D value is 1.5. Therefore, the time series' irregularity can be determined from the fractal dimension value, which aids in the short-term prediction [15].
For this research work, coastal regions (Balasore, Bhubaneswar, Chennai, Cuddalore, Kolkata, Mumbai, Surat, Thiruvananthapuram), hill regions (Agumbe, Bilaspur, Chattisgarh, Cherrapunji, Gairsain, Kodaikanal, Kohima, Lachen, Ladakh, Mawsynram, Munnar, Ooty, Shimla, Srinagar), Deserts (Jaisalmer, Sriganganagar), Islands (Kavaratti, Port Blair), Plateaus (Bengaluru, Bhopal, Mahabaleshwar, Ranchi, Nagpur), Plains (Amaravathi, Agartala, Banda, Brahmapuri, Chittoor, Delhi, Dispur, Gandhinagar, Kottayam, Ludhiana, Patna, Ramagundam, Udayanarayanpur), and Relief (Imphal) in India were selected under different terrains, and the following results were obtained from this research. Since the graphs of many locations are similar, the few location graphs are exhibited here as a sample. In the following Figures 2, 4, 6, and 8, the abscissas are the years from 1980 to 2021, and the ordinates are the respective parameters (MASP, MAT, MAWS, MAP) data. In the Figures 3, 5, 7, and 9, the abscissas are the logarithmic time spans of 3, 5, 10, and 20 years, and the ordinates are the logarithmic values of the corresponding average rescaleed range analyses.
Figure 2, shows the graph of MASP in kPa (Pascals). Its ordinate is the MASP data for the locations of (a) Agumbe, (b) Amaravathi, (c) Chennai, (d) Kottayam, (e) Mawsynram, (f) Mumbai, (g) Patna, (h) Ramagundam, and (i) Shimla. Even though the data graphs of Agumbe, Chennai, Kottayam, and Mumbai are similar, their extreme MASP values are different. The highest average of MASP is recorded in Kavaratti and the lowest average of MASP is in Ladakh. The data curve shows the complexity of the irregularity of the surface pressure. Figure 3, shows the graphs of logarithmic time span versus logarithms of the average MASP rescaled range analysis, for the locations mentioned above. From these nine samples, Agumbe, Kottayam, and Mumbai are similar, Amaravathi, Chennai, and Shimla are similar, and rest of the other three are similar for visualization, though their corresponding fractal dimension values are different from 1.0+ to 1.3+.
From Table 2, all the location's MASP fractal dimension values lie between 1<D<1.5. This means that it indicates a time series with a long-term positive autocorrelation. Except for Agumbe, Kottayam, and Mumbai, the other graphs of the logarithmic averages of the rescaling range analyses lie on or above the trend line; therefore, in the coming short-term years, the same MASP data or more is to be predicted. For Agumbe, Kottayam, and Mumbai, the graph line is comes down to the trendline, which shows that, in the next few years, the same MASP data or less than it (most probably reduced to one or two units) is to be predicted.
SI. No. | Location name | MASP | MAT | MAWS | MAP | |||||||
H | D | H | D | H | D | H | D | |||||
1 | Agartala | 0.7508 | 1.2492 | 0.8647 | 1.1353 | 0.9143 | 1.0857 | 0.8833 | 1.1167 | |||
2 | Agumbe | 0.6840 | 1.3160 | 0.8660 | 1.1340 | 0.7992 | 1.2008 | 0.8264 | 1.1736 | |||
3 | Amaravathi | 0.8679 | 1.1321 | 0.8516 | 1.1484 | 0.8732 | 1.1268 | 0.7998 | 1.2002 | |||
4 | Balasore | 0.8632 | 1.1368 | 0.7707 | 1.2293 | 0.8285 | 1.1715 | 0.8531 | 1.1469 | |||
5 | Bānda | 0.8109 | 1.1891 | 0.8127 | 1.1873 | 0.8401 | 1.1599 | 0.6169 | 1.3831 | |||
6 | Bengaluru | 0.7683 | 1.2317 | 0.9077 | 1.0923 | 0.8147 | 1.1853 | 0.8755 | 1.1245 | |||
7 | Bhopal | 0.7888 | 1.2112 | 0.8258 | 1.1742 | 0.8571 | 1.1429 | 0.5916 | 1.4084 | |||
8 | Bhubaneswar | 0.8963 | 1.1037 | 0.8217 | 1.1783 | 0.8229 | 1.1771 | 0.7118 | 1.2882 | |||
9 | Bilaspur | 0.8363 | 1.1637 | 0.7410 | 1.2590 | 0.7093 | 1.2907 | 0.7061 | 1.2939 | |||
10 | Bramhapuri | 0.8781 | 1.1219 | 0.7303 | 1.2697 | 0.7442 | 1.2558 | 0.6208 | 1.3792 | |||
11 | Chandigarh | 0.8521 | 1.1479 | 0.7818 | 1.2182 | 0.8659 | 1.1341 | 0.8657 | 1.1343 | |||
12 | Chennai | 0.7820 | 1.2180 | 0.7463 | 1.2537 | 0.7816 | 1.2184 | 0.7029 | 1.2971 | |||
13 | Cherrapunji | 0.8718 | 1.1282 | 0.8189 | 1.1811 | 0.9034 | 1.0966 | 0.9629 | 1.0371 | |||
14 | Chittoor | 0.7532 | 1.2468 | 0.7729 | 1.2271 | 0.7144 | 1.2856 | 0.7361 | 1.2639 | |||
15 | Cuddalore | 0.7437 | 1.2563 | 0.7931 | 1.2069 | 0.7823 | 1.2177 | 0.6662 | 1.3338 | |||
16 | Delhi | 0.8393 | 1.1607 | 0.7178 | 1.2822 | 0.8543 | 1.1457 | 0.6853 | 1.3147 | |||
17 | Dispur | 0.9022 | 1.0978 | 0.8408 | 1.1592 | 0.9648 | 1.0352 | 0.7955 | 1.2045 | |||
18 | Gairsain | 0.8999 | 1.1001 | 0.7348 | 1.2652 | 0.9194 | 1.0806 | 0.8868 | 1.1132 | |||
19 | Gandhinagar | 0.8946 | 1.1054 | 0.7378 | 1.2622 | 0.7000 | 1.3000 | 0.6842 | 1.3158 | |||
20 | Imphal | 0.8082 | 1.1918 | 0.8252 | 1.1748 | 0.8442 | 1.1558 | 0.8811 | 1.1189 | |||
21 | Jaisalmer | 0.8514 | 1.1486 | 0.8317 | 1.1683 | 0.8940 | 1.1060 | 0.7748 | 1.2252 | |||
22 | Kavaratti | 0.7065 | 1.2935 | 0.8879 | 1.1121 | 0.7792 | 1.2208 | 0.8319 | 1.1681 | |||
23 | Kodaikanal | 0.8106 | 1.1894 | 0.8287 | 1.1713 | 0.7363 | 1.2637 | 0.9094 | 1.0906 | |||
24 | Kohima | 0.8150 | 1.1850 | 0.8645 | 1.1355 | 0.7909 | 1.2091 | 0.9682 | 1.0318 | |||
25 | Kolkata | 0.7845 | 1.2155 | 0.8068 | 1.1932 | 0.8645 | 1.1355 | 0.7256 | 1.2744 | |||
26 | Kottayam | 0.6740 | 1.3260 | 0.8669 | 1.1331 | 0.7032 | 1.2968 | 0.8661 | 1.1339 | |||
27 | Lachen | 0.8426 | 1.1574 | 0.7908 | 1.2092 | 0.6325 | 1.3675 | 0.9694 | 1.0306 | |||
28 | Ladākh | 0.7650 | 1.2350 | 0.8673 | 1.1327 | 0.8326 | 1.1674 | 0.9341 | 1.0659 | |||
29 | Ludiana | 0.8786 | 1.1214 | 0.8463 | 1.1537 | 0.8199 | 1.1801 | 0.7934 | 1.2066 | |||
30 | Mahabaleshwar | 0.8920 | 1.1080 | 0.7386 | 1.2614 | 0.8454 | 1.1546 | 0.8702 | 1.1298 | |||
31 | Mawsynram | 0.8718 | 1.1282 | 0.8189 | 1.1811 | 0.9034 | 1.0966 | 0.9629 | 1.0371 | |||
32 | Mumbai | 0.7913 | 1.2087 | 0.7322 | 1.2678 | 0.8330 | 1.1670 | 0.7639 | 1.2361 | |||
33 | Munnar | 0.6839 | 1.3161 | 0.7814 | 1.2186 | 0.8236 | 1.1764 | 0.8812 | 1.1188 | |||
34 | Nagpur | 0.8041 | 1.1959 | 0.7647 | 1.2353 | 0.6321 | 1.3679 | 0.7150 | 1.2850 | |||
35 | Ooty | 0.7143 | 1.2857 | 0.8671 | 1.1329 | 0.7965 | 1.2035 | 0.8648 | 1.1352 | |||
36 | Patna | 0.6902 | 1.3098 | 0.8012 | 1.1988 | 0.7835 | 1.2165 | 0.7553 | 1.2447 | |||
37 | Port Blair | 0.8430 | 1.1570 | 0.8583 | 1.1417 | 0.9734 | 1.0266 | 0.8785 | 1.1215 | |||
38 | Ramagundam | 0.8486 | 1.1514 | 0.5940 | 1.4060 | 0.8039 | 1.1961 | 0.6113 | 1.3887 | |||
39 | Ranchi | 0.8808 | 1.1192 | 0.7978 | 1.2022 | 0.8412 | 1.1588 | 0.7855 | 1.2145 | |||
40 | Shimla | 0.9106 | 1.0894 | 0.7580 | 1.2420 | 0.9198 | 1.0802 | 0.8870 | 1.1130 | |||
41 | Sriganganagar | 0.8641 | 1.1359 | 0.8426 | 1.1574 | 0.7223 | 1.2777 | 0.7468 | 1.2532 | |||
42 | Srinagar | 0.7839 | 1.2161 | 0.8025 | 1.1975 | 0.7815 | 1.2185 | 0.8985 | 1.1015 | |||
43 | Surat | 0.8113 | 1.1887 | 0.7456 | 1.2544 | 0.7152 | 1.2848 | 0.8962 | 1.1038 | |||
44 | Tiruvananantapuram | 0.6761 | 1.3239 | 0.7976 | 1.2024 | 0.8670 | 1.1330 | 0.7793 | 1.2207 | |||
45 | Udaynarayanpur | 0.7845 | 1.2155 | 0.8068 | 1.1932 | 0.8645 | 1.1355 | 0.7256 | 1.2744 | |||
Mean Average | 0.8104 | 1.1896 | 0.8013 | 1.1987 | 0.8154 | 1.1846 | 0.8060 | 1.1940 |
In the past three years, India's highest temperature was recorded in Sriganganagar, Nagpur, Bilaspur, and Banda. From this research work, it is observed that their average temperature between 26 to 27 ∘C and the location Cuddalore has 28 ∘C. Additionally the lowest temperatures were recorded in Ladakh, Srinagar, and Lachen, which is the same as the research values. From Table 2, the MAT fractal dimension of Bengaluru is 1.0+. Bengaluru has received constant and moderate MAT for the past 41 years. In the rest of the 44 locations, 24 have 1.1+ as the fractal dimension, and 19 have 1.2+ as the fractal dimension. Ramagundam has a fractal dimension of 1.4+. Figure 4, shows the sample graphs of the MAT in unit Celsius, whose Y axis is the MAT data of the locations of (a) Banda, (b) Bengaluru, (c) Bramhapuri, (d) Chennai, (e) Delhi, (f) Ludiana, (g) Ramagundam, (h) Sriganganagar, and (i) Srinagar. All the graphs of Figure 4 are different. In India, the maximum temperature recorded from the selected location was 28.10 in Cuddalore, and the minimum temperature value was −3.13 in Ladakh.
Figure 5, shows the graphs of its log-log plot of time span versus the average MAT rescaled range, whose Y- axis is the logarithms of the average of the rescaled range analysis of the MAT for the locations mentioned above. From the diagram, it is observed that the fractal dimensions of Ramagundam and Sriganganagar are the same, and its extreme temperature values are also similar (more or less), though their curves are not the same. From the nine sample graphs, it is observed that some curves are pass above the trend line and some curves are below. From Table 2, all the fractal dimension values lie between 1<D<1.5. The lowest fractal dimension value of 1.0+ is obtained for Bengaluru, which means that it has a constant temperature for a long period of time. Therefore, the short-term prediction of MAT for Bengaluru will continue at the same temperature as in previous years. It may vary slightly by one or two units. The highest fractal dimension value of 1.4+ was obtained for Ramagundam, which means that it is closer to a random walk. Therefore, the prediction of MAT for Ramagundam is a little bit difficult task. Moreover, the MAT data for Ramagundam switched over from 26.83 to 28.23 for the past 41 years, so one should expect the same for the next few years, though it may have a chance to increase.
Figure 6, shows the graph of the MAWS data in units of m/s, whose ordinate is the MAWS data of the locations of (a) Agartala, (b) Chennai, (c) Gandhinagar, (d) Kottayam, (e) Lachen, (f) Mawsynram, (g) Nagpur, (h) Port Blair, and (i) Ramagundam. Figure 6, has different curves in all samples of the nine graphs, and their extreme wind speed values have also been changing for each graph. The maximum and minimum values of the MAWS from the selected locations were 5.86 in the island of Port Blair, and 0.95 in the hill station, Kohima. The maximum and minimum values of MAWS in Port Blair were 5.86 and 4.65, respectively. Since it is an island, the authors would like to compare it with another Island, Kavaratti, at 5.38 and 3.52, respectively which also have a high values. Therefore, it is observed that Islands are getting more MAWS. After seeing this, it is compared with all the other selected locations, not only the island, but also the Cuddalore coastal area, which also received high MAWS. Its maximum and minimum values were 5.28 and 4.59, respectively. The curves of the MAWS shows the irregularities and complexities which implies that, it is a fractal structure.
Figure 7 shows the graphs of their log-log plot of time span versus average MAWS rescaled range values for the locations mentioned above. From these graphs, the authors observed that Agartala, Mawsynram, and Port Blair have fractal dimensions of 1.0+. Even though the curve of the graphs deviated from the trend line, when it is merged with the trend line, it shows smooth and constant MAWS values for the past few years. The prediction for the next few years is easy, and as in previous years, it will continue with slight variation. Ramagundam has a fractal dimension of 1.1+, Chennai and Kottayam have fractal dimensions of 1.2+, and from their graphs, it is observed that they do not have much more deviations. Therefore, the prediction for the next few years is not difficult. It may be the same as the previous years, or a slight change may occur. Gandhinagar, Lachen, and Nagpur have a fractal dimension of 1.3+. From the graphs of these three locations, a deviation from the trend line is observed. Therefore, the prediction for these locations may not be accurate. Overall, from the selected locations, the maximum fractal dimension values obtained for Lachen and Nagpur are 1.3+, and the minimum fractal dimension value is 1.0+ in the locations of Agartala, Cherrapunji, Dispur, Gairsain, Mawsynram, Port Blair, and Shimla. In areas, whose fractal dimension is 1.0+, the future prediction is easy, which should be similar to previous years. In the locations, whose fractal dimension is more than 1.3+, the future prediction is difficult, and there is a greater chance to deviate from the predicted value. The 45 locations, H and D values of MAWS are displayed in the Table 2.
Figure 8, shows the graphs of the MAP data in mm/day for the locations of (a) Agumbe, (b) Balasore, (c) Chennai, (d) Jaisalmer, (e) Kavaratti, (f) Kottayam, (g) Ladakh, (h) Mawsynram, and (i) Port Blair, whose Y-axis is the MAP in units of mm/day. The maximum and minimum levels of precipitation at the selected locations were 14.65 mm/day and 0.03 mm/day, respectively. The maximum level of precipitation occurred as rainfall in the hill regions of Cherrapunji, and Mawsynram, which are villages in the state of Meghalaya, and receive the world's highest average annual precipitation. From the average of MAP, the maximum average value occurred at 9.01 mm/day in Agumbe, followed by 7.08 mm/day in Kottayam, then in Cherrapunji, Mawsynram, Mumbai, and Port Blair at 6.2 mm/day. Even though Agumbe, Kottayam, Mumbai, and Port Blair have higher average rainfall, they receive monsoon rains, while Cherrapunji and Mawsynram receive rainfall all over the year.
The greatest and lowest MAP values recorded for Cherrapunji and Mawsynram were 14.65 and 4.16 mm/day, respectively. Moreover, for Agumbe, the maximum and minimum values were 12.6 and 6.06 mm/day, respectively. Agartala's maximum and minimum MAP values reached were 13.19 and 2.99, respectively. However, its average MAP value was 5.26. Additionally, the MAP curve has more irregularities, which shows the fractal structure.
Figure 9, represents the graph of the log-log plot of the time span and the MAP logarithmic value of the average of the rescaled range analysis, whose ordinates are the logarithmic value of the average of the rescaled range analysis.
From Table 2, the fractal dimension of the MAP of the locations Cherrapunji, Kodaikanal, Kohima, Lachen, Ladakh, and Mawsynram is 1.0+. These locations have received constant annual rainfall for the past 41 years, and it is noticeable that these areas are hilly regions. The fractal dimension of the locations Agartala, Agumbe, Balasore, Bengaluru, Chandigarh, Chennai, Gairsain, Imphal, Kavaratti, Kottayam, Mahabaleshwar, Munnar, Ooty, Port Blair, Shimla, Srinagar, and Surat is nearly 1.1+. Additionally for the locations Amaravathi, Bhubaneshwar, Bilaspur, Dispur, Jaisalmer, Kolkata, Ludhiana, Mumbai, Nagpur, Patna, Ranchi, Sriganganagar, Thiruvananthapuram, and Udayanarayanpur, the fractal dimension is 1.2+. The locations Banda, Bramhapuri, Cuddalore, Delhi, Gandhinagar, and Ramagundam have a fractal dimension of 1.3+, and Bhopal has a fractal dimension of 1.4+. From the past 41 years, although Mawsynram and Ladakh have similar fractal dimensions of 1.0+, data graphs show that their extreme precipitation levels differ. That is, the maximum MAP level of Mawsynram was 16 mm/day and the minimum was 4 mm/day; however, for Ladakh, the maximum MAP was 1.4 mm/day and the minimum was 0.2 mm/day. Thus, the fractal dimension intimates that these places have similar persistent rainfall for the past few years. From the graphs of Figure 9, it is observed that Agumbe, Balasore, Kavarati, and Port Blair have slight deviations from the trend line whose fractal dimensions are 1.1+. The prediction for these locations is possible, and it should be as it was in previous years. The locations, Ladakh, Cherrapunji, and Mawsynram, have a fractal dimension of 1.0+, which leads to constant precipitation as in previous years. Bhopal has a fractal dimension of 1.4+, which means that the prediction is difficult and may have a deviation from the predicted value. Therefore, the precipitation is higher in hilly regions such as Agumbe, Cherrapunji, Mawsynram, and Kottayam. However, one of the highest peaks in the world, Ladakh, receives hardly any attention. The average MAP of the place is 0.5 only. The hill regions are not the only ones to receive more rainfall, but also the islands of Port Blair and Mumbai's coastal area too. After that, the authors wish to compare it with the deserts Jaisalmer and Sriganganagar, which receive 0.5 and 0.8, respectively, and both have fractal dimensions of 1.2+. From the fractal value, it is observed that for the past 41 years, these two places are received low-level precipitation only.
Table 2 clearly shows that MASP, MAT, MAWS, MAP are fractals. From the research work, it is observed that the average fractal dimension values of all parameters lie between 1<D<1.2. For the individual locations, the fractal dimension values lie between 1<D<1.5. The fractal dimension value of the MASP of Agumbe, Munnar, and Patna is 1.3, the fractal dimension value of the MAT in Ramagundam is 1.4, the fractal dimension value of the MAWS in Lachen, Munnar, is 1.3, and the fractal dimension value of the MAP in Banda, Bhopal, Bramhapuri, Cuddalore, Delhi, Gandhinagar, and Ramagundam is 1.3. A fractal dimension value nearer 1.5 indicates a rough and rugged one, where prediction is challenging; alternatively a value nearer 1 indicates a smooth one, where the prediction provides accuracy.
For the investigation of the interrelationship, twelve places were chosen from the selected locations on the basis of their fractal dimension of 1.0+, which means the parameter values are more or less constant throughout the 41 years. For this purpose, the authors find the average for the parameter data, and from the average values, they performed work. Additionally, two locations, Agumbe and Cuddalore, were selected on the basis of the maximum and minimum values of the average value of the parameters. Moreover, the location of Kavaratti is included for comparison with another island, Port Blair. A total of fifteen places were selected for this research work. For this work, the average values of the parameters are categorized as follows. For the average of the MASP (AMASP) values, 101−95 kPa is a high or greater (G) level, 94−90 kPa is moderate (M) level, and 89 kPa and below are low (L) levels. For the average of the MAT (AMAT) values, 28−24 ∘C is a high (G) level, 23−18 ∘C is a moderate (M) level, and 17 ∘C and below are low (L) levels.
The average of the MAWS (AMAWS) values, 4-5 m/s is a high (G) level, 3 m/s is a moderate (M) level, and 2 m/s and below are low (L) levels. Additionally for the average MAP (AMAP) values, 5-6 mm/day is a high (G) level, 3-4 mm/day is moderate (M), and 2 mm/day and below are low (L) levels. The average values of parameters are in Table 3, and the categorized values are in Table 4. Table 4 shows that when the AMASP is high, the AMAT is also high; in some cases, it is moderate, but not low. When the AMASP is low, the AMAP is high in Cherrapunji and Mawsynram only, but not in the locations of Gairsain, Kohima, Lachen, and Ladakh. While working deeply about these places, the terrain is too high from the sea level. Therefore, the places that are too high above sea level get less precipitation, and moreover, the precipitation is snowfall. When the AMAT is high, the AMAP is also high, and in a few places, it is moderate. However, in Cuddalore, the AMAT is high, and the AMAP is low. If the AMAT is high and the AMAWS is low, then the AMAP is high in Agartala, Agumbe, and Dispur, while at the same time, the AMSP is also high. Since Kodaikanal is a hill region, the AMASP, and the AMAT are high, though the AMAP is moderate and the AMWS is low. For the rest of the hill regions of Gairsain, Kohima, Lachen, and Ladakh, it is observed that all average parameter values were low except Kohima, whose AMAT and AMAP were moderate.
SI. No. | Locations | AMASP | AMAT | AMAWS | AMAP |
1 | Agartala | 100.49 | 25.44 | 2.75 | 5.26 |
2 | Agumbe | 96.88 | 25.22 | 2.64 | 9.01 |
3 | Bengaluru | 91.66 | 23.63 | 4.14 | 2.30 |
4 | Cherrapunji | 87.59 | 17.79 | 2.49 | 6.88 |
5 | Cuddalore | 100.81 | 28.11 | 4.98 | 2.78 |
6 | Dispur | 96.30 | 22.86 | 1.85 | 5.04 |
7 | Gairsain | 81.27 | 15.16 | 2.38 | 2.87 |
8 | Kavaratti | 101.00 | 27.83 | 4.77 | 4.36 |
9 | Kodaikanal | 93.12 | 23.60 | 2.68 | 3.23 |
10 | Kohima | 86.28 | 18.20 | 1.06 | 3.10 |
11 | Lachen | 69.27 | 7.24 | 2.42 | 2.62 |
12 | Ladākh | 58.35 | -3.13 | 2.26 | 0.57 |
13 | Mawsynram | 87.59 | 17.79 | 2.49 | 6.88 |
14 | Port Blair | 100.88 | 27.93 | 5.39 | 6.54 |
15 | Shimla | 90.95 | 21.10 | 2.84 | 2.67 |
SI. No. | Locations | AMASP | AMAT | AMAWS | AMAP |
1 | Agartala | G | G | L | G |
2 | Agumbe | G | G | L | G |
3 | Bengaluru | G | M | G | L |
4 | Cherrapunji | L | M | L | G |
5 | Cuddalore | G | G | G | L |
6 | Dispur | G | G | L | G |
7 | Gairsain | L | L | L | L |
8 | Kavaratti | G | G | G | G |
9 | Kodaikanal | G | M | L | M |
10 | Kohima | L | M | L | M |
11 | Lachen | L | L | L | L |
12 | Ladākh | L | L | L | L |
13 | Mawsynram | L | M | L | G |
14 | Port Blair | G | G | G | G |
15 | Shimla | G | M | M | L |
Now, they compare with the islands, both Port Blair and Kavaratti, where all the average parameter values were high. Another interrelationship between the AMAWS and the AMAP is observed: when the AMAWS is high, the AMAP is low, except for the islands.
Therefore, it is observed that the precipitation of India is not only based on these four parameters, but also on other parameters such as cloudiness, altitude, oceans, water bodies, mountains, etc. It's important to note that weather is a highly complex system, and the relationships between these parameters are influenced by numerous parameters, including regional geography, proximity to large bodies of water, and the presence of other atmospheric features such as fronts or storms. Meteorologists use sophisticated models and observations to analyze and understand these relationships, thus allowing for more accurate weather predictions.
The fractal dimension characteristics of four parameters time series were studied using the Hurst exponent. A fractal behavior existed in all four parameters and for all locations. From the above results, it was observed that if the fractal dimension values of the parameter was 1.0+, then it was confident that a constant parameter value will followed for a few more years. If the fractal dimension values was between 1.1+ to 1.3+, then they would also follow the values of the previous years with some irregularities. If the fractal dimension value was 1.4+, then it had more irregularities. Therefore, prediction would be difficult for these areas. The linear trend line obtained from the log−log plot of these locations for the four parameters didn't show any significant warning in the past 41 years. The conclusion of this research work reports that the research locations whose fractal dimension lies between 1<D<1.3 will maintain a level of the four parameter values as in the past two years. That is, whether the level of the MASP, MAT, MAWS, and MAP increases, decreases, or remains same, then it is same for the next two years. This research work also found that the precipitation level in India did not depend on the terrain. Due to the changes in these four parameters, the climate also changed. In India, high-temperature places changed to moderate temperatures, and the low-temperature area changed to the high-temperature area. During summer, sudden bursts of heavy rainfall may unexpectedly break through the intense heat. The seasons and monsoons also started earlier. Precipitation in the form of rainfall was higher, while snowfall was comparatively lower. This poses a serious problem because if all the snow melts, water bodies will receive an excess of water. If the oceans cannot accommodate this additional water, it may lead to flooding in low-lying areas. Additionally, it was observed that the Islands had high values for all parameters. It shows the danger sign of Islands submerging under the sea water. Meanwhile very high hill regions in the northern parts of India, far away from the sea, have low values for all parameters. Therefore, the authors conclude that the weather condition is complex and that these four parameters are not enough to identify it. In future research studies, the authors will include more parameters for the keen investigation using a multifractal analysis.
The authors declare they have not used Artificial Intelligence (AI) tools in the creation of this article.
The second author acknowledges the Vellore Institute of Technology (VIT) for providing "VIT–International Research Fund Scheme (VIN)–Sanctioned Order No.: VIN/2023-24/01/Date: 18 December 2023" for carrying out this research work.
All authors declare that they have no conflicts of interest.
[1] |
L. Schipper, M. Pelling, Disaster risk, climate change and international development: scope for, and challenges to, integration, Disasters, 30 (2006), 19–38. https://doi.org/10.1111/j.1467-9523.2006.00304.x doi: 10.1111/j.1467-9523.2006.00304.x
![]() |
[2] |
A. Gowrisankar, T. M. C. Priyanka, A. Saha, L. Rondoni, K. Hassan, S. Banerjee, Greenhouse gas emissions: a rapid submerge of the world, Chaos, 6 (2022), 1–32. https://doi.org/10.1063/5.0091843 doi: 10.1063/5.0091843
![]() |
[3] |
E. Ostrom, Polycentric systems for coping with collective action and global environmental change, Global Just., 20 (2017), 423–430. https://doi:10.1016/j.gloenvcha.2010.07.004 doi: 10.1016/j.gloenvcha.2010.07.004
![]() |
[4] |
V. P. Tewari, R. K.Verma, K. Von Gadow, Climate change effects in the Western Himalayan ecosystems of India: evidence and strategies, Forest Ecosyst., 4 (2017), 1–9. https://doi.org/10.1186/s40663-017-0100-4 doi: 10.1186/s40663-017-0100-4
![]() |
[5] |
B. Chapman, K. Rosemond, Seasonal climate summary for the southern hemisphere (autumn 2018): a weak La Nina fades, the austral autumn remains warmer and drier, J. South. Hemisphere Earth Syst. Sci., 70 (2020), 328–352. https://doi.org/10.1071/ES19039 doi: 10.1071/ES19039
![]() |
[6] |
A. M. Makarieva, V. G. Gorshkov, D. Sheil, A. D. Nobre, B. L. Li, Where do winds come from? A new theory on how water vapor condensation influences atmospheric pressure and dynamics, Atmos. Chem. Phys., 13 (2013), 1039–1056. https://doi.org/10.5194/acp-13-1039-2013 doi: 10.5194/acp-13-1039-2013
![]() |
[7] |
J. H. Van Hateren, A fractal climate response function can simulate global average temperature trends of the modern era and the past millennium, Clim. Dyn., 40 (2013), 2651–2670. https://doi.org/10.1007/s00382-012-1375-3 doi: 10.1007/s00382-012-1375-3
![]() |
[8] | S. Eichelberger, J. McCaa, B. Nijssen, A. Wood, Climate change effects on wind speed, North Amer. Windpower, 7 (2008), 68–72. |
[9] |
K. E. Trenberth, Changes in precipitation with climate change, Climate Res., 47 (2011) 123–138. https://doi.org/10.3354/cr00953 doi: 10.3354/cr00953
![]() |
[10] |
B. B. Mandelbrot, J. R. Wallis, Some long‐run properties of geophysical records, Water Resour. Res., 5 (1969), 321–340. https://doi.org/10.1029/WR005i002p00321 doi: 10.1029/WR005i002p00321
![]() |
[11] |
L. Bodri, Fractal analysis of climatic data: Mean annual temperature records in Hungary, Theor. Appl. Climatology, 49 (1994), 53–57. https://doi.org/10.1007/BF00866288 doi: 10.1007/BF00866288
![]() |
[12] |
R. Govindan, D. A. Sant, Fractal dimensional analysis of Indian climatic dynamics, Chaos Solitons Fract., 19 (2004), 285–291. https://doi.org/10.1016/S0960-0779(03)00042-0 doi: 10.1016/S0960-0779(03)00042-0
![]() |
[13] |
N. C. Sahu, D. Mishra, Analysis of perception and adaptability strategies of the farmers to climate change in Odisha, India, APCBEE Proc., 5 (2013), 123–127. https://doi.org/10.1016/j.apcbee.2013.05.022 doi: 10.1016/j.apcbee.2013.05.022
![]() |
[14] |
S. Rehman, A. H. Siddiqi, Wavelet based Hurst exponent and fractal dimensional analysis of Saudi climatic dynamics, Chaos Solitons Fract., 40 (2009), 1081–1090. https://doi.org/10.1016/j.chaos.2007.08.063 doi: 10.1016/j.chaos.2007.08.063
![]() |
[15] |
B. Cui, P. Huang, W. Xie, Fractal dimension characteristics of wind speed time series under typhoon climate, J. Wind Eng. Ind. Aerodyn., 229 (2022), 105144. https://doi.org/10.1016/j.jweia.2022.105144 doi: 10.1016/j.jweia.2022.105144
![]() |
[16] |
M. Li, Fractal time series–a tutorial review, Math. Probl. Eng., 2010 (2010), 157264. https://doi.org/10.1155/2010/157264 doi: 10.1155/2010/157264
![]() |
[17] | D. W. Stroock, Probability theory: an analytic view, Cambridge University Press, 2010. |
[18] | J. Feder, Fractals, Springer Science & Business Media, 2013. https://doi.org/10.1007/978-1-4899-2124-6 |
[19] | S. Banerjee, M. K. Hassan, S. Mukherjee, A. Gowrisankar, Fractal patterns in nonlinear dynamics and applications, CRC Press, 2020. |
[20] |
H. E. Hurst, Long-term storage capacity of reservoirs, Trans. Amer. Soc. Civ. Eng., 116 (1951), 770–799. https://doi.org/10.1061/TACEAT.0006518 doi: 10.1061/TACEAT.0006518
![]() |
[21] | B. Qian, K. Rasheed, Hurst exponent and financial market predictability IASTED Conference on Financial Engineering and Applications, 2004,203–209. |
[22] |
A. Akhrif, M. Romanos, K. Domschke, A. Schmitt-Boehrer, S. Neufang, Fractal analysis of BOLD time series in a network associated with waiting impulsivity, Front. Physiol., 9 (2018), 1378. https://doi.org/10.3389/fphys.2018.01378 doi: 10.3389/fphys.2018.01378
![]() |
[23] |
M. F. Barnsley, S. Demko, Iterated function systems and the global construction of fractals, Proc. R. Soc. London. A, 399 (1985), 243–275. https://doi.org/10.1098/rspa.1985.0057 doi: 10.1098/rspa.1985.0057
![]() |
[24] | M. F. Barnsley, Fractals everywhere, Academic Press, 2014. https://doi.org/10.1016/c2013-0-10335-2 |
[25] | S. Banerjee, D. Easwaramoorthy, A. Gowrisankar, Fractal functions, dimensions and signal analysis, Springer, 2021. https://doi.org/10.1007/978-3-030-62672-3 |
[26] |
B. B. Mandelbrot, Self-affine fractals and fractal dimension, Phys. Scr., 32 (1985), 257. https://doi.org/ 10.1088/0031-8949/32/4/001 doi: 10.1088/0031-8949/32/4/001
![]() |
[27] |
C. Thangaraj, D. Easwaramoorthy, Generalized fractal dimensions based comparison analysis of edge detection methods in CT images for estimating the infection of COVID-19 disease, Eur. Phys. J. Special Top., 231 (2022), 3717–3739. https://doi.org/10.1140/epjs/s11734-022-00651-1 doi: 10.1140/epjs/s11734-022-00651-1
![]() |
[28] | Y. Kohavi, H. Davdovich, Topological dimensions, Hausdorff dimensions & fractals, Bar-llan University, 2006. |
[29] |
I. Pilgrim, R. P. Taylor, Fractal analysis of time-series data sets: methods and challenges, Fractal Anal., 20 (2018), 5–30. https://doi.org/10.5772/intechopen.81958 doi: 10.5772/intechopen.81958
![]() |
[30] |
J. B. Bassingthwaighte, G. M. Raymond, Evaluating rescaled range analysis for time series, Ann. Biomed. Eng., 22, (1994), 432–444. https://doi.org/10.1007/BF02368250 doi: 10.1007/BF02368250
![]() |
[31] |
S. Lovejoy, B. B. Mandelbrot, Fractal properties of rain, and a fractal model, Tellus A, 37 (1985), 209–232. https://doi.org/10.1111/j.1600-0870.1985.tb00423.x doi: 10.1111/j.1600-0870.1985.tb00423.x
![]() |
[32] |
H. Tatli, Detecting persistence of meteorological drought via the Hurst exponent, Meteorol. Appl., 22 (2015), 763–769. https://doi.org/10.1002/met.1519 doi: 10.1002/met.1519
![]() |
SI. No. | Location name | Latitude | Longitude |
1 | Agartala, West Tripura, Tripura | 23.8296 | 91.2901 |
2 | Agumbe, Kundapura, Udupi, Karnataka | 13.508 | 75.0946 |
3 | Amaravathi, Guntur, Andhra Pradesh | 16.5703 | 80.3606 |
4 | Balasore, Odisha | 21.5053 | 86.9159 |
5 | Bānda, Uttar Pradesh | 25.4754 | 80.3356 |
6 | Bengaluru, Karnataka | 12.9703 | 77.5855 |
7 | Bhopal, Madhya Pradesh | 23.2644 | 77.4018 |
8 | Bhubaneswar, Odhisa | 20.2698 | 85.841 |
9 | Bilaspur, Chhattisgarh | 22.0787 | 82.1403 |
10 | Bramhapuri, Mahārāshtra | 20.6 | 79.8666 |
11 | Chandigarh, Harayana | 30.7306 | 76.8478 |
12 | Chennai, Tamil Nadu | 13.0549 | 80.2311 |
13 | Cherrapunji, East Khasi Hills, Meghalaya | 25.2851 | 91.7184 |
14 | Chittoor, Andhra Pradesh | 13.2067 | 79.0883 |
15 | Cuddalore, Tamil Nadu | 11.7462 | 79.7586 |
16 | Delhi, New Delhi, Delhi | 28.6342 | 77.2168 |
17 | Dispur, Kamrup Metropolitan, Assam | 26.136 | 91.8081 |
18 | Gairsain, Chamoli, Uttarakhand | 30.0554 | 79.2904 |
19 | Gandhinagar, Gujarat | 23.2413 | 72.638 |
20 | Imphal, Imphal West, Manipur | 24.8077 | 93.9273 |
21 | Jaisalmer, Rajasthan | 26.9194 | 70.9146 |
22 | Kavaratti Sub-Division, Lakshadweep | 10.5644 | 72.639 |
23 | Kodaikanal, Dindigul, Tamil Nadu | 10.2312 | 77.4882 |
24 | Kohima, Nagaland | 25.6631 | 94.0933 |
25 | Kolkata, West Bengal | 22.5708 | 88.372 |
26 | Kottayam, Kerala | 9.5947 | 76.5328 |
27 | Lachen, Northen sikkim | 27.7456 | 88.5014 |
28 | Ladākh, Jammu and Kashmir | 34.337 | 77.4057 |
29 | Ludiana, Punjab | 30.9063 | 75.8489 |
30 | Mahabaleshwar, Satara, Maharashtra | 17.9262 | 73.6593 |
31 | Mawsynram, East Khasi Hills, Meghalaya | 25.2996 | 91.5828 |
32 | Mumbai, Maharashtra | 18.9373 | 72.8349 |
33 | Munnar, Devikulam, Idukki, Kerala | 10.0742 | 77.0625 |
34 | Nagpur, Maharashtra | 21.1563 | 79.0821 |
35 | Ooty, The Nilgiris, Tamil Nadu | 11.4059 | 76.6991 |
36 | Patna, Bihar | 25.6015 | 85.1372 |
37 | Port Blair, Andaman & Nicobar Islands | 11.6629 | 92.7391 |
38 | Ramagundam, Peddapalle, Telangana | 18.7565 | 79.4843 |
39 | Ranchi, Jharkhand | 23.3515 | 85.3127 |
40 | Shimla, Himachal Pradesh | 31.1033 | 77.1581 |
41 | Sriganganagar, Rajasthan | 29.912 | 73.8756 |
42 | Srinagar, Jammu & Kashmir | 34.0862 | 74.7984 |
43 | Surat, Gujarat | 21.1857 | 72.837 |
44 | Tiruvananantapuram, Kerala | 8.5069 | 76.9569 |
45 | Udaynarayanpur, Haora, West Bengal | 22.7144 | 87.9761 |
SI. No. | Location name | MASP | MAT | MAWS | MAP | |||||||
H | D | H | D | H | D | H | D | |||||
1 | Agartala | 0.7508 | 1.2492 | 0.8647 | 1.1353 | 0.9143 | 1.0857 | 0.8833 | 1.1167 | |||
2 | Agumbe | 0.6840 | 1.3160 | 0.8660 | 1.1340 | 0.7992 | 1.2008 | 0.8264 | 1.1736 | |||
3 | Amaravathi | 0.8679 | 1.1321 | 0.8516 | 1.1484 | 0.8732 | 1.1268 | 0.7998 | 1.2002 | |||
4 | Balasore | 0.8632 | 1.1368 | 0.7707 | 1.2293 | 0.8285 | 1.1715 | 0.8531 | 1.1469 | |||
5 | Bānda | 0.8109 | 1.1891 | 0.8127 | 1.1873 | 0.8401 | 1.1599 | 0.6169 | 1.3831 | |||
6 | Bengaluru | 0.7683 | 1.2317 | 0.9077 | 1.0923 | 0.8147 | 1.1853 | 0.8755 | 1.1245 | |||
7 | Bhopal | 0.7888 | 1.2112 | 0.8258 | 1.1742 | 0.8571 | 1.1429 | 0.5916 | 1.4084 | |||
8 | Bhubaneswar | 0.8963 | 1.1037 | 0.8217 | 1.1783 | 0.8229 | 1.1771 | 0.7118 | 1.2882 | |||
9 | Bilaspur | 0.8363 | 1.1637 | 0.7410 | 1.2590 | 0.7093 | 1.2907 | 0.7061 | 1.2939 | |||
10 | Bramhapuri | 0.8781 | 1.1219 | 0.7303 | 1.2697 | 0.7442 | 1.2558 | 0.6208 | 1.3792 | |||
11 | Chandigarh | 0.8521 | 1.1479 | 0.7818 | 1.2182 | 0.8659 | 1.1341 | 0.8657 | 1.1343 | |||
12 | Chennai | 0.7820 | 1.2180 | 0.7463 | 1.2537 | 0.7816 | 1.2184 | 0.7029 | 1.2971 | |||
13 | Cherrapunji | 0.8718 | 1.1282 | 0.8189 | 1.1811 | 0.9034 | 1.0966 | 0.9629 | 1.0371 | |||
14 | Chittoor | 0.7532 | 1.2468 | 0.7729 | 1.2271 | 0.7144 | 1.2856 | 0.7361 | 1.2639 | |||
15 | Cuddalore | 0.7437 | 1.2563 | 0.7931 | 1.2069 | 0.7823 | 1.2177 | 0.6662 | 1.3338 | |||
16 | Delhi | 0.8393 | 1.1607 | 0.7178 | 1.2822 | 0.8543 | 1.1457 | 0.6853 | 1.3147 | |||
17 | Dispur | 0.9022 | 1.0978 | 0.8408 | 1.1592 | 0.9648 | 1.0352 | 0.7955 | 1.2045 | |||
18 | Gairsain | 0.8999 | 1.1001 | 0.7348 | 1.2652 | 0.9194 | 1.0806 | 0.8868 | 1.1132 | |||
19 | Gandhinagar | 0.8946 | 1.1054 | 0.7378 | 1.2622 | 0.7000 | 1.3000 | 0.6842 | 1.3158 | |||
20 | Imphal | 0.8082 | 1.1918 | 0.8252 | 1.1748 | 0.8442 | 1.1558 | 0.8811 | 1.1189 | |||
21 | Jaisalmer | 0.8514 | 1.1486 | 0.8317 | 1.1683 | 0.8940 | 1.1060 | 0.7748 | 1.2252 | |||
22 | Kavaratti | 0.7065 | 1.2935 | 0.8879 | 1.1121 | 0.7792 | 1.2208 | 0.8319 | 1.1681 | |||
23 | Kodaikanal | 0.8106 | 1.1894 | 0.8287 | 1.1713 | 0.7363 | 1.2637 | 0.9094 | 1.0906 | |||
24 | Kohima | 0.8150 | 1.1850 | 0.8645 | 1.1355 | 0.7909 | 1.2091 | 0.9682 | 1.0318 | |||
25 | Kolkata | 0.7845 | 1.2155 | 0.8068 | 1.1932 | 0.8645 | 1.1355 | 0.7256 | 1.2744 | |||
26 | Kottayam | 0.6740 | 1.3260 | 0.8669 | 1.1331 | 0.7032 | 1.2968 | 0.8661 | 1.1339 | |||
27 | Lachen | 0.8426 | 1.1574 | 0.7908 | 1.2092 | 0.6325 | 1.3675 | 0.9694 | 1.0306 | |||
28 | Ladākh | 0.7650 | 1.2350 | 0.8673 | 1.1327 | 0.8326 | 1.1674 | 0.9341 | 1.0659 | |||
29 | Ludiana | 0.8786 | 1.1214 | 0.8463 | 1.1537 | 0.8199 | 1.1801 | 0.7934 | 1.2066 | |||
30 | Mahabaleshwar | 0.8920 | 1.1080 | 0.7386 | 1.2614 | 0.8454 | 1.1546 | 0.8702 | 1.1298 | |||
31 | Mawsynram | 0.8718 | 1.1282 | 0.8189 | 1.1811 | 0.9034 | 1.0966 | 0.9629 | 1.0371 | |||
32 | Mumbai | 0.7913 | 1.2087 | 0.7322 | 1.2678 | 0.8330 | 1.1670 | 0.7639 | 1.2361 | |||
33 | Munnar | 0.6839 | 1.3161 | 0.7814 | 1.2186 | 0.8236 | 1.1764 | 0.8812 | 1.1188 | |||
34 | Nagpur | 0.8041 | 1.1959 | 0.7647 | 1.2353 | 0.6321 | 1.3679 | 0.7150 | 1.2850 | |||
35 | Ooty | 0.7143 | 1.2857 | 0.8671 | 1.1329 | 0.7965 | 1.2035 | 0.8648 | 1.1352 | |||
36 | Patna | 0.6902 | 1.3098 | 0.8012 | 1.1988 | 0.7835 | 1.2165 | 0.7553 | 1.2447 | |||
37 | Port Blair | 0.8430 | 1.1570 | 0.8583 | 1.1417 | 0.9734 | 1.0266 | 0.8785 | 1.1215 | |||
38 | Ramagundam | 0.8486 | 1.1514 | 0.5940 | 1.4060 | 0.8039 | 1.1961 | 0.6113 | 1.3887 | |||
39 | Ranchi | 0.8808 | 1.1192 | 0.7978 | 1.2022 | 0.8412 | 1.1588 | 0.7855 | 1.2145 | |||
40 | Shimla | 0.9106 | 1.0894 | 0.7580 | 1.2420 | 0.9198 | 1.0802 | 0.8870 | 1.1130 | |||
41 | Sriganganagar | 0.8641 | 1.1359 | 0.8426 | 1.1574 | 0.7223 | 1.2777 | 0.7468 | 1.2532 | |||
42 | Srinagar | 0.7839 | 1.2161 | 0.8025 | 1.1975 | 0.7815 | 1.2185 | 0.8985 | 1.1015 | |||
43 | Surat | 0.8113 | 1.1887 | 0.7456 | 1.2544 | 0.7152 | 1.2848 | 0.8962 | 1.1038 | |||
44 | Tiruvananantapuram | 0.6761 | 1.3239 | 0.7976 | 1.2024 | 0.8670 | 1.1330 | 0.7793 | 1.2207 | |||
45 | Udaynarayanpur | 0.7845 | 1.2155 | 0.8068 | 1.1932 | 0.8645 | 1.1355 | 0.7256 | 1.2744 | |||
Mean Average | 0.8104 | 1.1896 | 0.8013 | 1.1987 | 0.8154 | 1.1846 | 0.8060 | 1.1940 |
SI. No. | Locations | AMASP | AMAT | AMAWS | AMAP |
1 | Agartala | 100.49 | 25.44 | 2.75 | 5.26 |
2 | Agumbe | 96.88 | 25.22 | 2.64 | 9.01 |
3 | Bengaluru | 91.66 | 23.63 | 4.14 | 2.30 |
4 | Cherrapunji | 87.59 | 17.79 | 2.49 | 6.88 |
5 | Cuddalore | 100.81 | 28.11 | 4.98 | 2.78 |
6 | Dispur | 96.30 | 22.86 | 1.85 | 5.04 |
7 | Gairsain | 81.27 | 15.16 | 2.38 | 2.87 |
8 | Kavaratti | 101.00 | 27.83 | 4.77 | 4.36 |
9 | Kodaikanal | 93.12 | 23.60 | 2.68 | 3.23 |
10 | Kohima | 86.28 | 18.20 | 1.06 | 3.10 |
11 | Lachen | 69.27 | 7.24 | 2.42 | 2.62 |
12 | Ladākh | 58.35 | -3.13 | 2.26 | 0.57 |
13 | Mawsynram | 87.59 | 17.79 | 2.49 | 6.88 |
14 | Port Blair | 100.88 | 27.93 | 5.39 | 6.54 |
15 | Shimla | 90.95 | 21.10 | 2.84 | 2.67 |
SI. No. | Locations | AMASP | AMAT | AMAWS | AMAP |
1 | Agartala | G | G | L | G |
2 | Agumbe | G | G | L | G |
3 | Bengaluru | G | M | G | L |
4 | Cherrapunji | L | M | L | G |
5 | Cuddalore | G | G | G | L |
6 | Dispur | G | G | L | G |
7 | Gairsain | L | L | L | L |
8 | Kavaratti | G | G | G | G |
9 | Kodaikanal | G | M | L | M |
10 | Kohima | L | M | L | M |
11 | Lachen | L | L | L | L |
12 | Ladākh | L | L | L | L |
13 | Mawsynram | L | M | L | G |
14 | Port Blair | G | G | G | G |
15 | Shimla | G | M | M | L |
SI. No. | Location name | Latitude | Longitude |
1 | Agartala, West Tripura, Tripura | 23.8296 | 91.2901 |
2 | Agumbe, Kundapura, Udupi, Karnataka | 13.508 | 75.0946 |
3 | Amaravathi, Guntur, Andhra Pradesh | 16.5703 | 80.3606 |
4 | Balasore, Odisha | 21.5053 | 86.9159 |
5 | Bānda, Uttar Pradesh | 25.4754 | 80.3356 |
6 | Bengaluru, Karnataka | 12.9703 | 77.5855 |
7 | Bhopal, Madhya Pradesh | 23.2644 | 77.4018 |
8 | Bhubaneswar, Odhisa | 20.2698 | 85.841 |
9 | Bilaspur, Chhattisgarh | 22.0787 | 82.1403 |
10 | Bramhapuri, Mahārāshtra | 20.6 | 79.8666 |
11 | Chandigarh, Harayana | 30.7306 | 76.8478 |
12 | Chennai, Tamil Nadu | 13.0549 | 80.2311 |
13 | Cherrapunji, East Khasi Hills, Meghalaya | 25.2851 | 91.7184 |
14 | Chittoor, Andhra Pradesh | 13.2067 | 79.0883 |
15 | Cuddalore, Tamil Nadu | 11.7462 | 79.7586 |
16 | Delhi, New Delhi, Delhi | 28.6342 | 77.2168 |
17 | Dispur, Kamrup Metropolitan, Assam | 26.136 | 91.8081 |
18 | Gairsain, Chamoli, Uttarakhand | 30.0554 | 79.2904 |
19 | Gandhinagar, Gujarat | 23.2413 | 72.638 |
20 | Imphal, Imphal West, Manipur | 24.8077 | 93.9273 |
21 | Jaisalmer, Rajasthan | 26.9194 | 70.9146 |
22 | Kavaratti Sub-Division, Lakshadweep | 10.5644 | 72.639 |
23 | Kodaikanal, Dindigul, Tamil Nadu | 10.2312 | 77.4882 |
24 | Kohima, Nagaland | 25.6631 | 94.0933 |
25 | Kolkata, West Bengal | 22.5708 | 88.372 |
26 | Kottayam, Kerala | 9.5947 | 76.5328 |
27 | Lachen, Northen sikkim | 27.7456 | 88.5014 |
28 | Ladākh, Jammu and Kashmir | 34.337 | 77.4057 |
29 | Ludiana, Punjab | 30.9063 | 75.8489 |
30 | Mahabaleshwar, Satara, Maharashtra | 17.9262 | 73.6593 |
31 | Mawsynram, East Khasi Hills, Meghalaya | 25.2996 | 91.5828 |
32 | Mumbai, Maharashtra | 18.9373 | 72.8349 |
33 | Munnar, Devikulam, Idukki, Kerala | 10.0742 | 77.0625 |
34 | Nagpur, Maharashtra | 21.1563 | 79.0821 |
35 | Ooty, The Nilgiris, Tamil Nadu | 11.4059 | 76.6991 |
36 | Patna, Bihar | 25.6015 | 85.1372 |
37 | Port Blair, Andaman & Nicobar Islands | 11.6629 | 92.7391 |
38 | Ramagundam, Peddapalle, Telangana | 18.7565 | 79.4843 |
39 | Ranchi, Jharkhand | 23.3515 | 85.3127 |
40 | Shimla, Himachal Pradesh | 31.1033 | 77.1581 |
41 | Sriganganagar, Rajasthan | 29.912 | 73.8756 |
42 | Srinagar, Jammu & Kashmir | 34.0862 | 74.7984 |
43 | Surat, Gujarat | 21.1857 | 72.837 |
44 | Tiruvananantapuram, Kerala | 8.5069 | 76.9569 |
45 | Udaynarayanpur, Haora, West Bengal | 22.7144 | 87.9761 |
SI. No. | Location name | MASP | MAT | MAWS | MAP | |||||||
H | D | H | D | H | D | H | D | |||||
1 | Agartala | 0.7508 | 1.2492 | 0.8647 | 1.1353 | 0.9143 | 1.0857 | 0.8833 | 1.1167 | |||
2 | Agumbe | 0.6840 | 1.3160 | 0.8660 | 1.1340 | 0.7992 | 1.2008 | 0.8264 | 1.1736 | |||
3 | Amaravathi | 0.8679 | 1.1321 | 0.8516 | 1.1484 | 0.8732 | 1.1268 | 0.7998 | 1.2002 | |||
4 | Balasore | 0.8632 | 1.1368 | 0.7707 | 1.2293 | 0.8285 | 1.1715 | 0.8531 | 1.1469 | |||
5 | Bānda | 0.8109 | 1.1891 | 0.8127 | 1.1873 | 0.8401 | 1.1599 | 0.6169 | 1.3831 | |||
6 | Bengaluru | 0.7683 | 1.2317 | 0.9077 | 1.0923 | 0.8147 | 1.1853 | 0.8755 | 1.1245 | |||
7 | Bhopal | 0.7888 | 1.2112 | 0.8258 | 1.1742 | 0.8571 | 1.1429 | 0.5916 | 1.4084 | |||
8 | Bhubaneswar | 0.8963 | 1.1037 | 0.8217 | 1.1783 | 0.8229 | 1.1771 | 0.7118 | 1.2882 | |||
9 | Bilaspur | 0.8363 | 1.1637 | 0.7410 | 1.2590 | 0.7093 | 1.2907 | 0.7061 | 1.2939 | |||
10 | Bramhapuri | 0.8781 | 1.1219 | 0.7303 | 1.2697 | 0.7442 | 1.2558 | 0.6208 | 1.3792 | |||
11 | Chandigarh | 0.8521 | 1.1479 | 0.7818 | 1.2182 | 0.8659 | 1.1341 | 0.8657 | 1.1343 | |||
12 | Chennai | 0.7820 | 1.2180 | 0.7463 | 1.2537 | 0.7816 | 1.2184 | 0.7029 | 1.2971 | |||
13 | Cherrapunji | 0.8718 | 1.1282 | 0.8189 | 1.1811 | 0.9034 | 1.0966 | 0.9629 | 1.0371 | |||
14 | Chittoor | 0.7532 | 1.2468 | 0.7729 | 1.2271 | 0.7144 | 1.2856 | 0.7361 | 1.2639 | |||
15 | Cuddalore | 0.7437 | 1.2563 | 0.7931 | 1.2069 | 0.7823 | 1.2177 | 0.6662 | 1.3338 | |||
16 | Delhi | 0.8393 | 1.1607 | 0.7178 | 1.2822 | 0.8543 | 1.1457 | 0.6853 | 1.3147 | |||
17 | Dispur | 0.9022 | 1.0978 | 0.8408 | 1.1592 | 0.9648 | 1.0352 | 0.7955 | 1.2045 | |||
18 | Gairsain | 0.8999 | 1.1001 | 0.7348 | 1.2652 | 0.9194 | 1.0806 | 0.8868 | 1.1132 | |||
19 | Gandhinagar | 0.8946 | 1.1054 | 0.7378 | 1.2622 | 0.7000 | 1.3000 | 0.6842 | 1.3158 | |||
20 | Imphal | 0.8082 | 1.1918 | 0.8252 | 1.1748 | 0.8442 | 1.1558 | 0.8811 | 1.1189 | |||
21 | Jaisalmer | 0.8514 | 1.1486 | 0.8317 | 1.1683 | 0.8940 | 1.1060 | 0.7748 | 1.2252 | |||
22 | Kavaratti | 0.7065 | 1.2935 | 0.8879 | 1.1121 | 0.7792 | 1.2208 | 0.8319 | 1.1681 | |||
23 | Kodaikanal | 0.8106 | 1.1894 | 0.8287 | 1.1713 | 0.7363 | 1.2637 | 0.9094 | 1.0906 | |||
24 | Kohima | 0.8150 | 1.1850 | 0.8645 | 1.1355 | 0.7909 | 1.2091 | 0.9682 | 1.0318 | |||
25 | Kolkata | 0.7845 | 1.2155 | 0.8068 | 1.1932 | 0.8645 | 1.1355 | 0.7256 | 1.2744 | |||
26 | Kottayam | 0.6740 | 1.3260 | 0.8669 | 1.1331 | 0.7032 | 1.2968 | 0.8661 | 1.1339 | |||
27 | Lachen | 0.8426 | 1.1574 | 0.7908 | 1.2092 | 0.6325 | 1.3675 | 0.9694 | 1.0306 | |||
28 | Ladākh | 0.7650 | 1.2350 | 0.8673 | 1.1327 | 0.8326 | 1.1674 | 0.9341 | 1.0659 | |||
29 | Ludiana | 0.8786 | 1.1214 | 0.8463 | 1.1537 | 0.8199 | 1.1801 | 0.7934 | 1.2066 | |||
30 | Mahabaleshwar | 0.8920 | 1.1080 | 0.7386 | 1.2614 | 0.8454 | 1.1546 | 0.8702 | 1.1298 | |||
31 | Mawsynram | 0.8718 | 1.1282 | 0.8189 | 1.1811 | 0.9034 | 1.0966 | 0.9629 | 1.0371 | |||
32 | Mumbai | 0.7913 | 1.2087 | 0.7322 | 1.2678 | 0.8330 | 1.1670 | 0.7639 | 1.2361 | |||
33 | Munnar | 0.6839 | 1.3161 | 0.7814 | 1.2186 | 0.8236 | 1.1764 | 0.8812 | 1.1188 | |||
34 | Nagpur | 0.8041 | 1.1959 | 0.7647 | 1.2353 | 0.6321 | 1.3679 | 0.7150 | 1.2850 | |||
35 | Ooty | 0.7143 | 1.2857 | 0.8671 | 1.1329 | 0.7965 | 1.2035 | 0.8648 | 1.1352 | |||
36 | Patna | 0.6902 | 1.3098 | 0.8012 | 1.1988 | 0.7835 | 1.2165 | 0.7553 | 1.2447 | |||
37 | Port Blair | 0.8430 | 1.1570 | 0.8583 | 1.1417 | 0.9734 | 1.0266 | 0.8785 | 1.1215 | |||
38 | Ramagundam | 0.8486 | 1.1514 | 0.5940 | 1.4060 | 0.8039 | 1.1961 | 0.6113 | 1.3887 | |||
39 | Ranchi | 0.8808 | 1.1192 | 0.7978 | 1.2022 | 0.8412 | 1.1588 | 0.7855 | 1.2145 | |||
40 | Shimla | 0.9106 | 1.0894 | 0.7580 | 1.2420 | 0.9198 | 1.0802 | 0.8870 | 1.1130 | |||
41 | Sriganganagar | 0.8641 | 1.1359 | 0.8426 | 1.1574 | 0.7223 | 1.2777 | 0.7468 | 1.2532 | |||
42 | Srinagar | 0.7839 | 1.2161 | 0.8025 | 1.1975 | 0.7815 | 1.2185 | 0.8985 | 1.1015 | |||
43 | Surat | 0.8113 | 1.1887 | 0.7456 | 1.2544 | 0.7152 | 1.2848 | 0.8962 | 1.1038 | |||
44 | Tiruvananantapuram | 0.6761 | 1.3239 | 0.7976 | 1.2024 | 0.8670 | 1.1330 | 0.7793 | 1.2207 | |||
45 | Udaynarayanpur | 0.7845 | 1.2155 | 0.8068 | 1.1932 | 0.8645 | 1.1355 | 0.7256 | 1.2744 | |||
Mean Average | 0.8104 | 1.1896 | 0.8013 | 1.1987 | 0.8154 | 1.1846 | 0.8060 | 1.1940 |
SI. No. | Locations | AMASP | AMAT | AMAWS | AMAP |
1 | Agartala | 100.49 | 25.44 | 2.75 | 5.26 |
2 | Agumbe | 96.88 | 25.22 | 2.64 | 9.01 |
3 | Bengaluru | 91.66 | 23.63 | 4.14 | 2.30 |
4 | Cherrapunji | 87.59 | 17.79 | 2.49 | 6.88 |
5 | Cuddalore | 100.81 | 28.11 | 4.98 | 2.78 |
6 | Dispur | 96.30 | 22.86 | 1.85 | 5.04 |
7 | Gairsain | 81.27 | 15.16 | 2.38 | 2.87 |
8 | Kavaratti | 101.00 | 27.83 | 4.77 | 4.36 |
9 | Kodaikanal | 93.12 | 23.60 | 2.68 | 3.23 |
10 | Kohima | 86.28 | 18.20 | 1.06 | 3.10 |
11 | Lachen | 69.27 | 7.24 | 2.42 | 2.62 |
12 | Ladākh | 58.35 | -3.13 | 2.26 | 0.57 |
13 | Mawsynram | 87.59 | 17.79 | 2.49 | 6.88 |
14 | Port Blair | 100.88 | 27.93 | 5.39 | 6.54 |
15 | Shimla | 90.95 | 21.10 | 2.84 | 2.67 |
SI. No. | Locations | AMASP | AMAT | AMAWS | AMAP |
1 | Agartala | G | G | L | G |
2 | Agumbe | G | G | L | G |
3 | Bengaluru | G | M | G | L |
4 | Cherrapunji | L | M | L | G |
5 | Cuddalore | G | G | G | L |
6 | Dispur | G | G | L | G |
7 | Gairsain | L | L | L | L |
8 | Kavaratti | G | G | G | G |
9 | Kodaikanal | G | M | L | M |
10 | Kohima | L | M | L | M |
11 | Lachen | L | L | L | L |
12 | Ladākh | L | L | L | L |
13 | Mawsynram | L | M | L | G |
14 | Port Blair | G | G | G | G |
15 | Shimla | G | M | M | L |